A Database Model for Querying Visual Surveillance Videos by Integrating Semantic and Low-Le
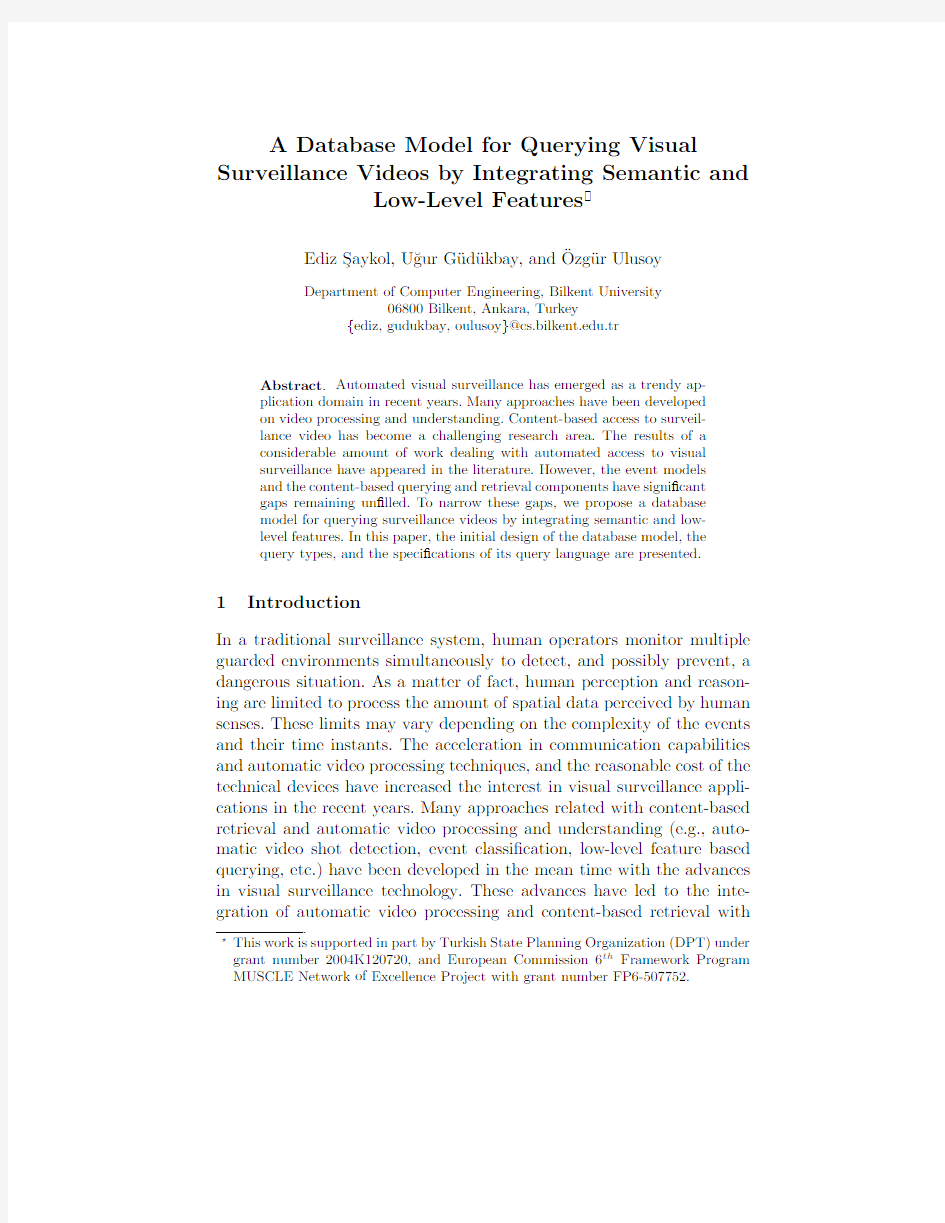

A Database Model for Querying Visual Surveillance Videos by Integrating Semantic and
Low-Level Features
Ediz S?aykol,U?g ur G¨u d¨u kbay,and¨Ozg¨u r Ulusoy
Department of Computer Engineering,Bilkent University
06800Bilkent,Ankara,Turkey
{ediz,gudukbay,oulusoy}@https://www.360docs.net/doc/109131085.html,.tr
Abstract.Automated visual surveillance has emerged as a trendy ap-
plication domain in recent years.Many approaches have been developed
on video processing and understanding.Content-based access to surveil-
lance video has become a challenging research area.The results of a
considerable amount of work dealing with automated access to visual
surveillance have appeared in the literature.However,the event models
and the content-based querying and retrieval components have signi?cant
gaps remaining un?lled.To narrow these gaps,we propose a database
model for querying surveillance videos by integrating semantic and low-
level features.In this paper,the initial design of the database model,the
query types,and the speci?cations of its query language are presented.
1Introduction
In a traditional surveillance system,human operators monitor multiple guarded environments simultaneously to detect,and possibly prevent,a dangerous situation.As a matter of fact,human perception and reason-ing are limited to process the amount of spatial data perceived by human senses.These limits may vary depending on the complexity of the events and their time instants.The acceleration in communication capabilities and automatic video processing techniques,and the reasonable cost of the technical devices have increased the interest in visual surveillance appli-cations in the recent years.Many approaches related with content-based retrieval and automatic video processing and understanding(e.g.,auto-matic video shot detection,event classi?cation,low-level feature based querying,etc.)have been developed in the mean time with the advances in visual surveillance technology.These advances have led to the inte-gration of automatic video processing and content-based retrieval with This work is supported in part by Turkish State Planning Organization(DPT)under grant number2004K120720,and European Commission6th Framework Program MUSCLE Network of Excellence Project with grant number FP6-507752.
visual surveillance systems.Due to the highly variable nature of visual surveillance videos,a need has arisen for robust scene processing and event recognition.
In our work,the main focus is on indoor monitoring,and a framework for querying surveillance videos by integrating semantic and low-level fea-tures is developed.Regarding this issue,some powerful systems(e.g.,[1–4])exist in the literature.Besides system-level integration,some methods have been proposed for smaller units(e.g.,detecting scene changes[5,6], moving object detection and tracking[7]).As far as the database index-ing and retrieval parts are concerned,the researchers generally designed simple database structures for events.The event descriptors stored in a database generally contain the start and?nish times,and the salient ob-ject labels.Indexing at the object feature level is not as frequent as the event level.In[8],the authors proposed an approach for tra?c surveil-lance and stored object motion in the database.There are also some ap-proaches that deal with the color information of the video objects(e.g.,[2, 4]).However,their use is either to keep track of the video objects or to classify the video objects.
The main contribution of the proposed framework is the querying capability by integrating semantic features(i.e.,events,sub-events,and salient objects)and low-level object features(i.e.,color,shape,and tex-ture)for surveillance videos.To the best of our knowledge,no systems ex-ist that are embedding object-based low-level features(e.g.,color,shape, and texture)to the indexing and retrieval module in visual surveillance domain.Moreover,the framework provides support for e?ective query speci?cation(e.g.,query-by-example,query-by-sketch)and retrieval as opposed to keyword-based database searches.In the following,we de-scribe our motivations and the basic assumptions we made in our work.–Enriching the querying module with low-level object features(color, shape,texture)is more meaningful for indoor surveillance.We might need to query an event to detect an intruder in a supermarket by specifying the color of his coat,texture on his shirt,etc.This low-level feature enrichment would possibly decrease the rate of false alarms.
For the intruder detection example,it is possible that there exist many innocent people making the same type of actions(events)in the su-permarket at that time,causing a signi?cant post-processing for the retrieved persons to?nd the intruder,or generate false alarms.
–Static camera and constant light source are assumed for the sake of simplicity in terms of database modeling.These assumptions?t most to indoor environments than the other categories.Although there are
some recent approaches assuming inputs from two?xed cameras for indoor environments[9],the way of processing object motions is very di?erent and sophisticated(e.g.,contour matching and tracking in 3D)for multi-camera surveillance approaches.
–The pre-processing steps for event/object indexing into the database for querying are more straightforward in indoor https://www.360docs.net/doc/109131085.html,plex background scenes are rare and the object motions(e.g.,motions of humans)are generally expectable.This is basically because of the fact that indoor environments are generally simpler than others.
The rest of this paper is organized as follows:Section2summarizes some related studies.The proposed database model is presented in Sec-tion3.Finally,Section4concludes the paper.
2Related Work
Video Surveillance and Monitoring(VSAM)system presented in[3]is one of the complete prototypes for object detection,object tracking and classi?cation,as well as calibrating a network of sensors for a surveillance environment.In that work,a hybrid algorithm was developed,which is based on an adaptive background subtraction by three-frame di?erenc-ing.The background update scheme is based on a classi?cation of pixels (either moving or non-moving)performed by a simple threshold test.A model is provided on temporal layers for pixels and pixel regions in order to be robust for detection of stop-and-go type of object motions.The background maintenance scheme we employ is similar to that of VSAM. However,in our framework,the extracted background is also used for both event annotation and object tracking.
