车牌识别英文文献2翻译
车牌识别外文文献翻译中英文

外文文献翻译(含:英文原文及中文译文)文献出处:Gao Q, Wang X, Xie G. License Plate Recognition Based On Prior Knowledge[C]// IEEE International Conference on Automation and Logistics. IEEE, 2007:2964-2968.英文原文License Plate Recognition Based On Prior KnowledgeQian Gao, Xinnian Wang and Gongfu XieAbstract - In this paper, a new algorithm based on improved BP (back propagation) neural network for Chinese vehicle license plate recognition (LPR) is described. The proposed approach provides a solution for the vehicle license plates (VLP) which were degraded severely. What it remarkably differs from the traditional methods is the application of prior knowledge of license plate to the procedure of location, segmentation and recognition. Color collocation is used to locate the license plate in the image. Dimensions of each character are constant, which is used to segment the character of VLPs. The Layout of the Chinese VLP is an important feature, which is used to construct a classifier for recognizing. The experimental results show that the improved algorithm is effective under the condition that the license plates were degraded severely.Index Terms - License plate recognition, prior knowledge, vehiclelicense plates, neural network.I. INTRODUCTIONV ehicle License-Plate (VLP) recognition is a very interesting but difficult problem. It is important in a number of applications such as weight-and-speed-limit, red traffic infringement, road surveys and park security [1]. VLP recognition system consists of the plate location, the characters segmentation, and the characters recognition. These tasks become more sophisticated when dealing with plate images taken in various inclined angles or under various lighting, weather condition and cleanliness of the plate. Because this problem is usually used in real-time systems, it requires not only accuracy but also fast processing. Most existing VLP recognition methods [2], [3], [4], [5] reduce the complexity and increase the recognition rate by using some specific features of local VLPs and establishing some constrains on the position, distance from the camera to vehicles, and the inclined angles. In addition, neural network was used to increase the recognition rate [6], [7] but the traditional recognition methods seldom consider the prior knowledge of the local VLPs. In this paper, we proposed a new improved learning method of BP algorithm based on specific features of Chinese VLPs. The proposed algorithm overcomes the low speed convergence of BP neural network [8] and remarkable increases the recognition rate especially under the condition that the license plate images were degrade severely.II. SPECIFIC FEA TURES OF CHINESE VLPSA. DimensionsAccording to the guideline for vehicle inspection [9], all license plates must be rectangular and have the dimensions and have all 7 characters written in a single line. Under practical environments, the distance from the camera to vehicles and the inclined angles are constant, so all characters of the license plate have a fixed width, and the distance between the medium axes of two adjoining characters is fixed and the ratio between width and height is nearly constant. Those features can be used to locate the plate and segment the individual character. B. Color collocation of the plateThere are four kinds of color collocation for the Chinese vehicle license plate .These color collocations are shown in table I.TABLE IMoreover, military vehicle and police wagon plates contain a red character which belongs to a specific character set. This feature can be used to improve the recognition rate.C. Layout of the Chinese VLPSThe criterion of the vehicle license plate defines the characters layout of Chinese license plate. All standard license plates contain Chinese characters, numbers and letters which are shown in Fig.1. The first one is a Chinese character which is an abbreviation of Chineseprovinces. The second one is a letter ranging from A to Z except the letter I. The third and fourth ones are letters or numbers. The fifth to seventh ones are numbers ranging from 0 to 9 only. However the first or the seventh ones may be red characters in special plates (as shown in Fig.1). After segmentation process the individual character is extracted. Taking advantage of the layout and color collocation prior knowledge, the individual character will enter one of the classes: abbreviations of Chinese provinces set, letters set, letters or numbers set, number set, special characters set.