表情识别应用1
人脸识别中的表情识别技术
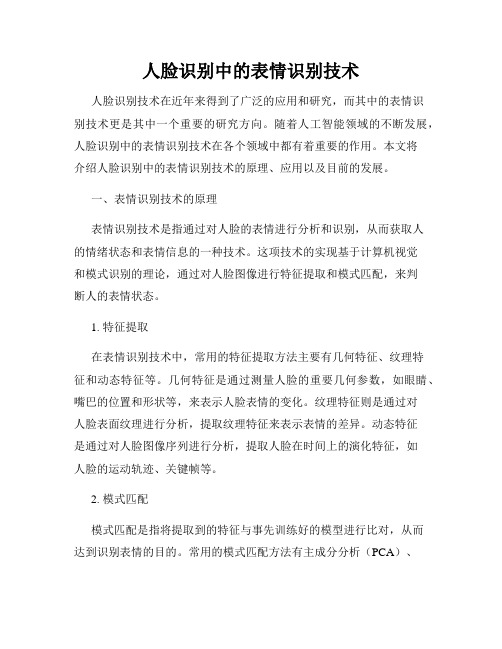
人脸识别中的表情识别技术人脸识别技术在近年来得到了广泛的应用和研究,而其中的表情识别技术更是其中一个重要的研究方向。
随着人工智能领域的不断发展,人脸识别中的表情识别技术在各个领域中都有着重要的作用。
本文将介绍人脸识别中的表情识别技术的原理、应用以及目前的发展。
一、表情识别技术的原理表情识别技术是指通过对人脸的表情进行分析和识别,从而获取人的情绪状态和表情信息的一种技术。
这项技术的实现基于计算机视觉和模式识别的理论,通过对人脸图像进行特征提取和模式匹配,来判断人的表情状态。
1. 特征提取在表情识别技术中,常用的特征提取方法主要有几何特征、纹理特征和动态特征等。
几何特征是通过测量人脸的重要几何参数,如眼睛、嘴巴的位置和形状等,来表示人脸表情的变化。
纹理特征则是通过对人脸表面纹理进行分析,提取纹理特征来表示表情的差异。
动态特征是通过对人脸图像序列进行分析,提取人脸在时间上的演化特征,如人脸的运动轨迹、关键帧等。
2. 模式匹配模式匹配是指将提取到的特征与事先训练好的模型进行比对,从而达到识别表情的目的。
常用的模式匹配方法有主成分分析(PCA)、独立成分分析(ICA)、支持向量机(SVM)等。
通过建立模型和训练样本的匹配关系,可以实现对人脸表情的识别。
二、表情识别技术的应用表情识别技术在人脸识别领域中有着广泛的应用,下面将介绍一些主要的应用场景。
1. 情感分析表情识别技术可以帮助我们判断人的情感状态,对于情感分析具有重要的意义。
比如在社交媒体中,通过分析用户在照片或视频中的表情,可以了解用户对某个事件或产品的态度和情感,从而为商家或企业提供有效的市场调研数据。
2. 人机交互表情识别技术可以应用于人机交互领域,通过对用户面部表情的识别,可以实现更加智能和自然的人机交互方式。
比如在游戏中,人脸表情的识别可以作为控制命令的输入方式,实现更加沉浸式的游戏体验。
3. 安防监控表情识别技术可以应用于安防监控领域,通过对行人或人群中的表情状态进行分析,可以判断是否存在可疑人员或异常行为。
人脸表情识别技术的使用教程与情绪分析应用
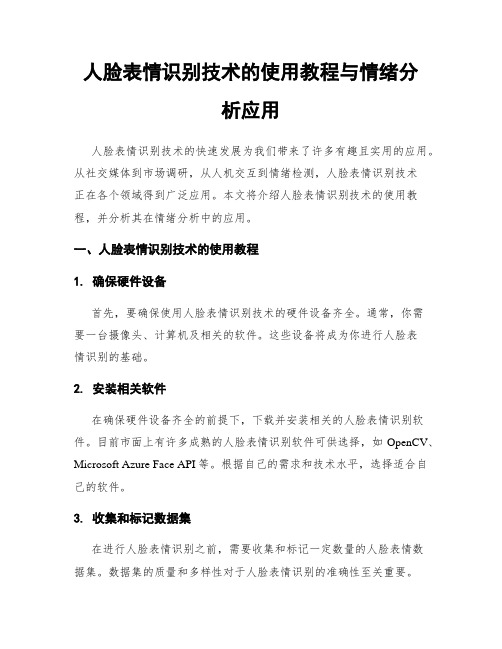
人脸表情识别技术的使用教程与情绪分析应用人脸表情识别技术的快速发展为我们带来了许多有趣且实用的应用。
从社交媒体到市场调研,从人机交互到情绪检测,人脸表情识别技术正在各个领域得到广泛应用。
本文将介绍人脸表情识别技术的使用教程,并分析其在情绪分析中的应用。
一、人脸表情识别技术的使用教程1. 确保硬件设备首先,要确保使用人脸表情识别技术的硬件设备齐全。
通常,你需要一台摄像头、计算机及相关的软件。
这些设备将成为你进行人脸表情识别的基础。
2. 安装相关软件在确保硬件设备齐全的前提下,下载并安装相关的人脸表情识别软件。
目前市面上有许多成熟的人脸表情识别软件可供选择,如OpenCV、Microsoft Azure Face API等。
根据自己的需求和技术水平,选择适合自己的软件。
3. 收集和标记数据集在进行人脸表情识别之前,需要收集和标记一定数量的人脸表情数据集。
数据集的质量和多样性对于人脸表情识别的准确性至关重要。
你可以通过在线数据库、社交媒体或者自己的照片进行数据集的搜集。
同时,要确保数据集中包含不同表情的照片,以提高模型的泛化能力。
4. 训练人脸表情识别模型在准备好数据集之后,你需要使用相关的机器学习算法来训练人脸表情识别模型。
常用的机器学习算法有支持向量机(SVM)、卷积神经网络(CNN)等。
根据选择的软件以及数据集的特点,你可以选择合适的算法进行训练。
5. 测试和优化模型在完成模型训练后,你需要进行模型的测试和优化。
通过测试模型在新数据上的表现,可以评估模型的准确性和鲁棒性。
如果模型表现不佳,你可以调整模型的参数、增加训练数据集的数量或进行其他优化策略。
6. 部署和应用模型当你对模型满意后,可以将其部署到实际应用中。
根据需求,你可以将人脸表情识别技术应用于社交媒体、人机交互、市场调研等领域。
例如,将人脸表情识别技术应用于视频聊天软件,可以实时识别对方的表情,提高交流质量。
二、人脸表情识别技术在情绪分析中的应用人脸表情识别技术在情绪分析中有广泛的应用。
面部表情识别技术在智慧医疗中的应用研究
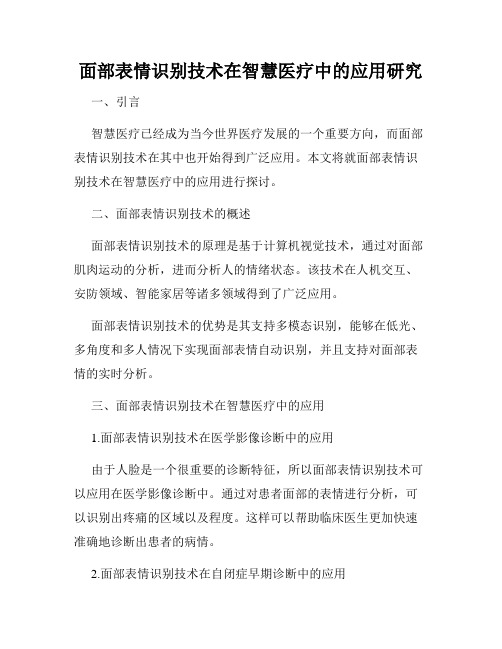
面部表情识别技术在智慧医疗中的应用研究一、引言智慧医疗已经成为当今世界医疗发展的一个重要方向,而面部表情识别技术在其中也开始得到广泛应用。
本文将就面部表情识别技术在智慧医疗中的应用进行探讨。
二、面部表情识别技术的概述面部表情识别技术的原理是基于计算机视觉技术,通过对面部肌肉运动的分析,进而分析人的情绪状态。
该技术在人机交互、安防领域、智能家居等诸多领域得到了广泛应用。
面部表情识别技术的优势是其支持多模态识别,能够在低光、多角度和多人情况下实现面部表情自动识别,并且支持对面部表情的实时分析。
三、面部表情识别技术在智慧医疗中的应用1.面部表情识别技术在医学影像诊断中的应用由于人脸是一个很重要的诊断特征,所以面部表情识别技术可以应用在医学影像诊断中。
通过对患者面部的表情进行分析,可以识别出疼痛的区域以及程度。
这样可以帮助临床医生更加快速准确地诊断出患者的病情。
2.面部表情识别技术在自闭症早期诊断中的应用自闭症是一种神经发育障碍,早期诊断是非常重要的。
而面部表情识别技术可以通过分析婴儿或儿童的面部表情来及早发现自闭症的症状,提高诊断的准确率。
3.面部表情识别技术在疾病预测中的应用面部表情识别技术可以分析患者的面部表情来预测患者的病情。
例如,通过分析患者的面部表情来判断是否患有抑郁症、焦虑症等疾病。
这样可以更早地发现患者的病情,及时进行干预和治疗。
4.面部表情识别技术在药物监测中的应用药物治疗是一种治疗疾病的手段。
然而药物治疗需要进行剂量调整,而面部表情识别技术可以被用于监测患者的情绪状态和病情变化等信息。
这样医生可以根据面部表情的分析结果来对治疗进行个性化的调整,以达到更好的治疗效果。
四、面部表情识别技术的应用前景展望随着智能技术的不断发展,面部表情识别技术也将得到更广泛的应用。
未来,这技术将会被应用于手术机器人的研发、通过分析患者面部表情来改善病房环境、以及通过分析医护人员面部表情来提高医患沟通效果等领域。
《计算机视觉》教学课件 第11章1-人脸检测、识别与表情识别1

• 自行构造人脸数据库和测试集
2024/7/13
6
项目任务
➢基于ResNet进行表情识别
➢使用Kaggle ICML表情数据集
• 包含35,887张48*48大小的表情灰度图片,共计七种类别:愤怒、厌恶、恐惧、高兴、悲伤、
惊讶和中性,并被保存在csv文件中(保存的是像素值)
MTCNN)
• 将人脸区域检测与人脸关键点检测放在一
起
• 这三个级联的网络
• P-Net生成候选框
• R-Net高精度候选框过滤选择
• O-Net生成最终候选框与人脸关键点
• 图像金字塔、非极大抑制
2024/7/13
13
知识链接-MTCNN
• P-Net,R-Net和O-Net的体系结构
• “MP”表示最大池化,“Conv”表示卷积
2024/7/13
18
知识链接-FaceNet
• Batch normalization 批归一化
• 对每层输出进行归一化处理
• 假设一个batch中有m个样本,在某一层的输出分别是 {1 , 2 , … , }, 可能是一维向量,
也可能是二维特征图
2024/7/13
19
知识链接-FaceNet
• 有三张图片参与计算
• 使得提取出来的特征,在相似图片上距离相近,不同图片上距离远
min
anchor
2024/7/13
positive
anchor negative
16
知识链接-FaceNet
2024/7/13
17
知识链接-FaceNet
• Batch normalization 批归一化