Stringa and Regazzoni[1,10,11]proposed a real-time surveillance sys-tem employing semantic video-shot detection and indexing.Lost objects are detected by the help of temporal rank order?ltering.The interesting video shots are detected by a hybrid approach based on low-level(color) and semantic features.The authors have adapted a change-detection mod-ule that processes the background image and the current image to detect the stationary object regions.Retrieving all the clips related to an alarm is the basic way of querying the system.The authors also mention about more complex query types including color and/or shape properties of the dangerous object.However,no details are provided for the storage of these low-level object features and their indexing and usage within complex queries.In our framework,we extract object-based low-level fea-
tures,and provide a scenario-based querying scheme for complex querying including color and shape descriptors of the objects.
In[12,13],an object-based video abstraction model was proposed.The authors employed a moving-edge detection scheme for video frames.The edge map of a frame is extracted?rst by using Canny edge-detector[14]. The extracted edge map is compared with the background edge map and the moving edges and regions are detected at the end of this process. They employed a semantic shot detection scheme to select object-based keyframes.When a change occurs in the number of moving regions,the current frame is declared as a keyframe indicating that an important event has occurred.This scheme also facilitates the detection of impor-tant events.If the number of moving objects remains the same for the next frame,then a shape-based change detector is applied to the con-secutive frames.A frame-based similarity metric is also de?ned to detect the distance between two frames.Our framework employs the strategy of moving object counting mentioned in[12]with rule-based extensions to help the event annotation process.
In[8],Jung et al.proposed a content-based event retrieval framework for tra?c surveillance videos using semantic scene interpretation tech-niques.They employed an adaptive background subtraction and update mechanism,where the background image eventually contains the tempo-ral median values of pixels.One of the important aspects of the work is that they designed the database indexing and retrieval in an object-based manner.However,since their primary concern is tra?c surveillance,the object trajectories and motion descriptors are stored in the database. Their query interface supports query-by-example,query-by-sketch,and query-by-weighting on the trajectory descriptors.The database is searched exhaustively to?nd the best matches for a given query.Our framework also includes object-based querying by providing examples or sketches. However,our querying module also enables speci?cation of more complex queries including low-level and directional descriptors.
Lyons et al.[15]developed video content analyzer(VCA),the main components of which are background subtraction,object tracking,event reasoning,graphical user interface,indexing,and retrieval.They adapted a non-parametric background subtraction approach based on[16].A?-nite state model was designed for object tracking.VCA discriminates people from objects and the main events recognized are as follows:en-tering scene,leaving scene,splitting,merging,and depositing/picking-up. The retrieval component was designed to retrieve video sequences based on event queries.The event categories are very similar to those we use
in our framework.However,as an additional feature,our framework also enables object-based querying that can be re?ned by providing low-level and/or directional descriptors.
Brodsky et al.[4]designed a system for indoor visual surveillance especially in the retail stores and in the houses.They assumed a stationary camera and used background subtraction technique described in[15].The pixels detected as foreground are grouped into connected components and tracked throughout the scene.Object tracking module handles merging and splitting of moving objects by making use of a color model extracted for each moving object.A list of events that the object is participated are stored for each object,where the events are simply entering,leaving, merging,and splitting.The system also includes a classi?cation module to distinguish people,pets,and other objects.One of the distinctions of this system and our framework is the use of color feature.In this system, the color feature of the objects is mainly used for reassigning labels for moving connected components,whereas,as an extension,we also provide object-based querying based on color and/or shape features.
Haritao?g lu et al.[17]proposed a real-time analysis of people activi-ties.Their model uses a stationary camera and background subtraction to detect the regions corresponding to person(s).Their system,called W4,uses shape information to locate people and their body parts(head, hands,feet,and torso).The system operates on monocular gray-scale video data,and no color cues are used.The creation of models of the appearance of person(s)helps the tracking process through people inter-action(e.g.,occlusions),and simultaneous activities of multiple people. The system uses a statistical background model holding bimodal distri-bution of intensity at each pixel to locate people.The system is capable of detecting single person,multiple persons,and multiple person groups in various postures.
In the literature,most of the systems have focused on(unattended)ob-ject detection and tracking moving object.Extracted event information, based on the object tracking and shot detection modules,is generally stored in a database and exhaustively searched when a query is submit-ted based on event information(e.g.,[15]).From another perspective, retrieving the video sequences related to a generated alarm is the basic way of querying the system(e.g.,[1]).In[1],the authors also mentioned more complex query types including color and/or shape properties of the salient objects.The querying module of[8]supports query-by-example, query-by-sketch,and query-by-weighting on the trajectory descriptors of moving objects.
Video
Database Population Process
Querying & Retrieval Process
Fig.1.The overall architecture of the framework.
3A Database Framework for an Integrated Querying of Visual Surveillance
We propose a framework which provides an integrated environment for querying indoor surveillance videos by semantic(event-based)and low-level(object-based)features.The overall architecture of the framework is shown in Figure1.The queries are handled by query processing mod-ule,which communicates with both the feature database and the content-based retrieval module.The database contains event and object features extracted by automated tools.The visual query interface is used to sub-mit queries to the system and to visualize the query results.The users should be able to specify event queries enriched with low-level features for objects and directional descriptors for events.
3.1Object Extraction and Tracking Module
A fully-automatic object detection and tracking module is designed and implemented,which employs an adaptive background maintenance scheme, similar to the one proposed in[3].At the pixel-level,the algorithm tries to identify the moving object pixels.The adaptive background subtraction technique is combined with a three-frame di?erencing technique to deter-mine the moving object pixels as a region and also to detect stop-and-go type of object motions.According to the three-frame di?erencing algo-rithm,an object is assumed to be moving if the intensities of the object
have changed between current and previous frames,and between current and next-to-previous frames.
Video data can be considered as a sequence of frames.Let I f(x,y)de-note the intensity value of a pixel at(x,y)at frame f.Hence,M f(x,y)=1 if(x,y)is moving at frame f,where M f(x,y)is a vector holding mov-ing pixels.A threshold vector T f(x,y)for a frame f is needed for de-tecting pixel motions.The basic test condition to detect moving pixels with respect to T f(x,y)can be formulated as follows:M f(x,y)=1if (|I f(x,y)?I f?1(x,y)|>T f(x,y))and(|I f(x,y)?I f?2(x,y)|>T f(x,y)).
The(moving)pixel intensities that are larger than the background intensities(B f(x,y))are used to?ll the region of a moving object.This step requires a background maintenance task based on the previous inten-sity values of the pixels.Similarly,the threshold is to be updated based on the observed moving pixel information at the current frame.A statis-tical background and threshold maintenance scheme is employed in the module as follows:
B0(x,y)=0,(1)
B f(x,y)=
αB f?1(x,y)+(1?α)I f?1(x,y),M f(x,y)=0,
B f?1(x,y),M f(x,y)=1,
(2)
T0(x,y)=1,(3)
T f(x,y)=
αT f?1(x,y)+(1?α)(k×|I f?1(x,y)?B f?1(x,y)|),M f(x,y)=0,
T f?1(x,y),M f(x,y)=1,
(4)
where k is set to5in Eq.4.As argued in[3],B f(x,y)is analogous to local temporal average of pixel intensities,and T f(x,y)is analogous to k times the local temporal standard deviation of pixel intensities computed with an in?nite impulse(IIR)?lter.A snapshot of object extraction and tracking module is shown in Figure2.
3.2Shot Boundary Detection Module
Object tracking and event classi?cation tasks directly bene?t from the extracted video shot s.Moving objects generally enter or leave the scene at shot boundaries.Hence,the shot boundary detection module is partic-ularly designed for detecting video shot boundaries,hence cut s.The shot boundary detection algorithm employed can be summarized as follows: Let f i and f i?1denote two consecutive frames in a video clip.Let I f denote the intensity histogram of a frame f.For a consecutive frame pair
Fig.2.Object extraction and tracking module user interface.Top-left image is the original image,top-right is the intensity layer image,bottom-left is the background layer image,and bottom-right is the object layer image where moving object pixel region can be seen.
(f i?1,f i),let d i denote the histogram intersection[18]distance between
I f
i?1and I f
i
.By using histogram intersection technique,d i can be found
by
d i=1?S I
f i,I f i?1=1?
n
i
min(I f
i
[i],I f
i?1
[i])
min(|I f
i
|,|I f
i?1
|)
,(5)
where|I f
i |denotes the L1-norm(i.e.,length)of an histogram I f
i
.If
d i≥t1,then i is introduced as a shot boundary,or a cut.Th
e value for t1is estimated by trial-error technique and it is found that0.3763gives best results for the video clips tested so far.A further improvement is made on the algorithm to increase the success rate.The algorithm starts with a lower t1value and extracts shot boundary candidates?rst.Then, it computes pixel-wise distance d p i between
f i?1and f i,where i is a shot boundary candidate.If d p i≥t2,then i is introduced as a shot boundary. The experiments show that t1=0.3and t2=0.13give promisin
g results.
3.3Event Information Extraction Module
This module is intended to‘annotate’video frame intervals based on event labels,objects appearing within the interval,and low-level feature descriptions for the event objects.There are some speci?c types of events, which are important for indoor visual surveillance to detect suspicious events.A person depositing or picking up an object and two people crossing over are examples for two important events.People/object entering or leaving the scene,people/object joining or splitting can be considered as sub-events to detect suspicious events[15].
The inputs to this module are the moving objects(or moving object groups)at a frame.While detecting moving object groups,our object tracking algorithm bene?ts from the algorithm discussed in[7].A moving object group is identi?ed as a moving object until the objects in the group are separated.Counting the number of moving objects also gives an important clue for detecting the events,since the number of moving objects changes at the time of the events.We also keep track of spatial (directional and topological)relations among the moving objects within the event detection algorithm.
