BP神经网络数据分类matlab程序代码
基于遗传算法的BP神经网络MATLAB代码
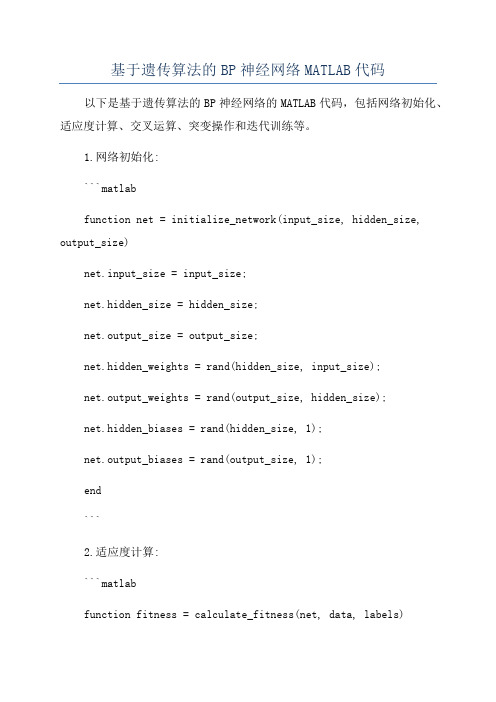
基于遗传算法的BP神经网络MATLAB代码以下是基于遗传算法的BP神经网络的MATLAB代码,包括网络初始化、适应度计算、交叉运算、突变操作和迭代训练等。
1.网络初始化:```matlabfunction net = initialize_network(input_size, hidden_size, output_size)net.input_size = input_size;net.hidden_size = hidden_size;net.output_size = output_size;net.hidden_weights = rand(hidden_size, input_size);net.output_weights = rand(output_size, hidden_size);net.hidden_biases = rand(hidden_size, 1);net.output_biases = rand(output_size, 1);end```2.适应度计算:```matlabfunction fitness = calculate_fitness(net, data, labels)output = forward_propagation(net, data);fitness = sum(sum(abs(output - labels)));end```3.前向传播:```matlabfunction output = forward_propagation(net, data)hidden_input = net.hidden_weights * data + net.hidden_biases;hidden_output = sigmoid(hidden_input);output_input = net.output_weights * hidden_output +net.output_biases;output = sigmoid(output_input);endfunction result = sigmoid(x)result = 1 ./ (1 + exp(-x));end```4.交叉运算:```matlabfunction offspring = crossover(parent1, parent2)point = randi([1 numel(parent1)]);offspring = [parent1(1:point) parent2((point + 1):end)]; end```5.突变操作:```matlabfunction mutated = mutation(individual, mutation_rate) for i = 1:numel(individual)if rand < mutation_ratemutated(i) = rand;elsemutated(i) = individual(i);endendend```6.迭代训练:```matlabfunction [best_individual, best_fitness] =train_network(data, labels, population_size, generations, mutation_rate)input_size = size(data, 1);hidden_size = round((input_size + size(labels, 1)) / 2);output_size = size(labels, 1);population = cell(population_size, 1);for i = 1:population_sizepopulation{i} = initialize_network(input_size, hidden_size, output_size);endbest_individual = population{1};best_fitness = calculate_fitness(best_individual, data, labels);for i = 1:generationsfor j = 1:population_sizefitness = calculate_fitness(population{j}, data, labels);if fitness < best_fitnessbest_individual = population{j};best_fitness = fitness;endendselected = selection(population, data, labels);for j = 1:population_sizeparent1 = selected{randi([1 numel(selected)])};parent2 = selected{randi([1 numel(selected)])};offspring = crossover(parent1, parent2);mutated_offspring = mutation(offspring, mutation_rate);population{j} = mutated_offspring;endendendfunction selected = selection(population, data, labels) fitnesses = zeros(length(population), 1);for i = 1:length(population)fitnesses(i) = calculate_fitness(population{i}, data, labels);end[~, indices] = sort(fitnesses);selected = population(indices(1:floor(length(population) / 2)));end```这是一个基于遗传算法的简化版BP神经网络的MATLAB代码,使用该代码可以初始化神经网络并进行迭代训练,以获得最佳适应度的网络参数。
MATLAB程序代码--BP神经网络的设计实例

MATLAB程序代码--BP神经网络的设计实例例1 采用动量梯度下降算法训练 BP 网络。
训练样本定义如下:输入矢量为p =[-1 -2 3 1-1 1 5 -3]目标矢量为t = [-1 -1 1 1]解:本例的 MATLAB 程序如下:close allclearecho onclc% NEWFF——生成一个新的前向神经网络% TRAIN——对 BP 神经网络进行训练% SIM——对 BP 神经网络进行仿真pause% 敲任意键开始clc% 定义训练样本% P 为输入矢量P=[-1, -2,3,1;-1,1,5, -3];% T 为目标矢量T=[-1, -1, 1, 1];pause;clc% 创建一个新的前向神经网络net=newff(minmax(P),[3,1],{'tansig','purelin'},'traingd m')% 当前输入层权值和阈值inputWeights=net.IW{1,1}inputbias=net.b{1}% 当前网络层权值和阈值layerWeights=net.LW{2,1} layerbias=net.b{2}pauseclc% 设置训练参数net.trainParam.show = 50; net.trainParam.lr = 0.05; net.trainParam.mc = 0.9;net.trainParam.epochs = 1000;net.trainParam.goal = 1e-3; pauseclc% 调用 TRAINGDM 算法训练 BP 网络[net,tr]=train(net,P,T);pauseclc% 对 BP 网络进行仿真A = sim(net,P)% 计算仿真误差E = T - AMSE=mse(E)pauseclcecho off例2 采用xx正则化算法提高 BP 网络的推广能力。
BP神经网络MATLAB代码
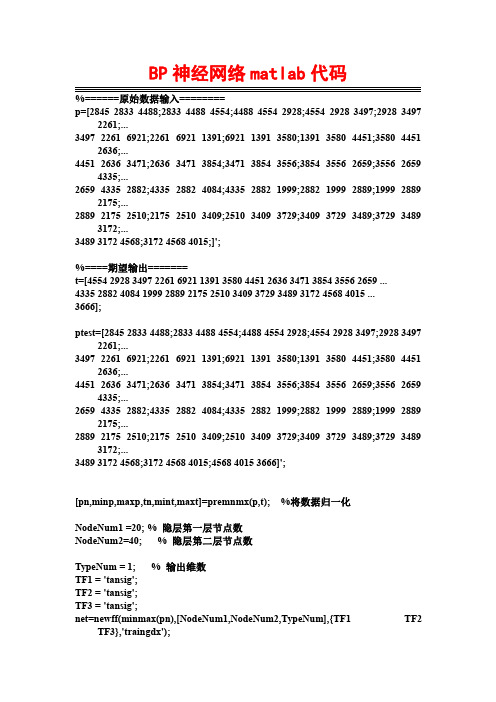
BP神经网络matlab代码p=[284528334488;283344884554;448845542928;455429283497;29283497 2261;...349722616921;226169211391;692113913580;139135804451;35804451 2636;...445126363471;263634713854;347138543556;385435562659;35562659 4335;...265943352882;433528824084;433528821999;288219992889;19992889 2175;...288921752510;217525103409;251034093729;340937293489;37293489 3172;...348931724568;317245684015;]';%====期望输出=======t=[4554292834972261692113913580445126363471385435562659... 4335288240841999288921752510340937293489317245684015... 