大数据与数据分析英文
大数据与数据分析的关系和区别

大数据与数据分析的关系和区别随着信息技术的快速发展,大数据和数据分析成为了当今互联网时代的热门话题。
大数据是指由数据量庞大、复杂多样的数据集合,而数据分析则是指对这些数据进行收集、处理和解释的过程。
本文将就大数据与数据分析的关系和区别进行探讨。
一、大数据的定义与特点大数据是指由庞大的、高速的和多样化的数据集组成的数据集合。
其所面临的挑战主要体现在三个方面:数据量大、数据处理速度快和数据种类多。
与传统数据相比,大数据具有以下特点:1. 数据量大:大数据的数据量通常以TB(1TB=1024GB)或PB(1PB=1024TB)为单位,数据量巨大,甚至可以达到万亿级别。
2. 数据来源多样化:大数据来自于各种各样的来源,包括社交媒体、物联网设备、传感器等,数据类型丰富多样。
3. 数据处理速度快:大数据需要在短时间内处理大量数据,因此对数据处理速度的要求较高。
4. 数据质量不确定:由于大数据的来源多样化,数据质量难以保证,可能存在噪声、重复和错误等问题。
二、数据分析的定义与过程数据分析是指通过收集、整理和解释数据,以获取有价值的信息、洞察和预测。
数据分析的过程通常包括以下几个步骤:1. 数据收集:收集需要分析的数据,包括内部数据和外部数据,如销售数据、用户行为数据等。
2. 数据清洗:对收集到的数据进行预处理,包括去重、去噪声、填补空缺等操作,以提高数据的质量。
3. 数据建模:根据具体的分析任务,选择合适的算法或模型,对数据进行建模和训练,以获取有用的模型。
4. 数据分析:通过运用统计学、机器学习、数据挖掘等方法,对数据进行分析和挖掘,以挖掘隐藏在数据中的有价值信息。
5. 结果解释:对分析结果进行解释和呈现,以便决策者能够理解和运用这些结果。
三、大数据与数据分析的关系大数据和数据分析之间存在紧密的关系,可以说大数据是数据分析的基础。
大数据提供了丰富的、多样化的数据资源,而数据分析则能够通过对这些数据进行分析和挖掘,揭示数据背后的规律和洞察,进而为决策提供支持。
基于大数据的用户行为分析与预测研究(英文中文双语版优质文档)

基于大数据的用户行为分析与预测研究(英文中文双语版优质文档)I. IntroductionWith the development of Internet technology, people are increasingly inseparable from the Internet, and more and more information is recorded, which is called big data. The mining and analysis of big data is crucial to the decision-making and development of enterprises, and user behavior data is a very important part of it. User behavior data refers to various data generated by users when using products or services, including user access records, click records, purchase records, etc. These data reflect users' needs, interests, and behavioral habits for products or services. Said that these data can be used for user behavior analysis and prediction.2. User Behavior AnalysisUser behavior analysis is to obtain the rules and trends of user behavior through statistics, analysis and mining of user behavior data, so as to provide a basis for enterprise decision-making. User behavior analysis mainly includes the following aspects:1. User interest analysisUser interest analysis refers to the analysis of user behavior data to understand the user's points of interest, so as to recommend personalized products or services for enterprises. Specifically, by analyzing the user's search records, purchase records, browsing records, etc., the user's points of interest and preferences can be obtained, and products or services that better meet the user's needs can be recommended for enterprises.2. User behavior path analysisUser behavior path analysis refers to the analysis of user behavior data