Data Analysis
数据分析 英语作文
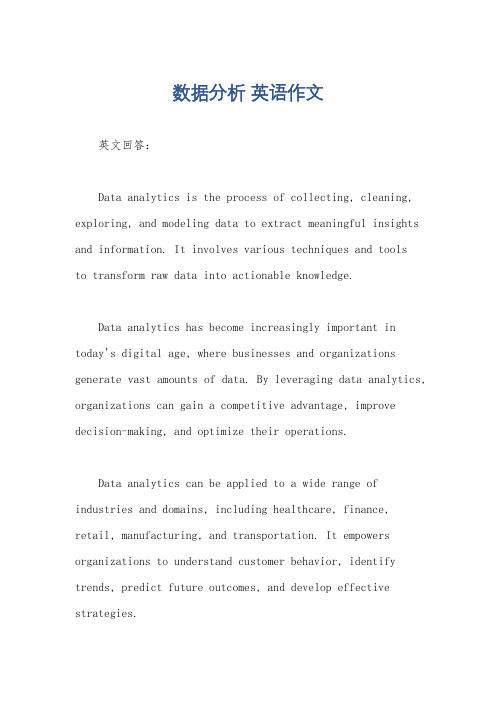
数据分析英语作文英文回答:Data analytics is the process of collecting, cleaning, exploring, and modeling data to extract meaningful insights and information. It involves various techniques and toolsto transform raw data into actionable knowledge.Data analytics has become increasingly important in today's digital age, where businesses and organizations generate vast amounts of data. By leveraging data analytics, organizations can gain a competitive advantage, improve decision-making, and optimize their operations.Data analytics can be applied to a wide range of industries and domains, including healthcare, finance, retail, manufacturing, and transportation. It empowers organizations to understand customer behavior, identify trends, predict future outcomes, and develop effective strategies.There are two main types of data analytics: descriptive and predictive. Descriptive analytics provides insightsinto historical data, while predictive analytics uses statistical models and machine learning algorithms to forecast future events and trends.Data analytics is a multidisciplinary field that draws on statistics, mathematics, computer science, and business knowledge. Data analysts use a variety of tools and techniques, including data visualization, statistical modeling, machine learning, and data mining.The process of data analytics typically involves the following steps:1. Data collection: Gathering data from various sources, such as databases, surveys, and sensors.2. Data cleaning: Removing errors, inconsistencies, and duplicate data.3. Data exploration: Analyzing the data to identify patterns, trends, and outliers.4. Data modeling: Developing statistical or machine learning models to predict future outcomes.5. Data visualization: Presenting the results of data analysis in a clear and understandable way.Data analytics has numerous benefits for organizations, including:Improved decision-making: Data-driven insights enable organizations to make more informed decisions.Increased efficiency: Data analytics can help organizations identify inefficiencies and optimize their processes.Enhanced customer satisfaction: By understanding customer behavior, organizations can improve their products and services.Competitive advantage: Data analytics can provide organizations with a competitive edge by identifying new opportunities and threats.中文回答:数据分析是一项收集、清洗、探索和建模数据的过程,以提取有意义的见解和信息。
Multivariate Data Analysis
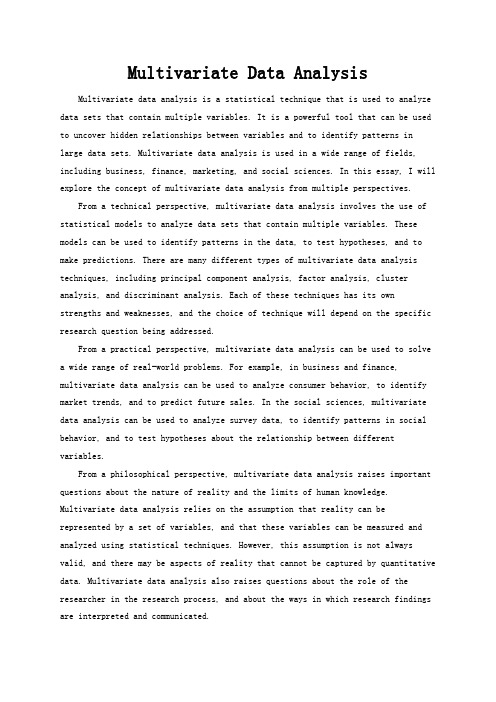
Multivariate Data Analysis Multivariate data analysis is a statistical technique that is used to analyze data sets that contain multiple variables. It is a powerful tool that can be used to uncover hidden relationships between variables and to identify patterns in large data sets. Multivariate data analysis is used in a wide range of fields, including business, finance, marketing, and social sciences. In this essay, I will explore the concept of multivariate data analysis from multiple perspectives.From a technical perspective, multivariate data analysis involves the use of statistical models to analyze data sets that contain multiple variables. These models can be used to identify