A Method for Squareness Error Verification on a Coordinate
[Fluent] Solver error原因及处理方法综整
![[Fluent] Solver error原因及处理方法综整](https://img.taocdn.com/s3/m/3dda6e0ade80d4d8d15a4f96.png)
5。网格划分有问题,两个三维流域交于一条线;或者二维流域交于一点;(有这个问题的, 如果选用湍流模型,计算必然发散)2 L9 b9 O& n" I. u* { 6。CFD 软件和分网格软件单位不匹配,导致物理模型失真。 --------by barggier#
Hirzebruch surfaces and Error-correcting Codes
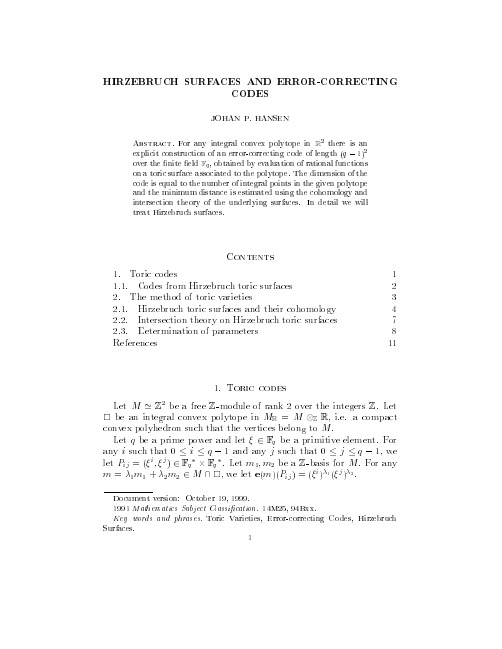
4
q-1
JOHAN P. HANSEN
e
d
q-1
Figure 2. The polytope of Theorem 1.5 is the polytope
with vertices (0; 0); (d; 0); (d; e + rd); (0; e):
Figure 3. The normal fan of the polytope of Theo-
De nition 1.1. The toric code C associated to is the linear code of length n = (q ? 1)2 generated by the vectors f(e(m)(Pij ))i=0;:::;q?1;j=0;:::;q?1 j m 2 M \ g: (1) In JPH] we presented a general method to obtain the dimension and a lower bound for the minimal distance of a toric code. In particular we obtained the following three results, where the second code is a subcode of the Reed Muller code on P2. Theorem 1.2. Let d be a positive integer and let be the polytope in MR with vertices (0; 0); (d; d); (0; 2d), see gure 1. Assume that 2d < q ? 1: The toric code C has length equal to (q ? 1)2 , dimension equal to #(M \ ) = (d + 1)2 ( the number of lattice points in ) and minimal distance greater or equal to (q ? 1)2 ? 2d(q ? 1). Theorem 1.3. Let d be a positive integer and let be the polytope in MR with vertices (0; 0); (d; 0); (0; d), see gure 1. Assume that d < q ?1: The toric code C has length equal to (q ? 1)2 , dimension equal to #(M \ ) = (d+1)(d+2) ( the number of lattice points in ) and minimal 2 distance greater or equal to (q ? 1)2 ? d(q ? 1). Theorem 1.4. Let d; e be positive integers and let be the polytope in MR with vertices (0; 0); (d; 0); (d; e); (0; e), see gure 1. Assume that d < q ? 1 and that e < q ? 1: The toric code C has length equal to (q ? 1)2 , dimension equal to #(M \ ) = (d + 1)(e + 1) ( the number of lattice points in ) and minimal distance greater or equal to (q ? 1)2 ? (d(q ? 1) + (q ? 1 ? d)e) = (q ? 1 ? d)(q ? 1 ? e).
bonferroni correction method -回复
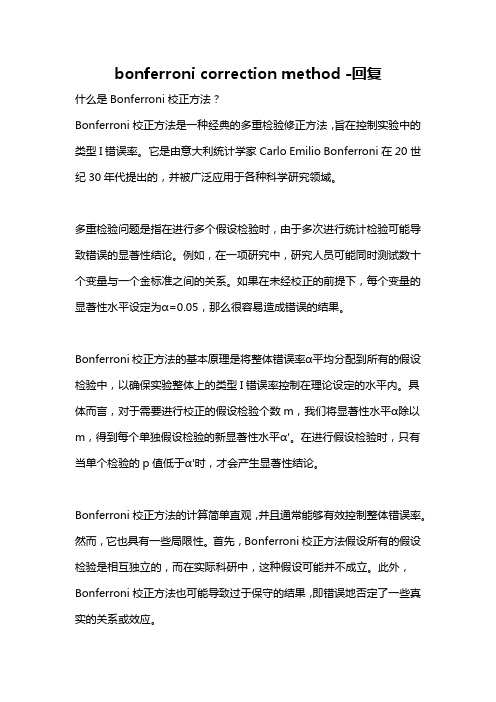
bonferroni correction method -回复什么是Bonferroni校正方法?Bonferroni校正方法是一种经典的多重检验修正方法,旨在控制实验中的类型I错误率。
它是由意大利统计学家Carlo Emilio Bonferroni在20世纪30年代提出的,并被广泛应用于各种科学研究领域。
多重检验问题是指在进行多个假设检验时,由于多次进行统计检验可能导致错误的显著性结论。
例如,在一项研究中,研究人员可能同时测试数十个变量与一个金标准之间的关系。
如果在未经校正的前提下,每个变量的显著性水平设定为α=0.05,那么很容易造成错误的结果。
Bonferroni校正方法的基本原理是将整体错误率α平均分配到所有的假设检验中,以确保实验整体上的类型I错误率控制在理论设定的水平内。
具体而言,对于需要进行校正的假设检验个数m,我们将显著性水平α除以m,得到每个单独假设检验的新显著性水平α'。
在进行假设检验时,只有当单个检验的p值低于α'时,才会产生显著性结论。
Bonferroni校正方法的计算简单直观,并且通常能够有效控制整体错误率。
然而,它也具有一些局限性。
首先,Bonferroni校正方法假设所有的假设检验是相互独立的,而在实际科研中,这种假设可能并不成立。
此外,Bonferroni校正方法也可能导致过于保守的结果,即错误地否定了一些真实的关系或效应。
为了克服Bonferroni校正方法的局限性,还有一些其他的多重检验方法被提出。
其中一种常见的方法是BH校正方法(Benjamini-Hochberg procedure),它在控制实验整体错误率的同时,还考虑了假设检验结果的相关性。
Bonferroni校正方法的应用范围十分广泛。
在医学研究中,它被用于分析临床试验和流行病学调查数据。
在基因组学领域,它被用于处理高通量基因表达数据中的差异分析。
此外,在社会科学研究和市场研究中,Bonferroni校正方法也常常用于控制多个统计检验的错误率。
一种实验验证方法英文
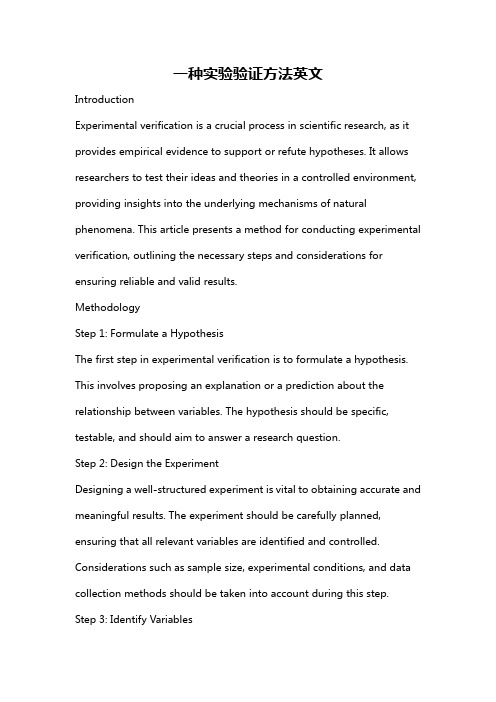
一种实验验证方法英文IntroductionExperimental verification is a crucial process in scientific research, as it provides empirical evidence to support or refute hypotheses. It allows researchers to test their ideas and theories in a controlled environment, providing insights into the underlying mechanisms of natural phenomena. This article presents a method for conducting experimental verification, outlining the necessary steps and considerations for ensuring reliable and valid results.MethodologyStep 1: Formulate a HypothesisThe first step in experimental verification is to formulate a hypothesis. This involves proposing an explanation or a prediction about the relationship between variables. The hypothesis should be specific, testable, and should aim to answer a research question.Step 2: Design the ExperimentDesigning a well-structured experiment