Learning Surface Text Patterns for a Question Answering System
新视野大学英语视听说教程2第三版BOOK2 UNIT4
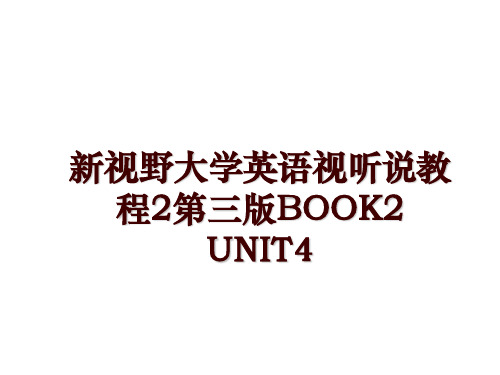
Listening to the world
Sharing
4 Watch Part 2 and fill in the blanks.
Additional Notes
Bob Dylan: He has been recording and performing
since the 1960s, mixing many of the traditions in American song, from folk, blues, and country to gospel, rock and roll. Hailed as the Shakespeare of his generation, Dylan sold more than 58 million albums, wrote more than 500 songs recorded by more than 2,000 artists, performed all over the world, and set the standard for lyric writing. As a songwriter and musician, he has received 11 Grammy Awards, one Academy Award and one Golden Globe Award. In 2000, he was awarded the Polar Music Prize, and in 2012 the Presidential Medal of Freedom.
Listening to the world
Sharing
4 Watch Part 2 and fill in the blanks.
Additional Notes
新编实用英语综合教程1Unit5Ourweatherandclimate

Unit Five Our W eather and ClimateSection I& II Listening and SpeakingTalking Face to FaceI W arm-up Questions1. Do you often listen to a weather forecast? Why or why not?2. What’s the use and importance of a weather forecast?II Class Activities1.The students read the Mini-Talks after the teacher, and then try to recitethem within five minutes in pairs.2.Students discuss in groups, summarizing the words, phrases andsentences frequently used according to the following topics with the help of the teacher. The students speak out the sentences under the guidance of the teacher, paying attention to the pronunciation and the intonation.1)Sentences for a weatherman to present weather forecasts:(1) Good morning. This is the local weather report.(2) Here is the national forecast.(3) Now let’s look at the weather across the country.(4) Rains will be expected tomorrow from the south to the north.(5) Snow is going to continue through tomorrow in this area.(6) The weatherman says that frost is on its way.2) Sentences for talking about weather changes:(1) It’ll be mild, and later turn to partly cloudy, with the southeast wind.(2) Today will be cool and partly cloudy, with a chance of rain this afternoon.(3) Tomorrow will be overcast with drizzle.(4) In the evening there’s good chance that we’ll get some snow.(5) Today is a cloudy and cool day with a low of 12 degrees.(6) The weather will change overnight with a high temperature of zerodegrees.3) Sentences about weather for starting a conversation:(1) What’s the weather like today?(2) What is the weather report?(3) What’s the temperature?(4) What’s it like outside?(5) Will it be a nice day?(6) Lovely day, isn’t it?(7) What do you think of the weather here?3. Act-Out Activities1) Students read the sample dialogues after the teacher, trying to imitate theteacher\s pronunciation and intonation.2) Ask the students to read the sample dialogues in pairs. Then categorize theexpressions for asking questions about the weather and describing the weather conditions.4. Do Exercises 5 and 6 in pairs.Being all earsⅢ. Learning Sentences for W orkplace Communication1.Warm–up:Give the students a few minutes to read through the printed materials for each listening item in Listen and Repeat, Listen and Match. While listening, students should try to remember the meaning of each of the sentences and pay more attention to the key words.fairly 相当地mild 温和的,暖和的2. Key to Listen and Match:1-f, 2-h, 3-i 4-j, 5-g, 6-c, 7-e, 8-d, 9-a, 10-bListen and RespondKey: 1-D 2-C 3-B 4-D 5-A 6-D3. Handling a Dialogue:Script:Li Hong: Hi, Pat! Why didn’t you go to the party last night?Pat: Because it was so cold and rainy.Li Hong: That’s too bad! It was a really good party. Hey, why don’t we go out for a walk this afternoon, Pat? I need some exercise.Pat: Go out for a walk? But its so cold out.Li Hong: Cold out? Wh at’s the temperature?Pat: About 13℃.Li Hong: 13℃? That’s not cold. Just wait until winter.Pat: Why?Li Hong: Well …it snows a lot and sometimes it’s very cold. Last winter it was 7 degree below zero for three weeks. And it was windy, too.Pat: That sounds awful!Li Hong: It wasn’t all that bad. The sun was out almost every day.4. Understanding a short Speech / TalkKey: 1. tourist cities 2. beautiful3. too cold in winter4. plenty of sunshine5. the sea6. thousands of7. from both home and abroad8. at the best time of yearUnit Five Our W eather and ClimateSection III Trying your handI. Sample Analysis1. The teacher summarize briefly the format and language used in weatherforecasts.2. A weather report or forecast is a very useful aid in our daily life. Knowingthe usual format for giving a weather forecast helps us a lot in understanding a weather forecast in English. Figures, measurement units, graphics, weather terms, and broken short passages are often used to forecast weather conditions. In general, the language used to forecast weather should be concise, clear, familiar and vivid.II. Simulated Writing1. The students read and translate the two sample weather forecasts intoChinese.Useful words and expressions for describing weather:1) to rain all day 全天有雨2) to be sunny / fine / cloudy / rainy / hot / cool天气将会是阳光充足、晴、多云、有雨、很热、凉爽3) to be going to warm up / clear up 天气要转暖/放晴4) quite a warm day with temperature around 24℃天气很暖和,气温在24度左右。
模具专业英语课件-unitfour
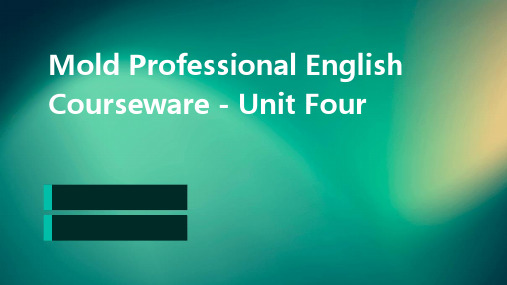
necessary adjustments before the mold is put into service."