人脸表情识别技术的情感分析应用研究
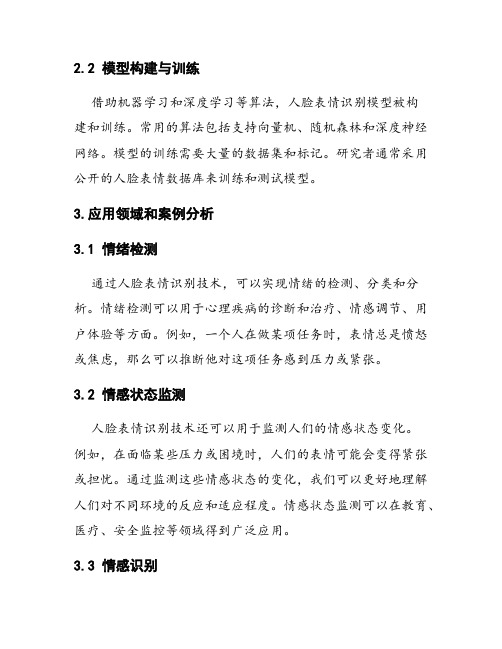
人脸表情识别技术的情感分析应用研究引言:随着科技的迅速发展,人脸表情识别技术成为了一个非常火热的研究领域。
这一技术在情感分析领域具有广泛的应用前景。
情感分析旨在通过分析人的表情来推断他们的情感状态。
本文将探讨人脸表情识别技术的应用和相关研究的进展。
1.人脸表情的情感分析意义人的表情是情感的重要表达方式,通过分析人脸表情,可以获取和推断个体的情感状态。
情感分析可以应用于情绪检测、情感状态监测、情感识别等领域。
2.人脸表情识别技术的方法2.1 特征提取特征提取是人脸表情识别的核心环节。
常用的方法包括图像处理、特征选择和特征提取。
图像处理技术可以去除背景杂乱干扰、调整图像大小和对比度,使图像更适合表情识别。
特征选择和特征提取方法可以帮助提取图像中最有代表性的特征。
2.2 模型构建与训练借助机器学习和深度学习等算法,人脸表情识别模型被构建和训练。
常用的算法包括支持向量机、随机森林和深度神经网络。
模型的训练需要大量的数据集和标记。
研究者通常采用公开的人脸表情数据库来训练和测试模型。
3.应用领域和案例分析3.1 情绪检测通过人脸表情识别技术,可以实现情绪的检测、分类和分析。
情绪检测可以用于心理疾病的诊断和治疗、情感调节、用户体验等方面。
例如,一个人在做某项任务时,表情总是愤怒或焦虑,那么可以推断他对这项任务感到压力或紧张。
3.2 情感状态监测人脸表情识别技术还可以用于监测人们的情感状态变化。
例如,在面临某些压力或困境时,人们的表情可能会变得紧张或担忧。
通过监测这些情感状态的变化,我们可以更好地理解人们对不同环境的反应和适应程度。
情感状态监测可以在教育、医疗、安全监控等领域得到广泛应用。
3.3 情感识别人脸表情识别技术的另一个重要应用领域是情感识别。
通过分析人的表情,可以准确推断他们的情感状态,例如高兴、悲伤、惊喜等。
情感识别可以应用于广告评估、市场调研、用户反馈等方面。
例如,通过分析消费者购物时的表情,可以了解他们对商品的兴趣度和满意度,从而改进产品的设计和销售策略。
人脸表情识别技术的应用教程
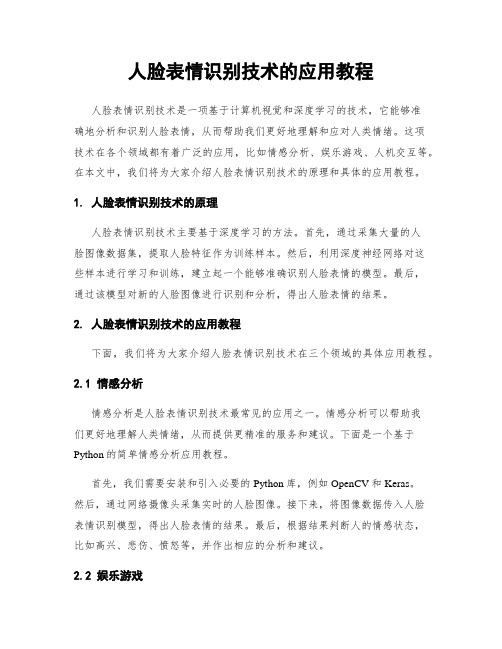
人脸表情识别技术的应用教程人脸表情识别技术是一项基于计算机视觉和深度学习的技术,它能够准确地分析和识别人脸表情,从而帮助我们更好地理解和应对人类情绪。
这项技术在各个领域都有着广泛的应用,比如情感分析、娱乐游戏、人机交互等。
在本文中,我们将为大家介绍人脸表情识别技术的原理和具体的应用教程。
1. 人脸表情识别技术的原理人脸表情识别技术主要基于深度学习的方法。
首先,通过采集大量的人脸图像数据集,提取人脸特征作为训练样本。
然后,利用深度神经网络对这些样本进行学习和训练,建立起一个能够准确识别人脸表情的模型。
最后,通过该模型对新的人脸图像进行识别和分析,得出人脸表情的结果。
2. 人脸表情识别技术的应用教程下面,我们将为大家介绍人脸表情识别技术在三个领域的具体应用教程。
2.1 情感分析情感分析是人脸表情识别技术最常见的应用之一。
情感分析可以帮助我们更好地理解人类情绪,从而提供更精准的服务和建议。
下面是一个基于Python的简单情感分析应用教程。
首先,我们需要安装和引入必要的Python库,例如OpenCV和Keras。
然后,通过网络摄像头采集实时的人脸图像。
接下来,将图像数据传入人脸表情识别模型,得出人脸表情的结果。
最后,根据结果判断人的情感状态,比如高兴、悲伤、愤怒等,并作出相应的分析和建议。
2.2 娱乐游戏人脸表情识别技术在娱乐游戏领域也有着广泛的应用。
通过该技术,我们可以开发出一些能够与人脸表情互动的娱乐游戏,例如拳击游戏、表情匹配等。
下面是一个基于Unity引擎的简单娱乐游戏应用教程。
首先,我们需要创建一个虚拟环境,并引入必要的游戏资源和编程代码。
然后,通过网络摄像头获取实时的人脸图像,并将其传递给人脸表情识别模型进行分析。
接下来,根据分析结果,将特定表情与游戏中的操作相匹配,从而与玩家进行互动。
最后,根据玩家的表情变化,不断更新游戏的状态和难度。
2.3 人机交互人脸表情识别技术还可以应用于人机交互领域,帮助我们更自然和便捷地与计算机进行交互。
面部表情识别技术的使用方法和常见问题解答

面部表情识别技术的使用方法和常见问题解答面部表情识别技术是一种通过人脸图像或视频中的面部表情信息来分析和识别人类情绪状态的技术。
它在计算机视觉和人机交互领域有着广泛的应用。
本文将介绍面部表情识别技术的使用方法,并提供一些常见问题的解答。
一、面部表情识别技术的使用方法1. 数据收集:在使用面部表情识别技术之前,需要收集一定量的数据用于模型训练。
这些数据通常包括人脸图像或视频,以及与之相关的情感标签。
可以通过从现有的面部表情数据集中收集样本,或者自己收集数据来满足训练需求。
2. 数据预处理:在数据收集之后,需要对数据进行预处理操作,以提高面部表情识别模型的准确度。
预处理操作包括对图像或视频进行裁剪、缩放、灰度化等处理。
3. 特征提取:面部表情识别技术的关键在于提取面部表情中蕴含的特征。
常用的特征提取方法包括使用光流法提取面部表情运动信息,利用神经网络模型提取高阶特征等。
4. 模型训练:通过采用监督学习方法,可以使用已标记的数据来训练面部表情识别模型。
常用的模型包括卷积神经网络(CNN)、支持向量机(SVM)等。
在训练过程中,可以使用交叉验证等技术对模型进行优化和验证。
5. 模型测试和应用:在模型训练完毕后,可以使用测试数据来评估模型的准确度和性能。
一旦模型通过测试,就可以将其应用于实际场景中,如情感分析、人机交互等。
二、常见问题解答1. 面部表情识别技术的准确度如何?面部表情识别技术的准确度受多种因素影响,包括数据质量、特征提取方法、模型训练参数等。
通常情况下,准确度可以达到较高水平,但仍存在误识别和漏识别的问题。
不同的应用场景和需求可以采用不同的指标来评估准确度。
2. 面部表情识别技术是否需要摄像头?面部表情识别技术的基础是人脸图像或视频,因此通常需要使用摄像头来采集人脸数据。
但是,在某些场景下,也可以使用已有的图像或视频数据进行识别,无需额外的摄像设备。
3. 面部表情识别技术是否能够识别不同人种、不同年龄段的面部表情?面部表情识别技术在设计和训练时通常考虑到抗干扰性和泛化能力,可以对不同人种和年龄段的面部表情进行识别。
面部表情识别技术在疾病诊断中的应用
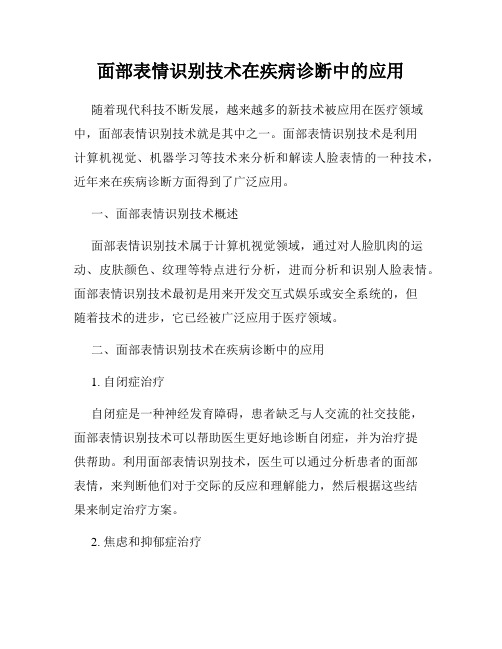
面部表情识别技术在疾病诊断中的应用随着现代科技不断发展,越来越多的新技术被应用在医疗领域中,面部表情识别技术就是其中之一。
面部表情识别技术是利用计算机视觉、机器学习等技术来分析和解读人脸表情的一种技术,近年来在疾病诊断方面得到了广泛应用。
一、面部表情识别技术概述面部表情识别技术属于计算机视觉领域,通过对人脸肌肉的运动、皮肤颜色、纹理等特点进行分析,进而分析和识别人脸表情。
面部表情识别技术最初是用来开发交互式娱乐或安全系统的,但随着技术的进步,它已经被广泛应用于医疗领域。
二、面部表情识别技术在疾病诊断中的应用1. 自闭症治疗自闭症是一种神经发育障碍,患者缺乏与人交流的社交技能,面部表情识别技术可以帮助医生更好地诊断自闭症,并为治疗提供帮助。
利用面部表情识别技术,医生可以通过分析患者的面部表情,来判断他们对于交际的反应和理解能力,然后根据这些结果来制定治疗方案。
2. 焦虑和抑郁症治疗面部表情识别技术也可以被用来诊断焦虑和抑郁症等精神疾病。
人的面部表情对于情绪状态的反应非常敏感,面部表情识别技术可以分析患者的面部表情来评估他们的情绪状态,以便制定准确的治疗方案。
3. 帕金森病治疗帕金森病是一种神经系统疾病,患者缺乏正常的运动协调能力。
利用面部表情识别技术,医生可以分析患者的面部表情来评估他们的运动能力和协调能力,从而更准确地诊断疾病,并制定更好的治疗方案。
