MS(Online)-2014(12)--Maximizing Stochastic Monotone Submodular Functions
Strong Lefschetz elements of the coinvariant rings of finite Coxeter groups
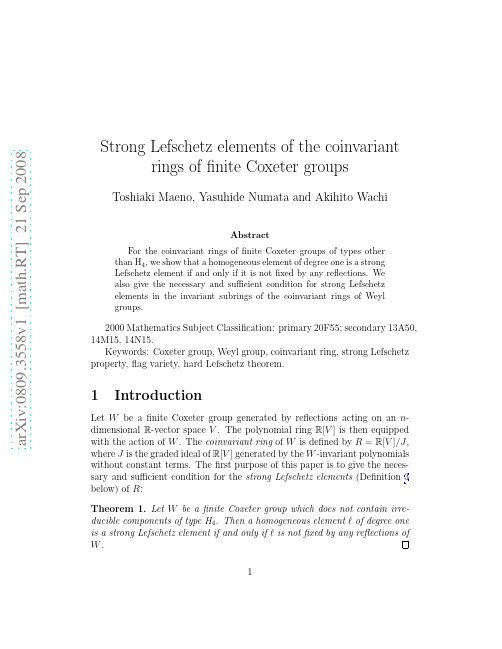
Keywords: Coxeter group, Weyl group, coinvariant ring, strong Lefschetz property, flag variety, hard Lefschetz theorem.
Theorem 1. Let W be a finite Coxeter group which does not contain irreducible components of type H4. Then a homogeneous element ℓ of degree one is a strong Lefschetz element if and only if ℓ is not fixed by any reflections of W.
In view of such a property of the cohomology ring, the strong Lefschetz prop-
erty is defined as follows:
Definition 3. A graded ring R =
m d=0
Rd
havபைடு நூலகம்ng
a
symmetric
tion 7. see [GH78], e.g.) tells us that the multiplication by the class of the K¨ahler form induces an isomorphism between Hi(X, R) and H2 dimC X−i(X, R).
成人高考升专科英语试卷

一、语音知识(共5小题,每题1.5分,共7.5分)1. 请选出下列单词中划线部分发音与其他三项不同的单词。
A. farmB. fanC. farD. family2. 请选出下列句子中划线部分发音与其他三项不同的句子。
A. She is a teacher.B. I have two brothers.C. He works hard.D. They are students.3. 请选出下列单词中划线部分发音与其他三项不同的单词。
A. mapB. catC. mapleD. match4. 请选出下列句子中划线部分发音与其他三项不同的句子。
A. It's a beautiful day.B. I like apples.C. She is in the classroom.D. They are watching TV.5. 请选出下列单词中划线部分发音与其他三项不同的单词。
A. homeB. hopeC. holeD. hold二、词汇与语法知识(共15小题,每题1.5分,共22.5分)1. Fill in the blanks with the proper form of the given verb in the brackets.They _______ (be) at the library when I called them.2. Choose the correct form of the given word to complete the sentence.She _______ (be) a teacher for 10 years.3. Choose the correct word to complete the sentence.He _______ (go) to the movies last night.4. Choose the correct word to complete the sentence.She _______ (not go) to the party because she was ill.5. Choose the correct word to complete the sentence.The weather _______ (not be) good today.6. Choose the correct word to complete the sentence.I _______ (not go) to the park because it's raining.7. Choose the correct word to complete the sentence.He _______ (not work) hard enough to pass the exam.8. Choose the correct word to complete the sentence.They _______ (not go) to the concert last night.9. Choose the correct word to complete the sentence.The movie _______ (not be) interesting.10. Choose the correct word to complete the sentence.She _______ (not go) to the party because she was tired.11. Choose the correct word to complete the sentence.The weather _______ (not be) good yesterday.12. Choose the correct word to complete the sentence.He _______ (not work) hard enough to pass the exam.13. Choose the correct word to complete the sentence.They _______ (not go) to the concert last night.14. Choose the correct word to complete the sentence.The movie _______ (not be) interesting.15. Choose the correct word to complete the sentence.She _______ (not go) to the party because she was tired.三、完形填空(共15小题,每题2分,共30分)Read the following passage and choose the best answer for each blank.When I was a child, I lived in a small town. There was a river running through the town, and it was my favorite place to play. Every day, I would go to the river with my friends, and we would fish, swim, and play games.One day, my father told me that the river was polluted, and it was not safe for us to play there anymore. I was sad and worried, because I loved the river so much. I decided to do something about it.I started by talking to my friends and asking them to join me in cleaning the river. We collected garbage and plastic waste from the riverbank and threw it in the trash. We also wrote letters to the local government, asking them to take action to clean the river.After a few months, the government finally responded. They began to clean the river and restore its natural beauty. The river became clean again, and we could play there without worrying about pollution.I learned a valuable lesson from this experience. I realized that even a small group of people can make a difference if we work together. I am grateful for the opportunity to help clean the river and make it a safe place for everyone to enjoy.1. The author's favorite place to play was the ___________.A. parkB. beachC. riverD. forest2. The author's father told him that the river was ___________.A. cleanB. pollutedC. dirtyD. beautiful3. The author decided to do something about the pollution by ___________.A. ignoring itB. writing letters to the governmentC. cleaning theriver himself D. moving to a different town4. The author and his friends collected garbage