全美数学建模大赛A论文-环岛城市交通
美国大学生数学建模竞赛优秀论文
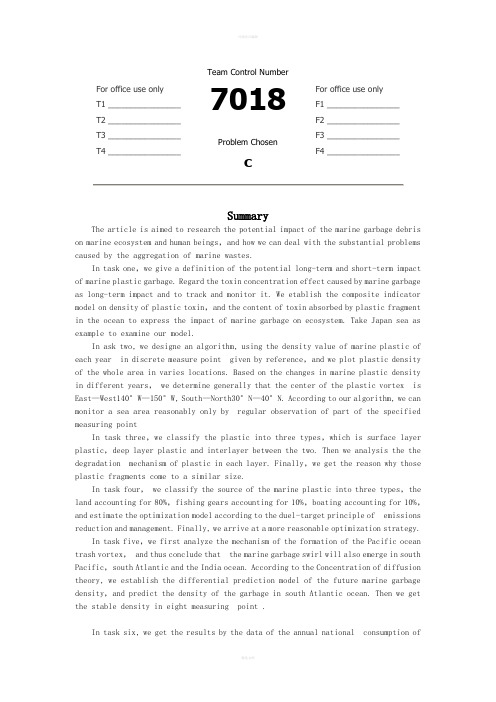
For office use onlyT1________________ T2________________ T3________________ T4________________Team Control Number7018Problem ChosencFor office use onlyF1________________F2________________F3________________F4________________ SummaryThe article is aimed to research the potential impact of the marine garbage debris on marine ecosystem and human beings,and how we can deal with the substantial problems caused by the aggregation of marine wastes.In task one,we give a definition of the potential long-term and short-term impact of marine plastic garbage. Regard the toxin concentration effect caused by marine garbage as long-term impact and to track and monitor it. We etablish the composite indicator model on density of plastic toxin,and the content of toxin absorbed by plastic fragment in the ocean to express the impact of marine garbage on ecosystem. Take Japan sea as example to examine our model.In ask two, we designe an algorithm, using the density value of marine plastic of each year in discrete measure point given by reference,and we plot plastic density of the whole area in varies locations. Based on the changes in marine plastic density in different years, we determine generally that the center of the plastic vortex is East—West140°W—150°W, South—North30°N—40°N. According to our algorithm, we can monitor a sea area reasonably only by regular observation of part of the specified measuring pointIn task three,we classify the plastic into three types,which is surface layer plastic,deep layer plastic and interlayer between the two. Then we analysis the the degradation mechanism of plastic in each layer. Finally,we get the reason why those plastic fragments come to a similar size.In task four, we classify the source of the marine plastic into three types,the land accounting for 80%,fishing gears accounting for 10%,boating accounting for 10%,and estimate the optimization model according to the duel-target principle of emissions reduction and management. Finally, we arrive at a more reasonable optimization strategy.In task five,we first analyze the mechanism of the formation of the Pacific ocean trash vortex, and thus conclude that the marine garbage swirl will also emerge in south Pacific,south Atlantic and the India ocean. According to the Concentration of diffusion theory, we establish the differential prediction model of the future marine garbage density,and predict the density of the garbage in south Atlantic ocean. Then we get the stable density in eight measuring point .In task six, we get the results by the data of the annual national consumption ofpolypropylene plastic packaging and the data fitting method, and predict the environmental benefit generated by the prohibition of polypropylene take-away food packaging in the next decade. By means of this model and our prediction,each nation will reduce releasing 1.31 million tons of plastic garbage in next decade.Finally, we submit a report to expediction leader,summarize our work and make some feasible suggestions to the policy- makers.Task 1:Definition:●Potential short-term effects of the plastic: the hazardeffects will be shown in the short term.●Potential long-term effects of the plastic: thepotential effects, of which hazards are great, willappear after a long time.The short- and long-term effects of the plastic on the ocean environment:In our definition, the short-term and long-term effects of the plastic on the ocean environment are as follows.Short-term effects:1)The plastic is eaten by marine animals or birds.2) Animals are wrapped by plastics, such as fishing nets, which hurt or even kill them.3)Deaden the way of the passing vessels.Long-term effects:1)Enrichment of toxins through the food chain: the waste plastic in the ocean has no natural degradation in theshort-term, which will first be broken down into tinyfragments through the role of light, waves,micro-organisms, while the molecular structure has notchanged. These "plastic sands", easy to be eaten byplankton, fish and other, are Seemingly very similar tomarine life’s food,causing the enrichment and delivery of toxins.2)Accelerate the greenhouse effect: after a long-term accumulation and pollution of plastics, the waterbecame turbid, which will seriously affect the marineplants (such as phytoplankton and algae) inphotosynthesis. A large number of plankton’s deathswould also lower the ability of the ocean to absorbcarbon dioxide, intensifying the greenhouse effect tosome extent.To monitor the impact of plastic rubbish on the marine ecosystem:According to the relevant literature, we know that plastic resin pellets accumulate toxic chemicals , such as PCBs、DDE , and nonylphenols , and may serve as a transport medium and soure of toxins to marine organisms that ingest them[]2. As it is difficult for the plastic garbage in the ocean to complete degradation in the short term, the plastic resin pellets in the water will increase over time and thus absorb more toxins, resulting in the enrichment of toxins and causing serious impact on the marine ecosystem.Therefore, we track the monitoring of the concentration of PCBs, DDE, and nonylphenols containing in the plastic resin pellets in the sea water, as an indicator to compare the extent of pollution in different regions of the sea, thus reflecting the impact of plastic rubbish on ecosystem.To establish pollution index evaluation model: For purposes of comparison, we unify the concentration indexes of PCBs, DDE, and nonylphenols in a comprehensive index.Preparations:1)Data Standardization2)Determination of the index weightBecause Japan has done researches on the contents of PCBs,DDE, and nonylphenols in the plastic resin pellets, we illustrate the survey conducted in Japanese waters by the University of Tokyo between 1997 and 1998.To standardize the concentration indexes of PCBs, DDE,and nonylphenols. We assume Kasai Sesside Park, KeihinCanal, Kugenuma Beach, Shioda Beach in the survey arethe first, second, third, fourth region; PCBs, DDE, andnonylphenols are the first, second, third indicators.Then to establish the standardized model:j j jij ij V V V V V min max min --= (1,2,3,4;1,2,3i j ==)wherej V max is the maximum of the measurement of j indicator in the four regions.j V min is the minimum of the measurement of j indicatorstandardized value of j indicator in i region.According to the literature [2], Japanese observationaldata is shown in Table 1.Table 1. PCBs, DDE, and, nonylphenols Contents in Marine PolypropyleneTable 1 Using the established standardized model to standardize, we have Table 2.In Table 2,the three indicators of Shioda Beach area are all 0, because the contents of PCBs, DDE, and nonylphenols in Polypropylene Plastic Resin Pellets in this area are the least, while 0 only relatively represents the smallest. Similarly, 1 indicates that in some area the value of a indicator is the largest.To determine the index weight of PCBs, DDE, and nonylphenolsWe use Analytic Hierarchy Process (AHP) to determine the weight of the three indicators in the general pollution indicator. AHP is an effective method which transforms semi-qualitative and semi-quantitative problems into quantitative calculation. It uses ideas of analysis and synthesis in decision-making, ideally suited for multi-index comprehensive evaluation.Hierarchy are shown in figure 1.Fig.1 Hierarchy of index factorsThen we determine the weight of each concentrationindicator in the generall pollution indicator, and the process are described as follows:To analyze the role of each concentration indicator, we haveestablished a matrix P to study the relative proportion.⎥⎥⎥⎦⎤⎢⎢⎢⎣⎡=111323123211312P P P P P P P Where mn P represents the relative importance of theconcentration indicators m B and n B . Usually we use 1,2,…,9 and their reciprocals to represent different importance. The greater the number is, the more important it is. Similarly, the relative importance of m B and n B is mn P /1(3,2,1,=n m ).Suppose the maximum eigenvalue of P is m ax λ, then theconsistency index is1max --=n nCI λThe average consistency index is RI , then the consistencyratio isRICI CR = For the matrix P of 3≥n , if 1.0<CR the consistency isthougt to be better, of which eigenvector can be used as the weight vector.We get the comparison matrix accoding to the harmful levelsof PCBs, DDE, and nonylphenols and the requirments ofEPA on the maximum concentration of the three toxins inseawater as follows:⎥⎥⎥⎦⎤⎢⎢⎢⎣⎡=165416131431P We get the maximum eigenvalue of P by MATLAB calculation0012.3max =λand the corresponding eigenvector of it is()2393.02975.09243.0,,=W1.0042.012.1047.0<===RI CI CR Therefore,we determine the degree of inconsistency formatrix P within the permissible range. With the eigenvectors of p as weights vector, we get thefinal weight vector by normalization ()1638.02036.06326.0',,=W . Defining the overall target of pollution for the No i oceanis i Q , among other things the standardized value of threeindicators for the No i ocean is ()321,,i i i i V V V V = and the weightvector is 'W ,Then we form the model for the overall target of marine pollution assessment, (3,2,1=i )By the model above, we obtained the Value of the totalpollution index for four regions in Japanese ocean in Table 3T B W Q '=In Table3, the value of the total pollution index is the hightest that means the concentration of toxins in Polypropylene Plastic Resin Pellets is the hightest, whereas the value of the total pollution index in Shioda Beach is the lowest(we point up 0 is only a relative value that’s not in the name of free of plastics pollution)Getting through the assessment method above, we can monitor the concentration of PCBs, DDE and nonylphenols in the plastic debris for the sake of reflecting the influence to ocean ecosystem.The highter the the concentration of toxins,the bigger influence of the marine organism which lead to the inrichment of food chain is more and more dramatic.Above all, the variation of toxins’ concentration simultaneously reflects the distribution and time-varying of marine litter. We can predict the future development of marine litter by regularly monitoring the content of these substances, to provide data for the sea expedition of the detection of marine litter and reference for government departments to make the policies for ocean governance.Task 2:In the North Pacific, the clockwise flow formed a never-ending maelstrom which rotates the plastic garbage. Over the years, the subtropical eddy current in North Pacific gathered together the garbage from the coast or the fleet, entrapped them in the whirlpool, and brought them to the center under the action of the centripetal force, forming an area of 3.43 million square kilometers (more than one-third of Europe) .As time goes by, the garbage in the whirlpool has the trend of increasing year by year in terms of breadth, density, and distribution. In order to clearly describe the variability of the increases over time and space, according to “Count Densities of Plastic Debris from Ocean Surface Samples North Pacific Gyre 1999—2008”, we analyze the data, exclude them with a great dispersion, and retain them with concentrated distribution, while the longitude values of the garbage locations in sampled regions of years serve as the x-coordinate value of a three-dimensional coordinates, latitude values as the y-coordinate value, the Plastic Count per cubic Meter of water of the position as the z-coordinate value. Further, we establish an irregular grid in the yx plane according to obtained data, and draw a grid line through all the data points. Using the inverse distance squared method with a factor, which can not only estimate the Plastic Count per cubic Meter of water of any position, but also calculate the trends of the Plastic Counts per cubic Meter of water between two original data points, we can obtain the unknown grid points approximately. When the data of all the irregular grid points are known (or approximately known, or obtained from the original data), we can draw the three-dimensional image with the Matlab software, which can fully reflect the variability of the increases in the garbage density over time and space.Preparations:First, to determine the coordinates of each year’s sampled garbage.The distribution range of garbage is about the East - West 120W-170W, South - North 18N-41N shown in the “Count Densities of Plastic Debris from Ocean Surface Samples North Pacific Gyre 1999--2008”, we divide a square in the picture into 100 grids in Figure (1) as follows:According to the position of the grid where the measuring point’s center is, we can identify the latitude and longitude for each point, which respectively serve as the x- and y- coordinate value of the three-dimensional coordinates.To determine the Plastic Count per cubic Meter of water. As the “Plastic Count per cubic Meter of water” provided by “Count Densities of P lastic Debris from Ocean Surface Samples North Pacific Gyre 1999--2008”are 5 density interval, to identify the exact values of the garbage density of one year’s different measuring points, we assume that the density is a random variable which obeys uniform distribution in each interval.Uniform distribution can be described as below:()⎪⎩⎪⎨⎧-=01a b x f ()others b a x ,∈We use the uniform function in Matlab to generatecontinuous uniformly distributed random numbers in each interval, which approximately serve as the exact values of the garbage density andz-coordinate values of the three-dimensional coordinates of the year’s measuring points.Assumptions(1)The data we get is accurate and reasonable.