Droplet coalescence by geometrically mediated flow
LES of steady spray flame and ignition sequences in aeronautical combustors
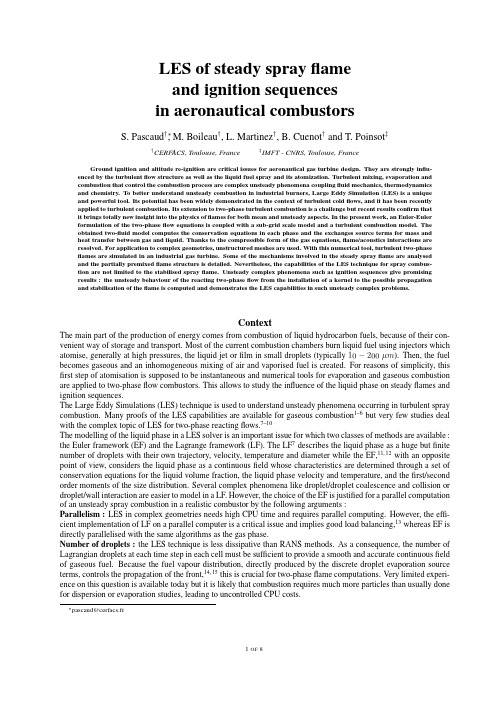
LES of steady sprayflameand ignition sequencesin aeronautical combustorsS.Pascaud†∗,M.Boileau†,L.Martinez†,B.Cuenot†and T.Poinsot‡†CERFACS,Toulouse,France‡IMFT-CNRS,Toulouse,FranceGround ignition and altitude re-ignition are critical issues for aeronautical gas turbine design.They are strongly influ-enced by the turbulentflow structure as well as the liquid fuel spray and its atomization.Turbulent mixing,evaporation and combustion that control the combustion process are complex unsteady phenomena couplingfluid mechanics,thermodynamics and chemistry.To better understand unsteady combustion in industrial burners,Large Eddy Simulation(LES)is a unique and powerful tool.Its potential has been widely demonstrated in the context of turbulent coldflows,and it has been recently applied to turbulent combustion.Its extension to two-phase turbulent combustion is a challenge but recent results confirm that it brings totally new insight into the physics offlames for both mean and unsteady aspects.In the present work,an Euler-Euler formulation of the two-phaseflow equations is coupled with a sub-grid scale model and a turbulent combustion model.The obtained two-fluid model computes the conservation equations in each phase and the exchanges source terms for mass and heat transfer between gas and liquid.Thanks to the compressible form of the gas equations,flame/acoustics interactions are resolved.For application to complex geometries,unstructured meshes are used.With this numerical tool,turbulent two-phase flames are simulated in an industrial gas turbine.Some of the mechanisms involved in the steady sprayflame are analysed and the partially premixedflame structure is detailed.Nevertheless,the capabilities of the LES technique for spray combus-tion are not limited to the stabilised sprayflame.Unsteady complex phenomena such as ignition sequences give promising results:the unsteady behaviour of the reacting two-phaseflow from the installation of a kernel to the possible propagation and stabilisation of theflame is computed and demonstrates the LES capabilities in such unsteady complex problems.ContextThe main part of the production of energy comes from combustion of liquid hydrocarbon fuels,because of their con-venient way of storage and transport.Most of the current combustion chambers burn liquid fuel using injectors which atomise,generally at high pressures,the liquid jet orfilm in small droplets(typically10−200µm).Then,the fuel becomes gaseous and an inhomogeneous mixing of air and vaporised fuel is created.For reasons of simplicity,this first step of atomisation is supposed to be instantaneous and numerical tools for evaporation and gaseous combustion are applied to two-phaseflow combustors.This allows to study the influence of the liquid phase on steadyflames and ignition sequences.The Large Eddy Simulations(LES)technique is used to understand unsteady phenomena occurring in turbulent spray combustion.Many proofs of the LES capabilities are available for gaseous combustion1–6but very few studies deal with the complex topic of LES for two-phase reactingflows.7–10The modelling of the liquid phase in a LES solver is an important issue for which two classes of methods are available: the Euler framework(EF)and the Lagrange framework(LF).The LF7describes the liquid phase as a huge butfinite number of droplets with their own trajectory,velocity,temperature and diameter while the EF,11,12with an opposite point of view,considers the liquid phase as a continuousfield whose characteristics are determined through a set of conservation equations for the liquid volume fraction,the liquid phase velocity and temperature,and thefirst/second order moments of the size distribution.Several complex phenomena like droplet/droplet coalescence and collision or droplet/wall interaction are easier to model in a LF.However,the choice of the EF is justified for a parallel computation of an unsteady spray combustion in a realistic combustor by the following arguments:Parallelism:LES in complex geometries needs high CPU time and requires parallel computing.However,the effi-cient implementation of LF on a parallel computer is a critical issue and implies good load balancing,13whereas EF is directly parallelised with the same algorithms as the gas phase.Number of droplets:the LES technique is less dissipative than RANS methods.As a consequence,the number of Lagrangian droplets at each time step in each cell must be sufficient to provide a smooth and accurate continuousfield of gaseous fuel.Because the fuel vapour distribution,directly produced by the discrete droplet evaporation source terms,controls the propagation of the front,14,15this is crucial for two-phaseflame computations.Very limited experi-ence on this question is available today but it is likely that combustion requires much more particles than usually done for dispersion or evaporation studies,leading to uncontrolled CPU costs.∗pascaud@cerfacs.frSize distribution:since the spray granulometry controls theflame regime,a droplet size distribution must be consid-ered.LF is better on this question because it naturally discretises droplets with different sizes.However,recent studies demonstrate the EF capabilities to include polydispersed sprays.16–18Inlet conditions:due to the atomisation complexity,the accurate determination of the spray characteristics is a criti-cal issue.Even if LF calculates droplet trajectories with precision,very approximate injection conditions will lead to rough results.Close to the injector,the liquid spray is organised as dense blobs19and LF can not be applied to these high-loaded zones.As a contrary,EF is more compatible with the physics of liquid injection.Numerical toolThe solver AVBP,developed at CERFACS,is a parallel fully compressible code which computes the turbulent react-ing two-phaseflows,on both structured and unstructured grids,for complex industrial applications such as ignition sequences or acoustic instabilities.Turbulent combustion modelling is ensured by the Dynamically Thickened Flame model,20using a thickening factor F and an efficacity function E to determine theflame front turbulent velocity.21 Subgrid scale turbulent viscosity is defined by the W ALE model,22derived from the classic Smagorinsky model. The Euler/Euler framework governing a turbulent reacting two-phaseflow is composed,for each phase,of a set of conservative equations defined by Eq.(1)and Eq.(2)and solved with the same numerical approach.Carrier phase ∂w∂t+∇·F=s(1)Dispersed phase ∂w l∂t+∇·F l=s l(2)For the carrier phase,the vector of conservative variables is defined by Eq.(3)withρthe density,(u1,u2,u3)the velocity components,E t the total non chemical energy and Y k the fuel mass fractions.Theflux tensor F is composed of viscous,inviscid and subgrid scale components and s is the source term defined by Eq.(4).Combustion terms are the reaction rate˙ωk and the heat release˙ωT modelled by an Arrhenius law.23Additional source terms representing exchanges between phases are the mass transferΓ,the momentum transfer I i and the enthalpy transferΠ.w=(ρ˜u1,ρ˜u2,ρ˜u3,ρ˜E t,ρ˜Y k)(3)s=(I1,I2,I3,EF˙ωT+I i˜u i+Π+˙ωspark,−EF˙ωk+ΓδkF)(4)For the dispersed phase,the vector of conservative variables w l is defined by Eq.(5)withαl the volume fraction, (u1,l,u2,l,u3,l)the velocity components,h s,l the sensible enthalpy and n l the droplet number density.Theflux tensor F l is only composed of convective terms and the source term s is defined by Eq.