Neural basis of learning and preference during social decision making
人工智能基础教材

人工智能基础教材以下是一些人工智能基础教材的推荐:1. 《人工智能:一种现代方法》(Artificial Intelligence: A Modern Approach)- 由Stuart Russell和Peter Norvig合著,是人工智能领域的经典教材之一,涵盖了人工智能的基本概念、算法和应用。
2. 《人工智能:方法、流程与应用》(Artificial Intelligence: Methods and Applications)- 由Farhad Hussain等人合著,介绍了人工智能的基本方法和应用领域,包括机器学习、自然语言处理、计算机视觉等。
3. 《统计学习方法》(Statistical Learning Methods)- 由李航编著,介绍了统计学习的基本概念、方法和算法,包括感知机、支持向量机、决策树、神经网络等,对机器学习算法有很好的讲解。
4. 《深度学习》(Deep Learning)- 由Ian Goodfellow、Yoshua Bengio和Aaron Courville合著,深入介绍了深度学习的基本原理、算法和应用,是学习深度学习的经典教材之一。
5. 《机器学习》(Machine Learning: A Probabilistic Perspective)- 由Kevin Murphy编著,介绍了机器学习的基本概念、方法和理论,包括概率图模型、无监督学习、强化学习等内容。
6. 《自然语言处理综论》(Speech and Language Processing)-由Daniel Jurafsky和James H. Martin合著,系统介绍了自然语言处理的基本概念、方法和应用,涵盖了文本处理、语音识别、机器翻译等领域。
这些教材涵盖了人工智能的基本知识和方法,适合初学者和进阶学习者。
读者可以根据自己的兴趣和需求选择合适的教材进行学习。
把传统的贝叶斯因果网络和知识图谱,与深度强化学习融合
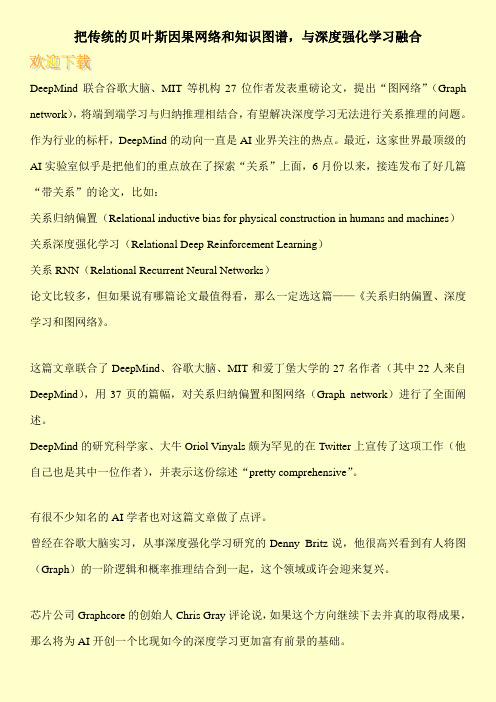
把传统的贝叶斯因果网络和知识图谱,与深度强化学习融合DeepMind联合谷歌大脑、MIT等机构27位作者发表重磅论文,提出“图网络”(Graph network),将端到端学习与归纳推理相结合,有望解决深度学习无法进行关系推理的问题。
作为行业的标杆,DeepMind的动向一直是AI业界关注的热点。
最近,这家世界最顶级的AI实验室似乎是把他们的重点放在了探索“关系”上面,6月份以来,接连发布了好几篇“带关系”的论文,比如:关系归纳偏置(Relational inductive bias for physical construction in humans and machines)关系深度强化学习(Relational Deep Reinforcement Learning)关系RNN(Relational Recurrent Neural Networks)论文比较多,但如果说有哪篇论文最值得看,那么一定选这篇——《关系归纳偏置、深度学习和图网络》。
这篇文章联合了DeepMind、谷歌大脑、MIT和爱丁堡大学的27名作者(其中22人来自DeepMind),用37页的篇幅,对关系归纳偏置和图网络(Graph network)进行了全面阐述。
DeepMind的研究科学家、大牛Oriol Vinyals颇为罕见的在Twitter上宣传了这项工作(他自己也是其中一位作者),并表示这份综述“pretty comprehensive”。
有很不少知名的AI学者也对这篇文章做了点评。
曾经在谷歌大脑实习,从事深度强化学习研究的Denny Britz说,他很高兴看到有人将图(Graph)的一阶逻辑和概率推理结合到一起,这个领域或许会迎来复兴。
芯片公司Graphcore的创始人Chris Gray评论说,如果这个方向继续下去并真的取得成果,那么将为AI开创一个比现如今的深度学习更加富有前景的基础。
ActivityTheory活动理论
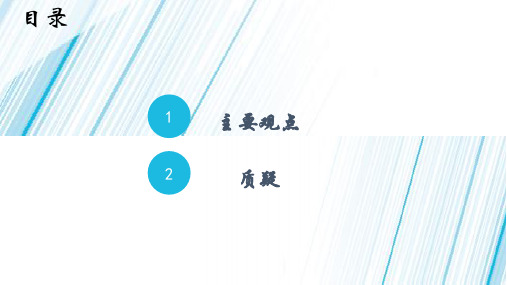
多重声音思想
多重声音思想认为各种不同观点的话语都要被融合接纳与利用, 而在传统的课堂中,一切学习活动目标与过程都已经被预先设定, 难以倾听学习者的多重声音,难以考虑学习者的不同差异,无法包 含多种持有不同理解、不同观点的主体与共同体,也就无法相互争 论、协商与融合。活动理论关注的不是知识传递,而是人们参与的 学习活动的过程,所以应该充分给予学生表达自己观点的机会,并 且构建课程学习活动共同体,建立教师之间、师生之间、学生之间 互助的学习共同体,通过沟通学习的模式,分享经验、表达观点, 促进多元声音融合。
理解:在拓展性学习中,学习者所学习的知识时并不是固定的, 先前就已经存在的,而是学习者通过集体活动,一边创造知识一 边学习知识,随后再将习得的知识运用于实践。这与一般意义上 的学习是有较大差别的。一般我们所说的学习的知识是比较固定 的,并且已经被他人证明过是正确且合理的。而拓展性学习主要 是探索“未知领域”,根据自己的实践总结出知识并学习。比如 说,陶行知先生所提出的“生活教育”就提倡拓展性学习。
举例:
在课堂中,老师正在讲解新课,突然有两个学生发生了争执。在 这种情况下,学生的争执就是进入课堂教学这个活动系统的新因素, 打破了原先正常教学的“平衡”,产生了矛盾。这时,活动系统就 因为这个矛盾而发生变化,从先前的讲课活动转化为处理学生争执 的活动。
媒介工具
从活动理论看,工具是学 习者和学习内容的中介,是学 习内容的制品,工具改变学习 活动中知识传递、呈现、处理 等的方式。工具是支持活动开 展的条件,在活动中使用媒介 工具,不仅使学习者获取知识, 也能培养他们的思维方式和价 值观念。如电化教学,慕课等 的推广,不仅帮助学生获取记 忆知识,而且也能使他们意识 到科技在生活中的作用。
拓展性学习
Ellis-Corrective-Feedback

T tries to elicit correct pronunciation and the corrects
S: alib[ai]
S fails again
T: okay, listen, listen, alb[ay] T models correct pronunciation
SS: alib(ay)
Theoretical perspectives
1. The Interaction Hypothesis (Long 1996) 2. The Output Hypothesis (Swain 1985;
1995) 3. The Noticing Hypothesis (Schmidt
1994; 2001) 4. Focus on form (Long 1991)
2. In the course of this, they produce errors. 3. They receive feedback that they recognize as
corrective. 4. The feedback causes them to notice the errors they
first row. (uptake)
The complexity of corrective feedback
Corrective feedback (CF) occurs frequently in instructional settings (but much less frequently in naturalistic settings)
Commentary
Initial focus on meaning Student perceives the feedback as corrective
人工智能:了解机器学习和深度学习的基础知识
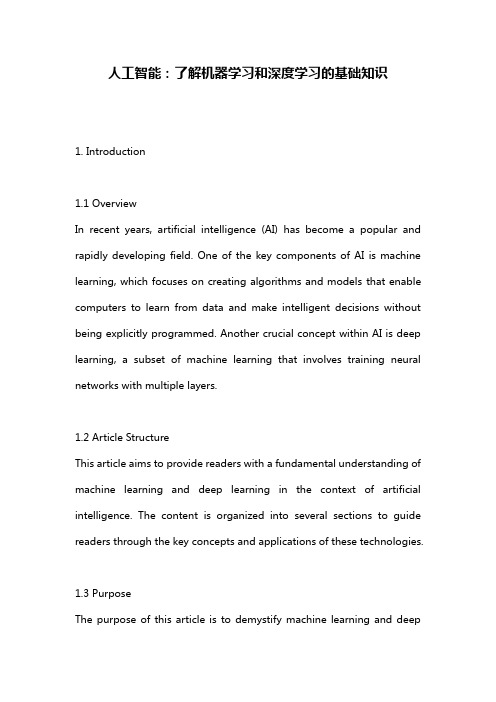
人工智能:了解机器学习和深度学习的基础知识1. Introduction1.1 OverviewIn recent years, artificial intelligence (AI) has become a popular and rapidly developing field. One of the key components of AI is machine learning, which focuses on creating algorithms and models that enable computers to learn from data and make intelligent decisions without being explicitly programmed. Another crucial concept within AI is deep learning, a subset of machine learning that involves training neural networks with multiple layers.1.2 Article StructureThis article aims to provide readers with a fundamental understanding of machine learning and deep learning in the context of artificial intelligence. The content is organized into several sections to guide readers through the key concepts and applications of these technologies.1.3 PurposeThe purpose of this article is to demystify machine learning and deeplearning by explaining their basic principles, highlighting their differences, discussing their main algorithms and frameworks, as well as examining the history, current status, trends, and challenges in the field of artificial intelligence. By reading this article, readers will gain a solid foundation in these subjects and be better prepared to explore further advancements in AI.(Note: The text above does not contain any URLs or website addresses.)2. 机器学习基础知识2.1 什么是机器学习机器学习(Machine Learning)是人工智能领域的一个重要分支,旨在通过计算机模拟人类学习过程,从数据中发现规律和模式,并利用这些规律和模式进行预测和决策。