(2)Plastic Count per cubic Meter of waterIn the oceanarea isa continuous change.(3)Density of the plastic in the gyre is a variable by region.Density of the plastic in the gyre and its surrounding area is interdependent , However, this dependence decreases with increasing distance . For our discussion issue, Each data point influences the point of each unknown around and the point of each unknown around is influenced by a given data point. The nearer a given data point from the unknown point, the larger the role.Establishing the modelFor the method described by the previous,we serve the distributions of garbage density in the “Count Pensities of Plastic Debris from Ocean Surface Samples North Pacific Gyre 1999--2008”as coordinates ()z y,, As Table 1:x,Through analysis and comparison, We excluded a number of data which has very large dispersion and retained the data that is under the more concentrated the distribution which, can be seen on Table 2.In this way, this is conducive for us to get more accurate density distribution map.Then we have a segmentation that is according to the arrangement of the composition of X direction and Y direction from small to large by using x co-ordinate value and y co-ordinate value of known data points n, in order to form a non-equidistant Segmentation which has n nodes. For the Segmentation we get above,we only know the density of the plastic known n nodes, therefore, we must find other density of the plastic garbage of n nodes.We only do the sampling survey of garbage density of the north pacificvortex,so only understand logically each known data point has a certain extent effect on the unknown node and the close-known points of density of the plastic garbage has high-impact than distant known point.In this respect,we use the weighted average format, that means using the adverse which with distance squared to express more important effects in close known points. There're two known points Q1 and Q2 in a line ,that is to say we have already known the plastic litter density in Q1 and Q2, then speculate the plastic litter density's affects between Q1、Q2 and the point G which in the connection of Q1 and Q2. It can be shown by a weighted average algorithm22212221111121GQ GQ GQ Z GQ Z Z Q Q G +*+*=in this formula GQ expresses the distance between the pointG and Q.We know that only use a weighted average close to the unknown point can not reflect the trend of the known points, we assume that any two given point of plastic garbage between the changes in the density of plastic impact the plastic garbage density of the unknown point and reflecting the density of plastic garbage changes in linear trend. So in the weighted average formula what is in order to presume an unknown point of plastic garbage density, we introduce the trend items. And because the greater impact at close range point, and thus the density of plastic wastes trends close points stronger. For the one-dimensional case, the calculation formula G Z in the previous example modify in the following format:2212122212212122211111112121Q Q GQ GQ GQ Q Q GQ Z GQ Z GQ Z Z Q Q Q Q G ++++*+*+*=Among them, 21Q Q known as the separation distance of the known point, 21Q Q Z is the density of plastic garbage which is the plastic waste density of 1Q and 2Q for the linear trend of point G . For the two-dimensional area, point G is not on the line 21Q Q , so we make a vertical from the point G and cross the line connect the point 1Q and 2Q , and get point P , the impact of point P to 1Q and 2Q just like one-dimensional, and the one-dimensional closer of G to P , the distant of G to P become farther, the smaller of the impact, so the weighting factor should also reflect the GP in inversely proportional to a certain way, then we adopt following format:221212222122121222211111112121Q Q GQ GP GQ GQ Q Q GQ GP Z GQ Z GQ Z Z P Q Q Q Q G ++++++*+*+*=Taken together, we speculated following roles:(1) Each known point data are influence the density of plastic garbage of each unknown point in the inversely proportional to the square of the distance;(2) the change of density of plastic garbage between any two known points data, for each unknown point are affected, and the influence to each particular point of their plastic garbage diffuse the straight line along the two known particular point; (3) the change of the density of plastic garbage between any two known data points impact a specific unknown points of the density of plastic litter depends on the three distances: a. the vertical distance to a straight line which is a specific point link to a known point;b. the distance between the latest known point to a specific unknown point;c. the separation distance between two known data points.If we mark 1Q ,2Q ,…,N Q as the location of known data points,G as an unknown node, ijG P is the intersection of the connection of i Q ,j Q and the vertical line from G to i Q ,j Q()G Q Q Z j i ,,is the density trend of i Q ,j Q in the of plasticgarbage points and prescribe ()G Q Q Z j i ,,is the testing point i Q ’ s density of plastic garbage ,so there are calculation formula:()()∑∑∑∑==-==++++*=Ni N ij ji i ijGji i ijG N i Nj j i G Q Q GQ GPQ Q GQ GP G Q Q Z Z 11222222111,,Here we plug each year’s observational data in schedule 1 into our model, and draw the three-dimensional images of the spatial distribution of the marine garbage ’s density with Matlab in Figure (2) as follows:199920002002200520062007-2008(1)It’s observed and analyzed that, from 1999 to 2008, the density of plastic garbage is increasing year by year and significantly in the region of East – West 140W-150W, south - north 30N-40N. Therefore, we can make sure that this region is probably the center of the marine litter whirlpool. Gathering process should be such that the dispersed garbage floating in the ocean move with the ocean currents and gradually close to the whirlpool region. At the beginning, the area close to the vortex will have obviously increasable about plastic litter density, because of this centripetal they keeping move to the center of the vortex ,then with the time accumulates ,the garbage density in the center of the vortex become much bigger and bigger , at last it becomes the Pacific rubbish island we have seen today.It can be seen that through our algorithm, as long as the reference to be able to detect the density in an area which has a number of discrete measuring points,Through tracking these density changes ,we Will be able to value out all the waters of the density measurement through our models to determine,This will reduce the workload of the marine expedition team monitoring marine pollution significantly, and also saving costs .Task 3:The degradation mechanism of marine plasticsWe know that light, mechanical force, heat, oxygen, water, microbes, chemicals, etc. can result in the degradation of plastics . In mechanism ,Factors result in the degradation can be summarized as optical ,biological,and chemical。
美赛数学建模文章交通建模方面

SummaryWith the rapidly developing of traffic, freeway gradually becomes the mainstream way of short-distance travel. In order to make the means of transportation become more perfect, we need to improve in as many aspects as possible. To measure the performance of a freeway, we must consider the following two factors: traffic flow and safety. These are the main aspects that we must take into consideration to weigh whether a freeway is good or bad.In order to better simulate the actual situation, we established a simulationmodel .We adopted the core ideas of the Cellular Automata Model, on whose basis, we established a new model suitable to the simulation of the performance on freeway. The key point of our model is regarding time and space to be discrete which is actually continuous. Every vehicle must be in certain discrete position. In this problem, we divide the road into many same-size rectangular grids, the vehicle must move in a fixed place. he number of grids stands for the distance, the number of the grids that a vehicle move per unit time stands for it‘s speed. According to different rules, different small models are respectively established to study which rule is better. In a word the model we designed has combined the advantages of the Cellular Automata Model and the most important aspects of the actual situation on the highway.To study the performance more accurately, We have studied under the following three conditions:1. under very light traffic load;2. under a medium traffic load (normal traffic conditions)(main part);3. under a very heavy traffic load.In each case, we have analyzed the performance on freeway and discussed the traffic flow both in theory and by simulation. We have also calculated how the drivers on freeway guarantee their safety quantitatively. After that, we examined tradeoffs between traffic flow and safety, and analyzed the how each case limit the speed and overtaking ratio. Through analysis, we have got relatively reasonable conclusions. Differently, in case1, we gave an actual example to test our model. In case 2, we respectively analyzed the following and passing phenomenon in detail.Safety on freeway is so important that we have studied how much the traffic flow and speed influence it, we have calculated the two safety correlation coefficients of the traffic flow and speed and conclude that speed influence safety most.We have made comprehensive evaluation of ―the rule that requires drivers to drivein the right-most lane unless they are passing another vehicle, in which case they move one lane to the left, pass, and return to their former travel lane‖ , and designed a new rule that―two lane used equally‖ to promote greater traffic flow while guaranteeing safe. The new rule has been tested by simulation.In countries where driving vehicles on the left is the norm, we have analyzed their performance on freeway,we found that my solution cannot be carried over with a simple change of orientation, additional requirements that the position of cab be changed should be needed.If vehicle transportation on the same roadway was fully under the control of an intelligent system, the most obvious change is the change of overtaking ratio (becomes almost 100%), this change will decrease traffic flow in our earlier analysis.ContentsAssumption and it‘s Rationality (5)1. Model (5)1.1 Basic model (5)1.2 