Using Link Analysis to Identify Aspects in Faceted Web Search
寻找原因的文案英语作文

In the pursuit of understanding,the quest to identify the root causesof various phenomena is a fundamental aspect of human curiosity and intellectual exploration.This essay delves into the significance of seeking reasons,the methods employed in this pursuit,and the impact such inquiries have on our lives.The human mind is naturally inclined to ask why?when faced with the unknown or the unexpected.This innate desire to understand the underlying causes of events is not merely an academic exercise it is a vital component of problemsolving and decisionmaking.Whether in the realm of science,where researchers seek to uncover the mechanisms behind natural phenomena,or in everyday life,where individuals attempt to make sense of their experiences,the search for reasons is a universal endeavor.One of the most compelling examples of this quest is the scientific method, which provides a structured approach to investigating the causes of events. Through observation,hypothesis formulation,experimentation,and analysis,scientists aim to establish causal relationships and develop theories that explain the world around us.This systematic approach has led to monumental breakthroughs in various fields,from the discovery of gravity by Isaac Newton to the understanding of DNA structure by Watson and Crick.However,the search for reasons is not limited to the scientific community. In everyday life,individuals often engage in a similar process of inquiry when faced with challenges or decisions.For instance,when a person experiences a setback,they may reflect on the factors that contributed tothe situation,seeking to learn from the experience and avoid similar outcomes in the future.This introspection is not only a means of selfimprovement but also a way to develop resilience and adaptability.The process of seeking reasons can also be seen in the field of social sciences,where researchers attempt to understand the complex interplay of factors that influence human behavior and societal structures.By examining historical events,cultural practices,and economic conditions, social scientists strive to identify the causes of social phenomena,such as the rise and fall of civilizations,the development of social norms,and the emergence of political ideologies.Moreover,the search for reasons is deeply intertwined with the concept of justice and fairness.In legal systems,the pursuit of truth and the determination of responsibility often hinge on the ability to establish causal relationships.For example,in a criminal trial,the prosecution must prove beyond a reasonable doubt that the defendants actions were the direct cause of the crime.This requirement ensures that justice is served based on a thorough understanding of the events in question.The impact of seeking reasons extends beyond the immediate resolution of problems or the acquisition of knowledge.It fosters critical thinking, encourages openmindedness,and promotes a deeper appreciation of the complexity of the world.By engaging in this process,individuals are better equipped to navigate the uncertainties of life and to make informed decisions.In conclusion,the quest to identify the root causes of events is a multifaceted endeavor that permeates various aspects of human life.From the scientific method to everyday problemsolving,the search for reasons is an essential tool for understanding,growth,and justice.As we continue to explore the world around us,it is this relentless pursuit of understanding that drives our progress and enriches our existence.。
财务分析英文
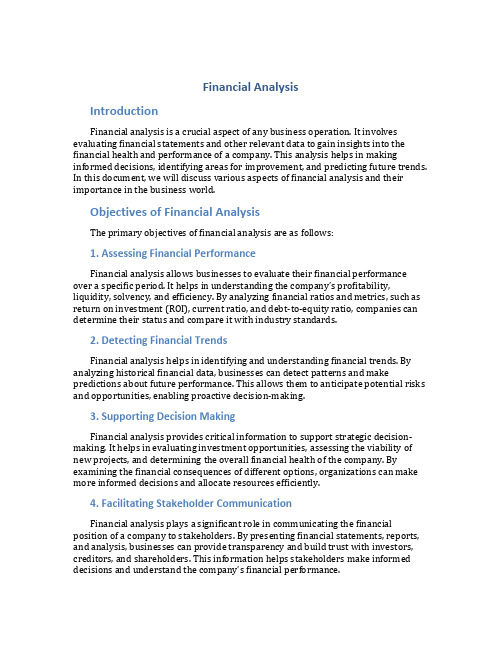
Financial AnalysisIntroductionFinancial analysis is a crucial aspect of any business operation. It involves evaluating financial statements and other relevant data to gain insights into the financial health and performance of a company. This analysis helps in making informed decisions, identifying areas for improvement, and predicting future trends. In this document, we will discuss various aspects of financial analysis and their importance in the business world.Objectives of Financial AnalysisThe primary objectives of financial analysis are as follows:1. Assessing Financial PerformanceFinancial analysis allows businesses to evaluate their financial performance over a specific period. It helps in understanding the company’s profitability, liquidity, solvency, and efficiency. By analyzing financial ratios and metrics, such as return on investment (ROI), current ratio, and debt-to-equity ratio, companies can determine their status and compare it with industry standards.2. Detecting Financial TrendsFinancial analysis helps in identifying and understanding financial trends. By analyzing historical financial data, businesses can detect patterns and make predictions about future performance. This allows them to anticipate potential risks and opportunities, enabling proactive decision-making.3. Supporting Decision MakingFinancial analysis provides critical information to support strategic decision-making. It helps in evaluating investment opportunities, assessing the viability of new projects, and determining the overall financial health of the company. By examining the financial consequences of different options, organizations can make more informed decisions and allocate resources efficiently.4. Facilitating Stakeholder CommunicationFinancial analysis plays a significant role in communicating the financial position of a company to stakeholders. By presenting financial statements, reports, and analysis, businesses can provide transparency and build trust with investors, creditors, and shareholders. This information helps stakeholders make informed decisions and understand the company’s financial performance.Methods of Financial AnalysisThere are various methods and tools used in financial analysis. Some of the commonly employed methods include:1. Ratio AnalysisRatio analysis involves assessing the relationship between different financial variables to evaluate a company’s performance. It helps in gauging profitability, liquidity, efficiency, and solvency. Example ratios include gross profit margin, return on assets, and inventory turnover ratio. By comparing these ratios with industry benchmarks, businesses can identify areas of improvement and assess their competitive position.2. Trend AnalysisTrend analysis involves analyzing financial data over a period to identify patterns and trends. It helps in understanding the direction in which various financial metrics are moving. By studying trends in revenue, expenses, and profitability, businesses can make predictions and take necessary actions to capitalize on opportunities or mitigate risks.3. Cash Flow AnalysisCash flow analysis assesses a company’s inflows and outflows of cash over a specific period. It helps in understanding the liquidity and cash position of the company. By analyzing cash flow statements, businesses can identify their ability to meet short-term obligations and fund operational activities. This analysis is essential for managing working capital and ensuring financial stability.4. Comparative AnalysisComparative analysis involves comparing the financial performance of a company with industry peers or competitors. It helps in benchmarking and understanding the company’s relative position in the market. By analyzing financial ratios, profitability, and growth metrics of competitors, businesses can identify areas for improvement and set realistic goals.5. Break-even AnalysisBreak-even analysis helps businesses determine the point at which their revenue equals their total costs. It identifies the level of sales required to cover both fixed and variable costs. By conducting break-even analysis, companies can assess the feasibility of a business venture, set pricing strategies, and evaluate the impact of changes in costs or sales volume.ConclusionFinancial analysis is an essential tool for businesses to evaluate their financial performance, detect trends, make informed decisions, and communicate with stakeholders. By utilizing various methods such as ratio analysis, trend analysis, cash flow analysis, comparative analysis, and break-even analysis, companies can gain valuable insights into their financial health and take necessary actions to improve their operations. Effective financial analysis forms the foundation for strategic planning and sustainable growth in today’s competitive business environment.。