(6).w l=(αlρl,αlρl˜u1,l,αlρl˜u2,l,αlρl˜u3,l,αlρl˜h s,l,n l)(5)s l=(−Γ,−I1,−I2,−I3,−Π,0)(6) The fully explicitfinite volume solver AVBP uses a cell-vertex discretisation and a second order time and space Lax-Wendroff centred numerical scheme.24Characteristic boundary conditions NSCBC25are used.ConfigurationThe computed configuration is a3D sector of22.5-degrees of an annular aeronautical gas turbine at atmospheric pressure.The kerosene liquid spray LS is located at the center of the main swirled inlet SI(Fig.1).An annular series of small holes H are located around the inlet to lift theflame and protect the injector from high temperatures.Then, several holes on the upper and lower walls are divided in two parts.Thefirst part of the combustor where combustion takes place is located between the injector and the primary jets PJ,which bring cold air to theflame.The second part called dilution zone is located between PJ and dilution jets DJ,that reduce and homogenise the outlet temperature to protect the turbine.The spark plug SP is located under the upper wall between two PJ(Fig.2).The geometry(Fig.3) also includes coolingfilms which protect upper and lower walls from theflame.The inlet and outlet boundary conditions are characteristic with relaxation coefficients to reduce reflexion.26The SI imposed velocityfield mimics the swirler influence.The other inlets are simple non-swirled jets.Non-slip conditions are used on the upper and lower walls while symmetry condition is used on the chamber sides.15µm-droplets are injected at the SI center through a specific condition which specifies a liquid volumic fractionαl 10−3.The droplets at288K are heated by the air at525K.The initial liquid velocity is equal to the gaseous velocity as the droplet Stokes number,based on the droplet relaxation time,is lower than one.The unstructured mesh is composed of 400000nodes and 2300000tetrahedra.The explicit time step is ∆t 0.22µs .The mesh is refined close to the inlets and in the combustion zone (Fig.4).The Arrhenius coefficients are fitted by a genetic algorithm 27from a reduced chemistry 28to the present one-step chemistry :JP 10+14O 2 10CO 2+8H 2O using criteria such as flame speed andthickness.Fig.1Geometry sketch :sideview Fig.2Geometry sketch :topviewFig.3Complexgeometry Fig.4Mesh refinement :central longitudinal viewSteady spray flamePrecessing Vortex CoreIn its review on vortex breakdown,Lucca-Negro 29classifies the hydrodynamic instabilities appearing in swirled flows.For high swirl numbers,the axial vortex breaks down at the stagnation point S and a spiral is created around a central recirculation zone CRZ (Fig.5):this vortex breakdown is the so-called precessing vortex core (PVC)existing in a large number of combustors.30The LES technique capture the vortex breakdown in the combustor and its frequency is evaluated with the backflow line on a transverse plane (Fig.6)at six successive times marked with a number from 1to 6and separated by 0.5ms.The turnover time is estimated at τswirl 3.5ms,corresponding to a frequency ofDispersion and evaporationThe 15µm droplets motion follows the carrier phase dynamics so that the CRZ of both zones are similar,as illustrated by both backflow lines on Fig.7.Maintained by this CRZ,the droplets accumulate and the droplet number density,presented with the liquid volumic fraction field on Fig.7,rises above its initial value.Increasing the residence time of these vaporising droplets,whose diameter field is presented on Fig.8,makes the local equivalence ratio distribution reach values higher than 10.The heat transfer linked to the phase change leads to the reduction of the gaseous temperature,as shown by the isoline T =450K on Fig.9,and an increase of the dispersed phase temperature.Thus,the CRZ,by trapping evaporating droplets,stabilise the vaporised fuel and the flame.Fig.7Dispersion Fig.8EvaporationCombustionThe flame front,illustrated on Fig.9by the heat release field,is influenced by both flow dynamics and evaporation rate.The main competitive phenomena for two-phase flame stabilisation are :1.the air velocity must be low enough to match the turbulent flame velocity :the dynamics of the carrier phase (and in particular the CRZ)stabilise the flame front on a stable pocket of hot gases2.zones where the local mixture fraction is within flammability limits must exist :combustion occurs between the fuel vapour radially dispersed by the swirl and the ambient air,where the equivalence ratio is low enough3.the heat release must be high enough to maintain evaporation and reaction :the sum of heat flux Πand heatrelease ˙ωT ,plotted on Fig.9,allows to identify the zone ()where the heat transfer due to evaporation extinguishes the flame :Π+˙ωT =0.In the present case,the flame front is stabilised by the CRZ (1.)but the heat release magnitude is reduced in the evaporation zone because of both effects (2.)and (3.).To determine the flame regime (premixed and/or diffusion),the Takeno index T =∇Y F .∇Y O and an indexed reaction rate ˙ω∗F =˙ωF T |∇Y F |.|∇Y O |are used.The flame structureis then divided into two parts :˙ω∗F =+˙ωF in the premixed regime part and ˙ω∗F =−˙ωF in the diffusion regime part(Fig.10).In the primary zone,the partially premixed regime is preponderant because of the unsteady inhomogeneous fuel vapour.In the dilution zone,the unburned fuel reacts with dilution jets through a diffusion flame,as confirmed by the coincidence between the flame and the stoichiometric line.Fig.9Flamefront Fig.10Flame structurePVC influenceThe PVC,defined on Fig.11a,controls the motions of both the vaporised fuel VF and theflame front.The cut plane, defined on Fig.11a,is presented on Fig.11b with the temperaturefield,the maximum fuel mass fraction(white lines) and theflame front(black isolines of reaction rate˙ωF).The CRZ stabilises hot gases and enhance evaporation leading to a cold annular zone where the maximum fuel mass fraction precesses.Theflame motion follows the PVC and the reaction rate is driven by the fuel vapour concentration.b.Fig.11PVC influence on evaporation and combustionIgnition sequenceSpark-like numerical methodThe numerical method used to mimic an ignition by spark plug in the combustion chamber is the addition of the source term ˙ωspark in Eq.(4).This source term,defined by Eq.(7),is a gaussian function located at (x 0,y 0,z 0)near the upper wall between both primary jets and deposited at t =t 0=0.The ignition delay,typical of industrial spark plugs,is σt =0.16ms .˙ωspark =E spark (2π)2σt σr 3e −12 (t −t 0σt )2+(x −x 0σr )2+(y −y 0σr )2+(z −z 0σr )2 (7)Temporal evolutionThe temporal evolution of the spark ignition is presented on Fig.12where the source term ˙ωspark ,the maximum heat release ˙ωT and the maximum temperature are plotted.First,the maximum temperature rises smoothly because of the source term on energy equation.When this temperature is sufficient,the reaction occurs between fuel vapour and air leading to a sudden increase of the heat release of the exothermic reaction and then,the maximum temperature rapidly rises.Once the source term is over,the maximum heat release decreases.The maximum temperature corresponds to the hot gases :the ignition is successful.15105ωspark 0.50.40.30.20.10.0time [ms]45004000350030002500200015001000500T [K]6420ωT [kg.mm -3.s -1] Source term ωspark Maximum heat release ωTMaximum temperatureFig.12Source term ˙ωspark ,maximum heat release ˙ωT ,maximum temperatureThe sequence of ignition is illustrated on the longitudinal central cut plane on Fig.13with the fuel mass fraction field and the reaction rate isolines,where the first image is presented at t =0.2ms and after,successive images are separated by ∆t =0.2ms .At the beginning of the computation,the 15µm droplets evaporate in the ambient air at T =525K creating a turbulent cloud of vaporised fuel in the whole primary zone.This fuel vapour distribution,whose stabilisation is ensured by the CRZ,propagates from the evaporation zone to the spark plug area.At t =0,the spark ignition occurs leatding to the creation of a hot kernel.The propagation of the flame front created by this pocket of hot gases is highly controlled by the fuel vapour distribution between t =0and t =1ms .Once the flame front reaches the CRZ (t 1ms ),there is no more vaporised fuel and the flame must evaporate the fuel droplets leading to an increase of the maximum fuel mass fraction in the CRZ.This evaporation process stabilise the flame front as explained in the previous section on steady spray flame.0.2ms 0.4ms 0.6ms 0.8ms1.0ms 1.2ms 1.4ms 1.6ms1.8ms2.0ms 2.2ms 2.4msFig.13Flame front propagation on fuel mass fraction field (white :0→black :0.35)ConclusionsA steady spray flame in a realistic aeronautical combustor has been computed using the parallel LES Euler/Euler solver A VBP.The influence of the dispersed phase on the flame motion has been highlighted,in particular the role of the evaporation process.The unsteady approach brings totally new insight into the physics of such complex reactive two-phase flows.Furthermore,it allows the computation of an ignition sequence from the formation of the first spherical flame front to the stabilisation of the turbulent spray flame.To conclude,the LES technique is a powerful tool to mimic ignition sequences and understand turbulent spray flame structure in realistic combustors.References1P.E.Desjardins and S.H.Frankel.Two dimensional large eddy simulation of soot formation in the nearfield of a strongly radiating nonpremixed acetylene-air jetflbust.Flame,119(1/2):121–133,1999.2O.Colin.Simulation aux Grandes Echelles de la Combustion Turbulente Pr´e m´e lang´e e dans les Stator´e acteurs.PhD thesis,INP Toulouse,2000. 