3666];ptest=[284528334488;283344884554;448845542928;455429283497;29283497 2261;...349722616921;226169211391;692113913580;139135804451;35804451 2636;...445126363471;263634713854;347138543556;385435562659;35562659 4335;...265943352882;433528824084;433528821999;288219992889;19992889 2175;...288921752510;217525103409;251034093729;340937293489;37293489 3172;...348931724568;317245684015;456840153666]';[pn,minp,maxp,tn,mint,maxt]=premnmx(p,t);%将数据归一化NodeNum1=20;%隐层第一层节点数NodeNum2=40;%隐层第二层节点数TypeNum=1;%输出维数TF1='tansig';TF2='tansig';TF3='tansig';net=newff(minmax(pn),[NodeNum1,NodeNum2,TypeNum],{TF1TF2 TF3},'traingdx');%网络创建traingdmnet.trainParam.show=50;net.trainParam.epochs=50000;%训练次数设置net.trainParam.goal=1e-5;%训练所要达到的精度net.trainParam.lr=0.01;%学习速率net=train(net,pn,tn);p2n=tramnmx(ptest,minp,maxp);%测试数据的归一化an=sim(net,p2n);[a]=postmnmx(an,mint,maxt)%数据的反归一化,即最终想得到的预测结果plot(1:length(t),t,'o',1:length(t)+1,a,'+');title('o表示预测值---*表示实际值')grid onm=length(a);%向量a的长度t1=[t,a(m)];error=t1-a;%误差向量figureplot(1:length(error),error,'-.')title('误差变化图')grid on%结束下面的是我把索%======ÔʼÊý¾ÝÊäÈë========p=[0.0358680.0385570.0220440.0171700.0104820.0120810.0037350.004663;0.0388910.0397780.0181150.0184190.0098570.0105140.0059380.006051;0.0374130.0384490.0185320.0171410.010221 0.0101030.0089230.009008;0.0332140.0371330.0206900.0145240.0111610.0106640.0066470.007862;0.0375960.0379980.0201270.0186020.0104910.0116430.0055090.004373;0.0303130.0340310.018255 0.0163330.0080200.0091640.0066560.004936;0.0366990.0371520.0202510.0154910.0121800.012924 0.0075200.008002;...0.2809400.1993590.1128910.0797500.0619890.0477980.0390930.035339;0.2352230.1675010.095537 0.0674510.0518210.0407670.0356960.028659;0.2258100.1612220.0914630.0637270.0492210.038668 0.0327730.030023;0.2282550.1619520.0921190.0654410.0509320.0381480.0341810.028801;0.230465 0.1639260.0936220.0676940.0514080.0377460.0347800.030020;0.2338510.1675610.0958520.068036 0.0507980.0399780.0350310.029043;0.2359590.1691130.0969030.0679360.0516860.0389350.034297 0.032020;...0.0182320.0140600.0059230.0040330.0007420.0058400.0018000.002859;0.0155060.0211870.008724 0.0067840.0025160.0024680.0042380.001484;0.0109790.0114670.0035370.0045270.0016230.002313 0.0019850.000662;0.0175400.0188050.0032210.0079770.0036650.0057530.0042850.003807;0.008817 0.0126570.0024340.0065130.0011920.0019030.0049040.003188;0.0124950.0161250.0064830.005098 