and ___________.A. recycled itB. threw it in the trashC. burned itD. buried it5. The author and his friends wrote letters to the ___________.A. local governmentB. school principalC. mayorD. river6. The government began to clean the river and ___________.A. ignored the problemB. restored its natural beautyC. destroyed the riverD. left it alone7. The river became clean again, and the author could play there___________.A. without worrying about pollutionB. with his friends onlyC. without his fatherD. with the government8. The author learned a valuable lesson from this experience, which was ___________.A. the importance of working togetherB. the importance of being aloneC. the importance of ignoring problemsD. the importance of moving to a different town9. The author is grateful for the opportunity to help clean the riverand ___________.A. move to a different townB. write letters to the governmentC. make the river a safe place for everyoneD. ignore the problem10. The author's story shows that ___________.A. a small group of people can't make a differenceB. working together can solve problemsC. it's important to move to a different townD. the government should always take action11. The author's friends ___________.A. helped him clean the riverB. ignored the problemC. moved to a different townD. wrote letters to the government12. The author's father ___________.A. supported his decision to clean the riverB. didn't believe the river was pollutedC. was worried about the river's pollutionD. ignored the problem13. The author's story took place in ___________.A. a big cityB. a small townC. a forestD. a beach14. The author's friends ___________.A. collected garbage and wrote letters to the governmentB. ignored the problemC. moved to a different townD. wrote letters to the mayor15. The author's story shows that ___________.A. a small group of people can't make a differenceB. working together can solve problemsC. it's important to move to a different townD. the government should always take action四、阅读理解(共15小题,每题3分,共45分)阅读下列短文,然后回答问题。
时间序列 数据清洗和预处理 数据分解 box-cox方法
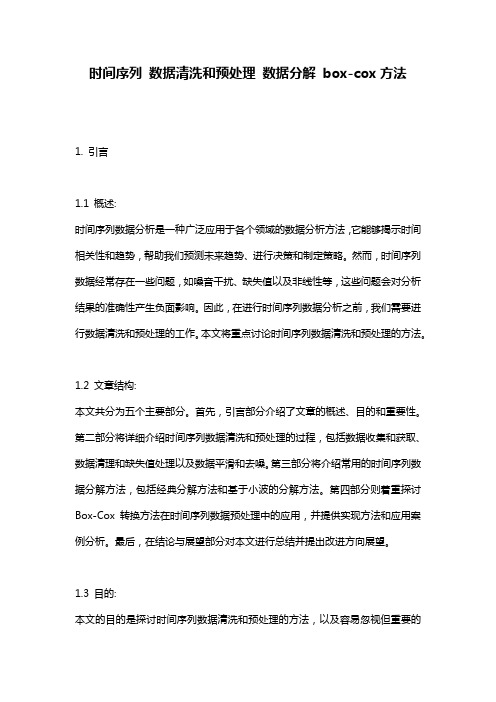
时间序列数据清洗和预处理数据分解box-cox方法1. 引言1.1 概述:时间序列数据分析是一种广泛应用于各个领域的数据分析方法,它能够揭示时间相关性和趋势,帮助我们预测未来趋势、进行决策和制定策略。
然而,时间序列数据经常存在一些问题,如噪音干扰、缺失值以及非线性等,这些问题会对分析结果的准确性产生负面影响。
因此,在进行时间序列数据分析之前,我们需要进行数据清洗和预处理的工作。
本文将重点讨论时间序列数据清洗和预处理的方法。
1.2 文章结构:本文共分为五个主要部分。
首先,引言部分介绍了文章的概述、目的和重要性。
第二部分将详细介绍时间序列数据清洗和预处理的过程,包括数据收集和获取、数据清理和缺失值处理以及数据平滑和去噪。
第三部分将介绍常用的时间序列数据分解方法,包括经典分解方法和基于小波的分解方法。
第四部分则着重探讨Box-Cox转换方法在时间序列数据预处理中的应用,并提供实现方法和应用案例分析。
最后,在结论与展望部分对本文进行总结并提出改进方向展望。
1.3 目的:本文的目的是探讨时间序列数据清洗和预处理的方法,以及容易忽视但重要的Box-Cox转换方法在时间序列数据分析中的应用。
通过深入了解和研究这些方法,读者将能够更好地理解如何有效地处理时间序列数据,降低噪音干扰、处理缺失值,并提高对数据趋势和相关性的理解能力。
此外,我们还将通过实际案例分析来展示这些方法在实际问题中的应用效果,帮助读者更好地理解其实际价值和应用场景。
最终,我们期望本文对时间序列数据分析领域的从业人员和学术研究者有所帮助,并为进一步研究和应用提供指导。
2. 时间序列数据清洗和预处理2.1 数据收集和获取数据收集是时间序列分析中的第一步,它涉及到获取可用于分析的原始时间序列数据。
常见的数据收集方法包括实时采集、历史数据提取和数据库查询等。
在进行数据收集之前,需要明确所需的时间范围、采样频率以及目标变量等。
2.2 数据清理和缺失值处理在时间序列数据中,经常会遇到许多问题,如异常值(outliers)、噪声(noise)以及缺失值(missing values)等。
微软消息分析器:一款高级网络包分析器说明书

Neil B MartinT est Manager WSSC-Interop and T oolsMicrosoft CorporationMicrosoft Message Analyzer Packet Analysis at a Higher LevelContent•Packet Analyzer -review •Abstracting views of protocols •Alternative data sources •ETW•Remote Capture•Bluetooth•USB•Evtx•Logs filesMessage Analyzer –What is it?• A packet analyzer is a computer program or a piece of computer hardware that can intercept and log traffic passing over all or part of a network•Packet analyzers capture network packets in real time and display them in human-readable format•WireShark, Microsoft NetMon3.4•These tools are dissectors•If they recognize a packet they dissect it and display the inner fields of the packet•The parsers are written based on the protocol specifications or in some cases through reverse engineering of the protocols whenno specification is available•Dissection and Abstraction•We want to allow a higher level of abstraction view of protcols•Pattern Matching•Match up request/response pairs where possible•Called an operation•Different Viewers and Charts•Addressing many of the challenges of diagnosing modern networks •Protocol Validation•Identify packets that do not match the specification•Data capture from multiple sources•NDIS, Bluetooth, USB, Windows Firewall Layer, Web Proxy•Header only network capture•Reduce data in volume scenarios•Correlation of data across multiple data sources and logs•Load and display multiple data source•Message Analyzer captures ETW •ETW -Event Trace for Windows •Message Capture from:•Traditional NDIS traffic from the Network Adapter •Windows Filtering Platform 9aka Firewall)•Web proxy•USB ports•Bluetooth•Windows SMB Client•Windows SMB Server ……•E vent T racing for W indows ETW•High-resolution (<<100µs)logging infrastructure allows any component to tell the outside world what it is currently doing by firing ETW events.