关于法医的英语作文

关于法医的英语作文英文回答:Forensic science is a fascinating and complex fieldthat combines science and law to investigate crimes. Forensic scientists use their expertise in a variety of fields, including biology, chemistry, physics, and computer science, to analyze evidence and provide expert testimony in court.Forensic scientists play a vital role in the criminal justice system by helping to solve crimes, exonerate the innocent, and convict the guilty. They work closely with law enforcement officers, prosecutors, and defense attorneys to provide objective and scientific analysis of evidence.There are many different types of forensic scientists, each with their own area of expertise. Some common types of forensic scientists include:Crime scene investigators: Crime scene investigators are responsible for collecting and preserving evidence at crime scenes. They document the scene with photographs, sketches, and notes, and collect any physical evidence that may be relevant to the investigation.Forensic pathologists: Forensic pathologists perform autopsies to determine the cause of death in criminal cases. They also examine injuries and other trauma to determine how the victim died.Forensic toxicologists: Forensic toxicologists analyze bodily fluids and tissues to determine if drugs or alcohol were present at the time of death. They can also determine the cause of death if the victim died from an overdose.Forensic serologists: Forensic serologists analyze blood and other bodily fluids to determine blood type, DNA, and other genetic information. This information can be used to identify victims, suspects, and perpetrators of crimes.Forensic document examiners: Forensic document examiners analyze handwriting, typewriting, and other documents to determine their authenticity. They can also help to identify forged or altered documents.Forensic scientists use a variety of scientific techniques to analyze evidence. These techniques include:DNA analysis: DNA analysis is a powerful tool that can be used to identify individuals from blood, saliva, hair, and other bodily fluids. DNA analysis can also be used to link suspects to crime scenes and victims.Fingerprinting: Fingerprinting is a unique form of identification that can be used to identify individualsfrom fingerprints left at crime scenes. Fingerprints are unique to each individual, and they do not change over time.Firearms analysis: Firearms analysis can be used to determine the type of firearm used in a crime, as well as the distance from which the gun was fired. Firearmsanalysis can also be used to identify the shooter if thegun has been used in multiple crimes.Toolmark analysis: Toolmark analysis can be used to identify the type of tool that was used to create a mark or impression. Toolmark analysis can also be used to linktools to crime scenes and suspects.Trace evidence analysis: Trace evidence analysis can be used to identify and analyze small pieces of evidence that may be relevant to a crime. Trace evidence can include fibers, hairs, paint chips, and glass fragments.Forensic science is a constantly evolving field, with new techniques being developed all the time. As technology advances, forensic scientists are able to analyze evidence more quickly and accurately than ever before. This has led to a significant increase in the number of criminal cases that can be solved using forensic evidence.Forensic science is a challenging but rewarding field that offers a unique opportunity to use science to make a difference in the world. If you are interested in a careerin forensic science, there are many different ways to get started. You can earn a degree in forensic science, work as an intern or volunteer in a forensic laboratory, or shadow a forensic scientist.中文回答:法医学是一门迷人且复杂的学科,它将科学和法律相结合以调查犯罪。
JS代码静态分析及挖掘

JS代码静态分析及挖掘JavaScript 已经成为现代 Web 浏览器开发中最普遍的技术之⼀。
使⽤客户端 JavaScript 框架(如 AngularJS,ReactJS 和 Vue.js)构建的应⽤程序已向前端输送了⼤量功能和逻辑。
随着客户端功能和逻辑的增加,客户端的攻击⾯也在逐渐增加。
作为安全测试⼈员,必须了解这些应⽤程序的攻击⾯。
对测试来说,了解要查找的信息,查找的位置以及如何查找那些能导致在应⽤程序中发现潜在安全问题的信息都很重要。
在这篇博⽂中,我们将介绍如何对客户端 JavaScript 代码进⾏静态分析来发现应⽤程序中潜在的安全问题。
我们特别感兴趣的事情是通过执⾏静态分析发现安全问题。
我们不会深⼊研究性能分析或功能测试。
Static analysis is analysing code without executing it.我们需要找哪些信息?作为渗透测试⼈员,对客户端 JavaScript 进⾏静态分析时,我们或多或少会对以下⼏类信息感兴趣:1. 会增加攻击⾯(URL,域等)的信息2. 敏感信息(密码,API 密钥,存储等)3. 代码中的潜在危险位置(eval,dangerouslySetInnerHTML 等)4. 具有已知漏洞的组件(过时的框架等)执⾏静态分析的步骤我们将执⾏静态分析分解为以下⼏个步骤:1. 识别和收集应⽤程序中的 JavaScript ⽂件2. 将收集的 JavaScript 代码进⾏可读处理(Unminify / Deobfuscate)3. 识别可能导致发现安全问题的信息收集 JavaScript ⽂件1.如果你使⽤的是 Burp Suite 测试应⽤程序,那么有多种⽅法可以收集应⽤程序中的所有 JavaScript ⽂件。
在 Appsecco 中,我们遵循⽤户驱动的⼯作流程来测试 Web 应⽤程序,我们通过模拟⽤户浏览整个应⽤程序来开始测试。
通过设置 Burp 代理在浏览应⽤程序时将产⽣的流量发送到Burp。
英文版电子商务概论课后习题

Chapter 11.Describe three factors that would cause a company to continue doing business intraditional ways and avoid electronic commerce.Traditional commerce is a better way to sell the items or services when personal selling skills are a factor, as in commercial real estate sales; or when the condition of the products is difficult to determine without making a personal inspection, as in the purchases of high-fashion clothing, antiques, or perishable food products.2.What are transaction costs and why are they important?Transaction costs are the total of all costs that a buyer and seller incur as they gather information and negotiate a purchase-sale transaction.6.How might a manager use SWOT analysis to identify new applications for electroniccommerce in their strategic business units?In SWOT analysis you list strengths and weaknesses of the business unit and then identify opportunities presented by the markets of the business unit and threats posed by the competitors of the business unit. This is accomplished by analyzing all the business operations into value adding activities and supporting activities.7.In about 200 words, explain the difference between language translation and languagelocalization.Language translation is the process of restating some text written in one language in a different language. In other words, to translate is examine some original text, written in what is called the source language, and to write a corresponding text in different language, called the target language, with the goal of preserving the tone and meaning of the original text.Language localization is a translation that considers multiple elements of the local environment, such as business and cultural practices, in addition to local dialect variations in the language. The cultural element is very important since it can affect—and sometimes completely change—the user’s interpretation of text.8.In a paragraph, describe the advantages of a flat-rate telecommunications access systemfor countries that want to encourage electronic commerce.In a flat-rate access system, the consumer or business pays one monthly fee for unlimited telephone line usage. Although many factors contributed to the rapid rise of U.S. electronic commerce, many industry analysts agree that flat-rate access has been one of the most important factors. As more European telecommunications providers have begun to offer flat-rate access, electronic commerce in those countries has increased dramatically.1.You have decided to buy a new laser printer for your home office. List specific activitiesthat you must undertake as you gather information about printer capabilities andfeatures. Use the CompUSA, , Office Depot, OfficeMax, and Staples Web sites to gather information. Write a short summary of the process you undertook so that others who plan to undertake a similar task can use your information.Answers will vary, but should include the following:∙Identify a search engine∙Visit Websites for information on features, attributes and benefits∙Interrogate Websites