A Real-Time Catadioptric Stereo System Using Planar Mirrors
基于X射线的小动物成像micro_CT系统_李昊
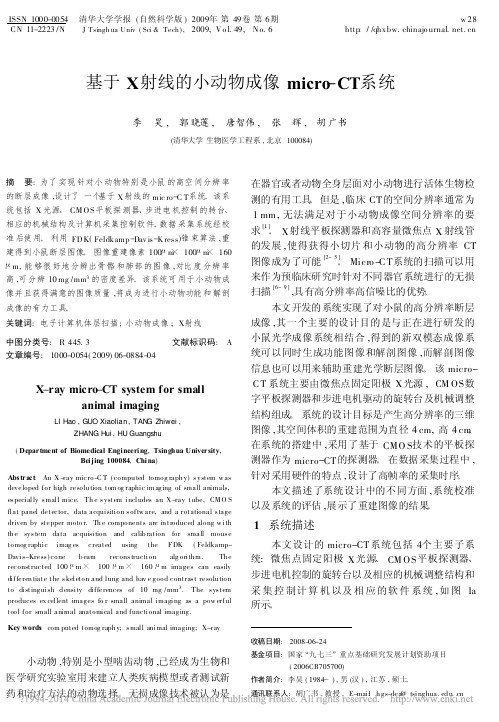
ISSN 1000-0054CN 11-2223/N 清华大学学报(自然科学版)J Tsingh ua Univ (Sci &Tech ),2009年第49卷第6期2009,V o l.49,N o.6w 28http ://qhx bw.chinajo 基于X 射线的小动物成像micro -CT 系统李 昊, 郭晓莲, 唐智伟, 张 辉, 胡广书(清华大学生物医学工程系,北京100084)收稿日期:2008-06-24基金项目:国家“九七三”重点基础研究发展计划资助项目(2006CB705700)作者简介:李昊(1984—),男(汉),江苏,硕士。
通讯联系人:胡广书,教授,E-mail :h gs-dea @tsinghua.ed 摘 要:为了实现针对小动物特别是小鼠的高空间分辨率的断层成像,设计了一个基于X 射线的mic ro -C T 系统。
该系统包括X 光源、CM O S 平板探测器、步进电机控制的转台、相应的机械结构及计算机采集控制软件。
数据采集系统经校准后使用。
利用FD K (Feldkamp -Dav is -K ress )锥束算法,重建得到小鼠断层图像。
图像重建像素100μm ×100μm ×160μm,能够很好地分辨出骨骼和肺部的图像,对比度分辨率高,可分辨10mg /mm 3的密度差异。
该系统可用于小动物成像并且获得满意的图像质量,将成为进行小动物功能和解剖成像的有力工具。
关键词:电子计算机体层扫描;小动物成像;X 射线中图分类号:R 445.3文献标识码:A文章编号:1000-0054(2009)06-0884-04X -ray micro -CT system for smallanimal imagingLI Hao ,G UO Xiaolian ,TAN G Zhiwei ,ZHANG Hui ,HU Guangshu(Department of Biomedical Engineering ,Tsinghua University ,Beijing 100084,China )Abs tract :An X-ray micro-C T (compu ted tomog raph y)s ystem w as developed for high resolu tion tom og raphic im aging of small animals,es pecially s mall mice.Th e s ystem includes an X-ray tube,CM O S flat panel detector,data acquisition s oftw are,and a rotational s tage driven by stepper moto r.Th e components are in troduced along w ith th e sys tem data acquisition and calib ration for small mous e tomog raphic imag es created using the FDK (Feldkamp-Davis-Kress )cone b eam recons truction alg orith m.Th e reconstructed 100μm ×100μm ×160μm images can easily differen tiate the s keleton and lung and hav e good contras t resolu tion to distinguish d ensity differences of 10mg /mm 3.The s ys tem produces ex cellent images fo r small animal imaging as a pow erful tool for small animal anatomical and functional imaging.Key words :com puted tomog raph y;s mall animal imaging;X-ray小动物,特别是小型啮齿动物,已经成为生物和医学研究实验室用来建立人类疾病模型或者测试新药和治疗方法的动物选择。
制冷型被动式消热差红外光学系统设计

文章编号 2097-1842(2023)04-0853-08制冷型被动式消热差红外光学系统设计李 康1,周 峰2 *,王保华1,宫 辉1,郑国宪1(1. 北京空间机电研究所, 北京 100076;2. 北京邮电大学,北京100876)摘要:在大温差条件下,由于温度剧烈变化导致红外光学系统成像质量变差。
用于机载林火监测的大视场中波红外相机工作环境变化剧烈,对杂散辐射要求较高。
为保证光学系统在要求的大视场和大温差条件下具有稳定的性能和良好的成像质量,通过基于消热差的设计方法和基于噪声等效温差的杂散辐射综合评价方法,设计了一套制冷型中波红外光学系统。
该光学系统由6片透镜和1片滤光片组成,工作波段为3.7~4.8 μm ,F 数为2.5,焦距为62.5 mm ,视场为14.36°×10.87°,探测器采用640×512 阵列中波制冷型探测器,通过采用硅、锗材料组合,合理分配光焦度,实现了消色差和消热差设计,通过冷反射优化和冷光阑匹配设计,较好地抑制了系统的杂散辐射噪声,通过引入少量非球面优化,在满足指标要求的情况下,对高阶像差进行了校正。
结果表明,光学系统在−55~+70 °C 温度范围内,成像质量稳定良好。
关 键 词:中波红外;制冷型探测器;消热差;冷反射中图分类号:TN216 文献标志码:A doi :10.37188/CO.2022-0205Passive athermalization design of a cooled infrared optical systemLI Kang 1,ZHOU Feng 2 *,WANG Bao-hua 1,GONG Hui 1,ZHENG Guo-xian 1(1. Beijing Institute of Space Mechanics and Electricity , Beijing 100076, China ;2. Beijing University of Posts and Telecommunications , Beijing 100876, China )* Corresponding author ,E-mail : zfsimon@Abstract : Under conditions with large temperature differences, the imaging quality of an infrared optical sys-tem will deteriorate due to severe temperature changes. Large field-of-view medium-wave infrared cameras for airborne forest fire monitoring work in drastically changing environments, so the optical system has high requirements for stray radiation. In order to ensure that the optical system performs stably and with good ima-ging quality in the large field-of-view and the required large temperature range, a cooled medium-wave in-frared optical system is designed based on athermalization and the comprehensive evaluation method of stray radiation based on noise equivalent temperature difference. The optical system consists of 6 lenses and 1 fil-ter with working wavelength of 3.7−4.8 μm, F-number 2.5, focal length 62.5 mm, and field of view 14.36°×10.87°, respectively. The pixel resolution of the medium-wave cooled detector is 640×512. By using a combination of silicon and germanium materials and reasonably distributing the optical power, achromatic aberration and athermalization designs are realized. Through cold reflection optimization and cold aperture matching, stray radiation noise in the system is well-suppressed. By a bit of aspheric optimization, higher-or-收稿日期:2022-09-28;修订日期:2022-11-02基金项目:高分辨率对地观测系统重大专项(民用部分)Supported by Major Projects for High Resolution Earth Observation System (Civilian Part)第 16 卷 第 4 期中国光学(中英文)Vol. 16 No. 42023年7月Chinese OpticsJul. 2023der aberrations are corrected based on the requirements. The results show that the imaging quality of the op-tical system is stable and good in the temperature range of −55~+70 °C.Key words: medium wave infrared;cooled detector;athermalization;cold reflection1 引 言森林是地表最重要的生态系统,基于飞艇机载的大视场中波红外成像系统是森林监测的重要工具。
LCOS微型投影仪中照明系统的设计
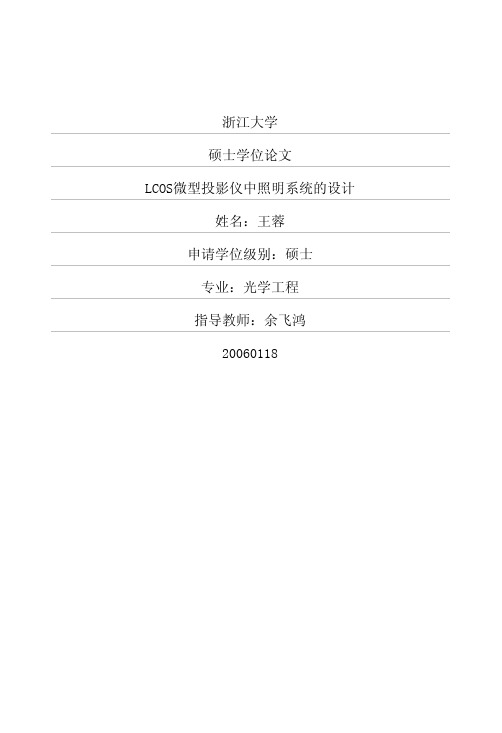
浙江大学硕士学住论文
被开发出来。它利用投影原理将阴极射线管的影像投射出去,其原理结构图1.4 所示。为了使图像达到足够的亮度,投影电视的阴极射线管亮度通常比直视型电 视要高,而显示的亮度又和加速电子的电压有关。高压下的辐射量越强,其危险 性叶相对提高。另外整个设备体积过于庞大,故不被普遍使用。
projectors,and different projecting view methods are classified.The structure and
