An Architecture for Video Surveillance Service based on P2P and Cloud Computing
海康威视HikCentral解决方案说明书
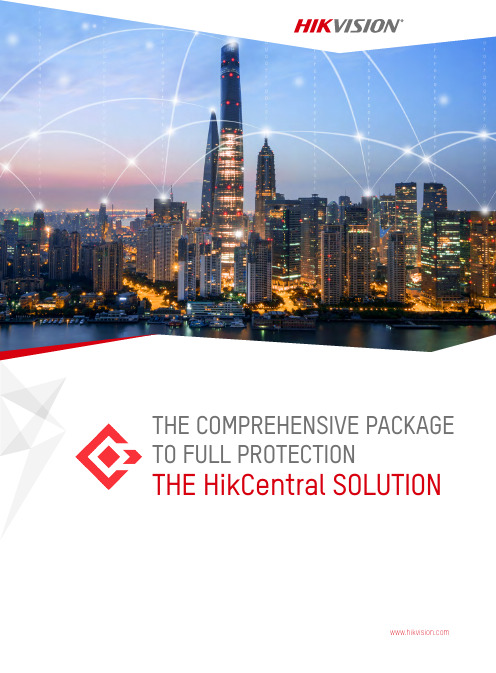
THE HikCentral SOLUTIONTHE COMPREHENSIVE PACKAGE TO FULL PROTECTIONContents17The HikCentralPlatform01CommonChallenges and Hikvision's Solutions The HikCentral SolutionVertical FeaturesHikCentralAdvantages0305 23300203COMMON CHALLENGESAND HIKVISION'S SOLUTIONS04Common ChallengesThe HikCentral Solution Advantages• Lacking resolution and clarity in low light • Video surveillance coverage impeded by blind angles• No intelligent features• Various vendor for different subsystems • High integration costs • High maintenance costs• Low efficiency with manual monitoring• Massive labor required when searching massive data • Complicated operation and troubleshootingA unified, connected, and intelligent platform solution is neededIn one word: unified. The HikCentral Solution from Hikvision keeps video surveillance, security systems, and access control units unified. Nothing ties together an extensive setup of critical security hardware like HikCentral. From front- to back-end equipment, and from perimeter protection to central command, the HikCentral Solution unifies whole systems – even integrating products from other manufacturers. It’s easy to install and configure, and offers impressive scalability. Its extensive backup systems provide solid, continuous operation. Continue reading to see just how powerful and effective this solution is both for security and for business enhancements in a multitude of vertical market applications.THE HikCentral SOLUTION 0506System ArchitectureAccess Control-Fingerprint, Card & Password Authentication -Attendance ReportVehicle Management-License Plate Number Recognition -Vehicle Chassis InspectionMonitoring Center-Unified Management -Smart Wall-Alarm Management -MaintenanceVideo Surveillance-Front-End -Back-End-Central StorageSolution for Common ScenarioDefogWDROptical Image Stabilization DarkfighterX Network IR Speed Dome0708Solution for Large ScenarioEntries & ExitsANPR Camera• 2 MP, CMOS, 1920 x 1080, built-in LPR algorithm • Integrated camera, housing, lens, power adaptor, supplement Lights • Motorized lens• Software one-key focus, easy debugging• Built in whitelist, LPR identification support, barrier / gate control , audio outputUVSS• 2048 *15000 resolution, support multiple pictures tiled display • Supports high speed and non-uniform movement with no motion blur• FOV 180°, designed for distortion optimization• Supports automatic trigger/ Sense coil trigger • Waterproof, anti-fog, and anti-corrosion• High-strength structure supports heavy vehicle passing • Professional illumination designPanoVu Series 360º Panoramic + PTZ CameraIntrusion Detection09Business IntelligenceStereo Vision Repeat Count Filtering Height FilteringFisheye Fisheye + 3 PTZ 2 x Panoramic 4 x PTZFacial RecognitionAccess ControlAnti Pass-backWith Anti Pass-back, an access card can only be used to enter again after it is swiped out.This function prevents multiple people from sharing cards to get into restricted areas.First CardFirst Card can be associated with different rules and be used in different scenarios. Forexample, schools can adopt First Card Open to keep the door open for a designated period during the rush hour after the first authorized swipe; offices can adopt FirstPerson Authorization, which only allows regular cards to enter after the first authorizedcard swipe in.Generate Attendance ReportThe platform can automatically generate attendance report, which is more cost-effectiveand accurate.Access Controller(1,2,4)Professional Card ReaderFingerprint and Card Reader Standalone Access Control TerminalMonitoring CenterStorageCloud Storage Node ClusterSingle ModeJoint ModeDivision ModeRoaming Mode Monitoring CenterSmart Wall Work FlowDisplay• Seamless viewing and large-scale screen• Point-by-point calibration• Nanosecond response time• Ultra-wide viewing angle and anti-dazzle• Video local view• PTZ control cameras• Management of screen displayKeyboardVideo StreamDecoderDisplay HikCentral ClientSwitchNVR / Hybrid SAN / Cloud StorageMonitoring CenterDecode, Display & ControlTHE HikCentral PLATFORMThe HikCentral Platform Video ManagementLive View & Playback• Mainstream and sub-stream automatic or manual switching• Supports camera status check• Supports channel playback on the live view screens duringsimultaneous live viewing on another channel• Rapid video clip acquisition around a specified target time;retrieves subsequent video by simply dragging timeline• Provides live viewing & playback via mobile appSmart Wall• Live viewing and playback mode• Supports manually sending alarm list to the Smart Wall• Alarm-related video can pop up onto Smart Wall automaticallyVideo Search• Multiple efficient ways to search video: by tag, by locked status, by interval, by time, by file, etc.• Thumbnail feature for quicker positioning• After-the-fact” VCA search of playback video available for convenient analysis -Motion Analysis-Line Crossing Detection -Intrusion DetectionEvent & Alarm Management• Motion Detection • Video Loss• Video Tampering Detection Email Alarm Output TagSmart Wall VideoMap Audio• Device Offline • HDD Full• R/W HDD Failure• Unsafe System Temperature • Unsafe CPU Temperature • Memory Issue or Anomaly• License Plate Event Match • License Plate Event Mismatch• TCP and/or UDP data packages• Alarm Input3rdHikCentralEvent & Alarm• Event & alarm configuration, including rules and linkage configuration • Event & alarm management • Alarm notification on mobile appComposite Alarm• Composite Alarm is able to lower the false alarm rate and increase alarmefficiency.You can customize the rules and priority levels for composite alarms according to different needs.Composite alarm is triggered when all the preset conditions are met. For example, a bird that only triggers line crossing will not trigger the composite alarm, thus lower the false alarm rate.Alarm EscalationMaintenance• Centralized display of general system health on dashboard for up-to-the-minute status reportVERTICAL FEATURESRetailPeople Counting• Real-time foot-traffic control• Intelligent analysis and statistics for visitors• Reports crowd traffic statisticsHeat Mapping• Improves decision making of sales based ondynamic analysis through advanced Heat Map• Provides insightful recommendations for locationsof commoditiesPOS IntegrationThe POS NVR provides multiple ways to connect with POS servers, making integration highly flexibleIO Server ( turn 232 signals into network signals )• Effective solution for loss prevention in retail sectors and for customer dispute resolving• Supports search by keywords contained in POS information• Links keywords to relevant POS videos which can beeasily found and exported for investigation purposeBanksATM IntegrationATM DVR• ATM cameras solve transaction disputes and create efficient management for transaction data • ATM smart search that based on keywords helps to locate relevant transaction videos quickly• Transaction video includes detailed information overlaid on the screenEducationFacial Recognition and Blacklist ComparisonPrevent unauthorized person to enter campusAccess ControlPerimeter Protection and Behavior Analysis• Manage student’s access to dangerous areas such as the lab room• Prevent irrelevant personnel to enterrestricted areas such as the finance center• Perimeter protection with advanced behavior analysis to prevent false alarmLab RoomFinance Center30HikCentral ADVANTAGES31Ease Of UseUnified PlatformEasy Set UpEasy ConfigurationInstall the system in minutes! The simple installation package design helps reduce the install time to minutes• One-step addition for DVRs, NVRs, and IP Cameras• One-step configuration automatically detects devices and allows quick Live Viewing and Playback of videoAutomatically creates logical areas by device nameSynchronizes camera namesSynchronize Recording ScheduleLive View & PlaybacKHikCentral is a unified platform that blends video surveillance, access control, ANPR, POS, and 3rd-party system integration into one highly efficient solutionEfficient & Effective Centralized ManagementANPRPeople Counting Heat Map Smart Wall Access Control Intrusion Detection VIP Recognition Blacklist AlarmSupports ONVIF Supports open SDKVideo Surveillance Applications Open PlatformSystem ScalabilityHQ Centralized Management via RSMHikCentral RSM Server HikCentral RSM ClientCorporate Branch 1Corporate Branch N Super-large project solution• Up to 1,024 HikCentral servers supported, covering 1,000 large branches• 1 RSM server for centralized management• Up to 100K+ camera access and managementBranch operator• Manage the branch video resource • 100 local clients supported HQ RSM• Manage the resource of all branches• 100 RSM clients supported3233System ReliabilityWith HikCentral VMS pre-installed and optimized for maximum performance, Hikvision Management Server provides advanced security solutions. These solutions are ideal for customers requiring highly reliable security.• Single-spot storage backup – Multi-RAID supported by embedded NVR and Hybrid SAN• Multi-spot storage backup – When more than 1 of 10 active Hybrid SANs are out of service, the standby Hybrid SAN can take over recording automaticallyIn extreme situations, a single standby Hybrid SAN can cover recording for 10 Hybrid SANs.