Preliminary Study of Analytic Sensitivities for CFD Codes Through Automatic Differentiation
sensitivity analysis学术语言-概述说明以及解释

sensitivity analysis学术语言-概述说明以及解释1.引言1.1 概述在学术研究和实践中,sensitivity analysis(敏感性分析)是一种重要的方法,用于评估模型输出结果对输入参数变化的敏感程度。
通过分析模型输入参数的变化对输出结果的影响,可以帮助研究人员更好地理解模型的稳定性和可靠性。
敏感性分析不仅可以帮助我们识别哪些参数对模型的结果影响最大,还可以帮助我们优化模型,提高模型的预测能力和可靠性。
本文将深入探讨sensitivity analysis的概念、在学术研究中的重要性以及方法及应用。
通过对这些内容的综合讨论,读者将能够了解敏感性分析在科研领域中的广泛应用和意义。
1.2 文章结构文章结构部分的内容可以按照以下内容进行编写:文章结构部分旨在介绍整篇文章的组织结构,为读者提供一个整体的概览。
本文共分为三大部分:引言、正文和结论。
在引言部分,我们将首先对sensitivity analysis的概念进行概述,说明其在学术研究中的重要性,并阐述本文的目的。
接着,正文部分将对sensitivity analysis的概念进行详细解释,阐述其在学术研究中的重要性,并介绍其方法及应用。
最后,在结论部分,我们将总结文章的主要内容,展望sensitivity analysis未来的发展方向,以及得出结论。
通过这样明确的结构安排,读者可以更清晰地了解本文的主要内容和逻辑顺序,有助于他们更好地理解和消化文章内容。
1.3 目的本文的主要目的在于探讨sensitivity analysis在学术研究中的重要性和应用。
通过对sensitivity analysis概念、方法及其在学术研究中的具体应用进行深入分析,希望能够进一步加深对该分析方法的理解,为研究人员提供更好的参考和指导。
同时,也旨在探讨sensitivity analysis在未来的发展方向和应用前景,为学术界提供启示和启发。
潜在类别模型在试题分析中的应用
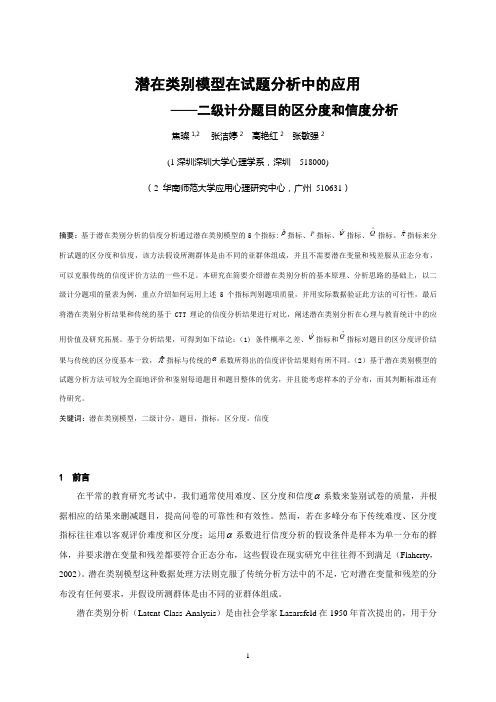
潜在类别模型在试题分析中的应用——二级计分题目的区分度和信度分析焦璨1,2 张洁婷2高艳红2张敏强2(1深圳深圳大学心理学系,深圳518000)(2 华南师范大学应用心理研究中心,广州510631)摘要:基于潜在类别分析的信度分析通过潜在类别模型的5个指标:ρˆ指标、P指标、ψˆ指标、Qˆ指标、πˆ指标来分析试题的区分度和信度,该方法假设所测群体是由不同的亚群体组成,并且不需要潜在变量和残差服从正态分布,可以克服传统的信度评价方法的一些不足。
本研究在简要介绍潜在类别分析的基本原理、分析思路的基础上,以二级计分题项的量表为例,重点介绍如何运用上述5个指标判别题项质量,并用实际数据验证此方法的可行性,最后将潜在类别分析结果和传统的基于CTT理论的信度分析结果进行对比,阐述潜在类别分析在心理与教育统计中的应用价值及研究拓展。
基于分析结果,可得到如下结论:(1) 条件概率之差、ˆψ指标和ˆQ指标对题目的区分度评价结果与传统的区分度基本一致,ˆπ指标与传统的α系数所得出的信度评价结果则有所不同。
(2)基于潜在类别模型的试题分析方法可较为全面地评价和鉴别每道题目和题目整体的优劣,并且能考虑样本的子分布,而其判断标准还有待研究。
关键词:潜在类别模型,二级计分,题目,指标,区分度,信度1 前言在平常的教育研究考试中,我们通常使用难度、区分度和信度α系数来鉴别试卷的质量,并根据相应的结果来删减题目,提高问卷的可靠性和有效性。
然而,若在多峰分布下传统难度、区分度指标往往难以客观评价难度和区分度;运用α系数进行信度分析的假设条件是样本为单一分布的群体,并要求潜在变量和残差都要符合正态分布,这些假设在现实研究中往往得不到满足(Flaherty,2002)。
潜在类别模型这种数据处理方法则克服了传统分析方法中的不足,它对潜在变量和残差的分布没有任何要求,并假设所测群体是由不同的亚群体组成。
潜在类别分析(Latent Class Analysis)是由社会学家Lazarsfeld在1950年首次提出的,用于分析态度量表中潜在变量的一种方法(Lazarsfeld & Henry,1986)。
preliminary study 和 pilot study 和 pretest

preliminary study 和pilot study 和pretest "Preliminary study"、"pilot study" 和"pretest" 都是研究设计和方法学上常用的术语,用于描述研究计划中的初步阶段。
以下是它们的一些基本概念:
1.Preliminary Study(初步研究):
•定义:Preliminary study 是在进行正式研究之前进行的一个阶段,用于获取关于研究主题的初步信息。
•目的:了解研究领域的背景,确定研究问题,收集初步数据,评估可行性等。
•特点:可能包括文献回顾、小规模调查、初步数据分析等。
2.Pilot Study(试点研究):
•定义:Pilot study 是在主要研究之前进行的小规模研究,用于测试研究设计、方法和工具的可行性。
•目的:确定研究设计的可行性、检测潜在问题、确定样本大小、测试数据收集工具等。
•特点:通常包含实际实验或调查,但规模较小,其结果不会被纳入最终分析。
3.Pretest(预测试):
•定义:Pretest 是在正式实验或调查之前对工具、问卷或实验条件进行的小规模测试。
•目的:评估测量工具的有效性、检查实验条件是否正常,
发现潜在问题。
•特点:通常关注于工具和程序的细节,以确保它们能够提供准确的测量和实验结果。
这些阶段的目标是确保主要研究的成功进行。
通过初步研究,研究者可以更好地了解研究领域,通过试点研究可以优化研究设计和方法,而预测试则有助于确保测量工具和程序的有效性。
survey--on sentiment detection of reviews
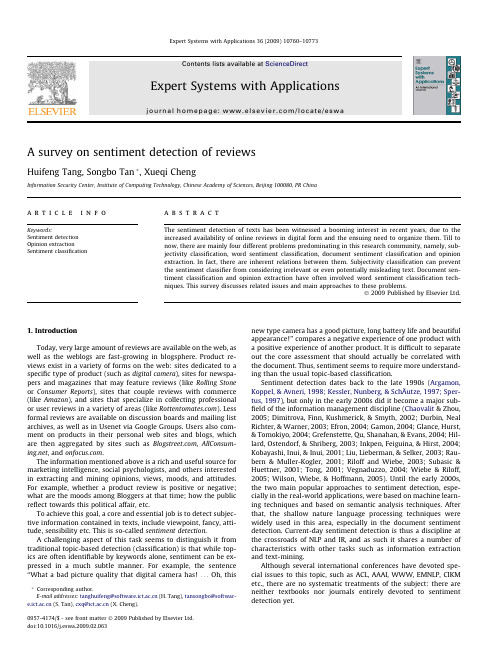
A survey on sentiment detection of reviewsHuifeng Tang,Songbo Tan *,Xueqi ChengInformation Security Center,Institute of Computing Technology,Chinese Academy of Sciences,Beijing 100080,PR Chinaa r t i c l e i n f o Keywords:Sentiment detection Opinion extractionSentiment classificationa b s t r a c tThe sentiment detection of texts has been witnessed a booming interest in recent years,due to the increased availability of online reviews in digital form and the ensuing need to organize them.Till to now,there are mainly four different problems predominating in this research community,namely,sub-jectivity classification,word sentiment classification,document sentiment classification and opinion extraction.In fact,there are inherent relations between them.Subjectivity classification can prevent the sentiment classifier from considering irrelevant or even potentially misleading text.Document sen-timent classification and opinion extraction have often involved word sentiment classification tech-niques.This survey discusses related issues and main approaches to these problems.Ó2009Published by Elsevier Ltd.1.IntroductionToday,very large amount of reviews are available on the web,as well as the weblogs are fast-growing in blogsphere.Product re-views exist in a variety of forms on the web:sites dedicated to a specific type of product (such as digital camera ),sites for newspa-pers and magazines that may feature reviews (like Rolling Stone or Consumer Reports ),sites that couple reviews with commerce (like Amazon ),and sites that specialize in collecting professional or user reviews in a variety of areas (like ).Less formal reviews are available on discussion boards and mailing list archives,as well as in Usenet via Google ers also com-ment on products in their personal web sites and blogs,which are then aggregated by sites such as , ,and .The information mentioned above is a rich and useful source for marketing intelligence,social psychologists,and others interested in extracting and mining opinions,views,moods,and attitudes.For example,whether a product review is positive or negative;what are the moods among Bloggers at that time;how the public reflect towards this political affair,etc.To achieve this goal,a core and essential job is to detect subjec-tive information contained in texts,include viewpoint,fancy,atti-tude,sensibility etc.This is so-called sentiment detection .A challenging aspect of this task seems to distinguish it from traditional topic-based detection (classification)is that while top-ics are often identifiable by keywords alone,sentiment can be ex-pressed in a much subtle manner.For example,the sentence ‘‘What a bad picture quality that digital camera