Princ.econs -lec 12
源自欧夏至草的成分的化妆品用途[发明专利]
![源自欧夏至草的成分的化妆品用途[发明专利]](https://img.taocdn.com/s3/m/744e55bb3b3567ec102d8ae9.png)
专利名称:源自欧夏至草的成分的化妆品用途专利类型:发明专利
发明人:C·林根巴赫,E·多瑞多特,P·蒙东
申请号:CN201780015321.0
申请日:20170321
公开号:CN108778242A
公开日:
20181109
专利内容由知识产权出版社提供
摘要:本发明提出了源自欧夏至草(Marrubium vulgare)的植物材料用于紧致皮肤毛孔的非治疗性美容处理的用途,所述植物材料包含有效量的连翘酯苷B作为活性分子。
所述植物材料优选由去除了细胞碎片的去分化植物细胞的细胞提取物组成。
这种治疗特别打算用于细化皮肤纹理和/或用于处理具有油性趋势的皮肤。
申请人:赛德玛公司
地址:法国勒佩雷-恩-伊林斯
国籍:FR
代理机构:中国国际贸易促进委员会专利商标事务所
代理人:李瑛
更多信息请下载全文后查看。
羟基酪醇对H2O2损伤的神经细胞株PC12凋亡影响及其机制
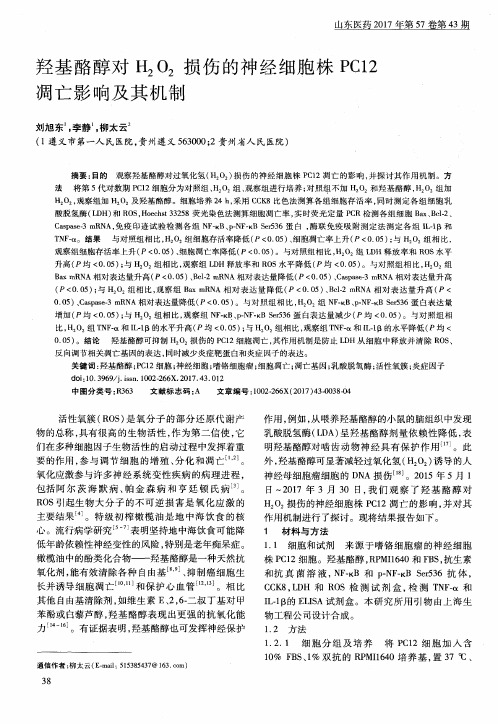
羟基酪醇对 H 2 O 2损 伤 的神 经 细 胞 株 P C 1 2
凋 亡 影 响及 其 机 制
刘 旭 东 , 李 静 , 柳 太 云
( 1 遵义市第一人民医院, 贵州遵义 5 6 3 0 0 0 ; 2贵州省人 民医院)
活性 氧簇 ( R O S ) 是 氧 分 子 的部 分 还 原 代 谢 产
作用 , 例如 , 从 喂养羟 基酪 醇 的小 鼠的脑组 织 中发 现 乳 酸脱 氢酶 ( L D A) 呈 羟 基酪 醇 剂 量 依 赖性 降低 , 表 明羟基 酪 醇对 啮齿 动 物 神 经 具 有 保 护 作 用 J 。此 外, 羟 基酪 醇可 显著 减轻 过氧 化氢 ( H: O : ) 诱 导 的人 神经母 细胞 瘤 细胞 的 D N A损 伤 。2 0 1 5年 5月 1 1 3—2 0 1 7年 3月 3 0 日, 我 们 观 察 了 羟 基 酪 醇 对 H 0 : 损 伤的神 经 细胞 株 P C 1 2凋亡 的影 响 , 并 对其
作用 机制 进行 了探讨 。现 将结 果报 告如 下 。
1 材 料 与方法
物的总称 , 具有很 高的生物活性 , 作为第二信使 , 它 们 在多 种 细胞 因子 生物 活性 的启 动过程 中发 挥着 重 要 的作 用 , 参 与 调节 细 胞 的增 殖 、 分化 和凋亡¨ I 2 j 。
酸脱氢酶 ( L D H) 和R O S , H o e c h s t 3 3 2 5 8荧光染色法测算细胞凋亡率 , 实 时荧 光定量 P C R检测各 组细胞 B a x 、 B c l - 2 、
C a s p a s e - 3 mR N A, 免疫 印迹试 验检 测各 组 N F — K B、 p — N F — K B S e r 5 3 6蛋 白 , 酶 联免 疫吸附 测定 法测 定各 组 I L — l B和 T N F 一 0 【 。结果 与对照组相 比 , H O 2 组细胞存活率降低 ( P< 0 . 0 5 ) 、 细胞凋 亡率 上升( P< 0 . 0 5 ) ; 与H : O 组 相 比, 观察组细胞存活率上升 ( P< 0 . 0 5 ) 、 细 胞凋亡 率降低 ( P< 0 . 0 5 ) 。与对照组相 比, H: O 组L D H 释放 率和 R O S水平 升高( P均 < 0 . 0 5 ) ; 与H O 2 组相 比, 观察组 L D H释放率 和 R O S水平 降低 ( P均 < 0 . 0 5 ) 。与对 照组相 比 , H O 组 B a x m R N A相对表达量 升高( P< 0 . 0 5 ) 、 B c l - 2 mR N A相对表达 量降低( P< 0 . 0 5 ) 、 C a s p a s e 一 3 m R N A相对表达量升高 ( P< 0 . 0 B a x m R N A相对 表达 量 降低 ( P< 0 . 0 5 ) 、 B c l 一 2 mR N A相 对表 达量 升高 ( P< 0 . 0 5 ) 、 C a s p a s e 一 3 m R N A相对表达量 降低 ( P< 0 . 0 5 ) 。与对照组 相 比 , H 2 O : 组N F ・ K B、 p - N F — K B S e r 5 3 6蛋 白表达量
Multicamera People Tracking with a Probabilistic Occupancy Map
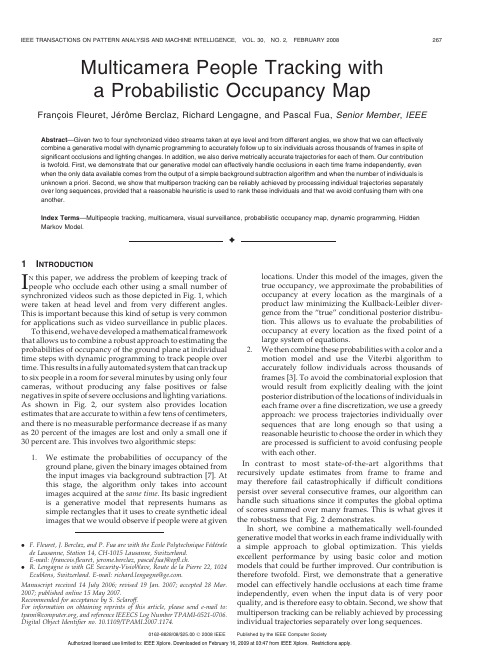
Multicamera People Tracking witha Probabilistic Occupancy MapFranc¸ois Fleuret,Je´roˆme Berclaz,Richard Lengagne,and Pascal Fua,Senior Member,IEEE Abstract—Given two to four synchronized video streams taken at eye level and from different angles,we show that we can effectively combine a generative model with dynamic programming to accurately follow up to six individuals across thousands of frames in spite of significant occlusions and lighting changes.In addition,we also derive metrically accurate trajectories for each of them.Our contribution is twofold.First,we demonstrate that our generative model can effectively handle occlusions in each time frame independently,even when the only data available comes from the output of a simple background subtraction algorithm and when the number of individuals is unknown a priori.Second,we show that multiperson tracking can be reliably achieved by processing individual trajectories separately over long sequences,provided that a reasonable heuristic is used to rank these individuals and that we avoid confusing them with one another.Index Terms—Multipeople tracking,multicamera,visual surveillance,probabilistic occupancy map,dynamic programming,Hidden Markov Model.Ç1I NTRODUCTIONI N this paper,we address the problem of keeping track of people who occlude each other using a small number of synchronized videos such as those depicted in Fig.1,which were taken at head level and from very different angles. This is important because this kind of setup is very common for applications such as video surveillance in public places.To this end,we have developed a mathematical framework that allows us to combine a robust approach to estimating the probabilities of occupancy of the ground plane at individual time steps with dynamic programming to track people over time.This results in a fully automated