5 Integrating Minimalistic Localization and Navigation for People with
晶体结构缺陷的类型
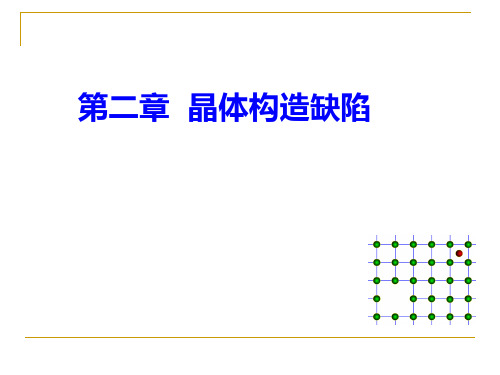
二 按缺陷产生旳原因分类
晶体缺陷
辐照缺陷 杂质缺陷
电荷缺陷 热缺陷 非化学计量缺陷
1. 热缺陷
定义:热缺陷亦称为本征缺陷,是指由热起伏旳原因所产生 旳空位或间隙质点(原子或离子)。
类型:弗仑克尔缺陷(Frenkel defect)和肖特基缺陷 (Schottky defect)
T E 热起伏(涨落) 原子脱离其平衡位置
面缺陷旳取向及分布与材料旳断裂韧性有关。
面缺陷-晶界
晶界示意图
亚晶界示意图
晶界: 晶界是两相邻晶粒间旳过渡界面。因为相邻晶粒 间彼此位向各不相同,故晶界处旳原子排列与晶内不同, 它们因同步受到相邻两侧晶粒不同位向旳综合影响,而做 无规则排列或近似于两者取向旳折衷位置旳排列,这就形 成了晶体中旳主要旳面缺陷。
-"extra" atoms positioned between atomic sites.
distortion of planes
selfinterstitiallids
Two outcomes if impurity (B) added to host (A):
• Solid solution of B in A (i.e., random dist. of point defects)
OR
Substitutional alloy (e.g., Cu in Ni)
Interstitial alloy (e.g., C in Fe)
Impurities in Ceramics
本章主要内容:
§2.1 晶体构造缺陷旳类型 §2. 2 点缺陷 §2.3 线缺陷 §2.4 面缺陷 §2.5 固溶体 §2.6 非化学计量化合物
Modeling the Spatial Dynamics of Regional Land Use_The CLUE-S Model

Modeling the Spatial Dynamics of Regional Land Use:The CLUE-S ModelPETER H.VERBURG*Department of Environmental Sciences Wageningen UniversityP.O.Box376700AA Wageningen,The NetherlandsandFaculty of Geographical SciencesUtrecht UniversityP.O.Box801153508TC Utrecht,The NetherlandsWELMOED SOEPBOERA.VELDKAMPDepartment of Environmental Sciences Wageningen UniversityP.O.Box376700AA Wageningen,The NetherlandsRAMIL LIMPIADAVICTORIA ESPALDONSchool of Environmental Science and Management University of the Philippines Los Ban˜osCollege,Laguna4031,Philippines SHARIFAH S.A.MASTURADepartment of GeographyUniversiti Kebangsaan Malaysia43600BangiSelangor,MalaysiaABSTRACT/Land-use change models are important tools for integrated environmental management.Through scenario analysis they can help to identify near-future critical locations in the face of environmental change.A dynamic,spatially ex-plicit,land-use change model is presented for the regional scale:CLUE-S.The model is specifically developed for the analysis of land use in small regions(e.g.,a watershed or province)at afine spatial resolution.The model structure is based on systems theory to allow the integrated analysis of land-use change in relation to socio-economic and biophysi-cal driving factors.The model explicitly addresses the hierar-chical organization of land use systems,spatial connectivity between locations and stability.Stability is incorporated by a set of variables that define the relative elasticity of the actual land-use type to conversion.The user can specify these set-tings based on expert knowledge or survey data.Two appli-cations of the model in the Philippines and Malaysia are used to illustrate the functioning of the model and its validation.Land-use change is central to environmental man-agement through its influence on biodiversity,water and radiation budgets,trace gas emissions,carbon cy-cling,and livelihoods(Lambin and others2000a, Turner1994).Land-use planning attempts to influence the land-use change dynamics so that land-use config-urations are achieved that balance environmental and stakeholder needs.Environmental management and land-use planning therefore need information about the dynamics of land use.Models can help to understand these dynamics and project near future land-use trajectories in order to target management decisions(Schoonenboom1995).Environmental management,and land-use planning specifically,take place at different spatial and organisa-tional levels,often corresponding with either eco-re-gional or administrative units,such as the national or provincial level.The information needed and the man-agement decisions made are different for the different levels of analysis.At the national level it is often suffi-cient to identify regions that qualify as“hot-spots”of land-use change,i.e.,areas that are likely to be faced with rapid land use conversions.Once these hot-spots are identified a more detailed land use change analysis is often needed at the regional level.At the regional level,the effects of land-use change on natural resources can be determined by a combina-tion of land use change analysis and specific models to assess the impact on natural resources.Examples of this type of model are water balance models(Schulze 2000),nutrient balance models(Priess and Koning 2001,Smaling and Fresco1993)and erosion/sedimen-tation models(Schoorl and Veldkamp2000).Most of-KEY WORDS:Land-use change;Modeling;Systems approach;Sce-nario analysis;Natural resources management*Author to whom correspondence should be addressed;email:pverburg@gissrv.iend.wau.nlDOI:10.1007/s00267-002-2630-x Environmental Management Vol.30,No.3,pp.391–405©2002Springer-Verlag New York Inc.ten these models need high-resolution data for land use to appropriately simulate the processes involved.Land-Use Change ModelsThe rising awareness of the need for spatially-ex-plicit land-use models within the Land-Use and Land-Cover Change research community(LUCC;Lambin and others2000a,Turner and others1995)has led to the development of a wide range of land-use change models.Whereas most models were originally devel-oped for deforestation(reviews by Kaimowitz and An-gelsen1998,Lambin1997)more recent efforts also address other land use conversions such as urbaniza-tion and agricultural intensification(Brown and others 2000,Engelen and others1995,Hilferink and Rietveld 1999,Lambin and others2000b).Spatially explicit ap-proaches are often based on cellular automata that simulate land use change as a function of land use in the neighborhood and a set of user-specified relations with driving factors(Balzter and others1998,Candau 2000,Engelen and others1995,Wu1998).The speci-fication of the neighborhood functions and transition rules is done either based on the user’s expert knowl-edge,which can be a problematic process due to a lack of quantitative understanding,or on empirical rela-tions between land use and driving factors(e.g.,Pi-janowski and others2000,Pontius and others2000).A probability surface,based on either logistic regression or neural network analysis of historic conversions,is made for future conversions.Projections of change are based on applying a cut-off value to this probability sur-face.Although appropriate for short-term projections,if the trend in land-use change continues,this methodology is incapable of projecting changes when the demands for different land-use types change,leading to a discontinua-tion of the trends.Moreover,these models are usually capable of simulating the conversion of one land-use type only(e.g.deforestation)because they do not address competition between land-use types explicitly.The CLUE Modeling FrameworkThe Conversion of Land Use and its Effects(CLUE) modeling framework(Veldkamp and Fresco1996,Ver-burg and others1999a)was developed to simulate land-use change using empirically quantified relations be-tween land use and its driving factors in combination with dynamic modeling.In contrast to most empirical models,it is possible to simulate multiple land-use types simultaneously through the dynamic simulation of competition between land-use types.This model was developed for the national and con-tinental level,applications are available for Central America(Kok and Winograd2001),Ecuador(de Kon-ing and others1999),China(Verburg and others 2000),and Java,Indonesia(Verburg and others 1999b).For study areas with such a large extent the spatial resolution of analysis was coarse(pixel size vary-ing between7ϫ7and32ϫ32km).This is a conse-quence of the impossibility to acquire data for land use and all driving factors atfiner spatial resolutions.A coarse spatial resolution requires a different data rep-resentation than the common representation for data with afine spatial resolution.Infine resolution grid-based approaches land use is defined by the most dom-inant land-use type within the pixel.However,such a data representation would lead to large biases in the land-use distribution as some class proportions will di-minish and other will increase with scale depending on the spatial and probability distributions of the cover types(Moody and Woodcock1994).In the applications of the CLUE model at the national or continental level we have,therefore,represented land use by designating the relative cover of each land-use type in each pixel, e.g.a pixel can contain30%cultivated land,40%grass-land,and30%forest.This data representation is di-rectly related to the information contained in the cen-sus data that underlie the applications.For each administrative unit,census data denote the number of hectares devoted to different land-use types.When studying areas with a relatively small spatial ex-tent,we often base our land-use data on land-use maps or remote sensing images that denote land-use types respec-tively by homogeneous polygons or classified pixels. When converted to a raster format this results in only one, dominant,land-use type occupying one unit of analysis. The validity of this data representation depends on the patchiness of the landscape and the pixel size chosen. Most sub-national land use studies use this representation of land use with pixel sizes varying between a few meters up to about1ϫ1km.The two different data represen-tations are shown in Figure1.Because of the differences in data representation and other features that are typical for regional appli-cations,the CLUE model can not directly be applied at the regional scale.This paper describes the mod-ified modeling approach for regional applications of the model,now called CLUE-S(the Conversion of Land Use and its Effects at Small regional extent). The next section describes the theories underlying the development of the model after which it is de-scribed how these concepts are incorporated in the simulation model.The functioning of the model is illustrated for two case-studies and is followed by a general discussion.392P.H.Verburg and othersCharacteristics of Land-Use SystemsThis section lists the main concepts and theories that are prevalent for describing the dynamics of land-use change being relevant for the development of land-use change models.Land-use systems are complex and operate at the interface of multiple social and ecological systems.The similarities between land use,social,and ecological systems allow us to use concepts that have proven to be useful for studying and simulating ecological systems in our analysis of land-use change (Loucks 1977,Adger 1999,Holling and Sanderson 1996).Among those con-cepts,connectivity is important.The concept of con-nectivity acknowledges that locations that are at a cer-tain distance are related to each other (Green 1994).Connectivity can be a direct result of biophysical pro-cesses,e.g.,sedimentation in the lowlands is a direct result of erosion in the uplands,but more often it is due to the movement of species or humans through the nd degradation at a certain location will trigger farmers to clear land at a new location.Thus,changes in land use at this new location are related to the land-use conditions in the other location.In other instances more complex relations exist that are rooted in the social and economic organization of the system.The hierarchical structure of social organization causes some lower level processes to be constrained by higher level dynamics,e.g.,the establishments of a new fruit-tree plantation in an area near to the market might in fluence prices in such a way that it is no longer pro fitable for farmers to produce fruits in more distant areas.For studying this situation an-other concept from ecology,hierarchy theory,is use-ful (Allen and Starr 1982,O ’Neill and others 1986).This theory states that higher level processes con-strain lower level processes whereas the higher level processes might emerge from lower level dynamics.This makes the analysis of the land-use system at different levels of analysis necessary.Connectivity implies that we cannot understand land use at a certain location by solely studying the site characteristics of that location.The situation atneigh-Figure 1.Data representation and land-use model used for respectively case-studies with a national/continental extent and local/regional extent.Modeling Regional Land-Use Change393boring or even more distant locations can be as impor-tant as the conditions at the location itself.Land-use and land-cover change are the result of many interacting processes.Each of these processes operates over a range of scales in space and time.These processes are driven by one or more of these variables that influence the actions of the agents of land-use and cover change involved.These variables are often re-ferred to as underlying driving forces which underpin the proximate causes of land-use change,such as wood extraction or agricultural expansion(Geist and Lambin 2001).These driving factors include demographic fac-tors(e.g.,population pressure),economic factors(e.g., economic growth),technological factors,policy and institutional factors,cultural factors,and biophysical factors(Turner and others1995,Kaimowitz and An-gelsen1998).These factors influence land-use change in different ways.Some of these factors directly influ-ence the rate and quantity of land-use change,e.g.the amount of forest cleared by new incoming migrants. Other factors determine the location of land-use change,e.g.the suitability