principles of LCOS projectors are analyzed and design requirement of the illuminating system ofmicro LCOS projectors are put forward based on the principles and its development.
the viewing angles
of LED,and FF system could improve the efficiency of surface light source like
LED.arrays.Principles of the polarization converter and its coupling with the light
图1.1幻灯机基本结构图 但是幻灯片的制作成本较高,过程复杂而且置换幻灯片的程序繁琐,公元 1895年,法国人卢米埃尔发明了拉片结构,一个可以连续播放资料的装置。从 此电影放映机(Motion pictureprojector)诞生了。其系统结构图如图1.2所示。 在早期是用手摇或者发条的方式来控制拉片机,最高的拉片速率只能达到每幅图 约为1/24秒,可是人眼的视觉暂留时间为1/16秒。因此,观众会感到屏幕有闪 烁的现象。其解决方法是在电影胶片前加上一个双叶片遮光器,每移动一个画面, 遮光器便旋转一次,叶片便遮光两次。如此,可以将闪烁频率从每秒24次提升 至每秒48次,观众便可欣赏到更为清晰、稳定的图像。由于电影放映机所使用 的光源为弧光灯,机器温度容易上升,而拉片机的拉片速度不够快,放映的胶片 很容易被烧毁。另外放映机体积庞大,一般只在电影院里使用。
摄像机标定算法综述
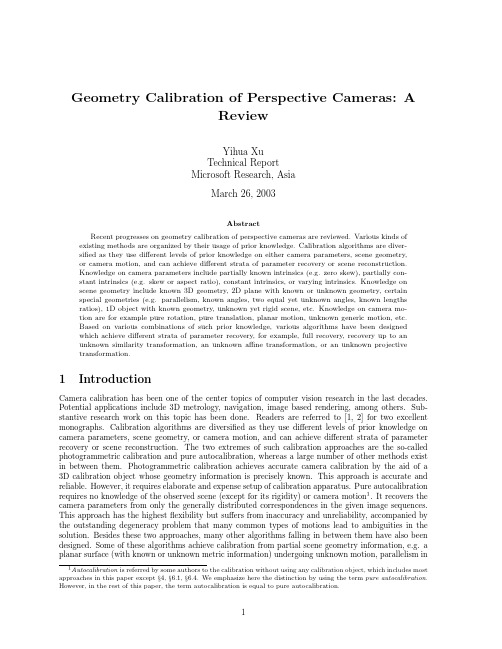
Geometry Calibration of Perspective Cameras:AReviewYihua XuTechnical ReportMicrosoft Research,AsiaMarch26,2003AbstractRecent progresses on geometry calibration of perspective cameras are reviewed.Various kinds of existing methods are organized by their usage of prior knowledge.Calibration algorithms are diver-sified as they use different levels of prior knowledge on either camera parameters,scene geometry,or camera motion,and can achieve different strata of parameter recovery or scene reconstruction.Knowledge on camera parameters include partially known intrinsics(e.g.zero skew),partially con-stant intrinsics(e.g.skew or aspect ratio),constant intrinsics,or varying intrinsics.Knowledge onscene geometry include known3D geometry,2D plane with known or unknown geometry,certainspecial geometries(e.g.parallelism,known angles,two equal yet unknown angles,known lengthsratios),1D object with known geometry,unknown yet rigid scene,etc.Knowledge on camera mo-tion are for example pure rotation,pure translation,planar motion,unknown generic motion,etc.Based on various combinations of such prior knowledge,various algorithms have been designedwhich achieve different strata of parameter recovery,for example,full recovery,recovery up to anunknown similarity transformation,an unknown affine transformation,or an unknown projectivetransformation.1IntroductionCamera calibration has been one of the center topics of computer vision research in the last decades. Potential applications include3D metrology,navigation,image based rendering,among others.Sub-stantive research work on this topic has been done.Readers are referred to[1,2]for two excellent monographs.Calibration algorithms are diversified as they use different levels of prior knowledge on camera parameters,scene geometry,or camera motion,and can achieve different strata of parameter recovery or scene reconstruction.The two extremes of such calibration approaches are the so-called photogrammetric calibration and pure autocalibration,whereas a large number of other methods exist in between them.Photogrammetric calibration achieves accurate camera calibration by the aid of a 3D calibration object whose geometry information is precisely known.This approach is accurate and reliable.However,it requires elaborate and expense setup of calibration apparatus.Pure autocalibration requires no knowledge of the observed scene(except for its rigidity)or camera motion1.It recovers the camera parameters from only the generally distributed correspondences in the given image sequences. This approach has the highestflexibility but suffers from inaccuracy and unreliability,accompanied by the outstanding degeneracy problem that many common types of motions lead to ambiguities in the solution.Besides these two approaches,many other algorithms falling in between them have also been designed.Some of these algorithms achieve calibration from partial scene geometry information,e.g.a planar surface(with known or unknown metric information)undergoing unknown motion,parallelism in 1Autocalibration is referred by some authors to the calibration without using any calibration object,which includes most approaches in this paper except§4,§6.1,§6.4.We emphasize here the distinction by using the term pure autocalibration. However,in the rest of this paper,the term autocalibration is equal to pure autocalibration.the scene,known angles,equal but unknown angles,known ratios of lengths,or multiple collinear points with known relative position.Some other algorithms calibrate the parameters by utilizing the controlled motions of the camera,e.g.pure rotation,planar motion,pure translation,or translation with known direction.In this paper,we confine the discussion to the calibration of perspective cameras.We will never consider the nonlinear camera models for e.g.wide-angle[3]or catadioptric cameras[4,5],and neither certain degenerate linear models,e.g.the affine camera[6,7]or 1D camera models[8,9].We also exclude a few other specific models,e.g.the two-plane camera model for direct reconstruction[10,11].The calibration framework using multi-view rank conditions[12,13]is not discussed.A few recent work that use statistics in geometry calibration are excluded[14,15].Various feature extraction methods (prior to camera calibration)are also never considered.2Basics and notationsIn this section we briefly introduce a handful of concepts that underpin the basic theory of camera calibration.Such concepts include camera projection matrix,intrinsic parameters,extrinsic parameters,absolute conic and quadric,planar homography,fundamental matrix,essential matrix,Kruppa equations,etc.Due to the space limitation,such introduction is compressed as much as possible.Readers are referred to the citations therein or [1,2]for further discussions.2.1Camera modelsFigure 1:The perspective camera modelConsider the widely used perspective camera model,as shown in Fig.1.Let the coordinates of a 3D point in the Euclidean world coordinate system be M =[x,y,z ]T and its projection in the retinal image coordinates be m =[u,v ]T .Their respective homogeneous coordinates ˜M=[x,y,z,1]T and ˜m =[u,v,1]T are related by a linear equation,˜m P ˜M (1)where the symbol means “equal up to a scale factor”,and P is a 3×4matrix called the camera projection matrix .P is defined up to scale and has 11degrees of freedom.It can be decomposed as:P A 100001000010R t 01 =A R t = AR At (2)where R is a 3×3orthogonal matrix,t is a 3D vector,and A is a 3×3upper triangular matrix.The decomposition is unique according to the QR factorization theory[16].The matrix R t 01describes the 3D displacement (rotation and translation)from the world coor-dinate system to the camera coordinate system.Rotation R and translation t are the camera’s extrinsicparameters .Matrix A is used to convert from the normalized image coordinates to the retinal image coordinates.It is called camera calibration matrix and encodes the camera’s all intrinsic parameters ,A = f λu 00τf v 0001(3)where f is the camera’s focal length ,τis the aspect ratio ,λskew factor ,and u 0,v 0principal point position.The skew factor of a usual camera is very small and is often set to be zero.Sometimes the aspect ratio is also simplified to be unit,and even the principal point to be the image center.However,contrary to the long-held general belief,a recent paper[17]points out that the calibration accuracy is rather sensitive to the accuracy of principal point position,at least in some configurations.Note that while the skew factor and aspect ratio are somehow constant for a camera system,the principal point often varies with zooming or focusing of a camera[18,19].The above rectilinear camera model has never accounted for the nonlinear lens distortions introduced by imperfections and misalignments in the optical system.Such distortions can be calibrated and corrected separately before calibrating the perspective model[20],or compensated as a posteriori using iterative nonlinear minimization techniques[21],if they are not weak enough to be neglected.A number of sophisticated models exist to account for different distortion factors,e.g.,radial distortion,de-centering distortion,and thin-prism distortion.Tsai[20]ever noted that any more elaborate distortion model than a radial one not only would not help increase accuracy but also would cause numerical instability.Yet some others[21]report,especially when wide angle cameras are used,a non radial distortion component in the distortion model improve accuracy.A generally used radial distortion model is a polynomial model, u =u +(u −u 0)(k 1r 2+k 2r 4+...)v =v +(v −v 0)(k 1r 2+k 2r 4+...)with r 2=(u −u 0)2+(v −v 0)2where u ,v denote the observed (distorted)image coordinates and x,y the undistorted image coordi-nates,u 0,v 0are the principal center position,r is the distance between (u,v )and (u 0,v 0),and k i are the distortion coefficients.It is usually sufficient to truncate the polynomials to the first two terms.Further discussion on nonlinear distortion are beyond the scope of this paper.2.2Absolute conic &quadricThe absolute conic (AC)is a degenerate conic lying on the plane at infinity.In Euclidean frame itis represented by a 4×4rank-3matrix Ωe = I 00T 0.A point ˜M is on the AC if and only if ˜MT Ω˜M =0.It can be seen that the AC does not contain any real point:it is composed entirely of complex points.The dual of AC,called absolute quadric [22],consists of planes tangent to the AC.It is a degenerate quadric whose unique null space is the planeat infinity.In Euclidean frame this quadric is represented by a 4×4rank-3matrix Ω∗e = I 00T 0.A plane Πis on the quadric if and only if ΠT ΩΠ=0.Consider an arbitrary nonsingular 4×4transformation T .It transforms a point ˜Mto T ˜M ,and transforms a plane Πto ΠT T −1.Thus it transforms the AC Ωe to T −T Ωe T −1,and transforms theabsolute quadric Ω∗e to T Ω∗e T T .It follows that Ωand Ω∗are invariant under Euclidean transformation,are scaled under similarities,take the form Q 0030(symmetric 3×3nonsingular Q )under affine ones,and become arbitrary symmetric 4×4rank-3matrices under projective ones.Let us consider the projection of the AC in the image plane (IAC).Given a point ˜M=[x,y,z,0]T on the AC,it has M T M =0,where M =[x,y,z ]T .Define a camera