• Strict system testing for pre-installed HikCentral on Hikvision Management Server • The system supports Hybrid SAN N+1hot-spare for storage reliability• Supports Windows Cluster and Rose Replicator solutions for system reliabilityCamera CameraActive Hybrid SANActive Hybrid SANStandbyHybrid SANN+1 Storage equipment device backupHeadquartersNo.555 Qianmo Road, Binjiang District, Hangzhou 310051, China T +86-571-8807-5998******************************Hikvision USAT +1-909-895-0400***********************Hikvision IndiaT +91-22-28469900************************Hikvision Europe T +31-23-5542770**********************Hikvision Italy T +39-0438-6902*********************Hikvision UAET +971-4-4432090*********************Hikvision Singapore T +65-6684-4718****************Hikvision Poland T +48-22-460-01-50*********************Hikvision Tashkent T +99-87-1238-9438****************Hikvision Indonesia T +62-21-2933759*****************************Hikvision Thailand T +662-275-9949****************************Hikvision UK & Ireland T +01628-902140**********************Hikvision FranceT +33(0)1-85-330-450*********************Hikvision Russia T +7-495-669-67-99********************Hikvision Australia T +61-2-8599-4233*********************Hikvision Brazil T +55 11 3318-0050***************************Hikvision Colombia****************************Hikvision Malaysia T +601-7652-2413**********************Hikvision Vietnam T +84-974270888**********************Hikvision South Africa Tel: +27 (10) 0351172*************************Hikvision SpainT +34-91-737-16-55*********************Hikvision Hong Kong T +852-2151-1761*********************Distributed byHikvision Canada T +1-866-200-6690**************************Hikvision KoreaT +82-(0)31-731-8817*************************Hikvision TurkeyT +90 (216)521 7070- 7074**********************Hikvision Kazakhstan T +7-727-9730667***************************HikvisionHikvisionHQHikvision_GlobalHikvisionCorporate ChannelHikvisionHQhikvisionhq Follow us on social media to get the latest product and solution informationTHE HikCentral SOLUTIONTHE COMPREHENSIVE PACKAGE TO FULL PROTECTION。
基于Windows Mobile的移动视频监控系统
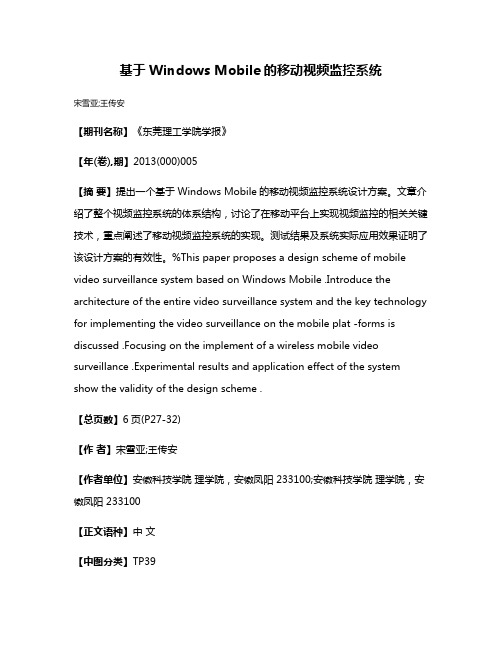
基于Windows Mobile的移动视频监控系统宋雪亚;王传安【期刊名称】《东莞理工学院学报》【年(卷),期】2013(000)005【摘要】提出一个基于Windows Mobile的移动视频监控系统设计方案。
文章介绍了整个视频监控系统的体系结构,讨论了在移动平台上实现视频监控的相关关键技术,重点阐述了移动视频监控系统的实现。
测试结果及系统实际应用效果证明了该设计方案的有效性。
%This paper proposes a design scheme of mobile video surveillance system based on Windows Mobile .Introduce the architecture of the entire video surveillance system and the key technology for implementing the video surveillance on the mobile plat -forms is discussed .Focusing on the implement of a wireless mobile video surveillance .Experimental results and application effect of the system show the validity of the design scheme .【总页数】6页(P27-32)【作者】宋雪亚;王传安【作者单位】安徽科技学院理学院,安徽凤阳 233100;安徽科技学院理学院,安徽凤阳 233100【正文语种】中文【中图分类】TP39【相关文献】1.基于Windows Mobile的移动视频监控系统 [J], 宋雪亚;王传安2.DIY Your Windows Mobile 6 Windows Mobile 6注册表修改大全 [J], 文田3.Windows Mobile文件监控系统的设计与实现 [J], 谢泽科;胡劲松4.嵌入式 Windows CE 系统下基于 LabVIEW Mobile模块的储液罐监控系统快速设计 [J], 潘世豪;王瑞波;王君丽;王永庆5.基于Windows Mobile的半导体CVD监控系统的设计与实现 [J], 刘颖;厉鲁卫;夏诗怡因版权原因,仅展示原文概要,查看原文内容请购买。
华为智能视频监控云IVS商业概述说明书
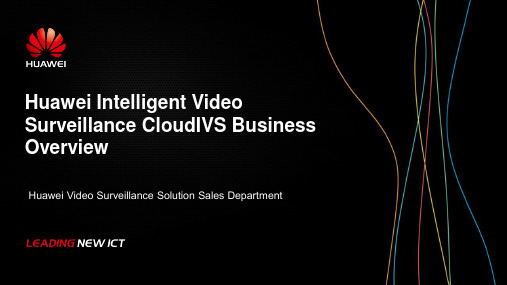
Huawei Intelligent Video Surveillance CloudIVS Business OverviewHuawei Video Surveillance Solution Sales DepartmentContentSuccess Cases Around the WorldVideo Surveillance Challenges and Trends123Huawei IVS Overview and CloudIVS SolutionChallenge 1: Video Big DataLondonPopulation 14 Million Area 8,382 km 2GDP US $531 Billion (2017)500,000Cameras43 PBVideo files per day1,200,000Movies per dayHow can the police find Valuable Data from massive amounts of video and make good use of it?London Metro Bombings56Killed2 weeksRecordingsManual Check4ArrestedIf this slide refers to the 2005 bombings, a total of seven people werearrested in connection with these acts, but that detail is of no consequence.Huawei had nothing to do with solving these crimes and will be seen as tasteless and deplorable for trying to leverage these terrible events to sell products. Please change this slide to a generic example of a terrorist attack on a major city that has 500,000 cameras. Delete the photos. Delete the number killed and the number arrested.Challenge 2: Network Security of Video SurveillanceForeign IPs ControlCase 1: In 2015, police in one Chinaprovince issued a notice to a surveillance system vendor demanding that system vulnerabilities be fixed.Case 2: In October 2016, hackers used millions of networked cameras and video recorder devices tolaunch a DDoS attack on an Internet DNS server, bringing down nearly half of the Internet in the United States.Level 3 Threat Research LabsSome of the devices were cameras and DVRs, but the devices also included printers, residential gateways, and baby monitors. Rather than being an example of surveillance system vulnerability, this is an example of the Vulnerability of Everything.Major Trends in Video Surveillance200820122016Analog →Digital IntelligentSD →HD NVR/DVRNetworkingVideo cloud20002020Digital eraNetwork eraAI eraVideo cloud technologies reduce Total Cost of Ownership (TCO) and protect existing CCTV investment .Video cloud supports multiple AI technologies to help find valuable data to prevent and solve crimes efficiently.1,0002,0003,0004,0005,0006,0007,0002015201620172018201920201,760.002,250.002,880.003,690.004,740.006,160.00Video Cloud Service is Growing Rapidly0.0500.01,000.01,500.02,000.02,500.03,000.020182019202020212022387.6655.31,075.41,702.22,627.1Deep-learning-enabled recorders and serversVideo cloud service and deep learning analytics market will reach US$7 Billion in 2020.The network security situation is getting more and more severe.Unit: US$MSource: TECHNAVIOCAGR 62.7%Unit: US$MSource: IHS ReportCAGR 28%Future of Video Surveillance Platform: Cloud-based + Deep Learning Artificial IntelligenceContentSuccess Cases Around the WorldVideo Surveillance Challenges and Trends123Huawei IVS Overview and SolutionInternational organizations Technical strengthInnovative chipset: Huawei-developed core technologyGlobal market share: 70% Innovation⚫Member of 123standards organizations, holding 148keypositions; has submitted 23,000+ standards proposals.⚫One of the eight OpenStack Platinum Members in cloudcomputing domain. Contributed2.7 million lines of code.⚫Standards: ITU, MPEG, 3GPP, CWPAN, AVS, and ONVIF⚫Chair of the HEVC video coding standard: Submitted100+accepted proposals. An H.265 main contributor.⚫Originator of the IETF network video storage standard.⚫Innovative video technologies: four major innovationsand 350+ video patents.Why Huawei Intelligent Video Surveillance?Most Secure: World’s first camera vendor to passinternational EAL3+ security certificationHuawei Builds Technology Leadership Based on Global R&D CentersMunichSan DiegoSilicon ValleyDallasOttawaParisMoscowBeijingShanghai Chengdu XianNanjingBangaloreHangzhouWuhanTurkey New JerseyChicagoUSA San Diego USA Dallas Russia MoscowIndia BangaloreGermany Munich Sweden Stockholm France Paris Italy MilanThe only company in the video surveillance industry with end-to-end core technology:chipset, algorithms, hardware, network, analytics, and cloud computingSwedenWireless and networkMilan Machine learningAudio and video algorithmProduct centerSolution centerVideo analyticsChipset designISP image processingHuawei Intelligent Video Surveillance Solution OverviewOpen PlatformUnified Resource SchedulingMatrix Intelligent Huawei Video Cloud and Global Partners Carrier Operator Government Health CampusEnergyPublic Safety Transportation Finance …Big Data AnalysisMedia AnalyticsMedia ManagementHuawei Software-Defined Camera…Person/vehicle checkpointPublic security videoSocial videoWi-Fi Huawei CloudIVS Video Cloud Platform Offers a Comprehensive Set of Capabilities Based on Four Key TechnologiesComputing PoolStorage PoolNetwork PoolCloudIVS SuperIoTVideo Image MetadataResource Sharing Service CoordinationVideo big dataVideo sharing Video analysis Service Agility3D MapDimensional cloud-based security applicationDynamic portrait checkpointMulti-dimensional data correlation analysisValued videocase association analysisAll CloudVersatile Networking•Cloud-based coordination for high efficiency and reliability •Hyper-converged cloud node•Multi-dimensional data connections•Shows real-time status of terminalsOpen Ecosystem•Agile enterprise integration gets ISVs online quickly•Multiple algorithms for many scenarios•Allows engine uploads to meet specific requirementsMatrix IntelligenceCloudIVS LiteVideo ManagementVideo AnalysisVideo RetrievalScenario-basedCloudIVS Lite Video Management Video Analysis Video RetrievalHuawei CloudIVS Lite: Quick-Start Coverage at Edge, Do More with LESSContentSuccess Cases Around the WorldVideo Surveillance Challenges and Trends123Huawei IVS Overview and SolutionSuccess Case 1: Huawei CloudIVS Assists City with Face-Recognition Big Data ApplicationsDeployed incity toprotect 14 million populationCloudOpen architecture to accesstens of thousandsof future camerasVideo CloudThousands offacial recognition algorithmsfrom multiple vendorsOpen EcosystemOpen SDK forsystem integratorto develop facebig data serviceCaseSolving Rate20%Success Case 2: Huawei CloudIVS Realizes Terminal, Edge, and Cloud Coordination for CityAll Cloud Distributed and coordinated architectureFront-EndStructuringThousands of vehiclestructure camerasand facial capturecamerasVideo CloudHundreds ofvehicle, facial, andhuman body recognitionalgorithmsDeployed ina cityand five towns to protect4 million populationCrime Rate30%“vehicle structured cameras” isn’tcorrect. Is it vehicle structure(recognizing) or vehicle-mounted?Success Case 3: Country’s CloudIVS Connects Public Video CamerasDeployed incity toprotect5 million populationCloudbased on OpenStackconnects nearly10,000camerasVideo CloudHuman body searchalgorithms and more infutureOpen EcosystemOpen SDK for integrationwith local servicesPublicSatisfaction40%Success Case 4: CloudIVS Unifies Management of Company’s Four CampusesAll CloudHQ + N divisions architectureDivision AutonomyThousands of cameras with local managementat division;video share to HQVideo Cloud EdgeHundreds ofvehicle and facial recognition algorithms for each campusDeployed infour citiesto protect 2 million m 2campus areaMember ofGlobal Fortune 500Cost15%Huawei Intelligent Video Surveillance solutions have servedpeople in countries and regionsGlobal Footprint of Huawei’s Intelligent Video Surveillance SolutionsGhanaNanjingEgyptChinaMexicoMongoliaPakistanAngolaEcuadorLaosTrinidad and TobagoIndonesiaKenyaEgyptMexicoRussiaGuangzhouShanghaiHefeiSerbia UKItalyVenezuela UgandaBrazilSingaporeTHANK YOUCopyright?All logos and images displayed in this document are the sole property of their respective copyright holders. No endorsement, partnership, or affiliation is suggested or implied.。