system that can track up to six people in a room for several minutes by using only four cameras,without producing any false positives or false negatives in spite of severe occlusions and lighting variations. As shown in Fig.2,our system also provides location estimates that are accurate to within a few tens of centimeters, and there is no measurable performance decrease if as many as20percent of the images are lost and only a small one if 30percent are.This involves two algorithmic steps:1.We estimate the probabilities of occupancy of theground plane,given the binary images obtained fromthe input images via background subtraction[7].Atthis stage,the algorithm only takes into accountimages acquired at the same time.Its basic ingredientis a generative model that represents humans assimple rectangles that it uses to create synthetic idealimages that we would observe if people were at givenlocations.Under this model of the images,given thetrue occupancy,we approximate the probabilities ofoccupancy at every location as the marginals of aproduct law minimizing the Kullback-Leibler diver-gence from the“true”conditional posterior distribu-tion.This allows us to evaluate the probabilities ofoccupancy at every location as the fixed point of alarge system of equations.2.We then combine these probabilities with a color and amotion model and use the Viterbi algorithm toaccurately follow individuals across thousands offrames[3].To avoid the combinatorial explosion thatwould result from explicitly dealing with the jointposterior distribution of the locations of individuals ineach frame over a fine discretization,we use a greedyapproach:we process trajectories individually oversequences that are long enough so that using areasonable heuristic to choose the order in which theyare processed is sufficient to avoid confusing peoplewith each other.In contrast to most state-of-the-art algorithms that recursively update estimates from frame to frame and may therefore fail catastrophically if difficult conditions persist over several consecutive frames,our algorithm can handle such situations since it computes the global optima of scores summed over many frames.This is what gives it the robustness that Fig.2demonstrates.In short,we combine a mathematically well-founded generative model that works in each frame individually with a simple approach to global optimization.This yields excellent performance by using basic color and motion models that could be further improved.Our contribution is therefore twofold.First,we demonstrate that a generative model can effectively handle occlusions at each time frame independently,even when the input data is of very poor quality,and is therefore easy to obtain.Second,we show that multiperson tracking can be reliably achieved by processing individual trajectories separately over long sequences.. F.Fleuret,J.Berclaz,and P.Fua are with the Ecole Polytechnique Fe´de´ralede Lausanne,Station14,CH-1015Lausanne,Switzerland.E-mail:{francois.fleuret,jerome.berclaz,pascal.fua}@epfl.ch..R.Lengagne is with GE Security-VisioWave,Route de la Pierre22,1024Ecublens,Switzerland.E-mail:richard.lengagne@.Manuscript received14July2006;revised19Jan.2007;accepted28Mar.2007;published online15May2007.Recommended for acceptance by S.Sclaroff.For information on obtaining reprints of this article,please send e-mail to:tpami@,and reference IEEECS Log Number TPAMI-0521-0706.Digital Object Identifier no.10.1109/TPAMI.2007.1174.0162-8828/08/$25.00ß2008IEEE Published by the IEEE Computer SocietyIn the remainder of the paper,we first briefly review related works.We then formulate our problem as estimat-ing the most probable state of a hidden Markov process and propose a model of the visible signal based on an estimate of an occupancy map in every time frame.Finally,we present our results on several long sequences.2R ELATED W ORKState-of-the-art methods can be divided