• A powerful diagnostic tool to log every methods/lines inside the code with reasonable performance fordebugging/troubleshooting.•MSDN on ETW/en-us/library/bb968803(VS.85).aspxAll Windows ETW Sources are available to Message Analyzer•Capability to perform remote capture •Select machine and give credentials•Collect data via ETW from NIC on remote machine•Powerful, extensible viewing and analysis •Browse, Select, View•Browse for messages from various sources (live, or stored)•Select a set of messages from those sources by characteristic(s)•View messages in a provided viewer, configure or build your own • A new high-level grid view•High level “Operations” view with automatic re-assembly•“Bubbling up” of errors in the stack to the top level•Ability to drill down the stack to underlying messages and/or packets•On the fly grouping, filtering, finding, or sorting by any message property •Payload rendering•V alidation of message structures, behavior, and architecture•Does the protocol comply with the specifications?•Over 450 published specifications for Windows Protocols(as of Windows 8.1)(/en-us/library/gg685446.aspx)Available online and as PDFContinue to publish new documents with each release of Windows •Continue to develop tools and technology to aid with the development of protocol documents, parsers and test technologyHow to get MA: /en-us/download/details.aspx?id=40308 How to get help: Blog,Operating Guide, T echnet Forum for Message Analyzer •We invite you to Explore Message Analyzer•Connect Community•https:///site216/。
REPSOL_VETTING_PROCESS_AND_CRITERIA_2014_tcm11-689923
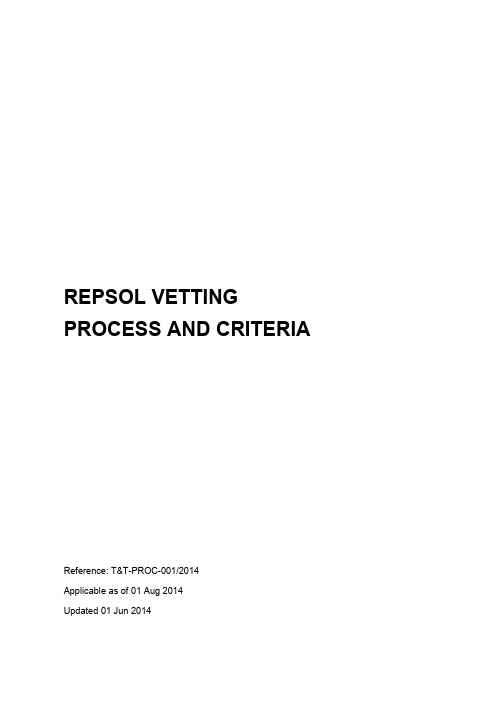
III. Effective Date
01 Aug 2014
Page 2 of 31
REPSOL VETTING PROCESS AND CRITERIA
IV. DEFINITIONS For the purpose of these procedures, the following definitions apply: Acceptable means the vessel can be used within the scope described above, and is the only rating that allows such use. This rating results from a favourable assessment based on information that we have deemed positive and sufficient. The rating of the vessel may be affected by relevant modifications concerning safety and operational systems, changes of name, technical operator, crew, flag, etc., as well as any incident, casualty or terminal negative feedback report, PSC detention or Memoranda or condition of Class. (See also “Vetting Assessment”.) Barge, for the purpose of these procedures, means a vessel carrying goods in rivers, inland navigation, lakes and ports, not sailing on open sea or bays and restricted by Flag Administration to inland water navigations. EBIS Barge: for the purpose of these procedures, means a vessel carrying goods in European rivers, not sailing on open sea or bay CAP (Condition Assessment Programme).- Independent and thorough scheme of inspections of the actual condition of a vessel. It is applicable as established in the present Vetting Process and Criteria and as defined in the Rules of the Classification Societies members of IACS. Cargo means any kind of material subject to a contract of transportation, mainly crude oil, oil products, chemical products, LPG, LNG, Lubricants, Liquid fertilizers and dry bulk cargoes. Charter Party means contract of affreightment signed between shipowner and charterer when hiring a vessel for the carriage of goods. Chief Officer and 2nd. Engineer terminology considered equivalent to 1st. Officer and 1st. Asst. Engineer for the purpose of these procedures. COA vessel means vessels included in a contract of affreightment to lift a fixed or determinable quantity of cargo of a specified type over a given period of time. EBIS the European Barge Inspection Scheme, is used to evaluate barges, tugs and dumb barges used to distribute oil and chemicals within Europe ESP (Enhanced Survey Programme).- It is applicable as established in SOLAS XI1/2 and as defined in Resolution A.744 (18). Heavy grade Oil: o o o crude oils, having a density at 15º C higher than 900 kg/m3; oils, other than crude oils, having either a density at 15º C higher than 900 kg/m3 or a kinematic viscosity at 50 º C higher than 180 mm2/s; or; bitumen, tar and their emulsions.