of the soils for agricultural land use.Especially the biophysical factors do pose constraints to land-use change at certain locations, leading to spatially differentiated pathways of change.It is not possible to classify all factors in groups that either influence the rate or location of land-use change.In some cases the same driving factor has both an influ-ence on the quantity of land-use change as well as on the location of land-use change.Population pressure is often an important driving factor of land-use conver-sions(Rudel and Roper1997).At the same time it is the relative population pressure that determines which land-use changes are taking place at a certain location. Intensively cultivated arable lands are commonly situ-ated at a limited distance from the villages while more extensively managed grasslands are often found at a larger distance from population concentrations,a rela-tion that can be explained by labor intensity,transport costs,and the quality of the products(Von Thu¨nen 1966).The determination of the driving factors of land use changes is often problematic and an issue of dis-cussion(Lambin and others2001).There is no unify-ing theory that includes all processes relevant to land-use change.Reviews of case studies show that it is not possible to simply relate land-use change to population growth,poverty,and infrastructure.Rather,the inter-play of several proximate as well as underlying factors drive land-use change in a synergetic way with large variations caused by location specific conditions (Lambin and others2001,Geist and Lambin2001).In regional modeling we often need to rely on poor data describing this complexity.Instead of using the under-lying driving factors it is needed to use proximate vari-ables that can represent the underlying driving factors. Especially for factors that are important in determining the location of change it is essential that the factor can be mapped quantitatively,representing its spatial vari-ation.The causality between the underlying driving factors and the(proximate)factors used in modeling (in this paper,also referred to as“driving factors”) should be certified.Other system properties that are relevant for land-use systems are stability and resilience,concepts often used to describe ecological systems and,to some extent, social systems(Adger2000,Holling1973,Levin and others1998).Resilience refers to the buffer capacity or the ability of the ecosystem or society to absorb pertur-bations,or the magnitude of disturbance that can be absorbed before a system changes its structure by changing the variables and processes that control be-havior(Holling1992).Stability and resilience are con-cepts that can also be used to describe the dynamics of land-use systems,that inherit these characteristics from both ecological and social systems.Due to stability and resilience of the system disturbances and external in-fluences will,mostly,not directly change the landscape structure(Conway1985).After a natural disaster lands might be abandoned and the population might tempo-rally migrate.However,people will in most cases return after some time and continue land-use management practices as before,recovering the land-use structure (Kok and others2002).Stability in the land-use struc-ture is also a result of the social,economic,and insti-tutional structure.Instead of a direct change in the land-use structure upon a fall in prices of a certain product,farmers will wait a few years,depending on the investments made,before they change their cropping system.These characteristics of land-use systems provide a number requirements for the modelling of land-use change that have been used in the development of the CLUE-S model,including:●Models should not analyze land use at a single scale,but rather include multiple,interconnected spatial scales because of the hierarchical organization of land-use systems.●Special attention should be given to the drivingfactors of land-use change,distinguishing drivers that determine the quantity of change from drivers of the location of change.●Sudden changes in driving factors should not di-rectly change the structure of the land-use system asa consequence of the resilience and stability of theland-use system.394P.H.Verburg and others●The model structure should allow spatial interac-tions between locations and feedbacks from higher levels of organization.Model DescriptionModel StructureThe model is sub-divided into two distinct modules,namely a non-spatial demand module and a spatially explicit allocation procedure (Figure 2).The non-spa-tial module calculates the area change for all land-use types at the aggregate level.Within the second part of the model these demands are translated into land-use changes at different locations within the study region using a raster-based system.For the land-use demand module,different alterna-tive model speci fications are possible,ranging from simple trend extrapolations to complex economic mod-els.The choice for a speci fic model is very much de-pendent on the nature of the most important land-use conversions taking place within the study area and the scenarios that need to be considered.Therefore,the demand calculations will differ between applications and scenarios and need to be decided by the user for the speci fic situation.The results from the demandmodule need to specify,on a yearly basis,the area covered by the different land-use types,which is a direct input for the allocation module.The rest of this paper focuses on the procedure to allocate these demands to land-use conversions at speci fic locations within the study area.The allocation is based upon a combination of em-pirical,spatial analysis,and dynamic modelling.Figure 3gives an overview of the procedure.The empirical analysis unravels the relations between the spatial dis-tribution of land use and a series of factors that are drivers and constraints of land use.The results of this empirical analysis are used within the model when sim-ulating the competition between land-use types for a speci fic location.In addition,a set of decision rules is speci fied by the user to restrict the conversions that can take place based on the actual land-use pattern.The different components of the procedure are now dis-cussed in more detail.Spatial AnalysisThe pattern of land use,as it can be observed from an airplane window or through remotely sensed im-ages,reveals the spatial organization of land use in relation to the underlying biophysical andsocio-eco-Figure 2.Overview of the modelingprocedure.Figure 3.Schematic represen-tation of the procedure to allo-cate changes in land use to a raster based map.Modeling Regional Land-Use Change395nomic conditions.These observations can be formal-ized by overlaying this land-use pattern with maps de-picting the variability in biophysical and socio-economic conditions.Geographical Information Systems(GIS)are used to process all spatial data and convert these into a regular grid.Apart from land use, data are gathered that represent the assumed driving forces of land use in the study area.The list of assumed driving forces is based on prevalent theories on driving factors of land-use change(Lambin and others2001, Kaimowitz and Angelsen1998,Turner and others 1993)and knowledge of the conditions in the study area.Data can originate from remote sensing(e.g., land use),secondary statistics(e.g.,population distri-bution),maps(e.g.,soil),and other sources.To allow a straightforward analysis,the data are converted into a grid based system with a cell size that depends on the resolution of the available data.This often involves the aggregation of one or more layers of thematic data,e.g. it does not make sense to use a30-m resolution if that is available for land-use data only,while the digital elevation model has a resolution of500m.Therefore, all data are aggregated to the same resolution that best represents the quality and resolution of the data.The relations between land use and its driving fac-tors are thereafter evaluated using stepwise logistic re-gression.Logistic regression is an often used method-ology in land-use change research(Geoghegan and others2001,Serneels and Lambin2001).In this study we use logistic regression to indicate the probability of a certain grid cell to be devoted to a land-use type given a set of driving factors following:LogͩP i1ϪP i ͪϭ0ϩ1X1,iϩ2X2,i......ϩn X n,iwhere P i is the probability of a grid cell for the occur-rence of the considered land-use type and the X’s are the driving factors.The stepwise procedure is used to help us select the relevant driving factors from a larger set of factors that are assumed to influence the land-use pattern.Variables that have no significant contribution to the explanation of the land-use pattern are excluded from thefinal regression equation.Where in ordinal least squares regression the R2 gives a measure of modelfit,there is no equivalent for logistic regression.Instead,the goodness offit can be evaluated with the ROC method(Pontius and Schnei-der2000,Swets1986)which evaluates the predicted probabilities by comparing them with the observed val-ues over the whole domain of predicted probabilities instead of only evaluating the percentage of correctly classified observations at afixed cut-off value.This is an appropriate methodology for our application,because we will use a wide range of probabilities within the model calculations.The influence of spatial autocorrelation on the re-gression results can be minimized by only performing the regression on a random sample of pixels at a certain minimum distance from one another.Such a selection method is adopted in order to maximize the distance between the selected pixels to attenuate the problem associated with spatial autocorrelation.For case-studies where autocorrelation has an important influence on the land-use structure it is possible to further exploit it by incorporating an autoregressive term in the regres-sion equation(Overmars and others2002).Based upon the regression results a probability map can be calculated for each land-use type.A new probabil-ity map is calculated every year with updated values for the driving factors that are projected to change in time,such as the population distribution or accessibility.Decision RulesLand-use type or location specific decision rules can be specified by the user.Location specific decision rules include the delineation of protected areas such as nature reserves.If a protected area is specified,no changes are allowed within this area.For each land-use type decision rules determine the conditions under which the land-use type is allowed to change in the next time step.These decision rules are implemented to give certain land-use types a certain resistance to change in order to generate the stability in the land-use structure that is typical for many landscapes.Three different situations can be distinguished and for each land-use type the user should specify which situation is most relevant for that land-use type:1.For some land-use types it is very unlikely that theyare converted into another land-use type after their first conversion;as soon as an agricultural area is urbanized it is not expected to return to agriculture or to be converted into forest cover.Unless a de-crease in area demand for this land-use type occurs the locations covered by this land use are no longer evaluated for potential land-use changes.If this situation is selected it also holds that if the demand for this land-use type decreases,there is no possi-bility for expansion in other areas.In other words, when this setting is applied to forest cover and deforestation needs to be allocated,it is impossible to reforest other areas at the same time.2.Other land-use types are converted more easily.Aswidden agriculture system is most likely to be con-verted into another land-use type soon after its396P.H.Verburg and othersinitial conversion.When this situation is selected for a land-use type no restrictions to change are considered in the allocation module.3.There is also a number of land-use types that oper-ate in between these two extremes.Permanent ag-riculture and plantations require an investment for their establishment.It is therefore not very likely that they will be converted very soon after into another land-use type.However,in the end,when another land-use type becomes more pro fitable,a conversion is possible.This situation is dealt with by de fining the relative elasticity for change (ELAS u )for the land-use type into any other land use type.The relative elasticity ranges between 0(similar to Situation 2)and 1(similar to Situation 1).The higher the de fined elasticity,the more dif ficult it gets to convert this land-use type.The elasticity should be de fined based on the user ’s knowledge of the situation,but can also be tuned during the calibration of the petition and Actual Allocation of Change Allocation of land-use change is made in an iterative procedure given the probability maps,the decision rules in combination with the actual land-use map,and the demand for the different land-use types (Figure 4).The following steps are followed in the calculation:1.The first step includes the determination