patterns in the data, to test hypotheses, and to make predictions. There are many different types of multivariate data analysis techniques, including principal component analysis, factor analysis, cluster analysis, and discriminant analysis. Each of these techniques has its own strengths and weaknesses, and the choice of technique will depend on the specific research question being addressed.From a practical perspective, multivariate data analysis can be used to solve a wide range of real-world problems. For example, in business and finance, multivariate data analysis can be used to analyze consumer behavior, to identify market trends, and to predict future sales. In the social sciences, multivariate data analysis can be used to analyze survey data, to identify patterns in social behavior, and to test hypotheses about the relationship between different variables.From a philosophical perspective, multivariate data analysis raises important questions about the nature of reality and the limits of human knowledge. Multivariate data analysis relies on the assumption that reality can be represented by a set of variables, and that these variables can be measured and analyzed using statistical techniques. However, this assumption is not always valid, and there may be aspects of reality that cannot be captured by quantitative data. Multivariate data analysis also raises questions about the role of the researcher in the research process, and about the ways in which research findings are interpreted and communicated.From a social perspective, multivariate data analysis has the potential toboth empower and disempower individuals and groups. On the one hand, multivariate data analysis can be used to identify patterns of inequality and discrimination, and to advocate for social change. On the other hand, multivariate data analysis can be used to reinforce existing power structures, and to perpetuate social inequality. It is important for researchers to be aware of these potential effects, and to use multivariate data analysis in a responsible and ethical manner.From a personal perspective, multivariate data analysis can be bothchallenging and rewarding. It requires a high level of technical skill and expertise, as well as a deep understanding of the research question being addressed. However, it can also be incredibly satisfying to uncover hidden relationships between variables, and to use statistical models to make predictions and test hypotheses. Multivariate data analysis also requires a high level of attention to detail and a willingness to engage in iterative processes of data cleaning, analysis, and interpretation.In conclusion, multivariate data analysis is a powerful tool that can be used to analyze complex data sets and to uncover hidden relationships between variables. It has many practical applications in a wide range of fields, and raises important questions about the nature of reality and the limits of human knowledge. It also has the potential to both empower and disempower individuals and groups, and requires researchers to use it in a responsible and ethical manner. From apersonal perspective, multivariate data analysis can be both challenging and rewarding, and requires a high level of technical skill and attention to detail.。
