DataStructureGraphrepresentation
基于柯西-施瓦茨不等式的知识图谱稠密表示方法
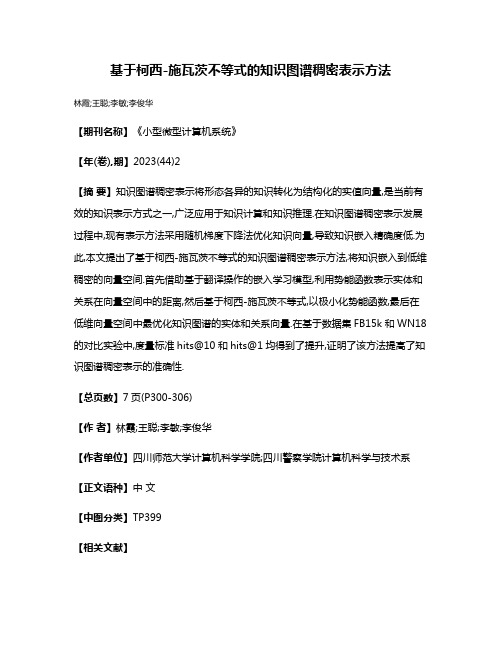
基于柯西-施瓦茨不等式的知识图谱稠密表示方法
林霞;王聪;李敏;李俊华
【期刊名称】《小型微型计算机系统》
【年(卷),期】2023(44)2
【摘要】知识图谱稠密表示将形态各异的知识转化为结构化的实值向量,是当前有效的知识表示方式之一,广泛应用于知识计算和知识推理.在知识图谱稠密表示发展过程中,现有表示方法采用随机梯度下降法优化知识向量,导致知识嵌入精确度低.为此,本文提出了基于柯西-施瓦茨不等式的知识图谱稠密表示方法,将知识嵌入到低维稠密的向量空间.首先借助基于翻译操作的嵌入学习模型,利用势能函数表示实体和关系在向量空间中的距离,然后基于柯西-施瓦茨不等式,以极小化势能函数,最后在低维向量空间中最优化知识图谱的实体和关系向量.在基于数据集FB15k和WN18的对比实验中,度量标准hits@10和hits@1均得到了提升,证明了该方法提高了知识图谱稠密表示的准确性.
【总页数】7页(P300-306)
【作者】林霞;王聪;李敏;李俊华
【作者单位】四川师范大学计算机科学学院;四川警察学院计算机科学与技术系【正文语种】中文
【中图分类】TP399
【相关文献】
1.柯西-施瓦茨不等式的三种证明
2.柯西不等式与施瓦茨不等式的推广
3.基于柯西-施瓦茨散度的多目标跟踪中的传感器选择
4.关于柯西-施瓦茨不等式证明
5.算子的柯西-施瓦茨范数不等式的改进
因版权原因,仅展示原文概要,查看原文内容请购买。
人工智能领域中英文专有名词汇总
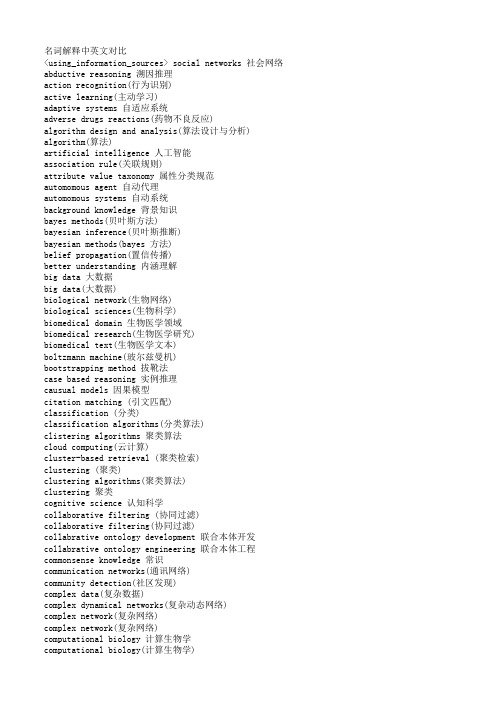
名词解释中英文对比<using_information_sources> social networks 社会网络abductive reasoning 溯因推理action recognition(行为识别)active learning(主动学习)adaptive systems 自适应系统adverse drugs reactions(药物不良反应)algorithm design and analysis(算法设计与分析) algorithm(算法)artificial intelligence 人工智能association rule(关联规则)attribute value taxonomy 属性分类规范automomous agent 自动代理automomous systems 自动系统background knowledge 背景知识bayes methods(贝叶斯方法)bayesian inference(贝叶斯推断)bayesian methods(bayes 方法)belief propagation(置信传播)better understanding 内涵理解big data 大数据big data(大数据)biological network(生物网络)biological sciences(生物科学)biomedical domain 生物医学领域biomedical research(生物医学研究)biomedical text(生物医学文本)boltzmann machine(玻尔兹曼机)bootstrapping method 拔靴法case based reasoning 实例推理causual models 因果模型citation matching (引文匹配)classification (分类)classification algorithms(分类算法)clistering algorithms 聚类算法cloud computing(云计算)cluster-based retrieval (聚类检索)clustering (聚类)clustering algorithms(聚类算法)clustering 聚类cognitive science 认知科学collaborative filtering (协同过滤)collaborative filtering(协同过滤)collabrative ontology development 联合本体开发collabrative ontology engineering 联合本体工程commonsense knowledge 常识communication networks(通讯网络)community detection(社区发现)complex data(复杂数据)complex dynamical networks(复杂动态网络)complex network(复杂网络)complex network(复杂网络)computational biology 计算生物学computational biology(计算生物学)computational complexity(计算复杂性) computational intelligence 智能计算computational modeling(计算模型)computer animation(计算机动画)computer networks(计算机网络)computer science 计算机科学concept clustering 概念聚类concept formation 概念形成concept learning 概念学习concept map 概念图concept model 概念模型concept modelling 概念模型conceptual model 概念模型conditional random field(条件随机场模型) conjunctive quries 合取查询constrained least squares (约束最小二乘) convex programming(凸规划)convolutional neural networks(卷积神经网络) customer relationship management(客户关系管理) data analysis(数据分析)data analysis(数据分析)data center(数据中心)data clustering (数据聚类)data compression(数据压缩)data envelopment analysis (数据包络分析)data fusion 数据融合data generation(数据生成)data handling(数据处理)data hierarchy (数据层次)data integration(数据整合)data integrity 数据完整性data intensive computing(数据密集型计算)data management 数据管理data management(数据管理)data management(数据管理)data miningdata mining 数据挖掘data model 数据模型data models(数据模型)data partitioning 数据划分data point(数据点)data privacy(数据隐私)data security(数据安全)data stream(数据流)data streams(数据流)data