Optimal Filters for the Detection of Continuous Gravitational Waves
Optimal Control and Estimation

Optimal Control and Estimation Optimal control and estimation are crucial concepts in the field ofengineering and mathematics, playing a significant role in various real-world applications such as robotics, aerospace, economics, and more. These concepts are essential for designing systems that can achieve the best performance while considering constraints and uncertainties. In this discussion, we will explore the importance of optimal control and estimation from multiple perspectives, considering their practical applications, theoretical foundations, and the challenges involved in implementing these techniques. From a practical standpoint, optimal control and estimation are essential for designing and controlling complex systems such as autonomous vehicles, industrial processes, and aerospace systems. For instance, in the context of autonomous vehicles, optimal control techniquesare used to plan the vehicle's trajectory while considering factors such astraffic conditions, safety constraints, and energy efficiency. Similarly, estimation techniques such as Kalman filtering are used for state estimation, allowing the vehicle to accurately perceive its environment and make informed decisions. These applications highlight the critical role of optimal control and estimation in enabling advanced technologies that have the potential to transform various industries. On a theoretical level, optimal control and estimation are grounded in mathematical optimization and statistical inference, drawing from disciplines such as control theory, optimization, and probability theory. Optimal control problems often involve finding the control inputs that minimize a certain cost function while satisfying system dynamics and constraints. This requires a deep understanding of optimization algorithms, dynamic programming, andPontryagin's maximum principle. On the other hand, estimation methods such as the Kalman filter and particle filters are rooted in Bayesian inference, involving the recursive update of probability distributions based on noisy measurements and system dynamics. The theoretical foundations of optimal control and estimation provide the necessary framework for developing and analyzing algorithms that can handle real-world complexities. However, the implementation of optimal controland estimation techniques is not without challenges. One of the primary challenges is the need for accurate models of the system dynamics and sensor measurements. Inmany real-world applications, the underlying dynamics may be complex and uncertain, leading to difficulties in formulating precise models for control and estimation. Additionally, the computational complexity of optimal control and estimation algorithms can be prohibitive, especially for real-time applications. Balancingthe trade-off between computational efficiency and optimality is a non-trivial task, requiring careful algorithm design and implementation. Moreover, the integration of optimal control and estimation techniques with modern technologies such as machine learning and deep learning presents both opportunities and challenges. While machine learning methods can potentially enhance the accuracy of system models and sensor data processing, integrating these techniques with traditional optimal control and estimation frameworks requires a deep understanding of both domains. Furthermore, ensuring the safety and reliability of autonomous systems that rely on optimal control and estimation poses ethical and societal challenges, especially in high-stakes domains such as healthcare and transportation. In conclusion, optimal control and estimation are fundamental concepts with far-reaching implications across various domains. From enabling the autonomy of vehicles to optimizing industrial processes, these techniques are essential for achieving superior performance in the presence of uncertainties and constraints. However, the practical implementation of optimal control and estimation poses significant challenges, ranging from modeling complexities to computational considerations. Addressing these challenges requires a multidisciplinary approach, combining expertise in control theory, optimization, statistics, and emerging technologies. As we continue to advance in the era of autonomous systems and smart technologies, the role of optimal control and estimation will only become more pronounced, shaping the future of engineering and beyond.。
信号处理 扩展卡尔曼滤波数据融合代码matlab

信号处理扩展卡尔曼滤波数据融合代码matlab 如何使用扩展卡尔曼滤波(Extended Kalman Filter, EKF)进行数据融合的问题,并提供MATLAB代码示例。
引言:现代技术的快速发展使得传感器的数量和种类越来越多。
数据融合是将多个传感器的测量结果进行合并,以得到更准确、更可靠的估计值的过程。
扩展卡尔曼滤波是一种常用的数据融合算法,特别适用于非线性系统的估计。
正文:扩展卡尔曼滤波是对卡尔曼滤波的一种扩展,它利用非线性系统的一阶泰勒展开,以线性化的形式近似非线性系统。
步骤一:构建状态方程和观测方程首先,我们需要构建状态方程和观测方程。
状态方程描述系统的动力学变化,而观测方程描述传感器对状态量的测量。
假设我们有一个非线性系统,其状态方程可以表示为:x(k) = f(x(k-1), u(k-1)) + w(k-1)其中,x(k)是系统在时刻k的状态量,f是非线性函数,u(k-1)是时刻k-1的控制量,w(k-1)是过程噪声。
观测方程可以表示为:z(k) = h(x(k)) + v(k)其中,z(k)是传感器在时刻k的测量值,h是非线性函数,v(k)是观测噪声。
步骤二:线性化模型由于扩展卡尔曼滤波是基于线性化模型的,我们需要对状态方程和观测方程进行线性化处理。
线性化可以使用一阶泰勒展开来近似非线性函数。
具体地,我们可以通过对状态方程和观测方程求一阶偏导数得到线性化模型。
步骤三:初始化滤波器扩展卡尔曼滤波的初始化包括初始化状态量估计和协方差矩阵。
初始状态量估计可以通过系统初始条件提供,而协方差矩阵可以设置为一个足够大的值,表示对初始估计的不确定性。
步骤四:预测步骤在预测步骤中,我们使用状态方程和控制量来预测时刻k的状态量估计。
同时,我们也需要更新状态量的协方差矩阵。
具体地,预测的状态量估计可以表示为:x^(k) = f(x^(k-1), u(k-1))预测的协方差矩阵可以表示为:P^(k) = A * P(k-1) * A' + Q(k-1)其中,x^(k)是时刻k的预测状态量估计,P^(k)是时刻k的预测协方差矩阵,A是状态方程的雅可比矩阵,Q(k-1)是过程噪声的协方差矩阵。
重型矿山设备手册 SANDVIK 重型颚式破碎机说明书

KEY FEATURESNew generation world-class Automation & Connectivity System (ACS)Automatically adapts the crusher to varying feed condi-tions ensuring maximum 24/7 performanceHydroset™ system Provides safety and settingadjustment functionsMainframe is built as a unibody without moving parts For optimal strength and less components requiring maintenanceTop serviceability Lifting from above minimizesrisks, and allows for quicker andsafer maintenanceAdjustable eccentric throw To exactly balance capacity tothe process thus harmonizingthe crushing stagesConstant liner profile Maintains the feed opening andperformance during the entireservice life of the linersWide range of crushing chambers suited for all types of applications Choose from extra coarse crushing chambers with the largest intake to extremely fine crushing chambersMechanical dump valve for tramp iron protection Reduces pressure peaks and mechanical stress on the crusher, greatly improving reliabilityFull lubrication monitoring and control Real-time monitoring of the crusher lubrication system for increased uptime and reliabilitySandvik CH840i is a technologically advanced, high capacity mid-range cone crusher for secondary and tertiary crushing, designed for crushing applications in mines or large sized quarries.Each crusher has a hydraulically supported main shaft which is supported at both ends. With a robust design, adjustable eccentric throw, a constant intake opening, high performance can be achieved by proper selection of a Sandvik OEM crushing chamber. The CH840i brings you a revolution in intelligent crushing. Connected via the My Sandvik portal,it offer 24/7 access to data generated by your connected Sandvik crusher fleet. Now you can make decisions based on facts, and clearly see areas where you can improve uptime and productivity. My Sandvik also gives you access to manuals and ane-commerce platform for easily and efficiently buying and reordering wear and spare parts. It lets you track and trace parts online to make maintenance planning simpler.The CH840i comes with the new generation Automation and Connectivity System (ACS) as standard. The system continuously monitors and optimizes crusher performance and controls the complete lubrication system, increasing uptimeand reliability. It can automatically adjust crusher settings to compensate for crushing chamber wear, ensuring consistent product size. Hydroset™ and the advanced dump valve automatically provide overload protection to let tramp iron or other uncrushable material pass through.Bolted rather than welded top and bottom shell liners reduce maintenance time and are much safer. It’s 90% faster to change liners compared to welding. The improved over-pressure system with dedicated air channel inlets keeps dust out to increase reliability. The standard off-line filter unit keeps lubrication oil cleaner with 24/7 fine filtration, reducing wear on your internal crusher components and extending oil life by up to 5 times.GENERAL DESIGN CRITERIA Crusher type Cone crusher, hydraulically adjusted Application Minerals processingCrushing stage Secondary, tertiary, quaternary Max. feed size 250 mm CSS range 10-48 mm Nominal capacity*103-427 mtphAmbient temperature -20°C to +40°C(Contact Sandvik if outside range)Altitude of site≤ 2,000 m(Contact Sandvik if outside range)* Capacity and possible CSS is dependent on the crushing chamber, the