0.0047510.0014300.0042500.004460;0.0081280.0200870.0064080.0102490.0045140.0027180.002576 0.002896;]';%====ÆÚÍûÊä³ö=======t=[000000000000001010101010101001010101010101];ptest=[0.0399850.0401500.0229320.0148890.0102450.0103300.0072850.008245;0.0395970.041301 0.0210420.0172060.0090620.0107090.0043790.007461;0.0347410.0382610.0230350.0185850.009328 0.0099880.0075210.006008;...0.2468790.1764940.1022420.0713890.0571930.0403130.0368370.030813;0.2569540.1800370.101773 0.0723390.0572040.0444510.0373170.033529;0.2809400.1993590.1128910.0797500.0619890.047798 0.0390930.035339;...0.0161200.0141350.0017940.0051930.0023760.0015300.0021690.001995;0.0163150.0207470.006796 0.0059240.0043230.0038880.0059570.002633;0.0024230.0293810.0174550.0108740.0032110.002222 0.0045590.002341;]';[pn,minp,maxp,tn,mint,maxt]=premnmx(p,t);%½«Êý¾Ý¹éÒ»»¯NodeNum1=20;%Òþ²ãµÚÒ»²ã½ÚµãÊýNodeNum2=40;%Òþ²ãµÚ¶þ²ã½ÚµãÊýTypeNum=1;%Êä³öάÊýTF1='tansig';TF2='tansig';TF3='tansig';net=newff(minmax(pn),[NodeNum1,NodeNum2,TypeNum],{TF1TF2TF3},'traingdx');%ÍøÂç´´½¨traingdmnet.trainParam.show=50;net.trainParam.epochs=50000;%ѵÁ·´ÎÊýÉèÖÃnet.trainParam.goal=1e5;%ѵÁ·ËùÒª´ïµ½µÄ¾«¶Ènet.trainParam.lr=0.01;%ѧϰËÙÂÊnet=train(net,pn,tn);p2n=tramnmx(ptest,minp,maxp);%²âÊÔÊý¾ÝµÄ¹éÒ»»¯an=sim(net,p2n);[a]=postmnmx(an,mint,maxt)%Êý¾ÝµÄ·´¹éÒ»»¯£¬¼´×îÖÕÏëµÃµ½µÄÔ¤²â½á¹ûplot(1:length(t),t,'o',1:length(t)+1,a,'+');title('o±íʾԤ²âÖµ*±íʾʵ¼ÊÖµ')grid onm=length(a);%ÏòÁ¿aµÄ³¤¶Èt1=[t,a(m)];error=t1a;%Îó²îÏòÁ¿figureplot(1:length(error),error,'.')title('Îó²î±ä»¯Í¼')grid on。
BP神经网络预测的matlab代码
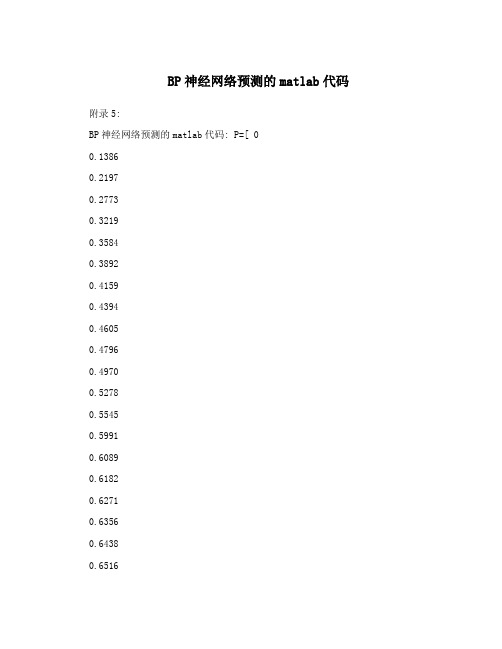
BP神经网络预测的matlab代码附录5:BP神经网络预测的matlab代码: P=[ 00.13860.21970.27730.32190.35840.38920.41590.43940.46050.47960.49700.52780.55450.59910.60890.61820.62710.63560.64380.65160.65920.66640.67350.72220.72750.73270.73780.74270.74750.75220.75680.76130.76570.7700]T=[0.4455 0.323 0.4116 0.3255 0.4486 0.2999 0.4926 0.2249 0.48930.2357 0.4866 0.22490.4819 0.2217 0.4997 0.2269 0.5027 0.217 0.5155 0.1918 0.5058 0.2395 0.4541 0.2408 0.4054 0.2701 0.3942 0.3316 0.2197 0.2963 0.5576 0.1061 0.4956 0.267 0.5126 0.2238 0.5314 0.2083 0.5191 0.208 0.5133 0.18480.5089 0.242 0.4812 0.2129 