海康威视HikCentral Master Lite V1.0.0数据手册说明书
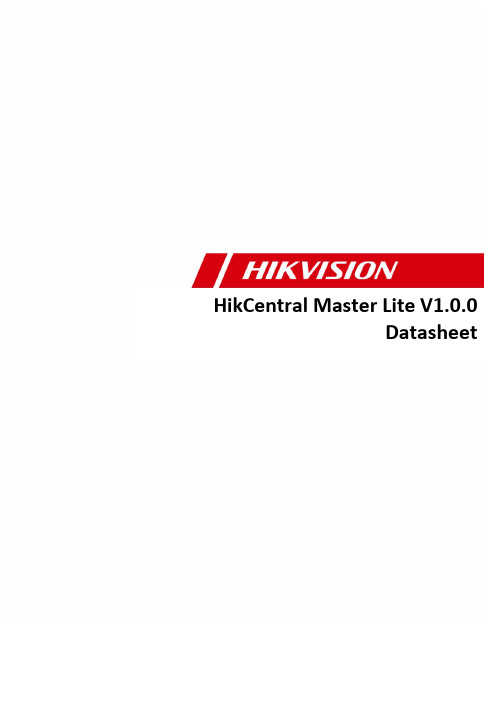
HikCentral Master Lite DatasheetHikCentral Master Lite V1.0.0DatasheetIntroductionHikCentral Master is an intelligent managementplatform under the architecture of AI Cloud,facing to edge domain. It focuses on dataapplication, data collection, storage, processing,and intelligent applications. It provides dataintegration, storage and analysis of multidimensional data in the scenario of Edge Domain and Cloud Center, integrated with technologies of Artificial Intelligence and Internet-of-Things (IoT).Key Components●Server Modules:Module ID Module NameHikCentral-Master Lite-VAS Video System ManagementHikCentral-Master Lite -METIS Application of Electronic MapHikCentral-Master Lite -BMS Vehicle ApplicationHikCentral-Master Lite -LANE Vehicle LaneHikCentral-Master Lite -VIDEO Video ChannelHikCentral Master Lite -VIOLATION Violation Management●Web Client/Control Client/Plug-in AssistantsSystem RequirementsFeature DescriptionOS for Server Linux Centos7.4(for hik)OS for Control Client Microsoft® Windows 7 (32/64-bit) Microsoft® Windows 10 (32/64-bit)Browser Version Chrome 68 and aboveFunction FeaturesPlatform●Intelligent Platform ManagementSupports on-stop installation, parameter configuration, server monitoring, and log analysis.Supports cluster management and authorization management.●Intelligent Applications: With the integration of multiple functionality and applicationsoftware, the platform extends its applications from video surveillance to the ones based on artificial intelligence algorithms, such as Automatic Number-Plate Recognition (ANPR) andfacial recognition. This makes the platform applicable to the usage scenarios such as city surveillance system and intelligent transportation system.●Open Platform: Supports integrating with third-party infrastructure, data, platform, andapplications based on open standards.●Comprehensive Security Mechanism: Provides security mechanism for device, network, hostcomputer, data, and applications, and supports multiple security control schemes.●Extensibility and ScalabilitySupports expanding the capacity of the services such as the maximum added device amount, stream storage, events, and data library.Increases capacity and stability by distributed deployment.Supports cluster deployment for some key services such as streaming gateway.Supports techniques such as clustered proxy, distributed cache, and load balancing to improve the response speed, and decrease performance losses caused by the interactionamong different components and modules, therefore improving the fluency of theplatform.●Multiple Usage Scenarios: Supports assembling different modules into different platformsbased on different usage scenarios. The platforms can be compatible with each other and cooperate with each other.Web Client●Video ApplicationE-map: Supports basic video application (video plan, video tracking, video linkage, three-circle monitoring, etc.), data analysis application (pattern analysis and area comparison),GPS application (route plan, GPS positioning, video relay, etc.), and situation analysis(alarm view and flow heat map).Video Search: Supports live video, video footage search, camera search, Favorites, and video plan management.●Search CenterVehicle Search: Supports searching passing vehicles by vehicle features.Device Alarm Search♦Supports displaying alarm information of all alarm devices, including device type, device name, alarm type, and alarm time.♦Supports filtering alarm information by device type, device name, device No., alarm type, etc.♦Supports view alarm details, including the basic information of the alarm and the alarm related picture (the picture can be enlarged).VCA Alarm Search♦Supports displaying all VCA alarm information, including analysis type, device name, alarm type, and alarm time.♦Supports filtering alarm information by analysis type, device name, alarm type, and alarm time, etc.♦Supports viewing alarm details, including basic information of the alarm and the alarm related picture (the picture can be enlarged).●Arming Alarm CenterVehicle Arming♦Supports creating a vehicle arming task basing on license plate, vehicle model, red list, and vehicle list library, and configuring client linkage and SMS message linkage(when the armed vehicles are detected, the platform will remind user by thelinkage).♦Supports operations including disarming, exporting, and approving arming task, etc.Target Alarm Search: Supports searching alarms basing on an arming task. The alarm details include alarm records, pattern on map, arming information, and pictureinformation.●Video MaintenanceOverview♦Supports displaying the data overview, including the total number of cameras, camera online rate, normal image rate, video integrity rate, resource running status, ,the camera running status trend, video exceptions, etc., in a visualized way.♦Supports quickly inspecting running status of the resources and ranking a selected area and its child areas based on theirs score in terms of resource health level.Video Surveillance♦Supports online status monitoring, video quality diagnosis, and recording check for cameras.♦Supports status monitoring and management of encoding devices, storage devices, and decoding devices, and viewing details of these devices.♦Supports customizing device topology tree and topology page functions.Alarm Search♦Supports viewing current day alarms, total alarm amount, and amount of each type of alarms, which includes alarm about resource status, recording exception, videoquality, and others.♦Supports viewing alarm details, and other operations including acknowledging, deleting, exporting and refreshing alarms, etc.♦Supports filtering alarms by alarm status, alarm source name, and alarm source, etc.Report Analysis: Supports generating area overview report, video quality report, recording status report, streaming status report, camera status report, and videoretention status report.●Analysis CenterVehicle Data Statistics♦Supports analyzing the passing vehicle data of a specific checkpoint in a specified time period, and displaying the results in the form of daily report and monthlyreport.♦Supports analyzing passing vehicle total number, top 5 checkpoint of passing vehicle (redirecting page is allowed to display more), top 5 frequent passing vehicles(redirecting page is allowed to display more) in a specified time, and peak hour;Calculating total amount of all vehicles, out-of-town vehicle, and first-time entryvehicle in specified time; Displaying in list or graph by registration location, brand,vehicle type and vehicle color.Control Client●Video WallSupports dividing video wall evenly into 1, 4, 6, 8, 9, or 16 windows.Supports jointing or cancel jointing of windows, opening window (i.e., open a layer of image to show the live image of a specific camera), roaming (i.e., drag window to adjustits size and position), and resizing a video wall screen to the whole video wall.Supports video wall image layer operations, such as sticking a specific image to the top or to the bottom.Supports dragging a specific camera to a window of the video wall to display thec amera’s live video.Supports audio control, PTZ control, configuring storage location and storage type, and local playback by the selected date.Supports playback on video wall and audio control during playback.Supports auto-switching cameras for live videos.Supports displaying system desktop of the PC where the Client is installed on video wall.Supports displaying live view of analog channel on video wall.Supports configuring stream type (main steam and sub-stream) for a camera whose video is displaying on video wall.Supports audio control for the output channel.Supports adding virtual textbox on the video wall and editing the text.Supports fuzzy search for cameras.Supports setting decoding resolution of the channel displayed on video wall to D1 resolution, 720P, or 1080P.●Smart LinkageSupports automatically detecting moving objects in the images and highlight the objects by red or green frames.Supports locating object in red frame through PTZ camera.Supports selecting object in green frame and relocating it through PTZ camera, and then tracking the target.Supports selecting an area in the images of the fixed camera, and then the PTZ camera will locate and track the area.