for additional information∙Place an order∙Inquire about shipping/payment terms2.Choose one of the Web sites listed in the previous question and identify three ways inwhich the company has reduced its transaction costs by using a Web site to provideinformation about printers. List these three transaction cost reduction elements and write a paragraph in which you discuss one transaction cost reduction opportunity that you believe the company missed.Answers will vary, but may include the following:∙Description of the item∙Employees no longer have to search for prices∙Delivery optionsStaples could include informational links such as: laser printer buying guide or laser printer maintenance.Chapter 21.What were the main forces that led to the commercialization of the Internet?Summarize your answer in about 100 words.The Internet was born out of the need for the U.S. government, specifically the Defense Department, to communicate with its weapons installation distributions all over the world.This idea, in the hands of researchers and scientists evolved even further allowing those researchers the capability to communicate with their colleagues at other universities. As personal computers became more popular and affordable, companies increasingly wanted to construct their own networks. This all led to the dramatic increase in business activity, but the commercialization of the Internet was really spurred by the emergence of the World Wide Web. The software that allowed computers to communicate while on the Internet is still the largest category of traffic today.2.Describe in two paragraphs the origins of HTML. Explain how markup tags workin HTML, and describe the role of at least one person involved with HTML’sdevelopment.SGML is a software language for describing electronic documents and how they should be formatted as well as displayed. This language is the precursor of HTML, which is used by all documents on the Web. Robert Calliau and Tim Berners-Lee independently invented HTML at the CERN research center in Switzerland. HTML’s document type definition is easier for users to learn and use for describing formatting and displaying electronic documents by Meta tag codes.3.In about 200 words, compare the POP e-mail protocol to the IMAP e-mail protocol.Describe situations in which you would prefer to use one protocol or the other andexplain the reasons for your preference.A POP message can tell the e-mail computer to send mail to the user’s computer and to dele teit from the e-mail computer; to send mail to the user’s computer and do not delete it; or simply to ask whether new mail has arrived. IMAP protocol performs the same basic functions as POP, but includes additional features that can instruct the e-mail server to send only selected messages to the client instead of all messages. It also allows the user to view only the headers and the e-mail sender’s name before deciding to download the entire message. One would choose IMAP if they have a need for a more robust system that allows them to access their email from different computers at different times.1.Bridgewater Engineering Company (BECO), a privately held machine shop, makesindustrial-quality, heavy-duty machinery for assembly lines in other factories. It sells its presses, grinders, and milling equipment using a few inside salespeople and telephones.This traditional approach worked well during the company’s start-up years, but BECO is getting a lot of competition from abroad. Because you worked for the company during the summers of your college years, BECO’s president, Tom Dalton, knows you andrealizes that you are Web savvy. He wants to form close relationships with the steelcompanies and small parts manufacturers that are BECO’s suppliers so that h e can tap into their ordering systems and request supplies when he needs them. Tom wants you to investigate how he can use the Internet to set up such electronic relationships. Use the Web and the links in the Online Companion to locate information about extranets and VPNs. Write a report that briefly describes how companies use extranets to link their systems with those of their suppliers, then write an evaluation of at least two companies (using information you have gathered in your Web searches) that could help develop an extranet that would work for Tom. Close the report with an overview of how BECO could use VPN technologies in this type of extranet. The three parts of your reportshould total about 700 words.Responses can vary significantly in this exercise. Any recommendation for systems development should include the infrastructure required to support a supply-chain management extranet, as well as the costs and the anticipated benefits. The infrastructure for a private network requires a TCP/IP network, Web authoring software, and a firewall server. The benefits include lower communication costs, and more timely and accessible information, as well as convenient use.2.Frieda Bannister is the IT manager for the State of Iowa’s Department of Transport ation(DOT). She is interested in finding ways to reduce the costs of operating the DOT’svehicle repair facilities. These facilities purchase replacement parts and repair supplies for all of the state’s cars, trucks, construction machinery, and road maint enanceequipment. Frieda has read about XML and thinks that it might help the DOT send orders to its many suppliers throughout the country more efficiently. Use the Online Companion links, the Web, and your library to conduct research on the use of XML in state, local, and federal government operations. Provide Frieda with a report of about 1000 words that includes sections that discuss what XML is and explain why XML shows promise for the ordering application Frieda envisions. Your report should also identify other DOT business processes or activities that might benefit from using XML. Thereport should also include a summary of the main disadvantages of using XML today for integrating business transactions. End the report with a brief summary of how the W3C Semantic Web project results might help the DOT operate more efficiently in the future.Responses should include the following points:▪XML uses markup tags to describe the meaning or semantics of the text.▪XML records are embedded in HTML documents.▪With XML, tags can be created that identify all the record details for the ordering application that Frieda envisions.▪The extensibility of XML is also its weakness. Sharing data across organizations means that the organizations must use the same tag na mes. For example, Frieda’s organizationmight create a tag called "PurchaseOrderNumber", and one of her suppliers might call the same item "OrderNum".▪The W3C Semantic Web will allow XML tags to be read by software agents, which will result in better, less-timely searching on the Web for information. This would allow theDOT to research pricing, availability, etc. of parts from suppliers.Chapter 31.Write a paragraph in which you describe the conditions under which a Web site canhope to become profitable if it relies exclusively on advertising revenue. In a secondparagraph, provide an example of a company not mentioned in the chapter that is using the advertising-supported model and that is likely to be successful in the long run.Explain why you think it will succeed.A Web site that relies exclusively on advertising revenues must contend with two majorproblems. First, no consensus has emerged on how to measure and charge for site views and the second problem is that very few Web sites have sufficient numbers of visitors to interest large advertisers. To be successful using the advertising-supported revenue model exclusively,a Web site must be large search engine, because they generate sufficient traffic to be profitable.Another alternative is to become a site that targets niche markets, for example employment-advertising sites.2.Describe two possible service-for-fee offerings that might become available tousers of Internet-enabled wireless devices (such as PDAs or mobile phones) in the near future. Write one paragraph for each service in