has!...Oh,thisnew type camera has a good picture,long battery life and beautiful appearance!”compares a negative experience of one product with a positive experience of another product.It is difficult to separate out the core assessment that should actually be correlated with the document.Thus,sentiment seems to require more understand-ing than the usual topic-based classification.Sentiment detection dates back to the late 1990s (Argamon,Koppel,&Avneri,1998;Kessler,Nunberg,&SchÄutze,1997;Sper-tus,1997),but only in the early 2000s did it become a major sub-field of the information management discipline (Chaovalit &Zhou,2005;Dimitrova,Finn,Kushmerick,&Smyth,2002;Durbin,Neal Richter,&Warner,2003;Efron,2004;Gamon,2004;Glance,Hurst,&Tomokiyo,2004;Grefenstette,Qu,Shanahan,&Evans,2004;Hil-lard,Ostendorf,&Shriberg,2003;Inkpen,Feiguina,&Hirst,2004;Kobayashi,Inui,&Inui,2001;Liu,Lieberman,&Selker,2003;Rau-bern &Muller-Kogler,2001;Riloff and Wiebe,2003;Subasic &Huettner,2001;Tong,2001;Vegnaduzzo,2004;Wiebe &Riloff,2005;Wilson,Wiebe,&Hoffmann,2005).Until the early 2000s,the two main popular approaches to sentiment detection,espe-cially in the real-world applications,were based on machine learn-ing techniques and based on semantic analysis techniques.After that,the shallow nature language processing techniques were widely used in this area,especially in the document sentiment detection.Current-day sentiment detection is thus a discipline at the crossroads of NLP and IR,and as such it shares a number of characteristics with other tasks such as information extraction and text-mining.Although several international conferences have devoted spe-cial issues to this topic,such as ACL,AAAI,WWW,EMNLP,CIKM etc.,there are no systematic treatments of the subject:there are neither textbooks nor journals entirely devoted to sentiment detection yet.0957-4174/$-see front matter Ó2009Published by Elsevier Ltd.doi:10.1016/j.eswa.2009.02.063*Corresponding author.E-mail addresses:tanghuifeng@ (H.Tang),tansongbo@ (S.Tan),cxq@ (X.Cheng).Expert Systems with Applications 36(2009)10760–10773Contents lists available at ScienceDirectExpert Systems with Applicationsjournal homepage:/locate/eswaThis paperfirst introduces the definitions of several problems that pertain to sentiment detection.Then we present some appli-cations of sentiment detection.Section4discusses the subjectivity classification problem.Section5introduces semantic orientation method.The sixth section examines the effectiveness of applying machine learning techniques to document sentiment classification. The seventh section discusses opinion extraction problem.The eighth part talks about evaluation of sentiment st sec-tion concludes with challenges and discussion of future work.2.Sentiment detection2.1.Subjectivity classificationSubjectivity in natural language refers to aspects of language used to express opinions and evaluations(Wiebe,1994).Subjectiv-ity classification is stated as follows:Let S={s1,...,s n}be a set of sentences in document D.The problem of subjectivity classification is to distinguish sentences used to present opinions and other forms of subjectivity(subjective sentences set S s)from sentences used to objectively present factual information(objective sen-tences set S o),where S s[S o=S.This task is especially relevant for news reporting and Internet forums,in which opinions of various agents are expressed.2.2.Sentiment classificationSentiment classification includes two kinds of classification forms,i.e.,binary sentiment classification and multi-class senti-ment classification.Given a document set D={d1,...,d n},and a pre-defined categories set C={positive,negative},binary senti-ment classification is to classify each d i in D,with a label expressed in C.If we set C*={strong positive,positive,neutral,negative,strong negative}and classify each d i in D with a label in C*,the problem changes to multi-class sentiment classification.Most prior work on learning to identify sentiment has focused on the binary distinction of positive vs.negative.But it is often helpful to have more information than this binary distinction pro-vides,especially if one is ranking items by recommendation or comparing several reviewers’opinions.Koppel and Schler(2005a, 2005b)show that it is crucial to use neutral examples in learning polarity for a variety of reasons.Learning from negative and posi-tive examples alone will not permit accurate classification of neu-tral examples.Moreover,the use of neutral training examples in learning facilitates better distinction between positive and nega-tive examples.3.Applications of sentiment detectionIn this section,we will expound some rising applications of sen-timent detection.3.1.Products comparisonIt is a common practice for online merchants to ask their cus-tomers to review the products that they have purchased.With more and more people using the Web to express opinions,the number of reviews that a product receives grows rapidly.Most of the researches about these reviews were focused on automatically classifying the products into‘‘recommended”or‘‘not recom-mended”(Pang,Lee,&Vaithyanathan,2002;Ranjan Das&Chen, 2001;Terveen,Hill,Amento,McDonald,&Creter,1997).But every product has several features,in which maybe only part of them people are interested.Moreover,a product has shortcomings in one aspect,probably has merits in another