into monocular and multiview approaches that we briefly review in this section.2.1Monocular ApproachesMonocular approaches rely on the input of a single camera to perform tracking.These methods provide a simple and easy-to-deploy setup but must compensate for the lack of 3D information in a single camera view.2.1.1Blob-Based MethodsMany algorithms rely on binary blobs extracted from single video[10],[5],[11].They combine shape analysis and tracking to locate people and maintain appearance models in order to track them,even in the presence of occlusions.The Bayesian Multiple-BLob tracker(BraMBLe)system[12],for example,is a multiblob tracker that generates a blob-likelihood based on a known background model and appearance models of the tracked people.It then uses a particle filter to implement the tracking for an unknown number of people.Approaches that track in a single view prior to computing correspondences across views extend this approach to multi camera setups.However,we view them as falling into the same category because they do not simultaneously exploit the information from multiple views.In[15],the limits of the field of view of each camera are computed in every other camera from motion information.When a person becomes visible in one camera,the system automatically searches for him in other views where he should be visible.In[4],a background/foreground segmentation is performed on calibrated images,followed by human shape extraction from foreground objects and feature point selection extraction. Feature points are tracked in a single view,and the system switches to another view when the current camera no longer has a good view of the person.2.1.2Color-Based MethodsTracking performance can be significantly increased by taking color into account.As shown in[6],the mean-shift pursuit technique based on a dissimilarity measure of color distributions can accurately track deformable objects in real time and in a monocular context.In[16],the images are segmented pixelwise into different classes,thus modeling people by continuously updated Gaussian mixtures.A standard tracking process is then performed using a Bayesian framework,which helps keep track of people,even when there are occlusions.In such a case,models of persons in front keep being updated, whereas the system stops updating occluded ones,which may cause trouble if their appearances have changed noticeably when they re-emerge.More recently,multiple humans have been simulta-neously detected and tracked in crowded scenes[20]by using Monte-Carlo-based methods to estimate their number and positions.In[23],multiple people are also detected and tracked in front of complex backgrounds by using mixture particle filters guided by people models learned by boosting.In[9],multicue3D object tracking is addressed by combining particle-filter-based Bayesian tracking and detection using learned spatiotemporal shapes.This ap-proach leads to impressive results but requires shape, texture,and image depth information as input.Finally, Smith et al.[25]propose a particle-filtering scheme that relies on Markov chain Monte Carlo(MCMC)optimization to handle entrances and departures.It also introduces a finer modeling of interactions between individuals as a product of pairwise potentials.2.2Multiview ApproachesDespite the effectiveness of such methods,the use of multiple cameras soon becomes necessary when one wishes to accurately detect and track multiple people and compute their precise3D locations in a complex environment. Occlusion handling is facilitated by using two sets of stereo color cameras[14].However,in most approaches that only take a set of2D views as input,occlusion is mainly handled by imposing temporal consistency in terms of a motion model,be it Kalman filtering or more general Markov models.As a result,these approaches may not always be able to recover if the process starts diverging.2.2.1Blob-Based