CDA_LEVEL_1试题及答案
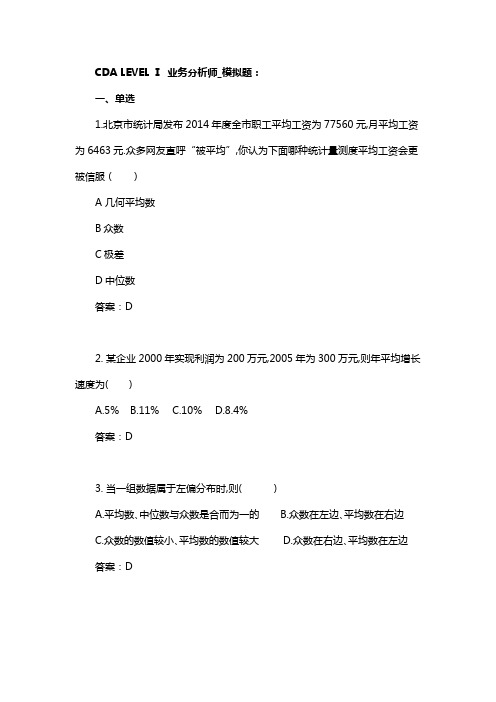
CDA LEVELⅠ业务分析师_模拟题:一、单选1.北京市统计局发布2014年度全市职工平均工资为77560元,月平均工资为6463元.众多网友直呼“被平均”,你认为下面哪种统计量测度平均工资会更被信服()A几何平均数B众数C极差D中位数答案:D2.某企业2000年实现利润为200万元,2005年为300万元,则年平均增长速度为()A.5%B.11%C.10%D.8.4%答案:D3.当一组数据属于左偏分布时,则()A.平均数、中位数与众数是合而为一的B.众数在左边、平均数在右边C.众数的数值较小、平均数的数值较大D.众数在右边、平均数在左边答案:D4.作为一家制造类企业,以下哪个图适合比较不同产品各年的销售变化情况()A.分组饼形图B.堆叠面积图C.堆叠柱形图D.分组柱形图答案:D5.横截面数据主要注意以下哪个数据问题()A.异方差B.不独立C.非正态分布D.不随机答案:A.6.以下叙述正确的是()A.极差较少受异常值的影响B.四分位差较少受异常值的影响C.方差较少受异常值的影响D.标准差较少受异常值的影响答案:B。
7.某汽车品牌预测到未来不同型号汽车的利润率和销售量会发生变化,希望根据利润最大化得到最优产量,这属于哪类数据分析过程()A.预测型建模B.预报C.优化D.报警答案:C8.为研究某种减肥茶减肥效果是否显著,可以采用()分析方法。
A、单样本t检验B、两独立样本t检验C、两配对样本t检验D、方差分析答案:C9.中心极限定理的假设不包括()A.样本相互独立B.样本具有相同分布C.样本足够大D.样本服从正态分布答案:D10.下列场合下,()适合用t检验统计量A.样本为小样本,且总体方差已知B.样本为大样本,且总体方差已知C.样本为小样本,且总体方差未知D.样本为大样本,且总体方差未知答案:C11.方差分析表表如下,值20应填在哪个位置上()方差来源离差平方和自由度均方差F值组间14245.8334748.61 2.16组内A B C总和D23答案:B12.某信用卡公司为了分析客户教育程度对授信额度是否有显著性差异,已知教育程度分为5种,每个教育程度取30个客户,则因素的水平为()A5B6C30D15013.给出下列结论:(1)在回归分析中,可用指数系数R方的值判断模型的拟合效果,R方越大,模型的拟合效果越好;(2)在回归分析中,可用残差平方和判断模型的拟合效果,残差平方和越大,模型的拟合效果越好;(3)在回归分析中,可用相关系数r的值判断模型的拟合效果,r越小,模型的拟合效果越好;(4)在回归分析中,可用残差图判断模型的拟合效果,残差点比较均匀地落在水平的带状区域中,说明这样的模型比较合适.带状区域的宽度越窄,说明模型的拟合精度越高.以上结论中,正确的有(B)个.A.1B.2C.3D.414.下列关系中,属于正相关关系的有()A.合理限度内,施肥量和平均单位产量之间的关系B.产品产量与单位产品成本之间的关系C.商品的流通费用与销售利润之间的关系D.流通费用率与商品销售量之间的关系答案:A15.下列关于SQL的说法错误的是()A SQL对大小写不敏感B SQL为非过程化编程语言C不同的数据库的SQL完全一致D一种数据库查询和程序设计语言,用于存取数据以及查询、更新和管理关系数据库系统答案:C16.要查询book表中所有书名中以“中国”开头的书籍的价格,可用()语句。
企业道德和社会责任 Business Ethics and Social Responsibilit

What Business Areas Does CSR Cover
• Ethics - discretionary actions. • Moral righteousness.
• Strategic brand management - Brand building, Brand insurance.’
Corporate social responsibility (CSR) is concerned with the ways in which an organisation exceeds its minimum obligations to stakeholders specified through regulation
• This poses a challenge as there are many different stakeholders with different, perhaps conflicting, expectations.
• Managers will need to take a view on: i. Which stakeholders have the greatest
development in LDCs. • Importance of global brands and corporate
reputations.
11
Key Drivers in CSR
• Changing social expectations - Consumers and society in general expect more from the companies whose products they buy. This sense has increased in the light of recent corporate scandals, which reduced public trust of corporations, and reduced public confidence in the ability of regulatory bodies and organisations to control corporate excess.
人教版英语七年级上册Unit1 SectionB1(1a-1d)课件

Parrot Beijing roast duck the UK Singapore guitar tennis
parro t
1a Wrhiatet dthoeywouorldeasrin athbeoubtoxthuentdweor stthuedceonrtrse?ct pictures.
Parrot Beijing roast duck the UK Singapore guitar tennis
in the UK. Now, I live in
Beibjinigg with my parents. My
fdauvcopkua.rIrittr'esofgtoroedati!s IBleikijeinmg ursoiacst
and I play the guitar in the
school band. Would you like
The Lion City is Singapore’s nickname(昵称). Merlion Park is the symbol of Singapore.
鱼尾狮 公园
the UK
Big Ben is the symbol of Singapore
the national flag of the UK
country
hobby
country
I think he/he lives in__S_in_g_a_p__o_re__. She has a __p_a_r_r_o_t___. She likes _te_sn_pn_o_is_rt.