数据分析英语怎么说
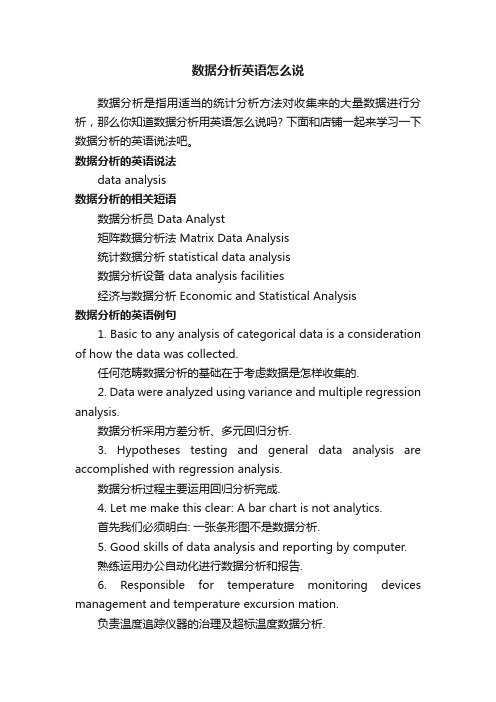
数据分析英语怎么说数据分析是指用适当的统计分析方法对收集来的大量数据进行分析,那么你知道数据分析用英语怎么说吗? 下面和店铺一起来学习一下数据分析的英语说法吧。
数据分析的英语说法data analysis数据分析的相关短语数据分析员 Data Analyst矩阵数据分析法 Matrix Data Analysis统计数据分析 statistical data analysis数据分析设备 data analysis facilities经济与数据分析 Economic and Statistical Analysis数据分析的英语例句1. Basic to any analysis of categorical data is a consideration of how the data was collected.任何范畴数据分析的基础在于考虑数据是怎样收集的.2. Data were analyzed using variance and multiple regression analysis.数据分析采用方差分析、多元回归分析.3. Hypotheses testing and general data analysis are accomplished with regression analysis.数据分析过程主要运用回归分析完成.4. Let me make this clear: A bar chart is not analytics.首先我们必须明白: 一张条形图不是数据分析.5. Good skills of data analysis and reporting by computer.熟练运用办公自动化进行数据分析和报告.6. Responsible for temperature monitoring devices management and temperature excursion mation.负责温度追踪仪器的治理及超标温度数据分析.7. Good ability at test design and operating, data analysis and summary.能独立进行测试计划设计,测试操作, 及进行数据分析汇总.8. In data analysis, congregate is a process of large amount original data.汇总被广泛应用于数据分析, 是一种对原始数据进行统计加工整理的过程.9. In chapter 6, the results of experiments and data analysis are provided.第六章给出了该系统在正式测试中的结果以及数据分析.10. SPM 99 was used to process data and localize functional areas.采用SPM99软件进行数据分析和脑功能区定位.11. Provide HR information analysis report and track the progress.提交人力资源数据分析报告并督导执行.12. VDA dynamically identifies different protocol segments in a packet.垂直数据分析(VDA)动态识别一个包中不同的协议段.13. The original analysis detected storm - driven swell shaking the ice.通过最初的数据分析发现,猛烈的膨胀会对冰产生动摇作用.14. Providing daily operational analysis report to relevant personals and project managers.为项目负责人及相关人员提供日常运营数据分析报告.15. The reliability life data analysis is an important basis of reliability engineering.可靠性寿命数据分析是可靠性工程研究的重要基础.数据分析相关阅读:数据会撒谎大数据并非万能Clay Christensen tells a good joke about a tour of heaven.克雷.克里斯坦森(Clay Christensen)讲了一个有关天堂旅游的有趣笑话。
qualitative data analysis a methods sourcebook

qualitative data analysis a methods sourcebook "Qualitative Data Analysis:A Methods Sourcebook"是一本关于定性数据分析方法的书籍。
它提供了深入的见解
和实用的指导,帮助读者理解和应用各种定性数据分析方法。
这本书详细介绍了定性数据分析的基本概念、方法和步骤,
包括数据收集、编码和分析等方面。
通过实例和案例研究,
它演示了如何使用不同的定性数据分析方法来解决实际问
题,并提供了一系列实用的技巧和工具,以帮助读者更好地
理解和解释定性数据。
此外,这本书还强调了定性数据分析在社会科学、市场营销、
组织研究和人力资源管理等领域中的应用,展示了如何利用
定性数据分析方法来探索和解释各种现象和问题。
总的来说,"Qualitative Data Analysis:A Methods Sourcebook"是一本对于学习和应用定性数据分析方法非常有用的书籍,它为读者提供了全面、深入和实用的指导和支持。
Multivariate Data Analysis

Multivariate Data Analysis Multivariate Data Analysis is a powerful statistical technique used to analyze and interpret data that involves multiple variables. It helps in understanding the relationships between different variables and making predictions based on these relationships. This technique is widely used in various fields such as finance, marketing, social sciences, and healthcare, among others. In this response, I will discuss the importance of multivariate data analysis from multiple perspectives. From a business perspective, multivariate data analysis is crucial for making informed decisions. Businesses collect a vast amount of data from various sources, such