●Real-Time Video AlarmSupports temporarily disarm alarm cameras and batch deleting alarm information.Supports displaying the alarm details, including alarm name, alarm priority, alarm source, alarm type, alarm time, etc.Supports viewing the live video of the selected alarm cameras and the video footage recorded during the alarm time.Supports filtering alarms by alarm name, alarm type, alarm priority, and alarm time. Operation and Management Center●Parameter ConfigurationCenter Server Configuration♦Supports configuring parameters for components and servers.♦Supports detecting port conflicts.♦Supports verifying the validity of the user-specified parameter values.Local Server Configuration♦Supports configuring parameters for local servers and components of the servers, and setting the parameters to be consistent with the corresponding parameters ofthe Operation and Management Center or not.♦Supports detecting port conflicts.♦Supports verifying the validity of the user-specified parameter values.Center Alarm Configuration♦Supports adjusting the alarm strategy♦Supports restoring the alarm strategy to its default value.Local Alarm Configuration♦Supports adjusting alarm strategy, which can be inconsistent with alarm strategy of the Operation and Management Center.♦Supports restoring the alarm strategy to the value same with the alarm strategy of the Operation and Management Center.Time Synchronization Configuration♦Supports setting NTP service in central management server or external servers.♦Supports automated time synchronization by server.♦Supports manual synchronization.Multi-Domain Configuration♦Supports filtering by service name, server name, port, and keywords.♦Supports creating new domains.♦Supports editing the multi-domain configuration of a specified service.●Software Running Status MonitoringDisplaying Status in Chart and List♦Supports displaying the topology and running status of the servers and components in charts, as well as alarms and status statistics.♦Supports displaying the running status of the current server and component on video wall.♦Supports scoring system running status.♦Supports statistics of server online rate and relevant details.♦Supports statistics of alarms (handled, ignored, and unhandled) in recent 7 days.♦Supports statistics of active users in recent 7 days.Server Status Monitoring♦Supports viewing the status of the servers, including CPU usage, memory usage, HDD usage, etc.♦Supports viewing alarms and handling alarms.♦Supports displaying the records of maintenance for software.♦Supports viewing the basic information of the servers.Component Status Monitoring♦Supports viewing the running status of the components, including CPU usage, andmemory usage.♦Supports enabling/disabling services within components.♦Supports self-inspection for the component status.♦Supports viewing alarms and handling alarms.♦Supports viewing maintenance records.♦Supports viewing basic information of the components.Enabling/Disabling Service♦Quickly enabling, disabling, or rebooting all services or the selected ones.♦Enabling, disabling, or rebooting a single service.●Alarm SolvingSupports searching alarms generated within the whole system.Supports batch solving alarms. Including batch handling alarms and batch ignoring alarms.Supports providing different handling suggestions for users to help them handle the detected exceptions. Users can save their methods and experience for handlingproblems to the knowledge base.Supports exporting the matched alarms after search.●Log AnalysisSystem Log♦Supports searching system logs by service, log time, log level, keywords, and categorizing the search results in different tabs by service types.♦Supports viewing error codes and call chains in the logs, as well as the related contents.♦Supports exporting the search results.Operation Log♦Supports searching logs by log time, operation result, and keywords.♦Supports exporting search results.●Knowledge BaseProvides a forum for maintenance personnel to communicate and share their experience in solving problems.Supports exporting and importing the shared experience.Supports searching articles in the knowledge base by keywords.●License ManagementSupports activating new product license, managing activated license file or activation code, deactivating license, and expanding license capacity.Supports viewing the current license status, including the authorized items and corresponding values.●Service ManagementSupports searching services by components, service type, server and keywords.Supports manually adding services, and other operations such as editing, viewing and deleting services.●Person Group ManagementSupports manually adding person information.Supports batch importing person information.Supports exporting person information.●Data BackupSupports manually backing up all data or the data of the specified components. And the backed up data can be manually deleted.Supports automatic backup and setting automatic backup strategy.。
电梯功能介绍英语作文

电梯功能介绍英语作文Elevators are an integral part of modern architecture, providing a convenient means of vertical transportation in buildings. Here's an introduction to the various functions of an elevator in English:Introduction to Elevator FunctionsElevators are not just simple boxes that move up and down; they are complex systems designed to ensure safe andefficient transportation of people and goods within multi-story buildings. Here are some key functions of an elevator:1. Vertical Transportation: The primary function of an elevator is to transport people and goods vertically between floors. This is achieved through a system of cables and pulleys that move the elevator car along a guide rail.2. Safety Features: Elevators are equipped with a range of safety features to prevent accidents and ensure passenger safety. These include emergency brakes, door interlocks that prevent the elevator from moving when doors are open, and emergency communication systems.3. Control Systems: Modern elevators are controlled by sophisticated computer systems that manage the operation ofthe elevator, including call signals, direction of travel, and speed. These systems also monitor the elevator's performance and alert maintenance personnel if any issues arise.4. Accessibility: Elevators are designed to be accessible to all users, including those with disabilities. This includes features such as audio announcements, braille buttons, and wider doors to accommodate wheelchairs.5. Energy Efficiency: Many elevators today are designed with energy efficiency in mind. This can include regenerative braking systems that convert kinetic energy back into electricity, reducing the overall energy consumption of the building.6. Emergency Power: In the event of a power failure, elevators are often equipped with emergency power systems that allow them to operate for a limited time, ensuring that passengers can safely exit the elevator.7. Maintenance and Monitoring: Elevators require regular maintenance to ensure they operate safely and efficiently. Many elevators are now equipped with remote monitoring systems that allow maintenance personnel to diagnose and address issues before they become critical.8. Environmental Control: Elevators are often equipped with air conditioning and ventilation systems to ensure a comfortable environment for passengers, regardless of the outside temperature.9. Security: For added security, elevators may have video surveillance and access control systems that restrict access to certain floors or require a keycard for operation.10. Design and Aesthetics: Elevators are not just functional; they are also designed to complement the aesthetics of the building they serve. This can include custom finishes, lighting, and even artwork.In conclusion, elevators are sophisticated pieces of machinery that offer a range of functions beyond just moving between floors. They are designed with safety, efficiency, accessibility, and aesthetics in mind, making them an essential component of modern buildings.This essay provides a comprehensive overview of the various functions that an elevator performs, ensuring that readers understand the complexity and importance of these systems in our daily lives.。
智能视频监控系统中改进YOLO算法的实现
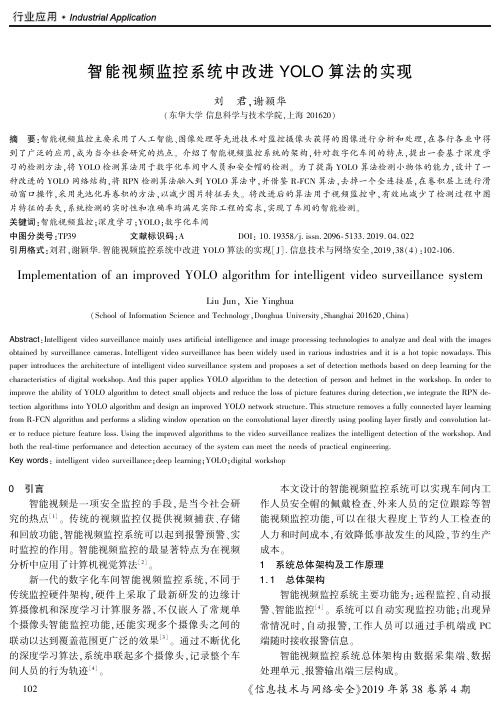