Figure3illustrates the detecting of events.For example,deposit event is detected as follows:The object tracking module detects A and B as a moving object group?rst at time T1.Then,they are identi?ed as two separate moving objects by the same module through some algorithms used in[7].Hence,at time T2,the objects A and B are detected as they are moving separately.At time T2+1,the only detected moving object is A.Therefore,a potential deposit event is detected at frame T2.The spatial relations among the moving objects are tracked in addition to the outputs of object tracking module,which helped the detection process. The detection process for the other types of events are similar to the deposit event.Re?nements on this event detection algorithm have been carried out continuously to end up with a better scheme.
The event information extraction module can be summarized as fol-lows:based on the above depositing event example,the event is detected at time T2.Hence,the event information is stored based on the status at time T2.This type of information storage is reasonable because the status at the time of the suspicious event is of interest while querying.For each frame that an event is identi?ed,the objects appearing on that frame(A and B in this example)are stored in Event and Object Database along with their low-level features and the spatial relations among them.The low-level object features stored are color vector and shape vector,which is a composition of angular span and distance span[21].
1 moving object group … T1
T2 T2+1 2 moving objects 1 moving object
(a)
1 moving object
…
T1 T2 T2+1 2 moving objects
1 moving object group (b)
2 moving objects T1
T2 T2+1 1 moving object group 2 moving objects
(c)
Fig.3.Detection of Events.(a)Deposit,(b)Pick Up,and (c)Crossover.
3.4Query Processing Module
One of the most important tasks in automated visual surveillance is the query processing module.Basically textual searches for event queries are supported in the existing systems.Some systems support object queries as well to some extent.It is observed that there might be a need for enhancing object queries with color and/or shape descriptions.In addition to this enhancement,allowing directional relation speci?cations among objects within suspicious events might be helpful in some domains.
Query Types:The main contribution of the querying module in our system is providing support to a wide range of event and object queries.
A classi?cation of the types of queries handled can be listed as follows:
–Single Object Queries,
?Object Entering/Leaving Scene,
–Multi-Object Queries,
?Object Depositing/Object Picking Up,
?Objects Crossing Over.
We plan to extend the framework to support queries based on sus-picious events in surveillance videos.By adding low-level and/or spa-tial sub-queries,more complex queries can be submitted to the system. Spatial sub-queries are more meaningful for queries involving multiple objects.However,low-level sub-queries can be supplied for each object. In real-life applications,low-level sub-query speci?cation with high-level descriptors might be su?cient(e.g.,a man with a black coat entering scene).
Query Language:An SQL-based querying language,which we call Vi-sual Surveillance Query Language(VSQL),is designed to provide support for integrated querying of indoor visual surveillance videos by spatial,se-mantic,and low-level features.The query processing module extends the querying capability by allowing low-level object feature speci?cations as well as spatial relationships among the objects.In short,VSQL provides support for semantic(suspicious event),spatial(relationships among ob-jects),and low-level(object features at a speci?c suspicious event)sub-queries for visual surveillance domain.Semantic sub-queries can be cou-pled with either spatial or low-level,or both,to form complex queries.A grammar for VSQL is presented in Appendix A.
VSQL identi?es two single-object event conditions(enter,leave),and three multi-object event conditions(deposit,pickup,and crossover)in a scenario.The moving objects are classi?ed into four categories,namely person,object,pet,other,by a classi?cation tool,which is developed out-side the scope of the work in this paper[7].The query processor counts the number of moving objects speci?ed in a query by tracing the enter event conditions.A timegap value can be speci?ed between event condi-tions.The order of the events is considered to be unimportant if a timegap value is not speci?ed for an event condition pair of the same type(i.e., pair of single-object conditions or pair of multi-object event conditions).
Query Processing:The event and object information is stored in the database is based on the frames at which an event is detected.This in-cludes the low-level features of the objects appearing on that frame and the spatial relations among them.By keeping the directional relations among the objects,event querying can be re?ned by specifying direc-tional predicates in the query.For example,while querying an event of ‘a person depositing an object’,the query can be enriched by directional predicates so that‘a person depositing an object to his west’can also be queried.Moreover,since the low-level features of moving objects are also stored,more detailed queries can be submitted to the system e?ectively.
Based on the observation that rule-based model is e?ective for query-ing video databases[19,20],a rule-based model is designed for query-ing visual surveillance videos.Our framework is to provide support for scenario-based queries which are very di?cult to handle in real-time sys-tems.The submitted queries are sent to Prolog,which processes the extracted event and object information is stored in the database(i.e., knowledge-base)based on a set of rules.Prior to the processing by Pro-log,the query string is passed through lexical analyzer and a parse tree is created.We are planning to embed a similarity-based metric in this querying process,which will be used as a con?dence value for the user.
3.5Content-Based Retrieval Module
In this section,some real-life querying scenarios are provided.We as-sumed a lobby of a hotel as our indoor environment for these scenarios. Since our system focuses on database querying and retrieval issues,it is assumed that there is a need to query the suspicious situation given in the scenarios.A real-time visual surveillance system has to detect such cases and inform the human operator for more security.It is also possible that the human operator does not see any danger in the situation at the time of the event.However,he may be informed later on that a danger-ous situation has happened in one of the events triggered by the system. Based on this argument,the assumption of the need to query suspicious situations is not super?cial.
Scenario1:A person with a black coat enters a lobby.
select objectA from1where
objectA=objdata(class=person,color=black)and enter(objectA)
The event is a simple object appearance type of query.It is assumed that the dominant color of the person is black,which is the color of his coat.
Scenario2:A person enters a lobby with a bag,deposits his bag,and leaves the lobby.
select segment from1where
objectA=objdata(class=person),objectB=objdata(class=object)
and enter(objectA)enter(objectB)deposit(objectA,objectB)leave(objectA) The event described in this scenario is very crucial because unattended bags are one of the primary sources of suspicious situations in indoor environments.Additional descriptors for both person and bag improve the quality of the retrieval.Directional descriptors can be added as well since the directional relations are stored.Hence,the query phrase‘deposits his bag’can be re?ned by‘deposits his bag to his west’,which might give better results.Then,the deposit condition will be as follows:
deposit(objectA,objectB,west)
Scenario3:A person enters a lobby with a bag,after3seconds another person enters the lobby,two persons meet and exchange the bag,then they leave the lobby.
select segment from1where
objectA=objdata(class=person),objectB=objdata(class=
object),
objectC=objdata(class=person)and
enter(objectA)enter(objectB)3enter(objectC)
crossover(objectA,objectC)deposit(objectA,objectB)
pickup(objectC,objectB)leave(objectA)leave(objectC)
This scenario is an example of a‘cross-over’,and generally real-time sys-tems detect such events and inform human operators.If we consider a quite crowded lobby,the number of crossovers is relatively large.Hence, providing additional descriptors for persons and/or bag(both low-level and directional)decreases the number of possible results of querying. This directly shortens the time and helps the security persons to catch the intruders.
Scenario4:A person with a black coat enters a lobby with a yellow bag, deposits the bag,another person with a white coat enters the lobby,picks up the bag.
select segment from1where
objectA=objdata(class=person,color=black),
objectB=objdata(class=object,color=yellow),
objectC=objdata(class=person,color=white)and
enter(objectA)enter(objectB)deposit(objectA,objectB)
enter(objectC)pickup(objectC,objectB)
This scenario describes a sequence of deposit and pickup events without crossover.Color descriptors are added for all of the objects acting in the scenario.The leaving times of the persons are not of interest for the querying of the event.
As mentioned in the scenarios,the suspicious events can be queried by adding low-level features to(moving)objects and/or directional relations for the event.This type of querying improves the retrieval quality and decreases the search space.These gains are more meaningful when the number of events to be searched in the database is relatively large.
4Conclusion
In this paper,we propose a database model for an integrated querying of visual surveillance videos by semantic and low-level features.The ap-plication domain chosen is indoor monitoring environment video,which ?ts most into our assumptions and which is simpler than other complex environments.The system has a database population process that ex-tracts necessary event and object information for e?ective querying.An SQL-based querying language is designed to express the query types.A wide range of event and object queries including semantic,spatial,and low-level is supported.
As the database modeling process is evolving,improvements will be made both in terms of database population and querying-and-retrieval processes.The design and implementation of the query processing module is an ongoing project,hence the above improvements will be employed accordingly to handle a wide range of query set e?ectively. References
1.Stringa,E.,Regazzoni,C.:Real-time video-shot detection for scene surveillance
applications.IEEE Trans.on Image Processing9(2000)69–79
2.Foresti,G.,Marcenaro,L.,Regazzoni,C.:Automatic detection and indexing of
video-event shots for surveillance applications.IEEE Trans.on Multimedia4 (2002)459–471
3.Collins,R.,Lipton,A.,Kanade,T.,Fujiyoshi,H.,Duggins,D.,Tsin,Y.,Tol-
liver,D.,Enomoto,N.,Hasegawa,O.,Burt,P.,Wixson,L.:A system for video surveillance and monitoring.Technical Report CMU-RI-TR-00-12,Carnegie Mel-lon University,The Robotics Institute(2000)
4.Brodsky,T.,Cohen,R.,Cohen-Solal,E.,Gutta,S.,Lyons,D.,Philomin,V.,Tra-
jkovic,M.:Visual surveillance in retail stores and in the home.In:Video-Based Surveillance Systems:Computer Vision and Distributed Processing,Kluwer Aca-demic Pub.(2001)51–65
https://www.360docs.net/doc/109131085.html,tecki,L.,Wen,X.,Ghubade,N.:Detection of changes in surveillance videos.