4. 认知障碍治疗认知障碍患者缺乏对周围环境的认知和理解能力,面部表情识别技术可以帮助医生更好地评估患者的认知能力和理解能力。
通过对患者面部表情的分析,医生可以更好地理解和评估患者的认知水平,为治疗提供更好的指导。
三、面部表情识别技术的优势与传统的疾病诊断方法相比,面部表情识别技术具有如下优势:1. 非侵入性面部表情识别技术不需要进行任何身体接触,也不会对患者身体造成任何伤害,因此非常适合应用于小儿科、老人科等人群的疾病诊断。
2. 测量准确面部表情识别技术可以测量非常微小的面部运动和表情变化,从而提高诊断的准确率。
- 1、下载文档前请自行甄别文档内容的完整性,平台不提供额外的编辑、内容补充、找答案等附加服务。
- 2、"仅部分预览"的文档,不可在线预览部分如存在完整性等问题,可反馈申请退款(可完整预览的文档不适用该条件!)。
- 3、如文档侵犯您的权益,请联系客服反馈,我们会尽快为您处理(人工客服工作时间:9:00-18:30)。
The Painful Face–Pain Expression Recognition UsingActive Appearance ModelsAhmed Bilal Ashraf∗bilal@Simon Lucey∗slucey@Jeffrey F.Cohn∗†jeffcohn@Tsuhan Chen∗tsuhan@Zara Ambadar†ambadar@Ken Prkachin‡kmprk@unbc.caPatty Solomon§solomon@mcmaster.caBarry-John Theobald¶b.theobald@uea.aABSTRACTPain is typically assessed by patient self-report.Self-reportedpain,however,is difficult to interpret and may be impairedor not even possible,as in young children or the severelyill.Behavioral scientists have identified reliable and validfacial indicators of pain.Until now they required manualmeasurement by highly skilled observers.We developed anapproach that automatically recognizes acute pain.Adultpatients with rotator cuffinjury were video-recorded whilea physiotherapist manipulated their affected and unaffectedshoulder.Skilled observers rated pain expression from thevideo on a5-point Likert-type scale.From these ratings,sequences were categorized as no-pain(rating of0),pain(rating of3,4,or5),and indeterminate(rating of1or2).We explored machine learning approaches for pain-no painclassification.Active Appearance Models(AAM)were usedto decouple shape and appearance parameters from the dig-itized face images.Support vector machines(SVM)wereused with several representations from the ing aleave-one-out procedure,we achieved an equal error rate of19%(hit rate=81%)using canonical appearance and shapefeatures.Thesefindings suggest the feasibility of automaticpain detection from video.e07(a)(b)(c)(d)Figure1:Examples of temporally subsampled sequences.(a)and(c):Pain;(b)and(d):No Painexpressions related to emotion[16,17].While much of this effort has used simulated emotion with little or no head mo-tion,several systems have reported success in facial action recognition in real-world facial behavior,such as people ly-ing or telling the truth,watching movie clips intended to elicit emotion,or engaging in social interaction[3,5,18].In real-world applications and especially in patients experienc-ing acute pain,out-of-plane head motion and rapid changes in head motion and expression are particularly challenging. Extending the approach of[14],we applied machine learn-ing to the task of automatic pain detection in a real-world clinical setting involving patients undergoing assessment for pain.In machine learning,the choice of representation is known to influence recognition performance[10].Both appear-ance and shape representations have been investigated.Ex-amples of appearance based representations are raw pixels and Gaborfilters[1,2].A drawback of appearance based approaches is that they lack shape registration,and thus cannot locate vital expression indicators,such as the eyes, brows,eyelids,and mouth.Shape-based representations,which include Active Shape Models[6]and Active Appearance Models(AAM)[6,15]ad-dress this concern.They decouple shape and appearance and perform well in the task of expression recognition,es-pecially in the context of