Feasibility and rationality of the model (6)1.3 How we set the parameters in the model (6)1.4 Simulation of different situation that we use in the article (6)1.4.1 Rules of the single-lane Cellular Automata model (6)1.4.2 The lane changing model (7)1.4.2.1 The lane changing rules (7)1.4.2.2 Explanations of the lane changingrules (7)1.4.3 Lane Changing Model verification (8)2. Different traffic density (8)2.1 Under very light traffic load (9)2.1.1 Traffic flow calculation and simulation (9)2.1.2 Safety guarantee (10)2.1.3Speed limit (11)2.1.4 Overtaking ratio limit (11)2.1.5An actual example (11)2.2 Medium traffic load (Normal traffic conditions) (12)2.2.1 Three factors influencing on traffic flow andsimulation (12)2.2.2 Safety guarantee (16)2.2.2.1 Following phenomenon (16)2.2.2.2 Overtaking phenomenon (17)2.2.3Speed limit (18)2.2.4 Overtaking ratio limit (18)2.3 Very heavy traffic load (18)2.3.1 Safety factors analysis (18)2.3.2Influence on traffic flow and simulation (19)2.3.3Speed limit(very low speed) (19)2.3.4 Overtaking ratio limit (19)2.4. Safety correlation coefficient (19)3. A better rule (20)3.1 Description (20)3.2 Simulation (21)4. For countries driving on the left (21)5. Intelligent controlled transportation system (22)Futher analysis of our model (22)Conclusion (23)Reference (24)Assumption and it’s RationalityAll the length is dispersive. Our model describes the movement of each individual vehicle according to the study for their interaction by taking vehicles as dispersive particles. Cellular Automata model divides a section of road into many cells of 2 meters in length.The time interval is one second. As length is dispersive, the time is dispersive. We make the minimum time interval is one second. One second is short enough to describe the motion of the car.The length of the car is the same. In some freeway the big truck is prohibited. The number of small cars dominates. We just study the situation that small car driving on the freeway. The length of the car is 4 meters or so. We take it as a average 2 cell length.The number of the cars on a selected section of the road is a constant. We only study a section of the road. We designed it as a closed loop which means one car gets out and one car enters. So the number of the cars on a selected section of the road is a constant. In this way, the density of the cars on the road is a constant.We ignore the factor of weather and season. Different weather may lead to different traffic. The situation is complex that we have to ignore these factors.The steering wheel is on the right side of the car. It is a common that n countries where driving vehicles on the left is the norm the steering wheel is on the right side of the car. It is also a fact in US and China.Passing is not allowed to single road. At the same time a cell can be occupied by only one car. So the car cannot pass another car in front on the same road. Analysis of the problem1. Model1.1 Basic modelThe key point of our model is regarding time and space as discrete which is actually continuous. Every vehicle must be in certain discrete position. In this problem, we divide the road into many of the same size rectangular grids, the vehicle must move in a fixed place.The number of grids stands for the distance, the number of the grids that a vehicle move per unit time stands for it‘s speed.1.2 Feasibility and rationality of the modelWhen we analyze the problem, the distance we consider is long enough, and time is also long enough, dividing time and space into many small parts will not influence the results of analysis and simulation so much. On the contrary, the way we make time and space discrete will simplify the analysis and calculation process to a great extent, it can also make simulation much more easy.1.3 How we set the parameters in the modelConsidering the various aspects of factors, the Basic parameter definiteness is as follows:In this model, the length of each cell is 2 meters, per 2 successive cells containone vehicle and these 2 successive cells are in the same state at moment t, i.e. thespeed of vehicle contained. Maximum speed of vehicle is 120km/h(33m/s). Minimum speed of vehicle is 80km/h(22m/s).Thus in this model, maximum speed (v m) is 16 cell length/second, minimum speed(v min) is 11 cell length/second. Speed value range is v min~v m and renewal time interval is 1 second.1.4 Simulation of different situation that we use in the article1.4.1 Rules of the single-lane Cellular Automata modelVariable symbols used in this Model are defined as follows.x n(t):the position of the vehicle at moment t;v n(t):the speed of the vehicle at moment t;a n(t):the acceleration of the vehicle at moment t~t+1;g n(t):the number offree sites ahead of the vehicle, i.e.g n(t)=x n-1(t)-x n(t)-2.The states of all vehicles on road conduct synchronous renewal according to the following rules.Acceleration Rule: if v n(t) ≤ g n(t), the vehicle will accelerate.If g n(t) - v n(t) < 2, then a n(t) = g n(t) - v n(t).If g n(t) - v n(t) ≥ 2, then a n(t) = 2.If v n(t) = v m, then a n(t) = 0.Deceleration Rule:If v n(t) >g n(t), the vehicle will decelerate.If g n(t) - v n(t) > -2, then a n(t) = g n(t) - v n(t).If g n(t) - v n(t) ≤ -2, then a n(t) = -2.Correction Rule:If the acceleration of the vehicle is a n(t) at moment t, on the assumption that the forward vehicle is decelerated at maximum deceleration, then at moment t+1.If v n(t+1) ≤ g n(t+1), then the acceleration of the vehicle is a n(t).If v n(t+1) >g n(t+1), then the acceleration of the vehicle is a n(t)-1, and recalculate the v n(t+1) and g n(t+1), until v n(t+1) ≤ g n(t+1).Thus, the actual acceleration of the vehicle is a corrected value.1.4.2 The lane changing modelLane changing is the emphasis and difficulty of multi-lane road traffic flow simulation. A lane change decision process is assumed to have the following three steps: production of lane changing desire, feasibility analysis on lane changing activity and implementation of lane changing activity (Zou,2002).Based on the single-lane NS model, K. Nagel has put forward the multi-lane traffic simulation model, in which, the vehicles moving in each lane shall conform to the NS rule and satisfy the Lane-changing rules (Nagel,1998/ Wagner,1997) when changing lanes. This article put forward a kind of lane-changing model that is suitable for vehicle movement in order on the urban roads under the unobstructed condition, which is shown to match the real vehicle activities well through computer. Simulation.1.4.2.1 The lane changing rulesVariable symbols used in this model are defined as follows.g n(t) = x n-1(t) - x n(t) - 2 (1) Here:g n(t)--the number of free sites ahead of the vehicle on the present lane at moment t g l(t)--the number of free sites between the vehicle and the forwardvehicle on target lane at moment tg b(t)--the number of free sites between the vehicle and the backward vehicle on target lane at moment tv l(t) --the speed of the forward vehicle on target lane at moment tv b(t)--the speed of the backward vehicle on target lane at moment ts b(t) --the emergency braking distance of the backward vehicle on target lane at moment tThe lane changing model is as follows:(1) ifg n(t) <v m, then the vehicle will produce lane changing desire(2) ifg l(t) ≥ g n(t) and v l (t) > v n-1 (t) and s b(t) ≤ s b(t), then the vehicle will change lane at v n(t) at probability pchangeHere, s b(t) = v b + max(v b - 2,0) + max(v b- 4,0) . (2)1.4.2.2 Explanations of the lane changing rulesIn this model, g n(t)<v m means due to the reason that the speed of forward vehicle isslower, that this vehicle will produce the desire of changing lane in order to reach faster speed and obtaining more free driving space.After producing the desire of changing lane, a vehicle will determine the feasibility of changing to adjacent lane according to observation. In general, a vehicle may chan- ge its lane only when the spaces between it and forward vehicle and it and backward vehicle are large enough. On condition of meeting g l(t)≥g n(t) and v l(t) > v n-1 (t), a vehicle can ensure that it will not collide with forward vehicle on target lane after changing its lane. On condition of meeting s b(t)≤s b(t), a vehicle will not collide with backward vehicle on target lane because the emergency braking distance of backward vehicle on target lane is less than the space between them. Only when meeting these conditions, a vehicle will implement lane changing activity at a certain probability. 1.4.3 Lane Changing Model verificationWe select 500-meter sections of two innermost lanes on the 4th Ring Road in Beijing as observation objectives to survey the lane changing condition at different time and under different flow in the condition of free flow. Observation period is 2 hours. Diamond shape points in Figure 1 are the survey number of lane changing under different volume. By linear regression fit, we can find that the relationship between number of lane changing and volume is linear.In accordance with the aforesaid lane-changing Cellular Automata model, we make a computer simulation for the lane-changing condition under the condition of free flow. During the simulation, we set up 500 cells, among which, 250 cells on the preparatory section (500m) and the other 250 cells on simulation section (500m), and the simulation time is 3900 seconds. The simulation within 0~300 seconds is the stage to clear up the bad effect, after a movement of 300 seconds, the road is full of vehicles. The simulation begins from the 301st second and simulation data is recorded after the first 250 cells, the flow diagram of lane-changing CellularAutomata model simulation is as follows.Simulations were conducted according to the above-mentioned process under different flows (i.e. 2500veh/h, 2600veh/h, 2700veh/h, 2800veh/h, 2900veh/h, 3000 veh/h), each flow is simulated for five times to acquire the average values, and thus, the lane-changing times under different flows are obtained. Comparing those simulated results (while p b=0.5 and p c=0.8)with the observed values, they are matching with each other by a large while p b and pc value are correctly selected so as to verify the validity of this Lane Changing Model.2. Different traffic densityThere‘s different performance under different traffic load so we must analyze in three parts:2.1 Under very light traffic loadWhen in light traffic, a vehicle is almost not constrained by other vehicles (free running). Drivers will run at a speed as much as possible to get the more benefits of driving such as shortening the travel time. It may raise traffic flow in some degree, however, this psychological state will cause certain threat to the safety. So the traffic flow and safety assessment in light traffic is necessary.2.1.1 Traffic flow calculation and simulationThough under low traffic load vehicles can run at a very high speed, the very low vehicle density plays a negative role. What‘s worse, the low vehicle density influence more on traffic flow in this case. In other words, the traffic flow will be very low.We assume that any vehicle can pass each other freely. The average interval of the vehicles is two thousand meters. The speed of vehicles varies from 80km/h to 120km/h. For the sake of simplicity, we choose only five kinds of speed: 80km/h, 90km/h, 100km/h, 110km/h and 120km/h. We assume that the quantity of each kind of speed of the vehicles is an equal.Compare this rule to the condition that all the vehicles run in one road without any pass.