place(Morinaga,Yamanishi,Tateishi,&Fukushima,2002;Taboada,Gillies,&McFe-tridge,2006).To analysis the online reviews and bring forward a visual man-ner to compare consumers’opinions of different products,i.e., merely with a single glance the user can clearly see the advantages and weaknesses of each product in the minds of consumers.For a potential customer,he/she can see a visual side-by-side and fea-ture-by-feature comparison of consumer opinions on these prod-ucts,which helps him/her to decide which product to buy.For a product manufacturer,the comparison enables it to easily gather marketing intelligence and product benchmarking information.Liu,Hu,and Cheng(2005)proposed a novel framework for ana-lyzing and comparing consumer opinions of competing products.A prototype system called Opinion Observer is implemented.To en-able the visualization,two tasks were performed:(1)Identifying product features that customers have expressed their opinions on,based on language pattern mining techniques.Such features form the basis for the comparison.(2)For each feature,identifying whether the opinion from each reviewer is positive or negative,if any.Different users can visualize and compare opinions of different products using a user interface.The user simply chooses the prod-ucts that he/she wishes to compare and the system then retrieves the analyzed results of these products and displays them in the interface.3.2.Opinion summarizationThe number of online reviews that a product receives grows rapidly,especially for some popular products.Furthermore,many reviews are long and have only a few sentences containing opin-ions on the product.This makes it hard for a potential customer to read them to make an informed decision on whether to purchase the product.The large number of reviews also makes it hard for product manufacturers to keep track of customer opinions of their products because many merchant sites may sell their products,and the manufacturer may produce many kinds of products.Opinion summarization(Ku,Lee,Wu,&Chen,2005;Philip et al., 2004)summarizes opinions of articles by telling sentiment polari-ties,degree and the correlated events.With opinion summariza-tion,a customer can easily see how the existing customers feel about a product,and the product manufacturer can get the reason why different stands people like it or what they complain about.Hu and Liu(2004a,2004b)conduct a work like that:Given a set of customer reviews of a particular product,the task involves three subtasks:(1)identifying features of the product that customers have expressed their opinions on(called product features);(2) for each feature,identifying review sentences that give positive or negative opinions;and(3)producing a summary using the dis-covered information.Ku,Liang,and Chen(2006)investigated both news and web blog articles.In their research,TREC,NTCIR and articles collected from web blogs serve as the information sources for opinion extraction.Documents related to the issue of animal cloning are selected as the experimental materials.Algorithms for opinion extraction at word,sentence and document level are proposed. The issue of relevant sentence selection is discussed,and then top-ical and opinionated information are summarized.Opinion sum-marizations are visualized by representative sentences.Finally, an opinionated curve showing supportive and non-supportive de-gree along the timeline is illustrated by an opinion tracking system.3.3.Opinion reason miningIn opinion analysis area,finding the polarity of opinions or aggregating and quantifying degree assessment of opinionsH.Tang et al./Expert Systems with Applications36(2009)10760–1077310761scattered throughout web pages is not enough.We can do more critical part of in-depth opinion assessment,such asfinding rea-sons in opinion-bearing texts.For example,infilm reviews,infor-mation such as‘‘found200positive reviews and150negative reviews”may not fully satisfy the information needs of different people.More useful information would be‘‘Thisfilm is great for its novel originality”or‘‘Poor acting,which makes thefilm awful”.Opinion reason mining tries to identify one of the critical ele-ments of online reviews to answer the question,‘‘What are the rea-sons that the author of this review likes or dislikes the product?”To answer this question,we should extract not only sentences that contain opinion-bearing expressions,but also sentences with rea-sons why an author of a review writes the review(Cardie,Wiebe, Wilson,&Litman,2003;Clarke&Terra,2003;Li&Yamanishi, 2001;Stoyanov,Cardie,Litman,&Wiebe,2004).Kim and Hovy(2005)proposed a method for detecting opinion-bearing expressions.In their subsequent work(Kim&Hovy,2006), they collected a large set of h review text,pros,cons i triplets from ,which explicitly state pros and cons phrases in their respective categories by each review’s author along with the re-view text.Their automatic labeling systemfirst collects phrases in pro and confields and then searches the main review text in or-der to collect sentences corresponding to those phrases.Then the system annotates this sentence with the appropriate‘‘pro”or‘‘con”label.All remaining sentences with neither label are marked as ‘‘neither”.After labeling all the data,they use it to train their pro and con sentence recognition system.3.4.Other applicationsThomas,Pang,and Lee(2006)try to determine from the