eccentric throw, the crusher’s setting and the feed material’s bulk density, crushability, size analysis, moisture content, etc.GENERAL CRUSHER DATA Weight 20,278 kgMain frameTwo-part unibody structure without moving parts.Cast steel.Top shell Two-arm design Bottom shell Five-arm designTwo inspection hatches Feed hopper Rubber / steel lined steel hopper Two inspection doors Feed level sensor Vegapuls 67Main shaftSupported at both ends Top spider bearing and eccentric bearing Eccentric bushings (Throws – mm)• 28, 32, 36• 36, 40, 44• 44, 48, 52Eccentric speed327 rpm (50 Hz, SPC-belt)332 rpm (60 Hz, SPC-belt)331 rpm (60 Hz, 8V-belt)Max. motor power 330 kW DriveV-Belt or DirectSafety coupling Omega (for Direct drive option)Pinion shaft speed1,470 rpm (50 Hz, SPC-belt)1,494 rpm (60 Hz, SPC-belt) 1,491 rpm (60 Hz 8V-belt)SubframeWith rubber dampersMaintenance tool boxExtractor for eccentric bushing Extractor for bottom shell bushing Extractor for step bearingAdditional lifting and maintenance tools includedGENERAL INFORMATIONMOTOR CHARACTERISTICSManufacturer WEG Model W22/HGFType Three-phase, squirrel cage Weight 1,850–2,650 Kg Rated power330 kW Frequency 50/60 Hz Poles4Vibration resistanceMotor is supplied with special winding that is reinforced in order to support the vibration levels Insulation class F Protection classIP55MECHANICAL DUMP VALVE System descriptionMechanical spring loaded hydraulic valveSYSTEM CHARACTERISTICS Type Dust seal air pressure Air input Blower Air quality Filtered Air flow <70 m³/h Air pressure<10 kPa Weight (blower, hoses)25 kg Motor power 0.75 kWMotor speed 2,800 rpm (50Hz) 3,350 rpm (60Hz)Phases 3Insulation class F Protection classIP55CRUSHER DRIVE SYSTEMCRUSHER DUST EXCLUSIONCRUSHER TRAMP IRON PROTECTIONUPPER FEED HOPPERNo. of rubber liners 16Max. weight 8 kgMaterialSandvik WT6000 rubber Fastening methodBoltedCONE LINER No. of rubber liners 20Max. weight 9-10 kg / 4-5 kgMaterialManganese steel or Sandvik WT6000 rubber (option)Fastening method BoltedTOP SHELL SPIDER CAP Max. weight 198 kg MaterialCarbon steelFastening methodBolted seal with O-ringTOP SHELL ARM SHIELDS No. of shields 2Max. weight 84 kgMaterialManganese steel Fastening methodBoltedBOTTOM SHELL BODY LINERS No. of liners 10Max. weight 16-24 kg / 5-7 kgMaterialWear-resistant hardened steel or Sandvik WT6000 rubber (option)Fastening methodBoltedBOTTOM SHELL ARM LINERS No. of liners 5Max. weight 80–83 kg MaterialManganese steel Fastening method Bolted (welding*)CRUSHER WEAR PROTECTIONCRUSHING CHAMBERS Mantle alternatives A, B, FlexiFeed B Concave alternatives EC, C, MC, M, F Alloys for mantles and concaves M1, M2, M7, M9Mantle and concave backing materialPlastic free, metallic contactLifting tools for mantles and concavesAvailable as option *No main frame weldingMONITORING FUNCTIONS Main/secondary lubrication circuit dataOil temperature Oil flowOil pressureOil tank temperature Oil levelDifferential pressure across filter Pinion shaft lubrication circuit dataOil pressureDifferential pressure across filterOver-pressure air system Filter monitoring functions Offline filter statusOPERATIONAL FUNCTIONSOil heatersMain lubrication oil pump Pinion lubrication oil pump Over-pressure fan Air/oil coolers Offline filter functionsELECTRICAL HARDWARE Lubrication controlConnection modules tank Cable kit CABINET DIMENSIONS Lubrication control cabinet (LxHxD)1200x800x250 mmHYDROSET SYSTEM System designSingle reversible pump Oil tank reservoir capacity 85 liters Pump design Gear pump Pump capacity10.4 l/min @50 Hz 12.6 l/min @60 HzOil filter Filter type Spin-on Filtration grade 10 µm Filter materialGlass fiber No. of filters 1Pump motor Type Three-phase, squirrel cage Power 3 kW @50 Hz 3.6 kW @60 Hz Speed 1,500 rpm @50 Hz 1,800 rpm @60 Hz Poles4Insulation class F Protection classIP55GENERAL DATAOil tank reservoir capacitySupplies oil to the main lubrica-tion system, Pinion lubrication systems and to the Hydroset system.No. of doors3No. of inspection hatches 2 located on top of unit Cabinet materialMetalTank unit dimensions (LxWxH)1,980x1,130x2,000 mm Dry weight865 kgMAIN CRUSHER LUBRICATION SYSTEM System designClosed circuit, single pump, gravity return Oil tank reservoir capacity 400 liters Pump design Gear pump Standby pump N/APump capacity112 l/min @50 Hz 135 l/min @60 HzOil filters Filter type Filter element insert Filtration grade 25 µm Filter material Glass fiber No. of filters1TANK UNITLUBRICATION CONTROL (ACS)SETTING REGULATIONSOFTWARE PACKAGE (OPTIONAL)PINIONSHAFT LUBRICATION SYSTEM System designClosed circuit, single pump, gravity return Oil tank reservoir capacity52 liters Pump design Gear pump Pump capacity0.9 l/min @50 Hz 1.1 l/min @60 HzOil filter Filter type Spin-on Filtration grade10 µm Filter material Glass fiber No. of filters 1Pump motor Type Three-phase, squirrel cage Power 0.12 kW @50 Hz / @60 Hz Speed1,500 rpm @50 Hz 1,800 rpm @60 Hz Insulation class F Protection classIP55OPERATOR’S PANEL Dimensions (LxHxD)316X251X72.5 mm Weight3.5 kgOperational temperature -25°C to +70°C Protection class IP65Power supply10–30 VDCMONITORING FUNCTIONS(AVAILABLE WITH METRIC AND IMPERIAL UNITS)Energy consumption Hydroset hydraulic pressure Main shaft positionCalculated CSS (based on main shaft position)Lubrication oil temperatureTemperature close to the spider bearing Liner wear Historical data logAutomatic liner wear compensation (Only available for CH-models)AUTOMATION & CONNECTIVITY SYSTEM (ACS) REGULATION FUNCTIONS (CRUSHING MODES)CSS (Auto CSS)Keep CSS constant Peak Pressure (Auto Load)Keep load constant Multi-CSS (Multi – CSS)Alternate between two CSS settings10 customized programs can be storedOTHER FUNCTIONS & CABINET DIMENSIONS Push button box for manual setting of CSS Setting regulation cabinet (LxHxD)1200x600x250 mm Connection box crusher (LxHxD)600x350x155 mm Network repeater box (LxHxD)(Recommended for distances over 100m)300x300x210 mmSAFETY FUNCTIONSProtects the crusher from overload by automatically regulating the crusher based on preset operational limits and the real-time input from the crusherAlarm severity levels: Direct Stop, Sequential Stop, Feeder stop, Notices and EventsSignal permitting operation of the crusher drive motor Alarm logELECTRICAL HARDWARE Setting regulation control Power measurement unit Customer interface gateway Connection box crusher Cable kitPump motor Type Three-phase, squirrel cage Power 4 kW @50 Hz 4.8 kW @60 Hz Speed1,500 rpm @50 Hz 1,800 rpm @60 Hz Insulation class F Protection class IP55Oil heaters No. of heaters 2 (Optional 3)Type Immersion heater Rating1.65 kWInstallation typeImmersion heater tube Phases3Communication gateway interfaceControlNet DeviceNet Ethernet/IP Modbus TCP Profibus ProfinetWINiOperating system compatibility:Windows 10, Windows 8, Windows 7, Windows Vista, Windows XP, Windows 2000Simultaneously control up to 9 different crushers with ACS from a PC via Ethernet networkControl the ACS remotely using the same graphical user interface ACS ReporterExport data from theAutomation & Connectivity System to a PC for analysis and storageOFFLINE FILTER UNIT FOR MAIN LUBRICATION MANUALSPurposeRemoves particles and water from the main lubrication system in a continuous slow offline filtration process Model 27/54Oil capacity20 litersDimensions (LxWxH)650x450x1,055mm Weight 100 kg Pump designGear wheelOil filter Filter type Filter Insert Filtration grade 3 µm Filter material Cellulose Filter housing material Cast iron No. of filters 2Pump motorType Three-phase, squirrel cage Capacity 200 @50 Hz 240 @60 Hz Speed915 rpm @50 Hz 1,120 rpm @60 Hz Protection classIP55Operator’s manual Any language Installation manualAny language Installation manual appendix Any language Maintenance manual Any language Spare parts catalogueEnglish onlyKg Lb Top shell assembly 6,35814,017Bottom shell assembly 5,77212,725Main shaft assembly4,2019,262Pinion shaft housing assembly 244538Hydroset cylinder assembly 1,0802,381Feed hopper assembly 1,4403,175Eccentric assembly 7951,753Dust collar assembly239527Hoses and protection assembly 51112Crusher weight 20,27844,706Subframe1,3843,051Electric motor (max.)2,6505,842Tolat weight(incl. subframe and drive)25,26855,707OIL COOLING SYSTEMS(FOR MAIN CRUSHER LUBRICATION)STANDARD AIR/OIL COOLERS No. of units1Dry weight (incl. stand)240 kg Material Aluminum Oil volume 12.8 liters Max. air flow2.8 kg/s @50 Hz3.3 kg/s @60 HzHOT CLIMATE AIR/OIL COOLERS No. of units1Dry weight (incl. stand)390 kg Material Aluminum Oil volume 19.0 liters Max. air flow7,8 kg/s @50 Hz 9,3 kg/s @60 HzAIR COOLER FAN MOTOR Type Three-phase, squirrel cage Power 2.2 kW @50 Hz 3.6 kW @60 Hz Speed1,500 rpm @50 Hz 1,800 rpm @60 HzAIR COOLER FAN MOTOR Type Three-phase, squirrel cage Power 5,5 kW @50 Hz 6,3 kW @60 Hz Speed1,500 rpm @50 Hz 1,800 rpm @60 HzCH840i CONNECTED – NOMINAL CAPACITY* (MTPH)ConcaveEC C MC M F Max. feed size (mm)F85**14111681--F901721411279773F10025020515912192Max. motor power (kW)330330*********Eccentric throw (mm)28-5228-5228-5228-5228-52CSS (mm)810131619222529323538414448---162-193174-272186-290198-309214-334226-352238-371250-366261-335273-324289---177-210190-297203-317216-337233-364246-384259-356272-349285-312298----187-254201-313214-334228-356246-384260-406274-427287-421301-385315-374333--155-213167-261180-280192-299204-299220-282233-254245----103-132109-170119-185128-200137-214147-215156-214169-200178-----MantleA/B/FFA/B/FFA/B/FFA/BA/B* based on material with bulk density of 1,600 kg/m 3** Additional feed size requirement applicable for FF mantle only (FlexiFeed)PERFORMANCEWEIGHT (KG)T S 5-1227:03/E N G /M E T R I C © S a n d v i k M i n i n g a n d R o c k T e c h n o l o g y 2019 S A N D V I K i s a r e g i s t e r e d t r a d e m a r k o w n e d b y S a n d v i k I n t e l l e c t u a l P r o p e r t y A B i n S w e d e n a n d o t h e r c o u n t r i e s.Sandvik Mining and Rock Technology reserves the right to make changes to the information on this data sheet without prior notification to users. Please contact a Sandvik representative for clarification on specifications and options.ROCKTECHNOLOGY.SANDVIK* Always refer to the installation manualsDIMENSIONS*4159 mm (Max)2160 mm3393 m m。