人工智能技术的知识点整理

人工智能技术的知识点整理人工智能(Artificial Intelligence,简称AI)是近年来发展迅猛的一门技术领域,它致力于使计算机系统具备类似人类智能的功能和能力。
在AI技术的发展过程中,各种知识点相互交织,形成了庞大而复杂的知识网络。
本文将对人工智能技术的知识点进行整理和梳理,以便更好地理解和掌握这一领域。
一、机器学习(Machine Learning)机器学习是人工智能领域的重要基石,它关注计算机系统如何通过经验学习来改善性能。
在机器学习中,主要有以下几个重要知识点:1. 监督学习(Supervised Learning):通过给定输入和对应的输出样本训练模型,从而使其能够预测未知输入的输出。
2. 无监督学习(Unsupervised Learning):通过从输入样本中发现模式和结构,从而提取隐藏的信息和知识。
3. 强化学习(Reinforcement Learning):通过与环境交互,通过奖励和惩罚的机制来学习最优决策策略。
4. 深度学习(Deep Learning):通过模仿人脑神经网络的结构和工作方式,实现复杂的模式识别和决策。
二、自然语言处理(Natural Language Processing)自然语言处理是AI技术中与人类语言相关的领域,主要研究计算机如何理解和处理人类的自然语言。
以下是自然语言处理的几个重点知识点:1. 词法分析(Lexical Analysis):将自然语言的连续字符序列切分成有意义的词汇单位,例如分词、词性标注等。
2. 句法分析(Syntactic Analysis):研究语言中词汇之间的关系,例如依存关系、语法结构等。
3. 语义分析(Semantic Analysis):理解自然语言句子的意义,例如命名实体识别、意图识别等。
4. 机器翻译(Machine Translation):将一种自然语言转化成另一种自然语言的技术。
三、计算机视觉(Computer Vision)计算机视觉是研究如何使计算机通过摄像头或相似的设备感知和理解图像或视频的过程。
人工智能的核心知识点
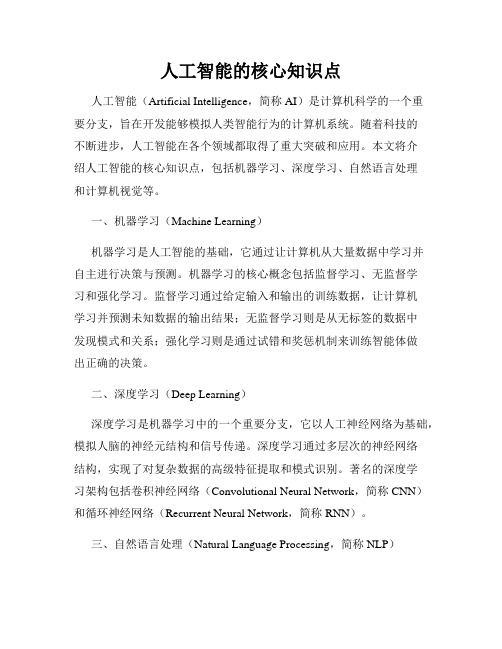
人工智能的核心知识点人工智能(Artificial Intelligence,简称AI)是计算机科学的一个重要分支,旨在开发能够模拟人类智能行为的计算机系统。
随着科技的不断进步,人工智能在各个领域都取得了重大突破和应用。
本文将介绍人工智能的核心知识点,包括机器学习、深度学习、自然语言处理和计算机视觉等。
一、机器学习(Machine Learning)机器学习是人工智能的基础,它通过让计算机从大量数据中学习并自主进行决策与预测。
机器学习的核心概念包括监督学习、无监督学习和强化学习。
监督学习通过给定输入和输出的训练数据,让计算机学习并预测未知数据的输出结果;无监督学习则是从无标签的数据中发现模式和关系;强化学习则是通过试错和奖惩机制来训练智能体做出正确的决策。
二、深度学习(Deep Learning)深度学习是机器学习中的一个重要分支,它以人工神经网络为基础,模拟人脑的神经元结构和信号传递。
深度学习通过多层次的神经网络结构,实现了对复杂数据的高级特征提取和模式识别。
著名的深度学习架构包括卷积神经网络(Convolutional Neural Network,简称CNN)和循环神经网络(Recurrent Neural Network,简称RNN)。
三、自然语言处理(Natural Language Processing,简称NLP)自然语言处理是指让计算机理解、处理和生成人类自然语言的技术。
NLP的关键任务包括语言识别、语义分析和机器翻译等。
语言识别通过识别和转录语音为文本;语义分析则是通过分析文本的结构和语义,理解其含义和情感;机器翻译则是将一种语言的文本自动翻译成另一种语言。
四、计算机视觉(Computer Vision)计算机视觉是指让计算机能够理解和解释图像和视频的技术。
计算机视觉的应用非常广泛,包括图像分类、目标检测和人脸识别等。
图像分类是将图像自动分类到不同的类别;目标检测则是在图像中定位并识别特定对象;人脸识别则是通过对人脸图像进行特征提取和匹配,实现人的身份认证等功能。
人工智能原理习题集及答案 北京大学 王文敏

Quizzes for Chapter 11单选(1分)图灵测试旨在给予哪一种令人满意的操作定义得分/总分• A.人类思考•B.人工智能•C.机器智能1.00/1.00•D.机器动作正确答案:C 你选对了2多选(1分)选择以下关于人工智能概念的正确表述得分/总分• A.人工智能旨在创造智能机器该题无法得分/1.00 •B.人工智能是研究和构建在给定环境下表现良好的智能体程序该题无法得分/1.00•C.人工智能将其定义为人类智能体的研究该题无法得分/1.00• D.人工智能是为了开发一类计算机使之能够完成通常由人类所能做的事该题无法得分/1.00 正确答案:A 、B 、D 你错选为A 、B 、C 、D3多选(1分)如下学科哪些是人工智能的基础?得分/总分• A.经济学0.25/1.00 • B.哲学0.25/1.00 •C.心理学0.25/1.00 •D.数学0.25/1.00正确答案:A 、B 、C、D 你选对了4多选(1分)下列陈述中哪些是描述强AI (通用AI )的正确答案?得分/总分• A.指的是一种机器,具有将智能应用于任何问题的能力0.50/1.00• B.是经过适当编程的具有正确输入和输出的计算机,因此有与人类同样判断力的头脑0.50/1.00•C.指的是一种机器,仅针对一个具体问题正确答案:A 、B 你选对了5多选(1分)选择下列计算机系统中属于人工智能的实例得分/总分•A.Web 搜索引擎 •B.超市条形码扫描器•C.声控电话菜单该题无法得分/1.00 •D.智能个人助理该题无法得分/1.00正确答案:A 、D 你错选为C 、D6多选(1分)选择下列哪些是人工智能的研究领域 得分/总分• A.人脸识别0.33/1.00• B.专家系统0.33/1.00 •C.图像理解•D.分布式计算正确答案:A、B 、C 你错选为A 、B7多选(1分)考察人工智能(AI)的一些应用,去发现目前下列哪些任务可以通过AI 来解决得分/总分• A.以竞技水平玩德州扑克游戏0.33/1.00• B.打一场像样的乒乓球比赛•C.在Web 上购买一周的食品杂货0.33/1.00•D.在市场上购买一周的食品杂货正确答案:A、B 、C 你错选为A 、C8填空(1分)理性指的是一个系统的属性,即在_________的环境下做正确的事。
- 1、下载文档前请自行甄别文档内容的完整性,平台不提供额外的编辑、内容补充、找答案等附加服务。
- 2、"仅部分预览"的文档,不可在线预览部分如存在完整性等问题,可反馈申请退款(可完整预览的文档不适用该条件!)。
- 3、如文档侵犯您的权益,请联系客服反馈,我们会尽快为您处理(人工客服工作时间:9:00-18:30)。
Neural basis of learning and preference during social decision-makingHyojung Seo and Daeyeol LeeSocial decision-making is arguably the most complex cognitive function performed by the human brain.This is due to two unique features of social decision-making.First,predicting the behaviors of others is extremely difficult.Second,humans often take into consideration the well-beings of others during decision-making,but this is influenced by many contextual factors.Despite such complexity,studies on the neural basis of social decision-making have made substantial progress in the last several years.They demonstrated that the core brain areas involved in reinforcement learning and valuation,such as the ventral striatum and orbitofrontal cortex,make important contribution to social decision-making.Furthermore,the contribution of brain systems implicated for theory ofmind during decision-making is being elucidated.Future studies are expected to provide additional detailsabout the nature of information channeled throughthese brain areas.AddressDepartment of Neurobiology,Yale University School of Medicine,333 Cedar Street,SHM B404,New Haven,CT06510,USA Corresponding author:Lee,Daeyeol(daeyeol.lee@)Current Opinion in Neurobiology2012,22:990–995This review comes from a themed issue on Decision making Edited by Kenji Doya and Michael N ShadlenFor a complete overview see the Issue