tran-scripts of US Congressionalfloor debates whether the speeches rep-resent support of or opposition to proposed legislation.Mullen and Malouf(2006)describe a statistical sentiment analysis method on political discussion group postings to judge whether there is oppos-ing political viewpoint to the original post.Moreover,there are some potential applications of sentiment detection,such as online message sentimentfiltering,E-mail sentiment classification,web-blog author’s attitude analysis,sentiment web search engine,etc.4.Subjectivity classificationSubjectivity classification is a task to investigate whether a par-agraph presents the opinion of its author or reports facts.In fact, most of the research showed there was very tight relation between subjectivity classification and document sentiment classification (Pang&Lee,2004;Wiebe,2000;Wiebe,Bruce,&O’Hara,1999; Wiebe,Wilson,Bruce,Bell,&Martin,2002;Yu&Hatzivassiloglou, 2003).Subjectivity classification can prevent the polarity classifier from considering irrelevant or even potentially misleading text. Pang and Lee(2004)find subjectivity detection can compress re-views into much shorter extracts that still retain polarity informa-tion at a level comparable to that of the full review.Much of the research in automated opinion detection has been performed and proposed for discriminating between subjective and objective text at the document and sentence levels(Bruce& Wiebe,1999;Finn,Kushmerick,&Smyth,2002;Hatzivassiloglou &Wiebe,2000;Wiebe,2000;Wiebe et al.,1999;Wiebe et al., 2002;Yu&Hatzivassiloglou,2003).In this section,we will discuss some approaches used to automatically assign one document as objective or subjective.4.1.Similarity approachSimilarity approach to classifying sentences as opinions or facts explores the hypothesis that,within a given topic,opinion sen-tences will be more similar to other opinion sentences than to fac-tual sentences(Yu&Hatzivassiloglou,2003).Similarity approach measures sentence similarity based on shared words,phrases, and WordNet synsets(Dagan,Shaul,&Markovitch,1993;Dagan, Pereira,&Lee,1994;Leacock&Chodorow,1998;Miller&Charles, 1991;Resnik,1995;Zhang,Xu,&Callan,2002).To measure the overall similarity of a sentence to the opinion or fact documents,we need to go through three steps.First,use IR method to acquire the documents that are on the same topic as the sentence in question.Second,calculate its similarity scores with each sentence in those documents and make an average va-lue.Third,assign the sentence to the category(opinion or fact) for which the average value is the highest.Alternatively,for the frequency variant,we can use the similarity scores or count how many of them for each category,and then compare it with a prede-termined threshold.4.2.Naive Bayes classifierNaive Bayes classifier is a commonly used supervised machine learning algorithm.This approach presupposes all sentences in opinion or factual articles as opinion or fact sentences.Naive Bayes uses the sentences in opinion and fact documents as the examples of the two categories.The features include words, bigrams,and trigrams,as well as the part of speech in each sen-tence.In addition,the presence of semantically oriented(positive and negative)words in a sentence is an indicator that the sentence is subjective.Therefore,it can include the counts of positive and negative words in the sentence,as well as counts of the polarities of sequences of semantically oriented words(e.g.,‘‘++”for two con-secutive positively oriented words).It also include the counts of parts of speech combined with polarity information(e.g.,‘‘JJ+”for positive adjectives),as well as features encoding the polarity(if any)of the head verb,the main subject,and their immediate modifiers.Generally speaking,Naive Bayes assigns a document d j(repre-sented by a vector dÃj)to the class c i that maximizes Pðc i j dÃjÞby applying Bayes’rule as follow,Pðc i j dÃjÞ¼Pðc iÞPðdÃjj c iÞPðdÃjÞð1Þwhere PðdÃjÞis the probability that a randomly picked document dhas vector dÃjas its representation,and P(c)is the probability that a randomly picked document belongs to class c.To estimate the term PðdÃjj cÞ,Naive Bayes decomposes it byassuming all the features in dÃj(represented by f i,i=1to m)are con-ditionally independent,i.e.,Pðc i j dÃjÞ¼Pðc iÞQ mi¼1Pðf i j c iÞÀÁPðdÃjÞð2Þ4.3.Multiple Naive Bayes classifierThe hypothesis of all sentences in opinion or factual articles as opinion or fact sentences is an approximation.To address this, multiple Naive Bayes classifier approach applies an algorithm using multiple classifiers,each relying on a different subset of fea-tures.The goal is to reduce the training set to the sentences that are most likely to be correctly labeled,thus boosting classification accuracy.Given separate sets of features F1,F2,...,F m,it train separate Na-ive Bayes classifiers C1,C2,...,C m corresponding to each feature set. Assuming as ground truth the information provided by the docu-ment labels and that all sentences inherit the status of their docu-ment as opinions or facts,itfirst train C1on the entire training set,10762H.Tang et al./Expert Systems with Applications36(2009)10760–10773then use the resulting classifier to predict labels for the training set.The sentences that receive a label different from the assumed truth are then removed,and train C2on the remaining sentences. This process