智能视频监控系统中改进YOLO算法的实现刘㊀君ꎬ谢颖华(东华大学信息科学与技术学院ꎬ上海201620)摘㊀要:智能视频监控主要采用了人工智能㊁图像处理等先进技术对监控摄像头获得的图像进行分析和处理ꎬ在各行各业中得到了广泛的应用ꎬ成为当今社会研究的热点ꎮ介绍了智能视频监控系统的架构ꎬ针对数字化车间的特点ꎬ提出一套基于深度学习的检测方法ꎬ将YOLO检测算法用于数字化车间中人员和安全帽的检测ꎮ为了提高YOLO算法检测小物体的能力ꎬ设计了一种改进的YOLO网络结构ꎬ将RPN检测算法融入到YOLO算法中ꎬ并借鉴R ̄FCN算法ꎬ去掉一个全连接层ꎬ在卷积层上进行滑动窗口操作ꎬ采用先池化再卷积的方法ꎬ以减少图片特征丢失ꎮ将改进后的算法用于视频监控中ꎬ有效地减少了检测过程中图片特征的丢失ꎬ系统检测的实时性和准确率均满足实际工程的需求ꎬ实现了车间的智能检测ꎮ关键词:智能视频监控ꎻ深度学习ꎻYOLOꎻ数字化车间中图分类号:TP39㊀㊀㊀㊀㊀㊀文献标识码:A㊀㊀㊀㊀㊀㊀DOI:10.19358/j.issn.2096 ̄5133.2019.04.022引用格式:刘君ꎬ谢颖华.智能视频监控系统中改进YOLO算法的实现[J].信息技术与网络安全ꎬ2019ꎬ38(4):102 ̄106.ImplementationofanimprovedYOLOalgorithmforintelligentvideosurveillancesystemLiuJunꎬXieYinghua(SchoolofInformationScienceandTechnologyꎬDonghuaUniversityꎬShanghai201620ꎬChina)Abstract:Intelligentvideosurveillancemainlyusesartificialintelligenceandimageprocessingtechnologiestoanalyzeanddealwiththeimagesobtainedbysurveillancecameras.Intelligentvideosurveillancehasbeenwidelyusedinvariousindustriesanditisahottopicnowadays.Thispaperintroducesthearchitectureofintelligentvideosurveillancesystemandproposesasetofdetectionmethodsbasedondeeplearningforthecharacteristicsofdigitalworkshop.AndthispaperappliesYOLOalgorithmtothedetectionofpersonandhelmetintheworkshop.InordertoimprovetheabilityofYOLOalgorithmtodetectsmallobjectsandreducethelossofpicturefeaturesduringdetectionꎬweintegratetheRPNde ̄tectionalgorithmsintoYOLOalgorithmanddesignanimprovedYOLOnetworkstructure.ThisstructureremovesafullyconnectedlayerlearningfromR ̄FCNalgorithmandperformsaslidingwindowoperationontheconvolutionallayerdirectlyusingpoolinglayerfirstlyandconvolutionlat ̄ertoreducepicturefeatureloss.Usingtheimprovedalgorithmstothevideosurveillancerealizestheintelligentdetectionoftheworkshop.Andboththereal ̄timeperformanceanddetectionaccuracyofthesystemcanmeettheneedsofpracticalengineering.Keywords:intelligentvideosurveillanceꎻdeeplearningꎻYOLOꎻdigitalworkshop0㊀引言智能视频是一项安全监控的手段ꎬ是当今社会研究的热点[1]ꎮ传统的视频监控仅提供视频捕获㊁存储和回放功能ꎬ智能视频监控系统可以起到报警预警㊁实时监控的作用ꎮ智能视频监控的最显著特点为在视频分析中应用了计算机视觉算法[2]ꎮ新一代的数字化车间智能视频监控系统ꎬ不同于传统监控硬件架构ꎬ硬件上采取了最新研发的边缘计算摄像机和深度学习计算服务器ꎬ不仅嵌入了常规单个摄像头智能监控功能ꎬ还能实现多个摄像头之间的联动以达到覆盖范围更广泛的效果[3]ꎮ通过不断优化的深度学习算法ꎬ系统串联起多个摄像头ꎬ记录整个车间人员的行为轨迹[4]本文设计的智能视频监控系统可以实现车间内工作人员安全帽的佩戴检查㊁外来人员的定位跟踪等智能视频监控功能ꎬ可以在很大程度上节约人工检查的人力和时间成本ꎬ有效降低事故发生的风险ꎬ节约生产成本ꎮ1㊀系统总体架构及工作原理1.1㊀总体架构智能视频监控系统主要功能为:远程监控㊁自动报警㊁智能监控[4]ꎮ系统可以自动实现监控功能ꎻ出现异常情况时ꎬ自动报警ꎬ工作人员可以通过手机端或PC端随时接收报警信息ꎮ智能视频监控系统总体架构由数据采集端㊁数据处理单元㊁报警输出端三层构成ꎮ数据采集端可以支持不同视频流输入ꎬ包括监控专网下的海康威视球机㊁枪机㊁半球机以及门禁系统中的抓拍机等ꎬ可以连接多个摄像头ꎬ实时监控车间场景ꎻ数据处理单元由GPU㊁服务器㊁智能监控软件HES构成ꎮGPU可以实现视频监控的智能化ꎬ分析多帧视频的监控情况ꎬ进行异常画面的捕捉和分析ꎬ并进行推送ꎻ服务器将做分布式处理ꎬ其硬盘为磁盘阵列ꎬ以便后续扩展ꎻ智能视频监控软件采用B/S架构ꎬ可利用网页和客户端软件发布监控信息ꎬ充分利用QT对实时监控的优化嵌入ꎻ报警输出端可以及时将报警信息推送给客户端[4]ꎮ1.2㊀系统工作原理智能视频监控技术的核心在于对视频中运动目标进行自动检测㊁提取特征㊁识别和行为分析[5]ꎮ智能视频监控系统可以实现多摄像头联动工作ꎬ四种摄像头分别为全局摄像头㊁人脸摄像头㊁行为摄像头㊁跟踪摄像头ꎮ该系统包括5个模块ꎬ分别为图像获取模块㊁图像预处理模块㊁数据压缩编码处理模块㊁图像分析模块以及图像理解模块[6]ꎮ图像采集模块主要用于获得四种摄像头拍摄的图像ꎻ图像预处理模块可以减少光照等因素的影响ꎬ提高图片质量ꎻ数据压缩编码处理模块用来融合多源图像信息ꎻ图像分析模块主要对图像中的目标进行检测跟踪ꎻ图像理解模块主要是研究图像中不同对象的性质以及它们之间的关系[7]ꎮ图1所示为系统工作原理图ꎮ图1㊀系统工作原理图2㊀YOLO算法及其改进2.1㊀YOLO算法YOLO(YouOnlyLookOnce)实时目标检测算法由JosephRedmon等于2016年5月提出[7]ꎮ该算法可同时预测目标物体的位置和类别ꎬ采用端到端的检测方法ꎬ检测速度快ꎮ本文中智能视频监控系统采用了YOLO是一种基于深度学习卷积神经网络的目标检测模型ꎮ卷积神经网络主要利用卷积层对输入的图片进行卷积运算ꎬ进而降低图片数据的维度ꎬ进行特征提取[8]ꎮ图2所示为深度卷积神经网络的构成ꎮ图2㊀深度卷积神经网络的构成YOLO检测具体实现过程如下:将图片分为SˑS个网格ꎬ当目标物体中心落在某一个格子时ꎬ该格子就负责预测目标物体ꎮ如图3所示ꎮ图3㊀网格图每个格子会预测B个边界框(boundingbox)以及边界框的置信度(confidencescore)ꎮ其中包括边界框的中心坐标(xꎬy)ꎬ边界框的长宽w㊁hꎬ以及边界框的准确度ꎮ置信度表示格子内是否有检测物体ꎬ定义置信度为:Pr(Object)ˑIOUtruthpred(1)其中ꎬIOU表示交集与并集的比值ꎬ为系统预测出来的边界框与物体实际的边界框的重合程度ꎮ若格子内存在目标物体ꎬ则Pr(Object)=1ꎬ置信度为IOUtruthpredꎻ若格子内不存在目标物体ꎬ则Pr(Object)=0ꎬ置信度为0ꎮ每个格子在输出边界框的同时还要输出各个网格存在物体所属分类的概率ꎬ记为:Pr(Classi|Object)(2)按照以下公式可以计算整张图片中ꎬ各个格子物体类别的概率:Pr(Classi|Object)ˑPr(Object)ˑIOUtruthpred=Pr(Classi)ˑIOUtruthpred(3)设定合适的概率阈值ꎬ高于概率阈值的就是识别出来的目标分类ꎬ利用非极大值抑制的方法ꎬ去掉概率较低的边界框ꎬ输出概率最高的边界框作为最后的预测结果ꎮ所以每个网格输出Bˑ(4+1)+C个预测值ꎬ卷积神经网络输出一个维度为SˑSˑ(Bˑ5+C)的固定张量ꎮS为划分网格数量ꎬB为每个网格预测的边界框数ꎬC为每个网格预测的物体类别个数ꎮYOLO的网络结构包括24个卷积层㊁4个池化层ꎬ以及2个全连接层ꎬ如图4所示ꎮ其中卷积层提取图像特征ꎬ全连接层预测目标物体位置以及物体类别概率ꎬ池化层负责压缩图片像素ꎬ利用Softmax回归分析输出目标类别概率ꎮ图4㊀YOLO网络结构图YOLO的损失函数计算公式为:λcoordðS2i=0ðBj=0Ιobjij[(xi-x^i)2+(yi-y^i)2]+λcoordðS2i=0ðBj=0Ιobjij[(wi-w^i)2+(hi-h^i)2]+ðS2i=0ðBj=0Ιobjij(ci-c^i)2+λnoobjðS2i=0ðBj=0Ιnoobjij(ci-c^i)2+ðS2i=0Ιobjiðcɪclasses(pi(c)-p^i(c))2(4)式(4)由3部分组成ꎬ第一部分为前两行ꎬ表示边界框的损失函数ꎻ第二部分为第三行ꎬ表示置信度损失函数ꎻ第三部分为最后一行ꎬ表示分类损失函数ꎮIobjij表示单元格i中第j个边界框负责检测目标物体ꎬ该边界框与物体实际的边界框交并比最大ꎻ(xiꎬyi)表示单元格i预测的边界框的中心点坐标ꎬ(x^iꎬy^i)表示目标物体实际边界框的中心点坐标ꎻwi表示单元格i预测的边界框的宽度ꎬw^i表示目标物体实际边界框的宽度ꎻhi表示单元格i预测的边界框的高度ꎬh^i表示目标物体实际边界框的高度ꎻInoobjij表示单元格i预测的第j个边界框未检测到目标物体ꎻci表示预测的边界框中单元格i的置信度ꎬc^i表示实际的边界框中单元格i的置信度ꎻΙobji表示单元格i中是否出现目标物体ꎻpi(c)和p^i(c)分别表示预测的和真实的边界框中单元格i中包含第c类对象的条件概率ꎮ因为一个单元格只负责预测一个物体ꎬ当多个物体的中心落在同一个格子中时ꎬ该格子很难区分物体类别ꎬ因此对于距离较近的物体识别效果不佳[9]ꎻ在图像预处理阶段ꎬ训练集图片经过卷积层后由高分辨率压缩为低分辨率ꎬ用于分类特征提取ꎬ在压缩过程中ꎬ图片中小物体的特征将很难保存ꎮ应用在车间中时ꎬ安全帽相对来说是较小的目标ꎬ当工作人员较近ꎬ或安全帽重叠时ꎬ识别效果较差ꎮ2.2㊀改进后的网络结构为了能够准确快速检测车间工作人员以及其佩带的工作帽子ꎬ需要提高YOLO算法检测小物体的能力ꎮ本文将目标检测方法RPN融入到YOLO算法中ꎬ并借鉴R ̄FCN方法设计了一种新型卷积神经网络结构ꎬ该网络结构中采用先池化再卷积的特征提取模式减少信息的丢失ꎬ将原先的两个全连接层变为一个ꎬ进而形成了一种具有较好的小目标检测能力的改进的YOLO算法[10]ꎮ最邻近的目标检测算法RPN(RegionProposalNet ̄works)的显著特点为利用滑动窗口提取特征[8]ꎮ神经网络对输入的图片进行卷积和池化操作ꎬ在最后的卷积层中ꎬ利用滑动窗口提取图片特征ꎬ输出图片的特征向量ꎬ利用softmax输出物体分类和边框回归ꎮ该算法对于单一目标检测速度快ꎬ准确率较高ꎮ全卷积神经网络R ̄FCN由卷积层和池化层组成ꎬ没有全连接层ꎬ具有良好的全局检测效果ꎬ可以保留更多的图像信息ꎬ因此本文中改进的网络结构借鉴R ̄FCN结构移除一个全连接层ꎬ只保留一个全连接层ꎬ以减少特征信息的丢失[9]ꎮ改进后的网络结构包含18个卷积层㊁6个池化层㊁1个全连接层与1个softmax输出层ꎮ其中卷积层用来提取图像特征ꎬ池化层用来压缩图片像素ꎮ如图5所示为改进后的网络结构ꎮ图5㊀改进后的网络结构输入图片后先采用2∗2最大池化层缩小图片尺寸ꎬ再进入卷积层ꎬ这样可以尽量保留图片的特征ꎻ图片经过多层卷积与池化后ꎬ输出的网格由7∗7变为14∗14ꎬ检测范围变广ꎮ图6为不同网格尺寸目标检测效果对比ꎬ7∗7网格只能检测到一个工作人员和一个安全帽ꎬ而改进后14∗14的网格可以检测到两个工作人员和一个安全帽ꎮ因此这种扩大了输出图片网格尺寸的结构具有更好的检测小目标的能力ꎮ图6㊀网格对比图2.3㊀改进后的算法流程将输入的图片集用图片打标工具labelImg打标ꎬ将图片中的人和安全帽标注出来ꎬ获得目标物体的真实坐标ꎬ制作数据集的标签ꎬ生成xml文件ꎻ之后进行图片预处理ꎬ运用基于RPN的滑动窗口算法获得每张图片的若干个目标边界框的矩阵向量ꎻ矩阵向量进入之后的神经网络进行卷积池化操作ꎻ之后输入全连接层ꎬ最后利用softmax分类函数ꎬ输出目标物体的类别ꎮ具体流程如图7所示ꎮ图7㊀改进后的算法流程3㊀实验分析基于卷积神经网络的目标检测方法需要从大量的样本中学习待检测物体的特征ꎮ本文采用的数据集为车间中四种摄像头采集到的图像数据集ꎬ四个摄像头各取1万张图片ꎬ每个图片的大小为500ˑ500ꎬ使用图片打标工具labelImg对数据集进行打标ꎮ数据集的40%作为训练集ꎬ10%用于验证ꎬ50%作为测试集ꎮ训练过程采用小批量梯度下降法和冲量ꎬ这样可以使训练过程更快收敛[11]ꎮ运用反向传播法使参数不断更新ꎬ直至损失函数收敛ꎮ网络训练参数设置:学习批次64ꎬ学习率0.001ꎬ冲量常数0.9ꎬ权值衰减系数0.0005ꎮ本文实验环境为:设备型号:DELLPowerEdge ̄R740ꎻCPU:Intel(R)Xeon(R)Silver4114CPU@2.20GHzꎻ内核版本:Linuxversion4.15.0 ̄32 ̄genericꎻ系统版本:Ubuntu16.04.03LTSꎻ内存:32GBꎻGPU:NVIDIAGe ̄ForceGTX1080TiꎻCUDA版本:9.0ꎻcuDNN版本:7.4.2ꎮ设置对照实验ꎬ使用Keras框架分别利用YOLO与改进YOLO进行检测ꎬ统计对象为工作人员与安全帽ꎮ本文选取召回率㊁准确率和检测速度作为算法性能衡量指标ꎮ两种算法召回率㊁准确率与检测速度对比如表1㊁表2ꎮ表1㊀工作人员检测对比结果表检测算法召回率/%准确率/%平均每帧处理时间/msYOLO88.3294.7422.421改进YOLO89.8695.2321.357表2㊀安全帽检测对比结果表检测算法召回率/%准确率/%平均每帧处理时间/msYOLO83.5788.8522.411改进YOLO87.9193.4220.659由实验结果看出ꎬ改进后的检测算法在召回率㊁准确率㊁快速性方面较原算法有所优化ꎮ尽管原算法检测速度较快ꎬ但是改进后速度也有了小幅度提高ꎬ可以更好地满足生产需求ꎻ在工作人员检测上ꎬ由于工作人员目标较大ꎬ故检测的召回率和准确率提高幅度较小ꎻ在安全帽检测上ꎬ召回率由83.57%提高到87.91%ꎬ准确率由88.85%提高到93.42%ꎬ有比较明显的优化ꎬ证明改进后的算法检测小物体的能力有了一定提高ꎮ4㊀结束语为了提高视频监控系统检测小物体的能力以及检测速度ꎬ更高效地实现车间监控ꎬ减少人力检查成本ꎬ本文提出了改进后的YOLO算法ꎬ将RPN算法与YO ̄LO算法相结合ꎬ借鉴R ̄FCN算法去掉一个全连接层ꎬ构成一种新的网络结构ꎬ更多地保留了图像特征ꎬ提高了检测小物体的能力ꎬ也提高了系统的综合检测能力ꎮ该算法应用于数字化车间智能视频监控系统中ꎬ具有较快的检测速度和较高的检测准确率ꎬ满足实时性要求ꎬ具有一定的应用价值ꎮ参考文献[1]刘佳.智能视频监控系统发展及应用研究[J].电子测试ꎬ2018(2):69ꎬ72.[2]TANAKAMꎬHORIUCHITꎬTOMINAGAS.ColorcontrolofalightingsystemusingRGBWLEDs[C].InternationalSocietyforOpticsandPhotonicsꎬ2011.[3]罗聪.基于人脸检测YOLO算法的专用型卷积神经网络推理加速器的研究与设计[D].广州:华南理工大学ꎬ2018. [4]王显龙ꎬ方明.一种智能视频监控系统架构方案设计[J].长春理工大学学报(自然科学版)ꎬ2018ꎬ41(1):123 ̄126. [5]朱明凌.面向智能视频监控系统的运动目标检测与异常行为分析[D].杭州:中国计量学院ꎬ2015.[6]康彬.面向智能监控的目标检测㊁追踪及图像融合算法研究[D].南京:南京邮电大学ꎬ2015.[7]REDMONJꎬDIVVALASꎬGIRSHICKRꎬetal.YouOnlyLookOnce:unifiedꎬreal ̄timeobjectdetection[C].ProceedingsoftheComputerVision&PatternRecognitionꎬ2016:779 ̄788.[8]RENSꎬHEKꎬGIRSHICKRꎬetal.FasterR ̄CNN:towardsreal ̄timeobjectdetectionwithregionproposalnetworks[C].Pro ̄ceedingsoftheInternationalConferenceonNeuralInformationProcessingSystemsꎬ2015:91 ̄99.[9]DAIJꎬYILꎬHEKꎬetal.R ̄FCN:objectdetectionviaregion ̄basedfullyconvolutionalNetworks[J].arXiv:160506409v2ꎬ2016.[10]杨观赐ꎬ杨静ꎬ苏志东ꎬ等.改进的YOLO特征提取算法及其在服务机器人隐私情境检测中的应用[J].自动化学报ꎬ2018ꎬ44(12):2238 ̄2249.[11]王宇宁ꎬ庞智恒ꎬ袁德明.基于YOLO算法的车辆实时检测[J].武汉理工大学学报ꎬ2016ꎬ38(10):41 ̄46.(收稿日期:2019 ̄02 ̄27)作者简介:刘君(1993-)ꎬ女ꎬ硕士研究生ꎬ主要研究方向:深度学习㊁目标检测ꎮ谢颖华(1972-)ꎬ女ꎬ硕士ꎬ副教授ꎬ主要研究方向:大数据㊁数据挖掘等ꎮ。
多摄像机目标跟踪系统综述
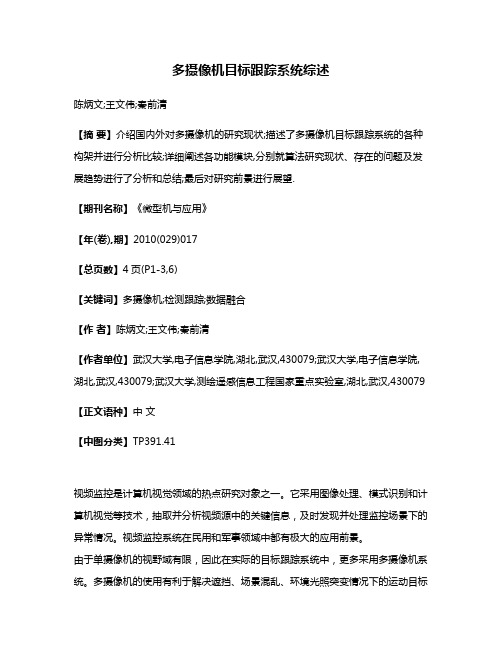
多摄像机目标跟踪系统综述陈炳文;王文伟;秦前清【摘要】介绍国内外对多摄像机的研究现状;描述了多摄像机目标跟踪系统的各种构架并进行分析比较;详细阐述各功能模块,分别就算法研究现状、存在的问题及发展趋势进行了分析和总结;最后对研究前景进行展望.【期刊名称】《微型机与应用》【年(卷),期】2010(029)017【总页数】4页(P1-3,6)【关键词】多摄像机;检测跟踪;数据融合【作者】陈炳文;王文伟;秦前清【作者单位】武汉大学,电子信息学院,湖北,武汉,430079;武汉大学,电子信息学院,湖北,武汉,430079;武汉大学,测绘遥感信息工程国家重点实验室,湖北,武汉,430079【正文语种】中文【中图分类】TP391.41视频监控是计算机视觉领域的热点研究对象之一。
它采用图像处理、模式识别和计算机视觉等技术,抽取并分析视频源中的关键信息,及时发现并处理监控场景下的异常情况。
视频监控系统在民用和军事领域中都有极大的应用前景。
由于单摄像机的视野域有限,因此在实际的目标跟踪系统中,更多采用多摄像机系统。
多摄像机的使用有利于解决遮挡、场景混乱、环境光照突变情况下的运动目标跟踪等问题,但它同时也带来了一些新难题,包括多摄像机之间的目标匹配、摄像机协作、摄像机之间的自动切换和数据融合等。
1 多摄像机跟踪系统构架1.1 集中式的系统构架在集中式系统结构[1]中,各个摄像机采集的视频数据直接被送到融合中心,在那里进行数据对准、数据相关、航迹记录、预测与综合跟踪等,而相机无自主处理能力。
这种结构的特点是信息损失小、精度高,但其对系统通信要求较高,融合中心计算负荷重,系统效率低,实时性差。
因此该结构的实用性差,生存能力低。
1.2 基于摄像机的系统构架基于摄像机的系统构架[2-4]主要分为传感器处理单元SPU(Sensor Processing Unit)、中央处理单元 CPU(Central Processing Unit)和用户接口GUI(Graphics User Interface)三部分。
外文翻译 视频监控系统
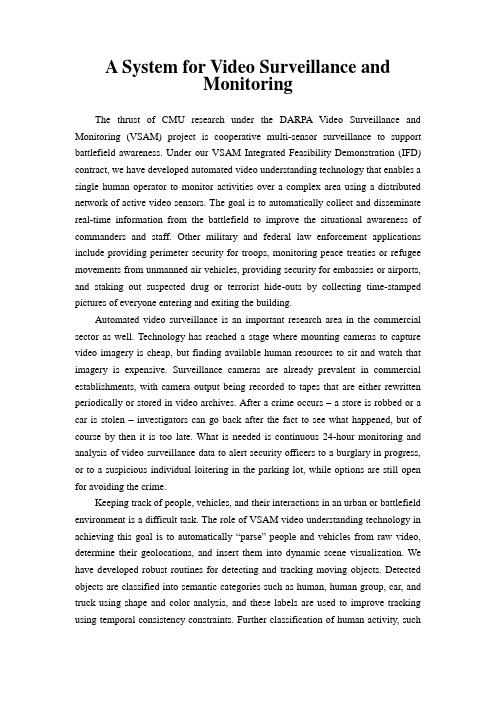
A System for Video Surveillance andMonitoringThe thrust of CMU research under the DARPA Video Surveillance and Monitoring (VSAM) project is cooperative multi-sensor surveillance to support battlefield awareness. Under our VSAM Integrated Feasibility Demonstration (IFD) contract, we have developed automated video understanding technology that enables a single human operator to monitor activities over a complex area using a distributed network of active video sensors. The goal is to automatically collect and disseminate real-time information from the battlefield to improve the situational awareness of commanders and staff. Other military and federal law enforcement applications include providing perimeter security for troops, monitoring peace treaties or refugee movements from unmanned air vehicles, providing security for embassies or airports, and staking out suspected drug or terrorist hide-outs by collecting time-stamped pictures of everyone entering and exiting the building.Automated video surveillance is an important research area in the commercial sector as well. Technology has reached a stage where mounting cameras to capture video imagery is cheap, but finding available human resources to sit and watch that imagery is expensive. Surveillance cameras are already prevalent in commercial establishments, with camera output being recorded to tapes that are either rewritten periodically or stored in video archives. After a crime occurs – a store is robbed or a car is stolen – investigators can go back after the fact to see what happened, but of course by then it is too late. What is needed is continuous 24-hour monitoring and analysis of video surveillance data to alert security officers to a burglary in progress, or to a suspicious individual loitering in the parking lot, while options are still open for avoiding the crime.Keeping track of people, vehicles, and their interactions in an urban or battlefield environment is a difficult task. The role of VSAM video understanding technology in achieving this goa l is to automatically “parse” people and vehicles from raw video, determine their geolocations, and insert them into dynamic