Machine Learning- Fundamentals
机器学习领域的知名人物和论文
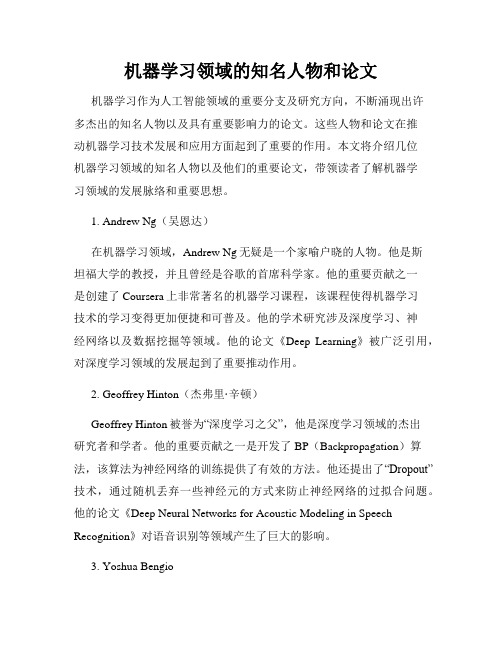
机器学习领域的知名人物和论文机器学习作为人工智能领域的重要分支及研究方向,不断涌现出许多杰出的知名人物以及具有重要影响力的论文。
这些人物和论文在推动机器学习技术发展和应用方面起到了重要的作用。
本文将介绍几位机器学习领域的知名人物以及他们的重要论文,带领读者了解机器学习领域的发展脉络和重要思想。
1. Andrew Ng(吴恩达)在机器学习领域,Andrew Ng无疑是一个家喻户晓的人物。
他是斯坦福大学的教授,并且曾经是谷歌的首席科学家。
他的重要贡献之一是创建了Coursera上非常著名的机器学习课程,该课程使得机器学习技术的学习变得更加便捷和可普及。
他的学术研究涉及深度学习、神经网络以及数据挖掘等领域。
他的论文《Deep Learning》被广泛引用,对深度学习领域的发展起到了重要推动作用。
2. Geoffrey Hinton(杰弗里·辛顿)Geoffrey Hinton被誉为“深度学习之父”,他是深度学习领域的杰出研究者和学者。
他的重要贡献之一是开发了BP(Backpropagation)算法,该算法为神经网络的训练提供了有效的方法。
他还提出了“Dropout”技术,通过随机丢弃一些神经元的方式来防止神经网络的过拟合问题。
他的论文《Deep Neural Networks for Acoustic Modeling in Speech Recognition》对语音识别等领域产生了巨大的影响。
3. Yoshua BengioYoshua Bengio是加拿大蒙特利尔大学教授,也是深度学习领域的重要人物之一。
他在深度学习领域的贡献源远流长。
他的论文《Learning Deep Architectures for AI》介绍了深度学习的概念和技术,并提出了一种深度置信网络(Deep Belief Networks)的训练方法。
这篇论文的发表引发了深度学习的研究和应用的热潮。
4. Ian GoodfellowIan Goodfellow是深度学习领域的年轻研究者,其主要贡献是提出了生成对抗网络(GAN)的概念。
周志华 机器学习ppt Chap01绪论
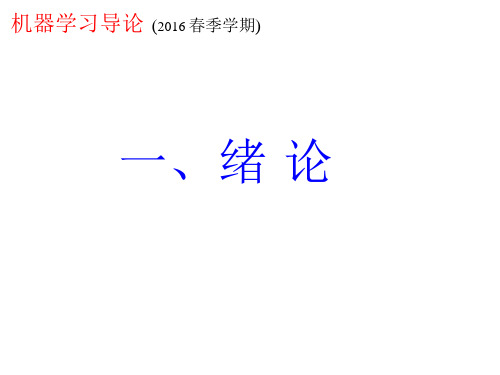
这个团队行动保密,定期向奥巴马报送结果; 被奥巴马公开称为总统竞选的
“核武器按钮”(“They are our nuclear codes”)
机器学习源自“人工智能”
Artificial Intelligence (AI), 1956 -
浊响
浊响
应该采用哪一个 模型(假设)?
归纳偏好 (inductive bias)
机器学习算法在学习过程中对某种类型假设的偏好
A更好? B更好?