0.4927 0.287 0.4832 0.2742 0.5969 0.24030.5056 0.2173 0.5364 0.1994 0.5278 0.2015 0.5164 0.2239 0.4489 0.2404 0.4869 0.2963 0.4898 0.1987 0.5075 0.2917 0.4943 0.2902 ]threshold=[0 1]net=newff(threshold,[11,2],{'tansig','logsig'},'trainlm');net.trainParam.epochs=6000net.trainParam.goal=0.01LP.lr=0.1;net=train(net,P',T')P_test=[ 0.77420.77840.78240.78640.79020.7941 ] out=sim(net,P_test')友情提示:以上面0.7742为例0.7742=ln(47+1)/5因为网络输入有一个元素,对应的是测试时间,所以P只有一列,Pi=log(t+1)/10,这样做的目的是使得这些数据的范围处在[0 1]区间之内,但是事实上对于logsin命令而言输入参数是正负区间的任意值,而将输出值限定于0到1之间。
BP神经网络实验详解(MATLAB实现)
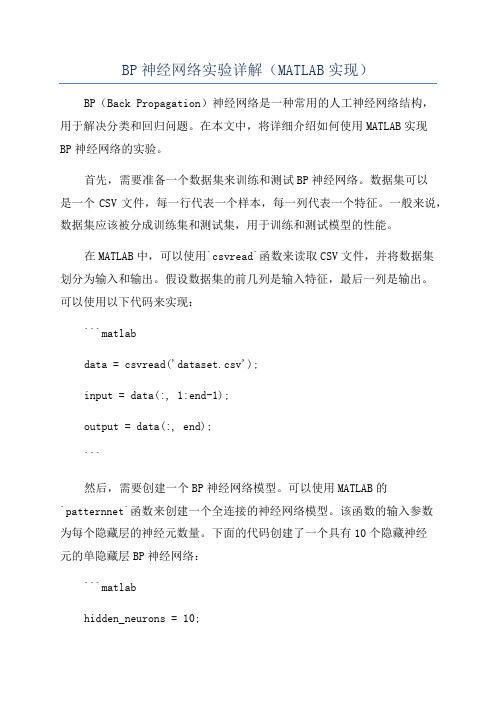
BP神经网络实验详解(MATLAB实现)BP(Back Propagation)神经网络是一种常用的人工神经网络结构,用于解决分类和回归问题。
在本文中,将详细介绍如何使用MATLAB实现BP神经网络的实验。
首先,需要准备一个数据集来训练和测试BP神经网络。
数据集可以是一个CSV文件,每一行代表一个样本,每一列代表一个特征。
一般来说,数据集应该被分成训练集和测试集,用于训练和测试模型的性能。
在MATLAB中,可以使用`csvread`函数来读取CSV文件,并将数据集划分为输入和输出。
假设数据集的前几列是输入特征,最后一列是输出。
可以使用以下代码来实现:```matlabdata = csvread('dataset.csv');input = data(:, 1:end-1);output = data(:, end);```然后,需要创建一个BP神经网络模型。
可以使用MATLAB的`patternnet`函数来创建一个全连接的神经网络模型。
该函数的输入参数为每个隐藏层的神经元数量。
下面的代码创建了一个具有10个隐藏神经元的单隐藏层BP神经网络:```matlabhidden_neurons = 10;net = patternnet(hidden_neurons);```接下来,需要对BP神经网络进行训练。
可以使用`train`函数来训练模型。
该函数的输入参数包括训练集的输入和输出,以及其他可选参数,如最大训练次数和停止条件。
下面的代码展示了如何使用`train`函数来训练模型:```matlabnet = train(net, input_train, output_train);```训练完成后,可以使用训练好的BP神经网络进行预测。
可以使用`net`模型的`sim`函数来进行预测。
下面的代码展示了如何使用`sim`函数预测测试集的输出:```matlaboutput_pred = sim(net, input_test);```最后,可以使用各种性能指标来评估预测的准确性。
matlab训练神经网络分类器方法,用神经网络做训练和分类的matlab代码
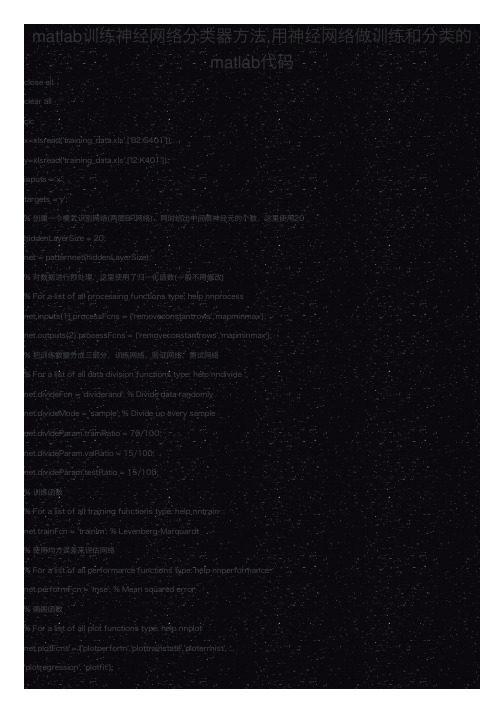
matlab训练神经⽹络分类器⽅法,⽤神经⽹络做训练和分类的matlab代码close allclear allclcx=xlsread('training_data.xls',['B2:G401']);y=xlsread('training_data.xls',['I2:K401']);inputs = x';targets = y';% 创建⼀个模式识别⽹络(两层BP⽹络),同时给出中间层神经元的个数,这⾥使⽤20hiddenLayerSize = 20;net = patternnet(hiddenLayerSize);% 对数据进⾏预处理,这⾥使⽤了归⼀化函数(⼀般不⽤修改)% For a list of all processing functions type: help nnprocessnet.inputs{1}.processFcns = {'removeconstantrows','mapminmax'};net.outputs{2}.processFcns = {'removeconstantrows','mapminmax'};% 把训练数据分成三部分,训练⽹络、验证⽹络、测试⽹络% For a list of all data division functions type: help nndividenet.divideFcn = 