驰盛D861B系列安全DVR配件说明书
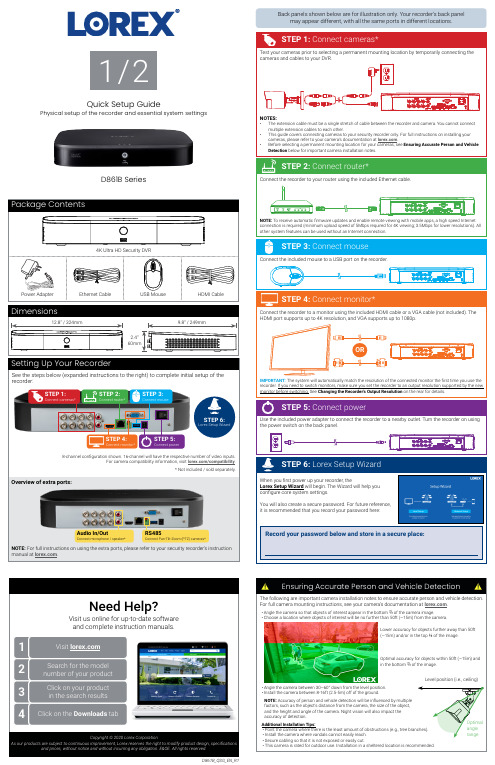
D861B_QSG_EN_R1D861B SeriesQuick Setup GuidePhysical setup of the recorder and essential system settingsBack panels shown below are for illustration only. Your recorder’s back panelmay appear different, with all the same ports in different locations.Record your password below and store in a secure place:recorder:STEP 1:Connect cameras*STEP 2:Connect router*STEP 3:Connect mouseSTEP 5:STEP 4:Overview of extra ports:Audio In/OutConnect microphone / speaker*RS485Connect Pan-Tilt-Zoom (PTZ) cameras*Ensuring Accurate Person and Vehicle DetectionThe following are important camera installation notes to ensure accurate person and vehicle detection. For full camera mounting instructions, see your camera’s documentation at .Optimal accuracy for objects within 50ft (~15m) and in the bottom ⅔ of the image.Additional Installation Tips:• Point the camera where there is the least amount of obstructions (e.g., tree branches).• Install the camera where vandals cannot easily reach.• Secure cabling so that it is not exposed or easily cut.• This camera is rated for outdoor use. Installation in a sheltered location is recommended.• Angle the camera between 30~60° down from the level position.• Install the camera between 8-16ft (2.5-5m) off of the ground.Level position (i.e., ceiling)Optimal angle NOTE: Accuracy of person and vehicle detection will be influenced by multiple factors, such as the object’s distance from the camera, the size of the object, and the height and angle of the camera. Night vision will also impact the accuracy of detection.Search through and play video recordings from the hard drive.Playback . If prompted, log in using the system user name (default:Back up recordings from the hard drive to a USB flash drive (not included).aba b c defgba cTo filter for person and vehicle recordings:Click to stopcurrent playback.d eHover over the icon. Check Person and Vehicle to filter available playback events.Wait a few seconds for the video bar to show detection events.Smart Motion Detection on any and all channels you would like to filter for person and vehicle detection. See the section Motion & Smart Motion Detection for details.a b dcgea. Select the channel you would like to configure.Click Enable .Click Setup next to Area to set an active area for motiondetection. See Figure 1 below for details.Click Setup next to Schedule to set the weekly schedulefor motion detection. See Figure 2 below for details.e. Set preferences for how the systemreacts to motion detection events.f. Click Smart Motion Detection toenable person and vehicle detection. See Figure 3 below for details.g. Click Apply to save changes.fFigure 1: Motion Detection Area• Click Enable to allow detection of people and vehicles The default schedule, shown in Figure 2, is active at all times. Click Modify to change the schedule for the corresponding day of the week.Click OK when finished.Figure 3: Smart Motion DetectionFigure 2: Motion Schedule• The camera image appears with a red grid overlay. This means the entire image is enabled for motion detection. • Click or click-and-drag to add / remove boxes from the active area. Cells that have been removed from the active area appear green.• Hover near the top of the image to reveal zone selection. You can set up to 3 different zones with different sensitivity and threshold values.• Right-click when finished.. If prompted, log in using the system user name (default: admin ) andon the far-left, then click the Deterrence tab.Set preferences for automatic warning light triggering on compatible Lorex deterrence cameras.For a complete list of compatible deterrence cameras, navigate to your recorder series at NOTE: For more information on configuring your Face Database andFace recognition set-up, refer to the DVR’s manual at .• The camera image appears with a grid overlay. The green area is the active area for deterrence. • Click or click-and-drag to add / remove boxes from the active area.• In Figure 4, only motion around the doorway will trigger warning light.Right-click when finished.• The default schedule, shown in Figure 5, is active during the night, between 5pm and 7am. • Click Modify to change the schedule for the corresponding day of the week.• Click OK when finished.a. Select the channel of a connecteddeterrence camera.b. Check Enable .c. Click Setup next to Warning Light toconfigure preferences.d. Click Setup next to Area to set ana ab bccd feactive area for automatic deterrence. See Figure 4 below for details.e. Click Setup next to Schedule to set the weekly schedule forautomatic deterrence. See Figure 5 below for details.f.Click Apply .Figure 5: Deterrence ScheduleFigure 4: Deterrence AreaConfiguring Deterrence SettingsTo set off all connected deterrence cameras’ warning lights and sirens, press and hold the front panel button for 3 seconds.Insert a formatted USB flash drive (not included) into a free USB port on the recorder.Main Menu . If prompted, log in using the system user name (default:To quickly open a window that displays vital system information such as device ID, model number, Quick Access to System InformationTap the button on the front panel of the recorder.Right-click to open the Quick Menu and click Info .ORIn live view, hover the mouse cursor over the top of the screen to open the Navigation Bar. Move the mouse cursor away from the top of the screen to close the Navigation Bar.During live view: Use the scroll wheel to zoom in / out.To configure face detection:Click Face Settings on the far-left, thenclick the Face Detection tab.Configure the following:。
SURFACTANT TOOLKit表面活性剂
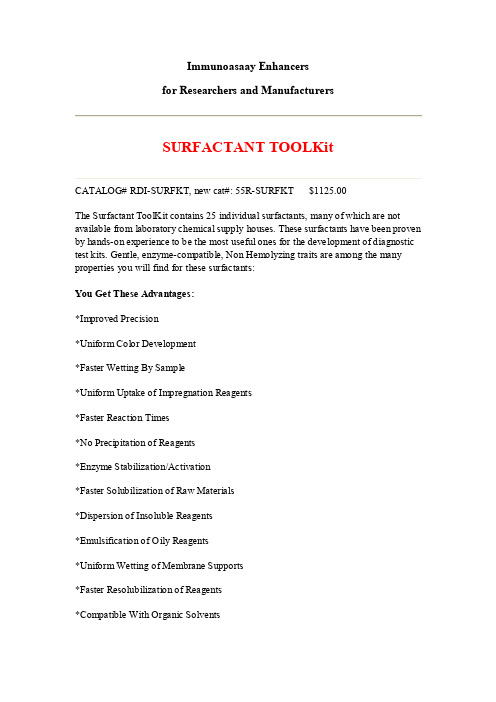
Immunoasaay Enhancersfor Researchers and ManufacturersSURFACTANT TOOLKitCATALOG# RDI-SURFKT, new cat#: 55R-SURFKT $1125.00The Surfactant ToolKit contains 25 individual surfactants, many of which are not available from laboratory chemical supply houses. These surfactants have been proven by hands-on experience to be the most useful ones for the development of diagnostic test kits. Gentle, enzyme-compatible, Non Hemolyzing traits are among the many properties you will find for these surfactants:You Get These Advantages:*Improved Precision*Uniform Color Development*Faster Wetting By Sample*Uniform Uptake of Impregnation Reagents*Faster Reaction Times*No Precipitation of Reagents*Enzyme Stabilization/Activation*Faster Solubilization of Raw Materials*Dispersion of Insoluble Reagents*Emulsification of Oily Reagents*Uniform Wetting of Membrane Supports*Faster Resolubilization of Reagents*Compatible With Organic Solvents*Reproducible Sample Uptake*No Air Bubbles in the SupportThe use of surfactants in in vitro diagnostic test kit is well known to those skilled in the art, but the surfactants may not be readily available to laboratory investigators who are new to this technolog. The Surfactant ToolKit distributed by RDI Division of Fitzgerald Industries Intl is the first step to solving this problem. Even experienced reagent developers will find new surfactants and properties which they have not used before.What You Will Receive.....We include 25 cationic, anionic, nonionic, and amphoteric surfactants. Bottles contain 20 to 25 grams of each surfactant. Included for each surfactant are its trade name, chemical names, HLB, formula weight, and examples of its function, all in a handy clamshell case. MSDS sheets are available for each surfactant.Are You In Any of these Areas? Then this kit is for YOU!