pet
I think he / she likes playing the __g_u_i_ta_r_. He is from
- 1、下载文档前请自行甄别文档内容的完整性,平台不提供额外的编辑、内容补充、找答案等附加服务。
- 2、"仅部分预览"的文档,不可在线预览部分如存在完整性等问题,可反馈申请退款(可完整预览的文档不适用该条件!)。
- 3、如文档侵犯您的权益,请联系客服反馈,我们会尽快为您处理(人工客服工作时间:9:00-18:30)。
Maximizing Stochastic Monotone Submodular Functions Arash Asadpour∗Hamid Nazerzadeh†Amin Saberi∗AbstractWe study the problem of maximizing a stochastic monotone submodular function with respect to a matroid constraint.We study the adaptivity gap-the ratio between the values of optimal adaptive andnon-adaptive policies-and show that it is equal to ee−1.This result implies that the benefit of adaptivityis bounded.We also study the myopic policy and show that it is a12-approximation.Furthermore,when thematroid is uniform,approximation ratio of the myopic policy becomes1−1ewhich is optimum.1IntroductionThe problem of maximizing submodular functions has been extensively studied in operations research and computer science.For a set A,the set function f:2A→R is submodular if for any two subsets S,T⊂A we havef(S∪T)+f(S∩T)≤f(S)+f(T)An equivalent definition is that the inequality below holds for any S⊆T⊆A and j∈Af(T+j)−f(T)≤f(S+j)−f(S)where f(·+j)denotes f(·∪{j}).Also,function f is monotone if for any two subsets S⊆T⊆A:f(S)≤f(T)A wide range of optimization problem that arise in the real world can be modeled as maximizing a monotone submodular functions with respect to some constraints.One instance is the welfare maximization problem[9,11,23]which is tofind an optimal allocation of resources to agents where the utilities of the agents are submodular.Submodularity corresponds to the law of diminishing return in economy.Another application of this problem is capital budgeting in which a risk-averse investor with a limited budget is interested infinding the optimal investment in different projects[24,2].The utility function of a risk averse investor is submodular.It is also naturally non-negative and monotone.Another example is the problem of viral marketing and maximizing influence through the network[14,18], where the goal is to choose an initial“active”set of people,so as to maximize the spread of a technology or behavior in a social network.It is well-known that under many models of influence propagation in networks (e.g.,cascade model[14]),the expected size of thefinal cascade is a submodular function of the set of initially activated individuals.Also,due to budget limitations,the number of people that we can activate in the beginning is bounded.Hence,the maximizing influence problem can be seen as a maximizing submodular function problem subject to cardinality constraints.Yet another example is the problem of optimal placement of sensors for environmental monitoring[16,17] where the objective is to place sensors in the environment in order to most effectively reduce uncertainty in observations.This problem can be modeled by entropy minimization and,due to the concavity of the entropy function,it is a special case of submodular optimization.∗Management Science and Engineering Department,Stanford University,Stanford,CA.{asadpour,saberi}@ †Microsoft Research,Cambridge,MA.hamidnz@1For the above problems and many others,the constraints can be model by a matroid.Afinite matroid M is defined by a pair(A,I),where I is a collection of subsets of A(called the independent sets)with the following properties:1.Every subset of an independent set is independent.2.If S and T are two independent sets and T has more elements than S,then there exists an element inT which is not in S and when added to S still gives an independent set.Two important special cases are uniform matroid and partition matroid.In a uniform matroid,all the subsets of A of size at most k,for a given k,are independent.Uniform matroids represent cardinality constraints.A partition matroid is defined over a partition of set A,where every independent set includes at most one element from each set in the partition.The celebrated result of Nemhauser et al.[20]shows that for maximizing nonnegative monotone sub-modular functions over uniform matroids,the greedy algorithm gives a(1−1e ≈0.632)-approximation ofthe optimal ter,they showed that for optimizing over matroids,the approximation ratio of thegreedy algorithm is12.Recently,Calinescu et al[5]proposed a better approximation algorithm with ratio1−1e .It also has been shown that this factor is optimal(in the value oracle model),if only a polynomialnumber of queries is allowed[19,10].However,these algorithms are designed for deterministic environments.In practice,one must deal with the stochasticity caused by the uncertain nature of the problem,the incomplete information about the environment,etc.For instance,in welfare maximization,the quality of the resources may be unknown in advance,or in the capital budgeting problem some projects taken by an investor may fail due to unexpected events in the market.As another example,in viral marketing some people in the initial set might not adopt the behavior.Also,in the environmental monitoring example,it is expected that a non-negligible fraction of sensors might not work properly for various reasons.All these possibilities motivate the problem of stochastic submodular maximization.In the stochastic setting,the outcome of the elements in the selected set are not known in advance and they will be only discovered after they are chosen.1.1Problem DefinitionIn defining the problem,we need to use some care to maintain generality.Consider a set A={X1,···,X n} of n independent random variables over a domain∆.The domain varies depending on the application.For instance,in the welfare maximization problem,X i denotes the quality of the resource or in viral marketing X i corresponds to the set of people who are influenced by person i.The distribution of each X i is potentially different and is given by a function g i.Let x i denote a realization of X i.Also,let vector s=<ˆx1,···,ˆx n>denote a realization of set S⊂A, whereˆx i=x i for X i∈S andˆx i=0for i/∈S.For a given function f:∆n→R+,we can define the stochastic function F:A→R+as F(S)=E[f(s)],where s is a realization of S and the expectation is taken with respect to the product distribution defined by g i’s.Also,consider a subset T∈A,and a realization t of T.We can define a conditional expectation E[f(s)|t]. In the distribution imposed by conditioning on t,s i=t i if its corresponding random variable is in S∩T. Otherwise s i is chosen independently with respect to the distribution defined by g i’s.Let us denote this conditional expectation with F(S,t).We call the set function F stochastic monotone submodular if F(·,t)is monotone submodular for every t. Observe that if f is monotone submodular then F is stochastic monotone submodular because it