一般原则: 奥卡姆剃刀
(Ocam’s razor)
任何一个有效的机器学习算法必有其偏好
学习算法的归纳偏好是否与问题本身匹配, 大多数时候直接决定了算法能否取得好的性能!
- 1947年出土 - 超过30,000个羊皮纸片段
Cairo Genizah - 19世纪末被发现 - 超过300,000个片段 - 散布于全球多家博物馆
高水平专家的大量精力 被用于古文献修复
[L. Wolf et al., IJCV 2011]
例如:古文献修复 (文化)
一个重要问题:
原书籍已经变成分散且混杂的多个书页,如何拼接相邻的书页?
文献筛选的故事为了降低昂贵的成本tufts医学中心引入了机器学习技术邀请专家阅读少量摘要标记为有关或无关分类模型对是否有关进行预测人类专家只需阅读50篇摘要系统的自动筛选精度就达到93人类专家阅读1000篇摘要则系统的自动筛选敏感度达到95人类专家以前需阅读33000篇摘要才能获得此效果色泽青绿蜷缩乌黑蜷缩青绿硬挺清脆决策树神经网络支持向量机boosting贝叶斯网
自动鉴定
分类模型 待鉴定画作
特有“笔迹”
人工智能领域机器学习理论和模型方面88个课题名称

人工智能领域机器学习理论和模型方面88个课题名称以下是人工智能领域机器学习理论和模型方面的88个课题名称:1. 支持向量机的理论与应用2. 决策树算法研究与优化3. 随机森林模型的改进与应用4. 基于神经网络的模型优化与训练算法5. 卷积神经网络的结构设计与参数优化6. 递归神经网络的理论研究与应用7. 长短时记忆网络的改进与应用8. Transformer模型的研究与应用9. 堆叠自编码器的理论与实践10. 受限波尔兹曼机的理论研究与应用11. 稀疏编码模型的改进与应用12. 深度置信网络的优化与应用13. 半监督学习理论与算法研究14. 强化学习的理论与应用研究15. 深度强化学习的模型与算法研究16. 迁移学习的理论与方法研究17. 对抗学习与生成模型的研究与应用18. 多任务学习的理论与算法优化19. 主动学习的模型改进与应用20. 弱监督学习的方法与应用研究21. 增量学习的理论与算法研究22. 大规模机器学习的优化与并行化23. 稳定性理论在机器学习中的应用研究24. 联邦学习的理论与算法研究25. 记忆增强型神经网络的研究与应用26. 多模态学习的理论与算法研究27. 深度学习与图像分析的交叉研究28. 深度学习在自然语言处理中的应用研究29. 深度学习在语音处理中的应用研究30. 深度学习在视频分析中的应用研究31. 深度学习在推荐系统中的应用研究32. 深度学习在医疗图像诊断中的应用研究33. 深度学习在生物信息学中的应用研究34. 深度学习在金融预测中的应用研究35. 深度学习在交通流预测中的应用研究36. 深度学习在智能驾驶中的应用研究37. 深度学习在智能物联网中的应用研究38. 深度学习在虚拟现实中的应用研究39. 深度学习在增强现实中的应用研究40. 深度学习在游戏设计中的应用研究41. 深度学习在人脸识别中的应用研究42. 深度学习在行为识别中的应用研究43. 深度学习在目标检测中的应用研究44. 深度学习在图像生成中的应用研究45. 深度学习在时序数据预测中的应用研究46. 深度学习在推荐系统中的应用研究47. 深度学习在异常检测中的应用研究48. 深度学习在网络安全中的应用研究49. 深度学习在自动驾驶中的应用研究50. 深度学习在自然语言生成中的应用研究51. 深度学习在机器翻译中的应用研究52. 深度学习在情感分析中的应用研究53. 深度学习在问答系统中的应用研究54. 深度学习在文本分类中的应用研究55. 深度学习在音乐生成中的应用研究56. 深度学习在文本生成中的应用研究58. 深度学习在药物发现中的应用研究59. 深度学习在股票预测中的应用研究60. 深度学习在风控与反欺诈中的应用研究61. 深度学习在客户关系管理中的应用研究62. 深度学习在供应链优化中的应用研究63. 深度学习在销售预测中的应用研究64. 深度学习在舆情分析中的应用研究65. 深度学习在信用评估中的应用研究66. 深度学习在环境监测中的应用研究67. 深度学习在智能辅助决策中的应用研究68. 深度学习在智能城市中的应用研究69. 深度学习在智能家居中的应用研究70. 深度学习在智慧医疗中的应用研究71. 深度学习在工业控制中的应用研究72. 深度学习在电力系统中的应用研究73. 深度学习在农业领域中的应用研究74. 深度学习在物流优化中的应用研究75. 深度学习在循环经济中的应用研究76. 深度学习在智慧旅游中的应用研究77. 深度学习在智能交通中的应用研究78. 深度学习在智能安防中的应用研究79. 深度学习在智慧零售中的应用研究80. 深度学习在智能金融中的应用研究81. 深度学习在智能教育中的应用研究82. 深度学习在智能体育中的应用研究83. 深度学习在智能制造中的应用研究84. 深度学习在智能文化遗产中的应用研究85. 深度学习在智能环保中的应用研究86. 深度学习在智能航空航天中的应用研究88. 深度学习在智能军事中的应用研究。
fundamentals翻译
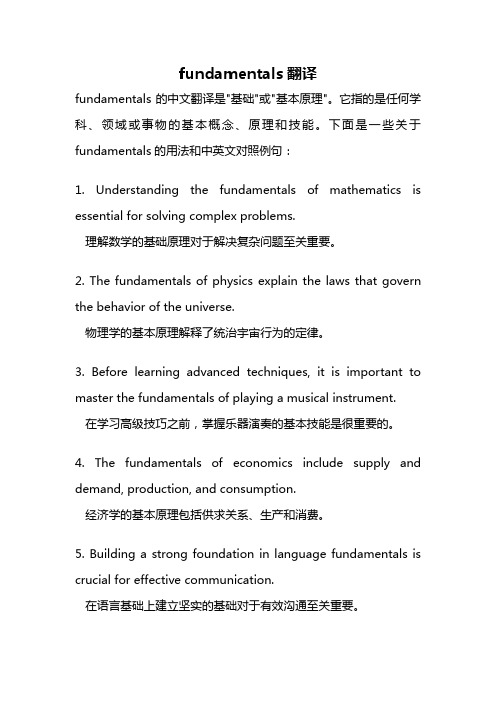
fundamentals翻译fundamentals的中文翻译是"基础"或"基本原理"。
它指的是任何学科、领域或事物的基本概念、原理和技能。
下面是一些关于fundamentals的用法和中英文对照例句:1. Understanding the fundamentals of mathematics is essential for solving complex problems.理解数学的基础原理对于解决复杂问题至关重要。
2. The fundamentals of physics explain the laws that govern the behavior of the universe.物理学的基本原理解释了统治宇宙行为的定律。
3. Before learning advanced techniques, it is important to master the fundamentals of playing a musical instrument.在学习高级技巧之前,掌握乐器演奏的基本技能是很重要的。
4. The fundamentals of economics include supply and demand, production, and consumption.经济学的基本原理包括供求关系、生产和消费。
5. Building a strong foundation in language fundamentals is crucial for effective communication.在语言基础上建立坚实的基础对于有效沟通至关重要。