MethodsIn[19],Kalman filtering is applied on3D points obtained by fusing in a least squares sense the image-to-world projections of points belonging to binary blobs.Similarly,in[1],a Kalman filter is used to simultaneously track in2D and3D,and objectFig.1.Images from two indoor and two outdoor multicamera video sequences that we use for our experiments.At each time step,we draw a box around people that we detect and assign to them an ID number that follows them throughout thesequence.Fig.2.Cumulative distributions of the position estimate error on a3,800-frame sequence(see Section6.4.1for details).locations are estimated through trajectory prediction during occlusion.In[8],a best hypothesis and a multiple-hypotheses approaches are compared to find people tracks from 3D locations obtained from foreground binary blobs ex-tracted from multiple calibrated views.In[21],a recursive Bayesian estimation approach is used to deal with occlusions while tracking multiple people in multiview.The algorithm tracks objects located in the intersections of2D visual angles,which are extracted from silhouettes obtained from different fixed views.When occlusion ambiguities occur,multiple occlusion hypotheses are generated,given predicted object states and previous hypotheses,and tested using a branch-and-merge strategy. The proposed framework is implemented using a customized particle filter to represent the distribution of object states.Recently,Morariu and Camps[17]proposed a method based on dimensionality reduction to learn a correspondence between the appearance of pedestrians across several views. This approach is able to cope with the severe occlusion in one view by exploiting the appearance of the same pedestrian on another view and the consistence across views.2.2.2Color-Based MethodsMittal and Davis[18]propose a system that segments,detects, and tracks multiple people in a scene by using a wide-baseline setup of up to16synchronized cameras.Intensity informa-tion is directly used to perform single-view pixel classifica-tion and match similarly labeled regions across views to derive3D people locations.Occlusion analysis is performed in two ways:First,during pixel classification,the computa-tion of prior probabilities takes occlusion into account. Second,evidence is gathered across cameras to compute a presence likelihood map on the ground plane that accounts for the visibility of each ground plane point in each view. Ground plane locations are then tracked over time by using a Kalman filter.In[13],individuals are tracked both in image planes and top view.The2D and3D positions of each individual are computed so as to maximize a joint probability defined as the product of a color-based appearance model and2D and 3D motion models derived from a Kalman filter.2.2.3Occupancy Map MethodsRecent techniques explicitly use a discretized occupancy map into which the objects detected in the camera images are back-projected.In[2],the authors rely on a standard detection of stereo disparities,which increase counters associated to square areas on the ground.A mixture of Gaussians is fitted to the resulting score map to estimate the likely location of individuals.This estimate is combined with a Kallman filter to model the motion.In[26],the occupancy map is computed with a standard visual hull procedure.One originality of the approach is to keep for each resulting connex component an upper and lower bound on the number of objects that it can contain. Based on motion consistency,the bounds on the various components are estimated at a certain time frame based on the bounds of the components at the previous time frame that spatially intersect with it.Although our own method shares many features with these techniques,it differs in two important respects that we will highlight:First,we combine the usual color and motion models with a sophisticated approach based on a