①Passing18090100110120/5052++++-=⨯=traffic flow hour (3) ②.No Passing ( the speed of almost all the cars is limited to under 80 km/h) 80/402traffic flow hour -== (4) This the result we get through calculation.Let‗s see the result of simulation:Fig1.under very low traffic loadThrough a certain tool, we can get the traffic flow (the number of vehicles through a cross-section we set in an hour).we have simulated 10 times and the data we got is as follows:42,44,49,38,45,46,44,43,47,51,45 (per hour)The average:40+42+38+44+40+41+42+45+44+43/=41.910traffic flow h -= (5) Comparing the theoretical calculation and simulation results, we can come to a similar conclusion with two methods, which has also supported our model.2.1.2 Safety guaranteeUnder very low traffic load, the main factor that influence safety is speed. Though there‘re other factors that may also influence, they are negligible relative to speed.The distance from the drivers found obstacles to the vehicle to a full stop is the sum of the reaction distance and braking distance. Shown in figure 2.Fig2. brake when finding an obstacleReaction distanceis the distance from the point where the driver finds an obstacle to the point where he starts to brake:1=t 3.6V S (6)Braking distance is the distance during the whole braking process:22=254(f+i)V S (7) The stopping sight distance:2=t+3.6254(f+i)V V S (8) Here:V--the speed of the vehicle (km/h);t--reaction time of the driver(s);f--coefficient of road adhesion (for dry pavement f=0.6);i--the tilt degree of the road (for level road i=0).L--the distance when the driver finds the obstacle between the obstacle and the vehicle.Suppose the safe distance is d, when +d >L S , t he vehicle will be in danger; when +d L S ≤, the vehicle is safe.2.1.3 Speed limitWhen the traffic load is light, vehicles can run as fast as possible under the biggest speed limit, however it will increase risk of accident; if the speed of some vehicles is too low, we can‘t make the best of the freeway thus reducing flow. So, under the premise of ensuring safety, we can run at a relatively high speed to increase traffic flow as possible as we can. A too high speed may lead to an accident.Speed is the main factor that influence the traffic flow.2.1.4Overtaking ratio limitSince the traffic load is very low, the possibility of overtaking phenomenon is very low, and in extreme cases the overtaking ratio can even be seen as zero.That is, in this case, overtaking is a very minor factor that influence traffic flow.2.1.5 An actual exampleWe have found an actual example in this case:2011, one day in July, Shanghai-Nanjing Freeway in Jiangsu province.Considering the weather and road condition, some experts major in it confirm that the coefficient of road adhesion f is 0.40. And according to the Shanghai-Nanjing Freeway designing information, the tilt degree of the road i is 0. The visibility that day is 55 meters. The safe distance is 5 meters.Substituting the data into equation :22.5d +53.6254(f+i)V S V +=+, (9) If the result we calculate is more than 55 meters, the vehicle can‘t brake in time, which will cause a collision between the vehicle and the obstacle.So under such condition, the speed of each vehicle must be limited.For example, a vehicle ran at V=80km/h that day on Shanghai-Nanjing Freeway,22.580d 80+5123.553.6254(0.40+0)S m +=⨯+≈ (10) This result is much higher than the visibility 55 meters, so V=80km/h is a very dangerous speed.We can also calculate the highest speed that is allowed under the terrible condition:Let:22.5d+5553.6254(f+i)VS V m+=+≤, (11)We get: V≤43.52km/h (12) We can see that in this example, drivers must control the speed under 43.52km/h in order to guarantee their safety.2.2 Medium traffic load (Normal traffic conditions)Medium traffic density is the most common case, that is to say, this is the most consistent with the actual situation under normal circumstances. Therefore, we personally think that studying this case makes the most common sense. When the traffic density is not so big or so small, due to the rule requiring drivers to drive in the right-most lane unless they overtake from the left lane, the motion of vehicles is not untrammeled,each one is in interference and constraints produced by others. The performance of vehicles on freeway is mainly following and overtaking, through practical experience.2.2.1 Three factors influencing on traffic flow and simulationThe main factors that influence the traffic flow are overtaking ratio, traffic density and speed of vehicles, the three factors are not completely independent, there‘s certain mutual restraint and influence between them.“overtaking ratio--- traffic flow” relationWe choose the overtaking ratio as the main verification index when we study the process of overtaking. Through a survey that has been made and a relative simulation, the traffic flow changes with the overtaking ratio. The survey method is as figure3,the survey conclusion is as the table 1.Fig 3.Sketch map of section observatin method for field surveyTab 1. Survey of traffic flow and overtaking ratioTo get more accurate result, we have made a simulation. To make sure how the traffic flow changes with the overtaking ratio, we set a series of overtaking ratios. Through the simulation, we get the ‖ traffic flow—overtaking changing curve.Fig 4. Traffic flow—overtaking changing curveComparing the results of the survey and the simulation, the rationality of the model can be made sure. According to the curve, the changing process is divided into two sections: the first section shows that in two-lane freeway, the overtaking ratio increases with the traffic flow raising, to the biggest; the second section shows that with the traffic flow raising, the overtaking ratio decreases, when the traffic flow increases to 2900pcu/h, the overtaking ratio is almost zero.“traffic density-- traffic flow” relationAccording to the equation:Q=KV (13)Here:Q---the traffic flow (pcu/h);K---traffic density (pcu/km);V---the average speed (km/h)If there‘s no special situation such as rear-ending, when V is a constant ,Q ∝K, the image is as figure 5.Fig5. Q-K relationHowever, our simulation result is as figure 6.and 7Fig 6 S imulation result of ―traffic density -- traffic flow‖ relationFig 7 . ―Traffic density-- traffic flow‖ relation curveDifference explanation :Increased density cannot be unlimited, Q = KV is the ideal case, the actual case will be affected by external factors, our simulation result is more realistic.Analysis :When the traffic density is less than the optimum density of traffic flow,traffic flow is in th e f ree driving state,the average speed of cars is high. Traffic flow does not reach the m aximum value.The increasing of density leads to the increasing of trafficflow;when th e traffic density is equal or close to the optimum density of traffic flow, traffic team fo llowing phenomenon appears, the speed will be limited. Different kinds of car approa- ching a speed constant speed, traffic volume will reach the maximum value; when the traffic density is greater than the optimum density of traffic flow, traffic flow is in the congestion state, because of traffic density increases gradually, vehicle speed and traff ic volume decrease at the same time and traffic jam happens or even parking phenome non.From the figure, we can get the following information:①When K=0,Q=0,the curve pass O of the coordinate system; ②0=dK dQ ,m j K K K ==21 ③From the point c ,if K become larger ,Q becomes smaller ,when K= K j ,V=0 Q=0④Drawing radius vector from the coordinate origin to any point on the curve, the slope of the radius vector stands for the average speed of the point.⑤ K ≤ Km: not crowded; K>Km: crowded.“speed -- traffic flow” relationAccording to the equation:Q=KVIf there‘s no special situation such as rear -ending, when K is a constant ,Q ∝V, the image is as figure 8.Fig 8 Q-A relationOur simulation also confirms the linear relationship.Further discussionThe equation Q=KV can be shown in an more unified way (figure 9)Fig9 .3-D image of Q=KVWe have known that )1(j f K K V V -=, so )1(jj V V K K -=, (14) So we can get a more specific form :)(2fj V V V K KV Q -==, (15) figure 9 has shown the equation.Fig 10.Q-V-K relation2.2.2 Safety guarantee2.2.2.1 Following phenomenonAccording to the accident statistics annals, rear-ending is the main part of the traffic accident, so we must guarantee the security when a vehicle follows another. We can easily come to a conclusion that the speed is the main factor influencing safety under the state of vehicle following the front one. When the front vehicle suddenly brake, whether the following vehicle can stop in time to avoid collision, and maintain at a safe distance determines the safety of the two vehicles. This process is shown infigure 11.Fig11.brake when the front vehicle brake suddenlyAccording to AASHTO parking stadia model, the distance that the front vehicle A run from starting braking to stopping completely is:2254(f+i)A A V S (16)After reaction time, vehicle B also start to brake, the distance that the front vehicle B run from starting braking to stopping completely is:2t 3.6254(f+i)B B B V V S =+(17)Here: S A the speed of the front vehicle A (km/h);S B --the speed of the latter vehicle B (km/h);t--the reaction time of driver (s);f--coefficient of road adhesion (for dry pavement f=0.6);i--the tilt degree of the road (for level road i=0).Suppose two vehicles are L away from each other when the front one brake suddenly, set safety distance d,When <A B S L S d ++, or 22<t +d 254(f+i) 3.6254(f+i)A BB V V V L ++, vehicle B can‘t brakein time, A and B can‘t hold a safe distance, accident may happen. Otherwise they are safe.2.2.2.2 Overtaking phenomenonWhen a freeway is in medium density, which is the most common case, overtaking often happens, in order to get rid of the limit of the slower vehicle in front of it. If an overtaking is successfully completed, the biggest driving satisfaction will be achieved. On freeway, overtaking will increase the traffic flow more or less, which is the main difference between single lane and two lanes. However, overtaking is a relatively dangerous behavior, we must make sure that we can safely finish a overtaking. This process is shown in figure 12.Fig 12. Overtaking phenomenon processVehicle P wants to overtake because there‘s a vehicle C from which P will be a dangerous distance away.We can put the overtaking process down into two lane changing process, which is the key to the analysis of overtaking.In the first lane changing process, the following inequalities must be satisfied:L1≤d;L2≤d; (18)L3≤d;In the second lane changing process, with the same reason, the following inequalities must be satisfied:L‖1≤d;L‖2≤d;L‖3≤d; (19)L‖4≤d;The L‖1, L‖2 , L‖3and L‖4 can be get by the following equations:L‖1=L1+V P t-V A t;L‖2= L2+V B t-V P t;L‖3=V P t-V C t-L3; (20)L‖4= L4+V D t-V P t.Here:L1 /L2/ L3/L4—the distance between A/B/C/D and P;d--safe distance;V A/V B/V C/V D/V P--the speed of vehicle A/B/C/D/P;t--total overtaking time.Now we can use these inequalities and equations to judge whether an overtaking is safe or not in theory.2.2.3Speed limitIn this case, the vehicles must run at a moderate speed, if an vehicle runs too fast, the risk of rear-ending will increase; if an vehicle runs too slow, it will increase the number of overtaking phenomenon per unit time per unit length thus security cannot be guaranteed.2.2.4 Overtaking ratio limitBecause of the vehicles around, the overtaking ratio can‘t be too low; the overtaking conditio n satisfied doesn‘t mean the happening of a successful overtaking, we must be aware that there‘s selective overtaking, that is, drivers may not overtake even if the safe condition has been ensured.2.3 Very heavy traffic loadWhen the freeway is under so heavy load that there‘s few overtaking behavior, the main factor that may limit the traffic flow is the overall movement speed. The performance on the freeway is mainly following, and the intervals are relatively very small.2.3.1 Safety factors analysis。
美赛数学的建模比赛论文实用模板
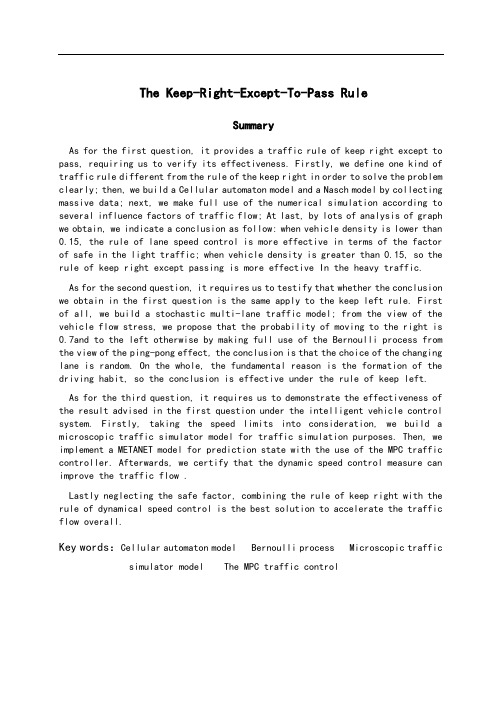