scene visualization. We have developed robust routines for detecting and tracking moving objects. Detected objects are classified into semantic categories such as human, human group, car, and truck using shape and color analysis, and these labels are used to improve tracking using temporal consistency constraints. Further classification of human activity, suchas walking and running, has also been achieved. Geolocations of labeled entities are determined from their image coordinates using either wide-baseline stereo from two or more overlapping camera views, or intersection of viewing rays with a terrain model from monocular views. These computed locations feed into a higher level tracking module that tasks multiple sensors with variable pan, tilt and zoom to cooperatively and continuously track an object through the scene. All resulting object hypotheses from all sensors are transmitted as symbolic data packets back to a central operator control unit, where they are displayed on a graphical user interface to give a broad overview of scene activities. These technologies have been demonstrated through a series of yearly demos, using a testbed system developed on the urban campus of CMU.Detection of moving objects in video streams is known to be a significant, and difficult, research problem. Aside from the intrinsic usefulness of being able to segment video streams into moving and background components, detecting moving blobs provides a focus of attention for recognition, classification, and activity analysis, making these later processes more efficient since only “moving” pixels need be considered.There are three conventional approaches to moving object detection: temporal differencing ; background subtraction; and optical flow. Temporal differencing is very adaptive to dynamic environments, but generally does a poor job of extracting all relevant feature pixels. Background subtraction provides the most complete feature data, but is extremely sensitive to dynamic scene changes due to lighting and extraneous events. Optical flow can be used to detect independently moving objects in the presence of camera motion; however, most optical flow computation methods are computationally complex, and cannot be applied to full-frame video streams in real-time without specialized hardware.Under the VSAM program, CMU has developed and implemented three methods for moving object detection on the VSAM testbed. The first is a combination of adaptive background subtraction and three-frame differencing . This hybrid algorithm is very fast, and surprisingly effective – indeed, it is the primary algorithm used by the majority of the SPUs in the VSAM system. In addition, two new prototype algorithms have been developed to address shortcomings of this standard approach. First, a mechanism for maintaining temporal object layers is developed to allow greater disambiguation of moving objects that stop for a while, are occluded by other objects,and that then resume motion. One limitation that affects both this method and the standard algorithm is that they only work for static cameras, or in a ”stepand stare” mode for pan-tilt cameras. To overcome this limitation, a second extension has beendeveloped to allow background subtraction from a continuously panning and tilting camera . Through clever accumulation of image evidence, this algorithm can be implemented in real-time on a conventional PC platform. A fourth approach to moving object detection from a moving airborne platform has also been developed, under a subcontract to the Sarnoff Corporation. This approach is based on image stabilization using special video processing hardware.The current VSAM IFD testbed system and suite of video understanding technologies are the end result of a three-year, evolutionary process. Impetus for this evolution was provided by a series of yearly demonstrations. The following tables provide a succinct synopsis of the progress made during the last three years in the areas of video understanding technology, VSAM testbed architecture, sensor control algorithms, and degree of user interaction. Although the program is over now, the VSAM IFD testbed continues to provide a valuable resource for the development and testing of new video understanding capabilities. Future work will be directed towards achieving the following goals:l better understanding of human motion, including segmentation and tracking of articulated body parts;l improved data logging and retrieval mechanisms to support 24/7 system operations;l bootstrapping functional site models through passive observation of scene activities;l better detection and classification of multi-agent events and activities;l better camera control to enable smooth object tracking at high zoom; andl acquisition and selection of “best views” with the eventual goal of recognizing individuals in the scene.视频监控系统在美国国防部高级研究计划局,视频监控系统项目下进行的一系列监控装置研究是一项合作性的多层传感监控,用以支持战场决策。
- 1、下载文档前请自行甄别文档内容的完整性,平台不提供额外的编辑、内容补充、找答案等附加服务。
- 2、"仅部分预览"的文档,不可在线预览部分如存在完整性等问题,可反馈申请退款(可完整预览的文档不适用该条件!)。
- 3、如文档侵犯您的权益,请联系客服反馈,我们会尽快为您处理(人工客服工作时间:9:00-18:30)。
An Architecture for Video Surveillance Service based on P2P and Cloud ComputingYu-Sheng WuDept. of CSIEĭġNational Taipei University New Taipei City, Taiwant123t23@Yue-Shan ChangDept. of CSIE,, National Taipei UniversityNew Taipei City, Taiwanysc@.twTong-Ying JuangDept. of CSIENational Taipei UniversityNew Taipei City, Taiwanjuang@.twJing-Shyang YenCriminal Investigation BureauTaipei City, TaiwanAbstract- Video Surveillance Services is increasingly becoming important part in daily live. For traditional distributed Video Surveillance Services, each video catcher will store its streaming data to server. It will create a great volume of data daily. The ap roach obviously incurs some p roblems in keep ing daily data into the data center (DC), such as limited bandwidth to DC, limited sp ace of DC, overloading to DC, reliability, scalability, and so on. In this p ap er, we p rop ose a novel architecture based on well-develop ed p eer to p eer technology and emerging cloud comp uting for solving the issues. The architecture exploits inherent characteristics of P2P and Cloud comp uting to p rovide an economic, scalable, reliable and efficient approach to store video data. We present the architecture, components, its operation flow, and depict the imp lementation issue in this p ap er. We believe that the architecture can improve significantly issues.Ke y words- P2PCloud, Video Surveillance, P2P streaming, Hadoop, HDFSI.I NTRODUCTIONSurveillance System (SS) is an important application around our life environment. I t is very useful to governments and law enforcement to maintain social control, recognize and monitor threats, and prevent/investigate criminal activity. I t may be applied to observation from a distance by means of electronic equipment (such as CCTV cameras), or interception of electronically transmitted information. There are increasingly deployed in the recent year.SS have evolved to third generation [7ĭġ8], which all applied digital device and storing the video in the pure data stream. They are converted and stored into digital and then delivered over networks. At first, the surveillance system adopted analog devices, such like CCT and closed-circuit TV, to capture the continuous scene and transmitted the video signal to other place. I n the second generation, the system begins to apply digital technologies to deal with each video, such as automatic event detection and alarm. Nowadays, the SS can compass the video stream to save the bandwidth for applied the communication networks.A video surveillance system is composed of three parts: front-end video capture device, the central control platform and user client.The front-end video contains the digital video recorder and the control to deal with the video dispatch. The communication of surveillance system is the important problem. Currently, the SS is a distributed architecture, every client stores front-end device’s video data into a centralized server [1]. Such scheme naturally has its advantage on management and use. But, it also has some inferiority on other aspect, such as scalability, reliability, and efficiency.For the scalability aspect, while the number of front-end device (FE) increasing, obviously, there are some problems will be risen. For example, the required bandwidth and access speed on the server side will be considerable increasing with positive proportional to the number of FE. This will limit the number of FE in the system. For the reliability aspect, the data center in the centralized server will be a critical component. Whole system cannot work well crash while the data center crash. For the efficiency problem, the data center is the bottleneck of the system while multiple clients currently access to the system. Therefore, proposing an approach to solve the above problems is an important issue.Figure 1: Macro view of the proposed architecturen this paper, we propose a novel architecture for surveillance system based on well-known peer to peer technology and emerging cloud computing, as shown in Figure 2. The architecture we adopt Hadoop file system1 (that is similar to Google file system) design philosophy and 1/2012 9th International Conference on Ubiquitous Intelligence and Computing and 9th International Conference on Autonomicand Trusted ComputingPeer to Peer (P2P) architecture to solve the mentioned problems. Due to the inherent characteristics of P2P and Hadoop file system, the proposed can naturally solve the problems mentioned