(a)Typical layout(b) Special characterFig.1 The layout of the Chinese license plateIII. THE PROPOSED ALGORITHMThis algorithm consists of four modules: VLP location, character segmentation, character classification and character recognition. The main steps of the flowchart of LPR system are shown in Fig. 2.Firstly the license plate is located in an input image and characters are segmented. Then every individual character image enters the classifier to decide which class it belongs to, and finally the BP network decides which character the character image represents.A. Preprocessing the license plate1) VLP LocationThis process sufficiently utilizes the color feature such as color collocation, color centers and distribution in the plate region, which are described in section II. These color features can be used to eliminate the disturbance o f the fake plate ’ s regions. The flowchart of the plate location is shown in Fig. 3.Fig.3 The flowchart of the plate location algorithmThe regions which structure and texture similar to the vehicle plate are extracted. The process is described as followed:Here, the Gaussian variance is set to be less than W/3 (W is the character stroke width), so 1P gets its maximum value M at the center of the stroke. After convolution, binarization is performed according to a threshold which equals T * M (T<0.5). Median filter is used to preserve the edge gradient and eliminate isolated noise of the binary image. An N * N rectangle median filter is set, and N represents the odd integer mostly close to W.Morphology closing operation can be used to extract the candidate region. The confidence degree of candidate region for being a license plate is verified according to the aspect ratio and areas. Here, the aspect ratio is set between 1.5 and 4 for the reason of inclination. The prior knowledge of color collocation is used to locate plate region exactly. The locating process of the license plate is shown in Fig. 4.2) Character segmentationThis part presents an algorithm for character segmentation based on prior knowledge, using character width, fixed number of characters, the ratio of height to width of a character, and so on. The flowchart of the character segmentation is shown in Fig. 5.Firstly, preprocess the license the plate image, such as uneven illumination correction, contrast enhancement, incline correction and edge enhancement operations; secondly, eliminating space mark which appears between the second character and the third character; thirdly, merging the segmented fragments of the characters. In China, all standard license plates contain only 7 characters (see Fig. 1). If the number of segmented characters is larger than seven, the merging process must be performed. Table II shows the merging process. Finally, extracting the individual character’ image based on the number and the width of the character. Fig. 6 shows the segmentation results. (a) The incline and broken plate image, (b) the incline and distort plate image, (c)the serious fade plate image, (d) the smut license plate image.where Nf is the number of character segments, MaxF is the number of the license plate, and i is the index of each character segment.The medium point of each segmented character is determined by:(3)where 1i Sis the initial coordinates for the character segment, and 2i S is thefinal coordinate for the character segment. The d istance between two consecutive medium points is calculated by:(4)Fig.6 The segmentation resultsB. Using specific prior knowledge for recognitionThe layout of the Chinese VLP is an important feature (as described in the section II), which can be used to construct a classifier for recognizing. The recognizing procedure adopted conjugate gradient descent fast learning method, which is an improved learning method of BP neural network[10]. Conjugate gradient descent, which employs a series of line searches in weight or parameter space. One picks the first descent direction and moves along that direction until the minimum in error is reached. The second descent direction is then computed: this direction the “ conjugate direction” is the one along which the gr adient does not change its direction will not “ spoil ” the contribution from the previous descent iterations. This algorithm adopted topology 625-35-N as shown in Fig. 7. The size of input value is 625 (25*25 ) and initial weights are with random values, desired output values have the same feature with the input values.As Fig. 7 shows, there is a three-layer network which contains working signal feed forward operation and reverse propagation of error processes. The target parameter is t and the length of network outputvectors is n. Sigmoid is the nonlinear transfer function, weights are initialized with random values, and changed in a direction that will reduce the errors.The algorithm was trained with 1000 images of different background and illumination most of which were degrade severely. After preprocessing process, the individual characters are stored. All characters used for training and testing have the same size (25*25 ).The integrated process for license plate recognition consists of the following steps:1) Feature extractingThe feature vectors from separated character images have direct effects on the recognition rate. Many methods can be used to extract feature of the image samples, e.g. statistics of data at vertical direction, edge and shape, framework and all pixels values. Based on extensive experiments, all pixels values method is used to construct feature vectors. Each character was reshaped into a column of 625 rows’ feature vector. These feature vectors are divided into two categories which can be used for training process and testing process.2) Training modelThe layout of the Chinese VLP is an important feature, which can be used to construct a classifier for training, so five categories are divided. The training process of numbers is shown in Fig. 8.As Fig. 8 shows, firstly the classifier decides the class of the inputfeature vector, and then the feature vector enters the neural network correspondingly. After the training process the optimum parameters of the net are stored for recognition. The training and testing process is summarized in Fig. 9.