which you outline the profit potential and risk of losses for each.Two possible fee-for-service offerings that might become available are medical and legal services. The profit potential for these services would be higher than the traditional brick and mortar services offered. There are limitations placed on these services because of licensure issues and dispensing legal and medical advice over the Internet is still a major hurdle. As technology and the Internet mature, it will be possible to offer these services offer the Internet.3.In two paragraphs, explain why a customer-centric Web site design is soimportant, yet is so difficult to accomplish.An important part of a successful electronic business operation is a Web site that meets the needs of potential customers. It is a significant challenge however, to design an effective website that introduces the company to different audiences (shareholders, the financial community, suppliers, potential alliance partners, potential customers, current customers and so on) with very different interests.4.Many real estate agents today have Web sites that list the properties they have for sale.These agents also advertise the properties in classified newspaper ads and sometimes in television ads. Write three paragraphs in which you briefly describe the things that realestate agents can best accomplish through (1) their Web sites, (2) mass media advertising, and (3) personal contact.Responses may be similar to the following:Web sites: Offer mortgage loan seekers online credit review and decisions within minutes.Mass media advertising: Offer property listings.Personal contact: Provide more detailed information about the property and about obtaining mortgages,1.Evaluate the usability of two Web sites that sell large-screen televisions. A list of links tocompanies that sell this product is included in the Online Companion for this exercise, but you may use other sites if you wish. In your evaluation, compare the sites on how easy it is to learn about the product and purchase the product. Your report shouldinclude a section of about 200 words in which you describe the criteria you used in your evaluation, a section of about 300 words that summarizes your findings, and a section of about 100 words in which you present your conclusion.Criteria that can be used to evaluate the Web sites include:∙Clarity of product information∙Prices relative to other online merchants∙Overall look and design∙Charges stated clearly before order submission∙Variety of shipping options∙Shipping chargesChapter 41.In about 600 words, explain the differences between customer acquisition and retentionand outline two marketing strategies that would help a company accomplish each ofthese two objectives. Be sure to present facts and logical arguments that support the use of each strategy for each objective.Customer retention is about making sure existing customers keep buying from you. On the Net, customer retention also means making sure your site visitors keep returning. Customer acquisition implies attracting new visitors to your Web site.2.Select a retail store with which you are familiar that has a Web site on which it sellsproducts or services similar to those it sells in its physical retail stores. Explore the Web site and examine it carefully for features that indicate the level of service it provides.Using your experience in the physical store and your review of the Web site, write a200-word evaluation of the company’s touchpoint consiste ncy.Responses will vary but a review should consider the following:The goal of providing the same quality of service is known as touchpoint consistency. The five levels are: awareness (customers who recognize the name of the company or its products), exploration (potential customers learning more about a company’s products and services), familiarity (customers who have completed several transactions and are aware of company policies), commitment (customers with preferences for the product; these customers are loyal and are willing to tell other potential customers), and separation (customers that are leaving, or separating, from the company for any reason).3.Many people have strong negative reactions to pop-up, pop-behind, interstitial, and richmedia ads. Write a 200-word letter to the editor of an Internet industry magazine inwhich you explain, from the advertiser’s viewpoint, why these ads can be effectiveadvertising media.Responses will vary, but the students might discuss how pop-behind ads remain visible after the browser has closed and keeps that information fresh in the user’s mind. In addition, a good point to bring up is that unlike pop-up ads, they do not cover the browser window.1.Visit the RedEnvelope Web site to examine how that company implements occasionsegmentation. Write a report of no more than 200 words in which you describe two clear examples of occasion segmentation on the site.Answers will vary, but Red Envelope has a section that displays various holidays, such as spring, Easter, and birthdays. Students should discuss the details of these different occasions.2.Marti Baron operates a small Web business, The Cannonball, that sells parts, repair kits,books, and accessories to hobbyists who restore antique model trains. Many model train hobbyists and collectors have created Web sites on which they share photos and other information about model trains. Marti is interested in creating an affiliate marketing program that would allow those hobbyists to place links on their sites to TheCannonball and be rewarded with commissions on sales that result from visitorsfollowing those links. Examine the services offered by Be Free, Commission Junction, LinkShare, and any other affiliate program brokers you can find on the Web.Recomme nd at least one affiliate program broker that would be a good fit for Marti’s business. In about 500 words, explain your recommendation. Be sure to consider thecharacteristics of Marti’s business in your analysis.The students should search different program brokers and try to find one that already deals in hobbies and collectibles. Be Free would be a good fit, since it caters to smaller businesses and is scalable as well.。
信息化侦查的流程

信息化侦查的流程Information gathering is a crucial step in the process of digital investigation. It involves collecting and analyzing data from various sources to gather evidence for criminal cases or intelligence purposes. In today's digital age, the amount of information available online is vast and diverse, making it essential for investigators to have the skills and tools to effectively navigate this data. This process can be both challenging and time-consuming, as investigators must sift through large amounts of data to identify relevant information.信息搜集是数字侦查过程中至关重要的一步。
它涉及从各种来源收集和分析数据,以搜集犯罪案件或情报目的的证据。
在当今的数字时代,在线上可获取的信息量庞大且多样化,这使得侦查人员必须具备有效地浏览这些数据的技能和工具至关重要。
这一过程既具有挑战性又耗时,因为侦查人员必须筛选大量数据,以确定相关信息。
One of the key aspects of the information gathering process is identifying the sources of information. This may include social media platforms, websites, online databases, and even physical sources such as documents or electronic devices. Investigators must be ableto determine which sources are reliable and relevant to the case at hand, as well as ensure the legality of their methods in obtaining the information. It is important to consider the integrity and authenticity of the sources, as well as any potential biases or inaccuracies that may be present.信息搜集过程中的一个关键方面是确定信息来源。
idmapping流程
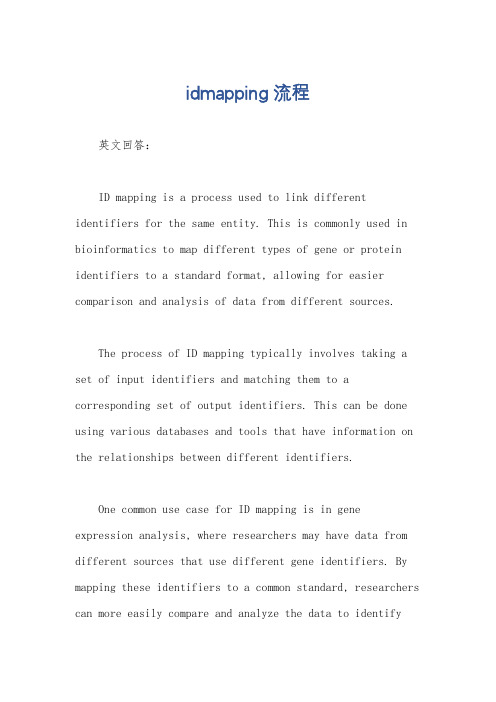
idmapping流程英文回答:ID mapping is a process used to link different identifiers for the same entity. This is commonly used in bioinformatics to map different types of gene or protein identifiers to a standard format, allowing for easier comparison and analysis of data from different sources.The process of ID mapping typically involves taking a set of input identifiers and matching them to a corresponding set of output identifiers. This can be done using various databases and tools that have information on the relationships between different identifiers.One common use case for ID mapping is in gene expression analysis, where researchers may have data from different sources that use different gene identifiers. By mapping these identifiers to a common standard, researchers can more easily compare and analyze the data to identifypatterns and relationships.Overall, ID mapping is a crucial step in integrating and analyzing data from multiple sources, and it plays a key role in bioinformatics research.中文回答:ID映射是一种用于链接同一实体的不同标识符的过程。