The Keep-Right-Except-To-Pass RuleSummaryAs for the first question, it provides a traffic rule of keep right except to pass, requiring us to verify its effectiveness. Firstly, we define one kind of traffic rule different from the rule of the keep right in order to solve the problem clearly; then, we build a Cellular automaton model and a Nasch model by collecting massive data; next, we make full use of the numerical simulation according to several influence factors of traffic flow; At last, by lots of analysis of graph we obtain, we indicate a conclusion as follow: when vehicle density is lower than 0.15, the rule of lane speed control is more effective in terms of the factor of safe in the light traffic; when vehicle density is greater than 0.15, so the rule of keep right except passing is more effective In the heavy traffic.As for the second question, it requires us to testify that whether the conclusion we obtain in the first question is the same apply to the keep left rule. First of all, we build a stochastic multi-lane traffic model; from the view of the vehicle flow stress, we propose that the probability of moving to the right is 0.7and to the left otherwise by making full use of the Bernoulli process from the view of the ping-pong effect, the conclusion is that the choice of the changing lane is random. On the whole, the fundamental reason is the formation of the driving habit, so the conclusion is effective under the rule of keep left.As for the third question, it requires us to demonstrate the effectiveness of the result advised in the first question under the intelligent vehicle control system. Firstly, taking the speed limits into consideration, we build a microscopic traffic simulator model for traffic simulation purposes. Then, we implement a METANET model for prediction state with the use of the MPC traffic controller. Afterwards, we certify that the dynamic speed control measure can improve the traffic flow .Lastly neglecting the safe factor, combining the rule of keep right with the rule of dynamical speed control is the best solution to accelerate the traffic flow overall.Key words:Cellular automaton model Bernoulli process Microscopic traffic simulator model The MPC traffic controlContentContent (2)1. Introduction (3)2. Analysis of the problem (3)3. Assumption (3)4. Symbol Definition (3)5. Models (4)5.1 Building of the Cellular automaton model (4)5.1.1 Verify the effectiveness of the keep right except to pass rule (4)5.1.2 Numerical simulation results and discussion (5)5.1.3 Conclusion (8)5.2 The solving of second question (8)5.2.1 The building of the stochastic multi-lane traffic model (9)5.2.2 Conclusion (9)5.3 Taking the an intelligent vehicle system into a account (9)5.3.1 Introduction of the Intelligent Vehicle Highway Systems (9)5.3.2 Control problem (9)5.3.3 Results and analysis (9)5.3.4 The comprehensive analysis of the result (10)6. Improvement of the model (11)6.1 strength and weakness (11)6.1.1 Strength (11)6.1.2 Weakness (11)6.2 Improvement of the model (11)7. Reference (13)1. IntroductionAs is known to all, it’s essential for us to drive automobiles, thus the driving rules is crucial important. In many countries like USA, China, drivers obey the rules which called “The Keep-Right-Except-To-Pass (that is, when driving automobiles, the rule requires drivers to drive in the right-most unless theyare passing another vehicle)”.2. Analysis of the problemFor the first question, we decide to use the Cellular automaton to build models,then analyze the performance of this rule in light and heavy traffic. Firstly,we mainly use the vehicle density to distinguish the light and heavy traffic; secondly, we consider the traffic flow and safe as the represent variable which denotes the light or heavy traffic; thirdly, we build and analyze a Cellular automaton model; finally, we judge the rule through two different driving rules,and then draw conclusions.3. AssumptionIn order to streamline our model we have made several key assumptions●The highway of double row three lanes that we study can representmulti-lane freeways.●The data that we refer to has certain representativeness and descriptive●Operation condition of the highway not be influenced by blizzard oraccidental factors●Ignore the driver's own abnormal factors, such as drunk driving andfatigue driving●The operation form of highway intelligent system that our analysis canreflect intelligent system●In the intelligent vehicle system, the result of the sampling data hashigh accuracy.4. Symbol Definitioni The number of vehiclest The time5. ModelsBy analyzing the problem, we decided to propose a solution with building a cellular automaton model.5.1 Building of the Cellular automaton modelThanks to its simple rules and convenience for computer simulation, cellular automaton model has been widely used in the study of traffic flow in recent years. Let )(t x i be the position of vehicle i at time t , )(t v i be the speed of vehicle i at time t , p be the random slowing down probability, and R be the proportion of trucks and buses, the distance between vehicle i and the front vehicle at time t is:1)()(1--=-t x t x gap i i i , if the front vehicle is a small vehicle.3)()(1--=-t x t x gap i i i , if the front vehicle is a truck or bus.5.1.1 Verify the effectiveness of the keep right except to pass ruleIn addition, according to the keep right except to pass rule, we define a new rule called: Control rules based on lane speed. The concrete explanation of the new rule as follow:There is no special passing lane under this rule. The speed of the first lane (the far left lane) is 120–100km/h (including 100 km/h);the speed of the second lane (the middle lane) is 100–80km8/h (including80km/h);the speed of the third lane (the far right lane) is below 80km/ h. The speeds of lanes decrease from left to right.● Lane changing rules based lane speed controlIf vehicle on the high-speed lane meets control v v <, ),1)(min()(max v t v t gap i f i +≥, safe b i gap t gap ≥)(, the vehicle will turn into the adjacent right lane, and the speed of the vehicle after lane changing remains unchanged, where control v is the minimum speed of the corresponding lane.● The application of the Nasch model evolutionLet d P be the lane changing probability (taking into account the actual situation that some drivers like driving in a certain lane, and will not takethe initiative to change lanes), )(t gap f i indicates the distance between the vehicle and the nearest front vehicle, )(t gap b i indicates the distance between the vehicle and the nearest following vehicle. In this article, we assume that the minimum safe distance gap safe of lane changing equals to the maximum speed of the following vehicle in the adjacent lanes.Lane changing rules based on keeping right except to passIn general, traffic flow going through a passing zone (Fig. 5.1.1) involves three processes: the diverging process (one traffic flow diverging into two flows), interacting process (interacting between the two flows), and merging process (the two flows merging into one) [4].Fig.5.1.1 Control plan of overtaking process(1) If vehicle on the first lane (passing lane) meets ),1)(min()(max v t v t gap i f i +≥ and safe b i gap t gap ≥)(, the vehicle will turn into the second lane, the speed of the vehicle after lane changing remains unchanged.5.1.2 Numerical simulation results and discussionIn order to facilitate the subsequent discussions, we define the space occupation rate as L N N p truck CAR ⨯⨯+=3/)3(, where CAR N indicates the number ofsmall vehicles on the driveway,truck N indicates the number of trucks and buses on the driveway, and L indicates the total length of the road. The vehicle flow volume Q is the number of vehicles passing a fixed point per unit time,T N Q T /=, where T N is the number of vehicles observed in time duration T .The average speed ∑∑⨯=T it i a v T N V 11)/1(, t i v is the speed of vehicle i at time t . Take overtaking ratio f p as the evaluation indicator of the safety of traffic flow, which is the ratio of the total number of overtaking and the number of vehicles observed. After 20,000 evolution steps, and averaging the last 2000 steps based on time, we have obtained the following experimental results. In order to eliminate the effect of randomicity, we take the systemic average of 20 samples [5].Overtaking ratio of different control rule conditionsBecause different control conditions of road will produce different overtaking ratio, so we first observe relationships among vehicle density, proportion of large vehicles and overtaking ratio under different control conditions.(a) Based on passing lane control (b) Based on speed control Fig.5.1.3Fig.5.1.3 Relationships among vehicle density, proportion of large vehicles and overtaking ratio under different control conditions.It can be seen from Fig. 5.1.3:(1) when the vehicle density is less than 0.05, the overtaking ratio will continue to rise with the increase of vehicle density; when the vehicle density is larger than 0.05, the overtaking ratio will decrease with the increase of vehicle density; when density is greater than 0.12, due to the crowding, it willbecome difficult to overtake, so the overtaking ratio is almost 0.(2) when the proportion of large vehicles is less than 0.5, the overtaking ratio will rise with the increase of large vehicles; when the proportion of large vehicles is about 0.5, the overtaking ratio will reach its peak value; when the proportion of large vehicles is larger than 0.5, the overtaking ratio will decrease with the increase of large vehicles, especially under lane-based control condition s the decline is very clear.● Concrete impact of under different control rules on overtaking ratioFig.5.1.4Fig.5.1.4 Relationships among vehicle density, proportion of large vehicles and overtaking ratio under different control conditions. (Figures in left-hand indicate the passing lane control, figures in right-hand indicate the speed control. 1f P is the overtaking ratio of small vehicles over large vehicles, 2f P is the overtaking ratio of small vehicles over small vehicles, 3f P is the overtaking ratio of large vehicles over small vehicles, 4f P is the overtaking ratio of large vehicles over large vehicles.). It can be seen from Fig. 5.1.4:(1) The overtaking ratio of small vehicles over large vehicles under passing lane control is much higher than that under speed control condition, which is because, under passing lane control condition, high-speed small vehicles have to surpass low-speed large vehicles by the passing lane, while under speed control condition, small vehicles are designed to travel on the high-speed lane, there is no low- speed vehicle in front, thus there is no need to overtake.● Impact of different control rules on vehicle speedFig. 5.1.5 Relationships among vehicle density, proportion of large vehicles and average speed under different control conditions. (Figures in left-hand indicates passing lane control, figures in right-hand indicates speed control.a X is the average speed of all the vehicles, 1a X is the average speed of all the small vehicles, 2a X is the average speed of all the buses and trucks.).It can be seen from Fig. 5.1.5:(1) The average speed will reduce with the increase of vehicle density and proportion of large vehicles.(2) When vehicle density is less than 0.15,a X ,1a X and 2a X are almost the same under both control conditions.Effect of different control conditions on traffic flowFig.5.1.6Fig. 5.1.6 Relationships among vehicle density, proportion of large vehicles and traffic flow under different control conditions. (Figure a1 indicates passing lane control, figure a2 indicates speed control, and figure b indicates the traffic flow difference between the two conditions.It can be seen from Fig. 5.1.6:(1) When vehicle density is lower than 0.15 and the proportion of large vehicles is from 0.4 to 1, the traffic flow of the two control conditions are basically the same.