and the EditorialAvailable online15th June20120959-4388/$–see front matter,#2012Elsevier Ltd.All rights reserved./10.1016/j.conb.2012.05.010IntroductionDecision-making can be understood as the process of selecting an option that is expected to produce the most desirable outcome.In most cases,the predictions for the outcomes from alternative actions are based on the previous experience of the decision maker.In addition, decision-making can be considered social,when its out-come depends jointly on the choices of multiple decision makers.For animals living in groups,including humans and other primates,purely individual decision-making is rare,and most decisions are made in social settings.This review focuses on recent neurobiologicalfindings that have begun to shed light on two important features of social decision-making.First,predicting the outcomes of different actions is difficult in social settings,as the actions of other decision makers change more unpredic-tably than inanimate objects in the animal’s environment. In this regard,the ability to infer about the intentions and knowledge of other animals,referred to as the theory of mind,is crucial.Second,social decision-making in humans and other primates can be influenced by other-regarding or social preferences.A central tenet in the classical game theory is that decision makers,or players, choose their actions purely on the basis of self-interest. However,such classic game theory often fails to predict actual human behaviors.Moreover,whether and how much the decision maker cares about the reward given to others is affected by a variety of neural and social factors[1–3].Model-based reinforcement learning and social decision-makingWhen humans and animals face an unfamiliar environ-ment or their environment changes unpredictably,their decision-making strategies will be adjusted accordingly. The reinforcement learning theory provides a parsimo-nious account of this process for many types of decision-making[4],including social decision-making.In this framework,the likelihood of selecting each action is determined by a set of value functions that are adjusted according to the animal’s experience.Algorithms in the reinforcement learning theory can be divided into two categories.In simple or model-free reinforcement learn-ing,changes in the value functions are driven by the actual outcomes resulting from the actions chosen by the decision maker.In model-based reinforcement learning, value functions for multiple actions can be changed simultaneously and moreflexibly according to the internal model of the decision maker about his or her environment without necessarily having to experience the outcome of each action(Figure1).Behaviors of human decision makers in a dynamic but non-social environment are best accounted for by hybrid learn-ing models that combine the features of model-free and model-based reinforcement learning models[5 ,6].In a social setting,decision makers might update their beliefs about the choices of other decision makers according to their newly observed behaviors,and utilize such infor-mation to guide their subsequent choices.This is an example of model-based reinforcement learning,and is referred to as belief learning in game theory[7].As in individual decision-making,human behaviors during iterative social interactions are also more consistent with hybrid learning models than with model-free reinforcementAvailable online at learning models or pure belief learning models[8,9 ]. Hybrid learning models also more accurately account for the choices of non-human primates during a computer-simulated rock-paper-scissors game[10,11 ].Much of our knowledge about the neural mechanisms of reinforcement learning is based on the results from experiments in which the observed behaviors of the subjects could not distinguish between these two differ-ent types of algorithms.For example,neurons modulating their activity according to the rewards expected from a particular state or action are widespread in the brain[12–16,17 ].In addition,signals related to the reward predic-tion error,namely,the difference between actual and expected rewards are also found in multiple brain areas, including the ventral tegmental area,substantia nigra pars compacta[18],striatum[19,20],anterior cingulate cortex (ACC)[21,22 ],and prefrontal cortex[23].However, whether such signals reflect the output of model-free or model-based reinforcement learning algorithm is still not well understood.Recently,several studies have begun to elucidate how signals related to the reward values and prediction errors computed