projection matrix as P =A [R t ].Since the AC is invariant to Euclidean transformation,the projection ˜m of ˜M satisfies ˜m P ˜M=AM .It follows that ˜m T A −T A −1˜m =0.So a point ˜m is on the IAC if and only if it lies on the conic representedby the matrix B A −T A −1.The projection of the absolute quadric in the image plane is the dualof IAC (DIAC),which consists of all lines tangent to the IAC.The DIAC is defined by the matrix K =B −1 AA T .A line l is tangent to the IAC if and only if l T Kl =0.Both B and K are symmetricand positive matrices,and defined up to a scale,hence they have only5independent unknowns.They uniquely determine and are uniquely determined(up to a scale)by the intrinsic parameters,withB A−T A−1=1τ2f2τ2γτ−fγτv0f−τ2u0γτ−fγ2+f2f2γ(γv0−τfu0)−f2−v0γτv0f−τ2u0γ(γv0−τfu0)f2−v0(γv0−τfu0)2f2+v20+τ2f2(4)andK AA T=γ2+u20+f2γτf+u0v0u0γτf+u0v0τ2f2+v20v0u0v01(5)Since K,B are symmetric and positive,the intrinsic parameters can be easily obtained from it by means of Cholesky factorization[16],or in closed form:A=K11−K213−121323K22−K223K12−K13K23√K22−K23K13K22−K223K23001(6)where K ij is the element of K at row i and column j,and K33=1.Or,A=λB11−λB212(B211B22−B11B212)B12(B11B23−B12B13)B211B22−B11B212−B13B1111B11B22−B212B12B13−B11B23B11B22−B212001(7)whereλ=B33−B213B11−(B12B13−B11B23)2B211B22−B11B212.Many calibration algorithmsfirst estimate K or B and thensolve the intrinsic parameters from them.2.3Planar HomographyIf we define the world coordinate system so that thefirst two axes define a space planeΠ,the projection of points ofΠcan be viewed as,u v1 TPx y01T=p1p2p4x y1Twhere p i denotes the i th column of the camera projection matrix P.If we use M=[x,y]T to denote a point ofΠand˜M=[x,y,1]T be its homogeneous coordinates,the above equation is simplified as,˜m H˜M with Hp1p2p4Ar1r2t(8)where r i denotes the i th column of the rotation matrix R,H is the planar homography from planeΠto the image plane.H is non-singular as long asΠdoes not pass through the camera’s optical center.The derivation of H under arbitrary world coordinate system can be found in[2].Because in(8)H has8degrees of freedom and there are6extrinsic parameters,an H applies2 constraints on the intrinsic ing the knowledge that r1and r2are orthonormal,we have,h T1Bh2=0,h T1Bh1=h T2Bh2with B A−T A−1(9) These constraints can also be derived from a geometric standpoint.It can be verified that a space plane z=0intersects the AC at two points:˜M∞=s[1,±i,0],where s is an arbitrary scale,i2=−1.These points are called the circular points of the plane,and their projection in the image are˜m∞=H˜M∞= h1±ih2.Since˜m∞must lie on the IAC,we get(h1±ih2)T B(h1±ih2)=0.Requiring that both real and imaginary parts be zero yields(9).Since B has5independent unknowns,3homographies arerequired to solve B(if it keeps constant).The extrinsic parameters can be computed from H if A is known,r1=λA−1h1,r2=λA−1h2,r3=r1×r2,t=λA−1h3(10) withλ=1/ A−1h1 =1/ A−1h2 .Consider a space planeΠin two-camera configuration.Let H and H be the respectively homogra-phies fromΠto thefirst and second image plane,in a common world coordinate system.Let m and m be the two respective images of point M ofΠat the two images.Thus˜m H˜M,˜m H ˜M.If H,H are non-singular,we obtain,˜m H H−1˜m=¯H˜m(11) where¯H are the homography induced byΠfrom thefirst image plane to the second.An important case occurs whenΠis a plane at infiniteΠ∞.Let H∞and H ∞be the respectively homographies fromΠ∞to thefirst and second image plane.These homographies can be expressed in a similar way as(8),but instead of setting z=0we define a world coordinate system in the Euclidean frame,so that,u v1 TPx y z0T=p1p2p3x y zTThus H∞,H ∞and¯H∞have particularly simple forms,H∞ AR,H ∞ A R ,¯H∞ A R R−1A−1(12) which yields,K ¯H∞K¯H T∞with K AA T,K A A T(13) This applies a set of constraints on K,K given¯H∞,from which only4are independent[23].This equation is important as it relates the infinity homographies with the intrinsic parameters,two entities of special use in camera calibration.2.4Epipolar geometryFigure2:Epipolar geometryThe epipolar geometry exists between any two camera system.Consider the case shown in Fig.2.Let C and C be the optical centers of thefirst and second cameras,respectively.Given a point m in thefirst image,its corresponding point in the second image in constrained to lie on a line called the epipolar line of m,denoted by l m.This line is the intersection of the second image plane I with the plane defined by m,C,and C (known as the epipolar plane).Furthermore,all epipolar lines of the points in thefirst image pass through a common point e ,which is the intersection of the line CC with the image plane I .This point is called the epipole.Above definitions are symmetric in the two cameras.The epipolar geometry constrains that the correspondence of a point in thefirst image must lie on its epipolar line in the second image.This is called the epipolar constraint.Let us express the epipolar geometry algebraically.Taking thefirst camera coordinate