Sentence patterns for mold usage and maintenance
Operating the mold
"When operating the mold, ensure that it is properly aligned and secured. Monitor the temperature and pressure during production runs."
Discussing mold design modifications
"After testing the mold, we've identified some areas that need to be modified for better part release. The ejector pins need to be adjusted and the gate location optimized."
Understanding mold design drawings
It is important to understand the purpose and function of each part of the mold, including the mold base, cavity, core, and other components. This helps in better understanding of the mold design and manufacturing process.
小学上册第二次英语第1单元寒假试卷[含答案]
![小学上册第二次英语第1单元寒假试卷[含答案]](https://img.taocdn.com/s3/m/2f4cba492bf90242a8956bec0975f46527d3a7f6.png)
小学上册英语第1单元寒假试卷[含答案]英语试题一、综合题(本题有100小题,每小题1分,共100分.每小题不选、错误,均不给分)1.What is the tallest building in the world?A. Burj KhalifaB. Shanghai TowerC. One World Trade CenterD. Taipei 101答案:A2.The brightest star in the night sky is called ______.3.中国的四大发明包括________ (papermaking) 和火药。
4.What do you call a place where you keep animals?A. ZooB. FarmC. SanctuaryD. All of the above5.The girl loves to ________.6.My favorite subject in school is ________ (地理) because I love learning about different ________ (国家).7. A reaction that occurs when two liquids are mixed is called a ______ reaction.8.What do you call the small, round fruit that is often red or green?A. CherryB. GrapeC. AppleD. Lemon9.I have lots of fun with my ____.10. A __________ is formed by the deposition of organic material over time.11. A rabbit's hearing is much better than ______ (人的).12.The chemical symbol for selenium is ______.13.The __________ (历史的完整性) is vital for accuracy.14.What is the capital of Burundi?A. GitegaB. BujumburaC. NgoziD. Muyinga答案:A15.Stellar evolution describes the life cycle of a _______.16.I like to go ________ (旅行) during the summer.17.________ (植物生长调控) is studied in labs.18.小龙) is often found in fairy tales. The ___19.The __________ can reveal patterns in sedimentation and erosion over time.20.My favorite season is ________ (春天). The flowers bloom, and the weather is ________ (温暖) and sunny.21.What do we call a young chicken?A. DucklingB. CalfC. ChickD. Foal22.I have a ___ (goal) to achieve.23. A ____ lives in a den and is clever.24.The chemical symbol for technetium is ______.25.In the morning, I feed my ______ (小狗) before going to ______ (学校).26.What do we call a collection of stories that are not real?A. FictionB. Non-fictionC. BiographyD. Autobiography27.My favorite food is _____ (pizza/salad).28.I like to _____ stories before bed. (tell)29.What is the capital of the Cook Islands?A. AvaruaB. RarotongaC. PalmerstonD. Mangaia答案:A Avarua30.My grandpa tells __________ (有趣的) jokes.31.My friend is a ______. He enjoys photography.32. (Declaration) of Independence was signed in 1776. The ____33.My brother likes to learn about ____ (space).34.The _____ (小猫) loves to curl up in a cozy spot.35.What is 14 5?A. 8B. 9C. 10D. 11答案:B36.The chemical symbol for mercury is ______.37.The _____ (植物相互作用) supports ecosystem balance.38.I feel proud of my ________ (玩具名) collection because it shows my interests.39.The ______ (肥料) supports plant growth.40.What do we call the planet we live on?A. MarsB. EarthC. VenusD. Jupiter答案:B41. A ____ is known for its beautiful patterns and can be found in gardens.42. A ______ (海星) can regenerate lost arms.43.What is the name of the famous tower in Pisa, Italy?A. Leaning Tower of PisaB. Eiffel TowerC. Tower of LondonD. Big Ben答案:A Leaning Tower of Pisa44.What shape has three sides?A. SquareB. TriangleC. CircleD. Rectangle答案:B45.The owl hunts silently through the ______ (森林).46.The _____ (植物) needs water to survive.47. A ______ (植物标本) can teach us about biodiversity.48.The ______ reads the news every morning.49.The _______ (The Civil Rights Act) aimed to end segregation in public places.50.The _____ (果树) blossoms in the spring.51.The chemical symbol for neodymium is ______.52. A desert is a place that receives very little _______.53.I like to ___ (explore) new hobbies.54.My favorite animal is a ________ because it swims.55.The boy has a new ________.56.The process of ______ can reshape the Earth's surface.57.We can _______ a picnic by the lake.58.The sloth is known for moving _________ (缓慢).munity innovation lab) develops creative solutions. The ____60.What do you call the amount of matter in an object?A. VolumeB. DensityC. MassD. Weight61.What do you call the sound made by a cat?A. BarkB. MeowC. RoarD. Quack答案:B Meow62.She likes to eat _____ (apples/television).63.What do you use to see far away?A. BinocularsB. Magnifying glassC. MicroscopeD. Telescope答案:D64.The main gas released by burning fossil fuels is ______ dioxide.65.I can _____ (dance/sing) very well.66.She is _____ (reading) a magazine.67. A __________ is a type of chemical reaction that produces energy in the form of light.68.The __________ is a large island in the Caribbean. (古巴)69.An ant is very _______ (勤劳).70.The sandwich is very ___ (tasty/dull).71.He is _____ (tall/short) than his brother.72.What do you call a large, slow-moving animal with a shell?A. TortoiseB. TurtleC. SnailD. Armadillo答案:A73.What is the name of the famous detective created by Arthur Conan Doyle?A. Hercule PoirotB. Sherlock HolmesC. Miss MarpleD. Sam Spade74.What do we call a large area of flat land?A. PlateauB. PlainC. ValleyD. Hill75.The __________ is located at the southernmost point of South America.76.The first human in space was _______. (尤里·加加林)77.What do we call the place where we buy food?A. SchoolB. LibraryC. Grocery storeD. Park答案:C78.I like to ______ on sunny days. (play outside)79.How many zeros are in one thousand?A. TwoB. ThreeC. FourD. Five答案:B80.I think it’s important to be curious. Asking questions helps us learn and grow. I love discovering new facts about __________ and sharing them with my friends.81.What do you call a book that tells you how to cook?A. Recipe BookB. NovelC. DiaryD. Encyclopedia82.The main