by model-based reinforcement learning algor-ithms are distributed in the brain.Signals related to reward prediction errors derived from model-free and model-based reinforcement learning algorithms might be co-localized in the striatum[5 ,6].Interestingly, during strategic social decision-making,activity in the rostral ACC was related only with reward prediction errors derived from a belief learning algorithm[9 ].These results raise the possibility that model-based reward pre-diction errors might be processed differently in the brain depending on the social features of the behavioral task. Additional brain areas,such as the hippocampus and prefrontal cortex,might be involved in predicting the outcomes of choices according to a model-based reinforcement learning algorithm in a non-social context [6].During social decision-making,inferences about the likely behaviors of other decision makers become recur-sive,as a group of decision makers try tofigure out how others in the group expect each other to behave.A set of brain areas associated with theory of mind,such as the medial prefrontal cortex(mPFC)and temporoparietal junction(TPJ),might be critically involved in such recursive strategic reasoning[24–26].For example, during the beauty contest game,in which the object is to pick a number as close to2/3times the average of all the numbers chosen by the participants,subjects who dis-played high levels of strategic reasoning also showed higher activity in the mPFC[27 ].Another study found that uncertainty in the inferences about the other decision maker’s strategy during a stag-hunt game recruited the rostral medial prefrontal cortex,while activity in the DLPFC increases with the depth or level of strategic reasoning[28 ].Activity in the DLPFC was also related to the level of strategic deception during a bargaining game[29 ].Thesefindings suggest that DLPFC activity during strategic reasoning might reflect the higher demands for working memory and cognitive control. Single-neuron recording studies in non-human primates have identified signals related to specific conjunctions of actions and their outcomes during computer-simulated competitive games[12,30 ].Activity related to action–outcome conjunctions provides the information necessary for updating the value functions for specific actions according to model-free reinforcement learning algor-ithms.Neurons involved in updating the value functions for unchosen actions according to their hypothetical out-comes are co-localized in the same brain areas that process actual outcomes and the corresponding reward prediction errors,such as the ACC[31 ]and prefrontal cortex[11 ]. These areas might provide converging inputs to the brain circuits responsible for updating the value functions for different actions.In particular,during a simulated rock-paper-scissors game,neurons in the orbitofrontal cortex and lateral prefrontal cortex often encode hypothetical Neural basis of social decision-making Seo and Lee991Figure 1Reward PhysicalObjectsOtherActors(VS, vmPFC)(M1,FEF, SC)(PFC, PPC, BG)(Hipp, PFC)(VTA, SNc)EnvironmentRewardPrediction ErrorValue FunctionsActionsSocial PreferenceMODELCurrent Opinion in Neurobiology Components of social decision-making and their possible neural substrates.The environment of a decision maker provides three different types of information useful for decision-making.First,it delivers reward, and its deviation from the predicted reward(reward prediction error) modifies the value functions used to select actions.Second,information about various physical objects can be used to update the decision maker’s model about his or her environment.Third,observed actions of other actors can be used to update the decision maker’s model about their likely future behaviors.In addition,social preference of the decision makers can also influence their value functions.FEF,frontal eye field; Hipp,hippocampus;M1,primary motor cortex;PFC,prefrontal cortex; PPC,posterior parietal cortex;SC,superior colliculus;SNc,substantia nigra pars compacta;vmPFC,ventromedial prefrontal cortex;VS, ventral striatum;VTA,ventral tegmental area.reward that could have been obtained from a particular action,in addition to specific conjunctions of chosen action and its actual outcome [11 ](Figure 2).In both humans and monkeys,neurons in the medial frontal cortex encode specific actions produced by others,and thereby might contribute to extracting the information about hypothetical outcomes associated with the same actions [32,33 ].Neural basis of social preferenceThe game theory,originally developed by von Neunman