6. The fundamentals of programming involve understanding algorithms, data structures, and coding languages.编程的基础包括理解算法、数据结构和编程语言。
andrew ng的讲义中文
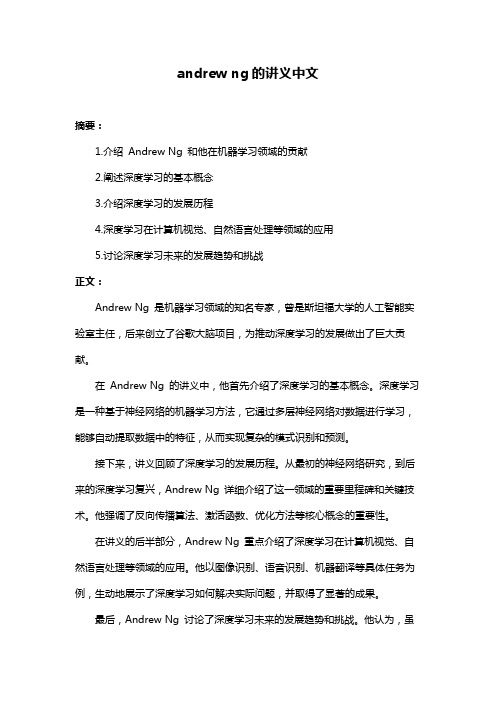
andrew ng的讲义中文
摘要:
1.介绍Andrew Ng 和他在机器学习领域的贡献
2.阐述深度学习的基本概念
3.介绍深度学习的发展历程
4.深度学习在计算机视觉、自然语言处理等领域的应用
5.讨论深度学习未来的发展趋势和挑战
正文:
Andrew Ng 是机器学习领域的知名专家,曾是斯坦福大学的人工智能实验室主任,后来创立了谷歌大脑项目,为推动深度学习的发展做出了巨大贡献。
在Andrew Ng 的讲义中,他首先介绍了深度学习的基本概念。
深度学习是一种基于神经网络的机器学习方法,它通过多层神经网络对数据进行学习,能够自动提取数据中的特征,从而实现复杂的模式识别和预测。
接下来,讲义回顾了深度学习的发展历程。
从最初的神经网络研究,到后来的深度学习复兴,Andrew Ng 详细介绍了这一领域的重要里程碑和关键技术。
他强调了反向传播算法、激活函数、优化方法等核心概念的重要性。
在讲义的后半部分,Andrew Ng 重点介绍了深度学习在计算机视觉、自然语言处理等领域的应用。
他以图像识别、语音识别、机器翻译等具体任务为例,生动地展示了深度学习如何解决实际问题,并取得了显著的成果。
最后,Andrew Ng 讨论了深度学习未来的发展趋势和挑战。
他认为,虽
然深度学习在许多领域取得了成功,但仍然存在一些问题,如模型的可解释性、数据依赖性等。
他呼吁研究人员继续探索新的方法,以解决这些问题,进一步推动深度学习的发展。
人工智能基础 汤晓鸥著 试题
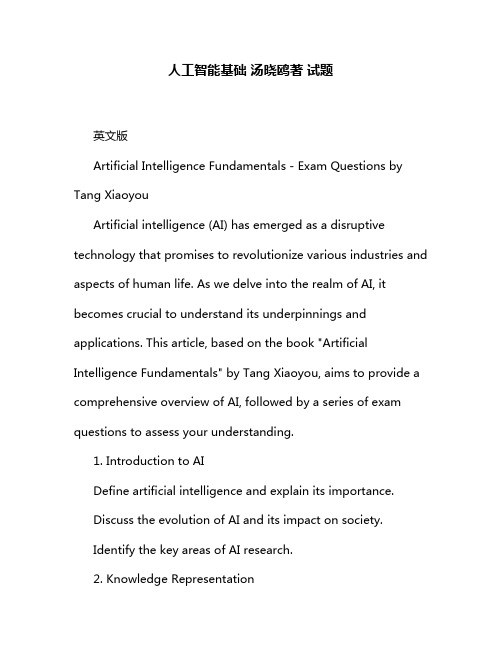
人工智能基础汤晓鸥著试题英文版Artificial Intelligence Fundamentals - Exam Questions by Tang XiaoyouArtificial intelligence (AI) has emerged as a disruptive technology that promises to revolutionize various industries and aspects of human life. As we delve into the realm of AI, it becomes crucial to understand its underpinnings and applications. This article, based on the book "Artificial Intelligence Fundamentals" by Tang Xiaoyou, aims to provide a comprehensive overview of AI, followed by a series of exam questions to assess your understanding.1. Introduction to AIDefine artificial intelligence and explain its importance.Discuss the evolution of AI and its impact on society.Identify the key areas of AI research.2. Knowledge RepresentationDescribe the different types of knowledge representation techniques.Explain the concept of ontologies and their role in AI.Discuss the limitations of knowledge representation.3. Problem Solving and ReasoningDefine problem-solving techniques in AI and provide examples.Describe the difference between deductive and inductive reasoning.Explain the working principle of expert systems.4. Machine LearningDefine machine learning and classify its different types.Discuss the fundamental concepts of supervised and unsupervised learning.Explain the principles of reinforcement learning and its applications.5. Neural Networks and Deep LearningDescribe the basic structure and working principle of neural networks.Explain the concept of deep learning and its applications in AI.Discuss the advantages and disadvantages of deep learning.6. Natural Language Processing (NLP)Define NLP and its role in AI.Describe the fundamental techniques used in NLP, such as tokenization, part-of-speech tagging, and parsing.Explain the principles of machine translation and its impact on language barriers.7. Computer VisionDefine computer vision and its applications in AI.Describe the techniques used in image recognition and analysis.Discuss the working principle of object detection and its importance in various fields.8. Ethical and