is repeated iteratively until no more sentences can be removed.Yu and Hatzivassiloglou(2003)report results using five feature sets,starting from words alone and adding in bigrams, trigrams,part-of-speech,and polarity.4.4.Cut-based classifierCut-based classifier approach put forward a hypothesis that, text spans(items)occurring near each other(within discourse boundaries)may share the same subjectivity status(Pang&Lee, 2004).Based on this hypothesis,Pang supplied his algorithm with pair-wise interaction information,e.g.,to specify that two particu-lar sentences should ideally receive the same subjectivity label. This algorithm uses an efficient and intuitive graph-based formula-tion relying onfinding minimum cuts.Suppose there are n items x1,x2,...,x n to divide into two classes C1and C2,here access to two types of information:ind j(x i):Individual scores.It is the non-negative estimates of each x i’s preference for being in C j based on just the features of x i alone;assoc(x i,x k):Association scores.It is the non-negative estimates of how important it is that x i and x k be in the same class.Then,this problem changes to calculate the maximization of each item’s score for one class:its individual score for the class it is assigned to,minus its individual score for the other class,then minus associated items into different classes for penalization. Thus,after some algebra,it arrives at the following optimization problem:assign the x i to C1and C2so as to minimize the partition cost:X x2C1ind2ðxÞþXx2C2ind1ðxÞþXx i2C1;x k2C2assocðx i;x kÞð3ÞThis situation can be represented in the following manner.Build an undirected graph G with vertices{v1,...,v n,s,t};the last two are, respectively,the source and sink.Add n edges(s,v i),each with weight ind1(x i),and n edges(v i,t),each with weight ind2(x i).Finally, addðC2nÞedges(v i,v k),each with weight assoc(x i,x k).A cut(S,T)of G is a partition of its nodes into sets S={s}US0and T={t}UT0,where s R S0,t R T0.Its cost cost(S,T)is the sum of the weights of all edges crossing from S to T.A minimum cut of G is one of minimum cost. Then,finding solution of this problem is changed into looking for a minimum cut of G.5.Word sentiment classificationThe task on document sentiment classification has usually in-volved the manual or semi-manual construction of semantic orien-tation word lexicons(Hatzivassiloglou&McKeown,1997; Hatzivassiloglou&Wiebe,2000;Lin,1998;Pereira,Tishby,&Lee, 1993;Riloff,Wiebe,&Wilson,2003;Turney&Littman,2002; Wiebe,2000),which built by word sentiment classification tech-niques.For instance,Das and Chen(2001)used a classifier on investor bulletin boards to see if apparently positive postings were correlated with stock price,in which several scoring methods were employed in conjunction with a manually crafted lexicon.Classify-ing the semantic orientation of individual words or phrases,such as whether it is positive or negative or has different intensities, generally using a pre-selected set of seed words,sometimes using linguistic heuristics(For example,Lin(1998)&Pereira et al.(1993) used linguistic co-locations to group words with similar uses or meanings).Some studies showed that restricting features to those adjec-tives for word sentiment classification would improve perfor-mance(Andreevskaia&Bergler,2006;Turney&Littman,2002; Wiebe,2000).However,more researches showed most of the adjectives and adverb,a small group of nouns and verbs possess semantic orientation(Andreevskaia&Bergler,2006;Esuli&Sebas-tiani,2005;Gamon&Aue,2005;Takamura,Inui,&Okumura, 2005;Turney&Littman,2003).Automatic methods of sentiment annotation at the word level can be grouped into two major categories:(1)corpus-based ap-proaches and(2)dictionary-based approaches.Thefirst group in-cludes methods that rely on syntactic or co-occurrence patterns of words in large texts to determine their sentiment(e.g.,Hatzi-vassiloglou&McKeown,1997;Turney&Littman,2002;Yu&Hat-zivassiloglou,2003and others).The second group uses WordNet (/)information,especially,synsets and hierarchies,to acquire sentiment-marked words(Hu&Liu, 2004a;Kim&Hovy,2004)or to measure the similarity between candidate words and sentiment-bearing words such as good and bad(Kamps,Marx,Mokken,&de Rijke,2004).5.1.Analysis by conjunctions between adjectivesThis method attempts to predict the orientation of subjective adjectives by analyzing pairs of adjectives(conjoined by and,or, but,either-or,or neither-nor)which are extracted from a large unlabelled document set.The underlying intuition is that the act of conjoining adjectives is subject to linguistic constraints on the orientation of the adjectives involved(e.g.and usually conjoins two adjectives of the same-orientation,while but conjoins two adjectives of opposite orientation).This is shown in the following three sentences(where thefirst two are perceived as correct and the third is perceived as incorrect)taken from Hatzivassiloglou and McKeown(1997):‘‘The tax proposal was simple and well received by the public”.‘‘The tax proposal was simplistic but well received by the public”.