above. We present the architecture, components, its operation flow, and depict the implementation issue in this paper. We believe that the architecture can improve significantly issues.The remainder of the paper is organized as follows. Section 2 surveys related works, especially on Hadoop, including its architecture and HDFS, and some surveillance system related works. Section 3 describes the proposed architecture, including Video Recording and Video Monitoring, as well as explaining the operation flow. Section 4 describes its implement issues. Finally we give a brief concluding remarks and feature work in Section 6.II.B ACKGROUND AND RELATED WORKłįHadoopThe Apache Hadoop [3][4] is a framework that allows distributed processing for large data sets across clusters of computers using a simple programming model. Hadoop is built up by two important parts, Mapreduce and Hadoop File System (HDFS) [5].In the Hadoop File System (HDFS), it provides global access to files in the cluster and is implemented by two kinds of node; the Name Node and the Data Node.The Name Node manages the all metadata of file system, while Data Node stores actual data.HDFS uses commodity hardware to distribute the system loading with characteristics of fault tolerance scalability and expandability.I n the HDFS, the NameNode is responsible for maintaining the HDFS directory tree, and is a centralized service. The NameNode execute the base system operation, just like renaming, opening, closing and others. NameNode also determines the mapping table for each block in the DataNode. The DataNode directly accesses the file. I t can serve reading and writing requests from the client.HDFS is designed to maintain and store large file data.I n HDFS, each file will be split into one or more small blocks, and those blocks are allowed to store in a set of DataNodes [6]. That means it will store each file as a sequence of blocks, which are expectedly split as the same size but not the last one. For the fault tolerance, each blocks of a file are replicated and by default, two backups of each block are stored by different DataNodes in the same rack and a third one is stored on a DataNode in a different rack. The NameNode decides the location of replication. The placement chosen for replica is to improve the reliability and performance.ŃįSurveillance SystemI n the [14], authors presented a layered generic organizational suite for the development of Sensor Management Framework (SMF) based on the service-oriented architecture. The sensor management system is studied from a layered perspective and the functional tasks carried by the SMF are categorized into three categories; sensor management, network management, and system management.I n the [15], authors proposed a design of large scale video surveillance system client based on p2p streaming. Given the particularity of video surveillance, such as high churn and the heterogeneity of user access network and delay-tolerant, authors also constructed the end-hosts into mesh application layer topology and adopt pull-push mode to deliver the data. Authors also designed the architecture of PPClient and gave details of its components. Through simulation and real system test it shows it is feasibility. PPClient conquered traditional server/client mode, which needs large number of infrastructure to support tens of thousands users. By using p2p streaming technology, users act as consumers and at the same time they provide service to other users. Then the system’s scalability is enhanced.I n the [10], authors proposed an overlay network architecture by application layer multicast with load balancing scheme to effectively provide ubiquitous video surveillance service. The proposed approach can provide I nternet users with scalable and stable surveillance video streaming, since the simulations and results demonstrate that our improved optimization of load balancing via both averaging bandwidth and life time has better performance in overhead of control message, service disruption, and tree depth than the other optimization criterion.I n this paper, we apply the data placement concept of ʼnadoop file system to provide fault tolerant and efficient video access and apply P2P technology to improve the scalability, reliability, robust, and server cost. Therefore, integrating both ʼnadoop concept and P2P technology can solve many issues of surveillance system.III.S YSTEM D ESIGNThis section will depict the design philosophy of the proposed architecture and explain how it can solve the problems mentioned in Section 1.łįSystem ArchitectureWe exploit Hadoop concept to design our system. The proposed system has two kinds of node. One is Directory Node (DN) which is responsible for managing all FEs, but does not keep all video data. The whole system can have multiple DNs. To simplify system architecture and its explanation, we assume the system only has one DN and it is deployed in administration department. The other is Peer Node (PN) which is responsible for storing the video datausing P2P technology. I n the Hadoop concept, a piece ofdata generally has three replicas. Therefore, each FE’s videodata in the architecture can be stored into three PNs; one iscalled Primary PN (P-PN) that of course is the node FE tiedup, and another two nodes are called Secondary PN (S-PN)that are selected by DN using distributed hashing table(DHT), as shown in Figure 2. In other words, the video dataof the FE in the P-PN is replicated to two S-PNs that areselected and managed by the DN. The three nodes naturallyform a replication group (RG).The concept of RG can be represented as Figure 2. Thevideo data of a PN (P-PN) can be replicated to any two PNs(S-PN). The two S-PNs are selected by the DN using P2Ptechnology.Figure 2: Replication GroupŃįComponents and FunctionalityAs well known, a surveillance system can be dividedinto two operation modes; one is Video Recording, and theother mode is Video Monitoring. We depict the componentsand their functionality according to the two modes.Video Recording is responsible for handling the videodata storing. The architecture is shown in Figure 3.•Directory Node(DN): The node provides the centralized directory services. I t contains followingcomponents: Auth enticator Module (AM),ReplicaManager (RM),Replica Sch eduler (RS), and a DNDatabase for the directory of whole system. I n theDN, a RM manages each PN and selects properlyreplicas for each FE of PN through RS. Every PN candirectly communicate with Replica Manager of DN.The Authentication Module is responsible forchecking the authentication of any PN as well asassigning authentication key to both P-PN andassociated S-PNs. The Replica Scheduler selects twoproper S-PNs for a P-PN according to P2P technology. I t will search for S-PNs. Directory NodeDatabase is to keep the information of every peernode.•Primary Peer Node (P-PN): The Primary Peer Node gets video data from FE directly. Therefore, it consistsof an FE (Camera or CCD), persistent storage, andthree extra components: Video Dispatch er,ReplicaManager and Auth entication Module, respectively.Video Dispatcher is responsible for transmitting andstoring video data into its RG currently. n otherwords, The Video Dispatcher will store the caughtvideo into local storage and deliver it to two replicas(S-PNs). The operation is similar with the writeoperation of Hadoop file system. The RM is tocommunicate with the RM of DN and other PNs forauthentication and getting associated information of S-PN. For example, it will get the location associated toS-PN from the DN, and try to build communicationchannel with them.AM stores all keys obtained fromDN and private information of its associated RGs.Figure 3: System Architecture•Secondary Peer Node (S-PN): Undoubtedly, an S-PN of a RG is also a P-PN of another RG. Therefore, allcomponents and their functionality are identical to theP-PN excepting for the operation flows. We willexplain their operations in next subsection.Such design obviously can have following advantages. First, the scalability can be improved because the data center is not a bottleneck yet. All video data of the system are stored into each PN and required bandwidth of data center can be significantly reduced. Secondly, the reliability can be enhanced due to replication mechanism used in the design (every video data is stored into a RG; one P-PN and two S-PNs). While the P-PN or a replicated S-PN failed, the DN can select another PN to take over the fail. Thirdly, the costof data center also can be reduced. Although it will alsoincrease the cost of individual PN, we think that is trivial comparing with expensive data center. Besides, the robustness of the system can be guaranteed because a PN failure will not affect the service of the system.The other operation mode- Video Monitoring, is designed for client to retrieve video data. The operation can be initiated by a client, namely Client Monitoring (CM), who is assumed that is a special PN has the same components; Video Dispatcher, Replica Manager and Authentication Module. These components can be run on desktop or PC. While the CM wants to retrieve desired video, it can retrieve from all replicas in parallel, which like P2P approach or [12]. Such design can be more efficiently retrieving video than from a centralized data center.n summary, the proposed architecture can naturally solve the problems mentioned in Section 1.