(2) Except that, the traffic flow under passing lane control condition is slightly larger than that of speed control condition.5.1.3 ConclusionIn this paper, we have established three-lane model of different control conditions, studied the overtaking ratio, speed and traffic flow under different control conditions, vehicle density and proportion of large vehicles.5.2 The solving of second question5.2.1 The building of the stochastic multi-lane traffic model5.2.2 ConclusionOn one hand, from the analysis of the model, in the case the stress is positive, we also consider the jam situation while making the decision. More specifically, if a driver is in a jam situation, applying ))(,2(x P B R results with a tendency of moving to the right lane for this driver. However in reality, drivers tend to find an emptier lane in a jam situation. For this reason, we apply a Bernoulli process )7.0,2(B where the probability of moving to the right is 0.7and to the left otherwise, and the conclusion is under the rule of keep left except to pass, So, the fundamental reason is the formation of the driving habit.5.3 Taking the an intelligent vehicle system into a accountFor the third question, if vehicle transportation on the same roadway was fully under the control of an intelligent system, we make some improvements for the solution proposed by us to perfect the performance of the freeway by lots of analysis.5.3.1 Introduction of the Intelligent Vehicle Highway SystemsWe will use the microscopic traffic simulator model for traffic simulation purposes. The MPC traffic controller that is implemented in the Matlab needs a traffic model to predict the states when the speed limits are applied in Fig.5.3.1. We implement a METANET model for prediction purpose[14].5.3.2 Control problemAs a constraint, the dynamic speed limits are given a maximum and minimum allowed value. The upper bound for the speed limits is 120 km/h, and the lower bound value is 40 km/h. For the calculation of the optimal control values, all speed limits are constrained to this range. When the optimal values are found, they are rounded to a multiplicity of 10 km/h, since this is more clear for human drivers, and also technically feasible without large investments.5.3.3 Results and analysisWhen the density is high, it is more difficult to control the traffic, since the mean speed might already be below the control speed. Therefore, simulations are done using densities at which the shock wave can dissolve without using control, and at densities where the shock wave remains. For each scenario, five simulations for three different cases are done, each with a duration of one hour. The results of the simulations are reported in Table 5.1, 5.2, 5.3. Table.5.1 measured results for the unenforced speed limit scenariodem q case#1 #2 #3 #4 #5 TTS:mean(std ) TPN 4700no shock 494.7452.1435.9414.8428.3445.21(6.9%) 5:4wave 3 5 8 8 0 14700nocontrolled520.42517.48536.13475.98539.58517.92(4.9%)6:364700 controlled 513.45488.43521.35479.75-486.5500.75(4.0%)6:244700 no shockwave493.9472.6492.78521.1489.43493.96(3.5%)6:034700 uncontrolled635.1584.92643.72571.85588.63604.84(5.3%)7:244700 controlled 575.3654.12589.77572.15586.46597.84(6.4%)7:19●Enforced speed limits●Intelligent speed adaptationFor the ISA scenario, the desired free-flow speed is about 100% of the speed limit. The desired free-flow speed is modeled as a Gaussian distribution, with a mean value of 100% of the speed limit, and a standard deviation of 5% of the speed limit. Based on this percentage, the influence of the dynamic speed limits is expected to be good[19].5.3.4 The comprehensive analysis of the resultFrom the analysis above, we indicate that adopting the intelligent speed control system can effectively decrease the travel times under the control of an intelligent system, in other words, the measures of dynamic speed control can improve the traffic flow.Evidently, under the intelligent speed control system, the effect of the dynamic speed control measure is better than that under the lane speed control mentioned in the first problem. Because of the application of the intelligent speed control system, it can provide the optimal speed limit in time. In addition, it can guarantee the safe condition with all kinds of detection device and the sensor under the intelligent speed system.On the whole, taking all the analysis from the first problem to the end into a account, when it is in light traffic, we can neglect the factor of safe with the help of the intelligent speed control system.Thus, under the state of the light traffic, we propose a new conclusion different from that in the first problem: the rule of keep right except to pass is more effective than that of lane speed control.And when it is in the heavy traffic, for sparing no effort to improve the operation efficiency of the freeway, we combine the dynamical speed control measure with the rule of keep right except to pass, drawing a conclusion that the application of the dynamical speed control can improve the performance ofthe freeway.What we should highlight is that we can make some different speed limit as for different section of road or different size of vehicle with the application of the Intelligent Vehicle Highway Systems.In fact, that how the freeway traffic operate is extremely complex, thereby, with the application of the Intelligent Vehicle Highway Systems, by adjusting our solution originally, we make it still effective to freeway traffic.6. Improvement of the model6.1 strength and weakness6.1.1 Strength●it is easy for computer simulating and can be modified flexibly to consideractual traffic conditions ,moreover a large number of images make the model more visual.●The result is effectively achieved all of the goals we set initially, meantimethe conclusion is more persuasive because of we used the Bernoulli equation.●We can get more accurate result as we apply Matlab.6.1.2 Weakness●The relationship between traffic flow and safety is not comprehensivelyanalysis.●Due to there are many traffic factors, we are only studied some of the factors,thus our model need further improved.6.2 Improvement of the modelWhile we compare models under two kinds of traffic rules, thereby we come to the efficiency of driving on the right to improve traffic flow in some circumstance. Due to the rules of comparing is too less, the conclusion is inadequate. In order to improve the accuracy, We further put forward a kinds of traffic rules: speed limit on different type of cars.The possibility of happening traffic accident for some vehicles is larger, and it also brings hidden safe troubles. So we need to consider separately about different or specific vehicle types from the angle of the speed limiting in order to reduce the occurrence of traffic accidents, the highway speed limit signs is in Fig.6.1.Fig .6.1Advantages of the improving model are that it is useful to improve the running condition safety of specific type of vehicle while considering the difference of different types of vehicles. However, we found that the rules may be reduce the road traffic flow through the analysis. In the implementation it should be at the 85V speed of each model as the main reference basis. In recent years, the 85V of some researchers for the typical countries from Table 6.1[ 21]: Table 6.1 Operating speed prediction modeAuthorCountry Model Ottesen andKrammes2000America LC DC L DC V C ⨯---=01.0012.057.144.10285Andueza2000Venezuel a ].[308.9486.7)/894()/2795(25.9885curve horizontal L DC Ra R V T ++--= ].[tan 819.27)/3032(69.10085gent L R V T +-= Jessen2001 America ][00239.0614.0279.080.86185LSD ADT G V V P --+=][00212.0432.010.7285NLSD ADT V V P -+=Donnell2001 America 22)2(8500724.040.10140.04.78T L G R V --+=22)3(85008369.048.10176.01.75T L G R V --+= 22)4(8500810.069.10176.05.74T L G R V --+=22)5(8500934.008.21.83T L G V --=BucchiA.BiasuzziK.And SimoneA.2005Italy DC V 124.0164.6685-= DC E V 4.046.3366.5585--= 2855.035.1119.0745.65DC E DC V ---= Fitzpatrick America KV 98.17507.11185-= Meanwhile, there are other vehicles driving rules such as speed limit in adverseweather conditions. This rule can improve the safety factor of the vehicle to some extent. At the same time, it limits the speed at the different levels.7. Reference[1] M. Rickert, K. Nagel, M. Schreckenberg, A. Latour, Two lane traffi csimulations using cellular automata, Physica A 231 (1996) 534–550.[20] J.T. Fokkema, Lakshmi Dhevi, Tamil Nadu Traffi c Management and Control inIntelligent Vehicle Highway Systems,18(2009).[21] Yang Li, New Variable Speed Control Approach for Freeway. (2011) 1-66。
美赛数学建模A题翻译版论文
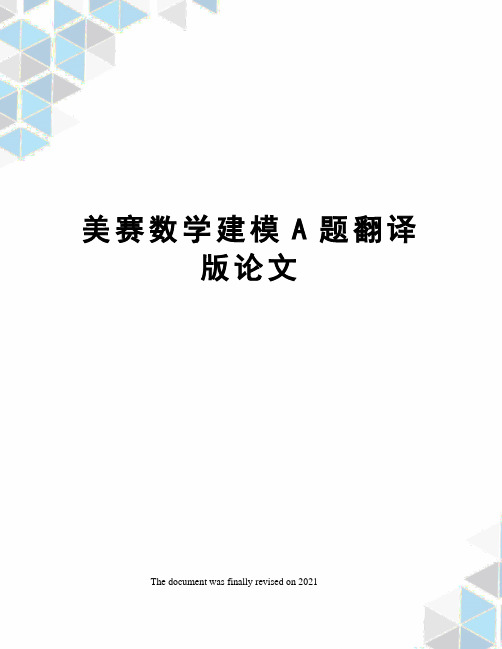
美赛数学建模A题翻译版论文The document was finally revised on 2021数学建模竞赛(MCM / ICM)汇总表基于细胞的高速公路交通模型自动机和蒙特卡罗方法总结基于元胞自动机和蒙特卡罗方法,我们建立一个模型来讨论“靠右行”规则的影响。
首先,我们打破汽车的运动过程和建立相应的子模型car-generation的流入模型,对于匀速行驶车辆,我们建立一个跟随模型,和超车模型。
然后我们设计规则来模拟车辆的运动模型。
我们进一步讨论我们的模型规则适应靠右的情况和,不受限制的情况, 和交通情况由智能控制系统的情况。
我们也设计一个道路的危险指数评价公式。
我们模拟双车道高速公路上交通(每个方向两个车道,一共四条车道),高速公路双向三车道(总共6车道)。
通过计算机和分析数据。
我们记录的平均速度,超车取代率、道路密度和危险指数和通过与不受规则限制的比较评估靠右行的性能。
我们利用不同的速度限制分析模型的敏感性和看到不同的限速的影响。
左手交通也进行了讨论。
根据我们的分析,我们提出一个新规则结合两个现有的规则(靠右的规则和无限制的规则)的智能系统来实现更好的的性能。
1介绍术语假设2模型设计的元胞自动机流入模型跟随模型超车模型超车概率超车条件危险指数两套规则CA模型靠右行无限制行驶规则3补充分析模型加速和减速概率分布的设计设计来避免碰撞4模型实现与计算机5数据分析和模型验证平均速度快车的平均速度密度超车几率危险指数6在不同速度限制下敏感性评价模型7驾驶在左边8交通智能系统智能系统的新规则模型的适应度智能系统结果9结论10优点和缺点优势弱点引用附录。
1 Introduction今天,大约65%的世界人口生活在右手交通的国家和35%在左手交通的国家交通流量。
[worldstandards。
欧盟,2013] 右手交通的国家,比如美国和中国,法规要求驾驶在靠路的右边行走。
多车道高速公路在这些国家经常使用一个规则,要求司机在最右边开车除非他们超过另一辆车,在这种情况下,他们移动到左边的车道、通过,返回到原来的车道。
环岛交通优化设计数学建模
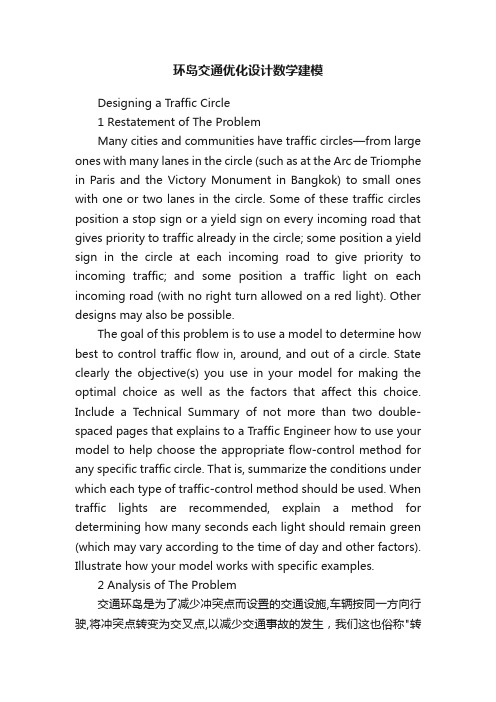
环岛交通优化设计数学建模Designing a Traffic Circle1 Restatement of The ProblemMany cities and communities have traffic circles—from large ones with many lanes in the circle (such as at the Arc de Triomphe in Paris and the Victory Monument in Bangkok) to small ones with one or two lanes in the circle. Some of these traffic circles position a stop sign or a yield sign on every incoming road that gives priority to traffic already in the circle; some position a yield sign in the circle at each incoming road to give priority to incoming traffic; and some position a traffic light on each incoming road (with no right turn allowed on a red light). Other designs may also be possible.The goal of this problem is to use a model to determine how best to control traffic flow in, around, and out of a circle. State clearly the objective(s) you use in your model for making the optimal choice as well as the factors that affect this choice. Include a Technical Summary of not more than two double-spaced pages that explains to a Traffic Engineer how to use your model to help choose the appropriate flow-control method for any specific traffic circle. That is, summarize the conditions under which each type of traffic-control method should be used. When traffic lights are recommended, explain a method for determining how many seconds each light should remain green (which may vary according to the time of day and other factors). Illustrate how your model works with specific examples.2 Analysis of The Problem交通环岛是为了减少冲突点而设置的交通设施,车辆按同一方向行驶,将冲突点转变为交叉点,以减少交通事故的发生,我们这也俗称"转盘"。
车道被占用对城市道路通行能力的影响_数学建模大赛a题优秀论文
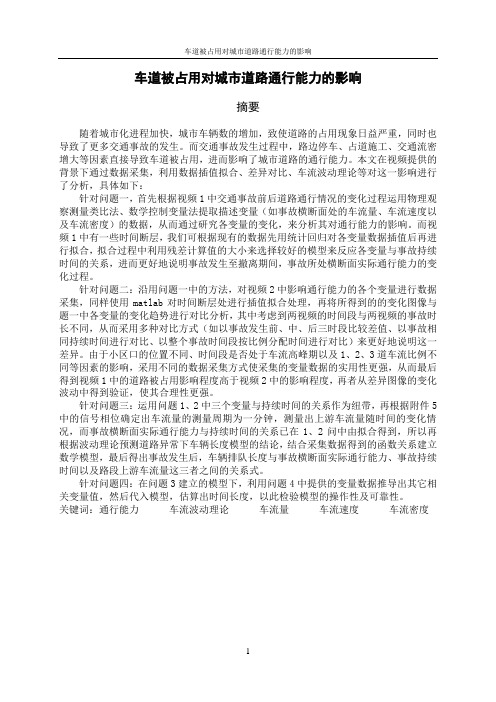
车道被占用对城市道路通行能力的影响摘要随着城市化进程加快,城市车辆数的增加,致使道路的占用现象日益严重,同时也导致了更多交通事故的发生。
而交通事故发生过程中,路边停车、占道施工、交通流密增大等因素直接导致车道被占用,进而影响了城市道路的通行能力。
本文在视频提供的背景下通过数据采集,利用数据插值拟合、差异对比、车流波动理论等对这一影响进行了分析,具体如下:针对问题一,首先根据视频1中交通事故前后道路通行情况的变化过程运用物理观察测量类比法、数学控制变量法提取描述变量(如事故横断面处的车流量、车流速度以及车流密度)的数据,从而通过研究各变量的变化,来分析其对通行能力的影响。
而视频1中有一些时间断层,我们可根据现有的数据先用统计回归对各变量数据插值后再进行拟合,拟合过程中利用残差计算值的大小来选择较好的模型来反应各变量与事故持续时间的关系,进而更好地说明事故发生至撤离期间,事故所处横断面实际通行能力的变化过程。
针对问题二:沿用问题一中的方法,对视频2中影响通行能力的各个变量进行数据采集,同样使用matlab对时间断层处进行插值拟合处理,再将所得到的的变化图像与题一中各变量的变化趋势进行对比分析,其中考虑到两视频的时间段与两视频的事故时长不同,从而采用多种对比方式(如以事故发生前、中、后三时段比较差值、以事故相同持续时间进行对比、以整个事故时间段按比例分配时间进行对比)来更好地说明这一差异。
由于小区口的位置不同、时间段是否处于车流高峰期以及1、2、3道车流比例不同等因素的影响,采用不同的数据采集方式使采集的变量数据的实用性更强,从而最后得到视频1中的道路被占用影响程度高于视频2中的影响程度,再者从差异图像的变化波动中得到验证,使其合理性更强。
针对问题三:运用问题1、2中三个变量与持续时间的关系作为纽带,再根据附件5中的信号相位确定出车流量的测量周期为一分钟,测量出上游车流量随时间的变化情况,而事故横断面实际通行能力与持续时间的关系已在1、2问中由拟合得到,所以再根据波动理论预测道路异常下车辆长度模型的结论,结合采集数据得到的函数关系建立数学模型,最后得出事故发生后,车辆排队长度与事故横断面实际通行能力、事故持续时间以及路段上游车流量这三者之间的关系式。
2104数学建模美赛A 交通流 安全性 参考资料 交通量
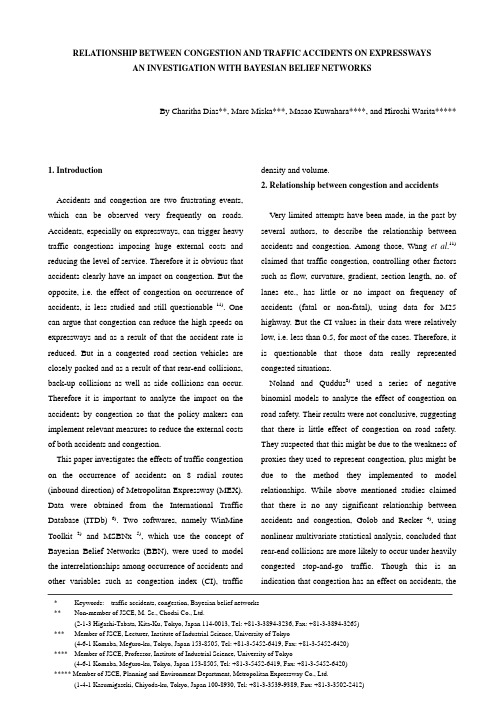