personalized page rank

Personalized PageRankIntroductionPageRank is an algorithm used by search engines to rank web pages based on their importance and relevance. It is a key component of the Google search engine and is widely used in the field of web search and web mining. The traditional PageRank algorithm ranks web pages solely based on their link structure, disregarding user preferences and personalized information.However, in recent years, there has been a growing need for personalized search results. Users often have different preferences and interests, and they expect search engines to provide them with tailored and relevant content. To address this need, personalized PageRank algorithms have been developed to incorporate user-specific information into the ranking process.Overview of Personalized PageRankPersonalized PageRank is an extension of the traditional PageRank algorithm that takes into account user preferences and interests. It assigns a personalized importance score to each web page based on its relevance to the user.The algorithm starts by assigning an initial importance score to each web page in the network. This can be done using various methods, such as uniformly distributing the scores or assigning higher scores to specific pages based on user preferences.Next, the algorithm iteratively updates the importance scores of the web pages based on their link structure and the user’s preferences. It takes into account both the importance of the pages that link to a given page and the importance of the user’s preferred pages. The process continues until the importance scores converge, indicating a stable ranking.Calculating Personalized PageRankThe calculation of personalized PageRank involves several steps:1.Define the user preferences: Users can provide their preferencesdirectly or implicitly through their search history, clickpatterns, or feedback. These preferences can be represented as apreference vector, where each element represents the user’sinterest in a specific topic or category.2.Construct the transition probability matrix: The transitionprobability matrix represents the likelihood of transitioning from one page to another in the network. It is calculated based on the link structure of the web pages. Each element of the matrixrepresents the probability of transitioning from one page toanother through a hyperlink. The matrix is typically sparse andcan be efficiently represented using sparse matrix techniques.3.Initialize the importance scores: The importance scores of the webpages are initialized based on the user preferences. Pages thatare more relevant to the user are assigned higher initial scores. 4.Update the importance scores iteratively: The importance scoresare updated iteratively using the transition probability matrix.At each iteration, the importance score of a page is calculated asa weighted sum of the importance scores of the pages that link toit, considering both the importance of the linking pages and theuser preferences.5.Convergence criteria: The iteration process continues until theimportance scores converge. A common convergence criterion is tostop the iterations when the L1 distance between the importancescores of consecutive iterations falls below a predeterminedthreshold.Advantages of Personalized PageRankPersonalized PageRank has several advantages over traditional PageRank: 1.Relevance: Personalized PageRank considers user preferences andinterests, resulting in search results that are more relevant and tailored to the user’s needs. Users are more likely to find theinformation they are looking for quickly and easily.2.Diversity: Personalized PageRank can also take into account theneed for diversity in search results. It can balance betweenproviding results that are highly relevant to the user’spreferences and presenting a diverse set of options to explore. er feedback incorporation: Personalized PageRank can incorporateuser feedback, such as clicks, likes, and dislikes, tocontinuously improve the search results. By learning from theuser’s interactions, the algorithm can adapt and provide betterrecommendations over time.4.Dynamic ranking: Personalized PageRank can be used to createdynamic rankings that adapt to changing user preferences andinterests. As the user’s preferences evolve, the algorithm canupdate the importance scores accordingly, ensuring that the search results remain up to date.Applications of Personalized PageRankPersonalized PageRank has various applications beyond web search:1.Recommender systems: Personalized PageRank can be used inrecommender systems to provide personalized recommendations tousers, based on their preferences and interests. It can help users discover new content that is relevant to their interests andincrease user engagement.2.Social networks: Personalized PageRank can be applied to socialnetwork analysis to identify influential users or groups of users.By considering the interactions between users and theirpreferences, the algorithm can identify the most important nodesin the network based on personalized influence.3.E-commerce: Personalized PageRank can be used in e-commerceplatforms to recommend relevant products to users. By taking into account the user’s preferences, purchase history, and browsingbehavior, the algorithm can generate personalized productrecommendations that increase conversion rates.rmation retrieval: Personalized PageRank can be used toimprove the effectiveness of information retrieval systems. Byincorporating user preferences, the algorithm can rank searchresults based on their relevance to the user, leading to betterretrieval performance.ConclusionPersonalized PageRank is a valuable algorithm for providing personalized and relevant search results. By incorporating user preferences and interests, it can generate tailored rankings that meet the individual needs of users. With its applications in various domains, personalized PageRank has the potential to enhance the user experience and improve the effectiveness of recommendation systems, social network analysis, and information retrieval.。
- 1、下载文档前请自行甄别文档内容的完整性,平台不提供额外的编辑、内容补充、找答案等附加服务。
- 2、"仅部分预览"的文档,不可在线预览部分如存在完整性等问题,可反馈申请退款(可完整预览的文档不适用该条件!)。
- 3、如文档侵犯您的权益,请联系客服反馈,我们会尽快为您处理(人工客服工作时间:9:00-18:30)。
Using Link Analysis to Identify Aspects in Faceted Web SearchChristian Kohlschütter L3S/University of Hannover Deutscher Pavillon,Expo Plaza1 30539Hannover,Germany kohlschuetter@l3s.dePaul-Alexandru ChiritaL3S/University of HannoverDeutscher Pavillon,Expo Plaza130539Hannover,Germanychirita@l3s.deWolfgang NejdlL3S/University of HannoverDeutscher Pavillon,Expo Plaza130539Hannover,Germanynejdl@l3s.deABSTRACTAre you looking for information about bush in the context of Politics or Gardening,with focus on government sources or news agencies?Faceted Search can improve your web search by o ering drill-down options towards such speci c aspects,particularly in controlled document collections with rich human-edited annotations.The Web,for the most part, however lacks appropriate metadata;only a small fraction is covered by taxonomies or folksonomies.In this paper,we show that the Web's intrinsic link structure is su cient to enable an automatic faceting on such a large and dynamic document collection,not being limited to topic detection only.We present an e cient method for identifying facets within full-text search.For each page,we allot fuzzy mem-bership values based on Personalized PageRank to all facets, then we compare them in order to derive context informa-tion.A prototype implementation is available online.1.INTRODUCTIONThe basic principle of web search is to nd