non-rigid head motion[19].In our previous work[14],we explored various representations derived from AAMs and concluded that this decoupling be-tween shape and appearance was indeed beneficial for action unit recognition.In this paper we extend our work based on AAM representations to pain expression recognition.2.IMAGE DATAImage data were from the UNBC-McMaster Shoulder Pain Expression Archive,which includes video clips for129sub-jects(63male,66female).Each subject was seen in“active”and“passive”conditions.In the active condition,subjects initiated shoulder rotation on their own;in passive,a phys-iotherapist was responsible for the movement.Camera angle for active tests was approximately frontal to start;camera angle for passive tests was approximately70degrees to start. Following each movement,subjects completed a10cm Vi-sual Analog Scale to indicate their level of subjective pain. The scale was presented on paper,with anchors of“no pain”and“worst pain imaginable”.Video of each trial was then rated by an observer with considerable training in the iden-tification of pain expression,using a0(no pain)–5(strong pain)Likert-type scale.Reliability of coding was estab-lished with a second coder,proficient in Facial Action Cod-ing System(F ACS)[11].The Pearson correlation between the observers’ratings on210randomly selected tests was 0.80.Correlation between observer rating and subject self-reported pain on VAS was0.74,for the tests used for recog-nition of pain from no pain.This correlation suggests mod-erate to high concurrent validity for pain intensity.For the current study,we included subjects in the active condition who had one or more pain ratings of both pain(0)and no-pain(3,4,or5).Fifty eight subjects met this initial cri-terion.Of these58,31were excluded for reasons including face out of frame,glasses,facial hair,bad image quality,oc-clusion,and hair in face.Thefinal sample consisted of21 subjects.Videos were captured at a resolution of320x240,out of which the face area spanned an average of approximately140x200(28000)pixels.Sample pain sequences are shown in Figure1.3.AAM SIn this section we briefly describe Active Appearance Mod-els(AAMs).The key dividend of AAMs is that they provide a decoupling between shape and appearance of a face image. Given a pre-defined linear shape model with linear appear-ance variation,AAMs have been demonstrated to produce excellent alignment of the shape model to an unseen im-age containing the object of interest.In general,AAMsfit their shape and appearance components through a gradient descent search,although other optimization methods have been employed with similar results[6].Keyframes within each video sequence were manually labeled,while the re-maining frames were automatically aligned using a gradient-descent AAMfit described in[15].3.1AAM Derived RepresentationsThe shape s of an AAM[6]is described by a2D trian-gulated mesh.In particular,the coordinates of the mesh vertices define the shape s(see row1,column(a),of Fig-ure2for examples of this mesh).These vertex locations correspond to a source appearance image,from which the shape was aligned(see row2,column(a),of Figure2).Since AAMs allow linear shape variation,the shape s can be ex-pressed as a base shape s0plus a linear combination of m shape vectors s i:s=s0+mi=1p i s i(1)where the coefficients p=(p1,...,p m)T are the shape pa-rameters.These shape