3C.Angelberger,F.Egolfopoulos,and rge eddy simulations of chemical and acoustic effects on combustion instabilities.Flow Turb.and Combustion,65(2):205–22,2000.4H.Pitsch and L.Duchamp de la rge eddy simulation of premixed turbulent combustion using a level-set approach.Proceedings of the Combustion Institute,29:in press,2002.5C.D.Pierce and P.Moin.Progress-variable approach for large eddy simulation of non-premixed turbulent combustion.J.Fluid Mech.,504:73–97,2004.6L.Selle.Simulation aux grandes´e chelles des couplages acoustique/combustion dans les turbines´a gaz.Phd thesis,INP Toulouse,2004.7K.Mahesh,G.Constantinescu,S.Apte,G.Iaccarino,F.Ham,and P.Moin.Progress towards large-eddy simulation of turbulent reacting and non-reactingflows in complex geometries.In Annual Research Briefs,pages115–142.Center for Turbulence Research,NASA Ames/Stanford Univ.,2002.8K.Mahesh,G.Constantinescu,and P.Moin.A numerical method for large-eddy simulation in complex put.Phys., 197:215–240,2004.9S.V.Apte,M.Gorokhovski,and rge-eddy simulation of atomizing spray with stochastic modeling of secondary breakup.In ASME Turbo Expo2003-Power for Land,Sea and Air,Atlanta,Georgia,USA,2003.10F.Ham,S.V.Apte,G.Iaccarino,X.Wu,M.Herrmann,G.Constantinescu,K.Mahesh,and P.Moin.Unstructured les of reacting multiphase flows in realistic gas turbine combustors.In Annual Research Briefs-Center for Turbulence Research,2003.11R.V.R.Pandya and F.Mashayek.Two-fluid large-eddy simulation approach for particle-laden turbulentflows.Int.J.of Heat and Mass transfer, 45:4753–4759,2002.12E.Riber,M.Moreau,O.Simonin,and B.Cuenot.Towards large eddy simulation of non-homogeneous particle laden turbulent gasflows using euler-euler approach.In11th Workshop on Two-Phase Flow Predictions,Merseburg,Germany,2005.13M.Garc´ıa,Y.Sommerer,T.Sch¨o nfeld,and T.Poinsot.Evaluation of euler/euler and euler/lagrange strategies for large eddy simulations of turbulent reactingflows.In ECCOMAS Thematic Conference on Computational Combustion,2005.14H.Pitsch and N.Peters.A consistentflamelet formulation for non-premixed combustion considering differential diffusion bust. Flame,114:26–40,1998.15L.Selle,rtigue,T.Poinsot,P.Kaufman,W.Krebs,and rge eddy simulation of turbulent combustion for gas turbines with reduced chemistry.In Proceedings of the Summer Program-Center for Turbulence Research,pages333–344,2002.16R.Borghi.Background on droplets and sprays.In Combustion and turbulence in two phaseflows,Lecture Series1996-02.V on Karman Institute for Fluid Dynamics,1996.17O.Delabroy,cas,begorre,and J-M.Samaniego.Param`e tres de similitude pour la combustion diphasique.Revue G´e n´e rale de Thermique,(37):934–953,1998.18J.R´e veillon,M.Massot,and C.Pera.Analysis and modeling of the dispersion of vaporizing polydispersed sprays in turbulentflows.In Proceedings of the Summer Program2002-Center for Turbulence Research,2002.19R.D.Reitz and R.Diwakar.Structure of high pressure fuel sprays.Technical Report Tech.Rep.870598,SAE Technical Paper,1987.20J.-Ph.L´e gier,T.Poinsot,and D.Veynante.Dynamically thickenedflame large eddy simulation model for premixed and non-premixed turbulent combustion.In Summer Program2000,pages157–168,Center for Turbulence Research,Stanford,USA,2000.21O.Colin,F.Ducros,D.Veynante,and T.Poinsot.A thickenedflame model for large eddy simulations of turbulent premixed combustion.Phys. Fluids,12(7):1843–1863,2000.22F.Nicoud and F.Ducros.Subgrid-scale stress modelling based on the square of the velocity gradient.Flow Turb.and Combustion,62(3):183–200,1999.23T.Poinsot and D.Veynante.Theoretical and numerical combustion,second edition.R.T.Edwards,2005.24C.Hirsch.Numerical Computation of Internal and External Flows.John Wiley&Sons,1989.25T.Poinsot and S.Lele.Boundary conditions for direct simulations of compressible viscousflput.Phys.,101(1):104–129,1992. 26L.Selle,F.Nicoud,and T.Poinsot.The actual impedance of non-reflecting boundary conditions:implications for the computation of resonators. AIAA Journal,42(5):958–964,2004.27C.Martin.Eporck user guide v1.8.Technical report,CERFACS,2004.28S.C.Li,B.Varatharajan,and F.A.Williams.The chemistry of jp-10ignition.AIAA,39(12):2351–2356,2001.29O.Lucca-Negro and T.O’Doherty.V ortex breakdown:a review.Prog.Energy Comb.Sci.,27:431–481,2001.30L.Selle,rtigue,T.Poinsot,R.Koch,K.-U.Schildmacher,W.Krebs,B.Prade,P.Kaufmann,and pressible large-eddy simulation of turbulent combustion in complex geometry on unstructured bust.Flame,137(4):489–505,2004.。
人工智能原理MOOC习题集及答案 北京大学 王文敏
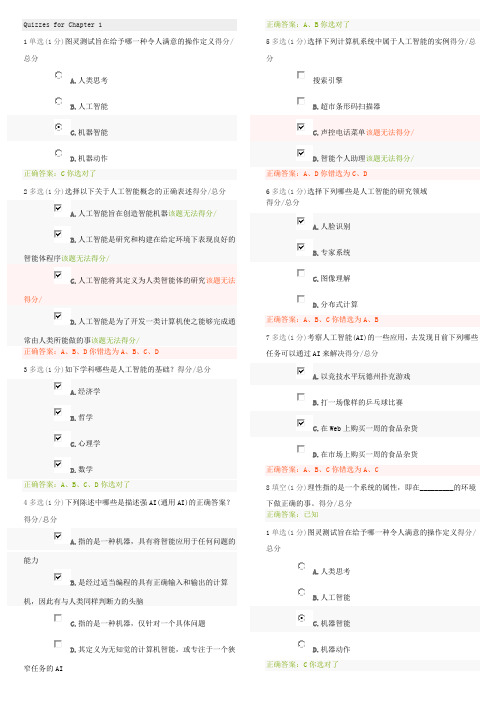
Quizzes for Chapter 11单选(1分)图灵测试旨在给予哪一种令人满意的操作定义得分/总分A.人类思考B.人工智能C.机器智能D.机器动作正确答案:C你选对了2多选(1分)选择以下关于人工智能概念的正确表述得分/总分A.人工智能旨在创造智能机器该题无法得分/B.人工智能是研究和构建在给定环境下表现良好的智能体程序该题无法得分/C.人工智能将其定义为人类智能体的研究该题无法得分/D.人工智能是为了开发一类计算机使之能够完成通常由人类所能做的事该题无法得分/正确答案:A、B、D你错选为A、B、C、D3多选(1分)如下学科哪些是人工智能的基础?得分/总分A.经济学B.哲学C.心理学D.数学正确答案:A、B、C、D你选对了4多选(1分)下列陈述中哪些是描述强AI(通用AI)的正确答案?得分/总分A.指的是一种机器,具有将智能应用于任何问题的能力B.是经过适当编程的具有正确输入和输出的计算机,因此有与人类同样判断力的头脑C.指的是一种机器,仅针对一个具体问题D.其定义为无知觉的计算机智能,或专注于一个狭窄任务的AI正确答案:A、B你选对了5多选(1分)选择下列计算机系统中属于人工智能的实例得分/总分搜索引擎B.超市条形码扫描器C.声控电话菜单该题无法得分/D.智能个人助理该题无法得分/正确答案:A、D你错选为C、D6多选(1分)选择下列哪些是人工智能的研究领域得分/总分A.人脸识别B.专家系统C.图像理解D.分布式计算正确答案:A、B、C你错选为A、B7多选(1分)考察人工智能(AI)的一些应用,去发现目前下列哪些任务可以通过AI来解决得分/总分A.以竞技水平玩德州扑克游戏B.打一场像样的乒乓球比赛C.在Web上购买一周的食品杂货D.在市场上购买一周的食品杂货正确答案:A、B、C你错选为A、C8填空(1分)理性指的是一个系统的属性,即在_________的环境下做正确的事。
得分/总分正确答案:已知1单选(1分)图灵测试旨在给予哪一种令人满意的操作定义得分/总分A.人类思考B.人工智能C.机器智能D.机器动作正确答案:C你选对了2多选(1分)选择以下关于人工智能概念的正确表述得分/总分A.人工智能旨在创造智能机器该题无法得分/B.人工智能是研究和构建在给定环境下表现良好的智能体程序该题无法得分/C.人工智能将其定义为人类智能体的研究该题无法得分/D.人工智能是为了开发一类计算机使之能够完成通常由人类所能做的事该题无法得分/正确答案:A、B、D你错选为A、B、C、D3多选(1分)如下学科哪些是人工智能的基础?得分/总分A.经济学B.哲学C.心理学D.数学正确答案:A、B、C、D你选对了4多选(1分)下列陈述中哪些是描述强AI(通用AI)的正确答案?得分/总分A.指的是一种机器,具有将智能应用于任何问题的能力B.是经过适当编程的具有正确输入和输出的计算机,因此有与人类同样判断力的头脑C.指的是一种机器,仅针对一个具体问题D.其定义为无知觉的计算机智能,或专注于一个狭窄任务的AI正确答案:A、B你选对了5多选(1分)选择下列计算机系统中属于人工智能的实例得分/总分搜索引擎B.超市条形码扫描器C.声控电话菜单该题无法得分/D.智能个人助理该题无法得分/正确答案:A、D你错选为C、D6多选(1分)选择下列哪些是人工智能的研究领域得分/总分A.人脸识别B.专家系统C.图像理解D.分布式计算正确答案:A、B、C你错选为A、B7多选(1分)考察人工智能(AI)的一些应用,去发现目前下列哪些任务可以通过AI来解决得分/总分A.以竞技水平玩德州扑克游戏B.打一场像样的乒乓球比赛C.在Web上购买一周的食品杂货D.在市场上购买一周的食品杂货正确答案:A、B、C你错选为A、C8填空(1分)理性指的是一个系统的属性,即在_________的环境下做正确的事。
商务数据挖掘与分析应用考核试卷

4. 在关联规则挖掘中,如果项集{X}的出现次数除以总项集的次数大于某个阈值,则称项集{X}具有高______度。
5. 在聚类分析中,______算法是基于距离的聚类方法,它试图找到最小化簇内距离和的最大化簇间距离的簇。
6. 在时间序列分析中,______模型是一种预测方法,它假设未来的值可以通过过去的值来预测。
得分:_________________ 判卷人:_________________
三、填空题(本题共10小题,每小题2分,共20分,请将正确答案填到题目空白处)
1. 在数据挖掘中,______是描述数据集中数据分布的统计量。
2. 在进行数据预处理时,______是指识别或删除数据集中的错误或不一致的过程。
得分:_________________ 判卷人:_________________
二、多选题(本题共20小题,每小题1.5分,共30分,在每小题给出的四个选项中,至少有一项是符合题目要求的)
1. 数据挖掘过程中常用的数据分析方法包括哪些?( )
A. 描述性分析
B. 探索性分析
C. 验证性分析
D. 预测性分析
A. 决策树
B. 逻辑回归
C. K-means
D. 支持向量机
14. 以下哪个算法常用于异常值检测?( )
A. 基于距离的聚类
B. 基于密度的聚类
C. 箱线图
D. 以上都是
15. 以下哪个不是数据挖掘中的数据类型?( )
A. 分类数据
B. 数值数据
C. 序列数据
D. 文本数据
16. 在商务数据分析中,以下哪个不是客户关系管理(CRM)的关键指标?( )
Shapes, contact angles, and line tensions of droplets on cylinders
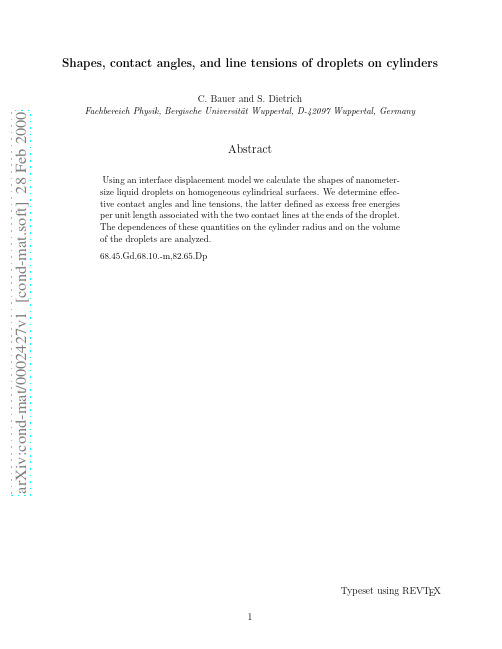
Typeset using REVTEX 1
I. INTRODUCTION