system as the world coordinate system,we can write the two camera projection matrices as:P A[I|0],P A [R|t], where R,t are the rotation and translation which bring points expressed in thefirst camera coordinate system to the second one.Let a space point M projecting onto both image planes to points˜m,˜m .We have˜m P˜M AM,and˜m P ˜M A RM+A bining together yields,˜m A RA−1˜m+sA t ¯H∞˜m+s˜e (14) where s is an unknown scale factor,¯H∞is the infinity homography as in(12),˜e =A t is the epipole. This equation means that˜m must lie on the epipolar line l m that goes through¯H∞˜m and˜e ,which yields,l m=F˜m or˜m T F˜m=0with F [˜e ]ׯH∞(15) where F is a3×3matrix called the fundamental matrix,[˜e ]×denotes the antisymmetric matrix defined by˜e such that[˜e ]×x=˜e ×x for any3D vector x.It is clear that F T˜e =0,thus F is of rank-2.Since F is also defined up to a scale factor,it has only7degrees of freedom.The fundamental matrix can also be expressed as,F A−T[t]×RA −1 A−T EA −1with E [t]×R(16) where E is called the essential matrix.It has5degrees of freedom and gives the extrinsic camera parameters up to a scale factor.Thus a fundamental matrix applies2independent constraints on the intrinsic parameters.If the two cameras have same intrinsic parameters,i.e.A=A ,3fundamental matrices are generally sufficient to solve A.E can be directly computed from F as long as the intrinsic parameters are known.2.5Kruppa equationsFigure3:The absolute conic and epipolar constraintConsider the two-camera configuration in Fig.3.LetΠbe a plane passing through the two optical centers C,C and tangent to the ACΩat infinite planeΠ∞.The intersection ofΠwith image planes I,I are lines l,l ,respectively.It follows that l is tangent to the IACωin I,l is tangent to the IAC ω in I ,and l,l are epipolar correspondent.The condition that the epipolar lines tangent to the IACs ω,ω correspond gives two constraints linking the epipolar geometry with the IACs.Kruppa equations are an algebraic version of these constraints.As shown in Fig.3,e,e are the two epipoles,m,m are the tangency points of l,l withω,ω , respectively.The condition that l(resp.l )is tangent toω(resp.ω )writes,l T Kl=l T K l =0, where K(resp.K )is the matrix of the dual conics toω(resp.ω ).Since l can be expressed asl =[˜e ]טm ,and from (15)we have l =F T ˜m ,thus ([˜e ]טm )T K ([˜e ]טm )=(F T ˜m )T K (F T ˜m )=0,which yields,[˜e ]T ×K [˜e ]× F KF T (17)From this equation we can obtain 2independent functions,called the Kruppa equations ,of degree two on the elements of K,K ,and hence of degree four on the elements of A,A .In the case that the two cameras have identical intrinsic parameters,i.e.K =K ,3fundamental matrices are generally sufficient to obtain a solution.The Kruppa equations were rediscovered and derived by Maybank and Faugeras[24,25].Since then a few different derivations have been proposed[26,27,28,29].Among them the simplest ones are based on the singular value decomposition (SVD)of the fundamental matrix[27,29].Let F be written asF =UDV T (with SVD),and U =[u T 1u T 2u T 3]T ,V =[v T 1v T 2v T 3]T ,and D =diag (r,s,0),then theKruppa equations are r 2v T 1Kv 1u T 2K u 2=rsv T 1Kv 2−u T 2K u 1=s 2v T 2Kv 2u T 1K u 1(18)From (18)one obtains two independent quadratic equations on the five coefficients of K .2.6Stratified reconstructionFull calibration of cameras’intrinsic and extrinsic parameters is often impossible.However,in some practical situations,partial calibration which yields 3D reconstruction up to certain unknown transfor-mation is often sufficient.We discuss here three levels of such partial reconstructions that are of special importance[23,2].They are,3D reconstruction up to an unknown projective transformation (called projective reconstruction ),up to an unknown affine transformation (called affine reconstruction ),and up to an unknown similarity transformation (called misleadingly Euclidean reconstruction ).Given the images taken by two cameras,the fundamental matrix F between the two cameras can be solved from sufficient general point correspondences.It can be verified that F leads directly to the projective reconstruction, P I 0 H PS s ˜e H with H = P ΠT (19)where ˜e is the epipole in the second image (given by F T ˜e =0),S =[˜e ]×F is called the special matrix ,s is an arbitrary scale,Πis an arbitrary 4D vector defined up to scale (with 3unknowns).s ,Πand P together have 15unknowns representing the projective ambiguity in reconstruction.An affine transformation is a particular projective transformation which preserves the plane at infinityΠ∞,whose coordinates in affine space is Πa ∞ [0,0,0,1]T .Given the projective reconstruction,if wecan identify Π∞expressed in the given projective frame Πp ∞,the problem of recovering affine structureis equivalent to finding an arbitrary 4×4projective matrix T a p which maps Πp ∞onto Πa ∞:Πp ∞T a −1p Πa ∞.Since Πp ∞is defined up to scale,we express it as Πp ∞ [r T ∞,1]T .It follows that a minimalparameterization of T a p is:T a p I 0r T ∞1.Applying