and Morgenstein [34],seeks to identify a set of strategies expected for a group of rational and selfish decisionmakers,and can provide useful approximations to human behaviors observed in a broad range of social interactions [7].However,there are many counter-examples violating the assumption of purely self-interested homo econom-icus.Not only are people often willing to give up some of their incomes to benefit others,but they can also choose costly actions to punish others acting unfairly.The neural correlates of such prosocial preferences are increasingly better understood.Charitable donations are common in human societies,and sharing valuable resources or donating them to others induces activity patterns in the brain that resemble those992Decision makingFigure 260top (b) OFC coding of hypothetical outcomes(a) Rock-paper-scissors taskWWWright leftchoicetargetfeedbackrewardcomputer 130410102RP S R P Sm o n k e ywinning target40200604020060W: winning payoff432l e f tf i r i ng r a t e (s p i k e s /s )r i g h tm o n k e y c h o i c et o p402000 0.5 0 0.5 0 0.5time from feedback onset (s)Current Opinion in NeurobiologyOrbitofrontal cortex encodes hypothetical outcomes during a competitive game.(a)Simulated rock-paper-scissors task performed by monkeys (adapted from [11 ]).The animal was required to shift its gaze towards one of the three peripheral targets when the white central target wasextinguished.After a short delay,the peripheral targets all changed their colors and indicated the amount of reward available from each location (e.g.purple =2drops of fruit juice),which was determined by the payoff matrix of a biased rock-paper-scissors game shown on the right (R,rock;P,paper;S,scissors).(b)A single neuron in the orbitofrontal cortex that encoded how much reward could have been earned from an unchosen winning target (indicated by ‘W’in the top panels).The spike density function of this neuron is shown separately according to the position of the winning target (columns),the position of the target chosen by the animal (rows),and the magnitude of the reward available from the winning target (colors).resulting from individual gains.For example,decisions to donate to charitable organizations increase the activity in the ventral striatum,whereas decisions to oppose such donations activate the lateral orbitofrontal cortex[35]. Furthermore,the ventral striatum and caudate nucleus showed greater activations when the monetary transfer to the charity was voluntary compared to when it was forced or taxed[36].The level of activity in the ventral striatum and ventromedial prefrontal cortex(vmPFC)is also cor-related with the subjective value of donation to others [37,38].Activity in these two areas is temporally corre-lated with the activity in other cortical areas implicated in empathy and agency perception,such as the ACC and posterior superior temporal cortex[37,38],suggesting that they might constitute a network of brain areas responsible for modulating social preference according to the nature of interpersonal relationship.A number of studies have used ultimatum games to investigate the nature of neurobiological correlates of fairness norms.During an ultimatum game,a proposer receives afixed amount of money and offers a proportion of it to the responder,who then chooses to accept or reject the offer.Although game theory predicts that a self-interested rational proposer would offer the smallest amount possible,such small offers are frequently rejected when humans play this game.Unfair offers leads to activation in the anterior insula,DLPFC,ACC,and amygdala of the responder[39,40].Among these areas, DLPFC is implicated for enforcing costly actions with the aim of achieving fair outcomes in the long run.For example,disrupting the DLPFC activity in the responder with repetitive transcranial stimulation(rTMS)during an ultimatum game makes it more likely for unfair offers to be accepted[41],and also reduces the activity related to unfair offers in the vmPFC[42 ].Similarly,the baseline activity of the lateral prefrontal cortex of the responder predicts the rate of acceptance[43].Faces of proposers that are judged to be trustworthy also increase the accept-ance rate,and this might be mediated by the lateral orbitofrontal cortex and its connections with the amygdala and insula[44].Non-selfish behaviors,including altruistic donations or punishment,can be accounted for by a model of inequity aversion[45].In this model,the utility for a particular distribution of wealth among the members in a group is diminished by both advantageous and disadvantageous