In:IEEE Conf.on Adv.Video and Signal Based Surv.(AVSS’03).(2003)237–242 6.Stefano,L.D.,Mattoccia,S.,Mola,M.:A change-detection algorithm based on
structure and colour.In:IEEE Conf.on Adv.Video and Signal Based Surv.
(AVSS’03).(2003)252–259
7.T¨o reyin,B.,C?etin,A.,Aksay,A.,Akhan,M.:Moving object detection in wavelet
compressed video.Signal Processing:Image Communication20(2005)255–264 8.Jung,Y.,Lee,K.,Ho,Y.:Content-based event retrieval using semantic scene in-
terpretation for automated tra?c surveillance.IEEE Trans.on Intelligent Trans-portation Systems2(2001)151–163
9.Eaton,R.,Scassellati,B.:ViSIT:Visual surveillance and interaction tracking.In:
https://www.360docs.net/doc/109131085.html,/classes/cs490/02-03a/ross.eaton/.(Social Robotics Laboratory,Yale University,accessed at February27,2005)
10.Stringa,E.,Regazzoni,C.:Content-based retrieval and real time detection from
video sequences acquired by surveillance systems.In:Int.Conf.on Image Process-ing.(1998)138–142
11.Regazzoni,C.,Sacchi,C.,Stringa,E.:Remote detection of abandoned objects in
unattended railway stations by using a DS/CDMA video surveillance system.In Regazzoni,C.,Fabri,G.,Vernezza,G.,eds.:Advanced Video-Based Surveillance System,Boston,MA:Kluwer(1998)165–178
12.Kim,C.,Hwang,J.:Fast and automatic video object segmentation and tracking
for content-based applications.IEEE Trans.on Circuits and Systems for Video Technology12(2002)122–129
13.Kim,C.,Hwang,J.:Object-based video abstraction for video surveillance systems.
IEEE Trans.on Circuits and Systems for Video Technology12(2002)1128–1138 14.Canny,J.:A computational approach to edge detection.IEEE Trans.on Pattern
Analysis and Machine Intelligence8(1986)679–698
15.Lyons,D.,Brodsky,T.,Cohen-Solal,E.,Elgammal,A.:Video content analysis for
surveillance applications.In:Philips Digital Video Technologies Workshop.(2000) 16.Elgammal,A.,Harwood,D.,Davis,L.:Non-parametric model for background
subtraction.In:Int.Conf.on Computer Vision and Pattern Recognition,Workshop on Motion.(1999)
17.Haritao?g lu,˙I.,Harwood,D.,Davis,L.:W4:Real-time surveillance of people and
their activities.IEEE Trans.on Pattern Analysis and Machine Intelligence22 (2000)809–830
18.Swain,M.,Ballard,D.:Color indexing.Int.J.of Comp.Vis.7(1991)11–32
19.D¨o nderler,M.,S?aykol,E.,Arslan,U.,Ulusoy,¨O.,G¨u d¨u kbay,U.:BilVideo:Design
and implementation of a video database management system.Multimedia Tools and Applications(accepted for publication)(2005)
20.D¨o nderler,M.,¨O.Ulusoy,G¨u d¨u kbay,U.:Rule-based spatio-temporal query pro-
cessing for video databases.The VLDB Journal13(2004)86–103
21.S?aykol,E.,Sinop,A.,G¨u d¨u kbay,U.,Ulusoy,¨O.,C?etin,E.:Content-based retrieval
of historical Ottoman documents stored as textual images.IEEE Trans.on Image Processing13(2004)314–325
A Grammar for Visual Surveillance Querying
Language(VSQL)
Visual Surveillance Query Language(VSQL)is designed to provide sup-port for integrated querying of indoor visual surveillance videos by spatial, semantic,and low-level features.
/*main query string*/
/*main query string components*/
|
/*single object query conditions*/
/*multi object query conditions*/
/*object condition*/
/*primitive types*/
第2章 数据库系统的数据模型
第2章数据库系统的数据模型 第二章数据库系统的数据模型 本章主要内容 数据库是个具有一定数据结构的数据集合,这个结构是根据现实世界中事物之间的联系来确定的。在数据库系统中不仅要存储和管理数据本身,还要保存和处理数据之间的联系,这种数据之间联系与就是实体之间的联系。研究如何表示和处理这种联系是数据库系统的一个核心问题,用以表示实体以及实体之间联系的数据库的数据结构称为数据模型。本章将着重介绍一下概念模型、层次模型、网状模型、关系模型、面向对象模型等数据库系统的数据模型的基本概念和设计方法,为后面的数据库设计打下基础。 2.1 数据模型概述 数据模型(Data Model)是对现实世界数据特征的抽象,是用来描述数据的一组概念和定义。 为了把现实世界的具体事物抽象、组织为某一DBMS现实世界支持的数据模型,通常首先把现实世界中的客观对象抽象 认识抽象为概念模型,然后把概念模型转换为某一DBMS支持的数 据模型,这一过程如图2,1所示。概念数据模型:信息世界 数据模型按不同的应用层次可划分为两类: 转换 (1)概念数据模型(又称概念模型) 是一种面向客观世界、面向用户的模型,独立于计算逻辑数据模型:DBMS支持的数据模型机系统的数据模型,完全不涉及信息在计算机中的表示,
只是用来描述某个特定组织所关心的信息结构。概念模型是按用户的观点对数据建模,是用户和数据设计人员之间进行交流的工具,主要是用于数据库设计。例如E,R模型、扩充E,R模型属于这一类模型。 (2)逻辑数据模型(又称数据模型) 是一种直接面向数据库系统的模型,主要用于DBMS的实现。例如层次模型、网状模型、关系模型均属于这一类模型。这类模型有严格的形式化定义,以便于在计算机系统中实现。 2.1.1 数据模型的基本组成 数据模型是现实世界中的事物及其间联系的一种抽象表示,是一种形式化描述数据、数据间联系以及有关语义约束规则的方法。通常一个数据库的数据模型由数据结构、数据操作和数据的约束条件三个部分组成。 (1)数据结构 是指对实体类型和实现间联系的表达实现。它是数据模型最基本的组织部分,规定了数据模型的静态特性。在数据库系统中通常按照数据结构的类型来命名数据模型,例如,采用层次型数据结构、网状型数据结构、关系型数据结构的数据模型分别称为层次模型、网状模型和关系模型。 (2)数据操作 是指对数据库进行的检索和更新(包括插入、删除和修改)两类操作。它规定了数据模型的动态操作。 (3)数据的约束条件 数据的约束条件是一组完整性规则的集合,它定义了给定数据模型中数据及其联系应具 1 有的制约和依赖规则。以确保数据库中数据的正确性、有效性和相容性。
数据库概论 习题参考答案
第1章绪论习题参考答案 1、试述数据、数据库、数据库管理系统、数据库系统的概念。(参见P3、4、5页) 参考答案: 描述事物的符号记录称为数据;数据库是长期储存在计算机内的、有组织的、可共享的数据集合;数据库管理系统是位于用户与操作系统之间的一层数据管理软件; 数据库系统是指在计算机系统中引入数据库后的系统,一般由数据库、数据库管理系统(及其开发工具)、应用系统、数据库管理员和用户构成。 2.使用数据库系统有什么好处?(参见P12页) 参考答案: 数据库系统使信息系统从以加工数据的程序为中心转向围绕共享的数据库为中心的阶段,这样既便于数据的集中管理,又有利于应用程序的研制和维护,提高了数据的利用率和相容性,提高了决策的可靠性。 3.试述文件系统与数据库系统的区别和联系。(8、9、10页) 参考答案: 1)数据结构化是数据库与文件系统的根本区别。 在文件系统中,相互独立的文件的记录内部是有结构的,管其记录内部已有了某些结构,但记录之间没有联系。数据库系统实现整体数据的结构化,是数据库的主要特征之一。 2)在文件系统中,数据的最小存取单位是记录,粒度不能细到数据项。而在数据库系统中,存取数据的方式也很灵活,可以存取数据库中的某一个数据项、一组数据项一个记录或或一组记录。 3)文件系统中的文件是为某一特定应用服务的,文件的逻辑结构对该应用程序来说是优化的,因此要想对现有的数据再增加一些新的应用会很困难,系统不容易扩充。而在数据库系统中数据不再针对某一应用,而是面向全组织,具有整体的结构化。5.试述数据库系统的特点。(9、10、11页) 参考答案: 数据结构化;数据的共享性高、冗余度低、易扩充;数据独立性高;数据由DBMS统一管理和控制。 6.数据库管理系统的主要功能有哪些? (4页)
SQLSERVER2008开启远程数据库访问图解
SQL SERVER2008开启远程数据库访问图解moon.翘楚 2012-02-19 8:52 转载自zhangcheng1 最终编辑zhangcheng1 tag:sql server2008远程数据库,远程连sql,IP远程连SQL SERVER,1433端口映射 这篇文章不错,我试验了确实可以,moon.翘楚在这里再进行一下图文增补,让大家更加明白。 讲解SQL Server 2008开启远程连接 作者:blue1000出处:IT专家网论坛2010-06-23 07:00 sql server 2008默认是不允许远程连接的,sa帐户默认禁用的,如果想要在本地用SSMS连接远程服务器上的SQL Server 2008,需要做两个部分的配置: sql server 2008默认是不允许远程连接的,sa帐户默认禁用的,如果想要在本地用SSMS连接远程服务器上的SQL Server 2008,需要做两个部分的配置: 1,SQL Server Management Studio Express(简写SSMS) 2,SQL Server 配置管理器/SQL Server Configuration Manager(简写SSCM) 有两点要注意: 1,2005的外围应用配置器在2008中换了地方 2,有些设置生效需要重启一下SQL Server step 1: 打开SSMS,用windows身份连接数据库,登录后,右键选择“属性” step 2: 左侧选择“安全性”,选中右侧的“SQL Server 和 Windows 身份验证模式”以启用混合登录模式 step 3: 选择“连接”,勾选“允许远程连接此服务器”,然后点“确定” step 4: 展开“安全性”->“登录名”->“sa”,右键选择“属性” step 5: 左侧选择“常规”,右侧选择“SQL Server 身份验证”,并设置密码 step 6: 选择“状态”,选中“启用”,点击“确定” step 7: 右击数据库选择“方面”
翻译 大型共享数据库的数据关系模型(精选.)