to understand the behavior path of users when using products or services, so as to provide better products or services for enterprises. Specifically, by analyzing the user's click records, browsing records, and purchase records in products or services, we can understand the user's behavior path and provide a basis for companies to improve products or services.3. User churn analysisUser churn analysis refers to the analysis of user behavior data to understand the reasons for user churn when using products or services, so as to provide enterprises with directions for improvement. Specifically, through the analysis of users' usage records, access records, evaluation records, etc. in products or services, we can understand the reasons for user loss and provide companies with improvement plans.3. User Behavior PredictionUser behavior prediction refers to the analysis and mining of user behavior data to obtain the future behavior trend of users, so as to provide decision-making basis for enterprises. User behavior prediction mainly includes the following aspects:1. User purchase predictionUser purchase prediction refers to the analysis and mining of behavior data such as user purchase records and browsing records to obtain the user's future purchase trends, so as to formulate better marketing strategies for enterprises. Specifically, by analyzing users' browsing records, click records, purchase records, etc. in products or services, we can understand users' purchasing preferences, purchasing power, purchasing cycle, etc., and provide personalized recommendations and marketing solutions for enterprises.2. User Churn PredictionUser churn prediction refers to the analysis and mining of user behavior data to obtain the trend and reasons for possible user loss in the future, so as to provide preventive measures for enterprises. Specifically, by analyzing the user's usage records, access records, evaluation records, etc. in products or services, we can understand the user's usage habits, satisfaction, etc., and provide personalized services and improvement plans for enterprises.3. User Conversion PredictionUser conversion prediction refers to the analysis and mining of user behavior data to obtain possible conversion trends of users in the future, so as to provide better conversion strategies for enterprises. Specifically, by analyzing users' usage records, browsing records, and click records in products or services, we can