as customer surveys, sales records, and market research. By applying multivariate data analysis techniques, businesses can uncover patterns and correlations within this data, which can help them identify key factorsinfluencing their success or failure. For example, a company may use multivariate analysis to determine the impact of different marketing strategies on sales performance. This information can then be used to optimize marketing campaigns and allocate resources effectively. From a scientific perspective, multivariate data analysis plays a vital role in research. Scientists often collect data from multiple variables in their experiments or studies. By employing multivariate analysis, they can examine the relationships between these variables and gain a deeper understanding of the underlying mechanisms or processes. For instance, in a medical study, researchers may use multivariate analysis to investigate the relationship between various risk factors and the occurrence of a particular disease. This analysis can help identify the most significant risk factors and develop preventive measures or treatment strategies accordingly. From a social sciences perspective, multivariate data analysis is essential for studying complex social phenomena. Social scientists often deal with data that involves multiple variables, such as demographic information, socioeconomic factors, and attitudes. By using multivariate analysis, they can explore the relationships between these variables and uncover underlying patterns or trends. For example, sociologists may use multivariate analysis to examine the impact of education, income, and race on social mobility. This analysis can provide valuable insights into the factors that contribute to or hinder social mobility, which can inform policy decisions andinterventions. From a healthcare perspective, multivariate data analysis is critical for improving patient outcomes and healthcare delivery. In the healthcare field, data is collected from various sources, including patient records, medical tests, and treatment outcomes. By applying multivariate analysis, healthcare professionals can identify risk factors, predict disease progression, and evaluate treatment effectiveness. For instance, in a clinical trial, researchers may use multivariate analysis to assess the impact of different treatment regimens on patient outcomes. This analysis can help determine the most effective treatment approach and improve patient care. In conclusion, multivariate data analysis is a valuable technique that offers numerous benefits from various perspectives. It enables businesses to make informed decisions, scientists to gain insights into complex phenomena, social scientists to understand social dynamics, and healthcare professionals to improve patient outcomes. By analyzing data from multiple variables, multivariate analysis provides a comprehensive and holistic understanding of the relationships between different factors. Its application is extensive and spans across different fields, making it an essential tool for data-driven decision-making and research.。
bruker data analysis 拟合分子量
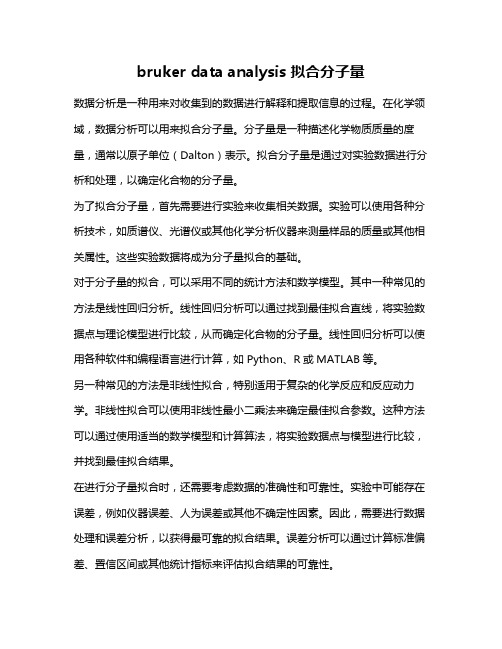