it into (19)we obtain the affine reconstruction, P I 0 A P¯H ∞se A with A = P 0T 1/s (20)where ¯H ∞is the planar homography induced by Π∞,given by ¯H ∞ S +˜e r T ∞.A is of course an affinetransformation matrix determined by 12unknown parameters.We are then seeking for reducing the ambiguity from an affine transformation to a similarity one.A similarity transformation is a particular affine transformation which keeps the absolute conic (up toscale).Thus the Euclidean reconstruction is solvable by finding an arbitrary 4×4affine matrix T e a mapping the absolute conic in affine space Ωa to its Euclidean form Ωe .This however makes little senseas direct identifying Ωa is often rather difficult.For clarity of presentation we instead give first the canonical form of the Euclidean stratum, P A I 0 S P A R st Swith S = R 1t 10T 1/s (21)where R 1,t 1are the rotation and translation from the world coordinate system to the first camera coordinate system,R,t are from the first camera coordinate system to the second one,S is a similaritymatrix with 7unknowns representing the reconstruction ambiguity.Therefore the upgrading matrix T e a should satisfy:[I ,0]T e a A [I ,0],and [¯H ∞,se ]T e a A [R,st ].Since we have ¯H ∞ A RA −1(12),and e A t (14),a minimal parameterization of T e a is easily obtained:T e a A 00T s 1.In summary,in a two-camera system,the projective stratum can be recovered if the fundamental matrix F is known.The affine stratum can be recovered from the projective one plus the infinity plane in the projective frame Πp ∞,or alternatively,from the infinity homography ¯H ∞plus the epipole in one camera ˜e .The Euclidean stratum can be recovered from the affine one plus the intrinsic parameters of one camera A ,or alternatively,from the intrinsics of both cameras A,A ,the in-between rotation R ,and the up-to-scale in-between translation t .The upgrading matrices are,T a p I 0r T ∞1 ,T e a A 00T s 1 ,T e p A 0r T ∞A s 1 (22)where [r ∞,1]T in the coordinates of the infinity plane in the projective stratum.3Fundamental matrix estimationThe problem being considered in this section is the estimation of the fundamental matrix F from a sufficiently large set of point correspondences.This problem is also known as weak calibration and many linear or nonlinear algorithms have been proposed,c.f.[30,31,32,33].It is of importance since given the fundamental matrix we can obtain a projective reconstruction of the scene[34,35],which is sufficient in some applications,c.f.[36,37],and is the basic of many further calibration techniques,c.f.[38].Readers are referred to [39]for a detailed review on this problem.Let a point m =[u,v ]T in the first image to be matched to a point m =[u ,v ]T in the second image.They must satisfy the the epipolar equation (15),which can be rewritten as a linear and homogeneous equation in the 9unknown coefficients of F :u T f =0,where u =[uu ,vu ,u ,uv ,vv ,v ,u,v,1]T ,f =[F 11,F 12,F 13,F 21,F 22,F 23,F 31,F 32,F 33]T ,F ij is the element of F at row i and column j .Thus given n point matches we have the following linear system to solve:Uf =0with U =[u 1,...,u n ]T (23)subject to det (F )=0,due to the rank-2constraint of F ,and e.g. f =1,as F is defined up to a scale.Since F has only 7degrees of freedom,7point matches are required to obtain a solution.However,in this case we may obtain at most 3solutions in that the rank-2constraint generates a cubic polynomial on the element of f .Thus 8point matches are demanded to obtain a unique solution,and in practice much more (say,20or more)should be used for combating noise.If firstly ignore the rank-2constraint,we get an analytic solution of f by solvingmin f Uf 2subject to f =1(24)The solution is the unit eigenvector of matrix U T U associated to the smallest eigenvalue.Then we can impose the rank-2constraint a posteriori,by replacing the solved F by the matrix ˆF which minimizes the Frobenius norm of F −ˆF subject to the constraint det (ˆF )=puting ˆF is quite efficient by using SVD F =USV T :ˆF=U ˆSV T ,where ˆS is obtained by replacing the least singular value in S to be zero.Faugeras[38]also proposed a linear algorithm that imposes the rank-2constraint during the minimization but still yields an analytic solution.The produced results are comparable to that of the above two-step method.Note that such methods that algebraically rearrange and so that analytically solve a linear system are called Direct Linear Transform methods (DLT),which will be used very often in the following sections.These methods are simple and efficient,however,they can not obtain a maximum likelihood estimation because the rearranged linear equations (23)are not corrupted by zero-mean independently distributed noises with identical variance,even under the assumption of independently identically distributed (i.i.d.)noises on point measures.Thus the results of DLT are biased and inaccurate for noisy data.Moreover,such algorithms are often numerical instable due to the intensive numerical manipulations.Hartley[40]has