is vital to obtaining accurate and meaningful results. The experiment should be carefully planned, ensuring that all relevant variables are identified and controlled. Considerations such as sample size, experimental conditions, and data collection methods should be taken into account during this step.Step 3: Identify VariablesIdentifying and controlling variables is crucial to minimize confounding factors and ensure that the observed effects are due to the manipulated variables. Variables can be classified as independent, dependent, or controlled. Independent variables are manipulated by the researcher, dependent variables are the outcomes or the observed effects, and controlled variables are kept constant to prevent their influence on the results.Step 4: Conduct the ExperimentOnce the experiment has been designed and variables have been identified, it is time to conduct the experiment. Follow the established protocol, collecting relevant data and observations. Take into consideration the ethical guidelines and safety precautions to ensure the integrity of the experiment and the well-being of participants.Step 5: Analyze and Interpret the ResultsAfter data collection, it is important to analyze and interpret the obtained results. Statistical analysis methods can be employed to determine the significance of the observed effects. Consider factors such as margin of error, confidence intervals, and p-values to draw meaningful conclusions.Step 6: Draw ConclusionsBased on the results obtained, draw conclusions regarding the hypothesis. If the data supports the hypothesis, it provides evidence tovalidate the proposed explanation. However, if the data contradicts the hypothesis, it suggests a revision of the initial hypothesis or the formulation of a new one. Ensure that the conclusions are based on sound scientific reasoning and are supported by the empirical evidence obtained from the experiment.Considerations and Potential Challenges- Sample size: Ensure that the sample size is sufficient to achieve statistical power and accurately capture the effects of variables.- Control group: Create a control group to serve as a basis of comparison, allowing the evaluation of the impact of the independent variable.- Replicability: Conduct the experiment multiple times to ensure the reliability of the results. Replicability adds strength to the validity of the findings.- Ethical considerations: Adhere to ethical guidelines and obtain necessary consent from participants to maintain the ethical integrity of the experiment.- External validity: Consider the generalizability of the results to the broader population or other settings. It is important to acknowledge any limitations or potential biases that might affect the external validity. ConclusionExperimental verification is a foundational aspect of scientific research. By following the outlined methodology and taking into account theconsiderations and potential challenges, researchers can conduct experiments that generate reliable and valid results. Through rigorous experimentation, scientists are able to advance knowledge, inspire further research, and make important contributions to their respective fields.。
模式识别课件prch5part3ding

i
j
then the fixed-increment rule for correcting L
k
is a (k 1) a (k) yk
i
i
a (k 1) a (k) yk
j
j
a (k 1) a (k), l i and l j
l
l
9
Remark:Kesler’s construction can also be used to extend all of results for the Perceptron and relaxation procedure to the multicategory case
Remarks: For an arbitrarily fixed b, MSE solution may not be a separating vector.
2
3
The Widrow-Hoff or LMS Procedure Need not converge to a separating hyperplane even if there exist one
Ya b
2
n
(at
y i
b )2 i
i 1
The gradient
n
J s
2(
a
t
y i
b )y
i
i
2Y t (Ya b)
i 1
Set it to zero, we get
Y tYa Y tb
If Y tY is nonsingular, a (Y tY)1Y tb Y b
The d by n matrix Y is call the pseudoinverse of Y.