'dividerand'; % Divide data randomlynet.divideMode = 'sample'; % Divide up every samplenet.divideParam.trainRatio = 70/100;net.divideParam.valRatio = 15/100;net.divideParam.testRatio = 15/100;% 训练函数% For a list of all training functions type: help nntrainnet.trainFcn = 'trainlm'; % Levenberg-Marquardt% 使⽤均⽅误差来评估⽹络% For a list of all performance functions type: help nnperformancenet.performFcn = 'mse'; % Mean squared error% 画图函数% For a list of all plot functions type: help nnplotnet.plotFcns = {'plotperform','plottrainstate','ploterrhist', ...'plotregression', 'plotfit'};% 开始训练⽹络(包含了训练和验证的过程)[net,tr] = train(net,inputs,targets);% 测试⽹络outputs = net(inputs);errors = gsubtract(targets,outputs);performance = perform(net,targets,outputs)% 获得训练、验证和测试的结果trainTargets = targets .* tr.trainMask{1};valTargets = targets .* tr.valMask{1};testTargets = targets .* tr.testMask{1};trainPerformance = perform(net,trainTargets,outputs) valPerformance = perform(net,valTargets,outputs) testPerformance = perform(net,testTargets,outputs)% 可以查看⽹络的各个参数view(net)% 根据画图的结果,决定是否满意% Uncomment these lines to enable various plots. figure, plotperform(tr)figure, plottrainstate(tr)figure, plotconfusion(targets,outputs)figure, ploterrhist(errors)%如果你对该次训练满意,可以保存训练好⽹络save('training_net.mat','net','tr');下⾯是⽤来分类的代码clear allclose allclcload 'training_net.mat'%% You can change the filename, sheet name, and range %导⼊测试数据new_input = xlsread('new_data.xls',['A2:F25']);new_output = round(net(new_input'));xlswrite('new_data.xls',new_output','result','G2');%把⼆进制转换成对应的类别。
BP神经网络MATLAB编程代码
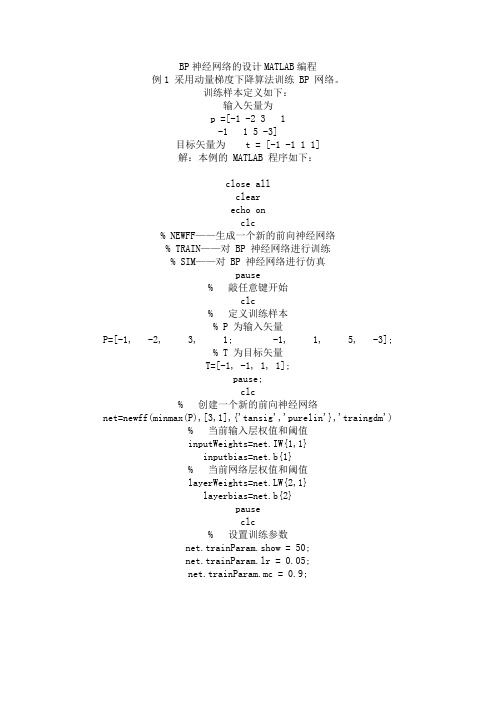
BP神经网络的设计MATLAB编程例1 采用动量梯度下降算法训练 BP 网络。
训练样本定义如下:输入矢量为p =[-1 -2 3 1-1 1 5 -3]目标矢量为 t = [-1 -1 1 1]解:本例的 MATLAB 程序如下:close allclearecho onclc% NEWFF——生成一个新的前向神经网络% TRAIN——对 BP 神经网络进行训练% SIM——对 BP 神经网络进行仿真pause% 敲任意键开始clc% 定义训练样本% P 为输入矢量P=[-1, -2, 3, 1; -1, 1, 5, -3];% T 为目标矢量T=[-1, -1, 1, 1];pause;clc% 创建一个新的前向神经网络net=newff(minmax(P),[3,1],{'tansig','purelin'},'traingdm')% 当前输入层权值和阈值inputWeights=net.IW{1,1}inputbias=net.b{1}% 当前网络层权值和阈值layerWeights=net.LW{2,1}layerbias=net.b{2}pauseclc% 设置训练参数net.trainParam.show = 50;net.trainParam.lr = 0.05;net.trainParam.mc = 0.9;net.trainParam.epochs = 1000;net.trainParam.goal = 1e-3;pauseclc% 调用 TRAINGDM 算法训练 BP 网络[net,tr]=train(net,P,T);pauseclc% 对 BP 网络进行仿真A = sim(net,P)% 计算仿真误差E = T - AMSE=mse(E)pauseclcecho off例2 采用贝叶斯正则化算法提高 BP 网络的推广能力。