structure( 数据结构)data structure(数据结构)data visualisation(数据可视化)data visualization 数据可视化data visualization(数据可视化)data warehouse(数据仓库)data warehouses(数据仓库)data warehousing(数据仓库)database management systems(数据库管理系统)database management(数据库管理)date interlinking 日期互联date linking 日期链接Decision analysis(决策分析)decision maker 决策者decision making (决策)decision models 决策模型decision models 决策模型decision rule 决策规则decision support system 决策支持系统decision support systems (决策支持系统) decision tree(决策树)decission tree 决策树deep belief network(深度信念网络)deep learning(深度学习)defult reasoning 默认推理density estimation(密度估计)design methodology 设计方法论dimension reduction(降维) dimensionality reduction(降维)directed graph(有向图)disaster management 灾害管理disastrous event(灾难性事件)discovery(知识发现)dissimilarity (相异性)distributed databases 分布式数据库distributed databases(分布式数据库) distributed query 分布式查询document clustering (文档聚类)domain experts 领域专家domain knowledge 领域知识domain specific language 领域专用语言dynamic databases(动态数据库)dynamic logic 动态逻辑dynamic network(动态网络)dynamic system(动态系统)earth mover's distance(EMD 距离) education 教育efficient algorithm(有效算法)electric commerce 电子商务electronic health records(电子健康档案) entity disambiguation 实体消歧entity recognition 实体识别entity recognition(实体识别)entity resolution 实体解析event detection 事件检测event detection(事件检测)event extraction 事件抽取event identificaton 事件识别exhaustive indexing 完整索引expert system 专家系统expert systems(专家系统)explanation based learning 解释学习factor graph(因子图)feature extraction 特征提取feature extraction(特征提取)feature extraction(特征提取)feature selection (特征选择)feature selection 特征选择feature selection(特征选择)feature space 特征空间first order logic 一阶逻辑formal logic 形式逻辑formal meaning prepresentation 形式意义表示formal semantics 形式语义formal specification 形式描述frame based system 框为本的系统frequent itemsets(频繁项目集)frequent pattern(频繁模式)fuzzy clustering (模糊聚类)fuzzy clustering (模糊聚类)fuzzy clustering (模糊聚类)fuzzy data mining(模糊数据挖掘)fuzzy logic 模糊逻辑fuzzy set theory(模糊集合论)fuzzy set(模糊集)fuzzy sets 模糊集合fuzzy systems 模糊系统gaussian processes(高斯过程)gene expression data 基因表达数据gene expression(基因表达)generative model(生成模型)generative model(生成模型)genetic algorithm 遗传算法genome wide association study(全基因组关联分析) graph classification(图分类)graph classification(图分类)graph clustering(图聚类)graph data(图数据)graph data(图形数据)graph database 图数据库graph database(图数据库)graph mining(图挖掘)graph mining(图挖掘)graph partitioning 图划分graph query 图查询graph structure(图结构)graph theory(图论)graph theory(图论)graph theory(图论)graph theroy 图论graph visualization(图形可视化)graphical user interface 图形用户界面graphical user interfaces(图形用户界面)health care 卫生保健health care(卫生保健)heterogeneous data source 异构数据源heterogeneous data(异构数据)heterogeneous database 异构数据库heterogeneous information network(异构信息网络) heterogeneous network(异构网络)heterogenous ontology 异构本体heuristic rule 启发式规则hidden markov model(隐马尔可夫模型)hidden markov model(隐马尔可夫模型)hidden markov models(隐马尔可夫模型) hierarchical clustering (层次聚类) homogeneous network(同构网络)human centered computing 人机交互技术human computer interaction 人机交互human interaction 人机交互human robot interaction 人机交互image classification(图像分类)image clustering (图像聚类)image mining( 图像挖掘)image reconstruction(图像重建)image retrieval (图像检索)image segmentation(图像分割)inconsistent ontology 本体不一致incremental learning(增量学习)inductive learning (归纳学习)inference mechanisms 推理机制inference mechanisms(推理机制)inference rule 推理规则information cascades(信息追随)information diffusion(信息扩散)information extraction 信息提取information filtering(信息过滤)information filtering(信息过滤)information integration(信息集成)information network analysis(信息网络分析) information network mining(信息网络挖掘) information network(信息网络)information processing 信息处理information processing 信息处理information resource management (信息资源管理) information retrieval models(信息检索模型) information retrieval 信息检索information retrieval(信息检索)information retrieval(信息检索)information science 情报科学information sources 信息源information system( 信息系统)information system(信息系统)information technology(信息技术)information visualization(信息可视化)instance matching 实例匹配intelligent assistant 智能辅助intelligent systems 智能系统interaction network(交互网络)interactive visualization(交互式可视化)kernel function(核函数)kernel operator (核算子)keyword search(关键字检索)knowledege reuse 知识再利用knowledgeknowledgeknowledge acquisitionknowledge base 知识库knowledge based system 知识系统knowledge building 知识建构knowledge capture 知识获取knowledge construction 知识建构knowledge discovery(知识发现)knowledge extraction 知识提取knowledge fusion 知识融合knowledge integrationknowledge management systems 知识管理系统knowledge management 知识管理knowledge management(知识管理)knowledge model 知识模型knowledge reasoningknowledge representationknowledge representation(知识表达) knowledge sharing 知识共享knowledge storageknowledge technology 知识技术knowledge verification 知识验证language model(语言模型)language modeling approach(语言模型方法) large graph(大图)large graph(大图)learning(无监督学习)life science 生命科学linear programming(线性规划)link analysis (链接分析)link prediction(链接预测)link prediction(链接预测)link prediction(链接预测)linked data(关联数据)location based service(基于位置的服务) loclation based services(基于位置的服务) logic programming 逻辑编程logical implication 逻辑蕴涵logistic regression(logistic 回归)machine learning 机器学习machine translation(机器翻译)management system(管理系统)management( 知识管理)manifold learning(流形学习)markov chains 马尔可夫链markov processes(马尔可夫过程)matching function 匹配函数matrix decomposition(矩阵分解)matrix decomposition(矩阵分解)maximum likelihood estimation(最大似然估计)medical research(医学研究)mixture of gaussians(混合高斯模型)mobile computing(移动计算)multi agnet systems 多智能体系统multiagent systems 多智能体系统multimedia 多媒体natural language processing 自然语言处理natural language processing(自然语言处理) nearest neighbor (近邻)network analysis( 网络分析)network analysis(网络分析)network analysis(网络分析)network formation(组网)network structure(网络结构)network theory(网络理论)network topology(网络拓扑)network visualization(网络可视化)neural network(神经网络)neural networks (神经网络)neural networks(神经网络)nonlinear dynamics(非线性动力学)nonmonotonic reasoning 非单调推理nonnegative matrix factorization (非负矩阵分解) nonnegative matrix factorization(非负矩阵分解) object detection(目标检测)object oriented 面向对象object recognition(目标识别)object recognition(目标识别)online community(网络社区)online social network(在线社交网络)online social networks(在线社交网络)ontology alignment 本体映射ontology development 本体开发ontology engineering 本体工程ontology evolution 本体演化ontology extraction 本体抽取ontology interoperablity 