20140219_Analytical_Procedures_and_Methods_Validation_for_Drugs_and_Biologics
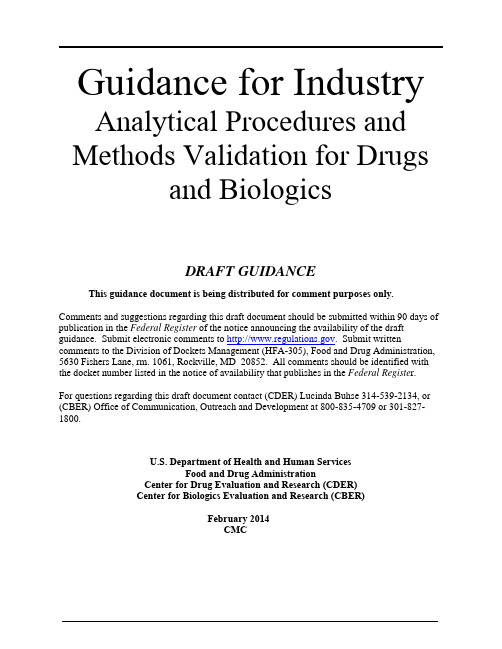
Analytical Procedures and Methods Validation for Drugsand BiologicsDRAFT GUIDANCEThis guidance document is being distributed for comment purposes only. Comments and suggestions regarding this draft document should be submitted within 90 days of publication in the Federal Register of the notice announcing the availability of the draft guidance. Submit electronic comments to . Submit written comments to the Division of Dockets Management (HFA-305), Food and Drug Administration, 5630 Fishers Lane, rm. 1061, Rockville, MD 20852. All comments should be identified with the docket number listed in the notice of availability that publishes in the Federal Registe r.For questions regarding this draft document contact (CDER) Lucinda Buhse 314-539-2134, or (CBER) Office of Communication, Outreach and Development at 800-835-4709 or 301-827-1800.U.S. Department of Health and Human ServicesFood and Drug AdministrationCenter for Drug Evaluation and Research (CDER)Center for Biologics Evaluation and Research (CBER)February 2014CMCAnalytical Procedures and Methods Validation for Drugsand BiologicsAdditional copies are available from:Office of CommunicationsDivision of Drug Information, WO51, Room 2201Center for Drug Evaluation and ResearchFood and Drug Administration10903 New Hampshire Ave., Silver Spring, MD 20993Phone: 301-796-3400; Fax: 301-847-8714druginfo@/Drugs/GuidanceComplianceRegulatoryInformation/Guidances/default.htmand/orOffice of Communication, Outreach andDevelopment, HFM-40Center for Biologics Evaluation and ResearchFood and Drug Administration1401 Rockville Pike, Rockville, MD 20852-1448ocod@/BiologicsBloodVaccines/GuidanceComplianceRegulatoryInformation/Guidances/default.htm(Tel) 800-835-4709 or 301-827-1800U.S. Department of Health and Human ServicesFood and Drug AdministrationCenter for Drug Evaluation and Research (CDER)Center for Biologics Evaluation and Research (CBER)Febr uary 2014CMCTABLE OF CONTENTSI.INTRODUCTION (1)II.BACKGROUND (2)III.ANALYTICAL METHODS DEVELOPMENT (3)IV.CONTENT OF ANALYTICAL PROCEDURES (3)A.Principle/Scope (4)B.Apparatus/Equipment (4)C.Operating Parameters (4)D.Reagents/Standards (4)E.Sample Preparation (4)F.Standards Control Solution Preparation (5)G.Procedure (5)H.System Suitability (5)I.Calculations (5)J.Data Reporting (5)V.REFERENCE STANDARDS AND MATERIALS (6)VI.ANALYTICAL METHOD VALIDATION FOR NDA, ANDAs, BLAs, AND DMFs (6)A.Noncompendial Analytical Procedures (6)B.Validation Characteristics (7)pendial Analytical Procedures (8)VII.STATISTICAL ANALYSIS AND MODELS (8)A.Statistics (8)B.Models (8)VIII.LIFE CYCLE MANAGEMENT OF ANALYTICAL PROCEDURES (9)A.Revalidation (9)B.Analytical Method Comparability Studies (10)1.Alternative Analytical Procedures (10)2.Analytical Methods Transfer Studies (11)C.Reporting Postmarketing Changes to an Approved NDA, ANDA, or BLA (11)IX.FDA METHODS VERIFICATION (12)X.REFERENCES (12)Guidance for Industry11Analytical Procedures and Methods Validation for Drugs and2Biologics345This draft guidance, when finalized, will represent the Food and Drug Administration’s (FDA’s) current 6thinking on this topic. It does not create or confer any rights for or on any person and does not operate to 7bind FDA or the public. You can use an alternative approach if the approach satisfies the requirements of 8the applicable statutes and regulations. If you want to discuss an alternative approach, contact the FDA9staff responsible for implementing this guidance. If you cannot identify the appropriate FDA staff, call 10the appropriate number listed on the title page of this guidance.11121314I. INTRODUCTION1516This revised draft guidance supersedes the 2000 draft guidance for industry on Analytical17Procedures and Methods Validation2,3 and, when finalized, will also replace the 1987 FDA18guidance for industry on Submitting Samples and Analytical Data for Methods Validation. It19provides recommendations on how you, the applicant, can submit analytical procedures4 and20methods validation data to support the documentation of the identity, strength, quality, purity,21and potency of drug substances and drug products.5It will help you assemble information and 22present data to support your analytical methodologies. The recommendations apply to drug23substances and drug products covered in new drug applications (NDAs), abbreviated new drug 24applications (ANDAs), biologics license applications (BLAs), and supplements to these25applications. The principles in this revised draft guidance also apply to drug substances and drug 26products covered in Type II drug master files (DMFs).2728This revised draft guidance complements the International Conference on Harmonisation (ICH) 29guidance Q2(R1)Validation of Analytical Procedures: Text and Methodology(Q2(R1)) for30developing and validating analytical methods.3132This revised draft guidance does not address investigational new drug application (IND) methods 33validation, but sponsors preparing INDs should consider the recommendations in this guidance.34For INDs, sufficient information is required at each phase of an investigation to ensure proper35identity, quality, purity, strength, and/or potency. The amount of information on analytical36procedures and methods validation will vary with the phase of the investigation.6 For general371 This guidance has been prepared by the Office of Pharmaceutical Science, in the Center for Drug Evaluation andResearch (CDER) and the Center for Biologics Evaluation and Research (CBER) at the Food and DrugAdministration.2 Sample submission is described in section IX, FDA Methods Verification.3 We update guidances periodically. To make sure you have the most recent version of a guidance, check the FDADrugs guidance Web page at/Drugs/GuidanceComplianceRegulatoryInformation/Guidances/default.htm.4Analytical procedure is interchangeable with a method or test procedure.5The terms drug substance and drug product, as used in this guidance, refer to human drugs and biologics.6 See 21 CFR 312.23(a)(7).guidance on analytical procedures and methods validation information to be submitted for phase 