Social Aspects of AIDiscuss the ethical considerations in the development and deployment of AI systems.Analyze the potential social impacts of AI on employment, privacy, and security.Propose strategies to address the ethical challenges associated with AI.ConclusionArtificial intelligence, being a rapidly evolving field, offers immense opportunities and challenges. The exam questions provided in this article aim to test your understanding of the fundamental concepts and applications of AI. By answering these questions, you can assess your readiness to delve deeper into the world of AI and its potential to revolutionize our lives.人工智能基础 - 汤晓鸥著试题英文版人工智能基础——汤晓鸥著试题人工智能(AI)已成为一种颠覆性技术,有望革命性地改变各个行业和人类生活的方方面面。
机器人学基础(全套课件470P)

Fundamentals of Robotics
智能科学基础系列课0
Fundamentals of Robotics
1
Ch. 1 Introduction 第1章 绪 论
Ch. 1 Introduction
2
Contents
Course Schedule Top 10 Robotics News of 2008 Development of Robotics Structure, Feature, and Classification of
讲授
2 讲授
2 课堂 报告
2 实验
9
教学进度安排(3)
月 日 周次 4 20 8
教学内容 机器人编程
教学 时数
2
教学 课外 备 方式 时数 注
讲授 2
4 23 8
机器人编程训练
2 训练
4 27 9 综合实验:智能机器人的路 2 综合
径规划与行为决策实验
实验
4 30 9
机器人应用
2 讲授
5 4 10
Ch. 1 Introduction
17
2 Robot ride on a wheelbarrow
➢ In September 2008 Japanese Murata Manufacturing Institute launched a new type of robot riding on a wheelbarrow, named "seiko". This new type of robot can maintain its balance through a series of sensors and gyroscopes, and easy to complete riding of a wheelbarrow.
深度学习,走向核心素养 英语 读后感

深度学习,走向核心素养英语读后感Embracing the Future: Exploring the Depth of Machine Learning and Its Impact on Core CompetenciesThe rapid advancements in technology have ushered in a new era of innovation, where the field of machine learning has emerged as a transformative force. As we delve into the realm of deep learning, a profound understanding of its implications on our core competencies becomes increasingly crucial. This essay aims to explore the depth of machine learning and its profound influence on the development of essential skills and knowledge.At the heart of deep learning lies the remarkable ability to extract meaningful patterns from vast amounts of data. Through the implementation of complex neural networks, machines can now mimic the human brain's learning processes, enabling them to tackle increasingly complex tasks with remarkable accuracy and efficiency. This paradigm shift has opened up new frontiers in various domains, from image recognition and natural language processing to predictive analytics and decision-making.One of the key advantages of deep learning lies in its capacity toautomate repetitive tasks and streamline workflows. By delegating mundane, rule-based activities to intelligent algorithms, individuals and organizations can redirect their focus towards more strategic and creative endeavors. This shift in the division of labor has profound implications for the development of core competencies, as it demands