*Immobilized Particles*Dry Reagent Strip Tests*Medical Diagnostic Test Kits*Environmental Test Kits*Immunoassay Test Kits*ELISA Test Kits*Bioremediation*Agricultural Test Kits*Contamination Residue Clean-up*Pesticide SolubilizationReagent Kit Optimization using the Surfactant ToolKitFirst, decide what basic problems are inherent in your test. Are they manufacturing or performance problems? Manufacturing problems are generally related to reagent solubility, poor stability of the stock reagent dip, uneven impregnation/coating of the web support, air bubbles in the device, wetability, or inadequate emulsification. Performance problems typically are poor precision, lack of lot-to-lot reproducibility, low signal to background noise ratio, limited range of response, and/or limited stability/shelf-life. All these are surfactant-related problems which could be preventing your test from performing better.Next, design a screening experiment that clearly measures the characteristics to be improved. It is best to screen all 25 of the surfactants in the kit, if possible.Incorporate the 25 surfactants individually into formulation at low and high concentration levels (if you don't know what to pick, try 0.3 and 0.8%). Don't neglect to use a surfactant in each solution which will be a separate component of the kit. some surfactants may not be soluble.You will now have as many as 50 formulations. Test these formulations under full assay conditions.Now, simply look for the formulation(s) with the best performance characteristics. You may want to fine-tune for the optimal surfactant concentration for the one chosen.It's possible that more than one surfactant will be desirable in your formulation, if you have multiple performance characteristics to fix; most surfactants can be combined in together. Don't forget to choose surfactant levels above or below the level that gave good results. You may want to use formula optimization techniques at ths point. Additional technical assistance and optimization protocols may be available on custom contract-inquire for costs and details.If you obtained some improved performance with one or more of the surfactants but desire even more improvement, contact us. We can make available to you surfactants which have properties similar to the ones in the kit but were not included. For each surfactant in the kit, many more are usually available which have similar but distinct structures. Don't stop short of the desired performance characteristics you are seeking. Surfactants are not the only ingredients to give improved performance & manufacturability. Polymers, Functional Materials,Biocides & Preservatives can also be key ingredients to accomplish your objectives. See our other developers kits.Surfactant Definitions and FunctionsSurfactant-an acronym for surface active agent. This is a more modern term than detergent, generally taking the place of the term detergent when referring to wetters, solubilizers, emulsifiers, and other items defined below. Surfactants almost always work to reduce attractive interactions between like particles and bring them to unlike surfaces. Surfactant is the name we use as a general term for all our Surfactant ToolKit items.Wetting- this is simply the action of a surfactant to reduce the surface tension of a liquid. It does this by reducing like attractions of molecules with each other (such as with water) and increase the attraction towards an unlike surface. This is extremely important when reconstituting dry powders, dry beads, or reagents in solid-phase devices. If the speed and evenness of reconstitution is important, then a good wetting agent is essential. Trition X305, Surfactant 10G, Silwet L7600, and BioTerge AS-40 are all very good wetters.Solubilizers - these are surfactants that can effect a solubilization of an otherwise insoluble material. If a concentration of surfactant is made high enough, micelle structures are formed. Insoluble molecules are then incorporated into the micelles and brought into apparent solution. Oftentimes reagent developers are faced with the dilemma of having to use water-insoluble substances in aqueous solutions, orwater-soluble substances in organic solvents. Surfactants with good solubilizer properties can solve either problem. We once used Ninate 411 to solubilize a diazonium salt in acetone.Emulsification - this is the property of surfactants to form a stable emulsion of two or more immiscible liquids. This is a little like micellular solubilization, but the resultant solubilizing particles are much bigger. Occasionally you might have a reagent in an oil form that needs to go into an aqueous solution.There are several surfactants such as Trition X45, BRIJ 35, the TWEENs. and Igepal CA210, that can help with this.Dispersion - an important property of surfactants that keeps insoluble particles in suspension. They do this by preventing insoluble particles from aggregating with each other. The smaller the particles, the more stable the dispersion formed. Have you ever scaled up a reagent prep and had the pilot supervisor point out a bunch of unsightly crud settling out, hithert unseen at the bench? Your worst nightmare! Sometimes even the best of efforts will not keep things in solution, and then what you need is a surfactant with good dispersing properties. Tetronic 1307,Geropon T-77, and Rhodasurf ON-870 are good choices here.Detergency - the ability of a surfactant to remove particles from a surface. We use this narrow definition to describe a detergent. Liken this function to the cleaning action of a surfactant to lift soil from a fabric. In the reagent development setting, it might bedesirable to release reagents from membranes, paper filters, glass surfces and the like upon wetting. Surfactants with long, straight carbon chains, such as Bio-TergeAS-40,Standapol ES-1, and Chemal LA-9, are often the most effective here.rev:July 14, 1997Surfactant Toolkit Contents: (subject to change without notice-see insert with each kit)1. Amine alkylbenzene sulfonate, Trade Name: NINATE 411, Class A, MW: 385,Function: Solvent compatible,good enulsifier.2. Poly(oxyethylene-co-oxypropylene) block polymer: Trade Name:Pluorinic (tm) F68, Class N, MW 8400, Function: excellent solubilizerand detergent; probablynon-hemolytic3. Telomer B monoether with polyethylene glycol,Trade name: Zonyl(tm)FSN 100, Class N, Function: Excellent wetter at very low concentration.Soluble in 30% IpOH, alcohols.4. Sodium dioctylsulfosuccinate, Trade name: Aerosol(tm) OT 100%. Class A, MW 445, Function: Useful in solvent/TMB systems,a strong wetter and emulsifier, good antifog, mold-release and dispersing properties.5. Sodium N-oleyl-N-methyltaurate, Trade name GEROPON(tm) T-77, Class A, MW 425, Function: Good wetting, dispersing properties.6. Sodium olefin(C(14)-C(16)) sulfonate, Trade name BIO-TERGE(tm) AS-40, Class A, MW 315, Function: Mild, solvent-compatible.7.Sodium polyoxyethylene(1) lauryl sulfate, Trade name STANDAPOLES-1, Class A, MW 346, Function: A strong anionic surfactant. Contains 2 parts in 5 sodium lauryl sulfate.8.`Benzalkonium (C(8)-C(18)) chloride, Class C, MW Mixture, Function, Antimicrobial,solvent compatible.9. Ethylenediamine alkoxlate block copolymer, Trade name, Tetronic(tm) 1307, Class M, HLB >24, MW 18600, Function: Non-hemolytic. solvent compatible, antistatic: good defoamer, disperser.10.2,4,7,9-Tetramethyl-5-decyne-4,7-diol ethoxylate (10), Trade name: Surfynol(tm) 465, Class: N, HLB: 13, MW: Mixture, Function: Non-hemolytic; good wetter and defoamer.11.2.4.7.9-Tetramethyl-5-decyne-4, 7-diol ethoxylate (30), Trade name: Surfynol(tm) 485, Class: N, HLB: 17, MW: Mixture, Function: Non-hemolytic, enzyme-compatible: good wetter and defoamer.12.Octylphenol ethoxylate (1.2), Trade name: IGEPAL(tm) CA210, Class: N, HLB: 4.6, MW: 272, Function: Solvent compatible, good emulsifier.13.Octylphenoxypolyethoxy (5)ethanol, Trade name: TRITON(tm) X-45, Class N, HLB: 10.4, MW: 426, Function: Solvent compatible: good emulsifier and dispersant.14.Octylphenoxypolyethoxy(9-10)ethanol, Trade name: TRITON(tm) X-100, Class: N, HLB: 13.5, MW: 625, Function: Emulsification, wetting, dispersing; very enzyme compatible.15.Octylphenoxypolyethoxy(30)ethanol, Trade Name: TRITON(tm) X305, Class: N, HLB: 17.3, MW: 1526, Function: Non-hemolytic, very water soluble: good wetter and emulsifier.16.Polydimethylsiloxane methylethoxylate, Trade name: SILWET(tm) L7600, Class N, HLB: 13-17, MW: 4000, Function: Non-hemolytic, silicone-based; water soluble, antifogging.17.Polyethoxylated(20) oleyl alcohol, Trade name: RHODASURF ON-870, Class: N, HLB: 15.4, MW: 1148, Function: Emulsifier,solubilizer, dispersant.18.Polyethoxylated(35) castor oil, Trade name: Cremophor(tm) EL, Class N, HLB:12-14, MW: NA, Function: Non-hemolytic, solvent compatible: emulsifier, solubilizer.19. Polyoxyethelene(20)sorbitan monolaurate, Trade name: TWEEN(tm) 20, Class: N, HLB: 16.7, MW: 1228, Function: Non-hemolytic, very hydrophillic; solubilizer, emulsifier.20.Polyoxyethelene(20)sorbitan monooleate, Trade name: TWEEN(tm)80,Class: N, HLB: 15 MW: 1310, Function: Non-hemolytic,very hydrophillic: solubilizer, emulsifier.21.Polyoxyethylene(23) dodecyl ether, Trade name: BRIJ(tm) 35, ClassN, HLB: 16.9, MW: 1200, Function: Good Emulsifier.22.Polyoxyethylene(9) lauryl alcohol, Trade name: CHEMAL LA-9, Class: N, HLB: 13.3, MW: 583, Function: Protein solubilization.Same structure as THESIT(tm).23.Poly(oxyethylene-co-oxypropylene) block copolymer, Trade name: Pluranic(tm)L64, Class: N, HLB: 12-18, MW: 2900, Function: Non-hemolytic, solvent compatible: excellent wetter,good emulsifier.24.p-[sonomylphenoxypoly(glycidol), Trade name: SURFACTANT 10G, Class: N, HLB: 12.4, MW: Mixture, Function: Non-hemolytic, v.enzyme-compatible, low foaming: good wetter.25.Sorbitan Monostearate, Trade name: SPAN(tm) 60, Class: N, HLB: 4.7, MW: 431, Function: Oil soluble, solvent-compatible, water insoluble.N = nonionic A = anionicC = cationic M = amphotericHLB = Hydrophile-Lipophile Balance Number. The higher the number, the more hydrophilic the surfactant. Only applies to non-ionics.NINATE(tm) and BioTerge(tm) are registered trademarks of Stephen Co. Aerosol(tm) is a registered trademark of American Cyanamide Co. Geropon(tm) and Igepal(tm) are registered trademarks of Rhone-Poulenc,Inc. Pluronic(tm), Tetronic(tm), and Cremophor(tm) are registered trademarks of BASF Corp. Surfynol(tm) is a registered trademark of Air Products and Chemicals Inc. TRITON(tm) and SILWET(tm) are registered trademarks of Union Carbide. TWEEN(tm), BRU(tm), and SPAN(tm) are registered trademarks of ICI Americas, Inc. Zonyl(tm) is a registered trademark of Du Pont Chemicals.Applications Notes (additional information providied with the kit=here is a sample): Bio-Terge AS-40. tmWe used this surfactant effectively in solving some wetting and solubility problems during the development of a dry-phase urinary test device. We were experiencing a mottled color response and somewhat slow reaction times. We had a water-insoluble indicator that had resolubilize in an aqueous biological fluid, knew that wetting and solubility were the chief problem areas. We also knew that our current surfactnt, being applied in a pH 9 aqueous system, was helping somewhat, and thus launched a screen over a dozen likely surfactant choices. Bio-Terge AS-40,a mild anionic surfactant, stood out head-and-shoulders above the other candidates. The color response was strikingly better in appearance, with significant reaction speed gains.Ninate 411. tmIn another impregnation mix, we were using methylisobutylketone (MIBK) as impregnation solvent. This was a highly desirable choice over acetone for both safety and reagent stability (MIBK does not absorb water) reasons. Unfortunately, the diazonium salt we were using precipitated out of solution during the impregnation run and could not be substituted by another. We had been using no surfactant at all, and in fact rarely if ever did in organic solvents. A screen for MIBK soluble surfactants produced 6 or 8 candidates, which we then tested to hold the diazonium salt in solution. Ninate 411 was the clear winner, and now afforded us unlimited pot-life times with regard to diazonium salt stability.Aerosol OT 100%. tmThis surfactant is essential for tetramethylbenzidine (TMB) stability. TMB is an oxidative-type indicator that readily overoxi-izes to a cruddy brown color that is undesirable for either visual or instrumental readout. Aerosol OT stops the oxidation process at the nice classic robins-egg blue color. Aerosol OT is believed to accomplish this through a complexing with the TMB. The complex is tightest at the first oxidation state of TMB, and is thus stabilized at that point. Aerosol OT/TMB systems are soluble in organic solvents such as toluene. It can also be used in aqueous solutions, in which case TMB-HCI would be the appropriate indicator form.Sodium cholate/deoxycholate.These are important surfactants for cholesterol chemistries. Cholates are effective cholesterol solubilizers and cholesterol enzyme activators due to the common steroidal-like backbone structure of these surfactants and cholesterol. It is a good idea to try more than one cholate in a screen, since solubility characteristics can vary with factors such as pH and ionic strength.SILWET L7600. tmThis is a unique siloxane-based surfactant with excellent wetting power. SILWETL7600 is among the few surfactants capable of wetting Teflon (PTF E) surfaces. It is highly soluble in both water and many organic solvents. We found this surfactant particularly useful once in a the development of a solid-phase whole-blood diagnostic device that was yielding biased numbers at high RBC hematocrit. A large array of surfactants was tested, and this was the only one that could eliminate the bias. It was thought that this surfactant through its powerful action at interfaces was interference by RBCs at the RBC-test surface interface. This surfactant is totally non-hemolytic. Typical use level is from 0.01 to 1%.Surfynot 465/485. tmThis pair of surfactants is also very gentle on red blood cells and can be placed directly in materials used to adsorb or filter RBCs. Both surfactants improve the stability of latex suspensions when used either as sole emulsifying agent or in conjunction with other emulsifying surfactants. They are low-foaming, high-wetting,and are the surfactants of choice for achieving an even coating of membranes withreagents.Surfybnot 485 has a higher HLB and is thus somewhat more water soluble, although both are soluble in water to levels greater than 1.0%. Surfynol 465 has slightly lower surface tension.Note: All products are for in vitro research or futher manufacturing use only. Use of any tradenames does not offer any license for their use. Tradenames are the property of their respective owners.。
ChannelEstimation
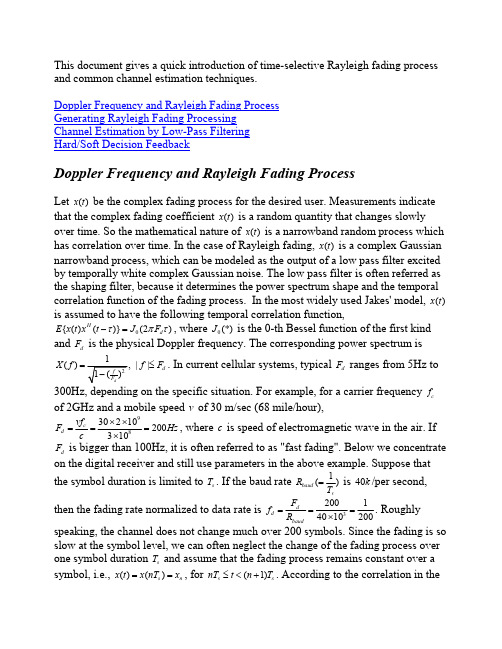
This document gives a quick introduction of time-selective Rayleigh fading process and common channel estimation techniques.Doppler Frequency and Rayleigh Fading Process Generating Rayleigh Fading Processing Channel Estimation by Low-Pass Filtering Hard/Soft Decision FeedbackDoppler Frequency and Rayleigh Fading ProcessLet ()x t be the complex fading process for the desired user. Measurements indicate that the complex fading coefficient ()x t is a random quantity that changes slowly over time. So the mathematical nature of ()x t is a narrowband random process which has correlation over time. In the case of Rayleigh fading, ()x t is a complex Gaussian narrowband process, which can be modeled as the output of a low pass filter excited by temporally white complex Gaussian noise. The low pass filter is often referred as the shaping filter, because it determines the power spectrum shape and the temporal correlation function of the fading process. In the most widely used Jakes' model, ()x t is assumed to have the following temporal correlation function,0{()()}(2)H d E x t x t J F τπτ−=, where 0(*)J is the 0-th Bessel function of the first kind and d Fis the physical Doppler frequency. The corresponding power spectrum is()||d X f f F =≤. In current cellular systems, typical d F ranges from 5Hz to300Hz, depending on the specific situation. For example, for a carrier frequency c f of 2GHz and a mobile speed v of 30 m/sec (68 mile/hour),9830210200310c d vf F Hz c××===×, where c is speed of electromagnetic wave in the air. If d F is bigger than 100Hz, it is often referred to as "fast fading". Below we concentrateon the digital receiver and still use parameters in the above example. Suppose that the symbol duration is limited to s T . If the baud rate 1()baud s R T =is 40k /per second, then the fading rate normalized to data rate is 320014010200dd baud F f R ===×. Roughly speaking, the channel does not change much over 200 symbols. Since the fading is so slow at the symbol level, we can often neglect the change of the fading process over one symbol duration s T and assume that the fading process remains constant over asymbol, i.e., ()()s n x t x nT x ==, for (1)s s nT t n T ≤<+. According to the correlation in thecontinuous case, the correlation function is 0{}(2)H n n m d E x x J f m π−=, whered d d s baudF f F T R == is the normalized Doppler frequency. The power spectrum of n xis ()||d X f f f =≤. At last, we point out that Jakes’ model in fact is mathematically derived rather than synthesized from field measurement. While Jakes’ model does fit some field measurement, other models from measurementcampaign might be more accurate in specific cases. For example, another normalized correlation function appears in a contribution to IEEE 802.16 specification for broadband wireless access, whose power spectrum is24()1 1.720.785,||d X f f f f f =−+≤.Generating Jakes’ Rayleigh Fading ProcessingA common method to generate n x is to sum up several sinusoids, as first suggested inJakes’ book. However, this method in fact generates a