bruker data analysis 拟合分子量数据分析是一种用来对收集到的数据进行解释和提取信息的过程。
在化学领域,数据分析可以用来拟合分子量。
分子量是一种描述化学物质质量的度量,通常以原子单位(Dalton)表示。
拟合分子量是通过对实验数据进行分析和处理,以确定化合物的分子量。
为了拟合分子量,首先需要进行实验来收集相关数据。
实验可以使用各种分析技术,如质谱仪、光谱仪或其他化学分析仪器来测量样品的质量或其他相关属性。
这些实验数据将成为分子量拟合的基础。
对于分子量的拟合,可以采用不同的统计方法和数学模型。
其中一种常见的方法是线性回归分析。
线性回归分析可以通过找到最佳拟合直线,将实验数据点与理论模型进行比较,从而确定化合物的分子量。
线性回归分析可以使用各种软件和编程语言进行计算,如Python、R或MATLAB等。
另一种常见的方法是非线性拟合,特别适用于复杂的化学反应和反应动力学。
非线性拟合可以使用非线性最小二乘法来确定最佳拟合参数。
这种方法可以通过使用适当的数学模型和计算算法,将实验数据点与模型进行比较,并找到最佳拟合结果。
在进行分子量拟合时,还需要考虑数据的准确性和可靠性。
实验中可能存在误差,例如仪器误差、人为误差或其他不确定性因素。
因此,需要进行数据处理和误差分析,以获得最可靠的拟合结果。
误差分析可以通过计算标准偏差、置信区间或其他统计指标来评估拟合结果的可靠性。
在实际应用中,分子量拟合可以用于许多化学研究和应用领域。
例如,在药物研发中,分子量拟合可以用来确定药物分子的质量,从而评估其活性和药理学性质。
在环境科学领域,分子量拟合可以用来研究有机物质的来源和分布。
在聚合物化学中,分子量拟合可以用来确定聚合物链的长度和分子量分布。
总之,数据分析是一种重要的工具,可以用来拟合分子量。
通过合理选择统计方法和数学模型,结合实验数据和误差分析,可以获得准确和可靠的分子量拟合结果。
这种方法在化学研究和实际应用中具有广泛的应用前景。
data analysis算质谱片段分子量
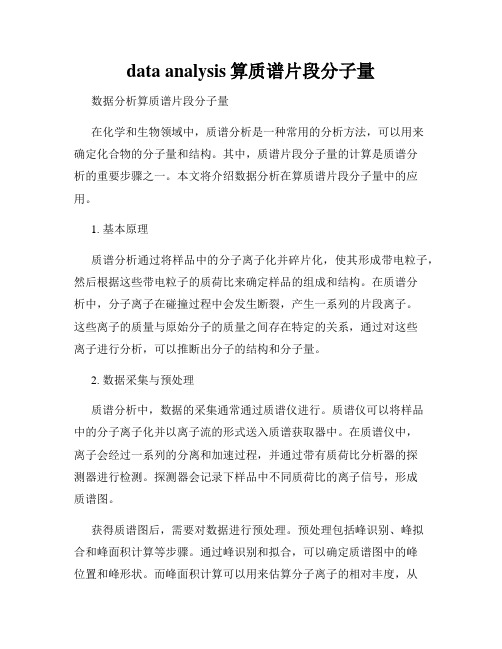
data analysis算质谱片段分子量数据分析算质谱片段分子量在化学和生物领域中,质谱分析是一种常用的分析方法,可以用来确定化合物的分子量和结构。
其中,质谱片段分子量的计算是质谱分析的重要步骤之一。
本文将介绍数据分析在算质谱片段分子量中的应用。
1. 基本原理质谱分析通过将样品中的分子离子化并碎片化,使其形成带电粒子,然后根据这些带电粒子的质荷比来确定样品的组成和结构。
在质谱分析中,分子离子在碰撞过程中会发生断裂,产生一系列的片段离子。
这些离子的质量与原始分子的质量之间存在特定的关系,通过对这些离子进行分析,可以推断出分子的结构和分子量。
2. 数据采集与预处理质谱分析中,数据的采集通常通过质谱仪进行。
质谱仪可以将样品中的分子离子化并以离子流的形式送入质谱获取器中。
在质谱仪中,离子会经过一系列的分离和加速过程,并通过带有质荷比分析器的探测器进行检测。
探测器会记录下样品中不同质荷比的离子信号,形成质谱图。
获得质谱图后,需要对数据进行预处理。
预处理包括峰识别、峰拟合和峰面积计算等步骤。
通过峰识别和拟合,可以确定质谱图中的峰位置和峰形状。
而峰面积计算可以用来估算分子离子的相对丰度,从而反映分子裂解的程度。
这些预处理步骤将为质谱片段分子量的计算提供必要的数据基础。
3. 片段分子量的计算方法质谱片段分子量的计算可以通过多种方法实现,其中最常用的方法是基于质谱数据与已知化合物库的比对。
这种方法利用已知化合物库中的质谱数据(包括分子离子峰和碎片离子峰)与待测化合物的质谱数据进行对比,通过计算两者之间的相似度来推断待测化合物的分子量。
此外,还可以利用分子离子峰和碎片离子峰之间的互相关关系来推断待测化合物的分子量。
这个方法基于碎片离子之间碰撞过程中的质能守恒关系,通过计算碎片离子之间的质荷比比值,可以推算出待测化合物的分子量。
4. 数据分析工具与软件为了实现质谱片段分子量的计算,需要借助一系列的数据分析工具与软件。
analysis名词
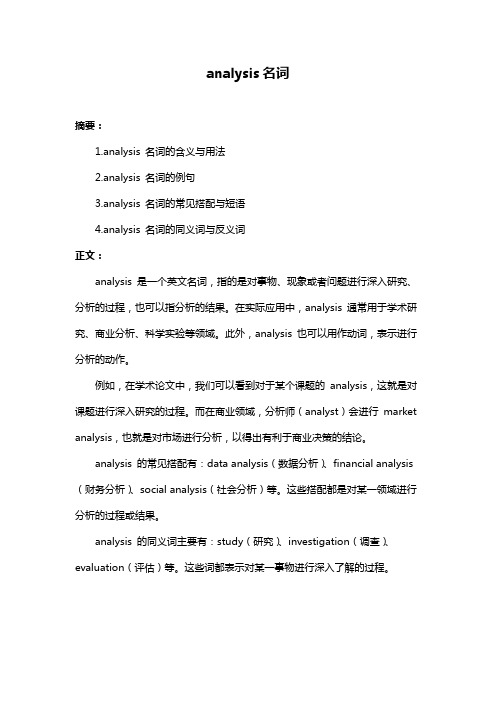
analysis名词
摘要:
1.analysis 名词的含义与用法
2.analysis 名词的例句
3.analysis 名词的常见搭配与短语
4.analysis 名词的同义词与反义词
正文:
analysis 是一个英文名词,指的是对事物、现象或者问题进行深入研究、分析的过程,也可以指分析的结果。
在实际应用中,analysis 通常用于学术研究、商业分析、科学实验等领域。
此外,analysis 也可以用作动词,表示进行分析的动作。
例如,在学术论文中,我们可以看到对于某个课题的analysis,这就是对课题进行深入研究的过程。
而在商业领域,分析师(analyst)会进行market analysis,也就是对市场进行分析,以得出有利于商业决策的结论。
analysis 的常见搭配有:data analysis(数据分析)、financial analysis (财务分析)、social analysis(社会分析)等。
这些搭配都是对某一领域进行分析的过程或结果。
analysis 的同义词主要有:study(研究)、investigation(调查)、evaluation(评估)等。
这些词都表示对某一事物进行深入了解的过程。
- 1、下载文档前请自行甄别文档内容的完整性,平台不提供额外的编辑、内容补充、找答案等附加服务。
- 2、"仅部分预览"的文档,不可在线预览部分如存在完整性等问题,可反馈申请退款(可完整预览的文档不适用该条件!)。
- 3、如文档侵犯您的权益,请联系客服反馈,我们会尽快为您处理(人工客服工作时间:9:00-18:30)。
Final Project of 805Jing Zhao1. DescriptionBefore I come to America from China, many people have told me that the public security is poor in some places of America. So I noticed the data set in my SAS book of my undergraduate study. (The name of the book is “The Methods of Data Analysis and The System of SAS”). And the data set is the ratio of commitment of 4 kinds of crime in 50 states of America. I have checked the reference of the book and noticed that the data is from /about-us/cjis/ucr/ucr/ which is the website of FBI. I want to analysis the relationship between those three kinds of crime which are rape crime, assault crime and robbery with the murder crime. Since the murder crime is more serious and more evil than those three. And