The wetting properties of a fiber in liquid matrices (e.g., dye mixtures, polymer melts, or molten resins) play an important role in the textile industry and in the fabrication of highperformance, fiber-reinforced composite materials. Since contact angles of liquid droplets on solid substrates provide a valuable characterization of such wetting properties there are numerous experimental and theoretical studies of the shape and the spreading of droplets deposited on a cylindrical substrate (see, e.g., Refs. [1–15]). The morphology of liquid drops on a fiber is particularly interesting insofar as on a planar substrate there is only one, spherical caplike droplet shape, whereas on a cylindrical substrate droplets may exhibit two, topologically different shapes, a “clamshell”- and a “barrel”-type one, depending on the droplet volume, the contact angle, and the cylinder radius [1–3]. The aforementioned studies deal with thick fibers and large drops, i.e., the length scales are µm and larger. In this range the fluid structures are determined by macroscopic properties alone, i.e., volume of the liquid, surface tension σ of the liquid vapor interface, Young’s contact angle θ∞ , and radius R of the cylinder. However, with the discovery of nanotubes the interest in such fluid structures has shifted to much smaller scales. There are several applications for which these small solid-fluid structures are very important. (i) For fabricating valuable composite materials involving nanotubes their wetting by the liquid host matrix is necessary to couple the inherent strength of the nanotubes to the matrix, reinforcing materials or fillers for plastics and ceramics [16]. (ii) Nanotubes can be used as supports for heterogeneous catalysis or as templates for creating small wires or tubular structures by coating them with metals or metal oxides in the liquid state [17] or by attaching inorganic and organic moieties to the nanotube surfaces [18]. (iii) In order to use nanotubes as “nanostraws” potential candidates for exploiting such capillarity must be screened by first seeing if the liquid wets the outside of nanotubes [19]. The performance of the nanotubes as catalysts, adsorbants, and deodorants can vary depending on whether they are composed of carbon, boron nitride, or oxides (SiO2 , Al2 O3 , V2 O5 , MoO3 , TiO2 ) [20]. This variety demonstrates, that the substrate potential of these tubes can be regarded as a tunable parameter. (iv) By using nanotubes as nanotweezers [21] it might be possible to grab and manipulate small liquid drops. For this application the substrate must be nonwettable. These small scales are comparable with the range of the substrate potential of the cylinders and of the molecular forces between the fluid particles adsorbing on them. Thus the droplets form under the action of the so-called effective interface potential ω which accounts for the net effect of the competition between the forces among the fluid particles and the substrate potential [22]. Accordingly the calculation of the corresponding deformed droplet shapes requires a more detailed theoretical description which takes the effective interface potential into account. To our knowledge there is only one, recent publication in which this effect of ω on the droplet shape on fibers has been analyzed [23]. It is the purpose of our study here to refine and to extend this analysis in various directions. If the radius R of the fiber reduces to a few nm, as it is the case for nanotubes, the effective interface potential itself will depend on R and thus deviate from that of the corresponding semiinfinite planar substrate used in Ref. [23]. Accordingly we present a systematic analysis of the dependence of the shape of the droplets and their suitably defined contact angles on 2
微积分介值定理的英文
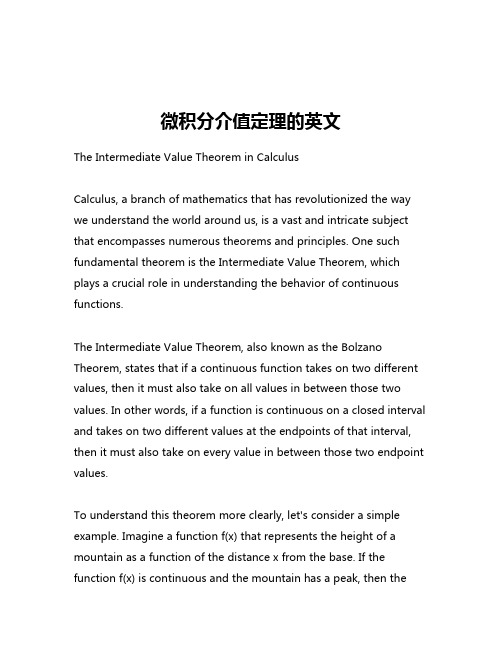
微积分介值定理的英文The Intermediate Value Theorem in CalculusCalculus, a branch of mathematics that has revolutionized the way we understand the world around us, is a vast and intricate subject that encompasses numerous theorems and principles. One such fundamental theorem is the Intermediate Value Theorem, which plays a crucial role in understanding the behavior of continuous functions.The Intermediate Value Theorem, also known as the Bolzano Theorem, states that if a continuous function takes on two different values, then it must also take on all values in between those two values. In other words, if a function is continuous on a closed interval and takes on two different values at the endpoints of that interval, then it must also take on every value in between those two endpoint values.To understand this theorem more clearly, let's consider a simple example. Imagine a function f(x) that represents the height of a mountain as a function of the distance x from the base. If the function f(x) is continuous and the mountain has a peak, then theIntermediate Value Theorem tells us that the function must take on every height value between the base and the peak.Mathematically, the Intermediate Value Theorem can be stated as follows: Let f(x) be a continuous function on a closed interval [a, b]. If f(a) and f(b) have opposite signs, then there exists a point c in the interval (a, b) such that f(c) = 0.The proof of the Intermediate Value Theorem is based on the properties of continuous functions and the completeness of the real number system. The key idea is that if a function changes sign on a closed interval, then it must pass through the value zero somewhere in that interval.One important application of the Intermediate Value Theorem is in the context of finding roots of equations. If a continuous function f(x) changes sign on a closed interval [a, b], then the Intermediate Value Theorem guarantees that there is at least one root (a value of x where f(x) = 0) within that interval. This is a powerful tool in numerical analysis and the study of nonlinear equations.Another application of the Intermediate Value Theorem is in the study of optimization problems. When maximizing or minimizing a continuous function on a closed interval, the Intermediate Value Theorem can be used to establish the existence of a maximum orminimum value within that interval.The Intermediate Value Theorem is also closely related to the concept of connectedness in topology. If a function is continuous on a closed interval, then the image of that interval under the function is a connected set. This means that the function "connects" the values at the endpoints of the interval, without any "gaps" in between.In addition to its theoretical importance, the Intermediate Value Theorem has practical applications in various fields, such as economics, biology, and physics. For example, in economics, the theorem can be used to show the existence of equilibrium prices in a market, where supply and demand curves intersect.In conclusion, the Intermediate Value Theorem is a fundamental result in calculus that has far-reaching implications in both theory and practice. Its ability to guarantee the existence of values between two extremes has made it an indispensable tool in the study of continuous functions and the analysis of complex systems. Understanding and applying this theorem is a crucial step in mastering the powerful concepts of calculus.。
湿度、云的形成和降水(英文)
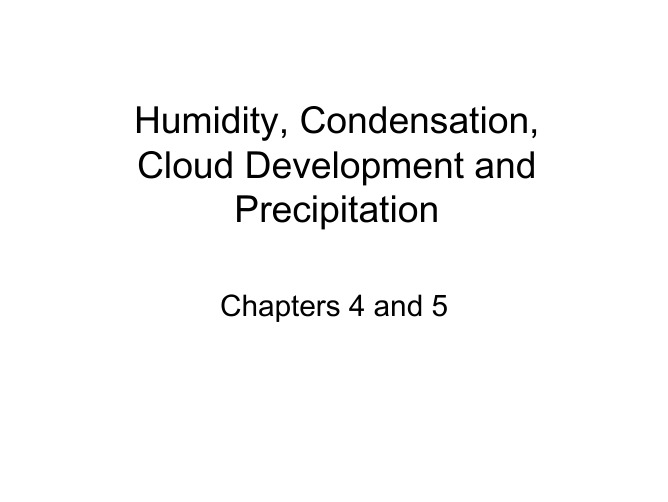
Condensation Nuclei
• If we examine the air above the water in the jar of the previous pictures, we would find that the air molecules are mixed with tiny (microscopic) bits of dust, smoke, ocean salt, etc. • Since these all serve as surfaces on which water vapor may condense they are called condensation nuclei. • Condensation is more likely to occur when air is cooled because the speed of the water vapor decreases.
•
•
Vapor Pressure
• Suppose our previous air parcel is near sea level and the air pressure inside the parcel is 1000 mb. (Total air pressure inside parcel is due to collision of all molecules against wall of parcel) • Parcel is 78% nitrogen, 21% oxygen and 1% water vapor. Pressure of nitrogen is 780 mb, oxygen 210 mb and water vapor is 10 mb. • So, the partial pressure of the water vapor (actualБайду номын сангаасvapor pressure) is 10 mb.