understand users' points of interest, conversion intentions, etc., and provide personalized conversion recommendations and services for enterprises.4. Application of Big Data Technology in User Behavior Analysis and PredictionBig data technology is the key to user behavior analysis and prediction. Big data technology can obtain the rules and trends of user behavior through the rapid processing and analysis of massive data, and improve the accuracy and efficiency of user behavior analysis and prediction. Big data technology mainly includes the following aspects:1. Data collectionData acquisition is the first step in big data analysis. Data collection needs to collect various behavioral data generated by users when using products or services, including user access records, click records, purchase records, etc. Data collection can be achieved through various channels, including websites, apps, social media, etc.2. Data storageData storage is an important part of big data analysis. Big data analysis needs to process massive amounts of data, so it needs to store massive amounts of data. Data storage can be implemented in various ways, including relational databases, NoSQL databases, distributed file systems, etc.3. Data cleaningData cleaning is a necessary step in big data analysis. Data cleaning requires deduplication, screening, conversion and other processing of the collected data to ensure the quality and accuracy of the data. Data cleaning can be achieved using various techniques, including ETL tools, data mining tools, etc.4. Data analysisData analysis is the core of big data technology. Data analysis requires statistics, analysis, mining and other processing of the collected data, so as to obtain the rules and trends of user behavior. Data analysis can be achieved using various techniques, including data mining, machine learning, deep learning, etc.5. Visual AnalysisVisual analysis is an important means of big data analysis. Visual analysis can present the analysis results in the form of charts, graphs, etc., and intuitively display the results of data analysis. Visual analysis can be implemented using various tools, including Tableau, Power BI, etc.6. Model buildingModel building is an important part of big data analysis. Model building needs to establish a model based on the collected data and analysis results, verify and optimize it, so as to obtain the prediction results of user behavior. Model building can