of all grid cells that are allowed to change.Grid cells that are either part of a protected area or under a land-use type that is not allowed to change (Situation 1,above)are excluded from further calculation.2.For each grid cell i the total probability (TPROP i,u )is calculated for each of the land-use types u accord-ing to:TPROP i,u ϭP i,u ϩELAS u ϩITER u ,where ITER u is an iteration variable that is speci fic to the land use.ELAS u is the relative elasticity for change speci fied in the decision rules (Situation 3de-scribed above)and is only given a value if grid-cell i is already under land use type u in the year considered.ELAS u equals zero if all changes are allowed (Situation 2).3.A preliminary allocation is made with an equalvalue of the iteration variable (ITER u )for all land-use types by allocating the land-use type with the highest total probability for the considered grid cell.This will cause a number of grid cells to change land use.4.The total allocated area of each land use is nowcompared to the demand.For land-use types where the allocated area is smaller than the demanded area the value of the iteration variable is increased.For land-use types for which too much is allocated the value is decreased.5.Steps 2to 4are repeated as long as the demandsare not correctly allocated.When allocation equals demand the final map is saved and the calculations can continue for the next yearly timestep.Figure 5shows the development of the iteration parameter ITER u for different land-use types during asimulation.Figure 4.Representation of the iterative procedure for land-use changeallocation.Figure 5.Change in the iteration parameter (ITER u )during the simulation within one time-step.The different lines rep-resent the iteration parameter for different land-use types.The parameter is changed for all land-use types synchronously until the allocated land use equals the demand.Modeling Regional Land-Use Change397Multi-Scale CharacteristicsOne of the requirements for land-use change mod-els are multi-scale characteristics.The above described model structure incorporates different types of scale interactions.Within the iterative procedure there is a continuous interaction between macro-scale demands and local land-use suitability as determined by the re-gression equations.When the demand changes,the iterative procedure will cause the land-use types for which demand increased to have a higher competitive capacity (higher value for ITER u )to ensure enough allocation of this land-use type.Instead of only being determined by the local conditions,captured by the logistic regressions,it is also the regional demand that affects the actually allocated changes.This allows the model to “overrule ”the local suitability,it is not always the land-use type with the highest probability according to the logistic regression equation (P i,u )that the grid cell is allocated to.Apart from these two distinct levels of analysis there are also driving forces that operate over a certain dis-tance instead of being locally important.Applying a neighborhood function that is able to represent the regional in fluence of the data incorporates this type of variable.Population pressure is an example of such a variable:often the in fluence of population acts over a certain distance.Therefore,it is not the exact location of peoples houses that determines the land-use pattern.The average population density over a larger area is often a more appropriate variable.Such a population density surface can be created by a neighborhood func-tion using detailed spatial data.The data generated this way can be included in the spatial analysis as anotherindependent factor.In the application of the model in the Philippines,described hereafter,we applied a 5ϫ5focal filter to the population map to generate a map representing the general population pressure.Instead of using these variables,generated by neighborhood analysis,it is also possible to use the more advanced technique of multi-level statistics (Goldstein 1995),which enable a model to include higher-level variables in a straightforward manner within the regression equa-tion (Polsky and Easterling 2001).Application of the ModelIn this paper,two examples of applications of the model are provided to illustrate its function.TheseTable nd-use classes and driving factors evaluated for Sibuyan IslandLand-use classes Driving factors (location)Forest Altitude (m)GrasslandSlope Coconut plantation AspectRice fieldsDistance to town Others (incl.mangrove and settlements)Distance to stream Distance to road Distance to coast Distance to port Erosion vulnerability GeologyPopulation density(neighborhood 5ϫ5)Figure 6.Location of the case-study areas.398P.H.Verburg and others。
Integrating Time Alignment and Self-Organizing Maps for Classifying Curves
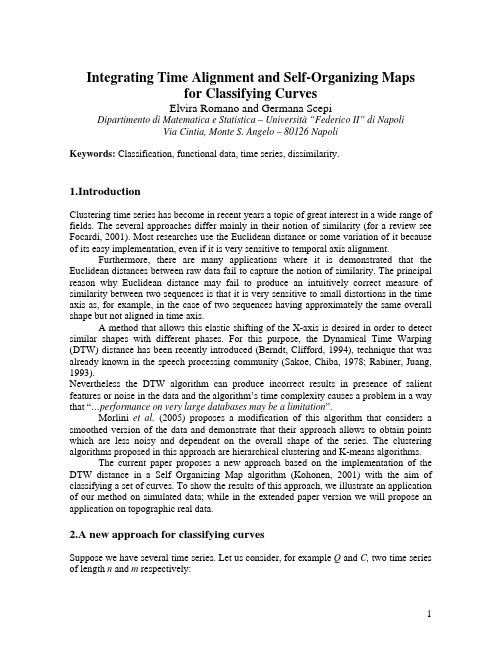
Integrating Time Alignment and Self-Organizing Mapsfor Classifying CurvesElvira Romano and Germana ScepiDipartimento di Matematica e Statistica – Università “Federico II” di NapoliVia Cintia, Monte S. Angelo – 80126 NapoliKeywords: Classification, functional data, time series, dissimilarity.1.IntroductionClustering time series has become in recent years a topic of great interest in a wide range of fields. The several approaches differ mainly in their notion of similarity (for a review see Focardi, 2001). Most researches use the Euclidean distance or some variation of it because of its easy implementation, even if it is very sensitive to temporal axis alignment.Furthermore, there are many applications where it is demonstrated that the Euclidean distances between raw data fail to capture the notion of similarity. The principal reason why Euclidean distance may fail to produce an intuitively correct measure of similarity between two sequences is that it is very sensitive to small distortions in the time axis as, for example, in the case of two sequences having approximately the same overall shape but not aligned in time axis.A method that allows this elastic shifting of the X-axis is desired in order to detect similar shapes with different phases. For this purpose, the Dynamical Time Warping (DTW) distance has been recently introduced (Berndt, Clifford, 1994), technique that was already known in the speech processing community (Sakoe, Chiba, 1978; Rabiner, Juang, 1993).Nevertheless the DTW algorithm can produce incorrect results in presence of salient features or noise in the data and the algorithm’s time complexity causes a problem in a way that “…performance on very large databases may be a limitation”.Morlini et al. (2005) proposes a modification of this algorithm that considers a smoothed version of the data and demonstrate that their approach allows to obtain points which are less noisy and dependent on the overall shape of the series. The clustering algorithms proposed in this approach are hierarchical clustering and K-means algorithms.The current paper proposes a new approach based on the implementation of the DTW distance in a Self Organizing Map algorithm (Kohonen, 2001) with the aim of classifying a set of curves. To show the results of this approach, we illustrate an application of our method on simulated data; while in the extended paper version we will propose an application on topographic real data.2.A new approach for classifying curvesSuppose we have several time series. Let us consider, for example Q and C, two time series of length n and m respectively:Q = q 1,q 2,…,q i ,…,q nC = c 1,c 2,…,c j ,…,c mThe first step of our approach consists in smoothing each series by a piecewise linear or cubic spline. Therefore our starting data are a set of curves, in the example:Q ’ = ''''12,,...,,...,i n q q q q C ’ =''''12 ,,...,,...,j m c c c cTo align the two obtained sequences using DTW, we construct an n-by-m matrix where the (i th ,j th ) element of the matrix contains the Euclidean distance between thetwo points and .Each matrix element (i,j ) corresponds to the alignment between thepoints.''i j (,)d q c 'i q 'j c A warping path, W , is a contiguous set of matrix elements that defines a mapping between Q ’ and C ’. The k -th element of W is defined as w k = (i,j )k , so we have:12,,...,,..., max(,)-1k K W w w w w m n K m n =≤≤+The warping path is typically subjected to several constraints-Boundary conditions: w 1 = (1,1) and w K = (m ,n ). Simply stated, this requires the warping path to start and finish in diagonally opposite corner cells of the matrix.- Continuity: Given w k = (a ,b ) then w k-1 = (a’,b’), where a–a' ≤1 and b-b' ≤1. This restricts the allowable steps in the warping path to adjacent cells (including diagonally adjacent cells).- Monotonicity: Given w k = (a ,b ) then w k-1 = (a',b'), where a–a' ≥0 and b-b'≥ 0. This forces the points in W to be monotonically spaced in time.We are interested only in the path that minimizes the warping cost:DTWC(',')min Q C = (1)Therefore in our approach, the data are the smoothed values of sequences and the dissimilarity between two elements is the Dynamic Time Warping Cost (DTWC). The clustering method is based on an adaptation of the Kohonen’s SOM algorithm for dissimilarity data (Golli et al, 2004).The SOM algorithm consists of neurons organized on a regular low dimensional map. More formally, the map is described by a graph (N ,Γ). N is a set of interconnectedneurons having a discrete topology defined by Γ. For each pair of neurons on the map, the distance is defined as the shortest path between them on the graph. This distance imposes a neighbourhood relation between neurons.The Dissimilarity SOM algorithm (DSOM) is an adaptation of the Kohonen’s SOM algorithm for dissimilarity data. It is a batch iterative algorithm in which the whole data set (Ω) is initially presented on the map. We denote with z l (l=1,…,N) the generic element of Ω and z l is the representation of this element in representative space D on which dissimilarity (denoted d ) is defined.Each neuron x is represented by a set of M elements of Ω , m 1,…,m g ,…,m M , called prototypes, where m g is a vector of z l element.In DSOM the prototypes associated to neurons as well as the neighbourhood function are evolving with the iterations. It starts by an initialization phase, in which the value of M is randomly chosen.The algorithm alternates affectation phases and representation phases until convergence. In the first phase each initial observation is assigned to the winning prototype according to the following assignment function:()(arg min ,T l g M)l g f z d z ∈=m (2)where the adequacy function is:()()()2,,s r T T l g l s g r r M z m d z m K d ϑ∈∈=∑∑,z z (3)with (),T g r K ϑ is the neighbourhood kernel around the neuron r and ,l s z z are the representations of the elements in the space D .At the generic h -th iteration we assign an observation to the winning prototype with the (2) and define the cluster associated to this prototype at the iteration h . The main drawback of the DSOM algorithm is the cost induced by the representation phase. A fast version of the DSOM algorithm that allows a an important reduction of its theoretical cost has been proposed by Conan-Guez et al. (2005).In our approach we aim to classify a set of curves by using the described clustering algorithm and the DTWC. Therefore in our algorithm the smoothed time series are classified by substituting the distance d in (3) with the DTWC (1).This approach allows us to have an easy visualization of data and it is computationally more efficient of the classical clustering algorithms, it deals with time series drawn from large data sets. The visualization of time series is very important for the detection of their own characteristics and gives us some information for representing each class.3. Experimental ResultsFor a first evaluation of our approach, we propose a simulation study on a small data set of 130 time series. We have generated 130 time series (Fig.1) of length 100 and in particular i) 60 time series with increasing trend, ii) 30 time series with a seasonal component only and iii) 40 time series with decreasing trend .Fig.1 The simulated time seriesWithout warping, the k-means algorithm is not often able to distinguish class i) from class ii), with a general misclassification of 53%.We wrote a Matlab program for generating the time series, smoothing each series with a cubic spline and implementing the DTW algorithm. Finally, we clustered the series on the basis of the DTWC with the DSOM algorithm.We have repeated the simulation study 150 times with different values of the smoothing parameter λ ranging from 0.05 to 0.20.The results show that (Fig.2) only the 10% of time series are misclassified (for λ=0.1) and there are very few cases of confusion between class i) and class ii).Fig.2 The classification results with the smoothed time series4. ConclusionsIn this short version of the paper, we have introduced a new approach for clustering smoothed time series, based on the joint use of the Dynamic Time Warping distance and the Dissimilarity SOM algorithm.This algorithm seems particularly promising in data mining problems and it can be applied on not aligned time points with a good visualisation of results.The forthcoming paper includes a more detailed analysis of our approach and, in particular, an application on a large set of real data, which is needed to investigate the robustness of the proposed approach in presence of irregular sampled data. A comparison, on the same data, with the algorithm proposed by Morlini et al. (2005) will be performed.In the further researches we aim to define a non parametric model for characterizing each obtained cluster. In other words, we will search for each cluster of smoothed time series a non parametric function synthesizing its elements.Main ReferencesBerndt, D., Clifford, J. (1994). Using dynamic time warping to find patterns in time series AAI -94 Workshop on Knowledge Discovery in Databases,229–248.Conan-Guez B., Rossi F., Golli A.E. (2005). A Fast Algorithm for the Self-Organizing Map on Dissimilarity Data,WSOM’05 Proceedings.Focardi S.M. (2001). Clustering delle serie storiche economiche: applicazioni e questioni computazionali, Technical Report, Supercalcolo in Economia e in Finanza Milano. Golli A.E., Conan-Guez B, Rossi F. (2004). Self-organizing maps and symbolic data CLUEB, Journal of Symbolic Data Analysis, 2, n.1, ISSN 1723–5081.Kohonen T. (2001). Self-Organizing Maps, Springer Series in Information Sciences, Springer.Molini I. (2005). On the Dynamic Time Warping for Computing the Dissimilarity Between Curves, Vichi et al. eds., New Developments in Classification and Data Analysis, Proceedings of the Meeting of the Classification and Data Analysis Group, Università di Bologna, Settembre 2003.Rabiner, L., Juang, B. (1993). Fundamentals of speech recognition, Englewood Cliffs, N.J, Prentice Hall.Sakoe, H., Chiba, S. (1978). Dynamic programming algorithm optimization for spoken word recognition. IEEE Trans. Acoustics, Speech, and Signal Processing., Volume 26, pp 143-165.。