互用性本体ontology language 本体语言ontology mapping 本体映射ontology matching 本体匹配ontology versioning 本体版本ontology 本体论open government data 政府公开数据opinion analysis(舆情分析)opinion mining(意见挖掘)opinion mining(意见挖掘)outlier detection(孤立点检测)parallel processing(并行处理)patient care(病人医疗护理)pattern classification(模式分类)pattern matching(模式匹配)pattern mining(模式挖掘)pattern recognition 模式识别pattern recognition(模式识别)pattern recognition(模式识别)personal data(个人数据)prediction algorithms(预测算法)predictive model 预测模型predictive models(预测模型)privacy preservation(隐私保护)probabilistic logic(概率逻辑)probabilistic logic(概率逻辑)probabilistic model(概率模型)probabilistic model(概率模型)probability distribution(概率分布)probability distribution(概率分布)project management(项目管理)pruning technique(修剪技术)quality management 质量管理query expansion(查询扩展)query language 查询语言query language(查询语言)query processing(查询处理)query rewrite 查询重写question answering system 问答系统random forest(随机森林)random graph(随机图)random processes(随机过程)random walk(随机游走)range query(范围查询)RDF database 资源描述框架数据库RDF query 资源描述框架查询RDF repository 资源描述框架存储库RDF storge 资源描述框架存储real time(实时)recommender system(推荐系统)recommender system(推荐系统)recommender systems 推荐系统recommender systems(推荐系统)record linkage 记录链接recurrent neural network(递归神经网络) regression(回归)reinforcement learning 强化学习reinforcement learning(强化学习)relation extraction 关系抽取relational database 关系数据库relational learning 关系学习relevance feedback (相关反馈)resource description framework 资源描述框架restricted boltzmann machines(受限玻尔兹曼机) retrieval models(检索模型)rough set theroy 粗糙集理论rough set 粗糙集rule based system 基于规则系统rule based 基于规则rule induction (规则归纳)rule learning (规则学习)rule learning 规则学习schema mapping 模式映射schema matching 模式匹配scientific domain 科学域search problems(搜索问题)semantic (web) technology 语义技术semantic analysis 语义分析semantic annotation 语义标注semantic computing 语义计算semantic integration 语义集成semantic interpretation 语义解释semantic model 语义模型semantic network 语义网络semantic relatedness 语义相关性semantic relation learning 语义关系学习semantic search 语义检索semantic similarity 语义相似度semantic similarity(语义相似度)semantic web rule language 语义网规则语言semantic web 语义网semantic web(语义网)semantic workflow 语义工作流semi supervised learning(半监督学习)sensor data(传感器数据)sensor networks(传感器网络)sentiment analysis(情感分析)sentiment analysis(情感分析)sequential pattern(序列模式)service oriented architecture 面向服务的体系结构shortest path(最短路径)similar kernel function(相似核函数)similarity measure(相似性度量)similarity relationship (相似关系)similarity search(相似搜索)similarity(相似性)situation aware 情境感知social behavior(社交行为)social influence(社会影响)social interaction(社交互动)social interaction(社交互动)social learning(社会学习)social life networks(社交生活网络)social machine 社交机器social media(社交媒体)social media(社交媒体)social media(社交媒体)social network analysis 社会网络分析social network analysis(社交网络分析)social network(社交网络)social network(社交网络)social science(社会科学)social tagging system(社交标签系统)social tagging(社交标签)social web(社交网页)sparse coding(稀疏编码)sparse matrices(稀疏矩阵)sparse representation(稀疏表示)spatial database(空间数据库)spatial reasoning 空间推理statistical analysis(统计分析)statistical model 统计模型string matching(串匹配)structural risk minimization (结构风险最小化) structured data 结构化数据subgraph matching 子图匹配subspace