gas produced during respiration is _______.83. A circuit can be powered by batteries or a ______ source.84.The discovery of gold led to the ______ (淘金热) in California.85.The _____ (breeze) feels nice.86.The process of electroplating deposits a layer of ______.87.The butterfly flutters from _____ to flower.88.My favorite season is ______ (春天) because the flowers ______ (开花) and the weather is ______ (温暖). I like to go outside and ______ (玩耍) with my friends.89. A ______ (刺猬) is small and covered in spikes.90.The dog is ________ in the park.91.The horse rides _________ in the field. (快)92. A _______ is a tool used to measure the weight of an object.93.What do we call a story that is passed down orally from generation to generation?A. MythB. LegendC. FolktaleD. Fable答案:C94.Which planet is known for its rings?A. EarthB. MarsC. SaturnD. Jupiter95.What is the common name for a wild horse?A. PonyB. MustangC. ZebraD. Donkey答案:B96.What do you call the person who repairs cars?A. DoctorB. MechanicC. TeacherD. Chef答案:B97.The starfish can regenerate its ________________ (臂).98.What is the main ingredient in pizza?A. RiceB. BreadC. DoughD. Pasta答案:C99.The __________ is a region known for its literary achievements. 100. A sound that is high-pitched has a high ______ (frequency).。
小学上册第六次英语第一单元期中试卷

小学上册英语第一单元期中试卷英语试题一、综合题(本题有100小题,每小题1分,共100分.每小题不选、错误,均不给分)1.What instrument do you blow to make music?A. PianoB. FluteC. DrumD. Violin2.What do you call a person who teaches students?A. DoctorB. TeacherC. FarmerD. Driver3.My ________ (玩具名称) is perfect for imaginative play.4.What is the opposite of "happy"?A. SadB. ExcitedC. JoyfulD. AngryA5.I love to read ______ books at night.6.I think it’s important to be grateful. Recognizing the good things in life bringshap piness. I often write down things I’m thankful for in my journal.7.Which animal is known for its long neck?A. ElephantB. GiraffeC. LionD. Zebra8.My mom is a dedicated __________ (教育者).9.What is the most widely spoken language in the world?A. EnglishB. MandarinC. SpanishD. HindiB10.The __________ can reveal patterns in sedimentation and erosion.11.The ____ is a small animal that collects food for the winter.12.She is ___ her homework. (doing)13.The Voyager spacecraft have traveled beyond our ______.14.My sister enjoys __________. (打球)15. A wolf's howl can be heard over long ________________ (距离).16.The clock is ________ ticking.17.What is the name of the famous wizard in J.K. Rowling's series?A. GandalfB. DumbledoreC. MerlinD. Harry PotterD18. A squirrel's front paws are used for ______ (抓取).19. A __________ is a reaction that absorbs heat.20.What is the name of the famous mountain in China?A. Mount EverestB. Mount HuangC. Mount FujiD. Mount Kilimanjaro21.The dog is ________ outside.22.The Earth's atmosphere protects us from _____ radiation.23.The __________ (历史的助力) drives progress.24. A liquid that can dissolve other substances is called a ______.25.I want to _____ (learn) about space.26.I like to ___ new things. (learn)27.The __________ (历史的力量) drives change.28.What is the term for the change of state from liquid to gas?A. FreezingB. MeltingC. CondensationD. EvaporationD29.The ________ (气候变化影响) our way of living.30.The stars are _____ in the night sky. (shining)31.We _______ (喜欢) to watch movies together.32.What do you call a baby elephant?A. CalfB. FoalC. CubD. KidA33. A _______ (海马) is often seen near coral reefs.34. A cheetah is the fastest _______ on land, running swiftly to catch its prey.35.What is the opposite of 'day'?A. MorningB. EveningC. NightD. Afternoon36.The ________ (记录) of our travels is fun to share.37.What is the capital of the Dominican Republic?A. Santo DomingoB. SantiagoC. Puerto PlataD. La RomanaA38.The first modern Olympic Games were held in ________ (1896).39.What is the term for a baby kangaroo?A. JoeyB. CalfC. KitD. CubA40.The sun sets in the ________.41.I enjoy planting _____ in my backyard.42.The _______ (Civil Rights Act of 1964) outlawed discrimination based on race.43.Chemical reactions can be classified into different ________.44.I call my friend “.”45.The __________ is a large area of land used for agriculture. (农田)46.The owl is a ______ (夜间) bird. It sleeps during the ______ (白天).47.The train is ______ (fast) and exciting.48.The country famous for its ancient civilization is ________ (埃及).49.The __________ is a famous city known for its culture.50.What is the capital of the Cayman Islands?A. George TownB. West BayC. Bodden TownD. North SideA51.What is the primary color of a fire truck?A. BlueB. YellowC. RedD. GreenC52.My brother loves playing __________ (电子游戏).53.I enjoy _____ (painting/drawing).54.I can ________ (navigate) using a map.55.What is the name of the famous detective created by Arthur Conan Doyle?A. Hercule PoirotB. Sherlock HolmesC. Miss MarpleD. Sam Spade56.What is the fastest land animal?A. CheetahB. LionC. HorseD. ElephantA57.She enjoys ________.58.The __________ (历史的身份认同) shapes group dynamics.59.What is the main ingredient in pizza?A. BreadB. DoughC. CheeseD. SauceC60.Which of these is a renewable resource?A. OilB. WaterC. CoalD. Gas61.The librarian helps us find ______. (books)62.The kitten likes to _______ (睡觉) on my lap.63.What do we call a vehicle with two wheels?A. CarB. BusC. BicycleD. Truck64.The weather is _______ for a picnic.65.I like to _____ (play) chess.66.I love to ______ (与朋友一起) explore.67.The __________ is a famous natural landmark in Arizona. (大峡谷)68.The dog is ___ (friendly/scary).69.The ____ is an animal that loves to dig.70.The main product of cellular respiration is ______.71.My ________ (玩具名称) is a great way to make new friends.72.The weather is so __________ that I can’t decide what to wear. (变化无常的)73.Substances that speed up chemical reactions without being consumed are called________.74.The park is ________ my house.75.Owls hunt for _______ at night.76.I want to be a __________ (作家) when I grow up.77.The Earth's surface is covered by a variety of ______, including forests and grasslands.78.What do you call a written message