inequity that is related to guilt and envy,respectively. This model has been corroborated and further elaborated by the results from neuroimaging studies.For example, inequitable monetary transfer activates the anterior insula [46],and decreases the activity in the ventral striatum and vmPFC[47 ].Envy and its dissolution(schadenfreude) also activate ACC and ventral striatum,respectively[48]. In addition,individual variability in the strength of social preference is correlated with the activation related to inequity in the amygdala[49].These results illustrate how effects of multiple contextual factors on social pre-ference might be mediated by a network of brain areas. Both prosocial and anti-social preference has been demon-strated in non-human primates[50 ,51 ],so this remains an important topic for further research.In addition,activity of neurons in the orbitofrontal cortex of monkeys is influ-enced by the reward given to another monkey,suggesting that the neural mechanisms underlying social preference in humans and other primates might overlap[51 ]. ConclusionsThe results from the studies summarized above suggest that the brain regions involved in the valuation of differ-ent options during individual decision-making,such as the ventral striatum and vmPFC,might perform similar functions during social decision-making.Other areas, such as the amygdala and insula,might also contribute to the emotional aspect of decision-making in both social and non-social context.Furthermore,areas involved in specific aspects of social perception and cognition,such as the TPJ,might be additionally recruited during social decision-making,as when decision makers are engaged in recursive reasoning to predict the actions of others. Recent studies showed that many of these areas thought to be important for social decision-making are enlarged when the size of the social group increases[52 ,53], suggesting that additional processing power in these brain areas might be beneficial during complex social inter-action.We expect that the future studies will provide more detailed insights into the features of our brain that make us socially competent.AcknowledgementsThe authors are supported by the National Institute of Health grants (DA024855,DA029330,DA027844).References and recommended readingPapers of particular interest,published within the period of review, have been highlighted as:of special interestof outstanding interest1.Herrmann B,Tho¨ni C,Ga¨chter S:Antisocial punishment acrosssocieties.Science2008,319:1362-1367.2.De Dreu CKW,Greer LL,Handgraaf MJJ,Shalvi S,Van Kleef GA,Baas M,Ten Velden FS,Van Dijk E,Feith SWW:The neuropeptide oxytocin regulates parochial altruism in intergroup conflictamong humans.Science2010,328:1408-1411.3.De Dreu CKW,Greer LL,Van Kleef GA,Shalvi S,Handgraaf MJJ:Oxytocin promotes human ethnocentrism.Proc Natl Acad Sci U S A2011,108:1262-1266.4.Sutton RS,Barto AG:Reinforcement Learning:An Introduction.MIT Press;1998.5.Daw ND,Gershman SJ,Seymour B,Dayan P,Dolan RJ:Model-based influences on humans’choices and striatal prediction errors.Neuron2011,69:1204-1215.This study showed that the reward prediction errors computed according to model-free and model-based reinforcement learning algorithms are both encoded in the ventral striatum,suggesting that some brain areas might be utilized for both types of reinforcement learning.Neural basis of social decision-making Seo and Lee9936.Simon DA,Daw ND:Neural correlates of forward planning in aspatial decision task in humans.J Neurosci2011,31:5526-5539.7.Camerer CF:Behavioral Game Theory.Princeton University Press;2003.8.Camerer C,Ho TH:Experience-weighted attraction learning innormal form games.Econometrica1999,67:827-874.9. Zhu L,Mathewson KE,Hsu M:Dissociable neural representations of reinforcement and belief prediction errors underlie strategic learning.Proc Natl Acad Sci U S A2012, 109:1419-1424.This study showed that during an iterative competitive game,rewardprediction errors estimated using a simple,model-free reinforcement learning algorithm and those estimated using a belief learning algorithmare separately localized in the ventral striatum and rostral anterior cingu-late cortex.10.Lee D,McGreevy BP,Barraclough DJ:Learning and decisionmaking in monkeys during a rock-paper-scissors game.Cogn Brain Res2005,25:416-430.11. Abe H,Lee D:Distributed coding of actual and hypothetical outcomes in the orbital and dorsolateral prefrontal cortex. Neuron2011,70:731-741.This study demonstrated that the behaviors of monkeys during a simu-lated rock-paper-scissors game are better accounted for by a