RELATIONSHIP BETWEEN CONGESTION AND TRAFFIC ACCIDENTS ON EXPRESSWAYS AN INVESTIGATION WITH BAYESIAN BELIEF NETWORKSBy Charitha Dias**, Marc Miska***, Masao Kuwahara****, and Hiroshi Warita*****1. IntroductionAccidents and congestion are two frustrating events, which can be observed very frequently on roads. Accidents, especially on expressways, can trigger heavy traffic congestions imposing huge external costs and reducing the level of service. Therefore it is obvious that accidents clearly have an impact on congestion. But the opposite, i.e. the effect of congestion on occurrence of accidents, is less studied and still questionable 11). One can argue that congestion can reduce the high speeds on expressways and as a result of that the accident rate is reduced. But in a congested road section vehicles are closely packed and as a result of that rear-end collisions, back-up collisions as well as side collisions can occur. Therefore it is important to analyze the impact on the accidents by congestion so that the policy makers can implement relevant measures to reduce the external costs of both accidents and congestion.This paper investigates the effects of traffic congestion on the occurrence of accidents on 8 radial routes (inbound direction) of Metropolitan Expressway (MEX). Data were obtained from the International Traffic Database (ITDb) 6). Two softwares, namely WinMine Toolkit 2) and MSBNx 5), which use the concept of Bayesian Belief Networks (BBN), were used to model the interrelationships among occurrence of accidents and other variables such as congestion index (CI), traffic density and volume.2. Relationship between congestion and accidentsVery limited attempts have been made, in the past by several authors, to describe the relationship between accidents and congestion. Among those, Wang et al.11) claimed that traffic congestion, controlling other factors such as flow, curvature, gradient, section length, no. of lanes etc., has little or no impact on frequency of accidents (fatal or non-fatal), using data for M25 highway. But the CI values in their data were relatively low, i.e. less than 0.5, for most of the cases. Therefore, it is questionable that those data really represented congested situations.Noland and Quddus8) used a series of negative binomial models to analyze the effect of congestion on road safety. Their results were not conclusive, suggesting that there is little effect of congestion on road safety. They suspected that this might be due to the weakness of proxies they used to represent congestion, plus might be due to the method they implemented to model relationships. While above mentioned studies claimed that there is no any significant relationship between accidents and congestion, Golob and Recker 4), using nonlinear multivariate statistical analysis, concluded that rear-end collisions are more likely to occur under heavily congested stop-and-go traffic. Though this is an indication that congestion has an effect on accidents, the* Keywords: traffic accidents, congestion, Bayesian belief networks** Non-member of JSCE, M. Sc., Chodai Co., Ltd.(2-1-3 Higashi-Tabata, Kita-Ku, Tokyo, Japan 114-0013, Tel: +81-3-3894-3236, Fax: +81-3-3894-3265) *** Member of JSCE, Lecturer, Institute of Industrial Science, University of Tokyo(4-6-1 Komaba, Meguro-ku, Tokyo, Japan 153-8505, Tel: +81-3-5452-6419, Fax: +81-3-5452-6420)**** Member of JSCE, Professor, Institute of Industrial Science, University of Tokyo(4-6-1 Komaba, Meguro-ku, Tokyo, Japan 153-8505, Tel: +81-3-5452-6419, Fax: +81-3-5452-6420)***** Member of JSCE, Planning and Environment Department, Metropolitan Expressway Co., Ltd.(1-4-1 Kasumigaseki, Chiyoda-ku, Tokyo, Japan 100-8930, Tel: +81-3-3539-9389, Fax: +81-3-3502-2412)link between accidents and congestion has not elaborated clearly.Though some other authors have attempted to explore the relationship between accidents and traffic volume or flow 1)3), these studies may not describe the relationship between accidents and congestion, because according to speed-flow characteristics not only flow but also speeds are also needed to determine whether the prevailing traffic condition is congested or un-congested.These limited previous studies suggest us the need for more studies to better understand the impact of congestion on accidents. In this study BBNs, which can model and graphically interpret the relationships in any system, were used to model the interrelationships among CI, volume, density and occurrence of accidents.3. Methodology(1) Data descriptionFive-minutes aggregated volume, average speed, occupancy and incident data on inbound direction of 8 radial routes (Route numbers 1, 2, 3, 4, 5, 6, 7 and 9) of the MEX, collected over one week were used. One-week data (from 5th June 2006 to 11th June 2006) of ITDb on MEX is free for public and that free data was used for this analysis. Format of raw data is shown in Figure 2.(2) Data arrangementa) Pre-accident period.First the other incidents such as car breakdowns, road works etc. were removed and the 15 minutes time period before an accident was defined as pre-accident period (Figure 2). Here, 15 minutes was selected just to represent the average traffic conditions on the road section before an accident. b) Congestion Index (CI)Congestion index expresses the congestion level of a given road section relative to the free-flow conditions. It is non-negative and dimensionless value. Average speed data was converted in to congestion data, i.e. congestion indexes (CI), with slightly modified version of Taylor’s 9) method, as follows.{tionroad the for speed flow Free tionroad the for speed Average Where ifand if CI VV VV V VV V V V FFFFFF FFFFFF sec sec ;;0;/)(−−>>≤−=Figure 1: Metropolitan expressway (MEX)networkc) V olume classes, CI classes and density classes V olumes, CI’s and occupancies were categorized in to classes as shown in Table 1. This was done because categorized data were needed to learn BBNs.Table 1: Categorized volumes, CIs and densitiesFigure 2: Available data(3) Bayesian belief Networks (BBN)BBNs are probabilistic graphical models that represent a set of variables and their conditional independencies via a directed acyclic graph 12). BBN is an effective technique to understand the relationships among variables because BBNs can model the interrelationships among variables with their conditional probabilities in any kind of a system and represent them graphically.Two applications namely WinMine Toolkit2) and MSBNx5), which use the BBN concept, were used to identify conditional independencies among variables and to perform inference, respectively. WinMine toolkit is set of tools, designed for Windows environment, that allow constructing statistical models from categorized data. WinMine has many advantages compared with other traditional methods such as bar graphs, contingency tables and odd ratios to mine for information in data because it can provide a better picture of the factor interrelationships among variables 7). MSBNx is a component-based application, which can be used to create, assess and evaluate Bayesian networks 5). It is an excellent tool for inference or updating probabilities. Categorized volume, CI, density and event for each route were fed in to WinMine toolkit separately in order to obtain the dependency network, which is very similar to Bayesian network. For inference purposes the conditional probabilities, determined with WinMine, were fed in to MSBNx. Figure 3 depicts dependency network along with the conditional probability table (CPT) for the node “Event”, constructed with WinMine, and Figure 4 depicts the BBN with un-conditional probability tables for all nodes, constructed with MSBNx, for the inbound direction of Route 4 of MEX.At a glance some important information can be drawn from these figures. Figure 3 describes the dependencies among the variables in data fed in to WinMine. Figure 4 shows how the inbound direction of Route 8 functions. 15% of the time, within the considered one week time period, it is congested or heavily congested, 76% of the time medium volumes and 81% of the times low densities could be observed. And there is around 1% of chance of an accident.Figure 3: Dependency network constructed with WinMineFigure 4: BBN constructed with MSBNxUn-conditional probabilities, which are shown in Figure 4, are probabilities before any evidence is observed and those are called prior probabilities. When evidence is observed, for example when it is known that the CI reflects heavily congested situation, probabilities for all other nodes can be updated and those updated probabilities are called posterior probabilities. And this process of updating probabilities is called inference. Inference was performed for BBNs for each considered routes with MSBNx and updated probabilities were noted. Results are described in Section 4.4. ResultsProbabilities of occurring a pre-accident period were plotted against congestion levels, as in Figure 5, and probability of occurring a pre-accident period was plotted against density levels, as in Figure 6. Figure 5clearly shows that the chance of an accident is increasedwith the congestion level.High density reflects high congestion levels. When occurrence of accidents is increased with the increase of density levels, as shown in Figure 6, it reflects that probability of accident occurrence is increased with congestion levels. Therefore both Figure 5 and Figure 6 describe that the accident occurrence is increased with congestion levels.Table 2 compares the congestion levels and density levels when a pre-accident condition is evidenced and non-pre-accident condition is evidenced.When a pre-accident situation is evidenced, the probability that the road section is in congested conditions is in the range of [60%, 87%]. And compared to that, when none (a non-pre-accident condition or any other incident) is observed the probability that the road section is congested is pretty low, i.e. in the range of [8%, 20%]. This reflects that when an accident occurred most of the time road section was congested.These results probably describe the drivers’ behavior at congested situations. On congested roads, where stop-and-go conditions are prevailed, frustrated drivers try to accelerate resulting rear-end collisions. And some drivers may try to change their lanes resulting side collisions plus back-up collisions. However, it should be kept in mind that fatalities are less in congested road sections compare to un-congested sections where operating speeds are high 10).Figure 5: Probability of observing a pre-accidentcondition given the congestion level5. Summary(1) ConclusionsFigure 6: Probability of observing a pre-accidentcondition given the density levelFlow, speed, occupancy and event data on inbound direction of 8 radial routes of MEX, collected over one week period, were used to explore the impact of congestion on occurrence of accidents. BBNs were used to model the relationships among pre-accident conditions and other variables. It was found that when a road section is getting congested, i.e. when the CI is increased and density is increased, the chance for an accident could be increased. Analysis of accident types will more clearly provide the answer for why probability of accident occurrence increases with congestion level.