I want to know which of the three are more important to the commitment of murder. And if any of those three crimes will cause more murder when the ratio of them increase. So I use the data set.I set the ratio of rape crime as x1, the ratio of robbery as x2 and the ratio of assault crime as x3 these three predictors. I also set murder as y. There will be 50 observationsin the data set.2. Data SetThe chance of commitments of four kinds of crime in 50 states of America in every 100,000 people.3. Analysis the data set(1). Select the modelWe want to know the relationship between murder crime and other three kinds of crime. So we let y equals the murder ratio, and x1 is the ratio of rape crime, and x2 is the ratio of robbery, and x3 is the ratio of assault crime.>mydata=read.table("C:/Users/jacky/Desktop/crime.txt")> y=mydata[,1] #murder>x1=mydata[,2] #rape crime>x2=mydata[,3] #robbery>x3=mydata[,4] #assaultSince we have 3 predictors so we will have 3217-=kinds of regressions.> LM1=lm(y~x1)> LM2=lm(y~x2)> LM3=lm(y~x3)> LM4=lm(y~x1+x2)> LM5=lm(y~x1+x3)> LM6=lm(y~x2+x3)> LM7=lm(y~x1+x2+x3)These are all possible models. And then we want to check which is the best and most simple one. We use the AIC method.> n=length(y)>AIC(LM1,k=2)[1] 259.6966>AIC(LM2,k=2)[1] 268.7988>AIC(LM3,k=2)[1] 254.836>AIC(LM4,k=2)[1] 259.6864>AIC(LM5,k=2)[1] 253.9535>AIC(LM6,k=2)[1] 254.9248>AIC(LM7,k=2)[1] 255.1303Now, we can see that LM5( That is y~x1+x3) is the best, since AIC(LM5,k=2)=253.9535 is the smallest AIC value.Then we use 2R and 2R to check the result.adj>summary(LM1)Call:lm(formula = y ~ x1)Residuals:Min 1Q Median 3Q Max-6.1400 -1.8733 -0.4707 1.7497 8.1813Coefficients:Estimate Std. Error t value Pr(>|t|)(Intercept) 1.88378 1.15443 1.632 0.109x1 0.21607 0.04145 5.213 3.89e-06 ***---Signif.codes: 0 ‘***’ 0.001 ‘**’ 0.01 ‘*’ 0.05 ‘.’ 0.1 ‘ ’ 1Residual standard error: 3.122 on 48 degrees of freedomMultiple R-squared: 0.3615, Adjusted R-squared: 0.3482F-statistic: 27.17 on 1 and 48 DF, p-value: 3.892e-06>summary(LM2)Call:lm(formula = y ~ x2)Residuals:Min 1Q Median 3Q Max-5.2968 -2.9440 -0.2921 2.2146 8.0922Coefficients:Estimate Std. Error t value Pr(>|t|) (Intercept) 4.816907 0.839399 5.739 6.27e-07 ***x2 0.021171 0.005529 3.829 0.000373 *** ---Signif.codes: 0 ‘***’ 0.001 ‘**’ 0.01 ‘*’ 0.05 ‘.’ 0.1 ‘ ’ 1Residual standard error: 3.419 on 48 degrees of freedom Multiple R-squared: 0.234, Adjusted R-squared: 0.218 F-statistic: 14.66 on 1 and 48 DF, p-value: 0.0003727>summary(LM3)Call:lm(formula = y ~ x3)Residuals:Min 1Q Median 3Q Max-4.8518 -2.2835 -0.5756 1.5642 7.4113Coefficients:Estimate Std. Error t value Pr(>|t|) (Intercept) 2.158405 0.989237 2.182 0.0340 *x3 0.025015 0.004238 5.903 3.52e-07 *** ---Signif.codes: 0 ‘***’ 0.001 ‘**’ 0.01 ‘*’ 0.05 ‘.’ 0.1 ‘ ’ 1Residual standard error: 2.974 on 48 degrees of freedom Multiple R-squared: 0.4206, Adjusted R-squared: 0.4085 F-statistic: 34.85 on 1 and 48 DF, p-value: 3.524e-07>summary(LM4)Call:lm(formula = y ~ x1 + x2)Residuals:Min 1Q Median 3Q Max-5.405 -1.775 -0.569 1.429 8.428Coefficients:Estimate Std. Error t value Pr(>|t|)(Intercept) 1.892178 1.143444 1.655 0.10463x1 0.174204 0.050935 3.420 0.00130 ** x2 0.008613 0.006203 1.389 0.17151---Signif.codes: 0 ‘***’ 0.001 ‘**’ 0.01 ‘*’ 0.05 ‘.’ 