parameters can typically be divided into similarity parameters p s and object-specific parame-ters p o,such that p T=[p T s,p T o].Similarity parameters are associated with the geometric similarity transform(i.e., translation,rotation and scale).The object-specific parame-ters,are the residual parameters representing geometric vari-ations associated with the actual object shape(e.g.,mouth opening,eyes shutting,etc.).Procrustes alignment[6]is employed to estimate the base shape s0.Once we have estimated the base shape and shape pa-rameters,we can normalize for various variables to achieve different representations as outlined in the following subsec-tions.3.1.1Similarity Normalized Shape,s nAs the name suggests,this representation gives the vertex locations after all similarity variation(translation,rotation and scale)has been removed.The similarity normalized shape s n can be obtained by synthesizing a shape instance of s,using Equation1,that ignores the similarity parameters of p.An example of this similarity normalized mesh can be seen in row1,column(b),of Figure2.3.1.2Similarity Normalized Appearance,a nThis representation contains appearance from which sim-ilarity variation has been removed.Once we have similarity normalized shape s n,as computed in section3.1.1,a sim-ilarity normalized appearance a n can then be synthesized by employing a piece-wise affine warp on each triangle patch appearance in the source image(see row2,column(b),of Figure2)so the appearance contained within s now aligns with the similarity normalized shape s n.3.1.3Shape Normalized Appearance,a0If we can remove all shape variation from an appearance, we’ll get a representation that can be called as shape nor-malized appearance,a0.a0can be synthesized in a similar fashion as a n was computed in section3.1.2,but instead ensuring the appearance contained within s now aligns with the base shape s0.We shall refer to this as the face’s canon-ical appearance(see row2,column(c),of Figure2for an example of this canonical appearance image)a0.3.2FeaturesBased on the AAM derived representations in Section3.1 we define three representations:S-PTS:similarity normalized shape s n representation(see Equation1)of the face and its facial features.Thereare68vertex points in s n for both x−and y−co-ordinates,resulting in a raw136dimensional featurevector.S-APP:similarity normalized appearance a n representa-tion.Due to the number of pixels in a n varying fromimage to image,we apply a mask based on s0so thatthe same number of pixels(approximately27,000)arein a n for each image.C-APP:canonical appearance a0representation where all shape variation has been removed from the sourceappearance except the base shape s0.This results in anapproximately27,000dimensional raw feature vectorbased on the pixel values within s0.The naming convention S-PTS,S-APP,and C-APP will be employed throughout the rest of this paper.4.SVM CLASSIFIERSSupport vector machines(SVMs)have been proven useful in a number of pattern recognition tasks including face and facial action recognition.Because they are binary classifiers, they are well suited to the task of Pain Vs No Pain classifica-tion.SVMs attempt tofind the hyper-plane that maximizes the margin between positive and negative observations for a specified class.A linear SVM classification decision is made for an unlabeled test observation x∗by,w T x∗true≷falseb(2)where