大型共享数据库的数据关系模型 E.F.Codd IBM Research Laboratory,SanJose,California 未来的数据库使用者一定是和数据在机器中的存储(即数据库的内部模式)相隔离的。而通过提示服务来提供信息是一个不太令人满意的解决方法。当数据的内部模式表示发生改变,甚至数据内部表示的多个方面发生改变时,终端用户和大多数应用程序的活动都不会受到影响。因此,查询、更新和报告存储信息类型的自然增长和变动都需要在数据表示中表现出来。 现存的不可推断的、格式化的数据系统给用户提供了树结构的文件或者更一般的网格模式的数据。本文在第一部分讨论这些模式的不足之处。并且会介绍一种基于n元组关系的模式,一种数据库关系的正式形式和通用数据子句的概念。第二部分将讨论一些关系的操作(不是逻辑层面的),并且把这些操作应用于用户模式上解决冗余和一致性问题。 1关系模式和一般模式 1.1简介 这篇文章是关于系统的基本关系原理的应用,这个原理提供了共享大型格式化数据库的方法。除了Childs[1]的文章有介绍外,用于数据库系统的关系的主要应用 还表现在演绎推理型的问-答系统中。Levein和Maron[2]提供了大量关于这个领域的参考资料。 相比之下,这里要解决的问题是一些数据独立性的问题——应用程序和终端活动之于数据类型增长和数据表示变动的独立性,而数据一致性问题即使在非演绎推 理型系统中也是很棘手的。 在目前流行的非推论性系统中,第一部分要介绍的数据的关系视图(或叫做模式)在一些方面似乎优于图模式和网格模式[3,4]。这种模式提供了一种根据数据的自然结构来描述描述数据的方式——也就是说,不用为了数据的机器表示而添加其 他的将结构。因此,这种模式为高水准的数据语言提供了基础,而这种数据语言机 制一方面可以达到最大化程序之间的独立性,另一方面也可以最大化数据的机器表 示和组织之间的独立性。 关系模式更高一级的优势在于它构成了关系处理可导性、冗余性和一致性的坚固基础——这些将在第二部分讨论。另一方面,网络模型产生了一些混淆,尤其是 把连接的源误作为关系的源(见第二部分“连接陷阱”) 最后,关系视图允许对目前格式化数据系统的范围和逻辑限制的更清晰的估算,并且有在单独的系统内竞争数据表示方式的优点(从逻辑的观点)。更清楚的这个观点的示例会在本文中的不同部分中被阐释。但是支持关系模式的系统实现不会讨论。 1.2目前系统的数据相关性 最近发展的信息系统中数据描述表的提供是向数据独立性目标[5,6,7]靠近的重要提高。这些表可以使改变数据库中数据表示的某些特征变得更容易些。但是,许 多数据表示特征可以在不逻辑地削弱一些应用程序的情况下被改变的功能仍受到相 当的限制。更进一步,与用户交互的数据模式仍然有一些散乱的代表性特征,特别
数据库系统原理课后答案 第九章
9.1 名词解释 (1)OODBS:是指面向对象数据库系统,它既具数据库管理的基本功能,又能支持面向对象的数据模型。 (2)ORDBS:基于对象关系数据模型的DBS称为对象关系数据库系统(ORDBS)。 (3)平面关系模型:传统的关系模型称为“平面关系模型”,它要求关系模式具有第一范式(1NF)性质,关系具有规范化的结构。也就是规定属性值是不可分解的,即不允许属性值具有复合结构(元组或关系)。 (4)嵌套关系模型:是从平面关系模型发展而成的。它允许关系的属性值又可以是一个关系,而且可以出现多次嵌套。嵌套关系突破了1NF的定义框架,是“非1NF关系”。 (5)复合对象模型:在嵌套关系模型上进一步放宽要求。在关系定义上,集合与元组不再有交替出现的严格限制,此时的关系中,属性类型可以是基本数据类型、结构类型(元组类型)或集体类型(即关系类型)。 (6)数据的泛化/细化:是对概念之间联系进行抽象的一种方法。当在较低层上的抽象表达了 与之联系的较高层上抽象的特殊情况时,就称较高层上抽象是较低层上抽象的"泛化",而较低层上抽象是较高层上抽象的"细化"。 (7)对象关系模型:在传统关系数据基础上,提供元组、数组、集合等更为丰富的数据类型及处理新数据类型操作的能力而形成的数据模型。(注:传统关系模型只支持字符、数值、字串,布尔值等等基本数据类型及其处理功能) (8)类型级继承性:当继承性发生在类型级时,子类型继承了超类型的属性。也就是说,超类型所具有的属性,在子类上也具有。 (9)表级继承性:继承性也可发生在表级,(就是元组集合上发生继承),子表继承超表全部属性,超表中每个元组最多可以与子表中一个元组对应,而子表中的每个元组在超表中恰有一个元组对应,并在继承的属性值上具有相同的值。 (10)引用类型:数据类型可以嵌套定义,在嵌套引用时,不是引用对象本身,而是个用对象标识符(即指针),这种指针被称为引用类型。 (11)对象:客观世界中的实体经过抽象称为问题空间中的对象,它是对一组信息及其操作的描述。 (12)类:是具有相同的变量名和类型、相同的消息和使用方法的对象的集合。 (13)单重继承性:一个子类继承某一个超类的结构和特性,称为单重继承性。 (14)多重继承性:一个子类继承多个超类的结构和特性,称为多重继承性。 (15)对象标识:在面向对象语言中,对象标识是一个指针一级的概念,在对象创建的瞬间,由系统赋给每个对象一个“标识”,即系统内的一个唯一的指针,在对象生存期内,这个标识不可改变。 (16)对象包含:不同类的对象之间存在的包含关系称为对象包含。包含是一种“一部分”(is part of)的联系。 (17)类继承层次图:表示类继承关系的图,由超类名、子类名和一组线条自上而下有序的表示。(18)类包含层次图:表示对象包含关系的图,由一些具有包含关系的对象和线条自上而下表示(下方的对象为其连线所指上方对象的一部分)。 (19)持久数据:是指创建这些数据的程序运行终止后数据依然存在于系统之中。数据库中的关系就是持久数据。 (20)持久对象:程序运行结束后,被保留下来的对象称为持久对象。 (21)持久指针:持久指针可看作是数据库中指向对象的指针。持久化指针不像内存中的指针,它在程序执行后及数据重组后仍保持有效。 (22)持久化C++系统: 基于C++的持久化扩充的OODBS。
用PLSQL远程连接Oracle数据库
PL/SQL Developer 远程连接Oracle数据库 1、配置服务器tnsnames.ora文件,如果本机上没有安装oracle,可以从安 装了oracle的机上拷贝一个(tnsnames.ora文件)放在指定目录下,因为我已安装oracle,所以直接配置该文件。 # tnsnames.ora Network Configuration File: D:\Oracle11g\product\11.2.0\dbhome_1\network\admin\tnsnames.ora # Generated by Oracle configuration tools. LISTENER_ORCL = (ADDRESS = (PROTOCOL = TCP)(HOST = localhost)(PORT = 1521)) ORACLR_CONNECTION_DATA = (DESCRIPTION = (ADDRESS_LIST = (ADDRESS = (PROTOCOL = IPC)(KEY = EXTPROC1521)) ) (CONNECT_DATA = (SID = CLRExtProc) (PRESENTATION = RO) ) ) ORCL = (DESCRIPTION = (ADDRESS = (PROTOCOL = TCP)(HOST = 192.168.25.224)(PORT = 1521)) (CONNECT_DATA = (SERVER = DEDICATED) (SERVICE_NAME = orcl) ) ) orcl为远程Oracle数据库的实例名,IP地址和端口也要配置正确。 2、配置完成后,Oracle客户端应该可以连接到远程Oracle 数据库上。
SQL2000数据库实现远程连接