deterministic process and not a truly random process. Nevertheless, it is still widely used due to its simplicity. Here are the C++ implementation and COM implementation of a modified Jakes’ model proposed in this paper by P. Dent et al . Another way is to pass white complex Gaussian noise n uthrough the following shaping filter, ()||d h f f f =≤,which is the square root of the power spectrum of n x . This filter is highly nonlinearand approximation has to be sought. Since the shaping filter has infinite impulseresponse, it is natural to seek an IIR filter for approximation. JTC recommends using a 32 order IIR filter to approximate ()h f with normalized fading rate d f of 12andthen generating slower fading process by interpolating the output from the IIR filter.A simpler method is to use an autoregressive (AR) filter to approximate ()h f , whose coefficients can be calculated from the correlation function of n x via the Yuler-Walker equation.Channel Estimation by Low-Pass FilteringThe model for flat fading channel can be expressed as, n n n n y x d v =+, where n y is the received signal and n v is the complex additive white Gaussian noise. n d is the n thtransmitted symbol and in this documentation is limited to BPSK signals taking values of 1±. We first assume that the transmitted data is known to the receiver, forexample, n d is either a known pilot symbol or a highly reliable decision. So we canalternatively write the model as H H n n n n n y d x v d =+, where we want to recover thenarrowband process n x buried in white noise H n n v d .Figure 1: Channel Estimation via Low-pass FilteringThe engineering approach is low-pass filtering of the modulation-free signal H n n y d . Low-pass filtering itself is a well studied problem, both mathematically andpractically. If the temporal correlation of the fading process is known, Wiener filter is the optimal filter. Particularly, if the fading process is generated by the IIR filter or AR filter, a Kalman filter can carry out the estimation recursively, which is computationally efficient. Note that temporal correlation is a function of thenormalized Doppler frequency and is unknown unless the vehicle speed is known. If the vehicle speed is unknown, adaptive algorithms can be used to extract the narrowband process n x from the background noise. A popular adaptive filter is thelinear predictor, where LMS or RLS is used to minimize the prediction error. There are also methods to estimate the coefficients of the AR filter when Kalman filtering is carried out. Approaches above are pursuing the optimality of the channel estimator by adapting the low-pass filter to the bandwidth/shape of the shaping filter. As a practical matter, the low-pass filter can be designed as a fixed filter to handle the maximum Doppler frequency possible, for example, a brick-wall low-pass filter with bandwidth max f . This worst-case design has been shown numerically to be robust invarious situations and the performance degradation from "optimal" filters is minimal. The reason is that typically the maximum normalized Doppler frequency is fairlysmall (for example, 1in the first paragraph) and the fixed low-pass filter can200average over sufficient number of symbols to obtain accurate channel estimate for detection. An "optimal" filter could have averaged over even more symbols to further improve the channel estimate, but bit-error-rate is dominated by the additive noisevn and will not be significantly lowered by the better channel estimate. On the other hand, if the bandwidth of the fixed low-pass filter is smaller than the true normalized Doppler frequency, the channel estimate can not keep up with channel variation and the BER degradation is more severe. Since channel estimation only incurs moderate complexity, most cellular systems employ coherent demodulation to take advantage of its 2-3dB gain over non-coherent demodulation.A useful BER expression can be found in the Appendix C of "Digital Communications" by John G. Proakis, which takes into account the impact of channel estimation error when maximal ratio combining is used. This article by me further analyzes the impact of channel estimation error in the presence of strong co-channel interference.Hard/Soft Decision FeedbackUp to now, we have assumed that the symbold is somehow known and we use n dnto remove the modulation fromy. While we can insert many pilot symbols to makenthis assumption true, the overhead consumes a lot of energy and bandwidth. A more attractive approach is to use decisions as addition pilot symbols as follows. Suppose the transmission is in blocks. After the initial channel estimate is carried out, the data block is detected. While we might make wrong symbol decisions, we still remove the modulation with these decisions and re-estimate the channel using the low-pass filter. Hopefully the correct decisions can outweigh wrong decisions and output of the low-pass filter is a better channel estimate than the initial channel estimate. This better channel estimate in turn can lead to better decisions. This procedure can be repeated several times. As found out by many practitioners, this iterative hard decision feedback method does provide decent BER even if the number of known pilot symbols is quite small. In fact, if the shaping filter is an IIR/AR filter and the channel estimator is a Kalman filter, it can be formally proved that each iteration can increase the likelihood of the demodulated symbol sequence, which justifies the validity of this intuitive idea of hard decision feedback.Figure 2: Iterative Receiver with Soft Decision FeedbackIn a fast fading environment, further improvement can be obtained by using "soft" decisions. That is, if the decision is unreliable, it is discounted in re-estimating the channel. For BPSK signals, the implementation is to multiply n y with {}H n E d , the expected value of the (conjugate of the) symbol. The rationale is that the closer the signal is to the decision boundary, the more unreliable the decision is and the smaller the amplitude of expected value of the symbol. So by using soft decision (the expected value) rather than hard decision, an unreliable decision has less impact in re-estimating the channel. Similar to the hard decision feedback case, if the shaping filter is an IIR/AR filter and the channel estimator is a Kalman filter, it can be formally proved that each iteration can increase the a posteriori probability of the channel estimate, which justifies the validity of this idea of soft decision feedback. Interested readers are referred to Chapter 4 of my thesis for the proof. Through extensive simulations we find that when the fading is slow (say, 150d f <), hard decision feedback has similar BER performance as soft decision feedback, whereas if the fading is fast, soft decision feedback is better than hard decision feedback. Our conjecture is that when fading is slow, the channel estimator can average over many symbols, so the effect of a few wrong decisions can be averaged out. However, if the fading is fast, the channel estimator can only average over limited number of symbols and the effect of wrong decisions is much harder to be averaged out.Consequently soft decision feedback is more favorable in a fast fading environment.A rigorous proof of why soft decision back is better still remains elusive, because it needs to deal with the convergence property of the expectation-maximization algorithm.from the Previous StageSoft Limiter。
数字通信中的多抽样率信号处理中英文翻译(部分)

数字通信中的多抽样率信号处理中英⽂翻译(部分)Multirate Signal Processing Concepts in Digital CommunicationsBojan VrceljIn Partial Fulfillment of the Requirementsfor the Degree ofDoctor of PhilosophyCalifornia Institute of TechnologyPasadena, California2004 (Submitted June 2, 2003)AbstractMultirate systems are building blocks commonly used in digital signal processing (DSP). Their function is to alter the rate of the discrete-time signals, which is achieved by adding or deleting a portion of the signal samples. Multirate systems play a central role in many areas of signal processing, such as filter bank theory and multiresolution theory. They are essential in various standard signal processing techniques such as signal analysis, denoising, compression and so forth. During the last decade, however, they have increasingly found applications in new and emerging areas of signal processing, as well as in several neighboring disciplines such as digital communications.The main contribution of this thesis is aimed towards better understanding of multirate systems and their use in modern communication systems. To this end, we first study a property of linear systems appearing in certain multirate structures. This property is called biorthogonal partnership and represents a terminology introduced recently to address a need for a descriptive term for such class of filters. In the thesis we especially focus on the extensions