PREFACE
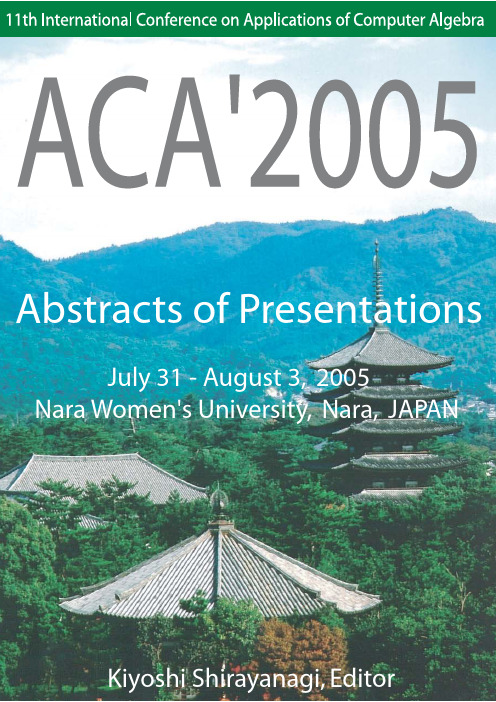
2
Nara Women’s University
3
Table of Contents
Session 1 Session 2 Session 3 Session 4 Session 5 Session 6 Session 7 Session 8 Session 9 Session 10 Session 11 Session 12 Session 13 Session 14
General Session Young Researchers Session Approximate Algebraic Computation Computational Algebraic Structures and Engineering Applications Computer Algebra and Coding Theory Computer Algebra in Quantum Information and Computation Computer Algebra in the Biological Sciences Computational Topology and Geometry Computer Algebra in Education Handling Large Expressions in Symbolic Computation High-Performance Computer Algebra Newton and Hensel Techniques in Scientific Computing Parametric and Nonconvex Constraint Solving Pen-Based Mathematical Computing
Abstracts of Presentations
集成梯度特征归属方法-概述说明以及解释
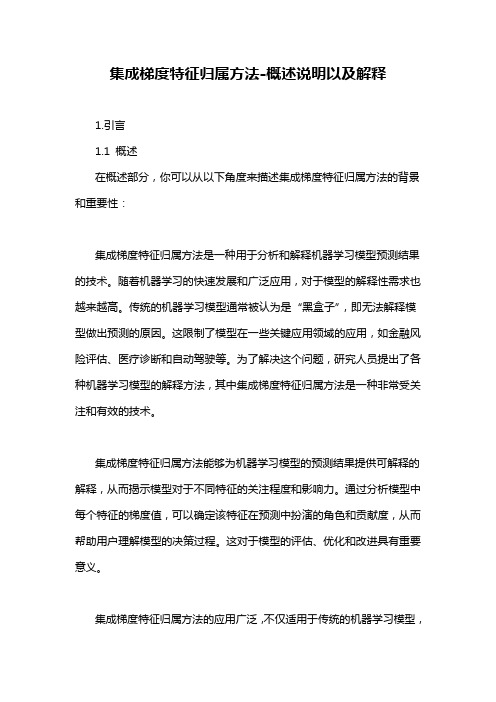
集成梯度特征归属方法-概述说明以及解释1.引言1.1 概述在概述部分,你可以从以下角度来描述集成梯度特征归属方法的背景和重要性:集成梯度特征归属方法是一种用于分析和解释机器学习模型预测结果的技术。
随着机器学习的快速发展和广泛应用,对于模型的解释性需求也越来越高。
传统的机器学习模型通常被认为是“黑盒子”,即无法解释模型做出预测的原因。
这限制了模型在一些关键应用领域的应用,如金融风险评估、医疗诊断和自动驾驶等。
为了解决这个问题,研究人员提出了各种机器学习模型的解释方法,其中集成梯度特征归属方法是一种非常受关注和有效的技术。
集成梯度特征归属方法能够为机器学习模型的预测结果提供可解释的解释,从而揭示模型对于不同特征的关注程度和影响力。
通过分析模型中每个特征的梯度值,可以确定该特征在预测中扮演的角色和贡献度,从而帮助用户理解模型的决策过程。
这对于模型的评估、优化和改进具有重要意义。
集成梯度特征归属方法的应用广泛,不仅适用于传统的机器学习模型,如决策树、支持向量机和逻辑回归等,也可以应用于深度学习模型,如神经网络和卷积神经网络等。
它能够为各种类型的特征,包括数值型特征和类别型特征,提供有益的信息和解释。
本文将对集成梯度特征归属方法的原理、应用优势和未来发展进行详细阐述,旨在为读者提供全面的了解和使用指南。
在接下来的章节中,我们将首先介绍集成梯度特征归属方法的基本原理和算法,然后探讨应用该方法的优势和实际应用场景。
最后,我们将总结该方法的重要性,并展望未来该方法的发展前景。
1.2文章结构文章结构内容应包括以下内容:文章的结构部分主要是对整篇文章的框架进行概述,指导读者在阅读过程中能够清晰地了解文章的组织结构和内容安排。
第一部分是引言,介绍了整篇文章的背景和意义。
其中,1.1小节概述文章所要讨论的主题,简要介绍了集成梯度特征归属方法的基本概念和应用领域。
1.2小节重点在于介绍文章的结构,将列出本文各个部分的标题和内容概要,方便读者快速了解文章的大致内容。
基于声学指数的神农架国家公园声音多样性动态变化
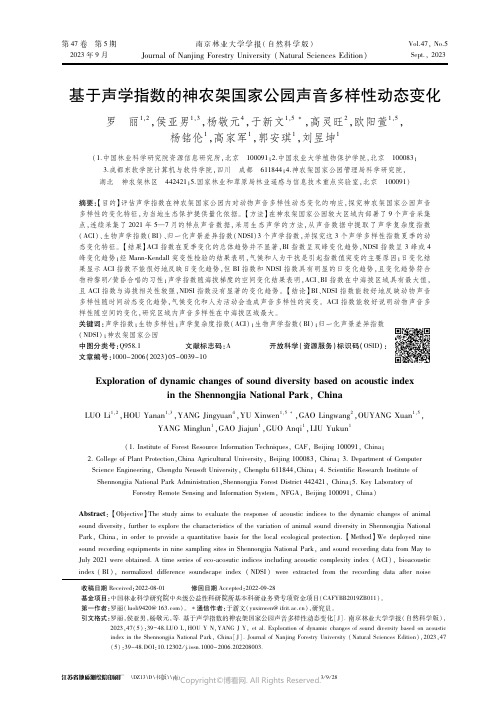
果显示 ACI 指数不能很好地反映日变化趋势,但 BI 指数和 NDSI 指数具有明显的日变化趋势,且变化趋势符合
物种黎明/ 黄昏合唱的习性;声学指数随海拔梯度的空间变化结果表明,ACI、BI 指数在中海拔区域具有最大值,
且 ACI 指数与海拔相关性较强,NDSI 指数没有显著的变化趋势。【结论】BI、NDSI 指数能较好地反映动物声音
:【 】 Abstract Objective The study aims to evaluate the response of acoustic indices to the dynamic changes of animal , sound diversity further to explore the characteristics of the variation of animal sound diversity in Shennongjia National , , 【 】 Park China in order to provide a quantitative basis for the local ecological protection. Method We deployed nine , sound recording equipments in nine sampling sites in Shennongjia National Park and sound recording data from May to ( ), July 2021 were obtained. A time series of ecoacosutic indices including acoustic complexity index ACI bioacoustic ( ), ( ) index BI normalized difference soundscape index NDSI were extracted from the recording data after noise
电容去离子除氯电极的构建及其脱盐性能研究进展

物 理 化 学 学 报Acta Phys. -Chim. Sin. 2022, 38 (5), 2006037 (1 of 12)Received: June 12, 2020; Revised: July 2, 2020; Accepted: July 7, 2020; Published online: July 13, 2020. *Correspondingauthor.Email:**************.cn.The project was supported by the National Natural Science Foundation of China (21777118). 国家自然科学基金(21777118)资助项目© Editorial office of Acta Physico-Chimica Sinica[Review] doi: 10.3866/PKU.WHXB202006037 Research Progress in Chlorine Ion Removal Electrodes for Desalination by Capacitive DeionizationYuecheng Xiong 1, Fei Yu 2, Jie Ma 1,3,*1 Key Laboratory of Yangtze River Water Environment, Tongji University, Shanghai 200092, China.2 College of Marine Ecology and Environment, Shanghai Ocean University, Shanghai 201306, China.3 Shanghai Institute of Pollution Control and Ecological Security, Shanghai 200092, China .Abstract: Sustainable freshwater supply is a grave challenge to the society because of the severe water scarcity and global pollution. Seawater is an inexhaustible source of industrial and potable water. The relevant desalination technologies with a high market share include reverse osmosis and thermal distillation, which are energy-intensive. Capacitive deionization (CDI) is a desalination technology that is gaining extensive attention because of its low energy consumption and low chemical intensity. In CDI, charged species are removed from the aqueous environment via applying a voltage onto the anode and cathode. For desalination, Na + and Cl − ions are removed by the cathode and anode, respectively. With the boom in electrode materials for rechargeable batteries, the Na + removal electrode (cathode) hasevolved from a carbon-based electrode to a faradaic electrode, and the desalination performance of CDI has also been significantly enhanced. A conventional carbon-based electrode captures ions in the electrical double layer (EDL) and suffers from low charge efficiency, thus being unsuitable for use in water with high salinity. On the other hand, a faradaic electrode stores Na + ions through a reversible redox process or intercalation, leading to high desalination capacity.However, the Cl − removal electrode (anode) has not yet seen notable development. Most research groups employ activated carbon to remove Cl −, and therefore, summarizing Cl − storage electrodes for CDI is necessary to guide the design of electrode systems with better desalination performance. First, this review outlines the evolution of CDI configuration based on the electrode materials, suggesting that the anode and cathode are of equal importance in CDI. Second, a systematic summary of the anode materials used in CDI and a comparison of the characteristics of different electrodes, including those based on Ag/AgCl, Bi/BiOCl, 2-dimensional (2D) materials (layered double hydroxide (LDH) and MXene), redox polymers, and electrolytes, are presented. Then, the underlying mechanism for Cl − storage is refined. Similar to the case of Na + storage, traditional carbon electrodes store Cl- via electrosorption based on the EDL. Ag/AgCl and Bi/BiOCl remove Cl − through a conversion reaction, i.e ., phase transformation during the reaction with Cl −. 2D materials store Cl − in the space between adjacent layers, a process referred as ion intercalation, with layered double hydroxide (LDH) and MXene showing higher Cl − storage potential. Redox polymers and electrolytes allow for Cl − storage via redox reactions. Among all the materials mentioned above, Bi/BiOCl and LDH are the most promising for the construction of CDI anodes because of their high capacity and low cost. Finally, to spur the development of novel anodes for CDI, the electrodes applied in a chlorine ion battery are introduced. This is the first paper to comb through reports on the development of anode materials for CDI, thus laying the theoretical foundation for future materials design. Key Words: Capacitive deionization; Desalination; Anode; Chlorineion; Battery电容去离子除氯电极的构建及其脱盐性能研究进展熊岳城1,于飞2,马杰1,3,*1同济大学长江水环境教育部重点实验室,上海 2000922上海海洋大学海洋生态与环境学院,上海 2013063上海污染控制与生态安全研究院,上海 200092摘要:电容去离子技术(Capacitive deionization,CDI)是一种新兴的脱盐技术,通过在电极两端施加较低的外加电场除去水中的带电离子和分子,由于其较低的能耗和可持续性而备受关注。
Accurate Passive Location Estimation Using TOA Measurements
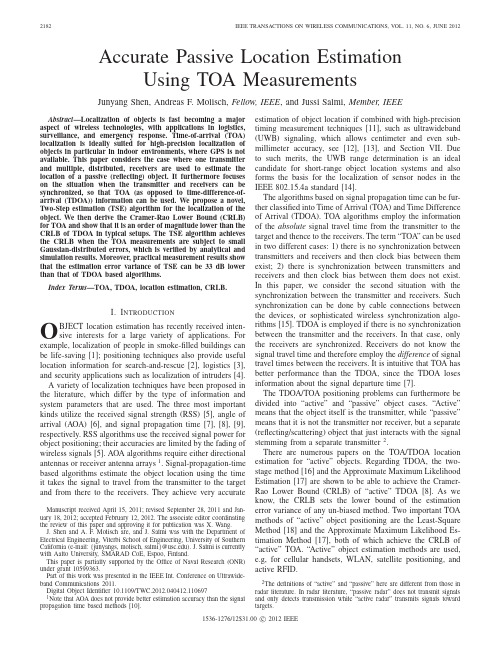
Accurate Passive Location Estimation Using TOA MeasurementsJunyang Shen,Andreas F.Molisch,Fellow,IEEE,and Jussi Salmi,Member,IEEEAbstract—Localization of objects is fast becoming a major aspect of wireless technologies,with applications in logistics, surveillance,and emergency response.Time-of-arrival(TOA) localization is ideally suited for high-precision localization of objects in particular in indoor environments,where GPS is not available.This paper considers the case where one transmitter and multiple,distributed,receivers are used to estimate the location of a passive(reflecting)object.It furthermore focuses on the situation when the transmitter and receivers can be synchronized,so that TOA(as opposed to time-difference-of-arrival(TDOA))information can be used.We propose a novel, Two-Step estimation(TSE)algorithm for the localization of the object.We then derive the Cramer-Rao Lower Bound(CRLB) for TOA and show that it is an order of magnitude lower than the CRLB of TDOA in typical setups.The TSE algorithm achieves the CRLB when the TOA measurements are subject to small Gaussian-distributed errors,which is verified by analytical and simulation results.Moreover,practical measurement results show that the estimation error variance of TSE can be33dB lower than that of TDOA based algorithms.Index Terms—TOA,TDOA,location estimation,CRLB.I.I NTRODUCTIONO BJECT location estimation has recently received inten-sive interests for a large variety of applications.For example,localization of people in smoke-filled buildings can be life-saving[1];positioning techniques also provide useful location information for search-and-rescue[2],logistics[3], and security applications such as localization of intruders[4].A variety of localization techniques have been proposed in the literature,which differ by the type of information and system parameters that are used.The three most important kinds utilize the received signal strength(RSS)[5],angle of arrival(AOA)[6],and signal propagation time[7],[8],[9], respectively.RSS algorithms use the received signal power for object positioning;their accuracies are limited by the fading of wireless signals[5].AOA algorithms require either directional antennas or receiver antenna arrays1.Signal-propagation-time based algorithms estimate the object location using the time it takes the signal to travel from the transmitter to the target and from there to the receivers.They achieve very accurate Manuscript received April15,2011;revised September28,2011and Jan-uary18,2012;accepted February12,2012.The associate editor coordinating the review of this paper and approving it for publication was X.Wang.J.Shen and A.F.Molisch are,and J.Salmi was with the Department of Electrical Engineering,Viterbi School of Engineering,University of Southern California(e-mail:{junyangs,molisch,salmi}@).J.Salmi is currently with Aalto University,SMARAD CoE,Espoo,Finland.This paper is partially supported by the Office of Naval Research(ONR) under grant10599363.Part of this work was presented in the IEEE Int.Conference on Ultrawide-band Communications2011.Digital Object Identifier10.1109/TWC.2012.040412.1106971Note that AOA does not provide better estimation accuracy than the signal propagation time based methods[10].estimation of object location if combined with high-precision timing measurement techniques[11],such as ultrawideband (UWB)signaling,which allows centimeter and even sub-millimeter accuracy,see[12],[13],and Section VII.Due to such merits,the UWB range determination is an ideal candidate for short-range object location systems and also forms the basis for the localization of sensor nodes in the IEEE802.15.4a standard[14].The algorithms based on signal propagation time can be fur-ther classified into Time of Arrival(TOA)and Time Difference of Arrival(TDOA).TOA algorithms employ the information of the absolute signal travel time from the transmitter to the target and thence to the receivers.The term“TOA”can be used in two different cases:1)there is no synchronization between transmitters and receivers and then clock bias between them exist;2)there is synchronization between transmitters and receivers and then clock bias between them does not exist. In this paper,we consider the second situation with the synchronization between the transmitter and receivers.Such synchronization can be done by cable connections between the devices,or sophisticated wireless synchronization algo-rithms[15].TDOA is employed if there is no synchronization between the transmitter and the receivers.In that case,only the receivers are synchronized.Receivers do not know the signal travel time and therefore employ the difference of signal travel times between the receivers.It is intuitive that TOA has better performance than the TDOA,since the TDOA loses information about the signal departure time[7].The TDOA/TOA positioning problems can furthermore be divided into“active”and“passive”object cases.