a greater emphasis on critical thinking, problem-solving, and adaptability.As the boundaries between human and machine intelligence continue to blur, the need for a deeper understanding of the underlying principles of deep learning becomes paramount. Mastering the fundamentals of machine learning, from data preprocessing to model architecture and optimization, empowers individuals to navigate the rapidly evolving technological landscape with confidence. By cultivating a robust comprehension of these concepts, professionals can leverage the power of deep learning to enhance their decision-making abilities, optimize workflows, and drive innovation within their respective fields.Moreover, the integration of deep learning into various industries has transformed the way we approach problem-solving and decision-making. In the realm of healthcare, for instance, deep learning algorithms have demonstrated remarkable capabilities in early disease detection, personalized treatment recommendations, and drug discovery. Similarly, in the financial sector, deep learningmodels have revolutionized risk assessment, fraud detection, and investment strategies. By understanding the potential of deep learning in these domains, individuals can develop specialized skills and knowledge that are highly sought after in the job market.Beyond the technical aspects of deep learning, the broader implications of this technology on our core competencies cannot be overlooked. As machines become increasingly adept at tasks that were once the exclusive domain of humans, the need for a well-rounded education and the cultivation of transferable skills becomes paramount. Critical thinking, creativity, emotional intelligence, and effective communication are just a few of the essential competencies that will continue to be highly valued in the age of deep learning.Embracing the depth of machine learning also requires a profound understanding of the ethical considerations that accompany this technological advancement. As deep learning systems become more pervasive, issues surrounding data privacy, algorithmic bias, and the responsible use of AI must be addressed. By developing a strong ethical foundation and a commitment to the responsible development of deep learning technologies, individuals can contribute to the creation of a more equitable and sustainable future.In conclusion, the exploration of deep learning and its impact on core competencies is a multifaceted endeavor. By delving into thetechnical intricacies of machine learning, cultivating specialized skills, and fostering a holistic understanding of the ethical implications, individuals can position themselves at the forefront of this technological revolution. As we navigate the challenges and opportunities presented by deep learning, the development of a comprehensive skillset and a nuanced perspective will be instrumental in shaping the future of our personal and professional lives.。