of this simple idea to the case of vector signals (MIMO biorthogonal partners) and to accommodate for nonintegral decimation ratios (fractional biorthogonal partners).Some of the main results developed here pertain to a better understanding of the biorthogonal partner relationship. These include the conditions for the existence of stable and of finite impulse response (FIR) biorthogonal partners. A major result that we establish states that under some generally mild conditions, MIMO and fractional biorthogonal partners exist. Moreover, when they exist, FIR solutions are not unique. We develop the parameterization of FIR solutions, which makes the search for the best partner in a given application analytically tractable. This proves very useful in the central application of biorthogonal partners, namely, channel equalization in digital communications with signal oversampling at the receiver. Sampling the received signal at a rate higher than that defined by the transmitter provides some flexibility in the design of the equalizer. A good channel equalizer in this context is one that helps neutralize the distortion on the signal introduced by the channel propagation but not at the expense of amplifying the channel noise. This presents the rationale behind the partner design problem which is formulated and solved. Theperformance of such equalizers is then compared to several other equalization methods by computer simulations. These findings point to the conclusion that the communication system performance can be improved at the expense of an increased implementational cost of the receiver.While the multirate DSP in the aforementioned communication systems serves to provide additional degrees of freedom in the design of the receiver, another important class of multirate structures is used at the transmitter side in order to introduce the redundancy in the data stream. This redundancy generally serves to facilitate the equalization process by forcing certain structure on the transmitted signal. If the channel is unknown, this procedure helps to identify it; if the channel is ill-conditioned, additional redundancy helpsVavoid severe noise amplification at the receiver, and so forth. In the second part of the thesis, we focus on this second group of multirate systems, derive some of their properties and introduce certain improvements of the communication systems in question.We first consider the transmission systems that introduce the redundancy in the form of a cyclic prefix. The examples of such systems include the discrete multitone (DMT) and the orthogonal frequency division multiplexing (OFDM) systems. The cyclic prefix insertion helps to effectively divide the channel in a certain number of nonoverlaping frequency bands. We study the problem of signal precoding in such systems that serves to adjust the signal properties in order to fully take advantage of the channel and noise properties across different bands. Our ultimate goal is to improve the overall system performance by minimizing the noise power at the receiver. The special case of our general solution corresponds to the white channel noise and the best precoder under these circumstances simply performs the optimal power allocation.Finally, we study a different class of communication systems with induced signal redundancy, namely, the multiuser systems based on code division multiple access (CDMA). We specifically focus on the special class of CDMA systems called `a mutually orthogonal usercode receiver' (AMOUR). These systems use the transmission redundancy to facilitate the user separation at the receiver regardless of the (different) communication channels. While the method also guarantees the existence of the zero-forcing equalizers irrespective of the channel zero locations, the performance of these equalizers can be further improved by exploiting the inherent flexibility in their design. Weshow how to find the best equalizer from the class of zero-forcing solutions and then increase the size of this class by employing alternative sampling strategies at the receiver. Our method retains the separability properties of AMOUR systems while improving their robustness in the noisy environment.Chapter 1 IntroductionThe theory of multirate digital signal processing (DSP) has traditionally been applied to the contexts of filter banks [61], [13], [50] and wavelets [31], [72]. These play a very important role in signal decomposition, analysis, modeling and reconstruction. Many areas of signal processing would be hard to envision without the use of digital filter banks. This is especially true for audio, video and image compression, digital audio processing, signal denoising, adaptive and statistical signal processing. However, multirate DSP has recently found increasing application in digital communications as well. Multirate building blocks are the crucial ingredient in many modern communication systems, for example, the discrete multitone (DMT), digital subscriber line (DSL) and the orthogonal frequency division multiplexing (OFDM) systems as well as general filter bank precoders, just to name a few. The interested reader is referred to numerous references on these subjects, such as [7]-[9], [17]-[18], [27], [30], [49], [64], [89], etc.This thesis presents a contribution to further understanding of multirate systems and their significance in digital communications. To that end, we introduce some new signal processing concepts and investigate their properties. We also consider some important problems in communications especially those that can be formulated using the multirate methodology. In this introductory chapter, we give a brief overview of the multirate systems and introduce some identities, notations and terminology that will prove useful in the rest of the thesis. Every attempt is made to make the present text as self-contained as possible and the introduction is meant to primarily serve this purpose. While some parts of the thesis, especially those that cover the theory of biorthogonal partners and their extensions provide a rather extensive treatment of the concepts, the material regarding the applications of the multirate theory in communication systems should be viewed as a contribution to a better understanding and by no means the exhaustive treatment of such systems. For a more comprehensive coverage the reader is referred to a range of extensive texts on the subject, for example, [71], [18], [19], [39], [38], [53], etc.1.1 Multirate systems 1.1.1 Basic building blocks The signals of interest in digital signal processing are discrete sequences of real or complex numbers denoted by x(n), y(n), etc. The sequence x(n) is often obtained by sampling a continuous-time signal x c(t). The majority of natural signals (like the audio signal reaching our ears or the optical signal reaching our eyes) are continuous-time. However, in order to facilitate their processing using DSP techniques, they need to be sampled and converted to digital signals. This conversion also includes signal quantization, i.e.,discretization in amplitude, however in practice it is safe to assume that the amplitude of x(n) can be any real or complexSignal processing analysis is often simplified by considering the frequency domain representation of signals and systems. Commonly used alternative representations of x(n) are its z-transform X (z) and the discrete-time Fourier transform X (O'). The z-transform is defined as X(z) = E _.x(n)z-"', and X (e j") is nothing but X(z) evaluated on the unit circle z = e3".Multirate DSP systems are usually composed of three basic building blocks, operating on a discrete-time signal x(n). Those are the linear time invariant (LTI) filter, the decimator and the expander. An LTI filter, like the one shown in Fig.1.1, is characterized by its impulse response h(n), or equivalently by its z-transform (also called the transfer function) H(z). Examples of the M-fold decimator and expander for M = 2 are shown in Fig.1.2. The rate of the signal at the output of an expander is M times higher than the rate at its input, while the converse is true for decimators. That is why the systems containing expanders and decimators are called `multirate' systems. Fig.1.2 demonstrates the behavior of the decimator andthe expander in both the time and the frequency domains.XE(z) = [X (z)]IM XD(z) = [X (z)]iM = X(z M)1 M-1 1 j2 k =M E X(z e n a)k=0for M-fold expander, and (1.1)for M-fold decimator. (1.2)The systems shown in Figs.1.1 and 1.2 operate on scalar signals and thus are called single input-single output (SISO) systems. The extensions to the case of vector signals are ratherstraightforward: the decimation and the expansion are performed on each element separately. The corresponding vector sequence decimators/expanders are denoted within square boxes in block diagrams. In Fig.1.3 this is demonstrated for vector expanders. The LTI systems operating on vector signals are called multiple input-multiple output (MIMO) systems and they are characterized by a (possibly rectangular) matrix transfer function H(z).1.1.2 Some multirate definitions and identitiesThe vector signals are sometimes obtained from the corresponding scalar signals by blocking. Conversely, the scalar signals can be recovered from the vector signals by unblocking. The blocking/unblocking operations can be defined using the delay or the advance chains [61], thus leading to two similar definitions. One way of defining these operations is shown in Fig.1.4, while the other is obtained trivially by switching the delay and the advance operators. Instead of drawing the complete delay/advance chain structure, we often use the simplified block notation as in Fig.1.4. It is usually clear from the context which of the two definitions数字通信中的多抽样率信号处理Bojan Vrcelj博⼠学位论⽂加州技术学会Pasadena, 加州2004 (委托于2003.6.2)摘要多抽样率系统普遍是被运⽤在处理数字信号⽅⾯。