‘‘The tax proposal was simplistic and well received by the public”.To infer the orientation of adjectives from analysis of conjunc-tions,a supervised learning algorithm can be performed as follow-ing steps:1.All conjunctions of adjectives are extracted from a set ofdocuments.2.Train a log-linear regression classifier and then classify pairs ofadjectives either as having the same or as having different ori-entation.The hypothesized same-orientation or different-orien-tation links between all pairs form a graph.3.A clustering algorithm partitions the graph produced in step2into two clusters.By using the intuition that positive adjectives tend to be used more frequently than negative ones,the cluster containing the terms of higher average frequency in the docu-ment set is deemed to contain the positive terms.The log-linear model offers an estimate of how good each pre-diction is,since it produces a value y between0and1,in which 1corresponds to same-orientation,and one minus the produced value y corresponds to dissimilarity.Same-and different-orienta-tion links between adjectives form a graph.To partition the graph nodes into subsets of the same-orientation,the clustering algo-rithm calculates an objective function U scoring each possible par-tition P of the adjectives into two subgroups C1and C2as,UðPÞ¼X2i¼11j C i jXx;y2C i;x–ydðx;yÞ!ð4Þwhere j C i j is the cardinality of cluster i,and d(x,y)is the dissimilarity between adjectives x and y.H.Tang et al./Expert Systems with Applications36(2009)10760–1077310763In general,because the model was unsupervised,it required an immense word corpus to function.5.2.Analysis by lexical relationsThis method presents a strategy for inferring semantic orienta-tion from semantic association between words and phrases.It fol-lows a hypothesis that two words tend to be the same semantic orientation if they have strong semantic association.Therefore,it focused on the use of lexical relations defined in WordNet to calcu-late the distance between adjectives.Generally speaking,we can defined a graph on the adjectives contained in the intersection between a term set(For example, TL term set(Turney&Littman,2003))and WordNet,adding a link between two adjectives whenever WordNet indicates the presence of a synonymy relation between them,and defining a distance measure using elementary notions from graph theory.In more de-tail,this approach can be realized as following steps:1.Construct relations at the level of words.The simplest approachhere is just to collect all words in WordNet,and relate words that can be synonymous(i.e.,they occurring in the same synset).2.Define a distance measure d(t1,t2)between terms t1and t2onthis graph,which amounts to the length of the shortest path that connects t1and t2(with d(t1,t2)=+1if t1and t2are not connected).3.Calculate the orientation of a term by its relative distance(Kamps et al.,2004)from the two seed terms good and bad,i.e.,SOðtÞ¼dðt;badÞÀdðt;goodÞdðgood;badÞð5Þ4.Get the result followed by this rules:The adjective t is deemedto belong to positive if SO(t)>0,and the absolute value of SO(t) determines,as usual,the strength of this orientation(the con-stant denominator d(good,bad)is a normalization factor that constrains all values of SO to belong to the[À1,1]range).5.3.Analysis by glossesThe characteristic of this method lies in the fact that it exploits the glosses(i.e.textual definitions)that one term has in an online ‘‘glossary”,or dictionary.Its basic assumption is that if a word is semantically oriented in one direction,then the words in its gloss tend to be oriented in the same direction(Esuli&Sebastiani,2005; Esuli&Sebastiani,2006a,2006b).For instance,the glosses of good and excellent will both contain appreciative expressions;while the glosses of bad and awful will both contain derogative expressions.Generally,this method can determine the orientation of a term based on the classification of its glosses.The process is composed of the following steps:1.A seed set(S p,S n),representative of the two categories positiveand negative,is provided as input.2.Search new terms to enrich S p and S e lexical relations(e.g.synonymy)with the terms contained in S p and S n from a thesau-rus,or online dictionary,tofind these new terms,and then append them to S p or S n.3.For each term t i in S0p [S0nor in the test set(i.e.the set of termsto be classified),a textual representation of t i is generated by collating all the glosses of t i as found in a machine-readable dic-tionary.Each such representation is converted into a vector by standard text indexing techniques.4.A binary text classifier is trained on the terms in S0p [S0nandthen applied to the terms in the test set.5.4.Analysis by both lexical relations and glossesThis method determines sentiment of words and phrases both relies on lexical relations(synonymy,antonymy and hyponymy) and glosses provided in WordNet.Andreevskaia and Bergler(2006)proposed an algorithm named ‘‘STEP”(Semantic Tag Extraction Program).This algorithm starts with a small set of seed words of known sentiment value(positive or negative)and implements the following steps:1.Extend the small set of seed words by adding synonyms,ant-onyms and hyponyms of the seed words supplied in WordNet.This step brings on average a5-fold increase in the size of the original list with the accuracy of the resulting list comparable to manual annotations.2.Go through all WordNet glosses,identifies the entries that con-tain in their definitions the sentiment-bearing words from the extended seed list,and adds these head words to the corre-sponding category–positive,negative or neutral.3.Disambiguate the glosses with part-of-speech tagger,and