is a convex combination of monotone submodular functions.Remark:We assume that either we can compute the value of function F up to a desired degree of accuracy explicitly,or F is given to us via an“oracle”.This is a natural assumption for all the applications mentioned in the paper.In fact,in most cases the expectations can be computed simply by using sampling.For example,sampling works when the probability distribution functions are constant Lipschitz continuous,or2when their support is a polynomial size set of discrete values.In both cases,a small o(1)error is introduced in the calculations that we ignore in the rest of the paper.Definition:[Maximizing a stochastic monotone submodular function]The set A={X1,···,X n}of n independent random variables,the matroid M=(A,I),and the stochastic monotone submodular set function F:2A→R+are given.Find a subset S∈I that maximizes F,i.e.,max S∈I E[F(S)]where the expectation is taken over the probability distribution of the sets chosen by the policy.A special case of the above problem is the stochastic max k-cover problem which is defined as follows. Suppose a collection A of random subsets of N={1,2,···,m}are given.Each element X i∈A is a random subset of N,and it is distribution is denoted by a probability distribution g i.In the stochastic maximum k-cover problem,the goal is to choose k elements of A such that their union has the maximum cardinality. We discuss this problem in more detail in Section2.1.For the problem of maximizing a stochastic monotone submodular function,we study two types of policies: adaptive and non-adaptive.A non-adaptive policy is represented by afixed subset of A.An adaptive policy is a decision tree.It assumes that the value of each random variable can be observed as soon as it is chosen and it uses the observed values of the previously chosen elements to determine the next element in the subset.We compare these policies by studying the adaptivity gap of the problem.The adaptivity gap is defined as the ratio between the expected values of optimal adaptive and non-adaptive policies.Adaptivity gap has been previously studied for stochastic maximization problems with respect to covering[13]and packing[7,8] constraints.1.2ResultsWe present approximately optimal policies for the stochastic monotone submodular maximization problem. First,in Section2,we compare the performance of the optimal adaptive and non-adaptive policies.Although non-adaptive policies may not perform as well as adaptive ones,they are particularly useful when it is difficult or time consuming to discover the outcome of an element.For example,in the capital budgeting problem, it is not possible for the investor to wait until the end of each project to measure the success,or in the environmental monitoring problem,it is not practical to measure the performance of sensors after placing each sensor in the environment.Surprisingly,we learn that the adaptivity gap of the problem is equal to ee−1≈1.59.In other words,there exists a non-adaptive policy which achieves at least e−1e fraction of the value of best adaptive policy.This result leads to a(e−1e )2≈40%approximation of the optimal adaptive policy by a non-adaptive policythat runs in polynomial time in n.We also give an example to show that our analysis of the adaptivity gap is tight.For that,we use a simple instance of the stochastic max k-cover problem.In Section3,we focus on natural myopic policies.We study the natural extension of the myopic policy studied in[5]in a stochastic environment.This policy iteratively chooses an element with the maximum expected marginal value,conditioned on the outcome of the previous elements.We show that the approximation ratio of this policy with respect to the optimal adaptive policy is12forgeneral matroids.We also prove that over uniform matroid(i.e.,subject to a cardinality constraint),theapproximation ratio of this policy is1−1e .1Due to the results of[19,10],the approximation ratio of1−1eis optimal only if a polynomial number of oracle accesses is allowed.The closest work to ours in the literature is by Chan and Farias[4].They mainly study the problem of stochastic submodular optimization over partition matroids.In their model,there is an ordering over the partitions and any adaptive policy has to choose one element from each partition according to the givenorder.They present a12-approximation of the optimal adaptive policy(that respects the ordering)using amyopic policy.In our setting,we do not have afixed ordering.In addition,we prove most of our results for general matroids.1The results for the uniform matroid has appeared in a preliminary version of this work[3].32The Adaptivity Gap of Stochastic Submodular Optimization ProblemIn this section,we analyze the optimal adaptive and non-adaptive policies and compare the performance of the two.First,observe that since non-adaptive policies do not observe the realized value of the items until the end,they may choose all the elements in one step.In other words,any non-adaptive policy can be represented by the set of chosen elements.On the other hand,an optimal adaptive policy selects the elements based on the realized values of the previously chosen elements.Note that the policy knows the probability distribution of the values of the elements that are not yet chosen,but not their actual values.Although an adaptive policy can clearly perform better than a non-adaptive policy,we show that its advantage is limited.The main result of this section is as follows:Theorem1The adaptivity gap of the stochastic monotone submodular maximization problem is equal to ee−1.In order to prove the above theorem,we start by establishing an upper bound on the adaptivity gap.In Section2.1,we give an example that shows our analysis of the adaptivity gap is tight.Before proving the theorem,observe that since F(S)is a submodular function,we can use the following result of Calinescu et.al.[5]:Theorem2(Calinescu et al[5])Given oracle access to F(see Remark1),there exists a polynomial time algorithm that achieves an approximation ratio of1−1e−o(1).The above theorem immediately implies thatCorollary3A(1−1e −o(1))-approximation of the optimal non-adaptive policy can be computed in polynomialtime.Theorem1and the above corollary imply that:Corollary4There is a policy that is non-adaptive and also runs in polynomial time and computes a solution that is within(e−1e)2of the optimal adaptive policy.In the rest of this section,we prove Theorem1.The proof is inspired by the techniques developed in Section3.5of[21]for submodular optimization(in a non-stochastic setting).For the sake of consistency,we use the same notation as[21]wherever possible.We start by making a few observations about adaptive policies.First,any adaptive policy can be described by a(possibly randomized)decision tree in which at each step an element is being added to the current selection.Consider an arbitrary adaptive policy Adapt.Each path from the root to a leaf of this tree corresponds to a realization s∈ˆI of the sequence of elements chosen by Adapt.Here,ˆI denotes the set of all possible realizations of sets in I.Let y=<y1,···,y n>represent the probability that each element of A is chosen by Adapt,i.e.,y i is the probability of choosing X i.These probabilities sum up to1.Also, letβs denote the probability density function for outcome s∈ˆI.Then,we have the following properties:1.s∈ˆIβs=1.2.