(a) Training process(b)Testing processFig.9 The recognition process3) Recognizing modelAfter training process there are five nets which were completely trained and the optimum parameters were stored. The untrained feature vectors are used to test the net, the performance of the recognition system is shown in Table III. The license plate recognition system is characterized by the recognition rate which is defined by equation (5).Recognition rate =(number of correctly read characters)/ (number of found characters) (5)IV. COMPARISON OF THE RECOGNITION RA TE WITH OTHER METHODSIn order to evaluate the proposed algorithm, two groups of experiments were conducted. One group is to compare the proposed method with the BP based recognition method [11]. The result is shown in table IV. The other group is to compare the proposed method with themethod based on SVM [12].The result is shown in table V. The same training and test data set are used. The comparison results show that the proposed method performs better than the BP neural network and SVM counterpart.V. CONCLUSIONIn this paper, we adopt a new improved learning method of BP algorithm based on specific features of Chinese VLPs. Color collocation and dimension are used in the preprocessing procedure, which makes location and segmentation more accurate. The Layout of the Chinese VLP is an important feature, which is used to construct a classifier for recognizing and makes the system performs well on scratch and inclined plate images. Experimental results show that the proposed method reduces the error rate and consumes less time. However, it still has a few errors when dealing with specially bad quality plates and characters similar to others. This often takes place among these characters (especially letter and number): 3—8 4—A 8—B D—0.In order to improve the incorrect recognizing problem we try to add template-based model [13] at the end of the neural network.中文译文基于先验知识的车牌识别Qian Gao, Xinnian Wang and Gongfu Xie摘要- 本文介绍了一种基于改进的BP(反向传播)神经网络的中国车牌识别(LPR)算法。
实时的车牌识别系统 中英文
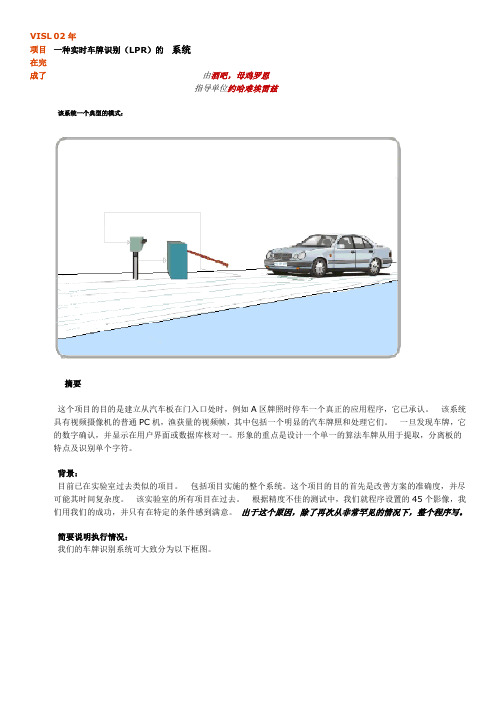
VISL 项目在完成了02年一种实时车牌识别(LPR)的系统由酒吧,母鸡罗恩指导单位约哈难埃雷兹该系统一个典型的模式:摘要这个项目的目的是建立从汽车板在门入口处时,例如A区牌照时停车一个真正的应用程序,它已承认。
该系统具有视频摄像机的普通PC机,渔获量的视频帧,其中包括一个明显的汽车牌照和处理它们。
一旦发现车牌,它的数字确认,并显示在用户界面或数据库核对一。
形象的重点是设计一个单一的算法车牌从用于提取,分离板的特点及识别单个字符。
背景:目前已在实验室过去类似的项目。
包括项目实施的整个系统。
这个项目的目的首先是改善方案的准确度,并尽可能其时间复杂度。
该实验室的所有项目在过去。
根据精度不佳的测试中,我们就程序设置的45个影像,我们用我们的成功,并只有在特定的条件感到满意。
出于这个原因,除了再次从非常罕见的情况下,整个程序写。
简要说明执行情况:我们的车牌识别系统可大致分为以下框图。
框图全球系统。
另外这个进程可以被看作是减少或地方的牌照抑制有害信息从携带信息的信号,这里是一个视频序列包含大量无关信息的特点,形式抽象符号的研究。
光学字符识别(OCR)已采用神经网络技术,采用神经元在输出层的前馈网络的3层,200个神经元在20输入层,中间神经元在10层,。
我们保留了神经网络数据集图像用在项目的先例,其中包括238位第我们的算法的详细步骤说明如下图:框图程序的子系统。
这里介绍捕获帧的一个给定的产出上面所述的主要步骤:示例捕获帧黄色区域捕获的帧过滤捕获帧地区扩张黄色车牌区域确定氡角度的变换板的使用改进的LP地区调整唱片轮廓-列和图调整唱片轮廓-线条和图唱片作物灰度唱片唱片二值化,均衡使用自适应阈值二进制唱片归唱片确定使用的LP水平轮廓图像总和先决行归唱片轮廓调节字符分割使用的山峰到山谷方法扩张型数位影像调整数字图像水平轮廓-线和图调整的数字图像轮廓调整大小的数字图像OCR的数字识别的神经网络方法工具该方案实施开发了基于Matlab。
车牌识别毕业论文
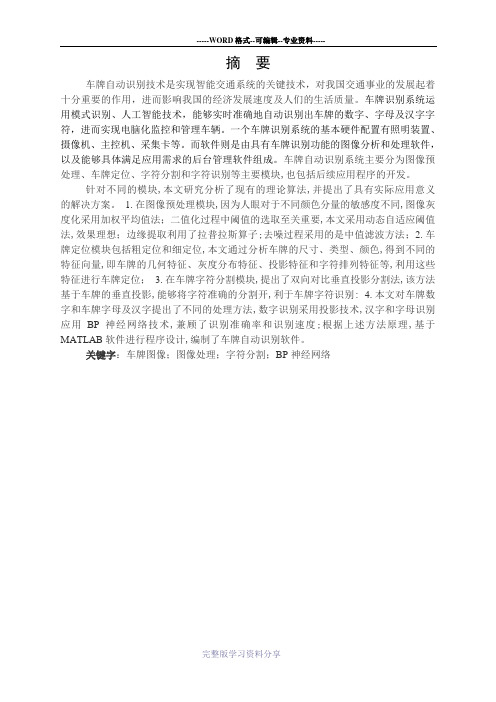
摘要车牌自动识别技术是实现智能交通系统的关键技术,对我国交通事业的发展起着十分重要的作用,进而影响我国的经济发展速度及人们的生活质量。
车牌识别系统运用模式识别、人工智能技术,能够实时准确地自动识别出车牌的数字、字母及汉字字符,进而实现电脑化监控和管理车辆。
一个车牌识别系统的基本硬件配置有照明装置、摄像机、主控机、采集卡等。
而软件则是由具有车牌识别功能的图像分析和处理软件,以及能够具体满足应用需求的后台管理软件组成。
车牌自动识别系统主要分为图像预处理、车牌定位、字符分割和字符识别等主要模块,也包括后续应用程序的开发。
针对不同的模块,本文研究分析了现有的理论算法,并提出了具有实际应用意义的解决方案。
1.在图像预处理模块,因为人眼对于不同颜色分量的敏感度不同,图像灰度化采用加权平均值法;二值化过程中阈值的选取至关重要,本文采用动态自适应阈值法,效果理想;边缘提取利用了拉普拉斯算子;去噪过程采用的是中值滤波方法;2.车牌定位模块包括粗定位和细定位,本文通过分析车牌的尺寸、类型、颜色,得到不同的特征向量,即车牌的几何特征、灰度分布特征、投影特征和字符排列特征等,利用这些特征进行车牌定位;3.在车牌字符分割模块,提出了双向对比垂直投影分割法,该方法基于车牌的垂直投影,能够将字符准确的分割开,利于车牌字符识别: 4.本文对车牌数字和车牌字母及汉字提出了不同的处理方法,数字识别采用投影技术,汉字和字母识别应用BP神经网络技术,兼顾了识别准确率和识别速度;根据上述方法原理,基于MATLAB软件进行程序设计,编制了车牌自动识别软件。
关键字:车牌图像;图像处理;字符分割;BP神经网络AbstractLicense plate recognition technology is to realize the key technology of intelligent transportation system of our country, the development of the cause of traffic plays a very important role, then affects the economic development of our country and speed and people's quality of life. License plate recognition system with pattern recognition, artificial intelligence technology, to real-time accurately recognize the license plate number of automatic, letters and Chinese characters, and achieve computerized monitoring and management vehicles. A license plate recognition system of basic hardware configuration have lighting devices, video camera, master control machine, acquisition card, etc. And software is with license plate identification function by the image analysis and processing software, and can meet the demand of the specific application background management software component. License plate recognition system mainly divided into the image preprocessing, license plate location, character segment and character recognition and other major modules, including the follow-up application development.In view of the different module, this paper analyzed the existing algorithm theory, and puts forward the practical significance of the solution. 