sent electronically?A. LetterB. EmailC. TextD. Note79.The chemical formula for copper(II) sulfate is _______.80.The ______ (生物多样性) of plants is essential for ecosystems.81.The _______ (鲸鱼) can dive deep into the ocean.82.The process of sublimation is when a solid changes directly to a _____.83.What do you call a person who studies the natural world?A. BiologistB. ChemistC. GeologistD. All of the aboveD84.What do we call a scientist who studies the weather?A. MeteorologistB. ClimatologistC. Environmental ScientistD. Geochemist85.The ancient Egyptians built ________ to honor their pharaohs.86.What is the name of the first human to walk on the moon?A. Neil ArmstrongB. Buzz AldrinC. Michael CollinsD. Yuri Gagarin87.What do we call the tool used to cut hair?A. ScissorsB. RazorC. ClipperD. Knife88.The _____ (crocodile) is in the river.89.Certain plants attract specific ______ (昆虫).90.What is the process of changing from a liquid to a gas called?A. FreezingB. MeltingC. EvaporationD. CondensationC91.What is the name of the famous scientist known for his laws of motion?A. GalileoB. Isaac NewtonC. Albert EinsteinD. Nikola TeslaB92.We have a ______ (丰富的) resource center.93.Napoleon Bonaparte became the emperor of France in ______ (1804年).94.Dogs are known for their _______ (忠诚).95.The ________ (socks) are in the drawer.96.I have a ______ of stickers in my collection. (book)97.My brother loves _______ (玩电子游戏).98.My cousin is very __________ (适应性强).99.The computer is very ___. (useful)100. A ______ (鸟) can sing sweet melodies in the morning.。
小学上册T卷英语第五单元真题试卷(有答案)

小学上册英语第五单元真题试卷(有答案)英语试题一、综合题(本题有100小题,每小题1分,共100分.每小题不选、错误,均不给分)1.Light travels faster than ______ (sound).2.The ________ (植物调查) gathers valuable data.3.The __________ (果实) of the tree is ripe for picking.4.The ________ was a famous philosopher who influenced Western thought.5.My sister is a _____ (作家) who explores diverse themes.6.My grandpa enjoys fishing with ____.7.What do we call a scientist who studies weather patterns?A. MeteorologistB. GeologistC. ClimatologistD. Ecologist答案: A8.The __________ is a famous area known for its wildlife reserves.9.In a single displacement reaction, one element replaces another in a _____.10.I want to be a __________ (医生) to help sick people.11.What is the name of the famous American monument on Mount Rushmore?A. Abraham LincolnB. George WashingtonC. Thomas JeffersonD. Teddy Roosevelt答案:B12.What do we call the part of the Earth where we live?A. AtmosphereB. LithosphereC. BiosphereD. Hydrosphere答案: C13.What is the capital city of Egypt?A. CairoB. AlexandriaC. GizaD. Luxor答案: A14.The Earth's surface is shaped by both ______ and human activity.15.The Milky Way is part of a larger ______.16. A _______ can be used to demonstrate the effects of gravity.17.I saw a ______ (rabbit) in the garden.18.I have a toy _____ that can roll.19.She is a _____ (历史学家) documenting significant events.20.I often ________ (动词) my toys after playing. It helps keep my room ________ (形容词). My favorite place to play is in my ________ (名词).21.My sister is a ______. She loves to help in the community.22.The __________ (历史的连接) link us to our roots.23.The __________ (文化对话) fosters peace.24.The ______ can be found in almost every habitat.25.I love to ______ (与朋友交往) at school.26.My _____ (阳台) has many flowers.27.The process of ______ can lead to changes in landforms.28.Gardening can provide a sense of ______ and satisfaction. (园艺可以带来成就感和满足感。
数字阅读英文作文

数字阅读英文作文1. I love numbers. They have this fascinating ability to tell stories without uttering a single word. Just by looking at a series of digits, you can uncover the secrets of the universe, decipher the mysteries of nature, and understand the complexities of human behavior. Numbers are like silent observers, silently recording the patterns and rhythms of life.2. Numbers can be deceiving. They have the power to create illusions and distort reality. Take statistics, for example. They can be manipulated to support any argument or agenda. A single statistic can be used to prove a point, but it can also be misleading if not put into context. So, it's important to approach numbers with a critical eye and question their validity.3. Numbers can be both comforting and terrifying. On one hand, they provide a sense of order and structure in a chaotic world. They give us a way to measure and quantifythings, making them more tangible and manageable. On the other hand, numbers can also be overwhelming. The sheer magnitude of certain numbers, like the national debt or the population of a city, can be mind-boggling and make us feel insignificant in the grand scheme of things.4. Numbers can be a source of competition and comparison. From grades in school to salaries in the workplace, numbers are often used to rank and evaluate individuals. This can create a sense of pressure and insecurity, as we constantly strive to measure up tocertain standards. However, it's important to remember that numbers don't define our worth or determine our success. They are just one way of assessing our progress.5. Numbers can be a language of their own. They have their own symbols, rules, and patterns. Just like learning a foreign language, understanding numbers requires practice and fluency. Once you become fluent in the language of numbers, you can unlock a whole new world of possibilities. You can analyze data, make predictions, and solve complex problems. It's like having a superpower that allows you tosee beyond the surface and delve into the depths of knowledge.6. Numbers can also be a source of inspiration and awe. Think about the beauty of mathematics, the elegance of equations, and the harmony of patterns. Numbers haveinspired artists, musicians, and poets throughout history. They have a certain magic that captivates our imagination and sparks our creativity. Whether it's the Fibonacci sequence in nature or the golden ratio in art, numbers have a way of revealing the hidden beauty in the world around us.7. In the end, numbers are just tools. They can be used for good or for harm, depending on how we wield them. It's up to us to use numbers responsibly, to question their meaning, and to seek a deeper understanding beyond the surface. Numbers may be silent, but they speak volumes ifwe're willing to listen.。