SQL 2000数据库怎么实现远程连接 SQL Server 2000无论是个人版、企业版还是标准版,只要细版本在8.00.1760以下,均不提供远程数据库连接,即使服务器端工具和客户端工具的设置均有TCP/IP设置和数据库连接属性也设置了RPC远程连接,仍然不能实现远程连接。该怎么解决呢? 解决方案如下: 首先,需要打SP4补丁,该补丁包含了以前sp1、2、3的补丁,安装此补丁后一般要重新启动计算机,再进行远程连接就可以了,如再企业管理器可远程注册服务器、查询分析可连接远程服务器、ODBC可连接远程服务器。 要点: 1.确认服务器端SQL服务端口号是否为:1433 2.如果不是1433,配置客户端 3.建立服务器端登录帐号,确保角色及管理数据库 一、查看服务器端情况 1. 看ping 服务器IP能否ping通。 这个实际上是看和远程sql server 服务器的物理连接是否存在。如果不行,请检查网络,查看配置,当然得确保远程sql server 服务器的IP拼写正确。 2. 在Dos或命令行下输入telnet 服务器IP 端口,看能否连通。 如telnet 202.114.100.100 1433 通常端口值是1433,因为1433是sql server 的对于Tcp/IP的默认侦听端口。如果有问题,通常这一步会出问题。通常的提示是“……无法打开连接,连接失败"。 如果这一步有问题,应该检查以下选项。 1) 检查远程服务器是否启动了sql server 服务。如果没有,则启动。 2) 检查sql server服务器端有没启用Tcp/IP协议,因为远程连接(通过因特网)需要靠这个协议。检查方法是,在服务器上打开开始菜单->程序->Microsoft SQL Server->服务器网络实用工具,看启用的协议里是否有tcp/ip协议,如果没有,则启用它。 3) 检查服务器的tcp/ip端口是否配置为1433端口。仍然在服务器网络实用工具里查看启用协议里面的tcp/ip的属性,确保默认端口为1433,并且隐藏服务器复选框没有勾上。 事实上,如果默认端口被修改,也是可以的,但是在客户端做telnet测试时,写服务器端口号时必须与服务器配置的端口号保持一致。如果隐藏sql server服务器复选框被勾选,则意味着客户端无法通过枚举服务器来看到这台服务器,起到了保护的作用,但不影响连接,但是Tcp/ip协议的默认端口将被隐式修改为2433,在客户端连接时必须作相应的改变(具体方式见二:设置客户端网络实用工具)。
Labview中远程数据库访问技术
摘要:介绍了在Labview中应用ADO技术访问本地和远程数据库的方案。对在局域网中以网络 共享方式访问远程Access数据库进行了详细介绍,最后给出了应用该方法开发的一个实例。关 键词:LabviewADO远程数据库引言Labview是美国NI公司开发的一种图形化编程语言,其主 要用于数据采集、仪器控制、过程监控等领域。Labview具有丰富的采集与分析函数库,在Labview环境下可以方便快捷地开发监控系统软件。现代监控系统一般构建于局域网内,且 摘要:介绍了在Labview中应用ADO技术访问本地和远程数据库的方案。对在局域网中以网络共享方式访问远程Access数据库进行了详细介绍,最后给出了应用 该方法开发的一个实例。 关键词:Labview ADO 远程数据库 引言 Labview 是美国NI公司开发的一种图形化编程语言,其主要用于数据采集、仪器控制、过程监控等领域。Labview具有丰富的采集与分析函数库,在Labview 环境下可以方便快捷地开发监控系统软件。现代监控系统一般构建于局域网内,且监控系统自身也是企业生产管理系统的一个子系统,因此不可避免地要访问本地或远程数据库。Labview开发版中并没有提供直接与通用数据库接口的工具,但可以 通过以下方法解决:购买NI公司开发的附加软件工具包;利用其它语言如VC++编写DLL访问数据库;利用ADO控件访问数据库。[1]Labview支持Activex控件,与其它方法相比基于ADO技术构建本地或远程数据库访问接口不失为一种简单而又经济 的方法。 1 ADO技术简介 ADO(Activex Data Objects)是Microsot为OLE DB设计的应用层接口,它为一致的数据访问接口提供了良好的扩展性而不局限于单一的数据源。ADO接口编程模型中包括连接(connection)、命令(command)、参数(parameter)、 记录集(recordset)、字段(field)、错误(error)、属性(property)、集合(collection)、事件(event)等元素。其中connection、command、recordset是三个主体对象,parameters、fields、errors、properties、是四个集合对象。ADO的一般操作方式为:1 连接到数据源;2 指定访问数据源的命令;3 执行命令;4 将命令执行后产生的结果保存在缓存中;5 更新数据源。ADO接口继承了OLE DB的高速性,可以高速访 问本地或远程的数据源。 2在Labview 中使用ADO接口 在Labview 中使用ADO接口访问数据库一般包括以下几个步骤:建立ADO控件;连接到数据源;生成SQL命令;执行命令;关闭连接。具体步骤如图1 所示。 2.1建立ADO控件 在前面板控件模板中选择Activex子模板单击Automation Refnum项,右键单击Select Activex Class的Browse从类型库中选择 Microsoft Activex Data Objects 2.7 library Version 2.7,之后可以向框图程序中加 入connection、command、recordset等对象。 2.2连接到数据源 首先在Diagram中单击鼠标右键,从Function模板选择communication 子模板,从中选择Activex项的Automation Open 与ADODB_Connection相连即 可打开连接。接着同样从communication子模板的Activex项中选择 Invoke node (方法节点)并与Automation Open 相连,在其上单击右键选择methods项的Open方法即出现图1中所示的节点。其中“Open”表示该节点为打开“连接对象”,“ConnectionString”是连接到数据源的字符串,“UserID”和“Password”
数据库的体系结构
数据库基础 ( 视频讲解:25分钟) 本章主要介绍数据库的相关概念,包括数据库系统的简介、数据库的体系结构、数据模型、常见关系数据库。通过本章的学习,读者应该掌握数据库系统、数据模型、数据库三级模式结构以及数据库规范化等概念,掌握常见的关系数据库。 通过阅读本章,您可以: 了解数据库技术的发展 掌握数据库系统的组成 掌握数据库的体系结构 熟悉数据模型 掌握常见的关系数据库 1 第 章
1.1 数据库系统简介 视频讲解:光盘\TM\lx\1\数据库系统简介.exe 数据库系统(DataBase System,DBS)是由数据库及其管理软件组成的系统,人们常把与数据库有关的硬件和软件系统称为数据库系统。 1.1.1 数据库技术的发展 数据库技术是应数据管理任务的需求而产生的,随着计算机技术的发展,对数据管理技术也不断地提出更高的要求,其先后经历了人工管理、文件系统、数据库系统等3个阶段,这3个阶段的特点分别如下所述。 (1)人工管理阶段 20世纪50年代中期以前,计算机主要用于科学计算。当时硬件和软件设备都很落后,数据基本依赖于人工管理,人工管理数据具有如下特点: ?数据不保存。 ?使用应用程序管理数据。 ?数据不共享。 ?数据不具有独立性。 (2)文件系统阶段 20世纪50年代后期到60年代中期,硬件和软件技术都有了进一步发展,出现了磁盘等存储设备和专门的数据管理软件即文件系统,文件系统具有如下特点: ?数据可以长期保存。 ?由文件系统管理数据。 ?共享性差,数据冗余大。 ?数据独立性差。 (3)数据库系统阶段 20世纪60年代后期以来,计算机应用于管理系统,而且规模越来越大,应用越来越广泛,数据量急剧增长,对共享功能的要求越来越强烈。这样使用文件系统管理数据已经不能满足要求,于是为了解决一系列问题,出现了数据库系统来统一管理数据。数据库系统满足了多用户、多应用共享数据的需求,它比文件系统具有明显的优点,标志着管理技术的飞跃。 1.1.2 数据库系统的组成 数据库系统是采用数据库技术的计算机系统,是由数据库(数据)、数据库管理系统(软件)、数
数据库系统 包括题目和答案
数据库系统原理复习题 第1章 一、选择题 1.数据库(DB)、数据库系统(DBS)和数据库管理系统(DBMS)之间的关系是(A )。 A. DBS包括DB和DBMS B. DBMS包括DB和DBS C. DB包括DBS和DBMS D. DBS就是DB,也就是DBMS 2.概念模型是现实世界的第一层抽象,这一类模型中最著名的模型是(D )。 A.层次模型 B. 关系模型 C. 网状模型 D. 实体-联系模型 3.目前,数据库管理系统最常用的逻辑数据模型是(C)。 A.网状模型B.层次模型 C.关系模型D.面向对象模型 4.下列四项中,不属于数据库系统特点的是(C)。 A.数据共享 B. 数据完整性 C. 数据冗余度高 D. 数据独立性高 5.数据模型的三个要素分别是(B )。 A.实体完整性、参照完整性、用户自定义完整性 B.数据结构、数据操作、数据完整性约束条件 C.插入数据、修改数据、删除数据 D.外模式、模式、内模式 6.数据库三级结构从内到外的3个层次依次为(B)。 A.外模式、模式、内模式 B. 内模式、模式、外模式 C. 模式、外模式、内模式 D. 内模式、外模式、模式 7.下列关于数据库系统的正确叙述是(A): A.数据库系统减少了数据冗余 B.数据库系统避免了一切冗余 C.数据库系统中数据的一致性是指数据类型的一致 D.数据库系统比文件系统能管理更多的数据 8.数据的逻辑独立性是指(B)。 A.外模式改变时保持应用程序不变B.模式改变时保持外模式不变 C.内模式改变时保持模式不变D.数据改变时保持应用程序不变