1. In the image preprocessing module, for the human eye to different color the sensitivity of the component is different, the image intensity by weighted average method; In the process of binary of the threshold is very important to select is adopted in this paper, dynamic adaptive threshold value method, the effect ideal; Using the Laplace operator edge extraction; Denoising the process is the median filtering method; 2. The license plate localization module contains coarse position and fine positioning, the paper analyzes the license plate size, type, color, get different characteristic vector, namely the geometrical characteristics of the license plate, gray distribution, projection characteristics and characters arrangement characteristics, use these characteristics of the license plate location; 3. In the license plate character segmentation module, and put forward the two-way contrast vertical projection segmentation method, this method is based on the license plate vertical projection, can make the character of accurate separated, beneficial to the license plate character recognition: 4. This article on license plate Numbers and letters and characters put forward different processing methods, number recognition by projection technology, Chinese characters and letters recognition application BP neural network technology, and taking account of the identification accuracy and recognition rate; According to the above method, based on the MATLAB software program design, compiled the license plate recognition software.Keywords License plate image, image processing, character segment, the BP neural network目录摘要............................................. 错误!未定义书签。
车辆牌照字符识别2
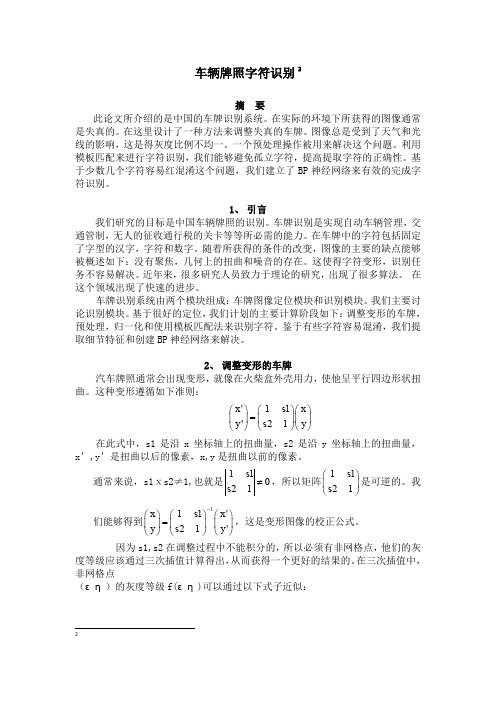
车辆牌照字符识别2摘 要此论文所介绍的是中国的车牌识别系统。
在实际的环境下所获得的图像通常是失真的。
在这里设计了一种方法来调整失真的车牌。
图像总是受到了天气和光线的影响,这是得灰度比例不均一。
一个预处理操作被用来解决这个问题。
利用模板匹配来进行字符识别,我们能够避免孤立字符,提高提取字符的正确性。
基于少数几个字符容易红混淆这个问题,我们建立了BP 神经网络来有效的完成字符识别。
1、 引言我们研究的目标是中国车辆牌照的识别。
车牌识别是实现自动车辆管理,交通管制,无人的征收通行税的关卡等等所必需的能力。
在车牌中的字符包括固定了字型的汉字,字符和数字。
随着所获得的条件的改变,图像的主要的缺点能够被概述如下:没有聚焦,几何上的扭曲和噪音的存在。
这使得字符变形,识别任务不容易解决。
近年来,很多研究人员致力于理论的研究,出现了很多算法。
在这个领域出现了快速的进步。
车牌识别系统由两个模块组成:车牌图像定位模块和识别模块。
我们主要讨论识别模块。
基于很好的定位,我们计划的主要计算阶段如下:调整变形的车牌,预处理,归一化和使用模板匹配法来识别字符。
鉴于有些字符容易混淆,我们提取细节特征和创建BP 神经网络来解决。
2、 调整变形的车牌汽车牌照通常会出现变形,就像在火柴盒外壳用力,使他呈平行四边形状扭曲。
这种变形遵循如下准则: '11'21x s x y s y ⎛⎫⎛⎫⎛⎫= ⎪ ⎪⎪⎝⎭⎝⎭⎝⎭在此式中,s1是沿x 坐标轴上的扭曲量,s2是沿y 坐标轴上的扭曲量,x ’,y ’是扭曲以后的像素,x,y 是扭曲以前的像素。
通常来说,s1ⅹs2≠1,也就是11021s s ≠,所以矩阵1121s s ⎛⎫ ⎪⎝⎭是可逆的。
我们能够得到111'21'x s x y s y -⎛⎫⎛⎫⎛⎫= ⎪ ⎪ ⎪⎝⎭⎝⎭⎝⎭,这是变形图像的校正公式。
因为s1,s2在调整过程中不能积分的,所以必须有非网格点,他们的灰度等级应该通过三次插值计算得出,从而获得一个更好的结果的。
汽车车牌识别系统毕业论文(带外文翻译)解析

汽车车牌识别系统---车牌定位子系统的设计与实现摘要汽车车牌识别系统是近几年发展起来的计算机视觉和模式识别技术在智能交通领域应用的重要研究课题之一。
在车牌自动识别系统中,首先要将车牌从所获取的图像中分割出来实现车牌定位,这是进行车牌字符识别的重要步骤,定位的准确与否直接影响车牌识别率。
本次毕业设计首先对车牌识别系统的现状和已有的技术进行了深入的研究,在此基础上设计并开发了一个基于MATLAB的车牌定位系统,通过编写MATLAB文件,对各种车辆图像处理方法进行分析、比较,最终确定了车牌预处理、车牌粗定位和精定位的方法。
本次设计采取的是基于微分的边缘检测,先从经过边缘提取后的车辆图像中提取车牌特征,进行分析处理,从而初步定出车牌的区域,再利用车牌的先验知识和分布特征对车牌区域二值化图像进行处理,从而得到车牌的精确区域,并且取得了较好的定位结果。
关键词:图像采集,图像预处理,边缘检测,二值化,车牌定位ENGLISH SUBJECTABSTRACTThe subject of the automatic recognition of license plate is one of the most significant subjects that are improved from the connection of computer vision and pattern recognition. In LPSR, the first step is for locating the license plate in the captured image which is very important for character recognition. The recognition correction rate of license plate is governed by accurate degree of license plate location.Firstly, the paper gives a deep research on the status and technique of the plate license recognition system. On the basis of research, a solution of plate license recognition system is proposed through the software MATLAB,by the M-files several of methods in image manipulation are compared and analyzed. The methods based on edge map and das differential analysis is used in the process of the localization of the license plate,extracting the characteristics of the license plate in the car images after being checked up for the edge, and then analyzing and processing until the probably area of license plate is extracted,then come out the resolutions for localization of the car plate.KEY WORDS:imageacquisition,image preprocessing,edge detection,binarization,licence,license plate location目录前言 (1)第1章绪论 (2)§1.1 课题研究的背景 (2)§1.2 车牌的特征 (2)§1.3 国内外车辆牌照识别技术现状 (3)§1.4车牌识别技术的应用情况 (4)§1.5 车牌识别技术的发展趋势 (5)§1.6车牌定位的意义 (6)第2章MATLAB简介 (7)§2.1 MATLAB发展历史 (7)§2.2 MATLAB的语言特点 (7)第3章图像预处理 (10)§3.1 灰度变换 (10)§3.2 图像增强 (11)§3. 3 图像边缘提取及二值化 (13)§3. 4 形态学滤波 (18)第4章车牌定位 (21)§4.1 车牌定位的主要方法 (21)§4.1.1基于直线检测的方法 (22)§4.1.2 基于阈值化的方法 (22)§4.1.3 基于灰度边缘检测方法 (22)§4.1.4 基于彩色图像的车牌定位方法 (25)§4.2 车牌提取 (26)结论 (30)参考文献 (31)致谢 (33)前言随着交通问题的日益严重,智能交通系统应运而生。
汽车牌照识别系统的车牌定位技术研究外文资料翻译(适用于毕业论文外文翻译+中英文对照)

建立一个自动车辆车牌识别系统车辆由于数量庞大的抽象,现代化的城市要建立有效的交通自动系统管理和调度.最有用的系统之一是车辆车牌识别系统,它能自动捕获车辆图像和阅读这些板块的号码在本文中,我们提出一个自动心室晚电位识别系统,ISeeCarRecognizer,阅读越南样颗粒在交通费的注册号码.我们的系统包括三个主要模块:心室晚电位检测,板数分割和车牌号码识别。
在心室晚电位检测模块,我们提出一个有效的边界线为基础Hough变换相结合的方法和轮廓算法.该方法优化速度和准确性处理图像取自不同职位。
然后,我们使用水平和垂直投影的车牌号码分开心室晚电位分段模块.最后,每个车牌号码将被OCR的识别模块实现了由隐马尔可夫模型。