pointed out that normalizing the input data (to improve the numerical conditioning of matrix U T U )prior to minimization will significantly improve the algorithms’stability.The basic idea of such normalization is to transform the points in each image so that their centroid is at the origin,and the two principal moments at axis u,v areboth unit.Let ˜m i be the i th of n points in an image,by Cholesky factorization we get i ˜m i ˜m T i =nLL T ,where L is a 3×3triangular matrix.Thus we have i L−1˜m i ˜m T i L −T =nI .It follows that L −1˜m i is the desired normalization transformation.While the above linear approaches give acceptable results if normalization is performed,practice has shown that more precise results with noisy data are obtained by certain nonlinear algorithms that minimize physically meaningful object functions,e.g.sum of distances between points and their corre-sponding epipolar lines,min F i(d 2(˜m i ,F ˜m i )+d 2(˜m i ,F T ˜m i ))(25)where d (˜m ,F ˜m )denotes the distance from point ˜m to line F ˜m .Standard nonlinear optimization techniques,such as Levenberg-Marquardt method[41],can be used to find the solution.Such nonlinear procedures demand an initial estimate of F ,which can be obtained from the above DLT methods.The above nonlinear least-squares methods obtain accurate results in case of i.i.d.noises on point measures.In practice,however,the given point matches often contain some outliers due to bad point locations or false matches.To tolerate such outliers robust estimate methods can be used [42,43].Among the most popular robust methods are the M-estimator,the least-median-of-squares (LMedS)method,and the random sample consensus (RANSAC)method.The M-estimator tries to reduce the impacts of outliers by replacing the squared residuals used in least-squares method by another function of the residuals,yielding,min i ρ(r i ),where ρis a robust function chosen to be less increasing than square.The LMedS method estimates the parameters by solving the nonlinear minimization problem:min median i (r 2i ).That is,the estimator yields the smallest value for the median,instead of the mean asin least-squares estimator,of squared residuals computed for the entire data set.This method is rather robust to outliers.However,the minimization is difficult and must be solved by a (random)search in the full solution space.RANSAC[44]tries to find,through random sampling of a minimal subset of the data,the parameter set which is consistent with a largest subset of data.It is very similar to LMedS except that RANSAC needs a threshold for consistent checking.It can deal with the case where the percentage of outliers is higher than 50%,while LMedS can not.If all the observed points are in some degenerate configurations,the fundamental matrices estimation may not have a unique solution,even with an arbitrarily large number of point correspondences.It has been shown [45]that this happens when all space points lie on a space quadric surface passing through the two optical centers,called critical surface .In this case the maximum number of ambiguous solutions is 3.A more special case is that all points lie on a single space plane,where infinite number of solutions will be produced.4Photogrammetric calibrationPhotogrammetric calibration are quite mature[20,46,47].It makes use of a calibration object whose geometry in 3D space is precisely known.The obtained results are usually accurate,relying on the precision of the calibration object and extracted features.Such method however requires expensive and elaborate laboratory setups.There are at least three types of calibration objects:(i)a plane pattern undergoing a precisely known motion (usually translation perpendicular to the plane).Such precise motion requires some special devices.(ii)an object consisting of two or three planes exactly orthogonal to each other.This is suitable for close-range applications that requires a small calibration object,whereas a large one for long range conditions is difficult to manufacture.(iii)a 3D test field with precisely measured markers.The measurement is done with for example theodolites,thus achieving high accuracy though time consuming.This is the best method regarding calibration accuracy for both close and long range applications.。
- 1、下载文档前请自行甄别文档内容的完整性,平台不提供额外的编辑、内容补充、找答案等附加服务。
- 2、"仅部分预览"的文档,不可在线预览部分如存在完整性等问题,可反馈申请退款(可完整预览的文档不适用该条件!)。
- 3、如文档侵犯您的权益,请联系客服反馈,我们会尽快为您处理(人工客服工作时间:9:00-18:30)。
AReal-TimeCatadioptricStereoSystemUsingPlanarMirrors∗JoshuaGluckmanandShreeK.NayarDepartmentofComputerScienceColumbiaUniversityNewYork,NY10027
AbstractByusingmirrorreflectionsofascene,stereoimagescanbecapturedwithasinglecamera.Singlecamerastereoprovidesbothgeometricandradiometricadvan-tagesovertraditionaltwocamerastereo.Inthispaper,wediscusstheseadvantagesandshowthattheepipolargeometryisrestrictedtotheclassofplanarmotions.Inadditionwehaveimplementedareal-timesystemwhichdemonstratestheviabilityofstereowithmirrorsasanalternativetotraditionaltwocamerastereo.