w is the vector normal to the separating hyperplane and b is the bias.Both w and b are estimated so that they minimize the structural risk of a train-set,thus avoiding the possibility of overfitting to the training data.Typically,w is not defined explicitly,but through a linear sum of support vectors.As a result SVMs offer additional appeal as they allow for the employment of non-linear combination func-tions through the use of kernel functions,such as the radial basis function(RBF),polynomial and sigmoid kernels.A linear kernel was used in our experiments due to its ability to generalize well to unseen data in many pattern recogni-tion tasks[13].Please refer to[13]for additional information on SVM estimation and kernel selection.Figure 2:Example of AAM Derived Representations (a)Top row :input shape(s),Bottom row :input image,(b)Top row:Similarity Normalized Shape(s n ),Bottom Row:Similarity Normalized Appearance(a n ),(c)Top Row:Base Shape(s 0),Bottom Row:Shape Normalized Appearance(a 0)Frame # 10 Frame # 20 Frame # 29 Frame # 150 Frame # 300(a)0.911.11.21.3(b)Figure 3:Example of Video Sequence Prediction (a)Sample frames from a pain-video sequence with theirframe indices,(b)Scores for individual frames.Points corresponding to the frames shown in (a)are high-lighted.For the example sequence shown,a cumulative score D sequence =T i =1d i =195was computed and compared to the derived equal error threshold of −3(as explained in Section 5.2)to yield an output decision of ‘Pain’5.EXPERIMENTS5.1Pain Model LearningTo ascertain the utility of various AAM representations, different classifiers were trained by using features of Sec-tion3.2in the following combinations:S-PTS:similarity normalized shape s nS-APP:similarity normalized appearance a nC-APP+S-PTS:canonical appearance a0combined with the similarity normalized shape s nTo check for subject generalization,a leave-one-subject-out strategy was employed for cross validation.Thus,there was no overlap of subjects between the training and test-ing set.The number of training frames from all the video sequences was prohibitively large to train an SVM,as the training time complexity for SVM is O(m3),where m is the number of training examples.In order to make the step of model learning practical,while making the best use of training data,each video sequence wasfirst clustered into a preset number of clusters.Standard K-Means clustering algorithm was used,with K set to a value that reduces the training set to a size manageable by SVM.Linear SVM training models were learned by by itera-tively leaving one subject out,which gives rise to N number of models,where N is the number of subjects.Since the ground truth was available at the level of an entire video sequence,all the clusters belonging to a pain containing se-quence were considered as positive(pain)examples,while clusters belonging to‘no pain’sequence were counted as neg-ative(no pain)training examples.5.2Video Sequence PredictionWhile learning was done on clustered video frames,testing was carried out on individual frames.The output for every frame was a score proportional to the distance of test-point from the separating hyperplane.In order to predict a video as a pain sequence or not,the output scores of individual frames were summed together to give a cumulative score for the entire sequence:D sequence=Ti=1d i(3)where d i is the output