9.数据的物理独立性是指(C)。 A.外模式改变时保持应用程序不变B.模式改变时保持外模式不变 C.内模式改变时保持模式不变D.数据改变时保持应用程序不变 10.公司有多个部门和多名职员,每个职员只能属于一个部门,一个部门可以有多名职 员,从部门到职员的联系类型是(D)。 A.多对多 B. 一对一 C. 多对一 D. 一对多 11.储蓄所有多个储户,储户在多个储蓄所之间存款,储户与储蓄所之间是(C)。 A.一对一联系 B. 一对多联系 C. 多对多联系 D. 不确定联系 12.描述数据库全体数据的全局逻辑结构和特性的是(A)。 A.模式 B. 内模式 C. 外模式 D. 以上三级模式 二、填空 1. 数据库系统一般由(数据库)、(数据库管理系统)、(应用程序)和(数据库管理员) 组成。 2. 数据库是长期存储在计算机中、有(组织)的、可(共享)的数据集合。 3. DBMS表示(DataBase Management System),它是位于(用户)和(操作系统)之 间的一层数据管理软件。 4. 实体之间的联系可抽象为三类,它们是(一对一)、(一对多)和(多对多)。 5. 数据模型的三要素包括(数据结构)、(数据操作)和(数据完整性约束条件)三部 分。 6. 根据数据模型的应用目的不同,数据模型分为(概念模型)、(逻辑模型)和(物理 模型)等。 7. 按照数据结构的类型命名,逻辑模型分为(关系模型)、(层次模型)和(网状模型) 等。 8. E-R图中,(矩形)表示实体,(椭圆)表示属性,(菱形)表示实体之间的联系。 三、简述题 1. 数据库是长期存贮在计算机内的、有组织的、可共享的大量数据的集合。 2. 数据库管理系统的主要功能包括: (1)数据定义功能, (2)数据的组织、存储和管理,
远程访问数据库
远程访问数据库 对于oracle数据库,可以通过建立dblink的方式,在一个数据库中访问获取另一个数据库的数据,具体实现方式如下: 1.建立DATABASE LINK: 在本机数据库中执行如下语句: Create public database link ERPDATABASE–远程数据库连接名 Connectto apps identifiedby apps Using '(DESCRIPTION = (ADDRESS_LIST= (ADDRESS = (PROTOCOL = TCP) (HOST = 192.168.0.100) (PORT = 1521) ) ) (CONNECT_DATA = (SERVICE_NAME = ERPTEST) ) ) ' ; 蓝色部分可以从数据库安装目录\product\10.2.0\db_1\network\ADMIN的路径下找到,拷贝tnsnames.ora文件中的对应信息。 以上连接信息可以用oracle客户端的Net Manager工具生成:
2.查看数据 成功之后,可以在本机数据库中查看ERPTEST数据库的数据: 其中需要注意的有: 1)ygfm为数据库用户名,因为dblink的连接信息中使用的apps用户是数据库dba,所以可以方位ygfm的数据,这里要注意的是apps需要有ygfm的访问权限。 2)注意需要使用’ @ERPDATABASE’标识远程数据库。 3.建立同义词 可以建立同义词减少输入: 执行以下语句: Create synonym ygfm_analyse_base for ygfm.ygfm_analyse_base@ERPDATABASE; 查询: select * from ygfm_analyse_base; 需要注意的是:这里建立的DATABASE LINK 不同于tnsnames.ora文件中的连接信息,这里是在数据库中保存连接信息,而tnsnames.ora文件中的连接信息是在数据库层面之上的。
第二章--关系数据库习题
第二章关系数据库 一、选择题: 1、对于关系模型叙述错误的是。 A.建立在严格的数学理论、集合论和谓词演算公式基础之一 B.微机DBMS绝大部分采取关系数据模型 C.用二维表表示关系模型是其一大特点 D.不具有连接操作的DBMS也可以是关系数据库管理系统 2、关系模式的任何属性。 A.不可再分B.可再分 C.命名在该关系模式中可以不唯一D.以上都不是 3、在通常情况下,下面的表达中不可以作为关系数据库的关系的是。 A.R1(学号,姓名,性别) B.R2(学号,姓名,班级号) C.R3(学号,姓名,宿舍号) D.R4(学号,姓名,简历) 4、关系数据库中的码是指。 A.能唯一关系的字段B.不能改动的专用保留字 C.关键的很重要的字段D.能惟一表示元组的属性或属性集合 5、根据关系模式的完整性规则,一个关系中的“主码”。 A.不能有两个B.不能成为另外一个关系的外码 C.不允许为空D.可以取值 6、关系数据库中能唯一识别元组的那个属性称为。 A.唯一性的属性B.不能改动的保留字段 C.关系元组的唯一性D.关键字段 7、在关系R(R#,RN,S#)和S(S#,SN,SD)中,R的主码是R#,S的主码是S#,则S#在R中称为。A.外码B.候选码 C.主码D.超码 8、关系模型中,一个码是。 A.可由多个任意属性组成 B.至多由一个属性组成 C.可由一个或多个其值能唯一标识该关系模式中任意元组的属性组成 D.以上都不是 9、一个关系数据库文件中的各条记录。 A.前后顺序不能任意颠倒,一定要按照输入的顺序排列 B.前后顺序可以任意颠倒,不影响库中的数据关系 C.前后顺序可以任意颠倒,但排列顺序不同,统计处理的结果可能不同 D.前后顺序不能任意颠倒,一定要按照码段的顺序排列 10、关系数据库管理系统应能实现的专门关系运算包括。 A.排序、索引、统计B.选择、投影、连接 C.关联、更新、排序D.显示、打印、制表 11、同一个关系模型的任意两个元组值。 A.不能全同B.可全同 C.必须全同D.以上都不是 12、自然连接是构成新关系的有效方法。一般情况下,当对关系R和S使用自然连接时,要求R和S含有一个或多个共有的。 A.元组B.行 C.记录D.属性 13、设关系R(A,B,C)和S(B,C,D),下列各关系代数表达式不成立的是。 A. ) ( ) (S R D A π π>< B.R S ? C. ) ( ) (S R B B π π? D.R>试述数据模型的概念
试述数据模型的概念,数据模型的作用和数据模型的三个要素: 答案: 模型是对现实世界的抽象。在数据库技术中,表示实体类型及实体类型间联系的模型称为“数据模型”。 数据模型是数据库管理的教学形式框架,是用来描述一组数据的概念和定义,包括三个方面: 1、概念数据模型(Conceptual Data Model):这是面向数据库用户的实现世界的数据模型,主要用来描述世界的概念化结构,它使数据库的设计人员在设计的初始阶段,摆脱计算机系统及DBMS的具体技术问题,集中精力分析数据以及数据之间的联系等,与具体的DBMS 无关。概念数据模型必须换成逻辑数据模型,才能在DBMS中实现。 2、逻辑数据模型(Logixal Data Model):这是用户从数据库所看到的数据模型,是具体的DBMS所支持的数据模型,如网状数据模型、层次数据模型等等。此模型既要面向拥护,又要面向系统。 3、物理数据模型(Physical Data Model):这是描述数据在储存介质上的组织结构的数据模型,它不但与具体的DBMS有关,而且还与操作系统和硬件有关。每一种逻辑数据模型在实现时都有起对应的物理数据模型。DBMS为了保证其独立性与可移植性,大部分物理数据模型的实现工作又系统自动完成,而设计者只设计索引、聚集等特殊结构。 数据模型的三要素: 一般而言,数据模型是严格定义的一组概念的集合,这些概念精确地描述了系统的静态特征(数据结构)、动态特征(数据操作)和完整性约束条件,这就是数据模型的三要素。 1。数据结构 数据结构是所研究的对象类型的集合。这些对象是数据库的组成成分,数据结构指对象和对象间联系的表达和实现,是对系统静态特征的描述,包括两个方面: (1)数据本身:类型、内容、性质。例如关系模型中的域、属性、关系等。 (2)数据之间的联系:数据之间是如何相互关联的,例如关系模型中的主码、外码联系等。 2 。数据操作 对数据库中对象的实例允许执行的操作集合,主要指检索和更新(插入、删除、修改)两类操作。数据模型必须定义这些操作的确切含义、操作符号、操作规则(如优先级)以及实现操作的语言。数据操作是对系统动态特性的描述。 3 。数据完整性约束 数据完整性约束是一组完整性规则的集合,规定数据库状态及状态变化所应满足的条件,以保证数据的正确性、有效性和相容性。
数据模型与数据库系统结构
数据模型与数据库系统结构 1.数据 为了了解世界,研究世界和交流信息,我们需要描述各种事物,用自然语言来描述虽然很直接,但是过于烦琐,不便于形式化,更不利于计算机去表达,为此,我们常常只抽取那些感兴趣的事物特征或属性来描述它。 例如:XX今天下课回到寝室,跟室友说,啊,兄弟们,我单身了!!~~~~准备请大家吃顿饭庆祝一下~~~~ 大家好奇的问 他叫小雪,21岁,是医护系的,护理专业和我是老乡,遵义人。 我们可以从胡锋的描述中获取到以下一条记录,小雪今年21岁遵义人是医护系护理专业的学生,那这种描述事物的符号记录我们称为数据。 数据有一定的格式,例如姓名在中国而言一般是4个汉字的字符(某些少数民族),性别呢是一个汉字字符,等等,那这些我们称为数据的语法,而数据的含义是数据的语义。我们通过解释、推论,归纳,分析和综合等等方法,从数据中获得有意义的内容称为信息。因此,数据是信息存在的一种形式,只有通过解释或处理才能成为有用的信息。 一般来说,数据库中的数据具有以下两个特征 1)数据的静态特征 包括数据的基本结构,数据间的联系和对数据取值范围的约束 学生管理的例子