38one studies, sponsors should refer to the FDA guidance for industry on Content and Format of39Investigational New Drug Applications (INDs) for Phase 1 Studies of Drugs, Including40Well-Characterized, Therapeutic, Biotechnology-Derived Products. General considerations for 41analytical procedures and method validation (e.g., bioassay) before conduct of phase three42studies are discussed in the FDA guidance for industry on IND Meetings for Human Drugs and 43Biologics, Chemistry, Manufacturing, and Controls Information.4445This revised draft guidance does not address specific method validation recommendations for46biological and immunochemical assays for characterization and quality control of many drug47substances and drug products. For example, some bioassays are based on animal challenge48models, and immunogenicity assessments or other immunoassays have unique features that49should be considered during development and validation.5051In addition, the need for revalidation of existing analytical methods may need to be considered 52when the manufacturing process changes during the product’s life cycle. For questions on53appropriate validation approaches for analytical procedures or submission of information not54addressed in this guidance, you should consult with the appropriate FDA product quality review 55staff.5657If you choose a different approach than those recommended in this revised draft guidance, we58encourage you to discuss the matter with the appropriate FDA product quality review staff before 59you submit your application.6061FDA’s guidance documents, including this guidance, do not establish legally enforceable62responsibilities. Instead, guidances describe the Agency’s current thinking on a topic and should 63be viewed only as recommendations, unless specific regulatory or statutory requirements are64cited. The use of the word should in Agency guidances means that something is suggested or65recommended, but not required.666768II.BACKGROUND6970Each NDA and ANDA must include the analytical procedures necessary to ensure the identity, 71strength, quality, purity, and potency of the drug substance and drug product.7 Each BLA must 72include a full description of the manufacturing methods, including analytical procedures that73demonstrate the manufactured product meets prescribed standards of identity, quality, safety,74purity, and potency.8 Data must be available to establish that the analytical procedures used in 75testing meet proper standards of accuracy and reliability and are suitable for their intended76purpose.9 For BLAs and their supplements, the analytical procedures and their validation are77submitted as part of license applications or supplements and are evaluated by FDA quality78review groups.79807 See 21 CFR 314.50(d)(1) and 314.94(a)(9)(i).8 See 21 CFR 601.2(a) and 601.2(c).9 See 21 CFR 211.165(e) and 211.194(a)(2).Analytical procedures and validation data should be submitted in the corresponding sections of 81the application in the ICH M2 eCTD: Electronic Common Technical Document Specification.108283When an analytical procedure is approved/licensed as part of the NDA, ANDA, or BLA, it84becomes the FDA approved analytical procedure for the approved product. This analytical85procedure may originate from FDA recognized sources (e.g., a compendial procedure from the 86United States Pharmacopeia/National Formulary (USP/NF)) or a validated procedure you87submitted that was determined to be acceptable by FDA. To apply an analytical method to a88different product, appropriate validation studies with the matrix of the new product should be89considered.909192III.ANALYTICAL METHODS DEVELOPMENT9394An analytical procedure is developed to test a defined characteristic of the drug substance or95drug product against established acceptance criteria for that characteristic. Early in the96development of a new analytical procedure, the choice of analytical instrumentation and97methodology should be selected based on the intended purpose and scope of the analytical98method. Parameters that may be evaluated during method development are specificity, linearity, 99limits of detection (LOD) and quantitation limits (LOQ), range, accuracy, and precision.100101During early stages of method development, the robustness of methods should be evaluated102because this characteristic can help you decide which method you will submit for approval.103Analytical procedures in the early stages of development are initially developed based on a104combination of mechanistic understanding of the basic methodology and prior experience.105Experimental data from early procedures can be used to guide further development. You should 106submit development data within the method validation section if they support the validation of 107the method.108109To fully understand the effect of changes in method parameters on an analytical procedure, you 110should adopt a systematic approach for method robustness study (e.g., a design of experiments 111with method parameters). You should begin with an initial risk assessment and follow with112multivariate experiments. Such approaches allow you to understand factorial parameter effects 113on method performance. Evaluation of a method’s performance may include analyses of114samples obtained from in-process manufacturing stages to the finished product. Knowledge115gained during these studies on the sources of method variation can help you assess the method 116performance.117118119IV.CONTENT OF ANALYTICAL PROCEDURES120121You should describe analytical procedures in sufficient detail to allow a competent analyst to 122reproduce the necessary conditions and obtain results within the proposed acceptance criteria. 