(完整版)BP神经网络matlab实例(简单而经典)
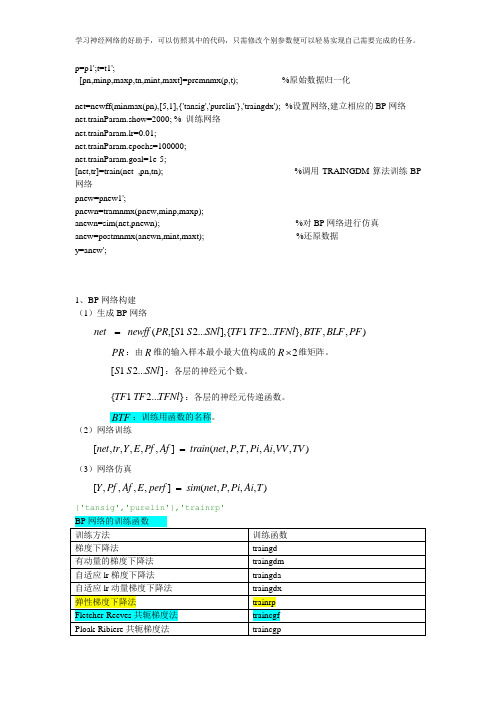
p=p1';t=t1';[pn,minp,maxp,tn,mint,maxt]=premnmx(p,t); %原始数据归一化net=newff(minmax(pn),[5,1],{'tansig','purelin'},'traingdx');%设置网络,建立相应的BP网络net.trainParam.show=2000; % 训练网络net.trainParam.lr=0.01;net.trainParam.epochs=100000;net.trainParam.goal=1e-5;[net,tr]=train(net ,pn,tn); %调用TRAINGDM算法训练BP 网络pnew=pnew1';pnewn=tramnmx(pnew,minp,maxp);anewn=sim(net,pnewn); %对BP网络进行仿真anew=postmnmx(anewn,mint,maxt); %还原数据y=anew';1、BP网络构建(1)生成BP网络=net newff PR S S SNl TF TF TFNl BTF BLF PF(,[1 2...],{ 1 2...},,,)PR:由R维的输入样本最小最大值构成的2R⨯维矩阵。
S S SNl:各层的神经元个数。
[ 1 2...]{ 1 2...}TF TF TFNl:各层的神经元传递函数。
BTF:训练用函数的名称。
(2)网络训练[,,,,,] (,,,,,,)=net tr Y E Pf Af train net P T Pi Ai VV TV(3)网络仿真=[,,,,] (,,,,)Y Pf Af E perf sim net P Pi Ai T{'tansig','purelin'},'trainrp'2、BP网络举例举例1、%traingdclear;clc;P=[-1 -1 2 2 4;0 5 0 5 7];T=[-1 -1 1 1 -1];%利用minmax函数求输入样本范围net = newff(minmax(P),T,[5,1],{'tansig','purelin'},'trainrp');net.trainParam.show=50;%net.trainParam.lr=0.05;net.trainParam.epochs=300;net.trainParam.goal=1e-5;[net,tr]=train(net,P,T);net.iw{1,1}%隐层权值net.b{1}%隐层阈值net.lw{2,1}%输出层权值net.b{2}%输出层阈值sim(net,P)举例2、利用三层BP神经网络来完成非线性函数的逼近任务,其中隐层神经元个数为五个。
- 1、下载文档前请自行甄别文档内容的完整性,平台不提供额外的编辑、内容补充、找答案等附加服务。
- 2、"仅部分预览"的文档,不可在线预览部分如存在完整性等问题,可反馈申请退款(可完整预览的文档不适用该条件!)。
- 3、如文档侵犯您的权益,请联系客服反馈,我们会尽快为您处理(人工客服工作时间:9:00-18:30)。
BP神经网络数据分类matlab程序代码BP神经网络数据分类
%把输出从1维变成4维
——语音信号特征分类 for i=1:2000
switch output1(i)
MatLab程序代码 case 1
output(i,:)=[1 0 0 0]; %% 清空环境变量
case 2 clc
output(i,:)=[0 1 0 0]; clear
case 3
output(i,:)=[0 0 1 0]; %% 训练数据预测数据提取及归一化
case 4
output(i,:)=[0 0 0 1]; %下载四类语音信号
end load data1 c1
end load data2 c2
load data3 c3
%随机提取1500个样本为训练样本,load data4 c4
500个样本为预测样本
input_train=input(n(1:1500),:)'; %四个特征信号矩阵合成一个矩阵
output_train=output(n(1:1500),:)'; data(1:500,:)=c1(1:500,:);
input_test=input(n(1501:2000),:)'; data(501:1000,:)=c2(1:500,:); output_test=output(n(1501:2000),:)'; data(1001:1500,:)=c3(1:500,:);