elim-inate errors of some words acquired in step1and from the seed list.At this step,it alsofilters out all those words that have been assigned contradicting.In this algorithm,for each word we need compute a Net Overlap Score by subtracting the total number of runs assigning this word a negative sentiment from the total of the runs that consider it posi-tive.In order to make the Net Overlap Score measure usable in sen-timent tagging of texts and phrases,the absolute values of this score should be normalized and mapped onto a standard[0,1] interval.STEP accomplishes this normalization by using the value of the Net Overlap Score as a parameter in the standard fuzzy mem-bership S-function(Zadeh,1987).This function maps the absolute values of the Net Overlap Score onto the interval from0to1,where 0corresponds to the absence of membership in the category of sentiment(in this case,these will be the neutral words)and1re-flects the highest degree of membership in this category.The func-tion can be defined as follows,Sðu;a;b;cÞ¼0if u6a2uÀac a2if a6u6b1À2uÀacÀa2if b6u6c1if u P c8>>>>>><>>>>>>:ð6Þwhere u is the Net Overlap Score for the word and a,b,c are the three adjustable parameters:a is set to1,c is set to15and b,which represents a crossover point,is defined as b=(a+c)/2=8.Defined this way,the S-function assigns highest degree of membership (=1)to words that have the Net Overlap Score u P15.Net Overlap Score can be used as a measure of the words degree of membership in the fuzzy category of sentiment:the core adjec-tives,which had the highest Net Overlap Score,were identified most accurately both by STEP and by human annotators,while the words on the periphery of the category had the lowest scores and were associated with low rates of inter-annotator agreement.5.5.Analysis by pointwise mutual informationThe general strategy of this method is to infer semantic orienta-tion from semantic association.The underlying assumption is that a phrase has a positive semantic orientation when it has good asso-ciations(e.g.,‘‘romantic ambience”)and a negative semantic orien-tation when it has bad associations(e.g.,‘‘horrific events”)(Turney, 2002).10764H.Tang et al./Expert Systems with Applications36(2009)10760–10773。
MEWS评分在急诊留观患者护理决策中的作用分析
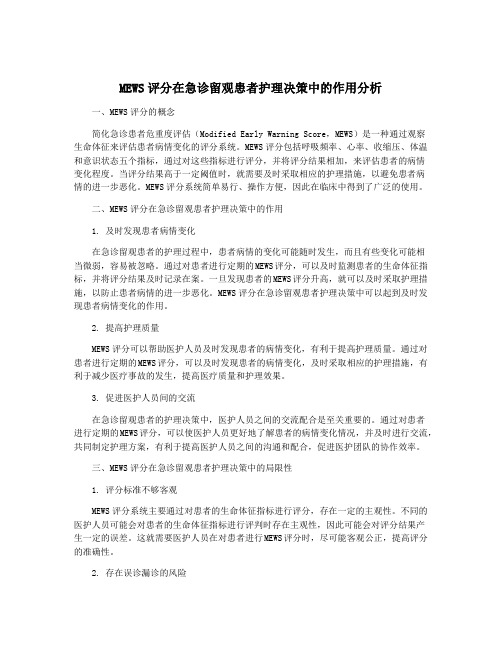
MEWS评分在急诊留观患者护理决策中的作用分析一、MEWS评分的概念简化急诊患者危重度评估(Modified Early Warning Score,MEWS)是一种通过观察生命体征来评估患者病情变化的评分系统。
MEWS评分包括呼吸频率、心率、收缩压、体温和意识状态五个指标,通过对这些指标进行评分,并将评分结果相加,来评估患者的病情变化程度。
当评分结果高于一定阈值时,就需要及时采取相应的护理措施,以避免患者病情的进一步恶化。
MEWS评分系统简单易行、操作方便,因此在临床中得到了广泛的使用。
二、MEWS评分在急诊留观患者护理决策中的作用1. 及时发现患者病情变化在急诊留观患者的护理过程中,患者病情的变化可能随时发生,而且有些变化可能相当微弱,容易被忽略。
通过对患者进行定期的MEWS评分,可以及时监测患者的生命体征指标,并将评分结果及时记录在案。
一旦发现患者的MEWS评分升高,就可以及时采取护理措施,以防止患者病情的进一步恶化。
MEWS评分在急诊留观患者护理决策中可以起到及时发现患者病情变化的作用。
2. 提高护理质量MEWS评分可以帮助医护人员及时发现患者的病情变化,有利于提高护理质量。
通过对患者进行定期的MEWS评分,可以及时发现患者的病情变化,及时采取相应的护理措施,有利于减少医疗事故的发生,提高医疗质量和护理效果。
3. 促进医护人员间的交流在急诊留观患者的护理决策中,医护人员之间的交流配合是至关重要的。
通过对患者进行定期的MEWS评分,可以使医护人员更好地了解患者的病情变化情况,并及时进行交流,共同制定护理方案,有利于提高医护人员之间的沟通和配合,促进医护团队的协作效率。
三、MEWS评分在急诊留观患者护理决策中的局限性1. 评分标准不够客观MEWS评分系统主要通过对患者的生命体征指标进行评分,存在一定的主观性。
不同的医护人员可能会对患者的生命体征指标进行评判时存在主观性,因此可能会对评分结果产生一定的误差。
研究力的三要素对效果的影响时研究方法

研究力的三要素对效果的影响时研究方法1.研究力的第一个要素是研究的深度和广度。
The first element of research ability is the depth and breadth of the research.2.对于一个问题或者主题的深入研究通常会产生更有价值的洞见。
In-depth research on a question or topic typically yields more valuable insights.3.同时,广泛的研究可以帮助研究者更好地理解整个领域的背景和相关因素。
Similarly, extensive research can help researchers better understand the background and related factors of the entire field.4.研究力的第二要素是研究方法的选择和应用。
The second element of research ability is the selection and application of research methods.5.合理的研究方法可以确保研究的可靠性和准确性。
Proper research methods can ensure the reliability and accuracy of the research.6.使用科学的研究方法也能够增加研究的可复制性和可验证性。
Using scientific research methods can also increase the replicability and verifiability of the research.7.此外,选择适当的研究方法还可以使研究过程更加高效和有效。
Furthermore, choosing the appropriate research methodscan make the research process more efficient and effective.8.研究力的第三要素是数据分析和解读的能力。
如何通过一篇文献做临床证据质量评价
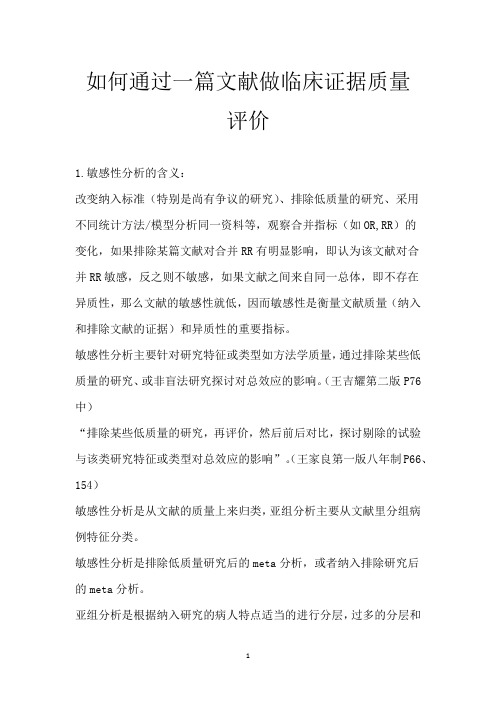
如何通过一篇文献做临床证据质量评价1.敏感性分析的含义:改变纳入标准(特别是尚有争议的研究)、排除低质量的研究、采用不同统计方法/模型分析同一资料等,观察合并指标(如OR,RR)的变化,如果排除某篇文献对合并RR有明显影响,即认为该文献对合并RR敏感,反之则不敏感,如果文献之间来自同一总体,即不存在异质性,那么文献的敏感性就低,因而敏感性是衡量文献质量(纳入和排除文献的证据)和异质性的重要指标。
敏感性分析主要针对研究特征或类型如方法学质量,通过排除某些低质量的研究、或非盲法研究探讨对总效应的影响。
(王吉耀第二版P76中)“排除某些低质量的研究,再评价,然后前后对比,探讨剔除的试验与该类研究特征或类型对总效应的影响”。
(王家良第一版八年制P66、154)敏感性分析是从文献的质量上来归类,亚组分析主要从文献里分组病例特征分类。
敏感性分析是排除低质量研究后的meta分析,或者纳入排除研究后的meta分析。
亚组分析是根据纳入研究的病人特点适当的进行分层,过多的分层和过少的分层都是不好的。
例如在排除某个低质量研究后,重新估计合并效应量,并与未排除前的Meta分析结果进行比较,探讨该研究对合并效应量影响程度及结果稳健性。
若排除后结果未发生大的变化,说明敏感性低,结果较为稳健可信;相反,若排除后得到差别较大甚至截然相反结论,说明敏感性较高,结果的稳健性较低,在解释结果和下结论的时候应非常慎重,提示存在与干预措施效果相关的、重要的、潜在的偏倚因素,需进一步明确争议的来源。
2.衡量方法和措施其实常用的就是选择不同的统计模型或进行亚组分析,并探讨可能的偏倚来源,慎重下结论。
亚组分析通常是指针对研究对象的某一特征如性别、年龄或疾病的亚型等进行的分析,以探讨这些因素对总效应的影响及影响程度。
而敏感性分析主要针对研究特征或类型如方法学质量,通过排除某些低质量的研究、或非盲法的研究以探讨对总效应的影响。
建议可以看参考王吉耀主编,科学出版社出版的《循证医学与临床实践》。
灵敏度分析

Sensitivity Analysis灵敏度分析
To study how changes in the parameters of a LP model affect the optimal solution. 研究线性规划模型的参数如何变化会影响 最优解。 It involves checking how sensitive the optimal solution is to the value of each parameters.最优解对每一参数变化的灵敏 度。
What is Sensitivity Analysis 什么是敏感性分析
Most of the important insights are gained while conducting analysis after finding an optional solution for the original version of the basic model.