123You should also describe aspects of the analytical procedures that require special attention. An 124analytical procedure may be referenced from FDA recognized sources (e.g., USP/NF,12510 See sections 3.2.S.4 Control of Drug Substance, 3.2.P.4 Control of Excipients, and 3.2.P.5 Control of DrugProduct.Association of Analytical Communities (AOAC) International)11 if the referenced analytical126procedure is not modified beyond what is allowed in the published method. You should provide 127in detail the procedures from other published sources. The following is a list of essential128information you should include for an analytical procedure:129130A.Principle/Scope131132A description of the basic principles of the analytical test/technology (separation, detection, etc.); 133target analyte(s) and sample(s) type (e.g., drug substance, drug product, impurities or compounds 134in biological fluids, etc.).135136B.Apparatus/Equipment137138All required qualified equipment and components (e.g., instrument type, detector, column type, 139dimensions, and alternative column, filter type, etc.).140141C.Operating Parameters142143Qualified optimal settings and ranges (allowed adjustments) critical to the analysis (e.g., flow144rate, components temperatures, run time, detector settings, gradient, head space sampler). A145drawing with experimental configuration and integration parameters may be used, as applicable. 146147D.Reagents/Standards148149The following should be listed:150151•Grade of chemical (e.g., USP/NF, American Chemical Society, High152Performance or Pressure Liquid Chromatography, or Gas153Chromatography and preservative free).154•Source (e.g., USP reference standard or qualified in-house reference material). 155•State (e.g., dried, undried, etc.) and concentration.156•Standard potencies (purity correction factors).157•Storage controls.158•Directions for safe use (as per current Safety Data Sheet).159•Validated or useable shelf life.160161New batches of biological reagents, such as monoclonal antibodies, polyclonal antisera, or cells, 162may need extensive qualification procedures included as part of the analytical procedure.163164E.Sample Preparation165166Procedures (e.g., extraction method, dilution or concentration, desalting procedures and mixing 167by sonication, shaking or sonication time, etc.) for the preparations for individual sample tests. 168A single preparation for qualitative and replicate preparations for quantitative tests with16911 See 21 CFR 211.194(a)(2).appropriate units of concentrations for working solutions (e.g., µg/ml or mg/ml) and information 170on stability of solutions and storage conditions.171172F.Standards Control Solution Preparation173174Procedures for the preparation and use of all standard and control solutions with appropriate175units of concentration and information on stability of standards and storage conditions,176including calibration standards, internal standards, system suitability standards, etc.177178G.Procedure179180A step-by-step description of the method (e.g., equilibration times, and scan/injection sequence 181with blanks, placeboes, samples, controls, sensitivity solution (for impurity method) and182standards to maintain validity of the system suitability during the span of analysis) and allowable 183operating ranges and adjustments if applicable.184185H.System Suitability186187Confirmatory test(s) procedures and parameters to ensure that the system (equipment,188electronics, and analytical operations and controls to be analyzed) will function correctly as an 189integrated system at the time of use. The system suitability acceptance criteria applied to190standards and controls, such as peak tailing, precision and resolution acceptance criteria, may be 191required as applicable. For system suitability of chromatographic systems, refer to CDER192reviewer guidance on Validation of Chromatographic Methods and USP General Chapter <621> 193Chromatography.194195I.Calculations196197The integration method and representative calculation formulas for data analysis (standards,198controls, samples) for tests based on label claim and specification (e.g., assay, specified and199unspecified impurities and relative response factors). This includes a description of any200mathematical transformations or formulas used in data analysis, along with a scientific201justification for any correction factors used.202203J.Data Reporting204205A presentation of numeric data that is consistent with instrumental capabilities and acceptance 206criteria. The method should indicate what format to use to report results (e.g., percentage label 207claim, weight/weight, and weight/volume etc.) with the specific number of significant figures 208needed. The American Society for Testing and Materials (ASTM) E29 describes a standard209practice for using significant digits in test data to determine conformance with specifications. For 210chromatographic methods, you should include retention times (RTs) for identification with211reference standard comparison basis, relative retention times (RRTs) (known and unknown212impurities) acceptable ranges and sample results reporting criteria.213214215V.REFERENCE STANDARDS AND MATERIALS216217Primary and secondary reference standards and materials are defined and discussed in the218following ICH guidances: Q6A Specifications: Test Procedures and Acceptance Criteria for 219New Drug Substances and New Drug Products: Chemical Substances (ICH Q6A), Q6B220Specifications: Test Procedures and Acceptance Criteria for Biotechnological/Biological221Products, and Q7 Good Manufacturing Practice Guidance for Active Pharmaceutical222Ingredients. For all standards, you should ensure the suitability for use. Reference standards for 223drug substances are particularly critical in validating specificity for an identity test. You should 224strictly follow storage, usage conditions, and handling instructions for reference standards to225avoid added impurities and inaccurate analysis. For biological products, you should include226information supporting any reference standards and materials that you intend to use in the BLA 227and in subsequent annual reports for subsequent reference standard qualifications. Information 228supporting reference standards and materials include qualification test protocols, reports, and 229certificates of analysis (including stability protocols and relevant known impurity profile230information, as applicable).231232Reference standards can often be obtained from USP and may also be available through the233European Pharmacopoeia, Japanese Pharmacopoeia, World Health Organization, or National 234Institute of Standards and Technology. Reference