score for the i th frame and T is the total number of frames in the sequence.Having computed the sequence level cumulative score in Equation3,we seek a decision rule of the form:D sequencepain≷no painT hreshold(4)Using this decision rule,a threshold was set such that false accept rate equaled false reject rate(i.e.,equal error rate point was computed).Output scores for a sample se-quence along with the implementation of decision rule is shown in Figure3.The score values track the pain expres-sion,with a peak response corresponding to frame29shown in Figure3(a).Features Hit Rate0.2899S-APP0.46550.1879Table1:Results of experiments performed in sec-tion 5.Column1indicates the features used for training,Columns2and3represent the correspond-ing Equal-Error and Hit Rates respectively6.RESULTSTable1shows equal-error rates(EER)using each of the representations.It is intuitively satisfying that the results highlight the importance of shape features for pain expres-sion.The best results(EER=0.1879,Hit Rate:0.8121) are for canonical appearance combined with similarity nor-malized shape(C-APP+S-PTS).This result is consistent with our previous work[14],in which we used AAMs for facial action unit recognition.It is surprising,however,that similarity normalized appear-ance features(S-APP)performed at close-to-chance levels despite the fact that this representation can be fully derived from canonical appearance and similarity normalized shape. S-PTS combined with C-APP may add additional dimen-sions that aid infinding an optimal separating hyperplane between two classes.7.DISCUSSIONIn this paper,we have explored various face representa-tions derived from AAMs for recognizing facial expression of pain.We have demonstrated the utility of AAM represen-tations for the task of pain-no pain classification.We have also presented a method to handle large training data when learning SVM models.Some conclusions of our experiments are:•Use of concatenated similarity normalized shape andshape normalized appearance(S-PTS+C-APP)is su-perior to either similarity normalized shape(S-PTS)orsimilarity normalized appearance(S-APP)alone.•Shape features have an important role to play in ex-pression recognition generally,and pain detection par-ticularly.•Automatic pain detection through video appears tobe a feasible task.Thisfinding suggests an oppor-tunity to build interactive systems to detect pain indiverse populations,including infants and speech im-paired adults.Additional applications for system de-velopment include pain-triggered automatic drug dis-pensation and discriminating between feigned and realpain.•Two limitations may be noted.One was the exclu-sion of subjects wearing glasses or having facial hair,which limits generalizability.A second limitation wasthe availability of ground-truth at the level of videorecorded tests instead of the level of individual frames.Not all frames in a pain sequence actually correspondto pain.Providing a frame-level ground-truth couldassist the model-learning step and thus improve recog-nition performance.This limitation can be overcomeeither by expert labeling of frames or by automatic re-finement of ground-truth by exploiting the information embedded in no-pain video sequences.Incorporating temporal information is also expected to positively in-fluence the performance.Based on our initialfindings, further improvements on above lines can pave way to-wards developing automatic pain detection tools for clinical use.8.ACKNOWLEDGMENTSThis research was supported by CIHR grant MOP77799 and NIMH grant MH514359.REFERENCES[1]M.Bartlett,G.Littlewort,inscesk,I.Fasel,andJ.Movellan.Machine learning methods for fullyautomatic recognition of facial expressions and facialactions.IEEE International Conference on Systems,Man and Cybernetics,pages592–597,October2004.