该系统在两个形象评价实证套并证明其有效性是适用于实际交通收费系统。
该系统也可适用于轻微改变一些其他类型的病毒样颗粒。
一.导言车牌识别的问题是一个非常有趣,且困难的一个问题.这在许多交通管理系统中是非常有用的。
心室晚电位识别需要一些复杂的任务,如车牌的检测,分割和识别。
这些任务变得更加复杂时,处理各种倾斜角度拍摄的图像或含有噪音的图像。
由于此问题通常是在实时系统中使用,它不仅需要准确性,而且要效率.大多数心室晚电位识别应用通过建立减少一些复杂的约束的位置和距离相机车辆,倾斜角度。
通过这种方式,车牌识别系统的识别率已得到明显改善.在此外,我们可以更准确地获得通过一些具体的当地样颗粒的功能,如字符数,行数在一板,或板的背景颜色或的宽度比为一板高。
二.相关工作心室晚电位的自动识别问题在20世纪90年代开始就有研究。
第一种方法是基于特征的边界线。
首次输入图像处理,以丰富的边界线的一些信息如梯度算法过滤器,导致在一边缘图像.这张照片是二值化处理,然后用某些算法,如Hough 变换,检测线。
最终,2平行线视为板候选人[4] [5]。
另一种方法是基于形态学[2]。
这种方法侧重于一些板块图像性质如亮度,对称,角度等。
英语作文中车牌号格式
英语作文中车牌号格式标题,The Format of Vehicle License Plate Numbers。
Introduction:Vehicle license plate numbers serve as uniqueidentifiers for vehicles around the world. Each country has its own format and regulations governing the structure of these numbers. In this essay, we will explore the different formats of vehicle license plate numbers and the significance behind their designs.1. The Structure of License Plate Numbers:Vehicle license plate numbers typically consist of a combination of letters and numbers arranged in a specific format. The structure varies from country to country, but there are some common elements. For example, in many countries, license plate numbers contain both letters and numbers, with the letters often representing the region orjurisdiction where the vehicle is registered.2. Examples of License Plate Formats:a. United States:In the United States, license plate numbers typically consist of a combination of letters and numbers. The format varies by state, but it often includes a combination of letters representing the state followed by numbers or a mix of numbers and letters. For example, in California, a license plate number might look like "ABC 1234", where "ABC" represents the state code and "1234" is a unique identifier.b. United Kingdom:In the United Kingdom, license plate numbers follow a specific format consisting of two letters, followed by two numbers, followed by three more letters. The first two letters represent the region where the vehicle is registered, the two numbers indicate the age ofthe vehicle, and the final three letters are randomly assigned. For example, a UK license plate number might appear as "AB12 CDE".c. China:In China, license plate numbers vary depending on the region where the vehicle is registered. In major cities like Beijing and Shanghai, license plates typically consist of a single letter followed by a series of numbers and then another letter. The first letter often represents the city or province, while the numbers are a unique identifier. For example, a license plate number in Beijing might be "京A12345", where "京" represents Beijing and "A" is a series code.3. Significance of License Plate Designs:The design of license plate numbers serves several purposes. Firstly, it allows for easy identification of vehicles by law enforcement officers, government agencies, and the general public. Secondly, it helps in regulatingand tracking vehicle registration and ownership. Additionally, the format of license plate numbers can have cultural or historical significance, reflecting regional identities or traditions.4. Conclusion:In conclusion, vehicle license plate numbers play a crucial role in identifying and regulating vehicles on the road. The format of these numbers varies by country, with each nation adopting its own system based on regulatory requirements and cultural norms. Understanding the structure and significance of license plate numbers provides insight into the complexities of vehicle registration and ownership worldwide.。
德汉汽车工程词典_车牌译名
附录 2 :车牌译名Acadiane阿卡迪恩Aska阿斯卡Accent爱可仙特Aspen白杨Accord阿科德,和睦Aspire希望Achieva成就Asti阿斯提Acron阿克朗Aston Martin阿斯通马丁Acty阿克泰Astra阿斯特拉Admiral海军大将Astro天体Aero bus空中客车Astron阿斯特朗Aero king空中之王Atlantique大西洋Aero medium迅达Atlas阿特拉斯Aero queen空中女皇Ato阿图Aerostar宇宙之星Atrai阿特雷Alero阿里罗Attante阿旦特Alfa Romeo阿尔法·罗密欧Attraction引力Alfasud阿尔法苏特Audi奥迪Alhambra阿尔汉布拉Auens奥恩斯Allante阿兰德Aurora奥罗拉Allegro阿莱格罗Auster南风Alliance结盟Austin Metro奥斯汀·地下铁道Almera阿美拉Avant阿万特Alpina阿尔卑斯Avella阿维拉Alpine阿尔平Avella Delta阿维拉·德尔塔Alto阿尔托Avenger复仇者Ambassador大使Avenir阿文尼尔Ami艾米Azure蓝天Amigo阿米戈Baleno百乐Anadol阿纳多尔Ballade叙事曲Angelia安琪利亚Balltt贝雷特Apollo阿波罗Bandeirant班带兰特Applause喝采Barchetta小风帆Arcadia阿卡迪亚Barina巴丽娜Ardenblan阿顿兰Basis基本型Argerta阿尔詹塔Bear熊Arise白羊座Beaufighter猛士Aristo贵族Beaulort博福特Armada阿玛达Beaver海狸Arosa阿瘳莎Bedford贝德福德Arrow利箭Bedford Contour贝德福德·康特Ascona阿斯科纳Beetle甲虫1048BellelBellel贝雷尔Calibra捷力Bentley本特里Camargue卡马克Beretta贝雷塔Camaro卡玛罗Berliet贝利埃Camira卡米拉Berlinetta伯林尼塔Campagnola野外Berling伯灵Camry卡姆利Beta贝塔Canter奔马Bighom彼虹Capella卡佩拉Bigua必佳Capital国都Bison野牛Cappuccino卡布西诺Biturlo比图尔拉Capri卡普里Blazer开辟者Caprice随想曲Blizzard狂风Caravan商队Bluebird青鸟Caravelle卡拉维尔,三桅帆Blues布鲁斯Cargo货运BMW宝马,巴依尔Carib卡利布Bocek鲍谢克Carina卡利那,船底座Bon Jovi帮·乔维Carisma凯斯马Bongo本格Carlton卡尔顿Bongo Brawny本格·布罗尼Caro卡罗Bongo Friendee本格·飞伦迪Carol颂歌Bonneville博纳维尔Carrera卡雷拉Bora北风Carry运载Boxer拳击家Catalina卡塔琳娜Boxster博克斯特Catera卡特拉Brasilia巴西利亚Catera凯帝Brava布拉瓦Cavalier骑士Bravada英勇者Cedric公子Bravo布拉沃Cefiro科费罗Break布瑞克Celebrity名人Breeze微风Celica赛利卡Brigadier准将Celson至尊Britania大英帝国,不列颠Century世纪Broadway百老汇,宽街Cerbera瑟伯拉Bronco烈马Cervo牡鹿Brooklands布罗克兰Challenger挑战者Brougham布劳汉姆Champ冠军Bruin熊先生Charade夏利,神奇Bulkmaster装载好手Charger战马Bulldog斗狗Chariot战车Caball卡布尔Charmant魅力Caballero骑士Chaser追击者Cabrio凯波Cherokee切诺基Cabstar卡布斯塔,出租车之星Cherry樱花Caddy凯迪Chevair雪维亚Calais卡莱斯Chevette雪维特Caldina卡尔蒂纳Chico驰高Dennis1049 