hybrid learning model than by a simple reinforcement or belief learning model.Italso showed that neurons in the orbital and lateral prefrontal cortex oftenencode specific conjunctions of counterfactual(unchosen)actions and hypothetical outcomes.12.Barraclough DJ,Conroy ML,Lee D:Prefrontal cortex anddecision making in a mixed-strategy game.Nat Neurosci2004, 7:404-410.13.Samejima K,Ueda Y,Doya K,Kimura M:Representation ofaction-specific reward values in the striatum.Science2005,310:1337-1340.u B,Glimcher PW:Value representations in the primatestriatum during matching behavior.Neuron2008,58:451-463.15.Hare TA,O’Doherty J,Camerer CF,Schultz W,Rangel A:Dissociating the role of the orbitofrontal cortex and thestriatum in the computation of goal values and predictionerrors.J Neurosci2008,28:5623-5630.16.Wunderlich K,Rangel A,O’Doherty JP:Neural computationsunderlying action-based decision making in the human brain.Proc Natl Acad Sci U S A2009,106:17199-17204.17. Vickery TJ,Chun MM,Lee D:Ubiquity and specificity of reinforcement signals throughout the human brain.Neuron 2011,72:166-177.Using a multi-voxel pattern analysis,this study demonstrated that the blood-oxygen-level-dependent signals related to decision outcomes are distributed throughout the entire human brain.18.Schultz W:Predictive reward signal of dopamine neurons.JNeurophysiol1998,80:1-27.19.Kim H,Sul JH,Huh N,Lee D,Jung MW:Role of striatum inupdating values of chosen actions.J Neurosci2009,29:14701-14712.20.Oyama K,Hernadi I,Iijima T,Tsutsui KI:Reward prediction errorcoding in dorsal striatal neurons.J Neurosci2010,30:11447-11457.21.Seo H,Lee D:Temporalfiltering of reward signals in the dorsalanterior cingulate cortex during a mixed-strategy game.JNeurosci2007,27:8366-8377.22. Kennerley SW,Behrens TE,Wallis JD:Double dissociation of value computations in orbitofrontal and anterior cingulate neurons.Nat Neurosci2011,14:1581-1589.This study showed that neurons in the anterior cingulate cortex tend to encode the reward prediction errors while those in the orbitofrontal cortex tend to encode the value of current reward relative to the animal’s reward history.23.Asaad WF,Eskandar EN:Encoding of both positive andnegative reward prediction errors by neurons of the primate lateral prefrontal cortex and caudate nucleus.J Neurosci2011, 31:17772-17787.24.Saxe R,Kanwisher N:People thinking about thinking people:the role of the temporo-parietal junction in‘‘theory of mind’’.NeuroImage2003,19:1835-1842.25.Hampton AN,Bossaerts P,O’Doherty JP:Neural correlates ofmentalizing-related computations during strategicinteractions in humans.Proc Natl Acad Sci U S A2008,105:6741-6746.26.Behrens TE,Hunt LT,Woolrich MW,Rushworth MF:Associativelearning of social value.Nature2008,456:245-249.27.Coricelli G,Nagel R:Neural correlates of depth of strategicreasoning in medial prefrontal cortex.Proc Natl Acad Sci U S A 2009,106:9163-9168.This study found that the degree of recursive strategic reasoning during a beauty-contest game is correlated with the activity in the medial fronal cortex.28.Yoshida W,Seymour B,Firston KJ,Dolan RJ:Neuralmechanisms of belief inference during cooperative games.J Neurosci2010,30:10744-10751.This study showed that during a stag hunt game,the uncertainty of inference regarding the strategy of other players and the depth of recursive strategy used to make such inference are reflected in the activity of medial and lateral prefrontal cortex,respectively.29.Bhatt MA,Lohrenz T,Camerer CF,Montague PR:Neuralsignatures of strategic types in a two-person bargaininggame.Proc Natl Acad Sci U S A2010,107:19720-19725.This study showed that the dorsolateral prefrontal cortex and Brodmann area10might be involved in strategic deception during a sender-receive game.In addition,it also found that the activity in the temporoparietal junction reflected the expected outcome of a deceptive strategy.30.Seo H,Lee D:Behavioral and neural changes after gainsand losses of conditioned reinforcers.J Neurosci2009,29:3627-3641.This study showed that during a computer-simulated biased matching pennies game,neurons in the medial frontal cortex often encoded the positive or negative outcomes resulting from specific choices.31.Hayden BY,Pearson JM,Platt ML:Fictive reward signals in the anterior cingulate cortex.Science2009,324:948-950.This study provided thefirst evidence that signals related to hypothetical rewards from unchosen actions are encoded by single neurons in the anterior cingulate cortex.32.Mukamel R,Ekstrom AD,Kaplan J,Iacoboni M,Fried I:Single-neuron responses in humans during execution andobservation of