0.1 ‘ ’ 1Residual standard error: 3.092 on 47 degrees of freedom Multiple R-squared: 0.3866, Adjusted R-squared: 0.3605 F-statistic: 14.81 on 2 and 47 DF, p-value: 1.027e-05>summary(LM5)Call:lm(formula = y ~ x1 + x3)Residuals:Min 1Q Median 3Q Max-5.221 -2.009 -0.512 1.771 7.832Coefficients:Estimate Std. Error t value Pr(>|t|) (Intercept) 1.296838 1.099797 1.179 0.24427x1 0.096301 0.057662 1.670 0.10155x3 0.017364 0.006189 2.806 0.00728 ** ---Signif.codes: 0 ‘***’ 0.001 ‘**’ 0.01 ‘*’ 0.05 ‘.’ 0.1 ‘ ’ 1Residual standard error: 2.92 on 47 degrees of freedom Multiple R-squared: 0.4531, Adjusted R-squared: 0.4298 F-statistic: 19.47 on 2 and 47 DF, p-value: 6.939e-07>summary(LM6)Call:lm(formula = y ~ x2 + x3)Residuals:Min 1Q Median 3Q Max-5.1240 -1.8898 -0.5108 1.8066 7.7803Coefficients:Estimate Std. Error t value Pr(>|t|) (Intercept) 2.000245 0.987719 2.025 0.048556 *x2 0.007769 0.005741 1.353 0.182448x3 0.021201 0.005059 4.191 0.000122 *** ---Signif.codes: 0 ‘***’ 0.001 ‘**’ 0.01 ‘*’ 0.05 ‘.’ 0.1 ‘ ’ 1Residual standard error: 2.948 on 47 degrees of freedomMultiple R-squared: 0.4423, Adjusted R-squared: 0.4186 F-statistic: 18.64 on 2 and 47 DF, p-value: 1.095e-06>summary(LM7) Call:lm(formula = y ~ x1 + x2 + x3)Residuals:Min 1Q Median 3Q Max -4.8858 -1.8890 -0.4094 1.8296 8.0070Coefficients:Estimate Std. Error t value Pr(>|t|) (Intercept) 1.343193 1.103849 1.217 0.2299 x1 0.079151 0.061048 1.297 0.2013 x2 0.005260 0.006019 0.874 0.3867 x3 0.016144 0.006359 2.539 0.0146 * ---Signif.codes: 0 ‘***’ 0.001 ‘**’ 0.01 ‘*’ 0.05 ‘.’ 0.1 ‘ ’ 1Residual standard error: 2.927 on 46 degrees of freedomMultiple R-squared: 0.462, Adjusted R-squared: 0.4269 F-statistic: 13.17 on 3 and 46 DF, p-value: 2.459e-06Now we can see that in the same size of predictor the 2R of LM5 is the largest and it equals 0.4531. And in the different size of predictor the 2adj R of LM5 is also the largest and it equals 0.4298. Also we know the model is131.2968380.096301*0.017364*Y X X =++(2). Use Hypothesis test to check the modelWe set 0212:0 :0H vs H ββ=≠. And the significant level 0.01α=>anova(LM7)Analysis of Variance TableResponse: yDf Sum Sq Mean Sq F value Pr(>F)x1 1 264.83 264.83 30.9064 1.318e-06 *** x2 1 18.44 18.44 2.1515 0.14924 x3 1 55.22 55.22 6.4450 0.01457 * Residuals 46 394.16 8.57 ---Signif.codes: 0 ‘***’ 0.001 ‘**’ 0.01 ‘*’ 0.05 ‘.’ 0.1 ‘ ’ 1 >qf(1-0.01,p-1,n-p) [1] 5.087373Now we can see that since F(=2.1515)<qf(1-0.01,p-1,n-p)=5.087373 so we include that we fail to reject H0. That is 20β=.(3). Check the multicollinearity of the modelNow we want to check the multicollinearity of the model131.2968380.096301*0.017364*Y X X =++.>source("/~cspark/805/R/VIF.R") >vif(LM5)x1 x32.212312 2.212312We can see that since max(VIF1,VIF2)<10. So there is no multicollinearity in the model.(4). Check the Boxcox transformation.