数据挖掘第三版第二章课后习题答案
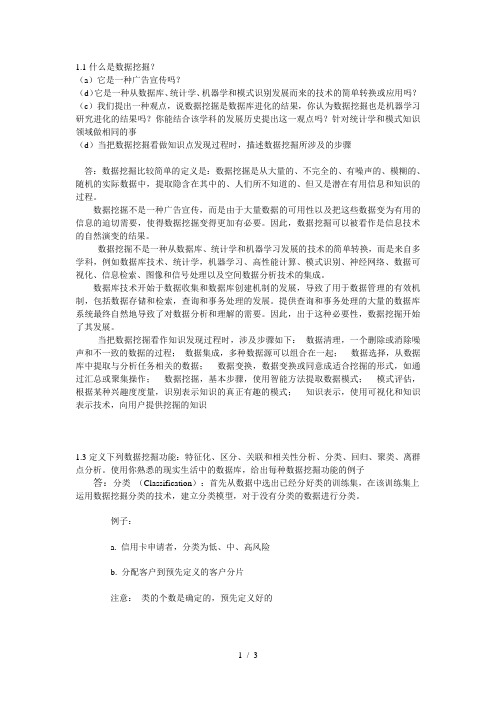
1.1什么是数据挖掘?(a)它是一种广告宣传吗?(d)它是一种从数据库、统计学、机器学和模式识别发展而来的技术的简单转换或应用吗?(c)我们提出一种观点,说数据挖掘是数据库进化的结果,你认为数据挖掘也是机器学习研究进化的结果吗?你能结合该学科的发展历史提出这一观点吗?针对统计学和模式知识领域做相同的事(d)当把数据挖掘看做知识点发现过程时,描述数据挖掘所涉及的步骤答:数据挖掘比较简单的定义是:数据挖掘是从大量的、不完全的、有噪声的、模糊的、随机的实际数据中,提取隐含在其中的、人们所不知道的、但又是潜在有用信息和知识的过程。
数据挖掘不是一种广告宣传,而是由于大量数据的可用性以及把这些数据变为有用的信息的迫切需要,使得数据挖掘变得更加有必要。
因此,数据挖掘可以被看作是信息技术的自然演变的结果。
数据挖掘不是一种从数据库、统计学和机器学习发展的技术的简单转换,而是来自多学科,例如数据库技术、统计学,机器学习、高性能计算、模式识别、神经网络、数据可视化、信息检索、图像和信号处理以及空间数据分析技术的集成。
数据库技术开始于数据收集和数据库创建机制的发展,导致了用于数据管理的有效机制,包括数据存储和检索,查询和事务处理的发展。
提供查询和事务处理的大量的数据库系统最终自然地导致了对数据分析和理解的需要。
因此,出于这种必要性,数据挖掘开始了其发展。
当把数据挖掘看作知识发现过程时,涉及步骤如下:数据清理,一个删除或消除噪声和不一致的数据的过程;数据集成,多种数据源可以组合在一起;数据选择,从数据库中提取与分析任务相关的数据;数据变换,数据变换或同意成适合挖掘的形式,如通过汇总或聚集操作;数据挖掘,基本步骤,使用智能方法提取数据模式;模式评估,根据某种兴趣度度量,识别表示知识的真正有趣的模式;知识表示,使用可视化和知识表示技术,向用户提供挖掘的知识1.3定义下列数据挖掘功能:特征化、区分、关联和相关性分析、分类、回归、聚类、离群点分析。
State Space Reconstruction for Multivariate Time Series Prediction
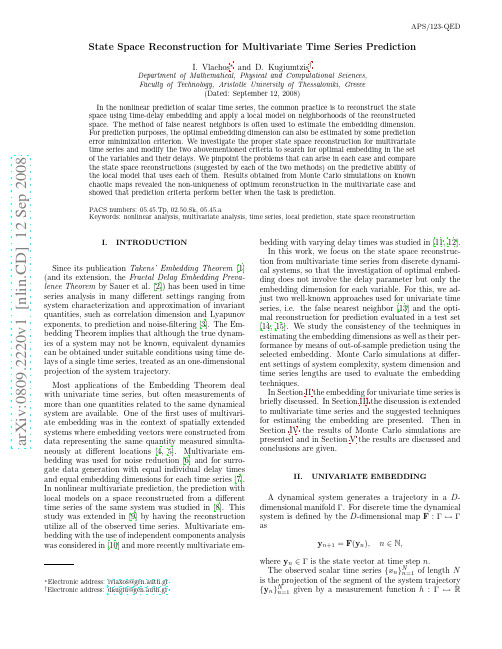
a r X i v :0809.2220v 1 [n l i n .C D ] 12 S e p 2008APS/123-QEDState Space Reconstruction for Multivariate Time Series PredictionI.Vlachos ∗and D.Kugiumtzis †Department of Mathematical,Physical and Computational Sciences,Faculty of Technology,Aristotle University of Thessaloniki,Greece(Dated:September 12,2008)In the nonlinear prediction of scalar time series,the common practice is to reconstruct the state space using time-delay embedding and apply a local model on neighborhoods of the reconstructed space.The method of false nearest neighbors is often used to estimate the embedding dimension.For prediction purposes,the optimal embedding dimension can also be estimated by some prediction error minimization criterion.We investigate the proper state space reconstruction for multivariate time series and modify the two abovementioned criteria to search for optimal embedding in the set of the variables and their delays.We pinpoint the problems that can arise in each case and compare the state space reconstructions (suggested by each of the two methods)on the predictive ability of the local model that uses each of them.Results obtained from Monte Carlo simulations on known chaotic maps revealed the non-uniqueness of optimum reconstruction in the multivariate case and showed that prediction criteria perform better when the task is prediction.PACS numbers:05.45.Tp,02.50.Sk,05.45.aKeywords:nonlinear analysis,multivariate analysis,time series,local prediction,state space reconstructionI.INTRODUCTIONSince its publication Takens’Embedding Theorem [1](and its extension,the Fractal Delay Embedding Preva-lence Theorem by Sauer et al.[2])has been used in time series analysis in many different settings ranging from system characterization and approximation of invariant quantities,such as correlation dimension and Lyapunov exponents,to prediction and noise-filtering [3].The Em-bedding Theorem implies that although the true dynam-ics of a system may not be known,equivalent dynamics can be obtained under suitable conditions using time de-lays of a single time series,treated as an one-dimensional projection of the system trajectory.Most applications of the Embedding Theorem deal with univariate time series,but often measurements of more than one quantities related to the same dynamical system are available.One of the first uses of multivari-ate embedding was in the context of spatially extended systems where embedding vectors were constructed from data representing the same quantity measured simulta-neously at different locations [4,5].Multivariate em-bedding was used for noise reduction [6]and for surro-gate data generation with equal individual delay times and equal embedding dimensions for each time series [7].In nonlinear multivariate prediction,the prediction with local models on a space reconstructed from a different time series of the same system was studied in [8].This study was extended in [9]by having the reconstruction utilize all of the observed time series.Multivariate em-bedding with the use of independent components analysis was considered in [10]and more recently multivariate em-2as x n=h(y n).Despite the apparent loss of information of the system dynamics by the projection,the system dynamics may be recovered through suitable state space reconstruction from the scalar time series.A.Reconstruction of the state space According to Taken’s embedding theorem a trajectory formed by the points x n of time-delayed components from the time series{x n}N n=1asx n=(x n−(m−1)τ,x n−(m−2)τ,...,x n),(1)under certain genericity assumptions,is an one-to-one mapping of the original trajectory of y n provided that m is large enough.Given that the dynamical system“lives”on an attrac-tor A⊂Γ,the reconstructed attractor˜A through the use of the time-delay vectors is topologically equivalent to A.A sufficient condition for an appropriate unfolding of the attractor is m≥2d+1where d is the box-counting dimension of A.The embedding process is visualized in the following graphy n∈A⊂ΓF→y n+1∈A⊂Γ↓h↓hx n∈R x n+1∈R↓e↓ex n∈˜A⊂R m G→x n+1∈˜A⊂R mwhere e is the embedding procedure creating the delay vectors from the time series and G is the reconstructed dynamical system on˜A.G preserves properties of the unknown F on the unknown attractor A that do not change under smooth coordinate transformations.B.Univariate local predictionFor a given state space reconstruction,the local predic-tion at a target point x n is made with a model estimated on the K nearest neighboring points to x n.The local model can have a simple form,such as the zeroth order model(the average of the images of the nearest neigh-bors),but here we consider the linear modelˆx n+1=a(n)x n+b(n),where the superscript(n)denotes the dependence of the model parameters(a(n)and b(n))on the neighborhood of x n.The neighborhood at each target point is defined either by afixed number K of nearest neighbors or by a distance determining the borders of the neighborhood giving a varying K with x n.C.Selection of embedding parametersThe two parameters of the delay embedding in(1)are the embedding dimension m,i.e.the number of compo-nents in x n and the delay timeτ.We skip the discussion on the selection ofτas it is typically set to1in the case of discrete systems that we focus on.Among the ap-proaches for the selection of m we choose the most popu-lar method of false nearest neighbors(FNN)and present it briefly below[13].The measurement function h projects distant points {y n}of the original attractor to close values of{x n}.A small m may still give badly projected points and we seek the reconstructed state space of the smallest embed-ding dimension m that unfolds the attractor.This idea is implemented as follows.For each point x m n in the m-dimensional reconstructed state space,the distance from its nearest neighbor x mn(1)is calculated,d(x m n,x mn(1))=x m n−x mn(1).The dimension of the reconstructed state space is augmented by1and the new distance of thesevectors is calculated,d(x m+1n,x m+1n(1))= x m+1n−x m+1n(1). If the ratio of the two distances exceeds a predefined tol-erance threshold r the two neighbors are classified as false neighbors,i.e.r n(m)=d(x m+1n,x m+1n(1))3 III.MULTIV ARIATE EMBEDDINGIn Section II we gave a summary of the reconstructiontechnique for a deterministic dynamical system from ascalar time series generated by the system.However,it ispossible that more than one time series are observed thatare possibly related to the system under investigation.For p time series measured simultaneously from the samedynamical system,a measurement function H:Γ→R pis decomposed to h i,i=1,...,p,defined as in Section II,giving each a time series{x i,n}N n=1.According to the dis-cussion on univariate embedding any of the p time seriescan be used for reconstruction of the system dynamics,or better,the most suitable time series could be selectedafter proper investigation.In a different approach all theavailable time series are considered and the analysis ofthe univariate time series is adjusted to the multivariatetime series.A.From univariate to multivariate embeddingGiven that there are p time series{x i,n}N n=1,i=1,...,p,the equivalent to the reconstructed state vec-tor in(1)for the case of multivariate embedding is of theformx n=(x1,n−(m1−1)τ1,x1,n−(m1−2)τ1,...,x1,n,x2,n−(m2−1)τ2,...,x2,n,...,x p,n)(3)and are defined by an embedding dimension vector m= (m1,...,m p)that indicates the number of components used from each time series and a time delay vector τ=(τ1,...,τp)that gives the delays for each time series. The corresponding graph for the multivariate embedding process is shown below.y n∈A⊂ΓF→y n+1∈A⊂Γւh1↓h2...ցhpւh1↓h2...ցhpx1,n x2,n...x p,n x1,n+1x2,n+1...x p,n+1ցe↓e...ւeցe↓e...ւex n∈˜A⊂R M G→x n+1∈˜A⊂R MThe total embedding dimension M is the sum of the individual embedding dimensions for each time seriesM= p i=1m i.Note that if redundant or irrelevant information is present in the p time series,only a sub-set of them may be represented in the optimal recon-structed points x n.The selection of m andτfollows the same principles as for the univariate case:the attrac-tor should be fully unfolded and the components of the embedding vectors should be uncorrelated.A simple se-lection rule suggests that all individual delay times and embedding dimensions are the same,i.e.m=m1and τ=τ1with1a p-vector of ones[6,7].Here,we set againτi=1,i=1,...,p,but we consider bothfixed and varying m i in the implementation of the FNN method (see Section III D).B.Multivariate local predictionThe prediction for each time series x i,n,i=1,...,p,is performed separately by p local models,estimated as in the case of univariate time series,but for reconstructed points formed potentially from all p time series as given in(3)(e.g.see[9]).We propose an extension of the NRMSE for the pre-diction of one time series to account for the error vec-tors comprised of the individual prediction errors for each of the predicted time series.If we have one step ahead predictions for the p available time series,i.e.