Chieftain领袖Cordoba科多巴Chimaera驰马拉Corniche险途Cielo蓝天Corolla花冠Ciera赛拉Corona光冠Cima西玛Corona Mark光冠·马克Cimarron西马伦Corona Premio光冠·普雷米奥Cinquecento文艺中兴Corsa奔驰 ,柯尔萨Cinquecento Happy文艺中兴·快乐Corsica可喜佳Cinquecento Young文艺中兴·青春Cortina科蒂那Ciolo西耶罗Corvette克尔维特Cirrus卷云Cosmos宇宙City城市Cougar美洲狮City Golf城市高尔夫Countach库塔什Cityliner城市班车Country故土Civic市民Country squire乡绅Civic Shattle市民班车Courier信使Civilian老百姓Cressida克雷西达Clarus克拉鲁斯Cresta登峰Classic经典Crew科锐Clio克里奥(历史女神)Croma克罗马Clipper快马Crown皇冠Club俱乐部Crown Majesta皇冠·庄严Clubliner俱乐部班机Crown Victoria维多利亚皇冠Clubman社交家Cultus卡尔特斯Clydersdale辕马Cuore库雷Coaster巡游者Curren卡伦Color Line彩色线条Custom民俗Colt小马Custom Cruiser卡斯特姆巡洋舰Combo康宝Cutlass短剑Comet彗星Cutlass Calais加莱短剑Comfort舒坦Cutlass Ciera谢耶拉短剑Commander司令官Cutlass Supreme上方短剑Comodoro海军准将,科莫多罗Cynos赛诺斯,北极星Compact紧凑Daily戴利Concord协和Daimler戴姆勒Condor神鹰Dart标枪Conguese征服者Dasher冲击者Consorte伴侣Datsan达特桑Constamnia葡萄酒Daytona戴托那Consul领事De Ville帝威Contessa伯爵夫人Deaville迪维尔Continental大陆Debonair快乐Contour康拓Dedra德拉Contractor承包人Defender卫士Cooper库珀Delica德利卡Corcel科塞尔Delta德尔塔Cordia科迪尔Dennis丹尼斯1050DerbyDerby赛马Eterna埃特那Deseo帝索Etude练习曲Deutz道依茨Evasion埃娃申Diablo鬼魅式Exa艾可萨Diamante达马迪Excel优越Dino迪诺Exclusive高级Diplomat外交家Executive董事Discovery发现者Expedition远征Dodge道奇Explorer探险号Dogan多甘Express迅速Domani明天Fairlady贵夫人Dominator统治者Fairland佳人国Domingo多明各Fairlane菲尔兰,佳人巷Dorchester多切斯特Fairmont菲尔蒙特,佳人峰Dream梦幻Falcon猎鹰Drogmoler Corsair德勒克默勒·海盗Familia家族Drogmoler Meteor德勒克默勒·流星Fargo远行Drogmoler Supercomet德勒克默勒·超级Faster法斯特彗星Feeling飞羚Drogmoler Superpullman德勒克默勒·超Felicia费丽西亚级卧车Feligia菲利伽Duna杜纳Feroza费罗沙Durango都兰戈Ferrari费雷丽Duster风衣Festiva菲丝蒂娃Dyna戴那Fiat菲亚特Dynasty王朝Fiero菲罗Eclipse日蚀Fiesta节日Econoline伊克诺莱恩Fifth Avenue纽约第五街Edsel埃塞尔Fiore费雷EL Camino埃尔·卡米诺Fiorino菲欧丽诺Elantra埃尔察Firebird火鸟Eldorado埃尔多拉多Firenza佛罗伦萨Electra厄勒克特拉Fleetwood弗利特伍德Elefantino埃莱伐森Florian御马ELF埃尔夫Florian Aska御马·阿斯卡Elfflatlow埃尔夫拉特罗Focus佛克斯Elgrand埃尔格拉德Ford福特Elise爱丽丝Forester丛林居民Encore重奏Forever芙沃Enterprise骄傲Forma菲尔玛Escort护卫者Forte强音Espace太空Forward行进Espero伊斯比罗Fox狐狸Esprit精灵Freighter货运者Estate伊斯塔特Fronte前驱者Esteem伊斯腾Frontera前卫Estima艾斯蒂玛FTO运动者Kelleners1051 Fuego富果Himalayar喜马拉雅Fury芙利(复仇女神)Hippo河马Fusca福斯卡Homer信鸽Fuso扶桑Homy安乐Futura福图拉,将来Honcho老板Gacel加赛尔Hongqi红旗Galant华美Horizon地平线Galaxie银河Hunter猎人Gamma伽马Hyrax蹄兔Gazelle羚羊Ibiza伊比扎Gemini双子星座Iltis白鼬General大将Impala英帕拉Ghia Shutter吉亚·快门Imperial帝国Ghia Trio吉亚·三重奏Impreza翼豹Ghia Vignale吉亚·维格纳尔Impuise冲击Ghibli吉百利Infiniti无穷Giulietta吉里耶塔Inspire英斯皮尔Gli Faicon猎隼Integra英蒂格拉Gloria荣耀Intrepid猛士Gloria Cima荣耀·西玛Intrigue密谋Gol高尔Ipanema依帕内玛Golf高尔夫,海弯风Ipsum爱普松Gorkha戈尔卡Ital意大利人Granada格雷那达IVECO依维柯Grand Am大艾姆Jaguar美洲豹,杰戈娃Grand Caravan大商队Jalpa佳尔帕Grand Cherokee大切诺基Jazz爵士尔Grand Fury大芙利Jeep Eagle鹰牌吉普Grand GEVR农场Jelpa杰尔帕Grand Marquis老候爵Jetliner喷气客机Grand Move大行动Jetta捷达Grand Prix大奖Jimmy吉米Grand View展望Jimny吉姆尼Grand Voyager老航海家Journey旅游Grandeur雄伟Joy欢欣Grandtour远游Jubilant欢欣Griffith格力菲斯Jubilaum庆典GT Spyder GT ·斯皮特Jumbocruiser巨型军舰Guara夸拉Junior少年Guest来客Justy贾斯蒂Guilia吉利亚Kadett士官生Guy盖K? fer甲壳虫Gypsy吉普塞Kangoo卡菇H-Ace Commnter海-艾斯·康姆特Kapitan船长Hiace海艾斯Kappa卡帕Hijet海捷特Kartal卡塔尔Hilux希鲁克斯Kelleners凯尔诺斯1052KhamsinKhamsin热风Lotus荷花,劳图斯Kitten小猫Luce光Kommando科曼多,突击队Lucino卢齐诺Kompressor考姆莱斯Lumina罗米娜Korrado科拉多Lupo小狼,路波Kraft力量Lynx山猫,天猫座Kyalami卡拉米Lyra天琴座Lada拉达Maestro艺术大师Lagonda拉贡达Magna玛格纳Laguna拉古娜Magnum马格纳姆Lanborghini蓝宝基尼Malaga马拉加Lancer枪骑兵Malibu马利布Lancer Celeste蓝色枪骑兵Maluch马路驰Lancer Fiore枪骑兵·菲奥里Mammoth长毛象Lancia兰西亚Manta曼塔Landau四轮马力Maranello马拉内罗Landcruiser陆地巡洋舰Marathon马拉松Langley兰利Marbella马尔贝加Lanos兰瑙March行进Lantis兰蒂斯Marcia玛尔西亚Lantra兰特拉Marea马丽Largo拉戈Marina玛丽娜Laser激光Mark马克Laurel桂冠Marquis侯爵Laurel Spirit荣誉精神Marshal主帅Le Baron男爵Maserati玛西拉蒂Le Mans勒·曼斯Master主人Lecar莱卡Masterace马斯特艾斯Leeza利扎Mastiff猛犬Legacy雄狮Matado玛塔多Leganza丽伽Matador斗牛士Legend传奇Matra马特拉Leopard猎豹Matra Bagheera马特拉·巴格西拉Lesabre马刀Matra Rancho马特拉·兰奇Levin霹雳Maverick野牛Lexus凌志Maxi马克西Leyland利兰Maxima马克西玛Leyland Cruiser利兰·巡洋舰Mchari梅哈丽Leyland Roadtrain利兰·车队Megane米加妮Libero自由人Merak梅拉克Liberta自由别墅Mercedes默谢台斯Life生活Merkur默克Lion狮Metro地下铁道Liteace莱特艾斯Micra米克拉Logus罗古斯Midge矫捷Longchamp隆前普Mighty Bog好少年Longliner长途班车Millenia米利妮亚Prelude1053 Mini米尼Octopus章鱼Mini City米尼·城市Olinpia奥林匹亚Mini Mayfair米尼·贵族宅区Omega欧米加Mini Metro米尼·地下铁道Omni欧姆尼Minica米尼卡Opala奥帕拉Minica Econo米尼卡·埃柯诺Opel欧宝Minicab米尼卡布Opti奥普蒂Mira米拉Orion猎户座 ,奥利安Mirada米拉达Pacer标兵Mirafiori米拉费欧丽Padmini帕米尼Mirage空中楼阁Pajero帕杰罗Mockvich莫斯科人Palio帕里奥Monaco摩纳哥Panda熊猫Monarch君主Panorama帕诺拉玛Mondeo蒙代欧Pantera潘特拉Mondi世界Parati帕拉蒂Montecarlo蒙特卡洛Parisienne巴黎女郎Montego蒙远古Park Avenue公园大道Monterey蒙特利Park Ward皇苑Montero蒙特罗Partner帕尼尔 ,伙伴Montez蒙泰兹Paseo帕赛奥Monza蒙扎Passat帕萨特,贸易风Morgan莫根Passport护照Morris莫利斯Pathfinder探路者Motion动感Patrol侦探兵Mountaineer爬山者Perdana佩达娜Move行动Personal公事员Murat姆拉特Peugeot别儒,标致Musso犀牛Phanton鬼魅Mustang野马Phoenix凤凰Mystique寰宇Piazza广场Nabian纳宾Picnic郊游Nana娜娜Pinto斑马National公民Plus普鲁斯Navajo纳瓦久Plymouth顺风Navigator领航员Pointer指针Neon霓虹Polara波拉勒Nevada内华达Polo马球,波罗New Power新动力Polo Classic马球·经典New Yorker纽约人Polonez波罗乃兹Nexia蓝天Pony小马Ninety-Eight九十八Popular大众Niva尼瓦Porsche鲍舍Nomad游牧者Potentia波登西亚Nova新星Power Ram壮公羊Nubira奴比拉Prairie大草原Octavia奥克塔维亚Prelude序曲1054PresidentPresident总统Robin Estate罗宾·庄园Previa前驱Robin Hatch罗宾·哈奇Pride骄傲,普里德Rocky罗基 ,盘石Primera普丽美拉Rocsta罗科斯塔Prince王子Rodeo竞技Princess公主Rogat罗盖特Prisma棱镜Ronda巡逻队Privs普丽吾丝Rose玫瑰Prizm奖品Routeman领航员Probe探究Rover罗孚Prowler神行者Royale皇家Publica公民Runner赛手Pullman普尔门Saab萨伯,绅宝Pulstar脉冲星Saber马刀Punto彭托Sable黑貂Quadrifoglio快追弗格里奥Sabre赛波Quantum Familia量子·家族Safari游猎Quattro夸特罗(四轮)Safrane萨夫兰Quattroporte夸特罗包尔特(四轮驱动)Sahin萨因Quint昆特Samara萨玛拉Rabbit兔牌Samara Baltic萨玛拉·巴帝克Racer赛艇Samba桑巴Racing赛跑Sambar麋鹿Rainbow彩虹Samurai武士Rally赛车会Santana桑塔纳Ram公羊Sapparo札幌Ramcharge装运者Saturn土星,农神Rampage猛冲Savanna草原Ranchero牧场主Saviem萨维姆Range Rover兰治·罗孚Saxo萨克索Ranger遨游者Scania斯堪尼亚Raum罗姆Scenic景观Reatta瑞塔Scimitar Sabre短弯刀Regal皇朝Scirocco西罗柯,海风Regata利加塔Scorpio天蝎座Reiver打劫者Scottsdale斯科茨戴尔Rekord创纪录Scrambler登攀者Reliance信心Scrum斯克茹姆Reliant里莱恩特Seat西特Renegade反叛者Sebring赛百灵Retona瑞图纳Seicengo赛申格Rio Verde里欧·维德Selecta塞雷科塔Ritmo节奏Senator议员Riviera里维埃拉Senda森达Roadmaster罗德马斯特Sentia西泰Roadpacer罗德佩瑟Sentra桑特拉Robin罗宾,知更鸟Sephia赛菲亚Tempest1055 Serena赛瑞纳Sprinter Carib短跑家·卡利勃Seventy Seven七十七Sprinter Trueno短跑家·托雷诺Seville赛威Spyder斯派德Sharan莎兰St· Regis圣·里吉斯Shelby Charger谢尔比·战马Stagea斯塔戈Sherpa舍帕Stanza斯坦札Shiguli日古里Starfire星火Shuttle舒特Starion斯塔里恩Siena西那Starlet小星Sienna锡耶纳Statesman政治家Sierra塞拉,山脉Stealth隐形者Silhouette雪尔悉脱Stellar明星Silver Dawn银曦Stout斯托特Silver Shadow银影Strada道路Silver Spirit银灵Strada Abareh道路·阿巴斯Silver Spur银剌Strato同温层Silver Wraith银魂Stratus层云Silverado西尔维拉多Subaru斯巴尔 ,昂星团Silvia西尔维亚Suburban郊区居民Simca西姆卡Summit巅峰Sintra森特Sumo速魔Sketch斯凯茨Sunbeam阳光Skoda斯柯达Sunbird金驹鸟Skylark云雀Sundance桑旦斯 ,太阳舞Skyline天际线Sunfire太阳火Skyliner航空班机Sunny小太阳Slawuta斯拉乌塔Sunny California小太阳·加利福尼亚Smart斯马特Sunny Vanette小太阳·瓦内特Solara太阳Sunseeker太阳探险者Soleil索莱尔Super Charged超级电子Sonata索那塔Super Seven超级·塞文Sovereign元首Supra萨普拉Space太空Sven Hedin文雅·赫丁Space-Arrow宇宙之箭SVX斯维克斯Space-Dream宇宙之梦Swift雨燕Spaceliner航天班机Taft塔夫特Space-Runner空中骏马Tagora塔古拉Space-Wing宇宙之翼Tahoe太和Spartana斯巴达那Taimar泰玛Spazio斯帕兹Talon魔爪Spider蜘蛛Targa达佳Spirit幽灵Tasmin塔斯明Sport Spider运动蜘蛛Taunus陶奴斯Sportage斯波达Taurus雪牛座Sportsman体育家Tavria塔伏利亚Sprint疾跑Telstar通讯卫星Sprinter短跑家Tempest狂风雨1056TempoTempo速度Uno乌诺Tempra天霸Urraco于拉科Tercel雄鹰Urraco Silhouette于拉科·西卢埃特Terios托瑞索Urvan郊野Terrano泰拉诺Van前锋Terrier士兵Vandenr Plas范登普拉斯Thames泰晤士Vandura范杜拉Thema主旋律Vanette瓦内特Think城市蜜蜂Vantage优势Thunderbird雷鸟Variant瓦里恩特Tico太克VAZ瓦斯Tiger虎Vaz-Oka瓦斯-欧卡Tigra迪拉 ,小虎Vectra威达Tipo蒂波Vega织女星Titan鼎力神,泰坦Vehi维海Today今天Velox维洛斯Toledo托莱多Vento文托,风Top Executive最高经理Ventora文托拉Toppo涛波Venturer冒险者Torana托拉那Verada维拉达Toronado托罗纳多Veraneio维拉尼奥Toros多罗斯Versailles凡尔赛Town & Country城乡Victor成功者Townace城市之花Victory成功Toyota ACE丰田之花Vienta维安塔Tracer追踪者,探究者Vigor气魄Tracker追踪者Viking海盗Tradesman商业家Villager田园Trafic运输Violet紫罗兰Transcontinental洲际Viper蝰蛇Transfer转运Visa维沙Transit特兰西特Vision幻影Transport运输Vista远景Transporter运输者Vitara维泰拉Trax Challenger特拉克斯·挑战人Viva万岁Trax Gurkha特拉克斯·古尔哈Vivace维瓦赛Tredia特雷迪亚Vivio维维欧Treker特里克Volante飞鼠Trevi特里维Volare飞奔Trooper骑兵Volga伏尔加Trueno特雷诺Volumex沃露梅克斯Tschaika海鸥Volvo沃尔沃Turismo图里斯莫Voyager航海家Twingo丽人行Wagoneer瓦戈尼尔,御夫座Ultima乌蒂玛Warrior猛士Ulysse优力塞Windom温多姆Unimog乌尼莫克Windstar风之星Zil1057 Wingroad威拉得Yunque云雀Wrangler雄辩者Zastav红旗X sara X·萨拉ZAZ扎波罗什Xantia桑蒂雅Zen泽恩Xedos爱克道斯Zeta赛达Xiali夏利Zil吉尔Yugo尤格,南方。
车牌识别英文文献1翻译
提出的模型本文的主要目的是要开发一个系统可以从没有虚假质量的复杂的现场图像中提取车牌号码,相机和车牌之间的距离,其中的相对车牌已被抓获在相机等。
对车牌识别系统概述见图1,在车辆图片由相机拍摄后,它会被传递到预先处理单位由系统作进一步处理。
其主要功能是消除图像采集子系统所造成的噪声,提升图像的其他两个子系统使用的功效。
图像将被板提取模块扫描以找到车辆的车牌。
下一阶段是对于车牌中字符的分割。
最后每个字符将传递给光学字符识别(OCR)模块来进行识别确定,最终结果将是ASCII字符和车牌号码。
3.1.预处理输入图像的最初处理是为了提高其质量,并为系统的下一阶段执行作准备。
首先,该系统使用的NTSC标准的方法将RGB图像转换为灰度图像。
G=0.299*R+0.587*G+0.114*B第二步,用中值滤波(5x5)对灰度图像进行处理,以消除噪音,同时也能保持图像的清晰度。
中值滤波是一种非线性滤波器,它用各像素5x5邻里的计算得出中位值来取代该像素的值。
3.2.板块提取板块提取处理包含五个不同的阶段进行,如图2所示;在这里每个阶段执行灰度图像分割过程以消除不属于车牌区域的多余的像素。
例如,水平定位阶段是负责寻找水平部分可能包含一个车牌。
在下面的讨论中,每一个阶段都会被细细展开讨论。
马来西亚车牌由一排白色字符在黑色的背景底色,所以我们可以说,车牌区域的特点是从一排黑色过渡到一排白色,反之亦然,这样转换被称为“边缘”。
从车牌字符到其背景在色彩强度上总的变化叫做边缘的强度。
最强边缘值,能够在从一个黑色像素过渡到一个白色像素或从白色像素变为黑色像素情况下被找到。
在理想的情况下,马来西亚的车牌是白色的字符绘制在黑色的背景上,因此这种搭配产生了高强度边缘值,用于查找出可能的板区域。
在本文中我们将使用Sobel算子来查找边缘。
Sobel运算在图片上执行一个2-D空间梯度测量。
通常它是用来寻找近似绝对的梯度幅度对在每一个点在输入的灰度图像上。
毕业设计BP神经网络方法对车牌照字符的识别(含外文翻译) (1)
摘要为了对车牌字符的识别,本文将BP神经网络应用于汽车车牌的自动识别,在车牌图像进行预处理后的基础上,重点讨论了用BP神经网络方法对车牌照字符的识别。
首先将训练样本做图像预处理,对车牌上的字符进行分割,得到单个字符。
对大小不一的字符做归一化后,对字符进行特征提取,把长为15,宽为25的归一化后的图像中的字符信息提取出来,图像中白点置为0,图像中的黑点置为1,这样就得到了15×25的特征向量,这个特征向量记录的就是字符的特征。
把这个特征向量送到BP网络中进行训练,得到了训练好的权值,把他保存到“win.dat”和“whi.dat”中。
然后打开要识别的图片(即车牌),对图像进行预处理后就可以识别了。
识别率也在90%以上,表明该方法的有效性。
关键字:车牌识别;LPR;字符识别;特征提取; BP神经网络;AbstractFor the discernment to the number plate character, this text applies BP neural network to the automatic discernment of the automobile number plate, on the basis that the number plate picture goes on in advance treated , is it use BP neural network method to car discernment , license plate of character to discuss especially. Will train samples to do the pretreatment of the picture at first, character in number plate cut apart, get the individual character. After making normalization to the character not of uniform size, drew the characteristic to the character 15, wide to draw out for character information of 25 picture behind the normalization, picture white point it puts to be 0, black point of picture is it as 1 , receive 15* 25 characteristic vector quantity like this to put, what the vector quantity of this characteristic is written down is the characteristic of the character . Send the characteristic vector quantity BP network train, get good right value of training, keep him in win.dat and whi.dat. Open picture (namely number