“Active”means that the object itself is the transmitter,while“passive”means that it is not the transmitter nor receiver,but a separate (reflecting/scattering)object that just interacts with the signal stemming from a separate transmitter2.There are numerous papers on the TOA/TDOA location estimation for“active”objects.Regarding TDOA,the two-stage method[16]and the Approximate Maximum Likelihood Estimation[17]are shown to be able to achieve the Cramer-Rao Lower Bound(CRLB)of“active”TDOA[8].As we know,the CRLB sets the lower bound of the estimation error variance of any un-biased method.Two important TOA methods of“active”object positioning are the Least-Square Method[18]and the Approximate Maximum Likelihood Es-timation Method[17],both of which achieve the CRLB of “active”TOA.“Active”object estimation methods are used, e.g,for cellular handsets,WLAN,satellite positioning,and active RFID.2The definitions of“active”and“passive”here are different from those in radar literature.In radar literature,“passive radar”does not transmit signals and only detects transmission while“active radar”transmits signals toward targets.1536-1276/12$31.00c 2012IEEE“Passive”positioning is necessary in many practical situa-tions like crime-prevention surveillance,assets tracking,and medical patient monitoring,where the target to be localized is neither transmitter nor receiver,but a separate(reflect-ing/scattering)object.The TDOA positioning algorithms for “passive”objects are essentially the same as for“active”objects.For TOA,however,the synchronization creates a fundamental difference between“active”and“passive”cases. Regarding the“passive”object positioning,to the best of our knowledge,no TOA algorithms have been developed.This paper aims tofill this gap by proposing a TOA algorithm for passive object location estimation,which furthermore achieves the CRLB of“passive”TOA.The key contributions are:•A novel,two step estimation(TSE)method for the passive TOA based location estimation.It borrows an idea from the TDOA algorithm of[16].•CRLB for passive TOA based location estimation.When the TOA measurement error is Gaussian and small,we prove that the TSE can achieve the CRLB.Besides,it is also shown that the estimated target locations by TSE are Gaussian random variables whose covariance matrix is the inverse of the Fisher Information Matrix(FIM)related to the CRLB.We also show that in typical situations the CRLB of TOA is much lower than that of TDOA.•Experimental study of the performances of TSE.With one transmitter and three receivers equipped with UWB antennas,we perform100experimental measurements with an aluminium pole as the target.After extracting the signal travel time by high-resolution algorithms,the location of the target is evaluated by TSE.We show that the variance of estimated target location by TSE is much (33dB)lower than that by the TDOA method in[16]. The remainder of this paper is organized as follows.Section II presents the architecture of positioning system.Section III derives the TSE,followed by comparison between CRLB of TOA and TDOA algorithms in Section IV.Section V analyzes the performance of TSE.Section VI presents the simulations results.Section VII evaluates the performance of TSE based on UWB measurement.Finally Section VIII draws the conclusions.Notation:Throughout this paper,a variable with“hat”ˆ•denotes the measured/estimated values,and the“bar”¯•denotes the mean value.Bold letters denote vectors/matrices. E(•)is the expectation operator.If not particularly specified,“TOA”in this paper denotes the“TOA”for a passive object.II.A RCHITECTURE OF L OCALIZATION S YSTEMIn this section,wefirst discuss the challenges of localization systems,and present the focus of this paper.Then,the system model of individual localization is discussed.A.Challenges for target localizationFor easy understanding,we consider an intruder localization system using UWB signals.Note that the intruder detection can also be performed using other methods such as the Device-free Passive(DfP)approach[19]and Radio Frequency Identification(RFID)method[20].However,both the DfP and RFID methods are based on preliminary environmental measurement information like“Radio Map Construction”[19] and“fingerprints”[20].On the other hand,the TOA based approach considered in our framework does not require the preliminary efforts for obtaining environmental information. With this example,we show the challenges of target po-sitioning system:Multiple Source Separation,Indirect Path Detection and Individual Target Localization.The intruder detection system localizes,and then directs a camera to capture the photo of the targets(intruders).This localization system consists of one transmitter and several receivers.The transmitter transmits signals which are reflected by the targets,then,the receivers localize the targets based on the received signals.Multiple Source Separation:If there are more than one intruders,the system needs to localize each of them.With multiple targets,each receiver receives impulses from several objects.Only the information(such as TOA)extracted from impulses reflected by the same target should be combined for localization.Thus,the Multiple Source Separation is very important for target localization and several techniques have been proposed for this purpose.In[21],a pattern recognition scheme is used to perform the Multiple Source Separation. Video imaging and blind source separation techniques are employed for target separation in[22].Indirect Path Detection:The transmitted signals are not only reflected by the intruders,but also by surrounding objects,such as walls and tables.To reduce the adverse impact of non-target objects in the localization of target, the localization process consists of two steps.In the initial/first stage,the system measures and then stores the channel impulses without the intruders.These impulses are reflected by non-target objects,which is referred to as reflectors here.The radio signal paths existing without the target are called background paths.When the intruders are present,the system performs the second measurement. To obtain the impulses related to the intruders,the system subtracts the second measurement with thefirst one. The remaining impulses after the subtraction can be through one of the following paths:a)transmitter-intruders-receivers,b)transmitter-reflectors-intruders-receivers,c) transmitter-intruders-reflectors-receivers,d)transmitter-reflectors-intruders-reflectors-receivers3.Thefirst kind of paths are called direct paths and the rest are called indirect paths.In most situations,only direct paths can be used for localization.In the literature,there are several methods proposed for indirect path identification[23],[24]. Individual Target Localization:After the Multiple Source Separation and Indirect Path Detection,the positioning system knows the signal impulses through the direct paths for each target.Then,the system extracts the characteristics of direct paths such as TOA and AOA.Based on these characteristics, the targets arefinally localized.Most researches on Individual Target Localization assumes that Multiple Source Separation and Indirect Path Detection are perfectly performed such as [16],[25]and[26].Note that the three challenges sometimes 3Note that here we omit the impulses having two or more interactions with the intruder because of the resulted low signal-to-noise radio(SNR)by multiple reflections.Cable for synchronizationFig.1.Illustration of TOA based Location Estimation System Model.are jointly addressed,so that the target locations are estimated in one step such as the method presented in [27].In this paper,we focus on the Individual Target Local-ization,under the same framework of [16],[25]and [26],assuming that Multiple Source Separation and Indirect Path Detection are perfectly performed in prior.In addition,we only use the TOA information for localization,which achieves very high accuracy with ultra-wideband signals.The method to ex-tract TOA information using background channel cancelation is described in details in [28]and also Section VII.B.System Model of Individual LocalizationFor ease of exposition,we consider the passive object (target)location estimation problem in a two-dimensional plane as shown in Fig.1.There is a target whose location [x,y ]is to be estimated by a system with one transmitter and M receivers.Without loss of generality,let the location of the transmitter be [0,0],and the location of the i th receiver be [a i ,b i ],1≤i ≤M .The transmitter transmits an impulse;the receivers subsequently receive the signal copies reflected from the target and other objects.We adopt the assumption also made in [16],[17]that the target reflects the signal into all ing (wired)backbone connections be-tween the transmitter and receivers,or high-accuracy wireless synchronization algorithms,the transmitter and receivers are synchronized.The errors of cable synchronization are negli-gible compared with the TOA measurement errors.Thus,at the estimation center,signal travel times can be obtained by comparing the departure time at the transmitter and the arrival time at the receivers.Let the TOA from the transmitter via the target to the i th receiver be t i ,and r i =c 0t i ,where c 0is the speed of light,1≤i ≤M .Then,r i = x 2+y 2+(x −a i )2+(y −b i )2i =1,...M.(1)For future use we define r =[r 1,r 2,...,r M ].Assuming each measurement involves an error,we haver i −ˆri =e i ,1≤i ≤M,where r i is the true value,ˆr i is the measured value and e i is the measurement error.In our model,the indirect paths areignored and we assume e i to be zero mean.The estimation system tries to find the [ˆx ,ˆy ],that best fits the above equations in the sense of minimizing the error varianceΔ=E [(ˆx −x )2+(ˆy −y )2].(2)Assuming the e i are Gaussian-distributed variables with zeromean and variances σ2i ,the conditional probability functionof the observations ˆr are formulated as follows:p (ˆr |z )=Ni =11√2πσi ·exp −(ˆr i −( x 2+y 2+ (x −a i )2+(y −b i )2))22σ2i,(3)where z =[x,y ].III.TSE M ETHODIn this section,we present the two steps of TSE andsummarize them in Algorithm 1.In the first step of TSE,we assume x ,y , x 2+y 2are independent of each other,and obtain temporary results for the target location based on this assumption.In the second step,we remove the assumption and update the estimation results.A.Step 1of TSEIn the first step of TSE,we obtain an initial estimate of[x,y, x 2+y 2],which is performed in two stages:Stage A and Stage B.The basic idea here is to utilize the linear approximation [16][29]to simplify the problem,considering that TOA measurement errors are small with UWB signals.Let v =x 2+y 2,taking the squares of both sides of (1)leads to2a i x +2b i y −2r i v =a 2i +b 2i −r 2i .Since r i −ˆr i =e i ,it follows that−a 2i +b 2i −ˆr 2i 2+a i x +b i y −ˆr i v=e i (v −ˆr i )−e 2i 2=e i (v −ˆr i )−O (e 2i ).(4)where O (•)is the Big O Notation meaning that f (α)=O (g (α))if and only if there exits a positive real number M and a real number αsuch that|f (α)|≤M |g (α)|for all α>α0.If e i is small,we can omit the second or higher order terms O (e 2i )in Eqn (4).In the following of this paper,we do this,leaving the linear (first order)term.Since there are M such equations,we can express them in a matrix form as followsh −S θ=Be +O (e 2)≈Be ,(5)whereh=⎡⎢⎢⎢⎢⎣−a21+b21−ˆr212−a22+b22−ˆr222...−a2M+b2M−ˆr2M2⎤⎥⎥⎥⎥⎦,S=−⎡⎢⎢⎢⎣a1b1−ˆr1a2b2−ˆr2...a Mb M−ˆr M⎤⎥⎥⎥⎦,θ=[x,y,v]T,e=[e1,e2,...,e M]T,andB=v·I−diag([r1,r2,...,r M]),(6) where O(e2)=[O(e21),O(e22),...,O(e2M)]T and diag(a) denotes the diagonal matrix with elements of vector a on its diagonal.For notational convenience,we define the error vectorϕ=h−Sθ.