ˆx i,n, i=1,...,p(for a range of current times n−1),we define the multivariate NRMSENRMSE=n (x1,n−¯x1,...,x p,n−¯x p) 2(4)where¯x i is the mean of the actual values of x i,n over all target times n.C.Problems and restrictions of multivariatereconstructionsA major problem in the multivariate case is the prob-lem of identification.There are often not unique m and τembedding parameters that unfold fully the attractor.A trivial example is the Henon map[17]x n+1=1.4−x2n+y ny n+1=0.3x n(5) It is known that for the state space reconstruction from the observable x n the appropriate embedding parame-ters are m=2andτ=1.Due to the fact that y n is a lagged multiple of x n the attractor can obviously be reconstructed from the bivariate time series{x n,y n} equally well with any of the following two-dimensional embedding schemesx n=(x n,x n−1)x n=(x n,y n)x n=(y n,y n−1) since they are essentially the same.This example shows also the problem of redundant information,e.g.the state space reconstruction would not improve by augmenting the delay vector x n=(x n,x n−1)with the component y n that actually duplicates x n−1.Redundancy is inevitable in multivariate time series as synchronous observations of the different time series are generally correlated and the fact that these observations are used as components in the same embedding vector adds redundant information in them.We note here that in the case of continuous dynamical systems,the delay parameterτi may be se-lected so that the components of the i time series are not correlated with each other,but this does not imply that they are not correlated to components from another time series.4 A different problem is that of irrelevance,whenseries that are not generated by the same dynamicaltem are included in the reconstruction procedure.may be the case even when a time series is connectedtime series generated by the system underAn issue of concern is also the fact thatdata don’t always have the same data ranges andtances calculated on delay vectors withdifferent ranges may depend highly on only some ofcomponents.So it is often preferred to scale all theto have either the same variance or be in the samerange.For our study we choose to scale the data torange[0,1].D.Selection of the embedding dimension vector Taking into account the problems in the state space reconstruction from multivariate time series,we present three methods for determining m,two based on the false nearest neighbor algorithm,which we name FNN1and FNN2,and one based on local models which we call pre-diction error minimization criterion(PEM).The main idea of the FNN algorithms is as for the univariate case.Starting from a small value the embed-ding dimension is increased by including delay compo-nents from the p time series and the percentage of the false nearest neighbors is calculated until it falls to the zero level.The difference of the two FNN methods is on the way that m is increased.For FNN1we restrict the state space reconstruction to use the same embedding dimension for each of the p time series,i.e.m=(m,m,...,m)for a given m.To assess whether m is sufficient,we consider all delay embeddings derived by augmenting the state vector of embedding di-mension vector(m,m,...,m)with a single delayed vari-able from any of the p time series.Thus the check for false nearest neighbors in(2)yields the increase from the embedding dimension vector(m,m,...,m)to each of the embedding dimension vectors(m+1,m,...,m), (m,m+1,...,m),...,(m,m,...,m+1).Then the algo-rithm stops at the optimal m=(m,m,...,m)if the zero level percentage of false nearest neighbors is obtained for all p cases.A sketch of thefirst two steps for a bivariate time series is shown in Figure1(a).This method has been commonly used in multivariate reconstruction and is more appropriate for spatiotem-porally distributed data(e.g.see the software package TISEAN[18]).A potential drawback of FNN1is that the selected total embedding dimension M is always a multiple of p,possibly introducing redundant informa-tion in the embedding vectors.We modify the algorithm of FNN1to account for any form of the embedding dimension vector m and the total embedding dimension M is increased by one at each step of the algorithm.Let us suppose that the algorithm has reached at some step the total embedding dimension M. For this M all the combinations of the components of the embedding dimension vector m=(m1,m2,...,m p)are considered under the condition M= p i=1m i.Then for each such m=(m1,m2,...,m p)all the possible augmen-tations with one dimension are checked for false nearest neighbors,i.e.(m1+1,m2,...,m p),(m1,m2+1,...,m p), ...,(m1,m2,...,m p+1).A sketch of thefirst two steps of the extended FNN algorithm,denoted as FNN2,for a bivariate time series is shown in Figure1(b).The termination criterion is the drop of the percent-age of false nearest neighbors to the zero level at every increase of M by one for at least one embedding dimen-sion vector(m1,m2,...,m p).If more than one embedding dimension vectors fulfill this criterion,the one with the smallest cumulative FNN percentage is selected,where the cumulative FNN percentage is the sum of the p FNN percentages for the increase by one of the respective com-ponent of the embedding dimension vector.The PEM criterion for the selection of m= (m1,m2,...,m p)is simply the extension of the goodness-of-fit or prediction criterion in the univariate case to account for the multiple ways the delay vector can be formed from the multivariate time series.Thus for all possible p-plets of(m1,m2,...,m p)from(1,0,...,0), (0,1,...,0),etc up to some vector of maximum embed-ding dimensions(m max,m max,...,m max),the respective reconstructed state spaces are created,local linear mod-els are applied and out-of-sample prediction errors are computed.So,totally p m max−1embedding dimension vectors are compared and the optimal is the one that gives the smallest multivariate NRMSE as defined in(4).IV.MONTE CARLO SIMULATIONS ANDRESULTSA.Monte Carlo setupWe test the three methods by performing Monte Carlo simulations on a variety of known nonlinear dynamical systems.The embedding dimension vectors are selected using the three methods on100different realizations of each system and the most frequently selected embedding dimension vectors for each method are tracked.Also,for each realization and selected embedding dimension vec-5ate NRMSE over the100realizations for each method is then used as an indicator of the performance of each method in prediction.The selection of the embedding dimension vector by FNN1,FNN2and PEM is done on thefirst three quarters of the data,N1=3N/4,and the multivariate NRMSE is computed on the last quarter of the data(N−N1).For PEM,the same split is used on the N1data,so that N2= 3N1/4data are used tofind the neighbors(training set) and the rest N1−N2are used to compute the multivariate NRMSE(test set)and decide for the optimal embedding dimension vector.A sketch of the split of the data is shown in Figure2.The number of neighbors for the local models in PEM varies with N and we set K N=10,25,50 for time series lengths N=512,2048,8192,respectively. The parameters of the local linear model are estimated by ordinary least squares.For all methods the investigation is restricted to m max=5.The multivariate time series are derived from nonlin-ear maps of varying dimension and complexity as well as spatially extended maps.The results are given below for each system.B.One and two Ikeda mapsThe Ikeda map is an example of a discrete low-dimensional chaotic system in two variables(x n,y n)de-fined by the equations[19]z n+1=1+0.9exp(0.4i−6i/(1+|z n|2)),x n=Re(z n),y n=Im(z n),where Re and Im denote the real and imaginary part,re-spectively,of the complex variable z n.Given the bivari-ate time series of(x n,y n),both FNN methods identify the original vector x n=(x n,y n)andfind m=(1,1)as optimal at all realizations,as shown in Table I.On the other hand,the PEM criterionfinds over-embedding as optimal,but this improves slightly the pre-diction,which as expected improves with the increase of N.Next we consider the sum of two Ikeda maps as a more complex and higher dimensional system.The bivariateI:Dimension vectors and NRMSE for the Ikeda map.2,3and4contain the embedding dimension vectorsby their respective frequency of occurrenceNRMSEFNN1PEM FNN2 512(1,1)1000.0510.032 (1,1)100(2,2)1000.028 8192(1,1)1000.0130.003II:Dimension vectors and NRMSE for the sum ofmapsNRMSEFNN1PEM FNN2 512(2,2)650.4560.447(1,3)26(3,3)95(2,3)540.365(2,2)3(2,2)448192(2,3)430.2600.251(1,4)37time series are generated asx n=Re(z1,n+z2,n),y n=Im(z1,n+z2,n).The results of the Monte Carlo simulations shown in Ta-ble II suggest that the prediction worsens dramatically from that in Table I and the total embedding dimension M increases with N.The FNN2criterion generally gives multiple optimal m structures across realizations and PEM does the same but only for small N.This indicates that high complex-ity degrades the performance of the algorithms for small sample sizes.PEM is again best for predictions but over-all we do not observe large differences in the three meth-ods.An interesting observation is that although FNN2finds two optimal m with high frequencies they both give the same M.This reflects the problem of identification, where different m unfold the attractor equally well.This feature cannot be observed in FNN1because the FNN1 algorithm inspects fewer possible vectors and only one for each M,where M can only be multiple of p(in this case(1,1)for M=2,(2,2)for M=4,etc).On the other hand,PEM criterion seems to converge to a single m for large N,which means that for the sum of the two Ikeda maps this particular structure gives best prediction re-sults.Note that there is no reason that the embedding dimension vectors derived from FNN2and PEM should match as they are selected under different conditions. Moreover,it is expected that the m selected by PEM gives always the lowest average of multivariate NRMSE as it is selected to optimize prediction.TABLE III:Dimension vectors and NRMSE for the KDR mapNRMSE FNN1PEM FNN2512(0,0,2,2)30(1,1,1,1)160.7760.629 (1,1,1,1)55(2,2,2,2)39(0,2,1,1)79(0,1,0,1)130.6598192(2,1,1,1)40(1,1,1,1)140.5580.373TABLE IV:Dimension vectors and NRMSE for system of Driver-Response Henon systemEmbedding dimensionsN FNN1PEM FNN2512(2,2)100(2,2)75(2,1)100.196(2,2)100(3,2)33(2,2)250.127(2,2)100(3,0)31(0,3)270.0122048(2,2)100(2,2)1000.093(2,2)100(3,3)45(4,3)450.084(2,2)100(0,3)20(3,0)190.0068192(2,2)100(2,2)1000.051(2,2)100(3,3)72(4,3)250.027(2,2)100(0,4)31(4,0)300.002TABLE