prevision tasks for specific patterns翻译版

I. Discuss the translation technique and the ways of applying the technique to the translation of the following sentences. Complete each of the Chinese translations.1. We have come to the last and most important step of the experiment.我们的实验现在已经到了最后(一步,也就是)最重要的阶段。
2. This is extruded through minute holes in a nozzle, and the threads of filaments produced and solidified in various ways. 现将该物质从喷嘴的小孔中挤压出来,(并)将产生的纤维丝利用各种方法固化。
3. Thousands of the electric power generators, often installed on windfarms in North America and Europe, now total over 800 megawatts of rated capacity, and their numbers are continuing to grow. 数以千计的风力发电机大多安装在北美和欧洲的风力发电场,其总额定功率现已达800兆瓦,(其)数量仍在继续增加。
4. Solid silicones serve, among other things, as a kind of artificial rubber, and liquid silicones have been used as hydraulic fluids. 固体硅酮的用途很广,其中之一是用作人工橡胶,(而)液体硅酮则被用作各种液压流体。
- 1、下载文档前请自行甄别文档内容的完整性,平台不提供额外的编辑、内容补充、找答案等附加服务。
- 2、"仅部分预览"的文档,不可在线预览部分如存在完整性等问题,可反馈申请退款(可完整预览的文档不适用该条件!)。
- 3、如文档侵犯您的权益,请联系客服反馈,我们会尽快为您处理(人工客服工作时间:9:00-18:30)。
Learning Surface Text Patterns for a Question Answering System Deepak Ravichandran and Eduard HovyInformation Sciences InstituteUniversity of Southern California4676 Admiralty WayMarina del Rey, CA 90292-6695USA{ravichan,hovy}@AbstractIn this paper we explore the power of surface text patterns for open-domain question answering systems. In order to obtain an optimal set of patterns, we have developed a method for learning such patterns automatically. A tagged corpus is built from the Internet in a bootstrapping process by providing a few hand-crafted examples of each question type to Altavista. Patterns are then automatically extracted from the returned documents and standardized. We calculate the precision of each pattern, and the average precision for each question type. These patterns are then applied to find answers to new questions. Using the TREC-10 question set, we report results for two cases: answers determined from the TREC-10 corpus and from the web.1 IntroductionMost of the recent open domain question-answering systems use external knowledge and tools for answer pinpointing. These may include named entity taggers, WordNet, parsers, hand-tagged corpora, and ontology lists (Srihari and Li, 00; Harabagiu et al., 01; Hovy et al., 01; Prager et al., 01). However, at the recent TREC-10 QA evaluation (Voorhees, 01), the winning system used just one resource: a fairly extensive list of surface patterns (Soubbotin and Soubbotin, 01). The apparent power of such patterns surprised many. We therefore decided to investigate their potential by acquiring patterns automatically and to measure their accuracy.It has been noted in several QA systems that certain types of answer are expressed using characteristic phrases (Lee et al., 01; Wang et al., 01). For example, for BIRTHDATEs (with questions like “When was X born?”), typical answers are“Mozart was born in 1756.”“Gandhi (1869–1948)…”These examples suggest that phrases like “<NAME> was born in <BIRTHDATE>”“<NAME> (<BIRTHDATE>–”when formulated as regular expressions, can be used to locate the correct answer.In this paper we present an approach for automatically learning such regular expressions (along with determining their precision) from the web, for given types of questions. Our method uses the machine learning technique of bootstrapping to build a large tagged corpus starting with only a few examples of QA pairs. Similar techniques have been investigated extensively in the field of information extraction (Riloff, 96). These techniques are greatly aided by the fact that there is no need to hand-tag a corpus, while the abundance of data on the web makes it easier to determine reliable statistical estimates.Our system assumes each sentence to be a simple sequence of words and searches for repeated word orderings as evidence forComputational Linguistics (ACL), Philadelphia, July 2002, pp. 41-47. Proceedings of the 40th Annual Meeting of the Association foruseful answer phrases. We use suffix trees for extracting substrings of optimal length. We borrow the idea of suffix trees from computational biology (Gusfield, 97) where it is primarily used for detecting DNA sequences. Suffix trees can be processed in time linear on the size of the corpus and, more importantly, they do not restrict the length of substrings. We then test the patterns learned by our system on new unseen questions from the TREC-10 set and evaluate their results to determine the precision of the patterns.2 Learning of PatternsWe describe the pattern-learning algorithm with an example. A table of patterns is constructed for each individual question type by the following procedure (Algorithm 1).1. Select an example for a given questiontype. Thus for BIRTHYEAR questions we select “Mozart 1756” (we refer to “Mozart” as the question term and “1756”as the answer term).2. Submit the question and the answer termas queries to a search engine. Thus, we give the query +“Mozart” +“1756” to AltaVista ().3. Download the top 1000 web documentsprovided by the search engine.4. Apply a sentence breaker to thedocuments.5. Retain only those sentences that containboth the question and the answer term.Tokenize the input text, smooth variations in white space characters, and remove html and other extraneous tags, to allow simple regular expression matching tools such as egrep to be used.6. Pass each retained sentence through asuffix tree constructor. This finds all substrings, of all lengths, along with their counts. For example consider the sentences “The great composer Mozart (1756–1791) achieved fame at a young age” “Mozart (1756–1791) was a genius”, and “The whole world would always be indebted to the great music of Mozart (1756–1791)”. The longest matching substring for all 3 sentences is “Mozart(1756–1791)”, which the suffix tree would extract as one of the outputs along with the score of 3.7. Pass each phrase in the suffix tree througha filter to retain only those phrases thatcontain both the question and the answer term. For the example, we extract only those phrases from the suffix tree that contain the words “Mozart” and “1756”. 