卡尔曼滤波算法英文
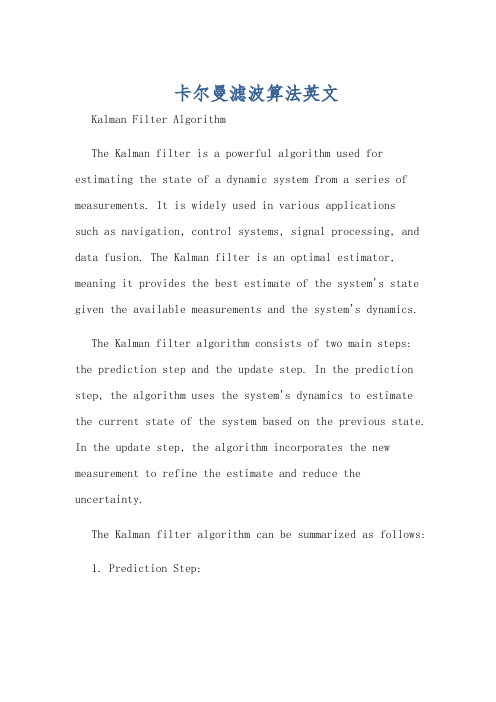
卡尔曼滤波算法英文Kalman Filter AlgorithmThe Kalman filter is a powerful algorithm used for estimating the state of a dynamic system from a series of measurements. It is widely used in various applicationssuch as navigation, control systems, signal processing, and data fusion. The Kalman filter is an optimal estimator, meaning it provides the best estimate of the system's state given the available measurements and the system's dynamics.The Kalman filter algorithm consists of two main steps: the prediction step and the update step. In the prediction step, the algorithm uses the system's dynamics to estimate the current state of the system based on the previous state. In the update step, the algorithm incorporates the new measurement to refine the estimate and reduce the uncertainty.The Kalman filter algorithm can be summarized as follows:1. Prediction Step:- State Prediction: The algorithm uses the system's dynamics to predict the current state of the system based on the previous state.- Covariance Prediction: The algorithm also predicts the covariance of the estimated state, which represents the uncertainty in the estimate.2. Update Step:- Kalman Gain Calculation: The algorithm calculates the Kalman gain, which determines the weight given to the new measurement in the update step.- State Update: The algorithm updates the estimated state by combining the predicted state and the new measurement, using the Kalman gain.- Covariance Update: The algorithm updates the covariance of the estimated state, reflecting the reduced uncertainty after incorporating the new measurement.The Kalman filter algorithm is recursive, meaning it can be implemented in an efficient and computationally-feasible manner. The algorithm only requires the current state and measurement, and it does not need to store or process allthe previous data. This makes the Kalman filterparticularly useful for real-time applications where computational resources are limited.The Kalman filter algorithm has several key properties that make it widely applicable:1. Optimality: The Kalman filter provides the optimal estimate of the system's state, given the available measurements and the system's dynamics.2. Robustness: The Kalman filter is robust to noise and uncertainties in the measurements and the system's dynamics.3. Versatility: The Kalman filter can be applied to a wide range of linear systems, including those with multiple inputs and outputs.4. Computational Efficiency: The Kalman filter algorithm can be implemented efficiently, making it suitable forreal-time applications.The Kalman filter algorithm has been extensively studied and applied in various fields, including:- Navigation: Kalman filters are used in GPS, inertial navigation systems, and autonomous vehicles to estimate the position, velocity, and orientation of the system.- Control Systems: Kalman filters are used to estimatethe state of a system and provide feedback for control algorithms, improving the system's performance andstability.- Signal Processing: Kalman filters are used to filter and smooth signals, removing noise and improving thesignal-to-noise ratio.- Data Fusion: Kalman filters are used to combinemultiple sources of information, such as sensors, toprovide a more accurate and reliable estimate of thesystem's state.Overall, the Kalman filter algorithm is a powerful and widely-used tool for estimating the state of dynamic systems. Its optimality, robustness, and computational efficiency make it a valuable asset in various applications.卡尔曼滤波算法卡尔曼滤波是一种用于估计动态系统状态的强大算法。
- 1、下载文档前请自行甄别文档内容的完整性,平台不提供额外的编辑、内容补充、找答案等附加服务。
- 2、"仅部分预览"的文档,不可在线预览部分如存在完整性等问题,可反馈申请退款(可完整预览的文档不适用该条件!)。
- 3、如文档侵犯您的权益,请联系客服反馈,我们会尽快为您处理(人工客服工作时间:9:00-18:30)。
Nadja S. Magalh˜ aes
Instituto Nacional de Pesquisas Espaciais C.P. 515, S˜ ao Jos´ e dos Campos - SP, 12201-970, BRAZIL
III. QUASI-MONOCHROMATIC SIGNALS
˜ (ω ) is well behaved. For example, if U (t) were a strictly Equation (2) is valid as long as U monochromatic wave like U (t) = h0 cos ω0 t, ˜ (ω ) = h0 δ (ω − ω0 ). it would be difficult to build this filter since U In order to use the optimal filter (2) in continuous gravitational wave detectors we will describe these waves as quasi-monochromatic useful signals. It means that the waves that reach the antenna will be of the form3 hxx (t) =
gravitational wave context yet.
II. THE FILTER THAT OPTIMIZES SNR
Linear, stationary filters obey the relation O(t) =
∞ −∞
k (t′ )I (t − t′ )dt′ .
k (t) is the impulse response function that characterizes the filter K , I (t) is the input at the filter and O(t) is the filter output. Generally1 I (t) has a useful part, U (t), and an unwanted part, N (t): I (t) = U (t) + N (t). We have a similar relation for the filter output, given by O(t) = U ′ (t) + N ′ (t). It is well known from noise theory [7] that the filter Ko that optimizes SNR at its output2 , SNR ≡ must have the following transfer function: Ko (ω ) = e−ıωt0 with Ko ≡
(4)
) ,t ≥ 0 2h0 e−at cos(ω0 t + π 4 2h0 e cos(ω0 t +
at π ) 4
,
πa , 2
(5)
,t ≤ 0 and the
The constant a is related to the signal spectral density bandwidth, ∆ωh = corresponding spectral density is of the form4 Sh ( ω ) = h2 2a 2a 0 . + 2 2 2 2 a + (ω − ω0 ) a + (ω + ω0 )2
arXiv:gr-qc/9312005v1 2 Dec 1993
Carlos O. Escobar
Instituto de F´ ısica Universidade de S˜ ao Paulo C.P. 20516, S˜ ao Paulo - SP, 01452-990, BRAZIL
Abstract
We determine the transfer functions of two kinds of filters that can be used in the detection of continuous gravitational radiation. The first one optimizes the signal-to-noise ratio, and the second reproduces the wave with minimum error. We analyse the behaviour of these filters in connection with actual detection schemes.
1
I. INTRODUCTION
The detection of gravitational waves (g.w.) is one of the most fascinating and challenging subjects in Physics research nowadays. Besides checking the General Relativity theory, the detection of this phenomenon will mark the beginning of a new phase in the comprehension of astrophysical phenomena by the use of gravitational wave astronomy. Although these waves were predicted at the beginning of the century [1], the research on their detection only started around 1960, with the studies of Joseph Weber [2]. The major obstacle to this detection is the tiny amplitude the g.w. have [3]. Even though the more sensitive detector now operating [4] is capable to detect amplitudes near h ∼ 5 × 10−19 , this value must be decreased by several orders of magnitude so that impulsive waves can be detected regularly. On the other hand, the discovery of pulsars with periods lying in the milliseconds range stimulated the investigations on the detection of gravitational waves of periodic origin. Although these waves generally have amplitudes even smaller than those emitted by impulsive sources, periodic sources are continuously emitting gravitational waves in space and they can be detected as soon as the correct sensitivity is reached. Since many of the resonant mass antennae now operating are designed to detect frequencies near 1000 Hz, the millisecond pulsars will probably be detected if these antennae ever become sensitive to amplitudes h ≤ 10−27 . This value is bigger if we consider the Crab pulsar (f ∼ 60Hz ): h ∼ 10−24 . There is a resonant mass detector with a torsional type antenna (CRAB IV) being developed by the Tokyo group [5] to detect gravitational waves emitted by the Crab pulsar. This group expects to reach h ∼ 10−22 soon. The main purpose of this paper is a contribution towards the increase in sensitivity of resonant mass continuous gravitational wave detectors looking at the use of adequate filters. We study two kinds of filters, the first optimizes the signal-to-noise ratio (SNR), and is normally used in the detection of impulsive waves [6]. The second filter reproduces the wave with minimum error. Both filters apparently were not investigated in the continuous 2