V:Dimension vectors and NRMSE for Lattice of3coupled Henon mapsEmbedding dimensionsN FNN1PEM FNN2512(2,2,2)94(1,1,1)6(1,2,1)29(1,1,2)230.298(2,2,2)98(1,1,1)2(2,0,2)44(2,1,1)220.2282048(2,2,2)100(1,2,2)34(2,2,1)300.203(2,2,2)100(2,1,2)48(2,0,2)410.1318192(2,2,2)100(2,2,2)97(3,2,3)30.174(2,2,2)100(2,1,2)79(3,2,3)190.084NRMSEC FNN2FNN1PEM0.4(1,1,1,1)42(1,0,2,1)170.2850.2880.8(1,1,1,1)40(1,0,1,2)170.3140.2910.4(1,1,1,1)88(1,1,1,2)70.2290.1900.8(1,1,1,1)36(1,0,2,1)330.2250.1630.4(1,1,1,1)85(1,2,1,1)80.1970.1370.8(1,2,0,1)31(1,0,2,1)220.1310.072 PEM cannot distinguish the two time series and selectswith almost equal frequencies vectors of the form(m,0)and(0,m)giving again over-embedding as N increases.Thus PEM does not reveal the coupling structure of theunderlying system and picks any embedding dimensionstructure among a range of structures that give essen-tially equivalent predictions.Here FNN2seems to de-tect sufficiently the underlying coupling structure in thesystem resulting in a smaller total embedding dimensionthat gives however the same level of prediction as thelarger M suggested by FNN1and slightly smaller thanthe even larger M found by PEM.ttices of coupled Henon mapsThe last system is an example of spatiotemporal chaosand is defined as a lattice of k coupled Henon maps{x i,n,y i,n}k i=1[22]specified by the equationsx i,n+1=1.4−((1−C)x i,n+C(x i−1,n+x i+1,n)ple size,at least for the sizes we used in the simulations. Such a feature shows lack of consistency of the PEM cri-terion and suggests that the selection is led from factors inherent in the prediction process rather than the quality of the reconstructed attractor.For example the increase of embedding dimension with the sample size can be ex-plained by the fact that more data lead to abundance of close neighbors used in local prediction models and this in turn suggests that augmenting the embedding vectors would allow to locate the K neighbors used in the model. On the other hand,the two schemes used here that ex-tend the method of false nearest neighbors(FNN)to mul-tivariate time series aim atfinding minimum embedding that unfolds the attractor,but often a higher embedding gives better prediction results.In particular,the sec-ond scheme(FNN2)that explores all possible embedding structures gives consistent selection of an embedding of smaller dimension than that selected by PEM.Moreover, this embedding could be justified by the underlying dy-namics of the known systems we tested.However,lack of consistency of the selected embedding was observed with all methods for small sample sizes(somehow expected due to large variance of any estimate)and for the cou-pled maps(probably due to the presence of more than one optimal embeddings).In this work,we used only a prediction performance criterion to assess the quality of state space reconstruc-tion,mainly because it has the most practical relevance. There is no reason to expect that PEM would be found best if the assessment was done using another criterion not based on prediction.However,the reference(true)value of other measures,such as the correlation dimen-sion,are not known for all systems used in this study.An-other constraint of this work is that only noise-free multi-variate time series from discrete systems are encountered, so that the delay parameter is not involved in the state space reconstruction and the effect of noise is not studied. It is expected that the addition of noise would perplex further the process of selecting optimal embedding di-mension and degrade the performance of the algorithms. For example,we found that in the case of the Henon map the addition of noise of equal magnitude to the two time series of the system makes the criteria to select any of the three equivalent embeddings((2,0),(0,2),(1,1))at random.It is in the purpose of the authors to extent this work and include noisy multivariate time series,also fromflows,and search for other measures to assess the performance of the embedding selection methods.AcknowledgmentsThis paper is part of the03ED748research project,im-plemented within the framework of the”Reinforcement Programme of Human Research Manpower”(PENED) and co-financed at90%by National and Community Funds(25%from the Greek Ministry of Development-General Secretariat of Research and Technology and75% from E.U.-European Social Fund)and at10%by Rik-shospitalet,Norway.[1]F.Takens,Lecture Notes in Mathematics898,365(1981).[2]T.Sauer,J.A.Yorke,and M.Casdagli,Journal of Sta-tistical Physics65,579(1991).[3]H.Kantz and T.Schreiber,Nonlinear Time Series Anal-ysis(Cambridge University Press,1997).[4]J.Guckenheimer and G.Buzyna,Physical Review Let-ters51,1438(1983).[5]M.Paluˇs,I.Dvoˇr ak,and I.David,Physica A StatisticalMechanics and its Applications185,433(1992).[6]R.Hegger and T.Schreiber,Physics Letters A170,305(1992).[7]D.Prichard and J.Theiler,Physical Review Letters73,951(1994).[8]H.D.I.Abarbanel,T.A.Carroll,,L.M.Pecora,J.J.Sidorowich,and L.S.Tsimring,Physical Review E49, 1840(1994).[9]L.Cao,A.Mees,and K.Judd,Physica D121,75(1998),ISSN0167-2789.[10]J.P.Barnard,C.Aldrich,and M.Gerber,Physical Re-view E64,046201(2001).[11]S.P.Garcia and J.S.Almeida,Physical Review E(Sta-tistical,Nonlinear,and Soft Matter Physics)72,027205 (2005).[12]Y.Hirata,H.Suzuki,and K.Aihara,Physical ReviewE(Statistical,Nonlinear,and Soft Matter Physics)74, 026202(2006).[13]M.B.Kennel,R.Brown,and H.D.I.Abarbanel,Phys-ical Review A45,3403(1992).[14]D.T.Kaplan,in Chaos in Communications,edited byL.M.Pecora(SPIE-The International Society for Optical Engineering,Bellingham,Washington,98227-0010,USA, 1993),pp.236–240.[15]B.Chun-Hua and N.Xin-Bao,Chinese Physics13,633(2004).[16]R.Hegger and H.Kantz,Physical Review E60,4970(1999).[17]M.H´e non,Communications in Mathematical Physics50,69(1976).[18]R.Hegger,H.Kantz,and T.Schreiber,Chaos:An Inter-disciplinary Journal of Nonlinear Science9,413(1999).[19]K.Ikeda,Optics Communications30,257(1979).[20]C.Grebogi,E.Kostelich,E.O.Ott,and J.A.Yorke,Physica D25(1987).[21]S.J.Schiff,P.So,T.Chang,R.E.Burke,and T.Sauer,Physical Review E54,6708(1996).[22]A.Politi and A.Torcini,Chaos:An InterdisciplinaryJournal of Nonlinear Science2,293(1992).。
- 1、下载文档前请自行甄别文档内容的完整性,平台不提供额外的编辑、内容补充、找答案等附加服务。
- 2、"仅部分预览"的文档,不可在线预览部分如存在完整性等问题,可反馈申请退款(可完整预览的文档不适用该条件!)。
- 3、如文档侵犯您的权益,请联系客服反馈,我们会尽快为您处理(人工客服工作时间:9:00-18:30)。
SHORT COMMUNICATIONDroplet coalescence by geometrically mediated flow in microfluidic channelsYung-Chieh Tan ÆYao Li Ho ÆAbraham Phillip LeeReceived:6September 2006/Accepted:7November 2006/Published online:30November 2006ÓSpringer-Verlag 2006Abstract Microfluidic flow is geometrically mediated at a trifurcating junction allowing periodically formed,equally spaced out emulsion droplets to redistribute and fuse consistently.This is achieved by controlling the ratio between the droplet transport time across the trifurcating junction and the drainage time of the fluid volume separating the droplets t r /t d .Three different microfluidic trifurcation geometries have been de-signed and compared for their droplet fusion efficien-cies.Fusion of up to six droplets has been observed in these devices.The fusion of two droplets occurs when t r /t d is equal to 1.25and the number of fused droplets increases with t r /t d .When the junction length (d )is 216l m fusion of 2–6six droplets are possible however when the junction length is increased to 360l m fusion of only two droplets is observed.Keywords Droplets ÁCoalescence ÁFusion ÁMixing ÁMicrofluidics1IntroductionSince Thorsen and Quake first demonstrated the for-mation of dynamic patterns of droplets in microfluidic channels (Thorsen et al.2001),droplet based micro-fluidics has become a rapidly growing platform with a variety of biological applications.Examples of theseapplications include on-chip PCR (Guttenberg et al.2004),protein crystallizations (Zheng et al.2003),and lab-on-chip bio-analytical devices (Srinivasan et al.2004).Anna and Stone (Anna et al.2003),Link et al.(2004),Lee et al.(Tan et al.2004,2005,2006a ,b ;Hung et al.2006)and many others (Utada et al.2005;Cha-bert et al.2005;Kohler et al.2004)have demonstrated a myriad of droplet manipulation techniques to enable applications that require more complex microfluidic device designs.Droplet fusion in microfluidic networks has been achieved by means of electrostatics (Chabert et al.2005),velocity difference between different size droplets (Olbricht and Kung 1987),passive trapping (Kohler et al.2004),and flow trapping (Tan et al.2004;Hung et al.2006).Droplet coalescence of two different sized droplets was first demonstrated in a capillary tube 20years ago by Olbricht and Kung (1987).However,the fusion of equal sized droplets is difficult since two identical droplets travel at the same velocity in capillary tubes (Hung et al.2006;Ho and Leal 1975).Here we describe a simple scaling criterion that can be used to design the trifurcating fluidic channels that would fuse identical droplets in large quantities through rearranging the original formation pattern of droplets in microfluidic channels.The setup can be used as a model to study the coalescence rate of droplets under different parameters or it can be used as a chemical mixing device.The periodic formation of droplets inherently indi-cates that droplets are separated by an equal and finite volume oil phase that varies according to the droplet size and generation rate.The draining of this separat-ing oil volume allows droplets to be ‘‘hydrodynamically trapped’’and allow droplets to fuse.Through varyingY.