be achieved using various techniques, including regression analysis, decision trees, neural networks, etc.In short, big data technology has played an important role in user behavior analysis and prediction, providing enterprises with more accurate and finer data analysis and prediction capabilities, thereby helping enterprises better understand user needs, optimize services, improve user experience and Market Competitiveness.一、引言随着互联网技术的发展,人们越来越离不开网络,越来越多的信息被记录下来,这些数据被称为大数据。
《大数据分析与挖掘》课程教学大纲

《大数据分析与挖掘》课程教学大纲一、课程基本信息课程代码:16054103课程名称:大数据分析与挖掘英文名称:Big data analysis and mining课程类别:专业选修课学时:48(理论课:32, 实验课:16)学 分:3适用对象: 软件工程专业、计算机科学与技术考核方式:考查先修课程:多媒体技术、程序设计、软件工程二、课程简介本课程从大数据挖掘分析技术实战的角度,结合理论和实践,全方位地介绍基于Python语言的大数据挖掘算法的原理与使用。
本课程涉及的主题包括基础篇和实战篇两部分, 其中基础篇包括:数据挖掘基础,Python数据分析简介,数据探索,数据预处理和挖掘建模;实战篇包括:电力窃漏电用户自动识别,航空公司客户价值分析,中医证型关联规则挖掘,基于水色图像的水质评价,家用电器用户行为分析与事件识别,应用系统负载分析与磁盘容量预测和电子商务网站用户行为分析及服务推荐。
本课程不是一个泛泛的理论性、概念性的介绍课程,而是针对问题讨论基于Python语言机器学习模型解决方案的深入课程。
教师对于上述领域有深入的理论研究与实践经验,在课程中将会针对这些问题与学员一起进行研究,在关键点上还会搭建实验环境进行实践研究,以加深对于这些解决方案的理解。
通过本课程学习,目的是让学生能够扎实地掌握大数据分析挖掘的理论与应用。
This course introduces the principle and application of big data mining algorithm based on Python language comprehensively from the perspective of big data mining analysis technology practice, combining theory and practice. This course covers two parts, the basic part and the practical part. The basic part includes: basic data mining, introduction to Python data analysis, data exploration, data preprocessing and mining modeling. Practical article included: electric power leakage automatic identification of the user, airlines customer value analysis, TCM syndrome association rule mining, based on water quality evaluation of color image, household electrical appliancesuser behavior analysis and event identification, load analysis and application system disk capacity prediction and e-commerce website user behavior analysis and recommendation service.This course is not a general theoretical, conceptual introduction, but rather an in-depth discussion of problem solving based on the Python language machine learning model. Teachers have in-depth theoretical research and practical experience in the above areas. In the course, they will study these problems together with students, and build experimental environment for practical research on key points to deepen their understanding of these solutions. Through the study of this course, students are expected to master the application of big data analysis and mining.三、课程性质与教学目的本课程是软件工程和计算机科学与技术专业的选修课。