a set of pages ranked by relevance to an idea or concept the user has in mind.These concepts are usually speci ed by keywords that must occur in any document deemed relevant.Obviously,it is often di cult to nd terms which precisely separate rele-vant from irrelevant pages,because they are either ambigu-ous or can at least be seen from di erent perspectives.While modern search engines may satisfy the average user's needs by focusing on general importance(i.e.,a keyword search for bush primarily returns pages about George W.Bush), as soon as other aspects are concerned,the only way out is to specify additional keywords(i.e.,do a search for bush gardening to focus on horticultural issues)and hope that these auxiliary terms are not too restrictive.But the more aspects are speci ed as terms(such as scienti c article and British ),chances are high that many highly relevant docu-ments are ltered out because not all terms are matched[10]. The emerging paradigm of Faceted Search[6,7,10,19]may help in this situation.Here,the system automatically de-Permission to make digital or hard copies of all or part of this work for personal or classroom use is granted without fee provided that copies are not made or distributed for profit or commercial advantage and that copies bear this notice and the full citation on thefirst page.To copy otherwise,to republish,to post on servers or to redistribute to lists,requires prior specific permission and/or a fee.SIGIR’2006Workshop on Faceted Search,Aug10,2006,Seattle,W A,USA. Copyright2006ACM X-XXXXX-XX-X/XX/XX...$5.00.termines possible aspects from the result set and presents them to the user,modeled as categories of orthogonal di-mensions like topic,cultural background or target audience and nally enables the user to iteratively narrow( drill-down )the search until he is satis ed with the results.These facets 1are represented as metadata,they do not interfere with full-text keywords as opposed to text-based clustering approaches[10].While faceted search works well in situations where facet metadata is annotated to the documents like in library sys-tems or enterprise search applications(e.g.,restaurant search), on dynamic,large scale collections of heterogeneous docu-ments such as the Web,the faceted classi cation of pages and the identi cation of facets within search results still are unresolved problems.The lack of annotations and the om-nipresence of noise within this collection hamper a direct adoption of enterprise faceted search.In this paper,we focus on the question how we can still utilize the few annotations from taxonomies or folksonomies like ODP or for faceted web search.We present an e cient method for automatically identifying facets in web search results solely by link analysis.First experiments have shown that we get a high precision of the suggested faceted classi cations without requiring additional data structures or categorization algorithms.The paper is organized as follows.In Section2,we give a short overview of faceted search,as well as of taxon-omy/folksonomy based web page tagging systems and the Personalized PageRank algorithm.In Section3,we describe an approach to classify web pages through PageRank-based link analysis.Based thereupon,Section4covers our algo-rithm for improving full-text search results by identifying facets with respect to the given keywords.In Section5,we describe our demo application and show the results of a pre-liminary evaluation.Section6concludes with a discussion of on-going and future work.2.RELEV ANT BACKGROUNDFaceted search.Faceted search is a relatively young research area,and thus there exist only few approaches to tackle the problems it raises,especially when generalizing this kind of search for the entire web environment.Facets allow for di erent views on the result set,which can be obtained through a specialized user interface[19],thus en-abling the user to choose di erent possible starting paths 1Note:In this paper,we use the term facet as a synonym to aspect or category ,whereas we call the orthogonal axes facet dimensions .for the exploration of the collection.The Flamenco Sys-tem2for example allows for both searching and browsing an image collection from various perspectives,such as gender, country of a liation for Nobel prize winners.There exist at least two types of facets:(1)Hierarchical and(2)Flat. The concept of Hierarchical Faceted Categories(HFC)was introduced by Hearst et al.in[11,19],and it relies on a set of category hierarchies(one per facet),built manually in advance.Thus,they classify the documents available in a collection space according to each of the hierarchies sep-arately,producing several navigation paths across the same set of points.Inherently,users will nd their sought docu-ments faster,as more routes towards them exist.The main approach we discuss in this paper,i.e.,using page topic(s) as one facet for categorizing web results,also falls in the HFC type.The second type of facets is a at one,in which there is no clear relation between the elements generated within the same facet dimension.We are not aware of any prior work creating this kind of facets from within textual web documents.Large scale taxonomies/folksonomies.One of the largest e orts to manually annotate web pages is the Open Directory Project(ODP)3.Over70,000editors helped to categorize more than5million web sites into almost600,000 hierarchical categories describing web sites'topics;however, these pages still make far less than0.1percent of the pub-licly accessible web.While in ODP,the taxonomy is clearly split into16root categories,such as Business,Computers or Sports, folksonomical organized platforms like 4 allow users to annotate arbitrary tags to web pages rather than using a xed taxonomy[8,14].Since both approaches allow to classify pages to more than just one facet(category or tag),faceted search could easily be implemented for the pages annotated in these collections,but not for the whole web graph.Personalized PageRank.In this paper,we focus on one speci c approach to exploit directly annotated information for supporting faceted search on large-scale web graphs:the so-called Personalized PageRank[15],a special version of the well-known PageRank web page popularity measure[15]. It promises an improvement in ranking with respect to in-dividual interests/facets,which are speci ed through a set of relevant pages.Regular PageRank computes a general importance score r(p)for every page p of the web graph G=(P,L),forming the PageRank vector r.It models the random surfer,who visits the web graph in a rather un-biased way:it starts from an arbitrarily chosen page and follows one random link from one page to another( walk ) until it gets bored,then it jumps to another random page in the graph,and so on.In Personalized PageRank,the jump now only addresses a given set of pages to which the compu-tation should be biased.A common representation of both approaches(random/biased)is the following:∀t∈P:r B(t)=(1−d)·πB(t)+d·X(s,t)∈L r B(s)|L(s)|(1)Here,for a given biasing set B⊆P the PageRank score 2/3/4/r B(t)for a page t is de ned recursively,based on a weighted5 linear combination of jump and walk action.The second summand describes the likelihood that the surfer reaches the page t by walking,which commonly is expressed by the sum of the ranks of all pages s linking to t,each divided by their number of outgoing links|L(s)|.Finally,πB(t)describes the likelihood that the surfer reaches page t from a jump.In the case of regular( unbiased )PageRank,we treat all pages of the web graph as being contained in B,so:πP(t)=|P|−1, in general it is:πB(t)=(1|B|i t∈B0otherwise(2)The distribution of scores in the rank vector r B follows the power law and thus is scale-free.Therefore multiplying a PageRank score with the keyword-based document rele-vance score W(d,q)(e.g.,based on the T F×IDF measure) causes important pages(with respect to the given biasing set B)to gain higher scoresρB.Note that the number of retrieved documents does not change when switching to an-other biasing set,since no facet-speci c keywords are added. Instead the document order is changed:ρB(d,q)=W(d,q)·r B(d)(3) Personalizing PageRank is in fact just a biasing towards an individualized set of scores.As a non-user speci c ap-proach,Haveliwala's Topic-Sensitive PageRank[9]makes use of linear combinations of just16Personalized PageR-ank vectors,each biased on one of the16top categories of ODP respectively.The weights for the linear combination are derived from each topic's term vector(hence,access to full-text information is required):r B =Xβ[ωβ rβ](4)Other,more recent approaches to generate personalized rankings do already exist.Qiu and Cho[16]for example also extend Topic-Sensitive PageRank to contain user spe-ci c weights for combining the16biased vectors.They learn these weights by applying machine learning on user's click history.Aktas et al.