clustering(子空间聚类)supervised learning( 有support vector machine 支持向量机support vector machines(支持向量机)system dynamics(系统动力学)tag recommendation(标签推荐)taxonmy induction 感应规范temporal logic 时态逻辑temporal reasoning 时序推理text analysis(文本分析)text anaylsis 文本分析text classification (文本分类)text data(文本数据)text mining technique(文本挖掘技术)text mining 文本挖掘text mining(文本挖掘)text summarization(文本摘要)thesaurus alignment 同义对齐time frequency analysis(时频分析)time series analysis( 时time series data(时间序列数据)time series data(时间序列数据)time series(时间序列)topic model(主题模型)topic modeling(主题模型)transfer learning 迁移学习triple store 三元组存储uncertainty reasoning 不精确推理undirected graph(无向图)unified modeling language 统一建模语言unsupervisedupper bound(上界)user behavior(用户行为)user generated content(用户生成内容)utility mining(效用挖掘)visual analytics(可视化分析)visual content(视觉内容)visual representation(视觉表征)visualisation(可视化)visualization technique(可视化技术) visualization tool(可视化工具)web 2.0(网络2.0)web forum(web 论坛)web mining(网络挖掘)web of data 数据网web ontology lanuage 网络本体语言web pages(web 页面)web resource 网络资源web science 万维科学web search (网络检索)web usage mining(web 使用挖掘)wireless networks 无线网络world knowledge 世界知识world wide web 万维网world wide web(万维网)xml database 可扩展标志语言数据库附录 2 Data Mining 知识图谱(共包含二级节点15 个,三级节点93 个)间序列分析)监督学习)领域 二级分类 三级分类。
储层表征
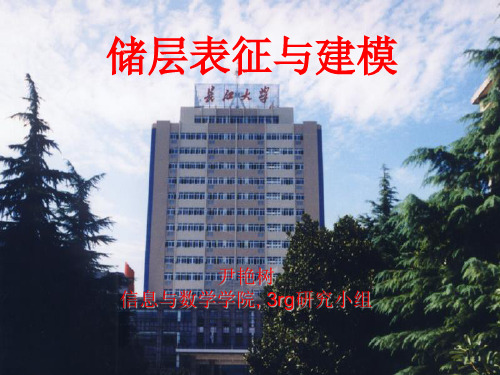
2、流动单元模型 概 念 : (C.L.Hearn etc 1984 ; W.J.Ebanks,1987) 影响流体流动的储层属性参数在 各处相似,且岩层特点也相似的 纵、横向连续的储集带单元。 流动单元不同,流体流动特征也 不同。 流动单元模型: •由许多流动单元块体镶嵌组合 而成,离散模型 •包括:流动单元划分,流动单 元间边界、单元内储层属性相似 •对油藏模拟及动态分析有很大 意义,对预测二次采油和三次采 油的生产性能亦意义重大。
胜坨油田胜二 区74小层不同 含水期渗透率 实现对比图
胜坨油 田胜二 区沙二 段74小 层不同 含水期 含油饱 和度模 型
内容提要
一、储层表征的概念
二、储层模型的分类 三、储层建模的概念 四、地质统计学基础知识 五、随机建模方法简介
六、随机建模步骤、策略
建模的目的
白化过程
测井信息与解释
地质信息与解释
油藏地质建模 是油藏描述的核心。
Reservoir description Reservoir characterization
 ¤Í ï Â Ï é ³ ±Ó Ì ³ 3¶ ¿ K2t1-K 2cÓ ² Æ Ã Í Í Ø Ê æ ¼
储层表征(Reservoir Characterization) 是由油藏描述(Reservoir Description) 向定量化方向发展演化出来的
1988年,SPE苏格兰会议,模拟是否有实际意义讨论
1991年,SPE科罗拉多会议,肯定方法,讨论方法适用性 2000年,Strebelle,多点地质统计学 国内 《国外储层建模技术》,原中国石油天然气总公司
1991年,裘怿楠教授 ,“储层地质模型”,石油学 报
内容提要
一、储层表征的概念
IT常用英文词汇

第一部分、计算机算法常用术语中英对照Data Structures 基本数据结构?Dictionaries 字典?Priority Queues 堆?Graph Data Structures 图?Set Data Structures 集合?Kd-Trees 线段树?Numerical Problems 数值问题?Solving Linear Equations 线性方程组?Bandwidth Reduction 带宽压缩?Matrix Multiplication 矩阵乘法?Determinants and Permanents 行列式?Constrained and Unconstrained Optimization 最值问题? Linear Programming 线性规划?Random Number Generation 随机数生成?Factoring and Primality Testing 因子分解/质数判定? Arbitrary Precision Arithmetic 高精度计算?Knapsack Problem 背包问题? Discrete Fourier Transform 离散Fourier变换? Combinatorial Problems 组合问题?Sorting 排序?Searching 查找?Median and Selection 中位数?Generating Permutations 排列生成? Generating Subsets 子集生成?Generating Partitions 划分生成?Generating Graphs 图的生成?Calendrical Calculations 日期?Job Scheduling 工程安排?Satisfiability 可满足性?Graph Problems -- polynomial 图论-多项式算法? Connected Components 连通分支? Topological Sorting 拓扑排序?Minimum Spanning Tree 最小生成树?Shortest Path 最短路径?Transitive Closure and Reduction 传递闭包?Matching 匹配?Eulerian Cycle / Chinese Postman Euler回路/中国邮路? Edge and Vertex Connectivity 割边/割点?Network Flow 网络流?Drawing