∀s:βs≥0.3.∀i,dx:s,s i∈dx iβs ds=y i g i(x i)dx i4Thefirst two properties hold becauseβdefines a probability measure on the space of all feasible outcomes. The third property implies that the probability that we observe outcome x i(a realized value of X i)among all possible outcome s is equal to the probability that X i is chosen(i.e.,y i)multiplied by the probability that the outcome is equal to x i.This property holds because of the independence among the random variables.Since every policy satisfies the above properties,we can establish an upper bound on the value of any adaptive policy.Hence,we define the function f+:[0,1]n→R as follows:f+(y)=supαs∈ˆIαs f(s):sαs=1,αs≥0,∀i,dx i:s,s i∈dx iαs ds=y i g i(x i)dx i.(1)Another observation is that for an optimal adaptive policy,vector y described above is in the base polytope of M(defined as follows).A set S∈I is called a basis for the matroid if|S|=max{|T|:T∈I}. The base polytope,B(M),is defined as:B(M)=conv{1S|S∈I,S is a basis}Here“conv”denotes the convex hull and1S is the characteristic vector of S,i.e.,1for elements in S and 0for other elements.Lemma5The expected value of the optimal adaptive policy is at most max y∈B(M){f+(y)},Proof:Note that an optimal adaptive policy only chooses independent sets.Due to monotonicity,all of these are independent sets are bases of the matroid.Hence,for an optimal adaptive policy vector y defined above is in B(M).Moreover,the expected value of the adaptive policy is bounded by f+(y),because the policy has to satisfy the3properties mentioned earlier.Now,we define an extension of set function F(S)to the domain of real numbers.For vector y∈[0,1]n, let Y denoted a random set where Y includes X i∈A with probability y i.With abuse of notation,we define the extension F:[0,1]n→R+as follows:F(y)=E[F(Y)]=Y is a basis of Ii∈Yy ii/∈Y(1−y i)F(Y).Function f+(y)sets an upper bound on the adaptive policies.We now establish a lower bound on the value of optimal non-adaptive policies via the following lemma from[21](Lemma3.4),which is based on pipage rounding[1].Lemma6[21]Any vector y∈B(M)can be rounded to an integral solution S∈I of value F(S)≥F(y).To complete the proof we need to show that for any vector y,the values of F(y)and f+(y)are within a constant factor of each other,which is established by combining Lemmas3.7and3.8from[21].Lemma7[21]For any monotone submodular function f and any vector y we havef+(y)≤(ee−1)F(y)Proof:[Theorem1]Lemma5shows that max y∈B(M)f+(y)is an upper-bound on the performance of the optimal adaptive policy.Consider y∗∈argmax y∈B(M)f+(y).By Lemma7,we have F(y∗)is at least a(1−1e )fraction of the expected value of an optimal adaptive policy.On the other hand,Lemma6impliesthat there exists a S∈I such that F(S)≥F(y∗).Note that F(S)is in fact the expected value gained by anon-adaptive policy that selects set S.Hence,S is a(1−1e )-approximation of the optimal adaptive policy.By Proposition10in the next section,this factor is tight.52.1A Tight Example:Stochastic Maximum k -CoverGiven a collection A of the subsets of N ={1,2,···,n },the goal of the max k -cover problem is to find k subsets from A such that their union has the maximum cardinality [10].In the stochastic version,the subset that an element of A would cover is revealed only after choosing the element,according to a given probability distribution.The following reduction shows that this problem is a special case of the stochastic monotone submodular maximization.For S ∈A ,let F (S )denote the expected number of elements covered by the subsets in S .Clearly,F is monotone.Consider two subsets S ⊆T ⊆A ,an element X ∈A ,and a realization y of an arbitrary subset of A .Because ∪A ∈S A ⊆∪B ∈T B ,for every realization y ,we have F (S +X )−F (S )≥F (T +X )−F (T ).In addition,M =(A ,{S ⊆A :|S |≤k })forms a uniform matroid.Therefore,the stochastic max k -cover problem is in fact a stochastic monotone submodular maximization problem.In this section,we define an instance of stochastic max k -cover problem that gives a lower bound on the adaptivity gap.This example has been brought to our attention by Vondr´a k [22].Consider the following instance:a ground set N ={1,2,···,n }and a collection A ={X (i )j |1≤i ≤n,1≤j ≤n 2}of its subsets are given.For every i,j ,define X (i )j to be the one-element subset {i }withprobability 1n and the empty set with probability 1−1n .The goal is to cover the maximum number of the elements of N by selecting at most k =n 2subsets from A .Lemma 8The optimal non-adaptive policy is to pick n subsets from each of the collections A (i )={X (i )j |1≤j ≤n 2}for every i .For large enough values of n ,the expected value of this policy is (arbitrarily close to)(1−1e )n .Proof :Consider an arbitrary non-adaptive policy which picks S ,containing n 2sets from A .For each i ,define k i =|S ∩A (i )|.Moreover,each element i ∈N is covered if and only if at least one of its correspondingchosen subsets are realized as a non-empty subset.Hence,it willbe covered with probability 1−(1−1n )k i .Therefore,the expected value of this policyis i 1−(1−1n )k i .Note that 1−(1−1n )x is a concave function with respect to x ,and also i k i =n 2.Hence,the expected value of the policy is maximized whenk 1=k 2=···=k n =n .In this case,the expected value is (1−(1−1n )n )n ≈(1−1e )n for large n .We now consider the following myopic adaptive policy P :Start with i =1and pick the elements of A (i )one by one until one of them is realized as {i }or all of elements in A (i )are chosen.Then increase i by one.Continue the iteration untill i =n +1.The following lemma gives a lower bound on the number of elements in N covered by the adaptive policy.Lemma 9The expected number of elements in N covered by P described above is (1−o (1))n .Proof :Let X k be the indicator random variable corresponding to the event that the subset chosen at the k -th step is realized as a non-empty subset for any 1≤k ≤n 2.Note that the number of elements covered by P is n 2k =1X k .Moreover,all X k ’s are independent random variables.By the description of P ,as long as k i =1X k <n 2,X k will be