- 1、下载文档前请自行甄别文档内容的完整性,平台不提供额外的编辑、内容补充、找答案等附加服务。
- 2、"仅部分预览"的文档,不可在线预览部分如存在完整性等问题,可反馈申请退款(可完整预览的文档不适用该条件!)。
- 3、如文档侵犯您的权益,请联系客服反馈,我们会尽快为您处理(人工客服工作时间:9:00-18:30)。
exhaustive alternatives
P(A + B) = P(“A or B”) P(AB) = P(A£B) = P(“A and B”) P(Ã) = P(“not A”) = 1 – P(A)
P(heads) ≈ #heads / #trials (can also quantify uncertainty) 1
0
❐ Bayesian: probability is a measure of belief. Use prior knowledge of coins to initially assume P(heads) = 0.5; revise this model of the coin in response to observations if necessary. (Bayes’ rule will tell us exactly how to do this.)
number of observations goes to infinity. It is purely descriptive. Example: “70% of November days in Ireland are rainy” ❐ Bayesian: probability is a “degree of confidence” that one attaches to an uncertain event (Bernoulli, 1654-1705). It can be manipulated, for instance by applying Bayes’ Rule. Example: “there is a 30% chance of rain tomorrow” Both respect the axioms of probability. For machine learning the Bayesian view is crucial, since it allows us to update measures of belief (models!) in response to observations – that is, to learn.
The mathematical/statistical foundation of machine learning: ❐ Bayesian inference: how to learn from observations (today) ❐ maximum likelihood: how to quantify the fit between a
Statistical Machine Learning
© Nic Schraudolph. All rights reserved.
5
Conditional Probability
P( A and B) P( A| B) = P(B)
P(A|B) is read “probability of A given B”, meaning: the probability of A, given that B has already occurred. Example: in a given country, 90% of households own a TV, and 54% own both a TV and DVD player. What is the probability that a household with TV also owns a DVD player?
model and observations (today) ❐ optimization: how to improve the fit of your model ❐ regularization, model selection: what is the best model?
Statistical Machine Learning
© Nic Schraudolph. All rights reserved.
2ቤተ መጻሕፍቲ ባይዱ
What is a Probability?
Technical answer: any number P that obeys the axioms of probability:
❐ must lie between zero and one: 0 · P · 1 ❐ must add up: P(A + B) = P(A) + P(B) – P(AB)
Statistical Machine Learning
© Nic Schraudolph. All rights reserved.
3
Interpretations of Probability
There are two interpretations of probability in statistics: ❐ frequentist: probability is the limit of observed frequency as
Statistical Machine Learning
© Nic Schraudolph. All rights reserved.
4
Example: Coin Tosses
For a given coin, what is the probability of coming up heads? ❐ frequentist: toss the coin many times
Machine Learning: Fundamentals
A model is a formal description of a belief about the world. Learning is the construction and/or revision of a model in
response to observations of the world.
© Nic Schraudolph. All rights reserved.
1
Statistical Machine Learning Nic Schraudolph
Bayesian Inference
Rev. Thomas Bayes (1702-1761)
Statistical Machine Learning