Graphs Nicely 图的描绘?Drawing Trees 树的描绘?Planarity Detection and Embedding 平面性检测和嵌入? Graph Problems -- hard 图论-NP问题?Clique 最大团?Independent Set 独立集?Vertex Cover 点覆盖?Traveling Salesman Problem 旅行商问题? Hamiltonian Cycle Hamilton回路?Graph Partition 图的划分?Vertex Coloring 点染色?Edge Coloring 边染色?Graph Isomorphism 同构?Steiner Tree Steiner树? Feedback Edge/Vertex Set 最大无环子图? Computational Geometry 计算几何? Convex Hull 凸包?Triangulation 三角剖分?Voronoi Diagrams Voronoi图?Nearest Neighbor Search 最近点对查询? Range Search 范围查询?Point Location 位置查询?Intersection Detection 碰撞测试?Bin Packing 装箱问题?Medial-Axis Transformation 中轴变换? Polygon Partitioning 多边形分割? Simplifying Polygons 多边形化简?Shape Similarity 相似多边形?Motion Planning 运动规划?Maintaining Line Arrangements 平面分割? Minkowski Sum Minkowski和?Set and String Problems 集合与串的问题?Set Cover 集合覆盖?Set Packing 集合配置?String Matching 模式匹配?Approximate String Matching 模糊匹配?Text Compression 压缩?Cryptography 密码?Finite State Machine Minimization 有穷自动机简化? Longest Common Substring 最长公共子串? Shortest Common Superstring 最短公共父串? DP——Dynamic Programming——动态规划? recursion ——递归?第二部分、编程词汇?A2A integration A2A整合?abstract 抽象的?abstract base class (ABC)抽象基类?abstract class 抽象类?abstraction 抽象、抽象物、抽象性? access 存取、访问?access level访问级别?access function 访问函数?account 账户?action 动作?activate 激活?active 活动的?actual parameter 实参?adapter 适配器?add-in 插件?address 地址?address space 地址空间?address-of operator 取地址操作符?ADL (argument-dependent lookup)?ADO(ActiveX Data Object)ActiveX数据对象? advancedaggregation 聚合、聚集?algorithm 算法?alias 别名?align 排列、对齐?allocate 分配、配置?allocator分配器、配置器?angle bracket 尖括号?annotation 注解、评注?API (Application Programming Interface) 应用(程序)编程接口?app domain (application domain)应用域?application 应用、应用程序?application framework 应用程序框架?appearance 外观?append 附加?architecture 架构、体系结构?archive file 归档文件、存档文件?argument引数(传给函式的值)。
结构化数据 向量表征
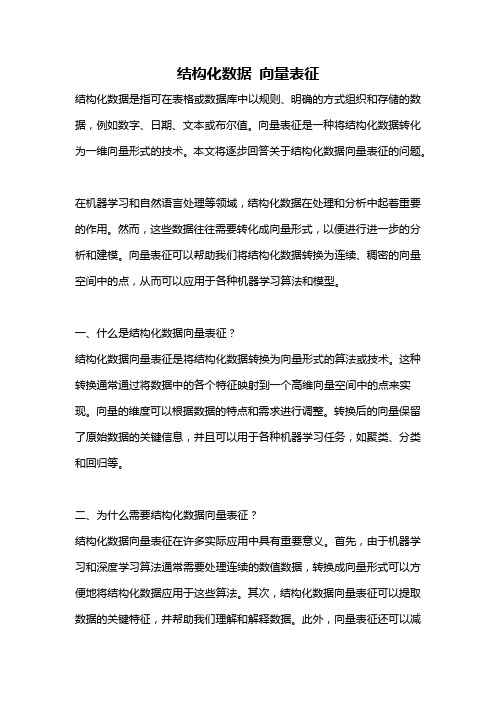
结构化数据向量表征结构化数据是指可在表格或数据库中以规则、明确的方式组织和存储的数据,例如数字、日期、文本或布尔值。
向量表征是一种将结构化数据转化为一维向量形式的技术。
本文将逐步回答关于结构化数据向量表征的问题。
在机器学习和自然语言处理等领域,结构化数据在处理和分析中起着重要的作用。
然而,这些数据往往需要转化成向量形式,以便进行进一步的分析和建模。
向量表征可以帮助我们将结构化数据转换为连续、稠密的向量空间中的点,从而可以应用于各种机器学习算法和模型。
一、什么是结构化数据向量表征?结构化数据向量表征是将结构化数据转换为向量形式的算法或技术。
这种转换通常通过将数据中的各个特征映射到一个高维向量空间中的点来实现。
向量的维度可以根据数据的特点和需求进行调整。
转换后的向量保留了原始数据的关键信息,并且可以用于各种机器学习任务,如聚类、分类和回归等。
二、为什么需要结构化数据向量表征?结构化数据向量表征在许多实际应用中具有重要意义。
首先,由于机器学习和深度学习算法通常需要处理连续的数值数据,转换成向量形式可以方便地将结构化数据应用于这些算法。
其次,结构化数据向量表征可以提取数据的关键特征,并帮助我们理解和解释数据。
此外,向量表征还可以减少数据存储和计算的开销,提高模型的训练和预测效率。
三、常用的结构化数据向量表征方法有哪些?1. One-hot编码:这是一种最常见且简单的向量表征方法。
对于具有离散取值的特征,我们可以使用One-hot编码将每个取值映射为一个二进制向量,向量中只有相应取值的位置为1,其余位置为0。
这样可以将特征转换为一个稀疏的向量。
2. 词袋模型:这是一种常用的文本特征向量表征方法。
它将文本中的词语作为特征,并统计每个词语在文本中出现的次数或频率。
通过构建词汇表,将每个词语映射为一个维度较大的向量,每个维度表示该词语在文本中出现的次数或频率。
3. 嵌入模型:这是一种将离散的结构化数据映射到连续空间的向量表征方法。
2 知识图谱表示与建模
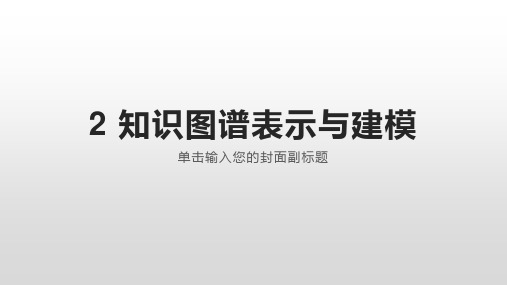
2.2.1 一阶谓词逻辑
● 1.一阶谓词逻辑优点 ● ●结构性。能把事物的属性以及事物间的各种语义联想显式地表示出来。 ● ●严密性。有形式化的语法和语义,以及相关的推理规则。 ● ●可实现性。可以转换为计算机内部形式,以便用算法实现。 ● 2.一阶谓词逻辑缺点 ● ●有限的可用性。一阶逻辑的逻辑归结只是半可判定性的。 ● ●无法表示不确定性知识。
2.2.2 霍恩子句和霍恩逻辑
● 霍恩子句(Horn Clause)得名于逻辑学家Alfred Horn[6]。 ● 一个子句是文字的析取。 ● 霍恩子句是带有最多一个肯定(positive)文字的子句,肯定文字指的是没有否定符号的文字。