(2) Further researchThese results may not totally describe the mechanism of occurrence of accidents and congestion on MEX. Broad analysis is needed, which considers all factors that may cause accidents and congestion such as weather, road geometry, driver characteristics etc., to get a betterTable 2: Conditional probabilities of CI and density ifpre-accident condition is observedpicture of pre-accident conditions.Although the probability of occurring a pre-accident situation is increased with the increase of congestion, accidents occur under congested situations are not fatal compared to accidents occur under high-speed situations. Here in this analysis, severity of accidents and types of accidents were not considered due to lack of data. Therefore, it is interesting to extend the analysis to consider the severity levels as well as types of accidents, too.6. AcknowledgementAll traffic measurement data for this study was provided by the International Traffic Database (ITDb). Data available to the public can be accessed through .References1) Ceder, A.: Relationships between road accidents and hourly traffic flow. II. Probabilistic approach, Accident Analysis and Prevention, 14 (1), pp.35–44, 1982.2) Chickering, D.: The WinMine Toolkit. Redmond, WA., Microsoft technical report no. MSR-TR- 2002-103, 2002.3) Dickerson, A., Peirson, J., Vickerman, R.: Road accidents and traffic flows: An econometric investigation, Economica, London School of Economics and Political Science, V ol. 67(265), pp. 101-21, 2000.4) Golob, T. F. and Recker, W. W.: Relationships Among Urban Freeway Accidents, Traffic Flow, Weather and Lighting Conditions, Institute of Transportation Studies, University of California, Berkeley, 2001.5) Kadie, C. M., Hovel, D., and Horvitz, H.: MSBNx: A Component-Centric Toolkit for Modeling and Inference with Bayesian Networks, Microsoft Research Technical Report MSR-TR-2001-67, 2001.6) Miska, M., et al.: The International Traffic Database Project, 14th World congress on intelligent transport systems, CD-ROM, 2007.7) Milburn, N. J. et al: Mining for information in accident data, Final report, Federal aviation administration, Civil Aerospace medical institute, Oklahoma city, 2006.8) Noland, R.B., Quddus, M.A.: Congestion and safety: a spatial analysis of London, Transportation Research Part A: Policy and Practice 39 (7-9), pp. 737-754, 2005.9) Taylor, M.A.P., Woolley, J.E., Zito, R.: Integration of the global positioning system and geographical information systems for traffic congestion studies. Transportation Research Part C: Emerging Technologies 8 (1-6), pp.257-285. 2000.10) Taylor, M. C.: The speeds of vehicles which are involved in fatal accidents, Traffic Engineering and Control 42(2), 2001.11) Wang, C., Quddus, M. A., Ison, S. G.: Impact of traffic congestion on road accidents: A spatial analysis of theM25motorway in England, Accident Analysis and Prevention, 2009.12) Wikipedia contributors: Bayesian network, Wikipedia, The Free Encyclopedia, /w/index.php?title=Bayesian_network&oldid=293220919 (accessed May 30, 2009).。
美国数学建模竞赛优秀论文阅读报告
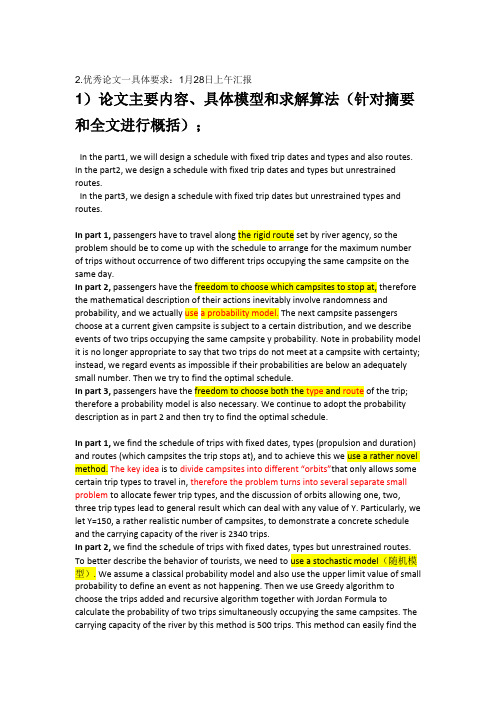
2.优秀论文一具体要求:1月28日上午汇报1)论文主要内容、具体模型和求解算法(针对摘要和全文进行概括);In the part1, we will design a schedule with fixed trip dates and types and also routes. In the part2, we design a schedule with fixed trip dates and types but unrestrained routes.In the part3, we design a schedule with fixed trip dates but unrestrained types and routes.In part 1, passengers have to travel along the rigid route set by river agency, so the problem should be to come up with the schedule to arrange for the maximum number of trips without occurrence of two different trips occupying the same campsite on the same day.In part 2, passengers have the freedom to choose which campsites to stop at, therefore the mathematical description of their actions inevitably involve randomness and probability, and we actually use a probability model. The next campsite passengers choose at a current given campsite is subject to a certain distribution, and we describe events of two trips occupying the same campsite y probability. Note in probability model it is no longer appropriate to say that two trips do not meet at a campsite with certainty; instead, we regard events as impossible if their probabilities are below an adequately small number. Then we try to find the optimal schedule.In part 3, passengers have the freedom to choose both the type and route of the trip; therefore a probability model is also necessary. We continue to adopt the probability description as in part 2 and then try to find the optimal schedule.In part 1, we find the schedule of trips with fixed dates, types (propulsion and duration) and routes (which campsites the trip stops at), and to achieve this we use a rather novel method. The key idea is to divide campsites into different “orbits”that only allows some certain trip types to travel in, therefore the problem turns into several separate small problem to allocate fewer trip types, and the discussion of orbits allowing one, two, three trip types lead to general result which can deal with any value of Y. Particularly, we let Y=150, a rather realistic number of campsites, to demonstrate a concrete schedule and the carrying capacity of the river is 2340 trips.In part 2, we find the schedule of trips with fixed dates, types but unrestrained routes. To better describe the behavior of tourists, we need to use a stochastic model(随机模型). We assume a classical probability model and also use the upper limit value of small probability to define an event as not happening. Then we use Greedy algorithm to choose the trips added and recursive algorithm together with Jordan Formula to calculate the probability of two trips simultaneously occupying the same campsites. The carrying capacity of the river by this method is 500 trips. This method can easily find theoptimal schedule with X given trips, no matter these X trips are with fixed routes or not. In part 3, we find the optimal schedule of trips with fixed dates and unrestrained types and routes. This is based on the probability model developed in part 2 and we assign the choice of trip types of the tourists with a uniform distribution to describe their freedom to choose and obtain the results similar to part 2. The carrying capacity of the river by this method is 493 trips. Also this method can easily find the optimal schedule with X given trips, no matter these X trips are with fixed routes or not.2)论文结构概述(列出提纲,分析优缺点,自己安排的结构);1 Introduction2 Definitions3 Specific formulation of problem4 Assumptions5 Part 1 Best schedule of trips with fixed dates, types and also routes.5.1 Method5.1.1 Motivation and justification5.1.2 Key ideas5.2 Development of the model5.2.1Every campsite set for every single trip type5.2.2 Every campsite set for every multiple trip types5.2.3One campsite set for all trip types6 Part 2 Best schedule of trips with fixed dates and types, but unrestrained routes.6.1 Method6.1.1 Motivation and justification6.1.2 Key ideas6.2 Development of the model6.2.1 Calculation of p(T,x,t)6.2.2 Best schedule using Greedy algorithm6.2.3 Application to situation where X trips are given7 Part 3 Best schedule of trips with fixed dates, but unrestrained types and routes.7.1 Method7.1.1 Motivation and justification7.1.2 Key ideas7.2 Development of the model8 Testing of the model----Sensitivity analysis8.1Stability with varying trip types chosen in 68.2The sensitivity analysis of the assumption 4④8.3 The sensitivity analysis of the assumption 4⑥9 Evaluation of the model9.1 Strengths and weaknesses9.1.1 Strengths9.1.2 Weakness9.2 Further discussion10 Conclusions11 References12 Letter to the river managers3)论文中出现的好词好句(做好记录);用于问题的转化We regard the carrying capacity of the river as the maximum total number of trips available each year, hence turning the task of the river managers into looking for the best schedule itself.表明我们在文中所做的工作We have examined many policies for different river…..问题的分解We mainly divide the problem into three parts and come up with three different….对我们工作的要求:Given the above considerations, we want to find the optimal。
- 1、下载文档前请自行甄别文档内容的完整性,平台不提供额外的编辑、内容补充、找答案等附加服务。
- 2、"仅部分预览"的文档,不可在线预览部分如存在完整性等问题,可反馈申请退款(可完整预览的文档不适用该条件!)。
- 3、如文档侵犯您的权益,请联系客服反馈,我们会尽快为您处理(人工客服工作时间:9:00-18:30)。
摘 要一、本文主要有三个数学模型:1. 通过环岛的理想模型,分析推出计算环岛的最大交通能力;对比设置停让交通标志控制以及信号灯控制对环岛通行能力。
得出:当经过环岛的实际流量'Q <环岛的最大通行能力Z Q ,应用指示牌控制法较宜,做法是在交通环岛的各个进口处设置指示牌,并设置环岛内交通车流的方向指示牌。
当'Z Q Q ≥时,宜采用信号灯控制法,并采用指示牌控制法予以辅助。
信号控制的目的在于最大限度地提高交叉口的使用效率。
2. 引入精英蚂蚁寻优策略模型。
针对城市道路交叉口的交通流特性,对单路口交通信号多相位实时控制的模型和算法进行研究。
采用能随交通需求的变化而实时变化的加权系数,将交叉口3 个优化目标函数转化为单目标函数优化的问题。
为提高模型的计算速度以及降低交叉口信号机的单机计算量,采用蚂蚁算法中的精英蚂蚁寻优3. 策略求解模型。
模型的目标方程为:ﻩ 42421411(1(/))min (,)2(1.0)[(1.0/)]/[2(1.0)](2(/))1.1(1.0)0.9(1.0/)/(1.0)2(3600/)(/)i i i i i i i i i i i i i i i Zl c Z x c s y Y c x c y l c s y Y c x c y c Y x c s Q ===-=⋅-⋅-⋅-++⋅⋅-⋅⋅⋅---⋅⋅⋅+∑∑∑4. 基于精英蚂蚁寻优策略模型,对其进行优化得到理想状态下计算信号灯系统中各路口的绿灯时间的目标方程z max (,)[2(3600/)(/)]Q i i Z x c c Y x c s =⋅⋅⋅+∑,引入算例,将算例所提供的数据代入优化得到的模型,使用软件求解。
当通过交通工程师通过观察法得到平稳期、高峰期的Y,S,当预设C 值,即可通过上述计算方法获得最大的通行量的四个信号的绿灯时长配置。
该优化模型可以将其应用到交通环岛各路口红绿灯时长的控制,并用交通标志配合控制交通流量。
验证了模型及其求解算法是合理的和有效的。
二、据我国交通规则,转盘内车道的行驶方向为逆时针方向。
我们在转盘入口处设置红 ﻩ绿灯控制车流进出,其中当c =101s ,Y =0.68时达到控制最优,Z =6932/pcu h , ﻩ第1x 相位绿灯亮15s ,第2x 相位绿灯亮90s ,第3x 相位绿灯亮65s ,第4x 相位 绿灯亮90s 。
转盘内运动的最佳方法是各驶入环岛车道的车辆匀速行驶,右转行 ﻩﻩ驶车辆不参与环岛车道的交织,左转行驶车辆靠内行驶,直行车辆靠外行驶,且未ﻩ 进环岛车道的车辆须等候环岛车辆经过,才可进入环岛车道。
关键词:平面环形交叉、通行能力、精英蚂蚁寻优策略( )、多相位交通信号( )目录一、问题的重述ﻩ错误!未指定书签。
二、问题的分析.............................................................................. 错误!未指定书签。
三、模型假设.................................................................................. 错误!未定义书签。
四、符号系统.................................................................................. 错误!未定义书签。
五、模型的建立ﻩ错误!未指定书签。
5.1 环岛最大通行能力计算模型( 1)ﻩ错误!未定义书签。
5.2、环岛交通控制两模型通行能力的比较分析ﻩ错误!未定义书签。
5.2.1 指示牌控制通行能力的计算............................ 错误!未定义书签。
5.2.2、信号控制通行能力计算.................................. 错误!未指定书签。
5.3、环岛信号灯控制模型( 2).......................................... 错误!未定义书签。
5.3.1 模型建立ﻩ错误!未定义书签。
5.3.2 精英蚂蚁寻优策略ﻩ错误!未指定书签。
5.3.3算法流程图...................................................... 错误!未指定书签。
5.4基于通行量最大的优化模型(3)............................ 错误!未定义书签。
六、模型的评价与推广.................................................................. 错误!未定义书签。
6.1模型的推广.................................................................. 错误!未指定书签。
6.2 模型的评价.................................................................. 错误!未指定书签。
6.2.1 优点:ﻩ错误!未指定书签。
6.2.2缺点:................................................................ 错误!未定义书签。
参考文献:.............................................................................. 错误!未定义书签。
一、问题的重述在许多城市和社区都建立有交通环岛,既有多条行车道的大型环岛,又有一至两条行车道的小型环岛。
转盘入口处的控制交通的方法各有不同,如:有些环岛在进入口设有“停车”标志或者让行标志,其目的是给已驶入环岛的车辆提供行车优先权;而在一些环岛的进入口的逆向一侧设立的让行标志是为了向即将驶入环岛的车辆提供行车优先权;还有一些环岛会在入口处设立交通灯;也可能会有其他的设计方案。
这个问题的要求是设计一个环岛,需要建立一个数学模型来分析控制车流进出以及在转盘内运动的最佳方法,以保证交通顺畅,且在此基础上达到通行量最大,充分发挥环岛的作用,要求清楚地解释建立模型的目的和影响最佳选择的因素,如:环交处的非机动车及行人交通量、相交道路的数量、环岛的半径大小、最小车头时距[7]、环岛处在的地形以及环境等[8]。
需要对交通工程师解释如何运用自己的模型来在某些特定的转盘处选择车流控制方法,恰当地选择使用交通标志还是红绿灯,若需要红绿灯,则需说明每个红绿灯绿灯亮的时间,要求能够使用自己的模型解决实际案例,最后对所使用的模型给予适当推广并陈述此模型控制方法的优缺点。
二、问题的分析本题研究的是建立一个数学模型来分析控制车流进出以及在环岛转盘内运动的最佳方法。
然后通过实例验证模型的准确性。
环形交叉口具有车辆连续行驶,安全,不需要设置管理设施,避免停车,节省燃料,噪声低,污染小等优点。
但同时其通行能力小于设置了交通信号灯的路口,不适用于交通流量较大的路口。
采用现有交通控制系统的控制策略,利用系统最优为目标,使得总延误最小[1]的控制思想。
对于设计的交通转盘,尝试直接假定在环道上有单条的理想状态以及双车道以上车道条件下建立环形交叉口通行能力的计算公式,在更加符合实际交通状况条件下确定环交最大总通行能力。
先后对设置交通标识法以及设置交通信号灯法所处的环形路口的两种模式分别进行分析研究比较。
当所使用的是交通信号灯控制法时,运用精英蚂蚁寻优策略及其最优化模型,计算出每个路口信号灯绿灯的时间。
最后通过结果的分析并且结合所用模型的优缺点,对之提一些参考性建议。
三、模型假设1、所计算的交通路段路口转盘处的交通状况正常,经过的车辆性能处于优的状态,不发生车祸,抛锚,追尾等交通事故。
2、车辆进入环岛转盘的行驶速度均相等。
3、进入环岛转盘车道的车辆之间的距离满足最小安全间距,且各车距相等。
4、经过的车辆都严格按照指示牌以及信号灯的指示行驶,不违反交通规则。
进入环岛行驶的车辆都严格按照逆时针方向行驶,依次驶出环岛转盘。
5、假设环岛的半径在理想的范围内,所设计的环岛内车道数不小于三条。
6、所研究计算的环岛交通流量处于稳态。
四、符号系统Q :直行车道的通行能力;'l β:一条直、左车道中的左转车所占比例;s T :直行车连续通过停车线的最小车头时距;first t :绿灯亮后,第一辆车起动并通过停车线的时间;j Q :环行道上靠环岛处绕行车道的通行能力;h Q :单道环岛总的通行能力;Z Q :多道环交的总通行能力;A Q :直、左、右行车辆驶过A 点的通过量;R Q :各进口道右转交通量之和;'Q :环岛交通环形交叉口的实际交通流量;P :右转交通量占环交总交通量的百分比;l :环岛内交织段长度;i l :为交叉口第i 相位损失时间;β:车辆分布不均匀系数,可采用0. 75~0. 85;i x :交叉口第i 相位有效绿灯时间;i y :交叉口第i 相位交通流量与饱和流量之比;i h :停车次数;n :交叉口信号控制相位数;c :交叉口信号系统的周期;'c :某交叉口一个信号灯的周期1i K 、2i K 、3i K :可随交通需求的不同而实时变化的性能指标加权系数; i s :第 i 相位道路通行能力;五、模型的建立5.1 环岛最大通行能力计算模型( 1)环岛转盘亦称环形平面交叉口。
首先假定环交路面上仅有1 条车道,进入环形平面交叉口的车辆(包括直行s 、右转r 和左转l 的车辆) 必须依此顺序在这1 条车道上通过。
如图1, 所有通过B B - 断面的直、左、右行车辆都必须依次驶过A 点的通过量A Q 为111223A r s l s l l Q Q Q Q Q Q Q =+++++ﻩﻩﻩ ﻩ ﻩﻩ (1)式中: is Q ,ir Q , il Q 分别为第i 个进口道的直行、右转和左转交通量(i = 1, 2, 3, 4).图1 环交口通行能力计算图式假定二,各向左、右转车辆比例相等且各向流量相等, (1)式可写为: 232()A r s l s r l Q Q Q Q Q Q Q =++=++因为整个环岛总的通行能力h Q 为:4()h s r l Q Q Q Q =++而 4()22()h s r l A s r l Q Q Q Q Q Q Q Q ++==++,所以2h A Q Q = /pcu h 对于6路交叉也可得出同样结论。
当环道上的车道数不止1 条时(一般≥3条) , 右转弯车辆不参与交织路段的交织,即不通过图中的A 点, 则给出的环交总的通行能力为2Z A R Q Q Q =+ ﻩ ﻩﻩﻩ ﻩ ﻩ ﻩ (2)式中: R Q 为各进口道右转交通量之和。