(7) According to(5)and(7),the mean ofϕis zero,and its covariance matrix is given byΨ=E(ϕϕT)=E(Bee T B T)+E(O(e2)e T B T)+E(Be O(e2)T)+E(O(e2)O(e2)T)≈¯BQ¯B T(8)where Q=diag[σ21,σ22,...,σ2M].Because¯B depends on the true values r,which are not obtainable,we use B(derived from the measurementsˆr)in our calculations.From(5)and the definition ofϕ,it follows thatϕis a vector of Gaussian variables;thus,the probability density function (pdf)ofϕgivenθisp(ϕ|θ)≈1(2π)M2|Ψ|12exp(−12ϕTΨ−1ϕ)=1(2π)M2|Ψ|12exp(−12(h−Sθ)TΨ−1(h−Sθ)).Then,lnp(ϕ|θ)≈−12(h−Sθ)TΨ−1(h−Sθ)+ln|Ψ|−M2ln2π(9)We assume for the moment that x,y,v are independent of each other(this clearly non-fulfilled assumption will be relaxed in the second step of the algorithm).Then,according to(9),the optimumθthat maximizes p(ϕ|θ)is equivalent to the one minimizingΠ=(h−Sθ)TΨ−1(h−Sθ)+ln|Ψ|. IfΨis a constant,the optimumθto minimizeΠsatisfies dΠdθθ=0.Taking the derivative ofΠoverθ,we havedΠdθθ=−2S TΨ−1h+2S TΨ−1Sθ.Fig.2.Illustration of estimation ofθin step1of TSE.Thus,the optimumθsatisfiesˆθ=arg minθ{Π}=(S TΨ−1S)−1S TΨ−1h,(10)which provides[ˆx,ˆy].Note that(10)also provides the leastsquares solution for non-Gaussian errors.However,for our problem,Ψis a function ofθsince Bdepends on the(unknown)values[x,y].For this reason,themaximum-likelihood(ML)estimation method in(10)can notbe directly used.Tofind the optimumθ,we perform theestimation in two stages:Stage A and Stage B.In Stage A,themissing data(Ψ)is calculated given the estimate of parameters(θ).Note thatθprovides the values of[x,y]and thus thevalue of B,therefore,Ψcan be calculated usingθby(8).In the Stage B,the parameters(θ)are updated according to(10)to maximize the likelihood function(which is equivalentto minimizingΠ).These two stages are iterated until con-vergence.Simulations in Section V show that commonly oneiteration is enough for TSE to closely approach the CRLB,which indicates that the global optimum is reached.B.Step2of TSEIn the above calculations,ˆθcontains three componentsˆx,ˆy andˆv.They were previously assumed to be independent;however,ˆx andˆy are clearly not independent ofˆv.As amatter of fact,we wish to eliminateˆv;this will be achievedby treatingˆx,ˆy,andˆv as random variables,and,knowing thelinear mapping of their squared values,the problem can besolved using the LS solution.Letˆθ=⎡⎣ˆxˆyˆv⎤⎦=⎡⎣x+n1y+n2v+n3⎤⎦(11)where n i(i=1,2,3)are the estimation errors of thefirststep.Obviously,the estimator(10)is an unbiased one,and themean of n i is zero.Before proceeding,we need the following Lemma.Lemma 1:By omitting the second or higher order errors,the covariance of ˆθcan be approximated as cov (ˆθ)=E (nn T )≈(¯S T Ψ−1¯S )−1.(12)where n =[n 1,n 2,n 3]T ,and Ψand ¯S(the mean value of S )use the true/mean values of x ,y,and r i .Proof:Please refer to the Appendix.Note that since the true values of x ,y,and r i are not obtain-able,we use the estimated/measured values in the calculationof cov (ˆθ).Let us now construct a vector g as followsg =ˆΘ−G Υ,(13)where ˆΘ=[ˆx 2,ˆy 2,ˆv 2]T ,Υ=[x 2,y 2]T and G =⎡⎣100111⎤⎦.Note that here ˆΘis the square of estimation result ˆθfrom the first step containing the estimated values ˆx ,ˆy and ˆv .Υis the vector to be estimated.If ˆΘis obtained without error,g =0and the location of the target is perfectly obtained.However,the error inevitably exists and we need to estimate Υ.Recalling that v =x 2+y 2,substituting (11)into (13),and omitting the second-order terms n 21,n 22,n 23,it follows that,g =⎡⎣2xn 1+O (n 21)2yn 2+O (n 22)2vn 3+O (n 23)⎤⎦≈⎡⎣2xn 12yn 22vn 3⎤⎦.Besides,following similar procedure as that in computing(8),we haveΩ=E (gg T )≈4¯D cov (ˆθ)¯D ,(14)where ¯D =diag ([¯x ,¯y ,¯v ]).Since x ,y are not known,¯Dis calculated as ˆD using the estimated values ˆx ,ˆy from the firststep.The vector g can be approximated as a vector of Gaussian variables.Thus the maximum likelihood estimation of Υis theone minimizing (ˆΘ−G Υ)T Ω−1(ˆΘ−G Υ),expressed by ˆΥ=(G T Ω−1G )−1G T Ω−1ˆΘ.(15)The value of Ωis calculated according to (14)using the valuesof ˆx and ˆy in the first step.Finally,the estimation of target location z is obtained byˆz =[ˆx ,ˆy ]=[±ˆΥ1,± ˆΥ2],(16)where ˆΥi is the i th item of Υ,i =1,2.To choose the correct one among the four values in (16),we can test the square error as followsχ=M i =1( ˆx 2+ˆy 2+ (ˆx −a i )2+(ˆy −b i )−ˆr i )2.(17)The value of z that minimizes χis considered as the final estimate of the target location.In summary,the procedure of TSE is listed in Algorithm 1:Note that one should avoid placing the receivers on a line,since in this case (S T Ψ−1S )−1can become nearly singular,and solving (10)is not accurate.Algorithm 1TSE Location Estimation Method1.In the first step,use algorithm as shown in Fig.2to obtain ˆθ,2.In the second step,use the values of ˆx and ˆy from ˆθ,generate ˆΘand D ,and calculate Ω.Then,calculate the value of ˆΥby (15),3.Among the four candidate values of ˆz =[ˆx ,ˆy ]obtained by (16),choose the one minimizing (17)as the final estimate for target location.IV.C OMPARISON OF CRLB BETWEEN TDOA AND TOA In this section,we derive the CRLB of TOA based estima-tion algorithms and show that it is much lower (can be 30dB lower)than the CRLB of TDOA algorithms.The CRLB of “active”TOA localization has been studied in [30].The “passive”localization has been studied before under the model of multistatic radar [31],[32],[33].The difference between our model and the radar model is that in our model the localization error is a function of errors of TOA measurements,while in the radar model the localization error is a function of signal SNR and waveform.The CRLB is related to the 2×2Fisher Information Matrix (FIM)[34],J ,whose components J 11,J 12,J 21,J 22are defined in (18)–(20)as follows J 11=−E (∂2ln(p (ˆr |z ))∂x 2)=ΣM i =11σ2i (x −a i (x −a i )2+(y −b i )2+xx 2+y2)2,(18)J 12=J 21=−E (∂2ln(p (ˆr |z ))∂x∂y )=ΣM i =11σ2i (x −a i (x −a i )2+(y −b i )2+x x 2+y 2)×(y −b i (x −a i )2+(y −b i )2+yx 2+y 2),(19)J 22=−E (∂2ln(p (ˆr |z ))∂y 2)=ΣM i =11σ2i (y −b i (x −a i )2+(y −b i )2+yx 2+y2)2.(20)This can be expressed asJ =U T Q −1U ,(21)where Q is defined after Eqn.(8),and the entries of U in the first and second column are{U }i,1=x ¯r i −a ix 2+y 2(x −a i )2+(y −b i )2 x 2+y 2,(22)and{U }i,2=y ¯r i −b ix 2+y 2(x −a i )2+(y −b i )2 x 2+y 2,(23)with ¯r i =(x −a i )2+(y −b i )2+ x 2+y 2.The CRLB sets the lower bound for the variance of esti-mation error of TOA algorithms,which can be expressed as [34]E [(ˆx −x )2+(ˆy −y )2]≥ J −1 1,1+J −1 2,2=CRLB T OA ,(24)where ˆx and ˆy are the estimated values of x and y ,respec-tively,and J −1 i,j is the (i,j )th element of the inverse matrix of J in (21).For the TDOA estimation,its CRLB has been derived in [16].The difference of signal travel time between several receivers are considered:(x −a i )2+(y −b i )2−(x −a 1)2+(y −b 1)2=r i −r 1=l i ,2≤i ≤M.(25)Let l =[l 2,l 3,...,l M ]T ,and t be the observa-tions/measurements of l ,then,the conditional probability density function of t is p (t |z )=1(2π)(M −1)/2|Z |12×exp(−12(t −l )T Z −1(t −l )),where Z is the correlation matrix of t ,Z =E (tt T ).Then,the FIM is expressed as [16]ˇJ=ˇU T Z −1ˇU (26)where ˇUis a M −1×2matrix defined as ˇU i,1=x −a i (x −a i )2+(y −b i )2−x −a 1(x −a 1)2+(y −b 1)2,ˇUi,2=y −b i (x −a i )2+(y −b i )2−y −b 1(x −a 1)2+(y −b 1)2.The CRLB sets the lower bound for the variance of esti-mation error of TDOA algorithms,which can be expressed as [34]:E [(ˆx −x )2+(ˆy −y )2]≥ ˇJ −1 1,1+ ˇJ −1 2,2=CRLB T DOA .(27)Note that the correlation matrix Q for TOA is different from the correlation matrix Z for TDOA.Assume the variance of TOA measurement at i th (1≤i ≤M )receiver is σ2i ,it follows that:Q (i,j )=σ2i i =j,0i =j.and Z (i,j )= σ21+σ2i +1i =j,σ21i =j.As an example,we consider a scenario wherethere is a transmitter at [0,0],and four receivers at [−6,2],[6.2,1.4],[1.5,4],[2,2.3].The range of the targetlocations is 1≤x ≤10,1≤y ≤10.The ratio of CRLB of TOA over that of TDOA is plotted in Fig.3.Fig.3(a)shows the contour plot while Fig.3(b)shows the color-coded plot.It can be observed that the CRLB of TOA is always —in most cases significantly —lower than that of TDOA.xy(a )xy0.10.20.30.40.50.60.70.80.9Fig.3.CRLB ratio of passive TOA over passive TDOA estimation:(a)contour plot;(b)pcolor plot.V.P ERFORMANCE OF TSEIn this section,we first prove that the TSE can achieve the CRLB of TOA algorithms by showing that the estimation error variance of TSE is the same as the CRLB of TOA algorithms.In addition,we show that,for small TOA error regions,the estimated target location is approximately a Gaussian random variable whose covariance matrix is the inverse of the Fisher Information Matrix (FIM),which in turn is related to the CRLB.Similar to the reasoning in Lemma 1,we can obtain the variance of error in the estimation of Υas follows:cov (ˆΥ)≈(G T Ω−1G )−1.(28)Let ˆx =x +e x ,ˆy=y +e y ,and insert them into Υ,omitting the second order errors,we obtainˆΥ1−x 2=2xe x +O (e 2x )≈2xe x ˆΥ2−y 2=2ye y +O (e 2y)≈2ye y (29)Then,the variance of the final estimate of target location ˆzis cov (ˆz )=E (e x e ye x e y )≈14C −1E ( Υ1−x 2Υ2−y 2Υ1−x 2Υ2−y 2 )C −1=14C −1cov (ˆΥ)C −1,(30)where C = x 00y.Substituting (14),(28),(12)and (8)into (30),we can rewrite cov (ˆz )as cov (ˆz )≈(W T Q −1W )−1(31)where W =B −1¯SD−1GC .Since we are computing an error variance,B (19),¯S(5)and D (14)are calculated using the true (mean)value of x ,y and r i .Using (19)and (1),we can rewrite B =−diag ([d 1,d 2,...,d M ]),whered i=(x−a i)2+(y−b i)2.Then B−1¯SD−1is given by B−1¯SD−1=⎡⎢⎢⎢⎢⎢⎣a1xd1b1yd1−¯r1√x2+y2d1a2xd2b2yd2−¯r2√x2+y2d2.........a Mxd Mb Myd M−¯r M√x2+y2d M⎤⎥⎥⎥⎥⎥⎦.(32)Consequently,we obtain the entries of W as{W}i,1=x¯r i−a ix2+y2(x−a i)2+(y−b i)2x2+y2,(33){W}i,2=y¯r i −b ix2+y2(x−a i)2+(y−b i)2x2+y2.(34)where{W}i,j denotes the entry at the i th row and j th column.From this we can see that W=paring(21)and (31),it followscov(ˆz)≈J−1.(35) Then,E[(ˆx−x)2+(ˆy−y)2]≈J−11,1+J−12,2.Therefore,the variance of the estimation error is the same as the CRLB.In the following,wefirst employ an example to show that[ˆx,ˆy]obtained by TSE are Gaussian distributed with covariance matrix J−1,and then give the explanation for this phenomenon.Let the transmitter be at[0,0],target at[0.699, 4.874]and four receivers at[-1,1],[2,1],[-31.1]and[4 0].The signal travel distance variance at four receivers are [0.1000,0.1300,0.1200,0.0950]×10−4.The two dimensional probability density function(PDF)of[ˆx,ˆy]is shown in Fig.4 (a).To verify the Gaussianity of[ˆx,ˆy],the difference between the PDF of[ˆx,ˆy]and the PDF of Gaussian distribution with mean[¯x,¯y]and covariance J−1is plotted in Fig.4(b).The Gaussianity of[ˆx,ˆy]can be explained as follows.Eqn.(35)means that the covariance of thefinal estimation of target location is the FIM related to CRLB.We could further study the distribution of[e x,e y].The basic idea is that by omitting the second or high order and nonlinear errors,[e x,e y]can be written as linear function of e:1)According to(29),[e x,e y]are approximately lineartransformations ofˆΥ.2)(15)means thatˆΥis approximately a linear transfor-mation ofˆΘ.Here we could omit the nonlinear errors occurred in the estimate/calculation ofΩ.3)According to(11),ˆΘ≈¯θ2+2¯θn+n2,thus,omittingthe second order error,thus,ˆΘis approximately a linear transformation of n.4)(10)and(39)mean that n is approximately a lineartransformation of e.Here we could omit the nonlinear errors accrued in the estimate of S andΨ.Thus,we could approximately write[e x,e y]as a linear trans-formation of e,thus,[e x,e y]can be approximated as Gaussian variables.Fig.4.(a):PDF of[ˆx,ˆy]by TSE(b):difference between the PDF of[ˆx,ˆy] by TSE and PDF of Gaussian distribution with mean[¯x,¯y]and covariance J−1.Fig.5.Simulation results of TSE for thefirst configuration.VI.S IMULATION R ESULTSIn this section,wefirst compare the performance of TSE with that TDOA algorithm proposed in[16]and CRLBs.Then, we show the performance of TSE at high TOA measurement error scenario.For comparison,the performance of a Quasi-Newton iterative method[35]is shown.To verify our theoretical analysis,six different system con-figurations are simulated.The transmitter is at[0,0]for all six configurations,and the receiver locations and error variances are listed in Table I.Figures5,6and7show simulation results comparing the distance to the target(Configuration1vs. Configuration2),the receiver separation(Configuration3vs. Configuration4)and the number of receivers(Configuration5 vs.Configuration6),respectively4.In eachfigure,10000trails are simulated and the estimation variance of TSE estimate is compared with the CRLB of TDOA and TOA based localization schemes.For comparison,the simulation results of error variance of the TDOA method proposed in[16]are also drawn in eachfigure.It can be observed that1)The localization error of TSE can closely approach theCRLB of TOA based positioning algorithms.4During the simulations,only one iteration is used for the calculation of B(19).。
- 1、下载文档前请自行甄别文档内容的完整性,平台不提供额外的编辑、内容补充、找答案等附加服务。
- 2、"仅部分预览"的文档,不可在线预览部分如存在完整性等问题,可反馈申请退款(可完整预览的文档不适用该条件!)。
- 3、如文档侵犯您的权益,请联系客服反馈,我们会尽快为您处理(人工客服工作时间:9:00-18:30)。