one with probability 1n and will be zero with probability 1−1n .Also,when t i =1X k =n ,we have already covered all the elements in N .Therefore,X t +1,···,X n 2will all be equal to zero.With this observation,we define i.i.d random variablesY 1,Y 2,···,Y n 2,where each Y i is set to be one with probability 1n and zero with probability 1n .Observe thatmin {n,Y = k Y k }has the same probability distribution as k X k .Note that E[Y ]=n .Using Chernoffbound,we havePr[Y ≤n −n 2/3]≤e −n 4/32n =e −n 1/3.Thus,with probability at least 1−e −n1/3we have Y >n −n 2/3.Hence,E[n 2 k =1X k ]=E[min {n,Y }]≥(1−e −n 1/3)(n −n 2/3)=n −o (n ),6The myopic adaptive policy:Initialize t=0,S0=∅,U0=∅While(A=U t∪S t)t←t+1S t←S t−1RepeatSelect X i∈argmax Xi ∈A\(U t−1∪S t−1)E[F(S t−1+X i)|s t−1]If S t−1∪{X i}/∈I thenU t←U t−1∪{X i}elseS t←S t−1∪{X i}U t←U t−1Observe x i and update s tUntil(A=U t−1∪S t−1)or(S t=S t−1)which completes the proof of the lemma.By combining the results of Lemmas8and9we have the following proposition:Proposition10For large enough n,the adaptivity gap of stochastic maximum coverage is at least ee−1.3Approximation Ratio of Simple Myopic PoliciesIn this section,we present an adaptive myopic policy with an approximation ratio of12with respect to anoptimal adaptive policy.In Section3.1,we show that the myopic policy achieves the approximation ratio of1−1e if the matroid is uniform.Note that even if the actual values were known,the problem of computingthe optimal policy is intractable.As mentioned before,the maximum k-cover is a special case of our problemand Feige[10]has shown that it is not possible tofind an approximation ratio better than1−1e for themaximum k-cover problem,unless NP⊂T IME(n O(log log n)).The policy is given in the abovefigure.At each iteration,from the elements in A that are not yet considered,the policy chooses an element with the maximum expected marginal value.We denote by S t the set of elements chosen by the adaptive policy up to iteration t.Let s t denote the realization of all these elements.Also,U t is the set of elements considered but not chosen by the policy due to the matroid constraint.Here is the main result of this section.Theorem11For general matroids,the approximation ratio of the myopic adaptive policy with respect to any optimal adaptive policy is12.Define∆t=F(S t)−F(S t−1).Also,let k be the number of elements chosen by the myopic policy(which is simply the rank of the matroid M).The basic idea of the proof is similar to Fisher et al.[12].But, the main difficulty is that the realized values of∆t are not always decreasing(due to the stochastic nature of the problem).In addition,the sequence of elements chosen by the optimal adaptive policy is random.7However,E[∆t|s t−1]≥E[∆t+1|s t−1](Note that E[∆t|s t]≥E[∆t+1|s t]does not necessary hold).Based on this observation,we prove the theorem.We will also use the following lemma from[12].Lemma12[12]For t=1,···,k,we have ti=1|C i|≤t.Note that T,U,and S are random sets,but the lemma holds for every realization because it is a consequence of the matroid constraint,not the realizations of the element chosen by the policy.We are now ready to prove the theorem.Proof:[Theorem11]Let P be the(random)set of elements chosen by the optimal adaptive policy. Also,for t=1,···,k,define C t=P∩(U t+1\U t).Consider a realization s t of S t.Because F is stochastic monotone submodular we haveE[F(P)|s t]≤E[l∈P\SF(S+l)|s t]The expectations,and in the rest of the proof,are taken over the probability distribution of all realizations of P such that the realized values of elements in P∩S t are according to s t.Since the above inequality holds for all s t,we haveE[F(P)]≤E[l∈P\SF(S+l)]E[F(P)]−E[F(S)]≤E[l∈P\S(F(S+l)−F(S))]Note that kt=1C t=P\S.Hence,E[F(P)]−E[F(S)]≤kt=1E[l∈C t(F(S+l)−F(S))]By expanding the expectation we haveE[F(P)]−E[F(S)]≤kt=1s t−1:S t−1∈IE[l∈C tF(S+l)−F(S)|s t−1]Pr[s t−1]ds t−1(2)Observe that conditioned on s t−1,because the myopic policy chooses an element with the maximum marginal value,we have∆t≥F(S+l)−F(S),l∈C t.Therefore,E[l∈C t F(S+l)−F(S)|s t−1]≤E[l∈C t∆t|s t−1]By plugging the above inequality into(2),we getE[F(P)]−E[F(S)]≤kt=1s t−1:S t−1∈IE[l∈C t∆t|s t−1]Pr[s t−1]ds t−1Using telescopic sums and the linearity of expectation we derive the following.Here∆k+1is defined0.E[F(P)]−E[F(S)]≤kt=1s t−1:S t−1∈IE[l∈C tkj=t(∆j−∆j+1)|s t−1]Pr[s t−1]ds t−1=kj=1jt=1s t−1:S t−1∈IE[l∈C t(∆j−∆j+1)|s t−1]Pr[s t−1]ds t−18Note that by using the Bayes’theorem and the law of total probability,for every t and j the integral term in the above is in fact equal to E[ l ∈C t (∆j −∆j +1)].Now,we can change the probability measure to calculate this expectation from s t −1to s j −1.Hence,we haveE[F (P )]−E[F (S )]≤kj =1j t =1 s j −1:S j −1∈I E[ l ∈C t(∆j −∆j +1)|s j −1]Pr[s j −1]ds j −1=kj =1j t =1 s j −1:S j −1∈I (E [|C t |E[∆j −∆j +1|s j −1]|s j −1])Pr[s j −1]ds j −1Note that conditioned on s j −1,the term E[∆j −∆j +1|s j −1]is by definition a constant and we can take it out from the outer expectation.Hence,E[F (P )]−E[F (S )]≤k j =1 s j −1:S j −1∈I E[j t =1|C t ||s j −1]E[∆j −∆j +1|s j −1] Pr[s j −1]ds j −1We now use Lemma 12which implies that in every realization j t =1|C t |≤j .We also use the fact that dueto the submodularity and the rule of the policy,we have E[(∆j −∆j +1)|s j −1]≥0.We conclude thatE[F (P )]−E[F (S )]≤kj =1 s j −1:S j −1∈I j E[(∆j −∆j +1)|s j −1]Pr[s j −1]ds j −1=kj =1 s j −1:S j −1∈I E[∆j |s j −1]Pr[s j −1]ds j −1=k j =1E[∆j ]=E[F (S )]Therefore,E[F (P )]≤2E[F (S )],as desired.Fisher et al.[12]have shown that even in the non-stochastic setting,in the worst-case,the approximation ratio of the greedy algorithm (hence the myopic policy)is equal to 12.Also,it is easy to see that that if Mis an intersection of κmatroids,then the approximation ratio of the myopic policy is equal to 11+κ.3.1Uniform MatroidsIn this section we show that the myopic policy described in the previous section has a better approximation ratio if the matroid is uniform.Theorem 13Consider the adaptive myopic policy that at each step selects an element with the maximum marginal value,conditioned on the realized value of the previously chosen elements.Over uniform matroids,the approximation ratio of this policy compared to the optimal adaptive policy is 1−1e .The proof presented here is similar to the proof of Kleinberg et al.[15]for submodular set functions.The main technical difficulty in our case is that the optimal adaptive policy here is a random set whose distribution depends on the realized values of the elements of A .Proof :Let P denote the (random)set chosen by an optimal adaptive policy.Also,denote the marginal value of the t -th element chosen by the myopic policy by ∆t ,i.e.,∆t =F (S t )−F (S t −1)9。