[2]M.S.Bartlett,G.Littlewort,M.Frank,inscesk,I.Fasel,and J.Movellan.Recognizing facialexpression:Machine learning and application tospontaneous behavior.IEEE Conference on Computer Vision and Pattern Recognition(CVPR),2:568–573,June2005.[3]M.S.Bartlett,G.Littlewort,M.Frank,inscsek,I.Fasel,and J.Movellan.Fully automatic facial actionrecognition in spontaneous behavior.Proceedings ofthe IEEE International Conference on Automatic Face and Gesture Recognition,pages223–228,2006.[4]J.F.Cohn,Z.Ambadar,and P.Ekman.Observer-based measurement of facial expression with the facial action coding system.In J.A.Coan andJ.B.Allen,editors,The handbook of emotionelicitation and assessment.Oxford University PressSeries in Affective Science,pages203–221.OxfordUniversity Press,New York,NY,2007.[5]J.F.Cohn and K.L.Schmidt.The timing of facialmotion in posed and spontaneous smiles.International Journal of Wavelets,Multiresolution and Information Processing,2:1–12,2004.[6]T.Cootes,G.Edwards,and C.Taylor.Activeappearance models.PAMI,23(6):681–685,2001. [7]R.R.Cornelius.The science of emotion.PrenticeHall,Upper Saddler River,New Jersey,1996.[8]K.D.Craig,K.M.Prkachin,and R.V.E.Grunau.The facial expression of pain.In D.C.Turk andR.M.,editors,Handbook of pain assessment.Guilford, New York,2nd edition,2001.[9]A.C.d.C.Williams,H.T.O.Davies,andY.Chadury.Simple pain rating scales hide complexidiosyncratic meanings.Pain,85:457–463.[10]R.O.Duda,P.E.Hart,and D.G.Stork.PatternClassification.John Wiley and Sons,Inc.,New York, NY,USA,2nd edition,2001.[11]P.Ekman,W.V.Friesen,and J.C.hager.Facialaction coding system:Research workResearch Information,Salt Lake City,UT,2002. [12]T.Hadjistavropoulos and K.D.Craig.Socialinfluences and the communication of pain.In Pain:Psychological perspectives,pages87–112.Erlbaum,New York,2004.[13]C.W.Hsu,C.C.Chang,and C.J.Lin.A practicalguide to support vector classification.TechnicalReport,2005.[14]S.Lucey,A.B.Ashraf,and J.Cohn.Investigatingspontaneous facial action recognition through AAMrepresentations of the face.In K.Kurihara,editor,Face Recognition Book.Pro Literatur Verlag,Mammendorf,Germany,April2007.[15]I.Matthews and S.Baker.Active appearance modelsrevisited.IJCV,60(2):135–164,2004.[16]M.Pantic,A.Pentland,A.Niholt,and T.S.Huang.Human computing and machine understanding ofhuman behavior:A survey.Proceedings of the ACMInternational Conference on Multimodal Interfaces,2006.[17]Y.Tian,J.F.Cohn,and T.Kanade.Facial expressionanalysis.In S.Z.Li and A.K.Jain,editors,Handbook of face recognition,pages247–276.Springer,NewYork,NY,2005.[18]M.F.Valstar,M.Pantic,Z.Ambadar,and J.F.Cohn.Spontaneous vs.posed facial behavior:Automatic analysis of brow actions.Proceedings of the ACM International Conference on MultimodalInterfaces,pages162–170,November2006.[19]J.Xiao,S.Baker,I.Matthews,and T.Kanade.(Inpress).2d vs.3d deformable face models:Representational power,construction,and real-timefitting.International Journal of Computer Vision,Springer Science.。