generative model to estimating the probabilities of occu-pancy,which explicitly handles complex occlusion interac-tions between detected individuals,as will be discussed in Section5.Second,we rely on dynamic programming to ensure greater stability in challenging situations by simul-taneously handling multiple frames.3P ROBLEM F ORMULATIONOur goal is to track an a priori unknown number of people from a few synchronized video streams taken at head level. In this section,we formulate this problem as one of finding the most probable state of a hidden Markov process,given the set of images acquired at each time step,which we will refer to as a temporal frame.We then briefly outline the computation of the relevant probabilities by using the notations summarized in Tables1and2,which we also use in the following two sections to discuss in more details the actual computation of those probabilities.3.1Computing the Optimal TrajectoriesWe process the video sequences by batches of T¼100frames, each of which includes C images,and we compute the most likely trajectory for each individual.To achieve consistency over successive batches,we only keep the result on the first 10frames and slide our temporal window.This is illustrated in Fig.3.We discretize the visible part of the ground plane into a finite number G of regularly spaced2D locations and we introduce a virtual hidden location H that will be used to model entrances and departures from and into the visible area.For a given batch,let L t¼ðL1t;...;L NÃtÞbe the hidden stochastic processes standing for the locations of individuals, whether visible or not.The number NÃstands for the maximum allowable number of individuals in our world.It is large enough so that conditioning on the number of visible ones does not change the probability of a new individual entering the scene.The L n t variables therefore take values in f1;...;G;Hg.Given I t¼ðI1t;...;I C tÞ,the images acquired at time t for 1t T,our task is to find the values of L1;...;L T that maximizePðL1;...;L T j I1;...;I TÞ:ð1ÞAs will be discussed in Section 4.1,we compute this maximum a posteriori in a greedy way,processing one individual at a time,including the hidden ones who can move into the visible scene or not.For each one,the algorithm performs the computation,under the constraint that no individual can be at a visible location occupied by an individual already processed.In theory,this approach could lead to undesirable local minima,for example,by connecting the trajectories of two separate people.However,this does not happen often because our batches are sufficiently long.To further reduce the chances of this,we process individual trajectories in an order that depends on a reliability score so that the most reliable ones are computed first,thereby reducing the potential for confusion when processing the remaining ones. This order also ensures that if an individual remains in the hidden location,then all the other people present in the hidden location will also stay there and,therefore,do not need to be processed.FLEURET ET AL.:MULTICAMERA PEOPLE TRACKING WITH A PROBABILISTIC OCCUPANCY MAP269Our experimental results show that our method does not suffer from the usual weaknesses of greedy algorithms such as a tendency to get caught in bad local minima.We thereforebelieve that it compares very favorably to stochastic optimization techniques in general and more specifically particle filtering,which usually requires careful tuning of metaparameters.3.2Stochastic ModelingWe will show in Section 4.2that since we process individual trajectories,the whole approach only requires us to define avalid motion model P ðL n t þ1j L nt ¼k Þand a sound appearance model P ðI t j L n t ¼k Þ.The motion model P ðL n t þ1j L nt ¼k Þ,which will be intro-duced in Section 4.3,is a distribution into a disc of limited radiusandcenter k ,whichcorresponds toalooseboundonthe maximum