8. Replace the word for the question term bythe tag “<NAME>” and the word for the answer term by the term “<ANSWER>”.This procedure is repeated for different examples of the same question type. For BIRTHDATE we also use “Gandhi 1869”, “Newton 1642”, etc.For BIRTHDATE, the above steps produce the following output:a. born in <ANSWER> , <NAME>b. <NAME> was born on <ANSWER> ,c. <NAME> ( <ANSWER> -d. <NAME> ( <ANSWER - )...These are some of the most common substrings of the extracted sentences that contain both <NAME> and <ANSWER>. Since the suffix tree records all substrings, partly overlapping strings such as c and d are separately saved, which allows us to obtain separate counts of their occurrence frequencies. As will be seen later, this allows us to differentiate patterns such as d (which records a still living person, and is quite precise) from its more general substring c (which is less precise).Algorithm 2: Calculating the precision of each pattern.1. Query the search engine by using only thequestion term (in the example, only “Mozart”).2. Download the top 1000 web documentsprovided by the search engine.3. As before, segment these documents intoindividual sentences.4. Retain only those sentences that containthe question term.5. For each pattern obtained from Algorithm1, check the presence of each pattern in thesentence obtained from above for two instances:i) Presence of the pattern with<ANSWER> tag matched by anyword.ii) Presence of the pattern in the sentence with <ANSWER> tag matched by thecorrect answer term.In our example, for the pattern “<NAME> was born in <ANSWER>” we check the presence of the following strings in the answer sentencei) Mozart was born in <ANY_WORD>ii) Mozart was born in 1756Calculate the precision of each pattern by the formula P = C a / C o whereC a = total number of patterns with theanswer term presentC o = total number of patterns presentwith answer term replaced by any word 6. Retain only the patterns matching asufficient number of examples (we choose the number of examples > 5).We obtain a table of regular expression patterns for a given question type, along with the precision of each pattern. This precision is the probability of each pattern containing the answer and follows directly from the principle of maximum likelihood estimation.For BIRTHDATE the following table is obtained:1.0 <NAME>( <ANSWER> - )0.85 <NAME> was born on <ANSWER>, 0.6 <NAME> was born in <ANSWER> 0.59 <NAME> was born <ANSWER>0.53 <ANSWER> <NAME> was born0.50 – <NAME> ( <ANSWER>0.36 <NAME> ( <ANSWER> -For a given question type a good range of patterns was obtained by giving the system as few as 10 examples. The rather long list of patterns obtained would have been very difficult for any human to come up with manually.The question term could appear in the documents obtained from the web in various ways. Thus “Mozart” could be written as “Wolfgang Amadeus Mozart”, “Mozart, Wolfgang Amadeus”, “Amadeus Mozart” or “Mozart”. To learn from such variations, in step 1 of Algorithm 1we specify the various ways in which the question term could be specified in the text. The presence of any of these names would cause it to be tagged as the original question term “Mozart”.The same arrangement is also done for the answer term so that presence of any variant of the answer term would cause it to be treated exactly like the original answer term. While easy to do for BIRTHDATE, this step can be problematic for question types such as DEFINITION, which may contain various acceptable answers. In general the input example terms have to be carefully selected so that the questions they represent do not have a long list of possible answers, as this would affect the confidence of the precision scores for each pattern. All the answers need to be enlisted to ensure a high confidence in the precision score of each pattern, in the present framework.The precision of the patterns obtained from one QA-pair example in algorithm 1 is calculated from the documents obtained in algorithm 2 for other examples of the same question type. In other words, the precision scores are calculated by cross-checking the patterns across various examples of the same type. This step proves to be very significant as it helps to eliminate dubious patterns, which may appear because the contents of two or more websites may be the same, or the same web document reappears in the search engine output for algorithms 1 and 2.Algorithm 1 does not explicitly specify any particular question type. Judicious choice of the QA example pair therefore allows it to be used for many question types without change.3 Finding AnswersUsing the patterns to answer a new question we employ the following algorithm:1. Determine the question type of the newquestion. We use our existing QA system (Hovy et al., 2002b; 2001) to do so.2. The question term in the question isidentified, also using our existing system.3. Create a query from the question term andperform IR (by using a given answer document corpus such as the TREC-10 collection or web search otherwise).4. Segment the documents obtained intosentences and smooth out white space variations and html and other tags, as before.5. Replace the question term in each sentenceby the question tag (“<NAME>”, in the case of BIRTHYEAR).6. Using the pattern table developed for thatparticular question type, search for the presence of each pattern. Select words matching the tag “<ANSWER>” as the answer.7. Sort these answers by their pattern’sprecision scores. Discard duplicates (by elementary string comparisons). Return the top 5 answers.4 ExperimentsFrom our Webclopedia QA Typology (Hovy et al., 2002a) we