[1]have successfully applied Personal-ized PageRank with respect to domain name features(coun-try and generic TLD topic assignment).Finally,Jeh and Widom[12]and more recently Sarlos et al.[17]have inves-tigated the means to compute Personalized PageRank in a scalable way for a large set of users.They both exploited the idea of decomposing the biasing set into small sets with a single non-zero entry followed by a linear combination of the resulted Personalized PageRank vectors.3.WEB PAGE CLASSIFICATIONUSING PERSONALIZED PAGERANK Several aspects of a single page may be considered rele-vant for faceted search;they can be ordered into facet di-mensions like thematical coverage,language,age etc.To automatically retrieve such facets,classi cation algorithms can be applied to receive the set of relevant facets M p for each page p in the web graph.If possible,a relevance mea-sure is assigned to each facet,resulting in a relevance vector 5d usually is around0.85in literature.m p whereas each dimension represents one of all available facets.In classical IR,to compute m p text-based mod-els are used,such as Naive Bayes or LSA.For the Web, one can consider the hyperlink structure of the web as an-other source of classi cation power.Several approaches ex-ist which either combine link structure and textual informa-tion or even attempt to derive a classi cation from the web graph only[3 5,13,18].While they may perform very well, in all cases the only purpose of the proposed algorithms is to generate a classi cation being not related to PageRank; the imposed additional payload(computational and storage requirements)to the search engine system may not be un-derestimated.In this section,we present an e cient method which re-uses the rank vector of Personalized PageRank for classi cation,avoiding such overhead .We are convinced that Personalized PageRank vectors themselves provide su cient information for classifying pages towards arbitrary facets.Because of the skewed jump in the PageRank computation,pages within the corresponding bi-asing set tend to get higher scores than pages outside.Via the link structure also pages being outside but nearby the biasing set(in terms of link hops)gain high scores[15].As-suming that pages mostly link to somehow related pages, there consequently must be a direct relationship between a page's facet-speci c PageRank score r f(p)and its member-ship m p(f)to that facet.m p(f)∝r f(p)(5) We can treat the values as quali able membership to the facet f(member of the set of available facets F);values above the average scoreµ≈1.0are treated as positive membership,values below as negative membership(i.e., non-relevant to the facet).One may assume that the mem-bership value is equal to the rank value,or that a high rank score automatically means a high relevance to the facet(as suggested in[9]).However,since there also is a fair amount of pages of general importance/interest which may also have a high score[4],directly proportional relationship is too imprecise.We therefore introduce the facet membership uncertaintyαf(p)for the page p:the more facets are pos-sible the less reliable the membership statements are.Since facet dimensions are orthogonal,this uncertainty depends on the number of facets in the same dimension dim as the considered facet f.In case that no facets could be deter-mined,the uncertainty is in nite:G f(p)={g∈F|dim(g)=dim(f)∧r g(p)≥µ}(6)αf(p)=(∞i G f(p)=∅|G f(p)|otherwise(7)To account for the scale-free nature of the rank score,αf should in uence r f exponentially:m p(f)=log r f(p)f(8)m p(f)now also quantitatively re ects positive or negative membership certainty6.Having said that,a value around 6We shall avoid the term probability;neither r f(p)norαf(p) are bounded.zero does not implicate that a page does not relate to a spe-ci c facet it is just unclear.This case applies to commonly linked pages like the Acrobat Reader download page,the ph-pBB bulletin board system website and others.On the other hand,specialty pages clearly bene t from this classi cation.4.IDENTIFYING FACETS FROMWEB SEARCH RESULTSWhen submitting a text query q on a PageRank-supported web search engine,the top-k of the returned result list rep-resents the k most relevant results for the query terms with respect to general importance(for Unbiased PageRank)or to speci c facets(for Personalized PageRank).For a su -ciently large k,this list could be regarded as a sample set S q of the whole result set D q;another approach to deter-mining S q,taking care of strongly over-represented facets, is shown in[2].Now,we can compute the facet membership vector m p for all pages p∈S q.From this,we can derive the facet membership vector m S,q,which represents the facet memberships for the given top-k subset S q of results for the query q.In other words,this vector represents the facets which are deemed to be contained in the full result set D q. In order to compute m S,q we use the following procedure: For each facet membership value(in each vector m p,for all pages p∈S q)which exceeds a certain thresholdϑ,we increment the corresponding value m S,q(f)by one; nally,m S,q is normalized to values between0and1.0:m S,q(f)=|{p∈S q|m p(f)≥ϑ}||S q|(9)As an improvement to facet diversity,we suggest to com-pute the membership values not only from one sample set but from multiple PageRank result sets.For example,with the ODP taxonomy,we start with sampling the top-k results from Unbiased PageRank as well as all Personalized PageR-ank vectors based on the taxonomy's16top categories.If the user has already chosen an ODP-topic as a facet,we take the corresponding child categories instead.Another way to extend the set of facets,which can also be applied to non-hierarchical facets,is to start from a custom seed of facets and then iteratively determine relevant facets using the formula above and extend this seed by newly de-tected facets D(see algorithm1).The user can now select from the facets given in D for the re nement of his search.If more than one facet is selected,Algorithm1Iterative identi cation of facetsλ=Membership threshold for newly discovered facets D←∅D ←Facet seedi max←Maximum number of iterationsi←0while i<i max∧D \D=∅<doi←i+1D←D∪DD ←{g∈F|m q,D(g)≥λ};m q,D(f)=Pd∈D|{p∈S d,q|m p(f)≥ϑ}|Pd∈D|S d,q|end whileFigure1:Screen-shot of the prototypea Personalized PageRank is used based on the linear combi-nation of the facets'rank vectors,as described in Section2. As opposed to Topic-Sensitive PageRank,we think it is not necessary to derive the combination weights from textual document statistics.Instead,we may propose pre-de ned weights on the level of facet dimensions(e.g.,prefer topic over origin),which can be altered by the user;facets of the same dimension may safely be equally balanced.5.IMPLEMENTATION AND EV ALUATION Right now,we have implemented facet detection for only one dimension,the topical membership of page,based on the ODP taxonomy.A screen-shot of the prototype is de-picted in gure1;the demo can be tested online,at http: //www.l3s.de/facetedsearch.We are currently extending the setup by two more dimensions,domain name features and type of information source(academia,business,govern-ment,media,private etc.).Our search engine prototype is based upon the Lucene7 information retrieval software library,which