standards for a number of biological products 235are also available from CBER. For certain biological products marketed in the U.S., reference 236standards authorized by CBER must be used before the product can be released to the market.12 237Reference materials from other sources should be characterized by procedures including routine 238and beyond routine release testing as described in ICH Q6A. You should consider orthogonal 239methods. Additional testing could include attributes to determine the suitability of the reference 240material not necessarily captured by the drug substance or product release tests (e.g., more241extensive structural identity and orthogonal techniques for purity and impurities, biological242activity).243244For biological reference standards and materials, we recommend that you follow a two-tiered 245approach when qualifying new reference standards to help prevent drift in the quality attributes 246and provide a long-term link to clinical trial material. A two-tiered approach involves a247comparison of each new working reference standard with a primary reference standard so that it 248is linked to clinical trial material and the current manufacturing process.249250251VI.ANALYTICAL METHOD VALIDATION FOR NDA, ANDAs, BLAs, AND 252DMFs253254A.Noncompendial Analytical Procedures255256Analytical method validation is the process of demonstrating that an analytical procedure is257suitable for its intended purpose. The methodology and objective of the analytical procedures 258should be clearly defined and understood before initiating validation studies. This understanding 25912 See 21 CFR 610.20.is obtained from scientifically-based method development and optimization studies. Validation 260data must be generated under an protocol approved by the sponsor following current good261manufacturing practices with the description of methodology of each characteristic test and262predetermined and justified acceptance criteria, using qualified instrumentation operated under 263current good manufacturing practices conditions.13 Protocols for both drug substance and264product analytes or mixture of analytes in respective matrices should be developed and executed. 265266ICH Q2(R1) is considered the primary reference for recommendations and definitions on267validation characteristics for analytical procedures. The FDA Reviewer Guidance: Validation of 268Chromatographic Methods is available as well.269270B.Validation Characteristics271272Although not all of the validation characteristics are applicable for all types of tests, typical273validation characteristics are:274275•Specificity276•Linearity277•Accuracy278•Precision (repeatability, intermediate precision, and reproducibility)279•Range280•Quantitation limit281•Detection limit282283If a procedure is a validated quantitative analytical procedure that can detect changes in a quality 284attribute(s) of the drug substance and drug product during storage, it is considered a stability285indicating assay. To demonstrate specificity of a stability-indicating assay, a combination of286challenges should be performed. Some challenges include the use of samples spiked with target 287analytes and all known interferences; samples that have undergone various laboratory stress288conditions; and actual product samples (produced by the final manufacturing process) that are289either aged or have been stored under accelerated temperature and humidity conditions.290291As the holder of the NDA, ANDA, or BLA, you must:14 (1) submit the data used to establish292that the analytical procedures used in testing meet proper standards of accuracy and reliability, 293and (2) notify the FDA about each change in each condition established in an approved294application beyond the variations already provided for in the application, including changes to 295analytical procedures and other established controls.296297The submitted data should include the results from the robustness evaluation of the method,298which is typically conducted during method development or as part of a planned validation299study.1530013 See 21 CFR 211.165(e); 21 CFR 314.50 (d), and for biologics see 21 CFR 601.2(a), 601.2(c), and 601.12(a).14 For drugs see 21 CFR 314.50 (d), 314.70(d), and for biologics see 21 CFR 601.2(a), 601.2(c), and 601.12(a). For aBLA, as discussed below, you must obtain prior approval from FDA before implementing a change in analyticalmethods if those methods are specified in FDA regulations15 See section III and ICH Q2(R1).pendial Analytical Procedures302303The suitability of an analytical procedure (e.g., USP/NF, the AOAC International Book of304Methods, or other recognized standard references) should be verified under actual conditions of 305use.16 Compendial general chapters, which are complex and mention multiple steps and/or306address multiple techniques, should be rationalized for the intended use and verified. Information 307to demonstrate that USP/NF analytical procedures are suitable for the drug product or drug308substance should be included in the submission and generated under a verification protocol.309310The verification protocol should include, but is not limited to: (1) compendial methodology to 311be verified with predetermined acceptance criteria, and (2) details of the methodology (e.g.,312suitability of reagent(s), equipment, component(s), chromatographic conditions, column, detector 313type(s), sensitivity of detector signal response, system suitability, sample preparation and314stability). The procedure and extent of verification should dictate which validation characteristic 315tests should be included in the protocol (e.g., specificity, LOD, LOQ, precision, accuracy, etc.). 