selected 6 different question types: BIRTHDATE, LOCATION, INVENTOR, DISCOVERER, DEFINITION, WHY-FAMOUS. The pattern table for each of these question types was constructed using Algorithm 1.Some of the patterns obtained along with their precision are as followsBIRTHYEAR1.0 <NAME> ( <ANSWER> - )0.85 <NAME> was born on <ANSWER> ,0.6 <NAME> was born in <ANSWER>0.59 <NAME> was born <ANSWER>0.53 <ANSWER> <NAME> was born0.5 - <NAME> ( <ANSWER>0.36 <NAME> ( <ANSWER> -0.32 <NAME> ( <ANSWER> ) ,0.28 born in <ANSWER> , <NAME>0.2 of <NAME> ( <ANSWER> INVENTOR1.0 <ANSWER> invents <NAME>1.0 the <NAME> was invented by<ANSWER>1.0 <ANSWER> invented the <NAME> in1.0 <ANSWER> ' s invention of the<NAME>1.0 <ANSWER> invents the <NAME> .1.0 <ANSWER> ' s <NAME> was1.0 <NAME> , invented by <ANSWER> 1.0 <ANSWER> ' s <NAME> and1.0 that <ANSWER> ' s <NAME>1.0 <NAME> was invented by <ANSWER> , DISCOVERER1.0 when <ANSWER> discovered<NAME>1.0 <ANSWER> ' s discovery of <NAME> 1.0 <ANSWER> , the discoverer of<NAME>1.0 <ANSWER> discovers <NAME> .1.0 <ANSWER> discover <NAME>1.0 <ANSWER> discovered <NAME> , the 1.0 discovery of <NAME> by <ANSWER>.0.95 <NAME> was discovered by<ANSWER>0.91 of <ANSWER> ' s <NAME>0.9 <NAME> was discovered by<ANSWER> inDEFINITION1.0 <NAME> and related <ANSWER>s1.0 <ANSWER> ( <NAME> ,1.0 <ANSWER> , <NAME> .1.0 , a <NAME> <ANSWER> ,1.0 ( <NAME> <ANSWER> ) ,1.0 form of <ANSWER> , <NAME>1.0 for <NAME> , <ANSWER> and1.0 cell <ANSWER> , <NAME>1.0 and <ANSWER> > <ANSWER> ><NAME>0.94 as <NAME> , <ANSWER> andWHY-FAMOUS1.0 <ANSWER> <NAME> called1.0 laureate <ANSWER> <NAME>1.0 by the <ANSWER> , <NAME> ,1.0 <NAME> - the <ANSWER> of1.0 <NAME> was the <ANSWER> of0.84 by the <ANSWER> <NAME> ,0.8 the famous <ANSWER> <NAME> ,0.73 the famous <ANSWER> <NAME>0.72 <ANSWER> > <NAME>0.71 <NAME> is the <ANSWER> of LOCATION1.0 <ANSWER> ' s <NAME> .1.0 regional : <ANSWER> : <NAME>1.0 to <ANSWER> ' s <NAME> ,1.0 <ANSWER> ' s <NAME> in1.0 in <ANSWER> ' s <NAME> ,1.0 of <ANSWER> ' s <NAME> ,1.0 at the <NAME> in <ANSWER>0.96 the <NAME> in <ANSWER> ,0.92 from <ANSWER> ' s <NAME>0.92 near <NAME> in <ANSWER>For each question type, we extracted the corresponding questions from the TREC-10 set. These questions were run through the testing phase of the algorithm. Two sets of experiments were performed. In the first case, the TREC corpus was used as the input source and IR was performed by the IR component of our QA system (Lin, 2002). In the second case, the web was the input source and the IR was performed by the AltaVista search engine.Results of the experiments, measured by Mean Reciprocal Rank (MRR) score (Voorhees, 01), are:TREC CorpusQuestion type Number ofquestionsMRR on TREC docsINVENTOR 6 0.17 DISCOVERER 4 0.13 DEFINITION 102 0.34 WHY-FAMOUS 3 0.33 LOCATION 16 0.75 WebQuestion type Number ofquestions MRR on theWebBIRTHYEAR 8 0.69 INVENTOR 6 0.58 DISCOVERER 4 0.88 DEFINITION 102 0.39 WHY-FAMOUS 3 0.00 LOCATION 16 0.86The results indicate that the system performs better on the Web data than on the TREC corpus. The abundance of data on the web makes it easier for the system to locate answers with high precision scores (the system finds many examples of correct answers among the top 20 when using the Web as the input source). A similar result for QA was obtained by Brill et al. (2001). The TREC corpus does not have enough candidate answers with high precision score and has to settle for answers extracted from sentences matched by low precision patterns. The WHY-FAMOUS question type is an exception and may be due to the fact that the system was tested on a small number of questions.5 Shortcoming and ExtensionsNo external knowledge has been added to these patterns. We frequently observe the need for matching part of speech and/or semantic types, however. For example, the question: “Where are the Rocky Mountains located?” is answered by “Denver’s new airport, topped with white fiberglass cones in imitation of the Rocky Mountains in the background, continues to lie empty”, because the system picked the answer “the background” using the pattern “the <NAME> in <ANSWER>,”. Using a named entity tagger and/or an ontology would enable the system to use the knowledge that “background” is not a location.DEFINITION questions pose a related problem. Frequently the system’s patterns match a term that is too general, though correct technically. For “what is nepotism?” the pattern “<ANSWER>, <NAME>” matches “…in the form of widespread bureaucratic abuses: graft, nepotism…”; for “what is sonar?” the pattern “<NAME> and related <ANSWER>s” matches “…while its sonar and related underseas systems are built…”.The patterns cannot handle long-distance dependencies. For example, for “Where is London?” the system cannot locate the answer in “London, which has one of the most busiest airports in the world, lies on the banks of the river Thames” due to the explosive danger of unrestricted wildcard matching, as would be required in the pattern “<QUESTION>, (<any_word>)*, lies on <ANSWER>”. This is one of the reasons why the system performsvery well on certain types of questions from the web but performs poorly with documents obtained from the TREC corpus. The abundance and variation of data on the Internet allows the system to find an instance of its patterns without losing answers to long-term dependencies. The TREC corpus, on the other hand, typically contains fewer candidate answers for a given question and many of the answers present may match only long-term dependency patterns.More information needs to be added to the text patterns regarding the length of the answer phrase to be expected. The system searches in the range of 50 bytes of the answer phrase to capture the pattern. It fails to perform under certain conditions as exemplified by the question “When was Lyndon B. Johnson born?”