例如,Øp1∨…∨Øpn∨ q是一个霍恩子句,它可以被等价地写为(p1∧…∧pn)→ q。Alfred Horn于1951年撰文指出这种子句的重要性。 ● 霍恩逻辑(Horn Logic)是一阶逻辑的子集。 ● 基于霍恩逻辑的知识库是一个霍恩规则的集合。 ● 一个霍恩规则由原子公式构成:B1∧…∧ Bn→ H, ● 其中H是头原子公式, B1,…,Bn是体原子公式。 ● 事实是霍恩规则的特例,它们是没有体原子公式且没有变量的霍恩规则。 ● 例如,→图灵奖得主(Tim Berners-Lee)是一个事实,可以简写为图灵奖得主(Tim BernersLee)。
组。 ● 由于所有的节点均通过联想弧彼此相连,语义网络可以通过图上的操作进行知识推理。
2.2.3 语义网络
● 1.语义网络的优点 ● 1)联想性。它最初是作为人类联想记忆模型提出来的。 ● 2)易用性。直观地把事物的属性及其语义联系表示出来,便于理解, ● 自然语言与语义网络的转换比较容易实现, ● 故语义网络表示法在自然语言理解系统中的应用最为广泛。 ● 3)结构性。语义网络是一种结构化的知识表示方法, ● 对数据子图特别有效。它能把事物的属性以及事物间的各种语义联想显式地表示出来。
1.通俗易懂解释知识图谱(KnowledgeGraph)

1.通俗易懂解释知识图谱(KnowledgeGraph)1. 前⾔从⼀开始的Google搜索,到现在的聊天机器⼈、⼤数据风控、证券投资、智能医疗、⾃适应教育、推荐系统,⽆⼀不跟知识图谱相关。
它在技术领域的热度也在逐年上升。
本⽂以通俗易懂的⽅式来讲解知识图谱相关的知识、尤其对从零开始搭建知识图谱过程当中需要经历的步骤以及每个阶段需要考虑的问题都给予了⽐较详细的解释。
知识图谱( Knowledge Graph)的概念由⾕歌2012年正式提出,旨在实现更智能的搜索引擎,并且于2013年以后开始在学术界和业界普及。
⽬前,随着智能信息服务应⽤的不断发展,知识图谱已被⼴泛应⽤于智能搜索、智能问答、个性化推荐、情报分析、反欺诈等领域。
另外,通过知识图谱能够将Web上的信息、数据以及链接关系聚集为知识,使信息资源更易于计算、理解以及评价,并且形成⼀套Web语义知识库。
知识图谱以其强⼤的语义处理能⼒与开放互联能⼒,可为万维⽹上的知识互联奠定扎实的基础,使Web 3.0提出的“知识之⽹”愿景成为了可能。
2. 知识图谱定义知识图谱:是结构化的语义知识库,⽤于迅速描述物理世界中的概念及其相互关系。
知识图谱通过对错综复杂的⽂档的数据进⾏有效的加⼯、处理、整合,转化为简单、清晰的“实体,关系,实体”的三元组,最后聚合⼤量知识,从⽽实现知识的快速响应和推理。
知识图谱有⾃顶向下和⾃底向上两种构建⽅式。
所谓⾃顶向下构建是借助百科类⽹站等结构化数据源,从⾼质量数据中提取本体和模式信息,加⼊到知识库中;所谓⾃底向上构建,则是借助⼀定的技术⼿段,从公开采集的数据中提取出资源模式,选择其中置信度较⾼的新模式,经⼈⼯审核之后,加⼊到知识库中。
看⼀张简单的知识图谱:如图所⽰,你可以看到,如果两个节点之间存在关系,他们就会被⼀条⽆向边连接在⼀起,那么这个节点,我们就称为实体(Entity),它们之间的这条边,我们就称为关系(Relationship)。
数据结构与算法 (2)

First started programming : have no ADT
–Writing the same code over and over
Data Sagtrauincture can be defined as:Exa•mAtpolemfiocr DADatTa: tahreecsoidneglteo arenadd the 1ea.Ak♠stcheWehcayAtebotoon•adohcmkAfAdwsoaafoanwtebrntwndinoadoatc-ihwha,ndmaiblsttatfiyetbauewcaihirspcstncahtheipaeotcdotaordeintimooaotniscforadnpcanofpnatoeotentaosdtprhidfriostmasaaetaheiatmystcahetlibopatokeidisrtlpcaenymedtatpogyeswedieepnesrdntaonanedhcadtttthaaapiiitesttsoneotayafai.ngaardndntresoseaeddt,,dthaaaaet.ar
bank toopdeertaetrimoninse tellers.
1-3 Model for an Abstract Data Type
In this section we provide a conceptual model for an Abstract Data Type (ADT).
t2rienyel.eApvwl–l♠adb3aiasqmoAene2raittsuehlbit7tevoeeo1ewa––s6{s•idauniut6rxdnFAt+Aens8mT123rneitetaboesuoasra,eegsn~s...th:r-mtinncefmhnanp,tti3DEDtaon*stcatoatyaeioipci,2ihtotpntgfeectc/ncisihotls,o…o7nnmeceuasccohiisienlfdmnn:6mtailliil(pneeaaeoscos}sgasg7ppruikrdhegrra:ioecfcattf}ssraaelaurhr,enadiaomintuiCcshmdudmttaalitsetrsaiipdagtleauooaatdtcfyabfrl:opncieetoieoiirannnnbtsaptvootatlioadmarrhaefuilgntte-ieotonnmoozeambinstnfrette.pntfhaisffheyhuetediwobdesto{tenrapdeo)eyoim-lenoneouasdgnbeapptfdtinfohricbamistotte.riedtainhahapaotteneotr.ipauthtkeaoetiagfrkathTltserndesretoaedlaeiahtsmniroapnyna.te,taenitepeatosanriasneusannod.t.ctfatsiohoatinoasnssa
- 1、下载文档前请自行甄别文档内容的完整性,平台不提供额外的编辑、内容补充、找答案等附加服务。
- 2、"仅部分预览"的文档,不可在线预览部分如存在完整性等问题,可反馈申请退款(可完整预览的文档不适用该条件!)。
- 3、如文档侵犯您的权益,请联系客服反馈,我们会尽快为您处理(人工客服工作时间:9:00-18:30)。
Root at 3
1 3
Back edge and cross edge 2
6
5
9
10
4
5
0
4 1
3
1
2
3
4
8
9
7 8
6 5
7 6
3
7
2
6
4
8
1
7
5
9
10
0
8
9
2
dfn() and low()
Observation
– 若root有兩個以上child, 則為articulation point – 若vertex u有任一child w, 使得w及w後代無法透
visited[w->vertex] = TRUE;
}
}
} 例如:地毯式搜索
}
Connected component
Is it a connected graph?