speed of a walking human.Entrance into the scene and departure from it are naturally modeled,thanks to the270IEEE TRANSACTIONS ON PATTERN ANALYSIS AND MACHINE INTELLIGENCE,VOL.30,NO.2,FEBRUARY 2008TABLE 2Notations (RandomQuantities)Fig.3.Video sequences are processed by batch of 100frames.Only the first 10percent of the optimization result is kept and the rest is discarded.The temporal window is then slid forward and the optimiza-tion is repeated on the new window.TABLE 1Notations (DeterministicQuantities)hiddenlocation H,forwhichweextendthemotionmodel.The probabilities to enter and to leave are similar to the transition probabilities between different ground plane locations.In Section4.4,we will show that the appearance model PðI t j L n t¼kÞcan be decomposed into two terms.The first, described in Section4.5,is a very generic color-histogram-based model for each individual.The second,described in Section5,approximates the marginal conditional probabil-ities of occupancy of the ground plane,given the results of a background subtractionalgorithm,in allviewsacquired atthe same time.This approximation is obtained by minimizing the Kullback-Leibler divergence between a product law and the true posterior.We show that this is equivalent to computing the marginal probabilities of occupancy so that under the product law,the images obtained by putting rectangles of human sizes at occupied locations are likely to be similar to the images actually produced by the background subtraction.This represents a departure from more classical ap-proaches to estimating probabilities of occupancy that rely on computing a visual hull[26].Such approaches tend to be pessimistic and do not exploit trade-offs between the presence of people at different locations.For instance,if due to noise in one camera,a person is not seen in a particular view,then he would be discarded,even if he were seen in all others.By contrast,in our probabilistic framework,sufficient evidence might be present to detect him.Similarly,the presence of someone at a specific location creates an occlusion that hides the presence behind,which is not accounted for by the hull techniques but is by our approach.Since these marginal probabilities are computed indepen-dently at each time step,they say nothing about identity or correspondence with past frames.The appearance similarity is entirely conveyed by the color histograms,which has experimentally proved sufficient for our purposes.4C OMPUTATION OF THE T RAJECTORIESIn Section4.1,we break the global optimization of several people’s trajectories into the estimation of optimal individual trajectories.In Section 4.2,we show how this can be performed using the classical Viterbi’s algorithm based on dynamic programming.This requires a motion model given in Section 4.3and an appearance model described in Section4.4,which combines a color model given in Section4.5 and a sophisticated estimation of the ground plane occu-pancy detailed in Section5.We partition the visible area into a regular grid of G locations,as shown in Figs.5c and6,and from the camera calibration,we define for each camera c a family of rectangular shapes A c1;...;A c G,which correspond to crude human silhouettes of height175cm and width50cm located at every position on the grid.4.1Multiple TrajectoriesRecall that we denote by L n¼ðL n1;...;L n TÞthe trajectory of individual n.Given a batch of T temporal frames I¼ðI1;...;I TÞ,we want to maximize the posterior conditional probability:PðL1¼l1;...;L Nül NÃj IÞ¼PðL1¼l1j IÞY NÃn¼2P L n¼l n j I;L1¼l1;...;L nÀ1¼l nÀ1ÀÁ:ð2ÞSimultaneous optimization of all the L i s would beintractable.Instead,we optimize one trajectory after theother,which amounts to looking for^l1¼arg maxlPðL1¼l j IÞ;ð3Þ^l2¼arg maxlPðL2¼l j I;L1¼^l1Þ;ð4Þ...^l Nüarg maxlPðL Nül j I;L1¼^l1;L2¼^l2;...