316Considerations that may influence what characteristic tests should be in the protocol may depend 317on situations such as whether specification limits are set tighter than compendial acceptance318criteria, or RT or RRT profiles are changing in chromatographic methods because of the319synthetic route of drug substance or differences in manufacturing process or matrix of drug320product. Robustness studies of compendial assays do not need to be included, if methods are 321followed without deviations.322323324VII.STATISTICAL ANALYSIS AND MODELS325326A.Statistics327328Statistical analysis of validation data can be used to evaluate validation characteristics against 329predetermined acceptance criteria. All statistical procedures and parameters used in the analysis 330of the data should be based on sound principles and appropriate for the intended evaluation.331Reportable statistics of linear regression analysis R (correlation coefficient), R square332(coefficient of determination), slope, least square, analysis of variance (ANOVA), confidence 333intervals, etc., should be provided with justification.For information on statistical techniques 334used in making comparisons, as well as other general information on the interpretation and335treatment of analytical data, appropriate literature or texts should be consulted.17336337B.Models338339Some analytical methods might use chemometric and/or multivariate models. When developing 340these models, you should include a statistically adequate number and range of samples for model 341development and comparable samples for model validation. Suitable software should be used for 342data analysis. Model parameters should be deliberately varied to test model robustness.34334416 See 21 CFR 211.194(a)(2) and USP General Chapter <1226> Verification of Compendial Procedures.17 See References section for examples including USP <1010> Analytical Data – Interpretation and Treatment.。
检测晶体常出现的错误

_diffrn_measured_fraction_theta_full Low ....... 0.93 采集数据的完成度偏低,通常要在0.97 以上。
最好是重新收集数据。
解决方法:omit0 50问题:No su's on H-atoms, but refinement reported as . mixed报告中显示对H 原子的修正方法是混合模式但没有列出H 原子相关的偏差。
解决方法:把.CIF 中的"mixed" 改成"constr" 就可以了。
问题:CIF Contains no X-H Bonds ...................... ?CIF Contains no X-Y-H or H-Y-H Angles .......... ?解决方法:在ins 文件中将Bond 改为Bond $h 命令,再在XL 中精修一下就可以了问题:PLAT080_ALERT_2_A Maximum Shift/Error ............................ 0.37 修正轮数不够多Maximum Shift/Error 值应该接近于0,至少要在0.02 以下。
解决方法:增加修正轮数(L.S. 后的数值改大)问题:PLAT220_ALERT_2_A Large Non-Solvent O Ueq(max)/Ueq(min) ... 7.95 Ratio PLAT222_ALERT_3_A Large Non-Solvent H Ueq(max)/Ueq(min) ... 7.78 Ratio 这二条是指原子有较大的非正定,说明没有修正好。
解决方法:可以考虑将这个有较大无序的O 进行分比(如占位率0.5:0.5)再修。
如果是衍射数据质量不够好而无法得到好的修正结果,那只有重新采集数据了。
问题:DENSX01_ALERT_1_A The ratio of the calculated to measured crystal density lies outside the range 0.80 <> 1.20Calculated density = 1.753Measured density = 0.000PLAT093_ALERT_1_A No su's on H-atoms, but refinement reported as . mixed解决方法:在cif 里将_exptl_crystal_density_meas 0 改为:_exptl_crystal_density_meas ?将_refine_ls_hydrogen_treatment mixed改为:_refine_ls_hydrogen_treatment constrPLAT211_ALERT_2_A ADP of Atom C3 is NPD ..................... ? 解决方法:原子非正定,无序或者空间群错误,或者数据不好。
Non-constanterrorvariance
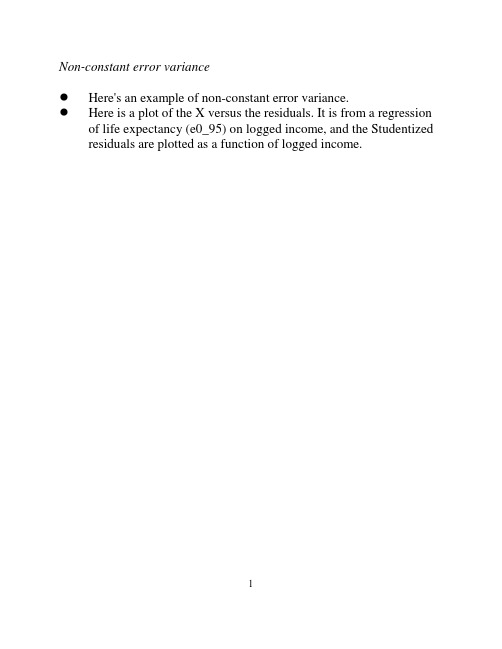
Non-constant error varianceHere's an example of non-constant error variance.Here is a plot of the X versus the residuals. It is from a regression of life expectancy (e0_95) on logged income, and the Studentized residuals are plotted as a function of logged income.12p r e s i dl P c G D P 95 3.5835210.6553-3.772373.2514F r a c t i o npresid - 3.772373.2514.207865Here we see that there is a pattern to the residuals.Note that this occurs even though the distribution of residuals appears sort of normal:We don’t seem to improve the situation much by transforming life expectancy. Here’s the residual plot after regressing logged lifeexpectancy:3p r e s i d 3lPcGDP95 3.5835210.6553-3.850283.0907p r e s i d 4lPcGDP953.5835210.1496-2.93662.00008Sometimes nonconstant error variance is a sign that something important hasn ’t been controlled for.Here ’s what we get after controlling for female illiteracy:Female illiteracy seems to have a strong association with life expectancy, and it varies more with countries at low levels ofincome.4CollinearityOne problem that can wreak havoc with a regression is collinearity , or as it is sometimes called, multicollinearity .This occurs when an independent variable has a strong association with one or more of the other independent variables. Why is this? Recall the formula for the sample variance of the least-squares estimate of the coefficient in a multiple regression:Remember that the numerator on the right is the error variance, and S j 2 is the variance of variable X j .R j 2 is the coefficient of multiple correlation from the regression of X j on the other independent variables in the model.As R j 2 approaches 1, i.e. the association between X j and the other independent variables becomes stronger, 1 over 1-R j 2 begins to increase quite rapidly. When R j 2 reaches 1, this term explodes completely.The implication is that as R j 2 approaches 1, the sampling variance of the coefficient B j becomes very large. The standard error on the estimate grows accordingly.The estimate of B j, in other words, is likely to vary considerably from one sample to the next. In some cases this leads to bizarrely large coefficient estimates.There are a variety of purported remedies for the problem of collinearity, and according to Fox most of them are suspect.Before we discuss them, let's clarify when collinearity is likely to present a problem.5yx 0.1.2.3.4.5.6.7.8.9112345678910When is collinearity a problem?The extent to which collinearity is a problem in a model depends on the precise value of the 1/(1-R j 2), which the book refers to as the VIF, or variance inflation factor.In practice, values of R j 2 up to 0.6 or 0.7 are not necessarily too problematic. Once you get above those values, however, problems escalate rapidly.The following, which plots 1/(1-R j 2) as a function of R j 2, clarifies why this is the case:Out to r 2 = 0.7 or 0.8, the sampling variance of the coefficient is only being doubled or tripled. This is bad, but not disastrous,especially if the sample is large.Past this, however, the sampling variance of the coefficient begins to skyrocket.How can it be detected?Pairwise correlations generated by the correlate command may identify situations where pairs of independent variables are highly correlated.Another tip-off that there may be a problem is the presence of coefficients with bizarrely large magnitudes and often opposingsigns.Also, coefficients that change