在学生基本信息中包括:学号,姓名,性别,出生日期,专业,家庭地址。 这些都是学生所具有的基本特征,是学生数据的基本结构。 学生选课信息中包括:学号,课程号,考试成绩等信息,其中选课信息和学生基本信息中的学号是有一定关联的,即选课信息中的学号所能选取的值必须在学生基本信息中的学号取值范围之内,只有这样,学生选课信息中所描述的学生选课情况才是有意义的。 说白一点,也就是这个学生要存在,他才会有选课信息。这个就是数据之间的联系。 最后,我们再来看看什么是数据取值范围的约束 例如,人的性别一项取值只能是男或女,课程的学分一般是大于0的整数值,而我们的考试成绩一般在0~100分范围内等,这些都是对某个列的数据取值范围进行的限制,目的是在数据库中存储正确的,有意义的数据,这就是对数据取值范围的约束 2)数据的动态特征 数据的动态特征是指对数据可以进行的操作以及操作规则。 对数据库数据的操作主要是有查询数据和更改数据,更改数据一般又包括对数据的插入,删除和修改 通常我们将数据的静态特征和动态特征的描述称为数据模型三要素。即描述数据时要包括数据的基本结构,数据的约束条件和定义在数据
数据库数据模型的发展及方向
[XXXX大学XXX学院XXX班] 数据库数据模型的 发展及方向 [ ] [学号: ] [摘要:近年来,随着计算机辅助设计(CAD)、计算机辅助制造(CAM)、计算机辅助软件工程 (CASE)、全球信息系统(GIS)、图像处理、超文本应用等领域的飞速发展及其在传统领域中应用的深化,要求数据库管理系统(database management system,DBMS)能够有效地管理复杂对象。比如在工程应用领域,一个客观复杂实体往往由数十个,甚至成百上千个简单实体组成,为了减小数据库应用系统的设计复杂度、提高其执行效率,要求DBMS不但能根据实体丰富的语义进行建模、提供有效的存储与操纵手段,以及模拟复杂实体的复杂行为,而且在逻辑上还要将一个复杂实体的表示和操纵作为一个整体看待,在操纵数据的同时考虑实体间的复合语义,即各简单实体的存在方式(独立或依赖)以及实体间的引用方式(共享或排他)。然而,传统RDBMS由于采用满足第一范式(first normal form,1NF)的平关系模型,在面对各种新的应用领域时存在以下不足。]
关键词:数据库,数据模型,扩展关系数据库,语义数据模型,面向对象的数据模型,XML数据模型 正文: 数据模型概述 数据(data)是描述事物的符号记录。模型(Model)是现实世界的抽象。数据模型(Data Model)是数据特征的抽象,是数据库管理的教学形式框架。数据库系统中用以提供信息表示和操作手段的形式构架。数据模型包括数据库数据的结构部分、数据库数据的操作部分和数据库数据的约束条件。 数据模型所描述的内容包括三个部分:数据结构、数据操作、数据约束。 1. 概念数据模型(Conceptual Model):这是面向数据库用户的实现世界的数据模型,主要用来描述世界的概念化结构,它使数据库的设计人员在设计的初始阶段,摆脱计算机系统及DBMS的具体技术问题,集中精力分析数据以及数据之间的联系等,与具体的DBMS无关。概念数据模型必须换成逻辑数据模型,才能在DBMS中实现。 2. 逻辑数据模型(Logical Data Model):这是用户从数据库看到的数据模型,是具体的DBMS所支持的数据模型,如网状数据模型、层次数据模型等等。此模型既要面向用户,又要面向系统。 3. 物理数据模型(Physical Data Model):这是描述数据在存储介质上的组织结构的数据模型它不但与具体的DBMS有关,而且还和操作系统以及硬件有关。每一种逻辑数据模型在实现时都有其对应的物理数据模型。DBMS为了保证其独立性与可移植性,大部分物理数据模型的实现工作由系统自动完成,而设计者只设计索引、聚集等特殊结构。 数据模型的三要素: 一般而言,数据模型是一组严格定义的概念的集合。这些概念精确地描述了系统的静态特征(数据结构)、动态特征(数据操作)和完整性约束条件,这就是数据模型的三要素。 1. 数据结构 数据结构是所研究的对象类型的集合。这些对象是数据库的组成部分,数据结构指对象和对象间联系的表达和实现,是系统静态特征的描述,包括两个方面:(1)数据本身:类型、内容、性质。例如关系模型中的域、属性、关系等。 (2)数据之间的联系:数据之间是如何相互联系的,例如关系模型中的主码、外码等联系。 2. 数据操作 对数据库中对象的实例允许执行的操作集合,主要指检索和更新(插入、删除、修改)两类操作。数据模型必须定义这些操作的确切含义、操作符号、操作规则
如何实现远程连接数据库服务器
如何实现远程连接数据库服务器 (SQL Server 2005 + Visual Studio 2005) (1)配置服务器端 打开“SQL Server 外围应用配置器”--》选择“服务和连接的外围应用配置器”–》选择“服务器端(SQLEXPRESS)”--》点击“远程连接”,选择“本地连接和远程连接”,选择“同时使用TCP/IP和Named pipes”; 启动“SQL Server Browser”,选择自动;
(2)进行服务器端网络配置 打开“SQL Server Configuration Manager”--》点击“SQL Server 2005网络
配置”–》点击“SQLEXPRESS的协议”--》确保“Named Pipes”和“TCP/IP”都启用–》右键点击“TCP/IP ”选择属性,选择“IP地址”,设置“TCP动态端口”为空,设置“TCP端口”为1433;
(3)进行客户端网络配置 打开“SQL Server Configuration Manager”--》点击“SQL Native Client配置” –》点击“客户端协议”--》确保“Named Pipes”和“TCP/IP”都启用–》右键点击“TCP/IP ”选择属性,选择“协议”,确认“默认端口”为1433;
(4)设置SQL Server服务器实例 右键点击服务器实例–》选择“属性”–》选择“连接”–》在“允许远程连接到此服务器”前打勾;
(5)防火墙设置 对系统中使用的防火墙(包括ESET和windows自带的防火墙),选择关闭,或者设置例外(允许某个程序或者某个端口) (6)检查客户端和服务器端是否连通,端口是否打开 telnet ip 1433 //ip即为服务器的IP地址,1433是sql server的默认端口,这
数据库系统复习题
复习题 第1章数据库系统基本概念 一、单项选择题 1.数据库系统是采用了数据库技术的计算机系统,数据库系统由数据库、数据库管理系统、应用系统和() A.系统分析员 B.程序员 C.数据库管理员 D.操作员 2.数据库(DB),数据库系统(DBS)和数据库管理系统(DBMS)之间的关系是()。 A.DBS包括DB和DBMS B.DBMS包括DB和DBS C.DB包括DBS和DBMS D.DBS就是DB,也就是DBMS 3.下面列出的数据库管理技术发展的三个阶段中,没有专门的软件对数据进行管理的是()。 I.人工管理阶段 II.文件系统阶段 III.数据库阶段 A.I 和 II B.只有 II C.II 和 III D.只有 I 4.下列四项中,不属于数据库系统特点的是()。 A.数据共享 B.数据完整性 C.数据冗余度高 D.数据独立性高 5.数据库系统的数据独立性体现在()。 A.不会因为数据的变化而影响到应用程序 B.不会因为数据存储结构与数据逻辑结构的变化而影响应用程序 C.不会因为存储策略的变化而影响存储结构 D.不会因为某些存储结构的变化而影响其他的存储结构 6. .描述数据库全体数据的全局逻辑结构和特性的是()。 A.模式 B.内模式 C.外模式 7.要保证数据库的数据独立性,需要修改的是()。 A.模式与外模式 B.模式与内模式 C.三级模式之间的两层映射 D.三层模式 8.要保证数据库的逻辑数据独立性,需要修改的是()。 A.模式与外模式之间的映射 B.模式与内模式之间的映射 C.模式 D.三级模式 9.用户或应用程序看到的那部分局部逻辑结构和特征的描述是()模式。 A.模式 B.物理模式 C.子模式 D.内模式 10.下述()不是DBA数据库管理员的职责。 A.完整性约束说明 B.定义数据库模式 C.数据库安全 D.数据库管理系统设计 11.概念模型是现实世界的第一层抽象,这一类模型中最著名的模型是()。 A.层次模型 B.关系模型 C.网状模型 D.实体-关系模型 12.区分不同实体的依据是()。 A.名称 B.属性 C.对象 D.概念 13.关系数据模型是目前最重要的一种数据模型,它的三个要素分别是()。 A.实体完整性、参照完整性、用户自定义完整性 B.数据结构、关系操作、完整性约束 C.数据增加、数据修改、数据查询