University of Nevada,RenoIntegrating Minimalistic Localization and Navigation for People with Visual ImpairmentsA thesis submitted in partial fulfillment of therequirements for the degree of Master of Sciencewith a major in Computer Science.byIlias ApostolopoulosDr.Kostas E.Bekris,Thesis AdvisorMay2011We recommend that the thesis prepared under our supervision byIlias ApostolopoulosentitledIntegrating Minimalistic Localization and Navigation for People withVisual Impairmentsbe accepted in partial fulfillment of the requirements for the degree ofMASTER OF SCIENCEDr.Kostas E.Bekris,Ph.D.,AdvisorDr.Eelke Folmer,Ph.D.,Committee MemberDr.Dwight Egbert,Ph.D.,Committee MemberDr.Daniel Cook,Ph.D.,Graduate School RepresentativeMarsha H.Read,Ph.D.,Associate Dean,Graduate SchoolMay2011AbstractIndoor localization and navigation systems for individuals with visual impair-ments(VI)typically rely upon extensive augmentation of the physical space or ex-pensive sensors;thus,few systems have been adopted.This work describes a system able to guide people with VI through buildings using inexpensive sensors,such as accelerometers,which are available in portable devices like smart phones.This ap-proach introduces some challenges due to the limited computational power of the portable devices and the highly erroneous sensors.The method takes advantage of feedback from the human user,who confirms the presence of landmarks.The system calculates the location of the user in real time and uses it to provide audio instructions on how to reach the desired destination.Afirst set of experiments suggested that the accuracy of the localization depends on the type of directions provided and the availability of good transition and observation models that describe the user’s behav-ior.During this initial set of experiments,the system was not executed in real time so the approach had to be improved.Towards an improved version of the method, a significant amount of computation was transferred offline in order to speed up the system’s online execution.Inspired by results in multi-model estimation,this work employs multiple particlefilters,where each one uses a different assumption for the user’s average step length.This helps to adaptively estimate the value of this pa-rameter on thefly.The system simultaneously estimates the step length of the user, as it varies between different people,from path to path,and during the execution of the path.Experiments are presented that evaluate the accuracy of the location estimation process and of the integrated direction provision method.Sighted people, that were blindfolded,participated in these experiments.Acknowledgements This work was supported by internal funds by UNR.ContentsAbstract i Acknowledgements ii List of Figures iv List of Tables v 1Introduction11.1Motivation (1)1.2Objective (2)1.3Challenges (2)1.4System Overview (3)2Background62.1Navigation and Cognitive Mapping (6)2.2Indoor Navigation Systems for Users with VI (7)2.2.1Localization (8)2.2.2Path planning (8)2.2.3Providing location information (8)2.2.4Interaction (9)2.3Localization Techniques (9)2.3.1Dead-Reckoning (9)2.3.2Beacon-based (9)2.3.3Sensor-based (9)2.4Bayesian methods (10)2.5Path planning (11)2.6Multi-model representation (11)3Initial Approach133.1Objectives (13)3.2High-level operation (13)3.2.1Direction Provision (14)3.3Localization (16)3.3.1Particle Filter (18)iv3.3.2Transition Model (18)3.3.3Observation Model (19)3.3.4Sampling (19)3.4Experiments (20)3.4.1Setup (20)3.4.2Participants (21)3.4.3Ground Truth (21)3.4.4Parameters (22)3.4.5Success Ratio of Direction Provision (22)3.4.6Localization Accuracy (23)4Improved Approach264.1Overview (26)4.2Offline Process (26)4.3Direction Provision (27)4.4Localization (28)4.4.1Transition Model (28)4.4.2Observation Model (29)4.4.3Sampling (29)4.5Experiments (30)4.5.1Setup (30)4.5.2Participants (30)4.5.3Ground Truth (32)4.5.4Parameters (32)4.5.5Success Ratio of Direction Provision (33)4.5.6Offcourse correction (34)4.5.7Computational overhead (35)5Conclusion375.1Summary (37)5.2Future work (38)List of Figures1.1An individual with visual impairments testing the system (2)1.2A communication graph between the components of the system (5)3.1The map of the environment and the paths traversed during the ex-perimental section (15)3.2An example path starting from255andfinishing at231 (16)3.3An illustration of the particle reseting process.The particle’s positionis moved close to the nearest landmark of the type that the user confirmed203.4a)Ground truth vs.dead-reckoning vs.particlefiltering.b)Error graph..244.1The map of thefirstfloor (31)4.2The map of the secondfloor (31)4.3A graph showing the localization error during the execution of a path.The different lines represent the distance of the different particlefiltersfrom the actual location of the user (35)List of Tables1.1Examples of model parameters (3)2.1Indoor Navigation Systems for Users with VI (7)3.1Table of parameters used in the case studies (22)3.2Average distance between destination and the user’s position uponcompletion(m) (23)3.3Average path duration(sec) (23)3.4Average error of dead reckoning infinal location(m) (24)3.5Average error of the proposed interactive localization process(m) (25)3.6Standard deviation for the error of the proposed interactive localizationprocess(m) (25)4.1Distance between destination and the user’s position upon completion(m) (33)4.2Distance between ground truth and destination(m) (34)4.3Profiling data(msec) (36)Chapter1IntroductionThe motivation of this work is presented here,along with the objectives,the chal-lenges faced and a high-level system overview.The following chapters include some background work,a description of an initial approach to the problem of guiding peo-ple with visual impairments,an improved approach based on the challenges faced during the initial implementation and,finally,a discussion section about the overall results and future work.1.1MotivationSighted people can navigate environments by primarily relying upon their visual senses tofind their way.Individuals with visual impairments(VI)have to rely upon their compensatory senses(e.g.touch,sound)for way-finding,resulting in reduced mobility.Unfamiliar environments are especially challenging as it is difficult to build a mental map of the surroundings using non-visual feedback[45].To increase the mobility of individuals with VI various navigation systems have been developed.While there are many solutions for outdoor navigation systems,in-door alternatives are more difficult to develop.Outdoor navigation systems typically use GPS,however GPS signals cannot be received in indoor environments.Exist-ing indoor navigation systems typically rely upon augmenting physical infrastructure with identifiers such as RFID tags[41,10,5].While RFID tags might be cheap, a large amount of them is required to cover a whole building.Often,RFID tags are installed under carpets on thefloor.Although this is possible,hallways or large open spaces with concretefloor or tiles render the installation of these tags more difficult.Other solutions employ laser sensors[30,29]or cameras[81].While these solutions often lead to sophisticated algorithms,they can be expensive,cumbersome, and computationally demanding alternatives.Figure1.1:An individual with visual impairments testing the system.1.2ObjectiveThis research describes an inexpensive solution that does not require physical infras-tructure and depends on cheap,light-weight sensors,such as an accelerometer and a compass,that are already available on popular devices,such as smart phones.Instead of depending on a physical infrastructure or expensive and cumbersome sensors,the system presented here only needs a virtual infrastructure,that can be created and updated very fast with low cost,and lightweight inexpensive sensors that can found in an everyday handheld device.1.3ChallengesThe proposed system has to deal with uncertainty at multiple levels of its operation. Uncertainty arises from:•The behavior of the user:e.g.,how quickly does the person move,how accuratelydoes one person turn when instructed to do so,how good is the person at identifying landmarks.For instance,while users can easily identify landmarks of different types,they cannot readily distinguish landmarks of the same type.When a user confirms a door,and there are a number of doors close one to each other,it is possible that the user did not confirm the correct one.The system has to take into consideration the possibility that the user confirmed an adjacent landmark.•The environment:The model of the environment may lead to an uncertain representation,as the annotation of the map,such as the actual location or the type of the landmarks,might be incorrect.•The sensors:Sensors used in mobile phones usually have low accuracy.The error due to these sensors must also be taken into consideration.The core of the research effort regarding the localization component is devoted to the definition and online learning of appropriate observation and transition models for individual users.Table1.1provides examples of potential parameters for these models.It is important for the models to be able to differentiate between users.This is especially important for this application,as different users will also have different types and degrees of visual impairments.Transition Model Observation ModelAverage Step Length Landmark Identification AccuracyStep Detection Accuracy Distance from Landmarkupon ConfirmationTurning Accuracy Confirmation EfficiencyTable1.1:Examples of model parameters1.4System OverviewTactile landmarks,such as doors,hallway intersections orfloor transitions,play an important role in the cognitive mapping of indoor spaces by users with VI[36,9].By incorporating the unique sensing capabilities of users with VI,the system aims to provide guidance in spaces for which the user does not have a prior cognitive map. The system assumes the availability of a2D map with addressing information(room numbers)and landmark locations.Then,it follows these steps:1.A user specifies a start and a destination room number to travel to.2.Given landmarks identifiable by users with VI on the map,the system computesthe shortest path using A*and identifies landmarks along the path.3.The user presses a button on the phone after successfully executing each direc-tion.4.Directions are provided iteratively upon the confirmation of each landmark,orwhen the user is presumed to be lost.The phone’s built-in speaker is used for the transmission of the direction.Figure1.2lists a high-level overview of the four different components of the sys-tem:(1)the cyber-representation component stores annotated models of indoor envi-ronments;(2)the localization component provides a location estimate of the user with VI;(3)the direction provision component provides directions to a user specified loca-tion;and(4)the interface component interacts with the user.All components have physical models of the users with VI with the exception of the cyber-representation component which explicitly models sighted users annotating the models.The landmarks used from the system are features that can be found in most buildings.Doors,hallway intersections,floor transitions,water coolers,ramps,stairs and elevators are incorporated to guide the user around the building.These landmarks are easily recognizable from users with VI by using touch and sound,thus creating no need for additional physical infrastructure.This research proposes a system that takes into consideration the sources of uncertainty previously mentioned and provides an integration of localization and path planning primitives.Multiple particlefilters are employed in order to deal with theFigure1.2:A communication graph between the components of the system. highly non-linear process of localization as well as learning and updating a model of the user’s behavior.To provide directions to the user,a path is created from start to goal using the A*algorithm.Then,turn-to-turn directions are provided based on the location estimation of the user provided by localization.Results show that this system is capable of successfully locating and guiding the user to the desired destination when a path with unique landmarks is provided.Chapter2BackgroundThere is a lot of work related to navigation systems for people with visual impair-ments,localization techniques,path planning,bayesian methods and multi-model estimation.2.1Navigation and Cognitive MappingNavigation relies on a combination of mobility and orientation skills[21].People employ either path integration,where they orient themselves relative to a starting position using proprioceptive date,or landmark-based navigation,where they rely upon perceptual cues together with an external or cognitive map[53,22,51].Path integration allows for exploring unfamiliar environments in which users may build a cognitive map by observing landmarks[82,53].Studies show small difference in path integration ability between sighted and individuals with VI[51],but cognitive mapping is significantly slower for users with VI[46,71].Cognitive mapping of outdoor environments has been extensively studied[21,68,28]and has reported to primarily rely upon landmarks that can be recognized by touch[71]in the users’immediate space[11],such as curbs or traffic lights.Pedestrian navigation systems for sighted users have been developed where users are localized by reporting visual landmarks,such as escalators[56]or churches[32].Recently,the cognitive mapping of indoor spaces by people with VI has been studied[36,77,26].Tactile landmarks easily sensed through touch,such as doors,hallway intersections andfloor transitions, play an important role in the cognitive mapping of indoor spaces[36,83].Sounds and smells also play a minor role[83].The use of virtual environments has been shown to aid cognitive mapping of users with VI[46,71,57].Table2.1:Indoor Navigation Systems for Users with VIAuthors Localization Directions Information Feedback 1998Sonnenblick[76]IR-Room name Speech 2001May[54]barcode--Braille2002Ross&Blasch [68]IR,RFID--Audio,Speech,Hap-tic2003Coroama andRothenbacher[17]RF Objects Central-2004Ran et al[62]Ultrasound Objects Room layout,objects SpeechHub et al[33]Wifi,Camera-Object name Speech Amemiya et al[5]RFID--Haptic(braille)2005Ross&Light-man[69]IR,RF,Au-dioLocations