data(1501:2000,:)=c4(1:500,:); %输入数据归一化
[inputn,inputps]=mapminmax(input_trai%从1到2000间随机排序
n); k=rand(1,2000);
[m,n]=sort(k);
%% 网络结构初始化
innum=24; %输入输出数据
midnum=25; input=data(:,2:25);
outnum=4; output1 =data(:,1);
%权值初始化 %计算误差
w1=rands(midnum,innum); e=output_train(:,i)-yn;
b1=rands(midnum,1); E(ii)=E(ii)+sum(abs(e)); w2=rands(midnum,outnum);
b2=rands(outnum,1); %计算权值变化率
dw2=e*Iout; w2_1=w2;w2_2=w2_1; db2=e';
w1_1=w1;w1_2=w1_1;
b1_1=b1;b1_2=b1_1; for j=1:1:midnum b2_1=b2;b2_2=b2_1; S=1/(1+exp(-
I(j)));
FI(j)=S*(1-S); %学习率 end
xite=0.1 for k=1:1:innum alfa=0.01; for j=1:1:midnum
dw1(k,j)=FI(j)*x(k)*(e(1)*w2(j,1)+e(2)%% 网络训练
*w2(j,2)+e(3)*w2(j,3)+e(4)*w2(j,4)); for ii=1:10
E(ii)=0;
db1(j)=FI(j)*(e(1)*w2(j,1)+e(2)*w2(j,2) for i=1:1:1500
+e(3)*w2(j,3)+e(4)*w2(j,4)); %% 网络预测输出
end x=inputn(:,i);
end % 隐含层输出
for j=1:1:midnum
w1=w1_1+xite*dw1'; I(j)=inputn(:,i)'*w1(j,:)'+b1(j);
b1=b1_1+xite*db1'; Iout(j)=1/(1+exp(-I(j)));
w2=w2_1+xite*dw2'; end
b2=b2_1+xite*db2'; % 输出层输出
w1_2=w1_1;w1_1=w1;
yn=w2'*Iout'+b2;
w2_2=w2_1;w2_1=w2;
b1_2=b1_1;b1_1=b1;
%% 权值阀值修正 b2_2=b2_1;b2_1=b2;
end %画出预测语音种类和实际语音种类end 的分类图
figure(1)
%% 语音特征信号分类 plot(output_fore,'r')
inputn_test=mapminmax('apply',input_tehold on
st,inputps); plot(output1(n(1501:2000))','b')
legend('预测语音类别','实际语音类别') for ii=1:1
for i=1:500%1500 %画出误差图
%隐含层输出 figure(2)
for j=1:1:midnum plot(error)
title('BP网络分类误差','fontsize',12)
I(j)=inputn_test(:,i)'*w1(j,:)'+b1(j); xlabel('语音信号','fontsize',12) Iout(j)=1/(1+exp(-I(j)));
ylabel('分类误差','fontsize',12) end
%print -dtiff -r600 1-4 fore(:,i)=w2'*Iout'+b2;
end
k=zeros(1,4); end
%找出判断错误的分类属于哪一类
for i=1:500 %% 结果分析
if error(i)~=0 %根据网络输出找出数据属于哪类
[b,c]=max(output_test(:,i));
for i=1:500
switch c
case 1 output_fore(i)=find(fore(:,i)==max(fore(
k(1)=k(1)+1;
:,i)));
case 2 end
k(2)=k(2)+1;
case 3 %BP网络预测误差 k(3)=k(3)+1;
error=output_fore-output1(n(1501:2000) case 4 )'; k(4)=k(4)+1;
end
end
end
%找出每类的个体和
kk=zeros(1,4);
for i=1:500
[b,c]=max(output_test(:,i));
switch c
case 1
kk(1)=kk(1)+1;
case 2
kk(2)=kk(2)+1;
case 3
kk(3)=kk(3)+1;
case 4
kk(4)=kk(4)+1;
end
end
%正确率
rightridio=(kk-k)./kk。