. The system selects the sentence “Tower gained national attention in 1960 when he lost to democratic Sen. Lyndon B. Johnson, who ran for both re-election and the vice presidency” using the pattern “<NAME> <ANSWER> –“. The system lacks the information that the <ANSWER> tag should be replaced exactly by one word. Simple extensions could be made to the system so that instead of searching in the range of 50 bytes for the answer phrase it could search for the answer in the range of 1–2 chunks (basic phrases in English such as simple NP, VP, PP, etc.).A more serious limitation is that the present framework can handle only one anchor point (the question term) in the candidate answer sentence. It cannot work for types of question that require multiple words from the question to be in the answer sentence, possibly apart from each other. For example, in “Which county does the city of Long Beach lie?”, the answer “Long Beach is situated in Los Angeles County” requires the pattern. “<QUESTION_TERM_1> situated in <ANSWER> <QUESTION_TERM_2>”, where <QUESTION_TERM_1> and <QUESTION_TERM_2> represent the terms “Long Beach” and “county” respectively. The performance of the system depends significantly on there being only one anchor word, which allows a single word match between the question and the candidate answer sentence. The presence of multiple anchor words would help to eliminate many of the candidate answers by simply using the condition that all the anchor words from the question must be present in the candidate answer sentence.The system does not classify or make any distinction between upper and lower case letters. For example, “What is micron?” is answered by “In Boise, Idaho, a spokesman for Micron, a maker of semiconductors, said Simms are ‘ a very high volume product for us …’ ”. The answer returned by the system would have been perfect if the word “micron” had been capitalized in the question.Canonicalization of words is also an issue. While giving examples in the bootstrapping procedure, say, for BIRTHDATE questions, the answer term could be written in many ways (for example, Gandhi’s birth date can be written as “1869”, “Oct. 2, 1869”, “2nd October 1869”, “October 2 1869”, and so on). Instead of enlisting all the possibilities a date tagger could be used to cluster all the variations and tag them with the same term. The same idea could also be extended for smoothing out the variations in the question term for names of persons (Gandhi could be written as “Mahatma Gandhi”, “Mohandas Karamchand Gandhi”, etc.).6 ConclusionThe web results easily outperform the TREC results. This suggests that there is a need to integrate the outputs of the Web and the TREC corpus. Since the output from the Web contains many correct answers among the top ones, a simple word count could help in eliminating many unlikely answers. This would work well for question types like BIRTHDATE or LOCATION but is not clear for question types like DEFINITION.The simplicity of this method makes it perfect for multilingual QA. Many tools required by sophisticated QA systems (named entity taggers, parsers, ontologies, etc.) are language specific and require significant effort to adapt to a new language. Since the answer patterns used in this method arelearned using only a small number of manual training terms, one can rapidly learn patterns for new languages, assuming the web search engine is appropriately switched.AcknowledgementsThis work was supported by the Advanced Research and Development Activity (ARDA)'s Advanced Question Answering for Intelligence (AQUAINT) Program under contract number MDA908-02-C-0007. ReferencesBrill, E., J. Lin, M. Banko, S. Dumais, and A. Ng.2001. Data-Intensive Question Answering.Proceedings of the TREC-10 Conference.NIST, Gaithersburg, MD, 183–189. Gusfield, D. 1997. Algorithms on Strings, Trees and Sequences: Computer Science and Computational Biology. Chapter 6: Linear Time construction of Suffix trees, 94–121. Harabagiu, S., D. Moldovan, M. Pasca, R.Mihalcea, M. Surdeanu, R. Buneascu, R. Gîrju, V. Rus and P. Morarescu. 2001. FALCON: Boosting Knowledge for Answer Engines.Proceedings of the 9th Text Retrieval Conference (TREC-9), NIST, 479–488. Hovy, E.H., U. Hermjakob, and C.-Y. Lin. 2001.The Use of External Knowledge in Factoid QA. Proceedings of the TREC-10 Conference. NIST, Gaithersburg, MD, 166–174.Hovy, E.H., U. Hermjakob, and D. Ravichandran.2002a. A Question/Answer Typology with Surface Text Patterns. Proceedings of the Human Language Technology (HLT) conference. San Diego, CA.Hovy, E.H., U. Hermjakob, C.-Y. Lin, and D.Ravichandran. 2002b. Using Knowledge to Facilitate Pinpointing of Factoid Answers.Proceedings of the COLING-2002 conference.Taipei, Taiwan.Lee, G.G., J. Seo, S. Lee, H. Jung, B-H. Cho, C.Lee, B-K. Kwak, J, Cha, D. Kim, J-H. An, H.Kim, and K. Kim. 2001. SiteQ: Engineering High Performance QA System Using Lexico-Semantic Pattern Matching and Shallow NLP.Proceedings of the TREC-10 Conference.NIST, Gaithersburg, MD, 437–446. Lin, C-Y. 2002. The Effectiveness of Dictionary and Web-Based Answer Reranking.Proceedings of the COLING-2002 conference.Taipei, Taiwan.Prager, J. and J. Chu-Carroll. 2001. Use of WordNet Hypernyms for Answering What-Is Questions. Proceedings of the TREC-10 Conference. NIST, Gaithersburg, MD, 309–316.Riloff, E. 1996. Automatically Generating Extraction Patterns from Untagged Text.Proceedings of the Thirteenth National Conference on Artificial Intelligence (AAAI-96), 1044–1049.Soubbotin, M.M. and S.M. Soubbotin. 2001.Patterns of Potential Answer Expressions as Clues to the Right Answer. Proceedings of the TREC-10 Conference. NIST, Gaithersburg, MD, 175–182.Srihari, R. and W. Li. 2000. A Question Answering System Supported by Information Extraction. Proceedings of the 1st Meeting of the North American Chapter of the Association for Computational Linguistics (ANLP-NAACL-00), Seattle, WA, 166–172. Voorhees, E. 2001. Overview of the Question Answering Track. Proceedings of the TREC-10 Conference. NIST, Gaithersburg, MD, 157–165.Wang, B., H. Xu, Z. Yang, Y. Liu, X. Cheng, D.Bu, and S. Bai. 2001. TREC-10 Experiments at CAS-ICT: Filtering, Web, and QA.Proceedings of the TREC-10 Conference.NIST, Gaithersburg, MD, 229–241.。