1IntroductionOpticalsystemsconsistingofacombinationofrefracting(lens)andreflecting(mirror)elementsarecalledcata-dioptricsystems[HechtandZajac,1974].Byusingtwoormoremirroredsurfaces,multipleviewsofascenecanbecapturedbyasinglecamera(catadioptricstereo).Sin-glecamerastereoprovidesseveraladvantagesovertradi-tionaltwocamerastereo.
•IdenticalSystemParameters:Lens,CCDanddigitizerparameterssuchasblurring,lensdistor-tions,focallength,spectralresponses,gain,offset,pixelsize,etc.areidenticalforthestereopair(as-sumingidealmirrors).Havingidenticalsystempa-rametersminimizesthedifferencesbetweenthetwoviews,thusfacilitatingrobuststereomatching.
•EaseofCalibration:Becauseonlyasinglecameraanddigitizerisused,thereisonlyonesetofintrinsiccalibrationparameters.Aswewillshow,theextrin-siccalibrationparametersareconstrainedbyplanarmotion.Togethertheseconstraintsreducethetotalnumberofcalibrationparametersfrom16to10.
•DataAcquisition:Camerasynchronizationisnotanissuebecauseonlyasinglecameraisused.Stereodatacaneasilybeacquiredandconvenientlystoredwithastandardvideorecorderwithouttheneedtosynchronizemultiplecameras.Mvv′M′fcP
mm′
pp′
Figure1:Stereoimageformationwithasinglecameraandtwoplanarmirrors.AscenepointPreflectedoffmirrorsMandMisimagedasifseenfromtwodiffer-entviewpointsvandv.
andGruver,1993],[Inabaetal.,1993]and[MathieuandDevernay,1993].Here,weanalyzethegeometryoftwomirrorsandasinglecamerawiththemirrorsplacedinanarbitraryconfiguration.
Figure1depictsthegeometryofacatadioptricsystemwithtwoplanarmirrors.AscenepointPisimagedasifseenfromtwodifferentviewpointsvandv.Theloca-tionofthetwovirtualpinholesisfoundbyreflectingthecamerapinholeabouteachmirror.Reflectingtheopticalaxisofthecameraaboutthemirrorsdeterminestheopti-calaxesandthustheorientationsofthetwovirtualcam-eras.Thevirtualimageplanesexistatadistancef,thefocallengthofthecamera,alongtheopticalaxesofthetwovirtualcameras.Therefore,thelocationsandorien-tationsofthetwovirtualcamerasaredeterminedbytheorientationsanddistancesofthetwomirrorswithrespecttothepinholeandopticalaxisofthecamera.
2.1RelativeOrientationIntraditionalstereowithtwocamerastherearenoquan-titativerestrictionsontherelativeorientationbetweenthetwocameras.However,constraintsdoexistforthetwovirtualcamerascreatedwhentwoplanarmirrorsareim-agedbyasinglecamera.Itturnsoutthattherelativeorientationisrestrictedtoplanarmotion(thedirectionoftranslationmustlieintheplanenormaltotheaxisofro-tation).Thisconstraintreducesthenumberofdegreesoffreedomofrelativeorientationfrom6to5(3forrotationand2fortranslationinaplane).Toderivethisresultweconsidertherelativeorientationbetweenthetworeflectedviewpointsvandv.Eachvirtualviewpointisrelatedtothecameracenterbythefollowingequations:v=D1c(1)andv=D2c,(2)whereD1andD2arereflectiontransformations.ThentherelativeorientationDbecomes,D=D2D−11.(3)Representingthetwomirrorsasplaneswithnormalsn1andn2anddistancesd1andd2measuredfromcthecameracenter,thereflectiontransformationsforthetwomirrorsaregivenbyD1=I−2n1nT12d1n101(4)andD2=I−2n2nT22d2n201.(5)Becausetheinverseofareflectiontransformationisit-self,therelativeorientationofthetwovirtualcamerasissimply,D=D2D1=Rt01(6)whereR=I+4(n1·n2)n1nT2−2n1nT1−2n2nT2,(7)andt=2d1n1−(2d1(n1·n2)+2d2)n2.(8)TherotationmatrixRhasarotationalaxisofn1×n2andfrom(8)thedirectionoftranslationliesintheplanedefinedbyn1andn2.Therefore,therotationalaxisisnormaltotheplanecontainingthedirectionoftranslation(planarmotion).Planarmotionhasbeenstudiedinthecontextofmobilerobotics[BeardsleyandZisserman,1995],wheremotionoveragroundplaneismodeledbyplanarmotion.ForsuchscenariosVievillandLingrand[1995]andArm-strongetal.[1996]haveusedplanarmotiontohelpcon-straintheself-calibrationproblem.
Aswehaveseen,singlecamerastereowithtwoplanarmirrorsconstrainstheexternalcalibrationparameterstoplanarmotion.Becauseonlyasinglecameraisused,theintrinsicparameters(focallength,pixelsize,imagecenter,skew)areexactlythesameforthetwostereoviews.Together,theseconstraintsplacerestrictionsontheepipolargeometry.