Parameters of the model 模型的参数(2)
Some factors cannot be estimated with real accuracy until long after the linear programming study has been completed and the new products have been on the market for some time. For example, the profits depend on many factors: the costs of raw materials, production, shipping, advertising, and so on, as well as such things as the market reception to the new products and the amount of competition encountered.
- 1、下载文档前请自行甄别文档内容的完整性,平台不提供额外的编辑、内容补充、找答案等附加服务。
- 2、"仅部分预览"的文档,不可在线预览部分如存在完整性等问题,可反馈申请退款(可完整预览的文档不适用该条件!)。
- 3、如文档侵犯您的权益,请联系客服反馈,我们会尽快为您处理(人工客服工作时间:9:00-18:30)。
Preliminary Study of Analytic Sensitivities for CFD Codes Through Automatic Di erentation
Alan Carle Mike Fagan
CRPC-TR96662 October 1996
Center for Research on Parallel Computation Rice University 6100 South Main Street CRPC - MS 41 Houston, TX 77005
computation cost for the Cray shows a penalty of 1tion evaluation | much more expensive than even two-sided di erences. Furthermore, the memory requirements for a derivative code can be given as O(I *M ), where I is the number of independent variables in the di erentiation, and M is the memory required for the function evaluation. Hence, for large problems, the memory requirements are likely to be the limiting resource with regard to derivative computation. The relatively steep cost of computing derivatives can be alleviated somewhat by use of extra processors using OVERFLOW's own PVM-based parallel capability. OVERFLOW is an overlapping multizone ow solver. Current parallel versions use one process per zone with one additional master process coordinating the zone interactions. To di erentiate parallel OVERFLOW, we used ADIFOR-MP, a new \message passing aware" version of ADIFOR. ADIFOR-MP processed parallel OVERFLOW, yielding a parallel multizone derivative code. The performance of the largest zone determines the overall performance of the parallel derivative code. For parallel OVERFLOW on the SP2, the ratio of derivative cost to function cost was still about 5.0, the same as for sequential OVERFLOW. The memory requirements, however, are reduced in the sense that each processor needs only enough memory for one zone, not the entire grid. In this way, function code parallelism enables parallel derivative calculation. As an additional bene t, for problems with large memory requirements, function grids could be divided into smaller zones, leaving more memory available for derivative computations.
Preliminary Study of Analytic Sensitivities for CFD Codes Through Automatic Di erentiation 1 CRPC-TR96662. Alan Carle ( carle@ ) Mike Fagan ( mfagan@ ) Rice University Center for Research on Parallel Computation 6100 Main St., MS 41 Houston, Texas 77005
1 Introduction
Modern engineering design depends on sensitivity calculations. To obtain these sensitivities, engineers have two choices: use nite di erences, or develop a program to compute the analytic derivatives. To avoid programming, engineers have typically chosen to use nite di erences. The accuracy of nite di erence calculations, however, depends on getting a \good" step size. A \good" step size, however, depends on two competing factors. To approximate the derivative well, the step size should be \small." On the other hand, to avoid critical cancellation, the step size should be \moderate." Finding an appropriate step size to satisfy both criteria can be di cult, especially for complex processes like CFD calculations. Furthermore, the step size exercise may need to be repeated for each new point in the design space. Analytic derivatives, by de nition, avoid the step size problem. Generating an analytic derivative program by hand, however, is tedious, time consuming, and prone to error. Fortunately, engineers can avoid the \by hand" part by using an automatic di erentiation (AD) tool. An AD tool automatically applies the derivative chain rule to all of the expressions in a program, thereby generating a program that computes both the desired analytic derivatives, in addition to the function value. The speci c AD tool we use for our research is ADIFOR 2.0, developed jointly by Argonne National Laboratory and Rice University. ADIFOR stands for Automatic Di erentiation of Fortran. Our hardware testbeds consisted of Rice's 8 node SP2 (RS6000 nodes), and Langley's Cray YMP. To nd out more about ADIFOR, see /adifor or /~adifor. In this work, we describe results obtained by application of automatic di erentiation to OVERFLOW, an extensively used CFD code from NASA Ames. OVERFLOW is currently a focal point of a NASA project that uses derivatives for shape optimization and inverse problems. The projected problems are expected to be large, and, more signi cantly, require a large number of derivatives. For these large problems, a naive (\black box") use of AD will probably be ine ective. Consequently, we have been exploring more sophisticated techniques for computing CFD derivatives. In particular, we have been exploring the use of stripmining to exploit available parallel resources. In addition, we have also explored the incremental iterative method (IIM) for computing derivatives. The IIM method reduces the amount of redundant computation that occurs with naive application of AD.