Objects Speech,brailleWillis&Helal[85]RFID Objects Room layout,ob-jects.Haptic(braille)2006Gifford et al[25]RFID-Room layout,objects Speech2008Bessho et al[10]RFID,IR Stations Layout of Station SpeechRiehle et al[64]WiFi Rooms-Speech Rajamaki et al[61]WiFi Rooms-SpeechD’Atri et al[18]RFID--Speech2.2Indoor Navigation Systems for Users with VINavigation systems for users with VI aim to allow safe navigation in unfamiliar en-vironments.This includes locating the user and optionally providing directions toa desired destination and/or describing the surroundings,such as obstacles or land-marks.Navigation systems can be differentiated into outdoor and indoor systems.Outdoor systems[52,69,68]typically use GPS for localization.Relatively few indoornavigation systems exist,as GPS signals cannot be received indoors,and alternativelocalization techniques must be used.Table2.1provides an overview of existing in-door navigation systems for VI and lists the specific techniques used for localization,path planning,providing location information and interaction with the system.By augmenting the physical infrastructure with identifiers,people can be localized when an identifier is sensed.Different technologies have been used,such as infrared (IR)[76,68,69],wireless[34,33,64,61],ultrasound[62],or radio frequency identifier (RFID)tags[5,68,25,10,18].There are limitations in this approach,as IR requires line of sight and the environment or the user may interfere with RFID readings[68]. Wireless based systems often suffer from multi-path effects[85]or cannot be used in certain spaces,e.g.,hospitals.A number of vision-based systems require little physical augmentation[33,58,35].Some of them may utilize wireless signals or RFID tags,or a virtual environment representation.There are also approaches that utilize magnetic compasses[78].Magnetic compasses are not very reliable when used in an indoors environment due to the noise created by the infrastructure.Although, there is a work that prerecords the signatures of different disturbances in a building and tries to match these signatures later in order to get a localization estimation.A recent approach uses a laser-rangefinder combined with odometry readings[31]. Relative to the last methods,the proposed approach aims to further reduce sensing requirements and avoid any environment augmentation with identifiers.2.2.2Path planningOnly three systems[62,69,10]provide global path planning where paths are com-puted using A*[70]and where the system updates the user’s position dynamically.2.2.3Providing location informationInformation on a user’s location varies from providing the name of a room[76]to detailed descriptions of the room’s layout,such as objects in that room[25,62]. Information is either stored locally[76,25],centrally[62,5,33,64]or distributedly [85,10].Users receive feedback using audio such as speech[76,25,10]or audio cues[68]to haptic solutions such as a tapping interface[68],pager belts[85]or haptic gloves[5].2.3Localization TechniquesCertain navigation devices focus on local hazard detection to provide obstacle-avoidance capabilities to users with VI[72,86].Most navigation systems,however,are able to locate the user and provide directions to a user-specified destination.Outdoor navi-gation systems[52,67]mainly use GPS to localize the user.Indoor systems cannot use GPS signals,as these are blocked by buildings.To surpass this issue,alternative localization techniques have been developed.2.3.1Dead-ReckoningDead-Reckoning techniques integrate measurements of the human’s motion.Ac-celerometers[14]and radar measurements[84]have been used for this purpose.With-out any external reference,however,the error in dead-reckoning grows unbounded.2.3.2Beacon-basedBeacon-based approaches augment the physical space with identifiers.Such beacons could be retro-reflective digital signs detected by a camera[81],infrared[67]or ultra-sound identifiers[62].A popular solution involves RFID tags[41,10,5].Nevertheless, locating identifiers may be hard,as beacons may require line of sight or close prox-imity to the human.Other beacons,such as wireless nodes[64,44,43],suffer from multi-path effects or interference.Another drawback is the significant time and cost spent installing and calibrating beacons.2.3.3Sensor-basedSensor-based solutions employ sensors,such as cameras[40],that can detect pre-existing features of indoor spaces,such as walls or doors.For instance,a multi-camerarig has been developed to estimate the6DOF pose of people with VI[20].A different camera system matches physical objects with objects in a virtual representation of the space[33].Nevertheless,cameras require good lighting conditions,and may impose a computational cost prohibitive for portable devices.An alternative makes use of a 2D laser scanner[30,29].This method achieves3D pose estimation by integrating data from an IMU unit,the laser scanner,and knowledge of the3D structure of the space.While laser scanners can robustly detect low-level features,this method has led to sophisticated algorithms for3D pose estimation and it depends on relatively expensive and heavy sensors.The proposed approach is also a sensor-based solution.It employs the user as a sensor together with information from light-weight,affordable devices,such as a pedometer.These sensors are available on smart phones and it is interesting to study the feasibility of using such popular devices to(i)interact effectively with a user with VI;and(ii)run in real-time localization primitives given their limited resources.To achieve this objective under the minimalistic and noisy nature of the available sensors, this work utilizes probabilistic tools that have been shown to be effective in robotics and evaluates their efficiency for different forms of direction provision.2.4Bayesian methodsBayesian methods for localization work incrementally,where given the previous belief about the agent’s location,the new belief is computed using the latest displacement and sensor reading.A transition model is used in order to advance the movement of the system and an observation model in order to compare the sensor readings with the state estimation.An important issue is how to represent and store the belief distribution.One method is the Extended Kalmanfilter(EKF)[37,75],which assumes normal distributions.Its purpose is to use measurements observed over time,containing noise(random variations)and other inaccuracies,and produce values that tend to be closer to the true values of the measurements and their associated calculated values.While Kalmanfilters provide a compact representation and returnthe optimum estimate under certain assumptions,a normal distribution may not be a good model,especially for multi-modal distributions.An alternative is to use particle filters[27,80,47,63,60,79],which sample estimates of the agent’s state.Particle filters keep a number of different estimations called particles.Each particle holds a state estimation and a weight.Particlefilters are able to represent multi-modal distributions at the expense of increased computation.Such distributions arise often in this research’s application,such as when a door is confirmed,where the belief increases in front of all of the doors in the vicinity of the last estimate.Thus,particle filters appear as an appropriate solution.This research shows that it is possible to achieve a sufficient,real-time solution with a particlefilter.2.5Path planningPopular path planning techniques include search methods,such as A*on grid-based maps[59,66,70],the visibility graph[24,19],the Voronoi graph or the medial axis [48,8],cell decomposition techniques[16,3]or potentialfield approaches[39,65]. Recent work has focused on solving complex high-dimensional challenges,giving rise to sampling-based methods[38,4,12,73,23].These methods sample collision-free configurations in order to construct a roadmap,a graph representing the connectivity of the obstacle-free space.While these methods are not complete,the probability the roadmap reflects the obstacle-free space connectivity increases exponentially fast to 1[42].Planning under uncertainty can be modeled by Partially Observable Markov Decision Process(POMDP)[50,13].2.6Multi-model representationThe user’s step length changes dynamically and these changes have to be taken into consideration during path execution.To achieve this,this project is building upon work in multi-model state estimation[1,55,49,74].Multi-model estimation is com-monly used to calculate both the state and the model of the system,when it is changing[15].Multi-model estimation systems though are also used to track thechanges in the environment itself[2].In thefirst case,multi-model estimation is commonly used to both track the location of the system and the value of a system variable that might be discrete[15]or continuous[49].In the second case,the system tries to determine the noise due to the environment.By doing so,the system can dynamically adapt in an environment with random properties[2].Most multi-model estimation systems use multiple Kalman filters to determine the system’s model.Multi-model estimations utilize multiple bayesian methods in order to estimate a model variable.The bayesian methods can be either multiple Extended Kalman filters or multiple particlefilters.Each method holds a unique value of a variable that needs to be approximated along with localization.After a few transitions and observations,the estimations of the variable converge to a value if the system model is stable.In case the system model changes,the variable estimations adopt dynamically to the correct values in order to match with the current observations of the system.The proposed system is trying to determine the system model by estimating the user’s step length.To achieve multi-model estimations,the system is maintaining multiple particlefilters,where each one has a different estimation for the step length of the user.Chapter3Initial ApproachAn initial approach was implemented to address the problem of indoor navigation. Experiments were executed to test this approach localization accuracy and the effect of different types of directions to the success rate and the execution time.3.1ObjectivesThe goal of this initial approach was to test if it possible to successfully localize the user and decide which type of directions is better based on accuracy and speed. This initial approach is not executed on the phone.Sensing data from the users are gathered during the execution of the experiments and are then processed offline to determine the location of the user.3.2High-level operationTactile landmarks,such as doors,intersections orfloor transitions,play an impor-tant role in the cognitive mapping of indoor spaces by users with VI[36,9].By incorporating the unique sensing capabilities of users with VI,the system aims to provide guidance in spaces for which the user does not have a prior cognitive map. The system assumes the availability of a2D map with addressing information(room numbers)and landmark locations.Then,it follows these steps:1.A user specifies a start and destination room number to travel to.2.The system computes the shortest path using A*andfinds landmarks along thepath.3.Directions are provided iteratively upon completion through the phone’s built-in speaker.The user presses a button on the phone after successfully executing each direction.3.2.1Direction ProvisionThe type of directions significantly effects the efficiency and reliability of navigation. Reliability is high when the user is required to confirm the presence of every single landmark along a path but this is detrimental to efficiency.Conversely,when the system solely relies on odometry,users have a smaller cognitive load but a high chance of getting lost,due to the inherent propagation of errors associated with dead reckoning.To gain a better insight in these tradeoffs two different types of direction provisions were tested:ndmark based directions,e.g.,“move forward until you reach a hallway onyour left”.No distance to a landmark is provided.Directions were subdivided based on the maximum distance between landmarks:(a)30ft,(b)50ft and(c) unlimited.Wall following and door counting strategies were employed for the first2cases(i.e.,“Follow the wall on your left until you reach the third door”).For the last case no wall following or door counting strategies were used for directions leading to a hallway.2.Metric based directions,e.g.,“Walk x steps until your reach a landmark onyour left/right”.Within this approach the maximum distance between land-marks was also varied with30ft,50ft and unlimited.For example:“Walk23 steps until you reach a door on your right”for the30ft limit.Both types of instructions contain a second type of direction with an action on a landmark,for example,“Turn right into the hallway”.The directions provided to the user were hardcoded into the system for each path.This initial approach did not generate automatically the instructions.Instead, the paths were predefined and the ability of the users to follow these instructions was tested.Here are some examples of instructions of different types generated to guide the user along the path in Figure3.4.。