>source("/~cspark/805/R/bxcx.R")>source("/~cspark/805/R/BoxCox.R") #Box cox trans> y1=fitted(LM5)> e1=y-y1> plot(y,e1)> lam=seq(-1,1,0.1)> SSE=BoxCox(y~x1+x3,lambda=lam,SSE=TRUE)>cbind(lam,SSE)lam SSE[1,] -1.0 1726.2062[2,] -0.9 1412.3691[3,] -0.8 1170.1879[4,] -0.7 982.4830[5,] -0.6 836.4204[6,] -0.5 722.3825[7,] -0.4 633.1395[8,] -0.3 563.2405[9,] -0.2 508.5651[10,] -0.1 465.9918[11,] 0.0 433.1527[12,] 0.1 408.2517[13,] 0.2 389.9292[14,] 0.3 377.1613[15,] 0.4 369.1853[16,] 0.5 365.4434[17,] 0.6 365.5417[18,] 0.7 369.2195[19,] 0.8 376.3275[20,] 0.9 386.8112[21,] 1.0 400.7009Since when lam=0.5 then the SSE is the smallest one which equals 365.4434. So lam should be 0.5. And then check if lambda=0.5 is better.>yprime=sqrt(y)>LM.prime=lm(yprime~x1+x3) > plot(x1+x3,yprime)>plot(lam,SSE)1002003004005001.01.52.02.53.03.54.x1 + x3y p r i m e-1.0-0.50.00.5 1.0400600800100012001400160lamS S E>qqnorm(e1)Now from the pictures above we know that when lambda=0.5 is better.-2-1012-4-202468Normal Q-Q PlotTheoretical QuantilesS a m p l e Q u a n t i l e s(5). Matrix Plot>colnames(mydata)=c("Murder","RapeCrime","Robbery","Assault") >pairs(mydata, cex=0.5, pch=1)>cor(mydata)Murder Rape Crime Robbery Assault Murder 1.0000000 0.6012205 0.4837076 0.6485505 Rape Crime 0.6012205 1.0000000 0.5918793 0.7402595 Robbery 0.4837076 0.5918793 1.0000000 0.5570782 Assault 0.6485505 0.7402595 0.5570782 1.0000000We confirm the model again from the Matrix plotMurder102030405010020030040050051015102030405Rape CrimeRobbery010030051015100200300400500100300Assault(6). Check the constancy of error varianceWe assume that0:H the error variance is constant vs1:H the error variance is not constant>source("/~cspark/805/R/Breusch-Pagan.R") #constancy of error variance>>BP.test(y~x1+x3)$test.stat[1] 0.8323462$df[1] 2$p.value[1] 0.6595661>qchisq(1-0.01,2)[1] 9.21034Since 0.8323462<qchisq(1-0.01,2)=9.21034, so we reject H1. That is the error variance is constant.(7). Check the independence of the predictorsNow we want to check the independence of x1 and x3.> LM8=lm(y~x3+x1)>anova(LM8)Analysis of Variance TableResponse: yDf Sum Sq Mean Sq F value Pr(>F)x3 1 308.16 308.16 36.1458 2.58e-07 ***x1 1 23.78 23.78 2.7892 0.1015Residuals 47 400.70 8.53---Signif.codes: 0 ‘***’ 0.001 ‘**’ 0.01 ‘*’ 0.05 ‘.’ 0.1 ‘ ’ 1>anova(LM3) # We can see that SSR(x3)=SSR(x3|x1) So independent. Analysis of Variance TableResponse: yDf Sum Sq Mean Sq F value Pr(>F)x3 1 308.16 308.16 34.847 3.524e-07 *** Residuals 48 424.48 8.84 ---Signif.codes : 0 ‘***’ 0.001 ‘**’ 0.01 ‘*’ 0.05 ‘.’ 0.1 ‘ ’ 1 >Since SSR(x3)=SSR(x3|x1), so the two predictors are independent.(8). Check the linearity of y and residual.> y1=fitted(LM5) > e1=y-y1 > plot(y,e1)51015-4-202468ye 1(9). Check if the residual follows the norm distribution> e2=resid(LM5) > c1=sort(e2)>LM.sum=summary(LM5) > s=LM.sum[["sigma"]] > k=1:n> c2=(k-0.375)/(n+0.25) > c3=qnorm(c2) > c4=s*c3 >plot(c4,c1) >lines(c4,c4)>cor=cor(c4,c1) >cor[1] 0.9860581We can see that the error terms almost normally distribute.-6-4-20246-4-202468c4c 1(10). Check if x1 and x3 are independent with residual.>plot(x1,e1)> plot(x3,e1)We can see that x1 or x3 are independent with residual.1020304050-4-202468x1e 1100200300400500-4-202468x3e 1(11). Check the median of the residuals and the maximum and minimum of the residuals.>boxplot(e1) #check the median of the residuals and the maximum and minimum of the residuals.We can obtain the median of the residuals and the maximum and minimum of the residuals from the picture.-4-202468。