dramatically with the addition or deletion of small numbers of cases may suggest a problem.Another way to detect collinearity is if the standard errors of the coefficients change a lot when you add other variables to theequation.If you suspect something, you can regress the independent variables on each other to check.In practice when is it likely to occur?Collinearity can be a frequent problem in certain types of time series analysis.In sociology, one area where this tends to crop up is in studies that attempt to include effects of age, period, and cohort, all at the same time.Interaction terms are also collinear (since they are justcombinations of variables already in the equation). It is generallyuseful to take them out of the equation if they are not significant.6What can be done about it?Increasing the sample size may help to reduce the sampling variance of the coefficient estimate.Better measurement of the variables may reduce the error variance. If collinearity is an issue because more than one variable are in theory measuring the same underlying dimension, combining ordiscarding variables may help.If the variables do not in theory measure the same underlying dimension, or dimensions, then you have a serious problem. You may need to reconsider the substantive questions you areattempting to investigate with the model, or seek additional data to add to the model.There are some purported 'fixes' available but using these may have a penalty. Either implicitly or explicitly, you may be adding constraints to the model. You may also be introducing biases inthe coefficient estimates.But, in general not too much will work, actually.7。
- 1、下载文档前请自行甄别文档内容的完整性,平台不提供额外的编辑、内容补充、找答案等附加服务。
- 2、"仅部分预览"的文档,不可在线预览部分如存在完整性等问题,可反馈申请退款(可完整预览的文档不适用该条件!)。
- 3、如文档侵犯您的权益,请联系客服反馈,我们会尽快为您处理(人工客服工作时间:9:00-18:30)。
then,
OP2 Ϫ OQ2 ⌬ = OP Ϫ OQ =
OP + OQ
and ␦ are small angles, so cos Ϸ 1, sin Ϸ tan Ϸ Ϸ 0 and sin ␦ Ϸ ␦,
⌬ Ϸ L ϫ (cos(2 Ϫ ␦) ϫ sin ␦ ϫ tan + tan2 sin(2 Ϫ ␦)
position and orientation of the body. If there is an error within the orthogonality (squareness error), the measured values will not be consistent. Therefore, some methods have been developed using these differences to find out the squareness error with uncalibrated reference artefacts [6]. Predominantly, these methods are 2D-based and require the artefact to be placed at positions of 90° to each other or 45° to the two axes ( in Fig. 1 is 45°). A formula can be given as:
ϫ
(OG2 (OG2
+ +
BE2) BE2)
Ϫ +
(CF2 (CF2
+ +
AD2) AD2)
XWY Ϸ
2 sin ␣ cos ␣
[rad] (4)
Both ␣ and  are arbitrary angles (Ͼ20°). They can be chosen to fit different CMM volumes and artefact lengths, and it is allowable to have slight errors (preferably less than 0.5°) of these two position angles at the different placements of the artefact (four diagonals).
3. Deduction of the Method
3.1 Small Angular Errors with Artefact Positions
Figure 3 presents a simplified 2D model. If the exact length is L and there is a small angular difference ␦, between two positions OP and OQ, then the CMM with a squareness error XWZ of will (wrongly) measures the same length at OP and OQ as:
Fig. 1. The layout of the method in 2D.
Squareness Error Verification 875
Fig. 2. Layout of the 3D method.
Fig. 3. Minor influence of small misalignment.
Now, if the angular errors (positive when they are more than 90° and negative when they are less than 90°) between axes are XWY, XWZ, and YWZ, then:
J.-P. Kruth, L. Zhou, C. Van den Bergh and P. Vanherck
K. U. Leuven, Division PMA, Celestijnenlaan 300B, 3001 Heverlee, Belgium
The face diagonal method for squareness error measurement is already well established. Because it is easy to apply, this method is widely used in CMMs (coordinate measuring machines). This paper introduces a new method, which reduces the set-up and measuring time for squareness error by applying four volumetric diagonal measurements instead of six face diagonal measurements. The method also makes it possible to use a single properly sized artefact, which fits the machine’s rectangular volume. The choice of artefacts used in the method is wide, and they need not be precalibrated before measurements.
sin ␦/2) Ϸ L × cos (2) ␦
cos(2 Ϫ ␦) Ϸ cos 2.
Suppose ␦ = Ϯ0.5°, = 1 ϫ 10Ϫ4 rad, and  = 30°, L = 1 m, then the difference between OP and OQ is less than 0.5 m. If a volumetric diagonal is measured with the above definitions unchanged, the difference will be less than 1 m when the ␣ error is less than Ϯ 0.5° and the squareness errors of X-Z and Y-Z are both 1 ϫ 10Ϫ4 rad. Because of these negligible differences, operators do not have to completely remove the small angular errors in placement, which saves much alignment effort. For higher accuracy, it is suggested that ␣ and  be reasonably close to 45° and the artefact be positioned as accurately as possible (small ␦) so that the differences will be minimised for better results.
Ϸ
OB2 OB2
Ϫ +
AC2 AC2
[rad]
is the squareness error, OB and AC are measured values of the same artefact at positions O-B and A-C.
As an extension, the new method first allows the angle  to be an arbitrary angle (though preferably not smaller than 20°), which means that the arms of OB and AC are not necessarily perpendicular. The squareness error (angle ) of the two axes (X and Z) is calculated as follows (Fig. 1):
Keywords: Artefact; CMM; Error compensation
1. Introduction
The performance verification of coordinate measuring machines (CMMs) has been extensively studied and many methods have been developed based on artefacts (i.e. gauge blocks, step gauges, engineer’s squares, ball plates, and ball bars) or optical systems [1–5]. The face diagonal method for squareness error measurement is one of the well-established methods. Because it is easy to apply, this method is widely used in CMMs. This paper introduces a new method, which reduces the set-up and measuring time for squareness error by applying four volumetric diagonal measurements instead of six face diagonal measurements. The method also makes it possible to use a single properly sized artefact, which fits the machine’s rectangular volume. The choice of artefacts for the method is wide, and they need not be precalibrated before measurements.