– BFS(v) or DFS(v)
Find out connected component
void connected(void){ int i; for (i=0;i<n;i++){
過back edge到u的祖先, 則為 articulation point
low(u): u及後代,其back edge可達vertex之最 小dfn()
– low(u) = min{ dfn(u), min{low(w)|w是u的child}, min{dfn(w)|(u,w)是back edge}}
A example: dfs() and low()
1
3
Vertex 0 1 2 3 4 5 6 7 8 9
2
6
dfn 5 4 3 1 2 6 7 8 9 10 4
5
low 5 1 1 1 0
2
6
0
4 1
3
1
2
3
4 2
8
9
4
8
1
7
7
8
5
9
10
6
0
8
9
5
•請自行Trace Biconnected() 7 •Hint: 將Unvisited edge跟back edge送 6 入Stack, 到Articulation Point 再一次輸出
Definition: Biconnected graph
– 定義為無Articulation point的connected graph
Definition: Biconnected component
– Graph G中的Biconnected component H, 為G中最 大的biconnected subgraph; 最大是指G中沒有其 他subgraph是biconnected且包含入H
typedef struct node {
int vertex;
0
node_ptr link; } node;
1
2
node_ptr graph[MAX_VERTICES]; 3
int n = 0; /* number of nodes */
G1
312 230 130 012
Adjacency lists, by array
int marked; int vertex1; int vertex2; edge_ptr path1; edge_ptr path2; } edge; edge_ptr graph[MAX_VERTICES];
N1
0 2 N2 N3
N2
0 3 NIL N4
N3
1 2 N4 N5
N4
1 3 NIL N5
if(!visited[i]){ dfs(i); printf(“\n”);
} }
Spanning Tree
A spanning tree is a minimal subgraph
G’, such that V(G’)=V(G) and G’ is
connected
312
Weight and MST 0
N5
2 3 NIL NIL
Weighted edges
Cost Weight field Network
6.2 Elementary graph operations
Outlines
Operations similar to tree traversals
– Depth-First Search (DFS) – Breadth-First Search (BFS)
6.1.3 Graph representation
常見的表示法
Adjacency matrices Adjacency lists Adjacency multilists
0
4
2
15
6
3
7
G4
0
1
2
3
G1
0 1
2 G3
Adjacency matrix
n*n 陣列 n*(n-1),即 O(n2) If the matrix is sparse ?
queue_ptr front, rear;
typedef struct queue {
front=rear=NULL;
int vertex;
printf(“%5d”,v);
queue_ptr link;
visited[v]=TRUE;
};
addq(&front, &rear, v);
void addq(queue_ptr *, queue_ptr *, int);
A connected graph and its biconnected components
0
8
9
0
1
7
1
2
3
5
4
6
1
8 9
7 7
7
2
33
55
4
6
為何沒有任一個邊可能存在於兩個或多個biconnected component中?
DFS spanning tree of the graph in 6.19(a)
Is it a connected graph? Spanning trees Biconnected components
Depth-First Search
int visited[MAX_VERTICES]; void dfs(int v) {
node_ptr w; visited[v] = TRUE; printf(“%5d”, v); for (w = graph[v]; w; w = w->link)
230
1
2
130
0
0
3
1
2
1
2
G1
012
3
3
DFS(0)
BFS(0)
Biconnected components
Definition: Articulation point (關節點)
– 原文請參閱課本 – 如果將vertex v以及連接的所有edge去除,產生
graph G’, G’至少有兩個connected component, 則 v稱為 articulation point
while(front) {
Int deleteq(queue_ptr);
v = deleteq(&front);
for(w=graph[v]; w; w=w->link)
if(!visited[w->vertex]) {
printf(“%5d”, w->vertex);
addq(&front, &rear, w->vertex);
0
0 1 0
1
1 0 1
2
0 0 0
G3
1 20
0123456 4577120
0
Adjacency multilists 1 2 3
m vertex1 vertex2 list1 list2
G1
N0
0 1 N1 N3
typedef struct edge *edge_ptr; Typedef struct edge {
– 大部分元素是0 – e << (n2/2)
0
1 3 G1
0
0 1 0 1 1 0 1 2 0 0 0
G3
0 1 1 1 2 1 0 1 1
1 1 0 1 1 1 1 0
Adjacency lists 0
1
n個linked list
1 20
2
#define MAX_VERTICES 50
G3
typedef struct node *node_ptr;
if(!visited[w->vertex]) dfs(w->vertex);
}
Adjacency list: O(e) Adjacency Mtx: O(n2) 例如:老鼠走迷宮
Breadth-First Search
void bfs(int v) {
node_ptr w;
typedef struct queue *queue_ptr;