actions.Curr Biol2010,20:750-756.33.Yoshida K,Saito N,Iriki A,Isoda M:Representation of others’action by neurons in monkey medial frontal cortex.Curr Biol2011,21:249-253.This study showed that neurons in the anterior cingulate cortex differ-entially encode actions executed by the self and others.34.von Neumann J,Morgenstern O:Theory of Games and EconomicBehavior.Princeton University Press;1944.35.Moll J,Krueger F,Zahn R,Pardini M,de Oliveira-Souza R,Grafman J:Human fronto-mesolimbic networks guidedecisions about charitable donation.Proc Natl Acad Sci U S A 2006,103:15623-15628.36.Harbaugh WT,Mayr U,Burghart DR:Neural responses totaxation and voluntary giving reveal motives for charitabledonations.Science2007,316:1622-1625.37.Mobbs D,Yu R,Meyer M,Passmonti L,Seymour B,Calder AJ,Schweizer S,Frith CD,Dalgleish T:A key role for similarity invicarious reward.Science2009,324:900.38.Hare T,Camerer CF,Knoepfle DT,O’Doherty JP,Rangel A:Valuecomputations in ventral medial prefrontal cortex duringcharitable decision making incorporate input from regionsinvolved in social cognition.J Neurosci2010,30:583-590.39.Sanfey AG,Rilling JK,Aronson JA,Nystrom LE,Cohen JD:Theneural basis of economic decision-making in the ultimatumgame.Science2003,300:1755-1758.40.Gospic K,Mohlin E,Fransson P,Petrovic P,Johannesson M,Ingvar M:Limbic justice–amygdala involvement in immediate rejection in the ultimatum game.PLoS Biol2011,9:e1001054.994Decision making41.Knoch D,Pascual-Leone A,Meyer K,Treyer V,Fehr E:Diminishing reciprocal fairness by disrupting the right prefrontal cortex.Science2006,314:829-832.42. Baumgartner T,Knoch D,Hotz P,Eisenegger C,Fehr E: Dorsolateral and ventromedial prefrontal cortex orchestrate normative choice.Nat Neurosci2011,14:1468-1474.This study demonstrated that repetitive transcranial magnetic stimulation applied to the right dorsolateral prefrontal cortex of responders in an ultimatum game subsequently reduce their rejection rate,and also diminished the activity in the same area and that in the ventromedial prefrontal cortex.43.Knoch D,Gianotti LRR,Baumgartner T,Fehr E:A neural markerof costly punishment behavior.Psychol Sci2010,21:337-342.44.Kim H,Choi MJ,Jang IJ:Lateral OFC activity predicts decisionbias due tofirst impressions during ultimatum games.J Cogn Neurosci2012,24:428-439.45.Fehr E,Schmidt KM:A theory of fairness,competition,andcooperation.Q J Econ1999,114:817-868.46.Zaki J,Mitchell JP:Equitable decision making is associatedwith neural markers of intrinsic value.Proc Natl Acad Sci U S A 2011,108:19761-19766.47. Tricomi E,Rangel A,Camerer CF,O’Doherty JP:Neural evidence for inequality-averse social preferences.Nature2010,463:1089-1091.This study showed that monetary transfer increases the activity in the ventral striatum and ventromedial prefrontal cortex when it reduces the inequity between self and others.48.Takahashi H,Kato M,Matsuura M,Mobbs D,Suhara T,Okubo Y:When your gain is my pain and your pain is mygain:neural correlates of envy and schadenfreude.Science 2009,323:937-939.49.Haruno M,Frith CD:Activity in the amygdala elicited by unfairdivisions predicts social value orientation.Nat Neurosci2010, 13:160-161.50.Chang SWC,Winecoff AA,Platt ML:Vicarious reinforcement in rhesus macaques(Macaca mulatta).Front Neurosci2011,5:27. This study evaluated the social preference of monkeys during a choice task,and found that the animal’s subjective value of reward received by another animal changed depending on whether the animal making the choice receives reward or not.51.Azzi JC,Sirigu A,Duhamel JR:Modulation of valuerepresentation by social context in the primate orbitofrontalcortex.Proc Natl Aca Sci U S A2012,109:2126-2131.This study found that the monkeys were more willing to work to obtain reward only for themselves compared to when the reward was given to themselves and another monkey.In addition,it also showed that the activity of neurons in the orbitofrontal cortex reflected the animal’s social preference.52.Sallet J,Mars RB,Noonan MP,Andersson JL,O’Reilly JX,Jbabdi S,Croxson PL,Jenkinson M,Miller KL,Rushworth MFS: Social network size affects neural circuits in macaques.Science2011,334:697-700.This study found that the size of gray matter in several brain areas implicated in social cognition,such as the superior temporal sulcus and rostral pre-frontal cortex,increases in animals housed in a large social group.53.Lewis PA,Rezaie R,Brown R,Roberts N,Dunbar RIM:Ventromedial prefrontal volume predicts understandingof others and social network size.NeuroImage2011,57:1624-1629.Neural basis of social decision-making Seo and Lee995。