人工智能与大数据分析
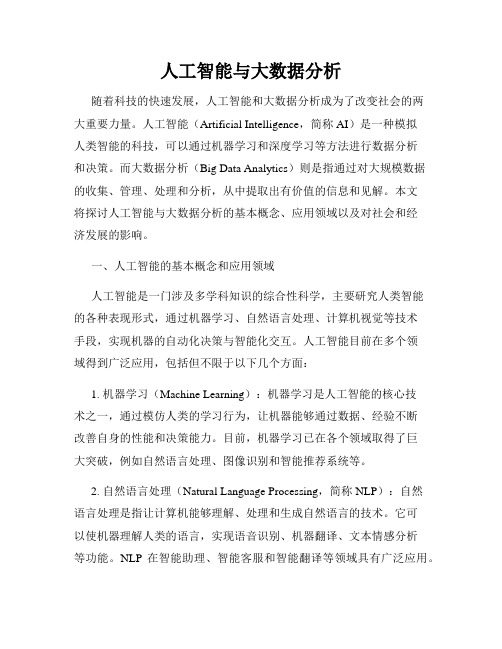
人工智能与大数据分析随着科技的快速发展,人工智能和大数据分析成为了改变社会的两大重要力量。
人工智能(Artificial Intelligence,简称AI)是一种模拟人类智能的科技,可以通过机器学习和深度学习等方法进行数据分析和决策。
而大数据分析(Big Data Analytics)则是指通过对大规模数据的收集、管理、处理和分析,从中提取出有价值的信息和见解。
本文将探讨人工智能与大数据分析的基本概念、应用领域以及对社会和经济发展的影响。
一、人工智能的基本概念和应用领域人工智能是一门涉及多学科知识的综合性科学,主要研究人类智能的各种表现形式,通过机器学习、自然语言处理、计算机视觉等技术手段,实现机器的自动化决策与智能化交互。
人工智能目前在多个领域得到广泛应用,包括但不限于以下几个方面:1. 机器学习(Machine Learning):机器学习是人工智能的核心技术之一,通过模仿人类的学习行为,让机器能够通过数据、经验不断改善自身的性能和决策能力。
目前,机器学习已在各个领域取得了巨大突破,例如自然语言处理、图像识别和智能推荐系统等。
2. 自然语言处理(Natural Language Processing,简称NLP):自然语言处理是指让计算机能够理解、处理和生成自然语言的技术。
它可以使机器理解人类的语言,实现语音识别、机器翻译、文本情感分析等功能。
NLP在智能助理、智能客服和智能翻译等领域具有广泛应用。
3. 计算机视觉(Computer Vision):计算机视觉是让机器能够理解和解释图像和视频的技术领域。
通过计算机视觉技术,机器可以实现图像识别、目标检测、人脸识别等功能。
计算机视觉广泛应用于人脸支付、智能监控和自动驾驶等领域。
二、大数据分析的基本概念和应用领域大数据分析是指通过对海量的、多样化的数据进行收集、管理和分析,以获得有价值的信息和见解的过程。
大数据分析需要运用特定的技术和工具,例如数据挖掘、机器学习和数据可视化等。
大数据4v

大数据(Big Data)是指“无法用现有的软件工具提取、存储、搜索、共享、分析和处理的海量的、复杂的数据集合。
”业界通常用4个V(即Volume、Variety、Value、Velocity)来概括大数据的特征。
一是数据体量巨大(Volume)。
截至目前,人类生产的所有印刷材料的数据量是200PB(1PB=210TB),而历史上全人类说过的所有的话的数据量大约是5EB(1EB=210PB)。
当前,典型个人计算机硬盘的容量为TB量级,而一些大企业的数据量已经接近EB量级。
二是数据类型繁多(Variety)。
这种类型的多样性也让数据被分为结构化数据和非结构化数据。
相对于以往便于存储的以文本为主的结构化数据,非结构化数据越来越多,包括网络日志、音频、视频、图片、地理位置信息等,这些多类型的数据对数据的处理能力提出了更高要求。
三是价值密度低(Value)。
价值密度的高低与数据总量的大小成反比。
以视频为例,一部1小时的视频,在连续不间断的监控中,有用数据可能仅有一二秒。
如何通过强大的机器算法更迅速地完成数据的价值“提纯”成为目前大数据背景下亟待解决的难题。
四是处理速度快(Velocity)。
这是大数据区分于传统数据挖掘的最显著特征。
根据IDC的“数字宇宙”的报告,预计到2020年,全球数据使用量将达到35.2ZB。
在如此海量的数据面前,处理数据的效率就是企业的生命。
2001年,高德纳分析员道格·莱尼在一份与其2001年的研究相关的演讲中指出,数据增长有三个方向的挑战和机遇:量(Volume),即数据多少;速(Velocity),即资料输入、输出的速度;类(Variety),即多样性。
在莱尼的理论基础上,IBM提出大数据的4V特征?得到了业界的广泛认可。
第一,数量(Volume),即数据巨大,从TB级别跃升到PB级别;第二,多样性(Variety),即数据类型繁多,不仅包括传统的格式化数据,还包括来自互联网的网络日志、视频、图片、地理位置信息等;第三,速度(Velocity),即处理速度快;第四,真实性(Veracity),即追求高质量的数据。
数据分析与大数据

数据分析与大数据随着信息技术的迅速发展,我们正逐渐进入一个大数据时代。
大数据作为一种重要的资源,被广泛应用于各个领域,尤其是数据分析。
数据分析是通过对大数据进行收集、处理、分析和挖掘,以发现潜在的规律和趋势,为决策提供支持和指导。
本文将从数据分析和大数据的定义、应用领域以及挑战等方面进行探讨。
一、数据分析和大数据的定义1. 数据分析的定义数据分析是指通过对数据进行收集、整理、处理、分析和解释,以获取有价值的信息和知识,从而为决策提供有力的支持和指导。
数据分析可以帮助人们理解数据背后的规律、趋势和关联性,并为企业、组织和个人提供决策依据。
2. 大数据的定义大数据是指规模庞大、复杂多样、高维度和高速度的数据集合。
大数据通常具有四个特点:可存储性、可处理性、可推断性和可挖掘性。
大数据的主要来源包括传感器数据、社交媒体数据、云计算数据等。
二、数据分析和大数据的应用领域1. 商业和市场领域数据分析和大数据在商业和市场领域有着广泛的应用。
通过对消费者行为数据和市场竞争数据的分析,可以为企业提供销售预测、市场定位、客户细分等方面的指导,从而帮助企业制定营销策略和提高市场竞争力。
2. 医疗和健康领域大数据在医疗和健康领域的应用正在逐渐增多。
通过对大量的医疗数据进行分析,可以帮助医生诊断疾病、制定治疗方案,并为科学研究提供依据。