we extended to hold multiple PageRank scores per document.We used a collection of9.2million documents which we have retrieved in2005using the Heritrix crawler8.The crawler's strategy was to nd pages from a variety of topics.We performed a broad crawl based on a seed of the pages from four of the16top-level categories of ODP,Business,Computers, Sports and Recreation.From them about one hundred bi-asing sub-categories were randomly chosen.This selection process was executed as follows:For each of the four top categories,three subcategories were randomly picked;then, for one of them,we again randomly took three subcategories and so on,until no deeper levels were available;almost all paths ended at level6(with level1being one of the ODP root categories).We then computed the corresponding100+ Personalized PageRank vectors(plus the Unbiased PageR-ank)using the pages residing in each of these categories (including sub-categories)as biasing sets.7/8/Any keyword search is conveyed using Unbiased PageR-ank by default;however the user can manually choose any available biasing set.The search results are classi ed on-line using equation8;since the classi cation is fuzzy and the op-eration can be performed quickly(no access to textual data is required),we decided not to store this information stat-ically in the index.For the top-k results(k=100per de-fault),we identify the contained facets having a membership certainty of at leastϑ(0.1per default).Both parameters can be adjusted by the expert user.The detected facets are presented next to the document descriptions;facets based on ODP topics are shown both as a tree as well as a sorted list,along with the facet membership value from algorithm 1.The user can choose from these facets by clicking on the corresponding facet name(facets with a membership of at least25%are highlighted).The search is then repeated using the corresponding Personalized PageRank. Unbiased PageRank is treated as a special facet.It may also receive a membership value.Intuitively,this facet can be regarded as general importance.If no other facet has been retrieved for the keyword query,we conclude that we lack the proper Personalized PageRank.From this perspec-tive,the membership to general importance can be inter-preted as uncertainty as well.We performed a preliminary evaluation of the facet de-tection algorithm.We determined a set of phrase queries which are relevant to speci c ODP categories(33in our test set,5keywords each)and searched for these phrases with our system(i max was1).In order to avoid falsi ed results,we did not distill these queries from our own data set,but utilized Google's AdWords service9(we randomly selected5suggested queries;an excerpt is shown in the ap-pendix).Then we compared the category recommendations from AdWords with the topical facet recommendations from our system.Exact phrase search returned results in155out of the165cases.Only in13cases,the algorithm did neither retrieve the desired category nor any ancestor category(this makes a precision of91.6%).9https:///6.CONCLUSIONS&ONGOING WORKIn this paper we proposed to drill-down from hierarchical faceted search and to simply re-order web search results ac-cording to a speci c category within a facet using Personal-ized PageRank.Our current implementation covers output categories for the topic facet,thus enabling users to on-the- y switch to result rankings according to PageRank biased on some Open Directory topic.We have also proposed a new simple technique to infer the most relevant topics as-sociated to a user query.Experimental results have shown this approach to yield precise identi cation of facets.We are currently implementing the generation of a sec-ond type of categories,according to the source type facet. This would enable biasing PageRank on news pages,on user home pages,on university sites etc.For completeness,we also think about adding facets that can be directly retrieved from the documents themselves,such as document size and language.We plan to nish this before giving the demo at the workshop.7.REFERENCES[1]Mehmet S.Aktas,Mehmet A.Nacar,and FilippoMenczer.Personalizing pagerank based on domainpro les.In WEBKDD'04:Proceedings of the sixthWEBKDD workshop:Webmining and Web UsageAnalysis(WEBKDD'04),Seattle,USA,pages83 90,August2004.[2]Aris Anagnostopoulos,Andrei Z.Broder,and DavidCarmel.Sampling search-engine results.In WWW'05: Proceedings of the14th international conference onWorld Wide Web,pages245 256,New York,NY,USA,2005.ACM Press.[3]Pavel Calado,Marco Cristo,Marcos Andre;Goncalves,Edleno S.de Moura,BerthierRibeiro-Neto,and Nivio Ziviani.Link-based similarity measures for the classi cation of web documents.J.Am.Soc.Inf.Sci.Technol.,57(2):208 221,2006. [4]Soumen Chakrabarti,Byron Dom,and Piotr Indyk.Enhanced hypertext categorization using hyperlinks.In SIGMOD'98:Proceedings of the1998ACMSIGMOD international conference on Management of data,pages307 318,New York,NY,US,1998.ACMPress.[5]Je rey Dean and Monika R.Henzinger.Findingrelated pages in the World Wide puterNetworks(Amsterdam,Netherlands:1999),31(11 16):1467 1479,1999.[6]William Denton.How to make a faceted classi cationand put it on the web./ library/facet-web-howto.pdf,November2003. [7]William Denton.Putting facets on the web:Anannotated bibliography.http:///library/facet-biblio.html, October2003.[8]Scott Golder and Bernardo A.Huberman.Thestructure of collaborative tagging systems.Technicalreport,Information Dynamics Lab,HP Labs,2005. [9]Taher H.Haveliwala.Topic-sensitive PageRank.InProc.of the eleventh International Conference onWorld Wide Web,pages517 526.ACM Press,2002.[10]Marti A.Hearst.Clustering versus faceted categoriesfor information mun.ACM,49(4):59 61,2006.[11]Marti A.Hearst,Ame Elliott,Jennifer English,Rashmi R.Sinha,Kirsten Swearingen,and Ka-PingYee.Finding the ow in web site mun.ofthe ACM,45(9):42 49,2002.[12]Glen Jeh and Jennifer Widom.Scaling personalizedweb search.In WWW'03:Proceedings of the12thinternational conference on World Wide Web,pages271 279,New York,NY,USA,2003.ACM Press. [13]Qing Lu and Lise Getoor.Link-based textclassi cation.Text-Mining&Link-Analysis Workshop TextLink2003,2003.[14]Adam Mathes.Folksonomies-cooperativeclassi cation and communication through sharedputer Mediated Communication-LIS590CMC,Graduate School of Library andInformation Science,University of IllinoisUrbana-Champagin,December2004.[15]Lawrence Page,Sergey Brin,Rajeev Motwani,andTerry Winograd.The PageRank citation ranking:Bringing order to the web.Technical report,Stanford Digital Library Technologies Project,1998.[16]Feng Qiu and Junghoo Cho.Automatic identi cationof user interest for personalized search.In Proc.of the 15th international World Wide Web conference,2006.[17]Tam�s Sarl�s,Andr�s A.Bencz�r,K�rolyCsalog�ny,D�niel Fogaras,and Bal�zs R�cz.To randomize or not to randomize:Space optimalsummaries for hyperlink analysis.In Proc.of the15th international World Wide Web conference,2006. [18]Yiming Yang,Sean Slattery,and Rayid Ghani.Astudy of approaches to hypertext categorization.Journal of Intelligent Information Systems,18(2-3):219 241,2002.[19]Ka-Ping Yee,Kirsten Swearingen,Kevin Li,andMarti A.Hearst.Faceted metadata for image searchand browsing.In Proc.of the SIGCHI Conf.onHuman Factors in Computing Systems,pages401 408,2003.Table1:Sample keywords from Google AdWordsBusiness:information technology;market share;strategic planning;supply chain management;swot analysis Business/Textiles and Nonwovens/Textiles/ Carpets:rugs; oor covering;persian carpet;oriental weavers;hardwood oorComputers:laptops;workstations; at screen;second hand computers;midwest microComputers/Internet/Searching:search the web;web browser;front crawl;search engines;internet searching Recreation:recreation jobs;parks and recreation;nude recreation;recreation and leisure;lake mead recreation Recreation/Travel/Travelogues:travelogue game; travel diary;travelogues;travel adventures;travel phots Sports:n ;athletics;stadiums;formula one;basketball Sports/Soccer/UEF A/England/Women:women soccer uk;football girls;ladies soccer;girls fc;manchester ladies。