plate) discerned to want, go on to picture in advance treated to can discern. The discerning rate is above 90% too; show the validity of this method.Key word:The number plate discerning;The character discerning;LPR;The characteristic is drawn;BP neural network;目录摘要 (Ⅰ)ABSTRACT (Ⅱ)第一章概述 (1)1.1 基本概念 (1)1.2 字符识别简介 (2)1.2.1字符识别发展概况 (2)1.2.2字符识别系统用到的方法 (3)1.2.3字符识别原理 (4)1.3 国内外研究现状和发展趋势 (5)1.4 基于神经网络的字符识别系统 (6)1.4.1 系统简介 (6)1.4.2 系统的基本技术要求 (7)1.4.3系统的软硬件平台 (7)第二章字符识别系统中的关键技术 (8)2.1 特征提取 (8)2.1.1 基本概念 (8)2.1.2 区域内部的数字特征 (10)2.1.3 基于边界的形状特征 (13)2.2 神经网络 (18)2.2.1 人工神经元 (18)2.2.2 人工神经网络构成 (22)2.2.3 人工神经网络的学习规则 (23)2.2.4 BP神经网络 (24)第三章系统的实现 (31)3.1 系统流程图 (31)3.2 程序实现 (31)3.3 程序的总体框架 (36)第四章系统使用说明、测试及注意事项 (37)4.1 系统使用说明 (37)4.2 系统测试 (39)4.2.1 数字识别 (39)4.2.2 字母识别 (40)4.2.3 汉字识别 (40)4.2.4 车牌识别 (41)4.3 注意事项 (41)第五章结论和展望 (42)致谢 (43)参考文献 (44)外文原文与译文 (46)●外文原文 (46)●译文 (57)第一章概述1.1 基本概念随着21世纪经济全球化和信息时代的到来,计算机技术、通信技术和计算机网络技术迅猛发展,自动化的信息处理能力和水平不断提高,并在人们社会活动和生活的各个领域得到广泛应用。
- 1、下载文档前请自行甄别文档内容的完整性,平台不提供额外的编辑、内容补充、找答案等附加服务。
- 2、"仅部分预览"的文档,不可在线预览部分如存在完整性等问题,可反馈申请退款(可完整预览的文档不适用该条件!)。
- 3、如文档侵犯您的权益,请联系客服反馈,我们会尽快为您处理(人工客服工作时间:9:00-18:30)。
实时车辆的车牌识别系统摘要本文中阐述的是一个简炼的用于车牌识别系统的算法。
基于模式匹配,该算法可以应用于对车牌实时检测数据采集,测绘或一些特定应用目的。
拟议的系统原型已经使用C++和实验结果已证明认可阿尔伯塔车牌。
1.介绍车辆的车牌识别系统已经成为在视频监控领域中一个特殊的热门领域超过10年左右。
随着先进的用于交通管理应用的视频车辆检测系统的的到来,车牌识别系统被发现可以适合用在相当多的领域内,并非只是控制访问点或收费停车场。
现在它可以被集成到视频车辆检测系统,该系统通常安装在需要的地方用于十字路口控制,交通监控等,以确定该车辆是否违反交通法规或找到被盗车辆。
一些用于识别车牌的技术到目前为止有如BAM(双向联想回忆)神经网络字符识别[1],模式匹配[2]等技术。
应用于系统的技术是基于模式匹配,该系统快速,准确足以在相应的请求时间内完成,更重要的是在于阿尔伯塔车牌识别在字母和数字方位确认上的优先发展。
由于车牌号码的字体和方位因国家/州/省份的不同而不同,该算法需要作相应的修改保持其结构完整,如果我们想请求系统识别这些地方的车牌。
本文其余部分的组织如下:第2节探讨了在识别过程中涉及的系统的结构和步骤,第3节解释了算法对于车牌号码的实时检测,第4节为实验结果,第5节总结了全文包括致谢和参考文献。
2.系统架构系统将被用来作为十字路口的交通视频监控摄像系统一个组成部分来进行分析。
图1显示了卡尔加里一个典型的交叉口。
只有一个车牌用在艾伯塔,连接到背面的车辆照相机将被用于跟踪此背面车牌。
图1 卡尔加里一个的典型交叉口系统架构包含三个相异部分:室外部分,室内部分和通信链路。
室外部分是安装摄像头在拍摄图像的不同需要的路口。
室内部分是中央控制站,从所有这些安装摄像头中,接收,存储和分析所拍摄图像。
通信链路就是高速电缆或光纤连接到所有这些相机中央控制站。
几乎所有的算法的开发程度迄今按以下类似的步骤进行。
一般的7个处理步骤已被确定为所有号牌识别算法[3] 共有。
它们是:触发:这可能是硬件或软件触发。
硬件触发是旧的方式,即感应圈用于触发和这个表述了图像通过检测车牌的存在何时应该被捕获。
硬件触发现在在操作上在许多地方被软件触发取代。
在软件触发,图像分为区,通过图像对于分析的车辆的检测的执行。
图像采集:硬件或软件触发启动图像捕捉设备来捕捉和存储图像来进一步的分析。
车辆的存在:这一步是只需要如果在确认一定时间间隔后触发完成不需要知道车辆存在于捕获的图像中。
这一步背景图像与捕获的图片作比较,并检测是否有任何重大改变。
如果没有,拍摄的图像被忽略,否则进入到下一个步骤。
寻找车牌:此步骤是在捕获的图像中定位车牌。
一些技术的可用于这一步,例如颜色检测[4],特征分析[5],边缘检测[6]等。
在捕获的图像中的任何倾斜是纠正在这一步。
一旦车牌已被定位,图像即准备进行字符识别。
字符分割:分割可以通过检测浓到淡或者淡到浓的过渡层。
车牌中的每个灰色字符产生了一个灰色带。
因此,通过检测类似灰度带每个字符可以被分割出来。
识别过程:这是光学字符识别的一步。
一些技术可以被用于到这一步包括模式匹配[2],特征匹配[7][8]和神经网络分类[9]。
发布过程:这是应用程序的特有的一步。
根据应用此步骤可保存已被检测出来的车牌用于交通数据收集,尝试匹配号牌与被盗车辆数据库或在停车场中为认可停车的车辆打开汽车门等等。
3.算法该算法用于在处理捕获的图像和车牌检测后的车牌字符识别。
基于模式匹配,系统沿用了一个智能算法用于艾伯塔车牌字母和数字的识别。
图2显示了一个艾伯塔省车牌样本其中包含三个字母,3个数字和破折号在内。
所以通过基本的字符确认方法,模糊的字符比如有:数字'0'和字母'O',数字'8'和字母'B已被解决。
此外,由于前三个字符是字母,所以只需与A-Z这一段的字母作比较比较。
类似的,在最后三个字符,它门只需与0-9这一段数字作比较。
图2. 阿尔伯塔省的车牌首先字符识别问题是要找出字符的印刷区域。
这一区域通常是垂直和水平居中的。
因此,通过采取颜色的浓度,我们可以得到字符垂直的顶部和底部。
一旦图像中字符的顶部和底部位置被找到,该区域可以从生成的图像中分割出来,生成图3一样的图像。
这个图像现在为字符分割和识别作准备。
图3. 分割的图像只包含字符作进一步处理字符分割可通过横向颜色的浓度来进行。
为了模式匹配有效地进行,需要在车牌上找到一个与之相匹配的字体。
Arial字体在阿尔伯塔省的车牌字符识别用起来相当好。
在用到这种字体时一个库首先被建立起来。
这个库包含直方图字母AZ和数字0-9。
15个不同的直方图已为了库生成各自相应的字符。
它们是:水平直方图对应的(1)全尺寸,(2)下半部分,(3)上半部分,(4)下部三分之一,(5)上部三分之一,(6)下部四分之一,(7)上部四分之一,(8)上部的三分之二的字符和垂直直方图对应的(9)全尺寸,(10)左半部,(11)右半部,(12)左边三分之一,(13)右边三分之一,(14)左边四分之一以及(15)右边四分之一的字符。
识别的流程图已在图4中给出。
如图所示,3段在每次用于识别以及库在每次被调用时取决于这‘三段’是否被采用。
如果3段设定被检测的为字母,'字母'库将被调用来进行比较,否则就是'数'库被调用来进行比较。
有15个不同的直方图每个字母的排序为A-Z在‘字母'库中与15位不同的直方图每个字符排序为从0-9在‘数字’中。
图4中所示的算法要运行两次,将‘三段’设置各自运行一次,为了完整地识别车牌。
i在流程图中迭代算子。
s 和p是匹配的参数。
图4. 字符识别的流程图i的值随着每个循环而改变并且这个值指示了库中的哪个直方图应该被用来作比较。
如流程图中所示,从段提取的直方图(通过i的变化而定)在作比较之前应该首先被正常化。
一旦正常化过程完成后,该段准备与存储在库中的模式作匹配。
每个匹配过程提供了一套在检查下与段相似匹配的字符。
因此,用不同的直方图模式通过进行几次这样的过程,最不可能的字符被过滤掉留下最正确的。
4.实验结果系统已经使用C++建立原型并且用艾伯塔省的车牌样本进行测试。
图5显示通过采取图像中垂直颜色浓度来确定车牌字符位置的过程。
从中心到上和从中心到下进行水平扫描,图像中字符顶端(H1)和底端(H2)的位置找到。
图5。
垂直颜色浓度图6显示了字符分割的过程。
这是通过利用颜色的浓度水平进行完成的。
因为我们知道,前三个字符是字母而最后三个字符是数字,我们可以很容易在分割后将他们分组进行下一步:模式匹配。
图6。
字符分割如图所示的流程图中的15个不同的模式在系统中使用的是随i的值而定,并此方式分配:0(水平直方图,全尺寸),1(垂直直方图,全尺寸),2(水平直方图,上半部),3(垂直直方图,左半部),4(垂直直方图,右半部),5(水平直方图,下半部),6(水平直方图,下部三分之一),7(垂直直方图,右三分之一),8(水平直方图,上部三分之一),9(垂直直方图,左三分之一),10(水平直方图,下部四分之一),11(水平直方图,上部四分之一),12(水平直方图,上部三分之二),13(垂直直方图,右四分之一),14(垂直直方图,左四分之一)。
从段提取的直方图在作比较之前应该首先被正常化。
图7.正常化进程正常化通过段的宽度与库作比较来完成。
例如,如果拿水平直方图来进行比较,三段中水平方向的最大宽度要与库中的最大宽度进行比较。
如果该段的宽度更大,直方图通过邻近位置的直方图的平均值在水平方向均匀缩小。
类似的过程已被用于放大,如果是偏小的。
图7说明正常化时,段的宽度比库的要大。
图7(a)显示了库中字母F的水平全直方图。
图7(b)显示了字母F的水平直方图在段中被找到。
如果F的直方图的宽度在库中最大(在16的情况下),从段中找到的直方图宽度(在19的情况下)在进行比较之前应该被缩减到16这种情况。
这个过程完成并表述在图7(c)中。
由于宽度的差值为3,直方图3这个段直方图中均匀分配位置的值将被删除,计算邻域的平均值。
如图7(c)中所示,5号,10号与15号位置的值被删除通过对4号,6号,9号,11号,14号与16号位置值的平均计算。
4号位置的新值是原来4号与5号位置的值的平均值。
类似的,5号位置的新值是原来5号和6号位置原来的值的平均值等。
经过规范化,进行模式匹配。
这是通过比较每个直方图中两个比率来完成。
一个来自段,另一个来自库。
该比率是直方图每个位置的值对应图中的最大值。
如果这两个比率的差值在某值设置通过参数s以内,匹配计数增加。
因此,通过在横向(水平直方图)/垂直(垂直直方图)的位置部分,我们得到一个匹配计数说明段与字符如何密切匹配。
对于库中的每一个字符重复这个过程,获得库中每一个字符的匹配计数。
现在,通过假设最高匹配计算为100%匹配,字符的匹配小于70%(由参数 p设定)的算法过滤器。
因此,下一次,当算法采用不同的直方图时,将这段与先前检测到的字符作比较。
如果在做这些比较进行了15个不同的直方图之后,仍有存在多个匹配,整个过程将重复进行伴随具有较高的灵敏度(S随灵敏度增加而下降),直到找到一个。
5.结论本文提出了一种实时的车牌识别系统,突出的一些地区在此应用系统执行都可以。
该系统结构对于识别识别过程中涉及的复杂的步骤进行了讨论。
该实验已经进行,澄清了系统作为一个潜在的候选用于实时识别。
实验表明了本文假定的理想的天气条件。
研究的各种假设天气状况正在进展中。
该原型系统将整合到路口监控录像作流量测量或一些应用在特定用途的文件中进行讨论。
6.致谢作者在此感谢支持这项研究的自然科学加拿大与工程研究理事会(NSERC),卡尔加里大学和卡尔加里市。