-C.Tan (&)ÁY.L.Ho ÁA.P.Lee Department of Biomedical Engineering,University of California,Irvine,CA,USA e-mail:mtan@ Y.L.Hoe-mail:aplee@Microfluid Nanofluid (2007)3:495–499DOI 10.1007/s10404-006-0136-1the drainage rate,longer hydrodynamic trapping time increases the number of fused droplets and the original droplet formation pattern is rearranged accordingly.Thus a formation pattern of individual small droplets distributed evenly in a straight channel can enter the trifurcating junction and form a pattern of fused dou-blets,triplets,and etc.The droplet fusion design is shown in Fig.1.Droplet fusion occurs at the trifurcating junction and t r corre-sponds to the required transport time for the droplets to travel horizontally across the junction.The lengths of the branches of the three trifurcated exit channels determine Q d by the resistances of the channels.Due to the symmetric channel geometry at the trifurcating junction,the shear forces opposite in directions cancels out and balance the traveling droplet in the center of the trifurcating junction.Since droplets travel hori-zontally across the channel t r is proportional to the length of the channel trifurcating junction (d )and in-versely proportional to Q c /Q d ,the ratio of the droplet transporting flow rate and the drainage flow rate.Since the drainage time must be shorter than the transport time across the trifurcating junction a scaling criterion,t d ¼Q i t gQ dt r ð1Þis derived to design the fluidic channel.t d is the time required to drain the oil volume separating the two droplets,Q i is the total flow rate of the continuous oil phase,t g is the time required to generate a single droplet,Q d is the drainage flow rate,and t r is the droplet’s transport time during fluid drainage in the trifurcating junction.Equation (1)can be expressed as 1£t r /t d since fusion at the junction can only occur when the separating fluid volume is drained while the droplets are still crossing the junction.2Materials and methodsThe PDMS microfluidic channel is molded from a SU-8master fabricated using UV-lithography and subse-quently bonded to a glass microscopic slide after oxy-gen plasma oxidation.Five different channels are fabricated each with varying ratios of channel lengths or variations in the junction length.Immiscible fluids,ultra purified deionized water are used as the dispersed phase and oleic acid (viscosity 27.64mPa and interfa-cial tension 15.6dyn/cm)is used as the continuous phase.Droplets are generated by the shear viscous stress interactions in a flow focusing channel geometry where droplets break-up at the junction (Fig.1left).The flow rate of each fluid is controlled by an inde-pendent pump that injects the fluid at a constant flow rate.A fast speed imaging system (Fastcam PCI-10K ,Photron Ltd.)is used to record movies of droplet transport and fusion in the microfluidic channels.From the recorded movie,the velocity of the droplets,the droplet sizes,and the droplet generation frequencies are measured using digital processing software (Meta-Morph v.6by Universal Imaging Corporation).3Results and discussionsThe velocities of the droplet plugs are found to be independent of droplet sizes.This permits that the ratio of flow rates specific to channel geometry be determined by the ratio of the velocities of every droplet measured at the point before and after the junction.Since the shape of the droplets are identical before and after the junction,Q c /Q d are approximated from the velocities of droplet before and after fusion.The measured Q c /Q d are found to be approximately equal to the values predicted from the resistances of the trifurcated channels as indicated in Table 1.Fig.1Geometry of the droplet fusion device.Left Droplets are created at the generation site using the flow focusing geometry.Right Droplets then travel down stream to the junction where they fuse according to the designed geometric ratios.Q d is the sum of the upper fluid drainage rate and the bottom fluid drainage rateDroplet volumes ranging from 0.16to 0.99nL have been shown to fuse with 100%accuracy.Up to six droplets can be combined simultaneously.We observed three types of droplet fusion events as shown in Fig.2,the medial fusion occurs when the droplets are perfectly aligned to the middle of the junction before fusion and during fusion;the lateral fusion occurs when one droplet is in contact with the side of the other droplet but is not aligned center to center.The induced fusion occurs when the deforma-tion of the front droplet initiates the fusion of the following droplet.When droplets fuse the shape of droplets are tem-porarily deformed then restored which characterize the completion of fusion.The fusion of two droplets con-taining the same fluids is completed in approximately 16ms.This time scale is different from the classical droplet coalescence by film drainage in which the film drainage time is on the scale of seconds (Klaseboer et al.2000).From the five channel designs (A–E)each with a uniform depth of 50l m and with variations in d and Q c /Q d as indicated in Table 1,the observed fusion criteria agree with 1£t r /t d such that the minimum va-Table 1Flow rate ratiosd (l m)Q c /Q d (est)Q c /Q d (exp)A 2160.640.75B 2160.890.89C 216 1.5 1.22D 3600.890.89E3601.51.4Fig.2Three types of fusion events are observed in the microfluidic device.Thearrow indicates the traveling direction of the droplet.Medial fusion and lateral fusion differs in the positions of the droplets during fusion.During induced fusion the channel inlet deforms the droplet causing thecoalescence of the subsequent drop.Shown in the last row is the sequential fusion of multiple droplets.Up to six droplets have been shown to fuse with thisprocesslue of t r /t d observed,when fusion occurs,is 1.29.The results in Fig.3show the effect of t r /t d and junction geometry on droplet fusion.Each point is derived from a different set of dispersed and continuous flow rates.The flow rates are adjusted until droplet fusion occurs.The dispersed phase flow rate used in the experiment ranges from 0.1to 0.7l L/min and the continuous phase flow rate ranges from 0.8to 4.2l L/min.The droplet transport time across the junction ranges from 80to 372ms and the drainage time ranges from 60to 300ms.When Q c /Q d increases,the oil volume separating the droplets are drained at a lower rate and the number of droplet fused at the junction decreases stly,when the length of junction (d )is 216l m the fusing of 1–5droplets are reproducible,however when d is increased to 360l m the number of fused droplets decreases significantly.While an increased d would increase the droplet transport time,the widened junc-tion also reduces the magnitude of the fluidic shear force that aligns the droplets to the center of the channel.This allows droplets to form a zigzag pattern causing lateral fusion as demonstrated in Fig.2and consequently prevents the fusion of three or more droplets.One of the most important applications for droplet fusion devices is the mixing of reagents.When droplets containing different reagents are generated sequen-tially,reagent mixing can be achieved rapidly upon fusion.Figure 4demonstrates the reagent fusing of two and three droplets containing different reagents.Upon fusion of two droplets a portion of each droplet is rapidly exchanged forming a tri-band pattern within 8ms.It is interesting to note that in the fusion between a droplet dye and a pure water droplet,the pure water droplet is always rapidly absorbed into the dyed droplet regardless of the position or the orientation of the two droplets.This is probably due to the difference in the surface tension of the droplets.4ConclusionFrom the scaling of 1£t r /t d a series of channel designs with variations in fusion efficiencies can be derived to manipulate the post formation spacing pattern of droplets in the microfluidic channel.The dynamic formation pattern of droplets is altered accordingly that the periodically generated single droplets become fused droplets separated by identical spacing in be-tween.The rapid mixing rate,the quantized volume addition,the high mixing efficiency,and the simplicity of fabrication and design may be utilized as a study model for droplet coalescence or as a mixer for nano-liter reagents.ReferencesAnna SL,Bontoux N,Stone HA (2003)Formation of dispersionsusing ‘flow-focusing’in microchannels.Appl Phys Lett 82:364–366Chabert M,Dorfman KD,Viovy JL (2005)Droplet fusion byalternating current (AC)field electrocoalescence in micro-channels.Electrophoresis 26:3706–3715Guttenberg Z,Mu¨ller H,Habermu ¨ller H,Geisbauer A,Pipper J,Felbel J,Kielpinski M,Scriba J,Wixforth A (2004)Planar chip device for PCR and hybridization with surface acoustic wave b Chip 5:308–317Ho BP,Leal LG (1975)The creeping motion of liquid dropsthrough a circular tube of comparable diameter.J Fluid Mech 71:361–383Hung LH,Choi KM,Tseng WY,Tan YC,Shea KJ,Lee AP(2006)Alternating droplet generation and controlled dynamic droplet fusion in microfluidic device for CdS nanoparticles b Chip 6:174–178Klaseboer E,Chevaillier JP,Gourdon C,Masbernat O (2000)Film drainage between colliding drops at constant approach velocity experiments and modeling.J Colloid Interface Sci 229:274–285Kohler JM,Henkel T,Grodrian A,Kirner T,Roth M,Martin K,Metze J (2004)Digital reaction technology by micro segmented flow—components,concepts and applications.Chem Eng J 101:201–216Link DR,Anna SL,Weitz DA,Stone HA (2004)Geometricallymediated breakup of drops in microfluidic devices.Phys Rev Lett92:05403–05404Fig.4Fusion of droplets containing different reagents can be achieved within millisecondsOlbricht WL,Kung DM(1987)The interaction and coalescence of liquid drops inflow through a capillary tube.J Colloid Interface Sci120:229–244Srinivasan V,Pamula VK,Fair RB(2004)An integrated digital microfluidic lab-on-a-chip for clinical diagnostics on human physiologicalflb Chip4:310–315Tan YC,Fisher JS,Lee AI,Cristini V,Lee AP(2004)Design of microfluidic channel geometries for the control of droplet volume,chemical concentration,and b Chip 4:292–298Tan YC,Lee AP(2005)Microfluidic separation of satellite droplets as the basis of a monodispersed micron and submicron emulsification b Chip5:1178–1183 Tan YC,Cristini V,Lee AP(2006a)Monodispersed microfluidic droplet generation by shear focusing microfluidic device.Sens Actuators B114:350–356Tan YC,Hettiarachchi K,Siu M,Pan YR,Lee AP(2006b) Controlled microfluidic encapsulation of cells,proteins and microbeads in lipid vesicles.JACS128:5656–5658 Thorsen T,Roberts RW,Arnold FH,Quake SR(2001)Dynamic pattern formation in a vesicle-generating microfluidic device.Phys Rev Lett86:4163–4166Utada AS,Lorenceau E,Link DR,Kaplan PD,Stone HA,Weitz DA(2005)Monodisperse double emulsions generated froma microcapillary device.Science308:537–541Zheng B,Roach LS,Ismagilov(2003)RF,screening of protein crystallization conditions on a microfluidic chip using nanoliter-size droplets.J Am Chem Soc125:11170–11171。