同时,大数据还可以用于监测公共卫生事件和疾病爆发,及时采取相应的防控措施。
3. 城市规划和交通管理大数据在城市规划和交通管理方面有着重要的应用。
通过对城市居民的出行行为数据进行分析,可以帮助城市规划师规划交通网络,优化交通流量,提高城市交通效率。
同时,大数据还可以用于智能交通管理,实现交通信号的智能调控和交通拥堵预测等功能。
4. 金融风控和投资决策大数据在金融行业尤其是风险控制和投资决策方面具有重要作用。
通过对大量金融交易数据的分析,可以帮助金融机构识别风险、预测市场波动、制定投资策略,并提高风险管理能力和资金利用效率。
常见的大数据术语表(中英文对照版)
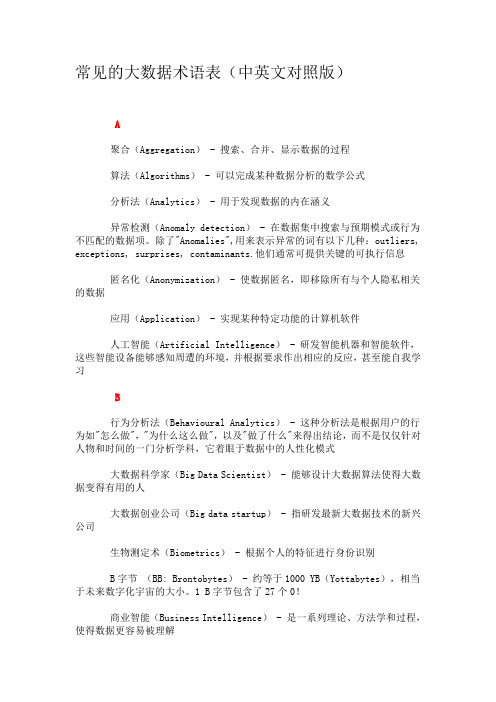
常见的大数据术语表(中英文对照版)A聚合(Aggregation) - 搜索、合并、显示数据的过程算法(Algorithms) - 可以完成某种数据分析的数学公式分析法(Analytics) - 用于发现数据的内在涵义异常检测(Anomaly detection) - 在数据集中搜索与预期模式或行为不匹配的数据项。
除了"Anomalies",用来表示异常的词有以下几种:outliers, exceptions, surprises, contaminants.他们通常可提供关键的可执行信息匿名化(Anonymization) - 使数据匿名,即移除所有与个人隐私相关的数据应用(Application) - 实现某种特定功能的计算机软件人工智能(Artificial Intelligence) - 研发智能机器和智能软件,这些智能设备能够感知周遭的环境,并根据要求作出相应的反应,甚至能自我学习B行为分析法(Behavioural Analytics) - 这种分析法是根据用户的行为如"怎么做","为什么这么做",以及"做了什么"来得出结论,而不是仅仅针对人物和时间的一门分析学科,它着眼于数据中的人性化模式大数据科学家(Big Data Scientist) - 能够设计大数据算法使得大数据变得有用的人大数据创业公司(Big data startup) - 指研发最新大数据技术的新兴公司生物测定术(Biometrics) - 根据个人的特征进行身份识别B字节(BB: Brontobytes) - 约等于1000 YB(Yottabytes),相当于未来数字化宇宙的大小。
1 B字节包含了27个0!商业智能(Business Intelligence) - 是一系列理论、方法学和过程,使得数据更容易被理解C分类分析(Classification analysis) - 从数据中获得重要的相关性信息的系统化过程;这类数据也被称为元数据(meta data),是描述数据的数据云计算(Cloud computing) - 构建在网络上的分布式计算系统,数据是存储于机房外的(即云端)聚类分析(Clustering analysis) - 它是将相似的对象聚合在一起,每类相似的对象组合成一个聚类(也叫作簇)的过程。
常用大数据词汇中英文对照表
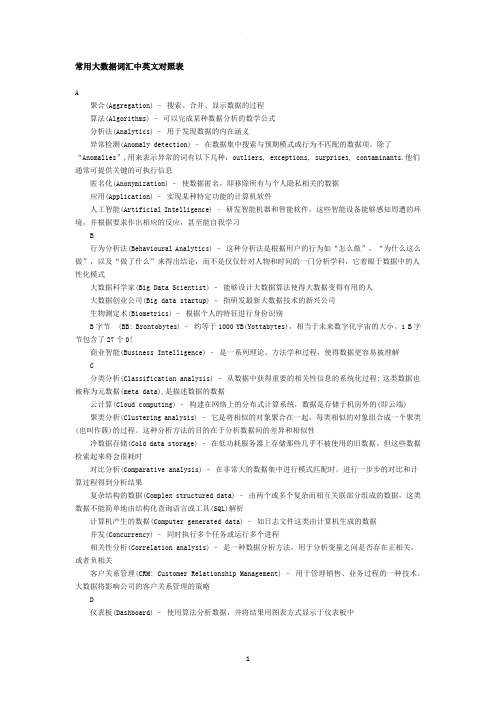
常用大数据词汇中英文对照表A聚合(Aggregation)–搜索、合并、显示数据的过程算法(Algorithms)–可以完成某种数据分析的数学公式分析法(Analytics)–用于发现数据的内在涵义异常检测(Anomaly detection)–在数据集中搜索与预期模式或行为不匹配的数据项。
除了“Anomalies”,用来表示异常的词有以下几种:outliers, exceptions, surprises, contaminants.他们通常可提供关键的可执行信息匿名化(Anonymization)–使数据匿名,即移除所有与个人隐私相关的数据应用(Application)–实现某种特定功能的计算机软件人工智能(Artificial Intelligence)–研发智能机器和智能软件,这些智能设备能够感知周遭的环境,并根据要求作出相应的反应,甚至能自我学习B行为分析法(Behavioural Analytics)–这种分析法是根据用户的行为如“怎么做”,“为什么这么做”,以及“做了什么”来得出结论,而不是仅仅针对人物和时间的一门分析学科,它着眼于数据中的人性化模式大数据科学家(Big Data Scientist)–能够设计大数据算法使得大数据变得有用的人大数据创业公司(Big data startup)–指研发最新大数据技术的新兴公司生物测定术(Biometrics)–根据个人的特征进行身份识别B字节(BB: Brontobytes)–约等于1000 YB(Yottabytes),相当于未来数字化宇宙的大小。
1 B字节包含了27个0!商业智能(Business Intelligence)–是一系列理论、方法学和过程,使得数据更容易被理解C分类分析(Classification analysis)–从数据中获得重要的相关性信息的系统化过程;这类数据也被称为元数据(meta data),是描述数据的数据云计算(Cloud computing)–构建在网络上的分布式计算系统,数据是存储于机房外的(即云端) 聚类分析(Clustering analysis)–它是将相似的对象聚合在一起,每类相似的对象组合成一个聚类(也叫作簇)的过程。
- 1、下载文档前请自行甄别文档内容的完整性,平台不提供额外的编辑、内容补充、找答案等附加服务。
- 2、"仅部分预览"的文档,不可在线预览部分如存在完整性等问题,可反馈申请退款(可完整预览的文档不适用该条件!)。
- 3、如文档侵犯您的权益,请联系客服反馈,我们会尽快为您处理(人工客服工作时间:9:00-18:30)。