ńįOperation FlowThis section presents the operation flow for video recording and monitoring. First the video recording is presented and the details are shown in Figure 4.In general, an activatied PN needs to register itself to DN for future management. The registration message is sent by RM to the RM of DN.The message includes some private data about the node, such as Node I D, address, hardware information, authentication key, and so on; and it will be stored into AM of DN. While the registration is completed, the DN will reply the registration back to the PN. The PN can then send a request for find suitable S-PNs from DN for delivering replicated video data.Figure 4: Operation Flow of Video RecordingThe DN will check the states of all PNs to find available PNs for replicating video data. The peer node states contain peer node’s Replica state, its bandwidth, connection stability and peer node group. If available PN can be found and the number is enough, the DN will select suitable S-PN using DHT function. And then the DN generates an authentication key and sends it to chosen S-PN for the request and for future request messages from P-PN(PN of initiating the request).Next, the DN replys the P-PN the node’s information of S-PNs; so that the P-PN can create communication channel to S-PNs according to the node’s information and authentication key. Finally the P-PN can store video data to local storage and deliver the to the S-PNs.In the design, we hope that the client can be any device and at any where. Therefore, a client need to get the authentication key in each video monitoring request. Following describes the steps of video monitoring, the details are shown as in Figure 5.Figure 5: Operation flow of video monitoringWhile a client wants to access his/her own video data, he/she can send a request with account and password to DN. The DN will check its legality by inquiring AM for the request. If the client is authorized, the DN first reply client the request and then later send an authentication key for access video data from RG. In the meantime, the RM of DN will get the authentication key from AM and all states of the PNs in the RG. After client receives the authentication key, it can create communication channels with authentication key to all nodes of the RG. If the request is authorized by allnodes, he/she can get the desired video data from all nodes (three replicas) of the RG in parallel. Obviously, the design can improve the performance of video access.Of course, client can get equally video data size from each replica. Due to the bandwidth variance of PNs, each replica’s bandwidth may be difference. Therefore, we can apply adaptive algorithm [12] to dynamically adjust video data size form each replica according to some criteria, such as bandwidth, connection stability, distance, and PN’s computing capability etc.IV.I MPLEMENTATION I SSUESAccording to the design, we depict some implementation issues in this section. In general, a PN node can be implemented using an embedded system with embedded Linux OS.łįPeer Node StateHere, we present some information and states inside a PN used for PN selection and video data access. The information comprises of peer node state contains the Unique I D, the peer node group, bandwidth, peer node’s replica state, authentication key and authorized state, as follows:•Unique ID (UID): Every PN has a unique ID in the System, and they are recoded in the database ofDN.•Group ID (GID) of Peer Node: It is the ID of RG, which identifies the group of replication. The PeerNodes have the same replica will be grouped in thesame group; so that they have the same GID. If aPN has N replicas, it will maintain N GID s foreach group.•Bandwidth: Each peer node may have different bandwidth in different situation. It can be used forcalculating the transmitting size of video datawhile client asks for his own video.•Peer Node’s Replica State: The state indicates the PN is available for replicating other PN’s video. If“Yes”, it means that it has room for doing so.•Authorized State: The peer node will store the Authorized Key from DN. Each key is mapped toa PN for further access, and it also recodes therelationship with the peer node. DN will check thisstate for the stability of connection between thesame replicas.ŃįThe PNs Scheduler in Video RecordingWe utilize the same replication scheme with Hadoop-like file system to store video data. When a PN registers itself into the DN, it will be grouped together with other PNs (replicas) using our PN scheduling algorithm that provides a lookup service which according to the Peer Node’s storage space state and bandwidth. I n the Hadoop, a file will have three replicas. Therefore, we utilize this algorithm to select two S-PNs for a certain P-PN.Figure 6: PN scheduling algorithmThe algorithm of the Replica Scheduler can be found in Figure 6. The algorithm firstly executes unFullPNList()to get a PNList (PeerNode List) that maintains the registered PN which is available for replicating other video data in the system. And then sorts the list by executing ReplicaStateSort() to sort the list according to the storage space of PN. The part of the same storage space in the list, we will sort again by executing BandwidthSort() according to the bandwidth of PN.In order to balance the load, we first try to find an S-PN from the member of PNlist(PeerNode List). I f we cannot find any PN to serve the replication, we then try the next peer node in the list.T ABLE1:S YSTEM A NALYSISAccording to the different design for the system, they have different advantage. We compare the SMF [14], PPClient [15], UVS [10] and our P2PCloud. SMF is hard to join new sensor node into the system, but it robustness is strong. PPClinet has higher scalability, but the video of each Media Distribution Subsystem doesn’t make any replicas toavoid the error occur. UVS utilize UVSMON tree rotatewhen the new node join or broken, but the system frequently revise is not good for robustness. The SMF and UVS are centralized storage, but PPClient and P2PCloud are distributed storage. P2PCloud has higher scalability, fault tolerant and robustness, but lower bandwidthV.C ONCLUSION AND F UTURE W ORKI n this paper, we have proposed an architecture for video surveillance service by integrating P2P and hadoop-like file system technology. Adapting P2P is used for connecting with each PN and storing video data to replicas.I t can improve scalability, cost and efficiency, while Hadoop is to improve reliability and efficiency. We presented the system design, components and their functionality, operation flows, and implementation issues. According to our explanation and analysis, it is obviously that such design can improve some issues; such as scalability, reliability, robust, efficiency, and cost.I n the future, we want to implement the system to various embedded platform; and turn and evaluate the performance of the system.A CKNOWLEDGEMENTThis work was supported by the Nation Science Council of Republic of China under Grant No. NSC 100-2221-E-305-013.R EFERENCE:[1]H. Dias, J. Rocha, P. Silva, C. Leao, and L.P. Reis, “DistributedSurveillance System”, Proc. of the Portuguese Conf. on ArtificialIntelligence, Covilha, Portugal, 5-8 Dec. 2005[2]X. Cao, Z. Wang, R. Hu, and J.Chen , “Distributed VideoSurveillance System Based on Overlay Network,” I n Proc. I EEEFuture Generation Communication and Networking (FGCN’07),pp.368-373, 6-8 Dec. 2007[3]Hadoop. /, 2012.[4] D. Borthakur. “The hadoop distributed file system: Architecture anddesign”, Hadoop Project Website, 2007.[5]HDFS (hadoop distributed file system) architecture./common/docs/r1.0.2/#HDFS, 2012. [6]J. Shafer, S. Rixner, and A. L. Cox. “The Hadoop DistributedFilesystem: Balancing Portability and Performance”, In Proceedingsof the 2010 I EEE I nternational Symposium on PerformanceAnalysis of Systems and Software (ISPASS’10), pp. 122–133, 2010.[7]Michael Bramberger, Andreas Doblander, Arnold Maier, BernhardRinner, Helmut Schwabach, “Distributed Embedded Smart Cameras for Surveillance Applications”, Computer, vol. 39, no. 2,pp. 68-75, Feb. 2006.[8]L.F. Marcenaro et al.,“Distributed Architectures and Logical-TaskDecomposition in Multimedia Surveillance Systems,” Proc. I EEE,pp.1419-1440, Oct. 2001.[9]Y.Hongyun, H.Ruiming, C.Jun. “Design and I mplementation ofLarge-scale Distributed Video Surveillance System”, the ThirdI nternational Conference on Computer Science & Education(ICCSE'2008), Kaifeng, China, May 20,2008.[10]Chia-Hui Wang, Haw-Yun Shin, Wu-Hsiao Hsu. “Load-sharingoverlay network design for ubiquitous video surveillance services”,International Conference on Ultra Modern Telecommunications &Workshops, 12-14 Oct. 2009, pp. 1-7.[11]Whitman, D. “The need and capability of a Surveillance DataDistribution System”, I ntegrated Communications, Navigation andSurveillance Conference, 2009. I CNS '09, pp. 1 – 6, 13-15 May2009.[12]Yue-Shan Chang, Guo-Jie Zou, Ching-lung Chang “RARS: AResource-Aware Replica Selection and co-allocation scheme forMobile Grid,” I nternational Journal of Ad Hoc and UbiquitousComputing, Vol. 6, No. 2, 2010, pp. 99-113.[13]P. Maymounkov and D. Mazieres, “Kademlia: A peer-to-peerinformation system based on the xor metric”, I n Proceedings ofIPTPS02, Cambridge, USA, Mar. 2002.[14] A.R. Hilal, A. Khamis, and O. Basir, ”A Service-OrientedArchitecture Suite for Sensor Management in Distributed Surveillance Systems,“ 2011 International Conference on Computerand Management (CAMAN), 2011, pp. 1 – 6.[15]Xun Zhu, Hongtao Deng, Zheng Chen, Hongyun Yang “Design ofLarge-Scale Video Surveillance System Based on P2P Streaming,”2011 3rd I nternational Workshop on I ntelligent Systems andApplications (ISA), 28-29 May 2011, pp. 1 – 4.。