Þ:ð5ÞNote that under our model,conditioning one trajectory,given other ones,simply means that it will go through noalready occupied location.In other words,PðL n¼l j I;L1¼^l1;...;L nÀ1¼^l nÀ1Þ¼PðL n¼l j I;8k<n;8t;L n t¼^l k tÞ;ð6Þwhich is PðL n¼l j IÞwith a reduced set of the admissiblegrid locations.Such a procedure is recursively correct:If all trajectoriesestimated up to step n are correct,then the conditioning onlyimproves the estimate of the optimal remaining trajectories.This would suffice if the image data were informative enoughso that locations could be unambiguously associated toindividuals.In practice,this is obviously rarely the case.Therefore,this greedy approach to optimization has un-desired side effects.For example,due to partly missinglocalization information for a given trajectory,the algorithmmight mistakenly start following another person’s trajectory.This is especially likely to happen if the tracked individualsare located close to each other.To avoid this kind of failure,we process the images bybatches of T¼100and first extend the trajectories that havebeen found with high confidence,as defined below,in theprevious batches.We then process the lower confidenceones.As a result,a trajectory that was problematic in thepast and is likely to be problematic in the current batch willbe optimized last and,thus,prevented from“stealing”somebody else’s location.Furthermore,this approachincreases the spatial constraints on such a trajectory whenwe finally get around to estimating it.We use as a confidence score the concordance of theestimated trajectories in the previous batches and thelocalization cue provided by the estimation of the probabil-istic occupancy map(POM)described in Section5.Moreprecisely,the score is the number of time frames where theestimated trajectory passes through a local maximum of theestimated probability of occupancy.When the POM does notdetect a person on a few frames,the score will naturallydecrease,indicating a deterioration of the localizationinformation.Since there is a high degree of overlappingbetween successive batches,the challenging segment of atrajectory,which is due to the failure of the backgroundsubtraction or change in illumination,for instance,is met inseveral batches before it actually happens during the10keptframes.Thus,the heuristic would have ranked the corre-sponding individual in the last ones to be processed whensuch problem occurs.FLEURET ET AL.:MULTICAMERA PEOPLE TRACKING WITH A PROBABILISTIC OCCUPANCY MAP2714.2Single TrajectoryLet us now consider only the trajectory L n ¼ðL n 1;...;L nT Þof individual n over T temporal frames.We are looking for thevalues ðl n 1;...;l nT Þin the subset of free locations of f 1;...;G;Hg .The initial location l n 1is either a known visible location if the individual is visible in the first frame of the batch or H if he is not.We therefore seek to maximizeP ðL n 1¼l n 1;...;L n T ¼l nt j I 1;...;I T Þ¼P ðI 1;L n 1¼l n 1;...;I T ;L n T ¼l nT ÞP ðI 1;...;I T Þ:ð7ÞSince the denominator is constant with respect to l n ,we simply maximize the numerator,that is,the probability of both the trajectories and the images.Let us introduce the maximum of the probability of both the observations and the trajectory ending up at location k at time t :Èt ðk Þ¼max l n 1;...;l nt À1P ðI 1;L n 1¼l n 1;...;I t ;L nt ¼k Þ:ð8ÞWe model jointly the processes L n t and I t with a hidden Markov model,that isP ðL n t þ1j L n t ;L n t À1;...Þ¼P ðL n t þ1j L nt Þð9ÞandP ðI t ;I t À1;...j L n t ;L nt À1;...Þ¼YtP ðI t j L n t Þ:ð10ÞUnder such a model,we have the classical recursive expressionÈt ðk Þ¼P ðI t j L n t ¼k Þ|fflfflfflfflfflfflfflfflffl{zfflfflfflfflfflfflfflfflffl}Appearance modelmax P ðL n t ¼k j L nt À1¼ Þ|fflfflfflfflfflfflfflfflfflfflfflfflfflfflfflfflffl{zfflfflfflfflfflfflfflfflfflfflfflfflfflfflfflfflffl}Motion modelÈt À1ð Þð11Þto perform a global search with dynamic programming,which yields the classic Viterbi algorithm.This is straight-forward,since the L n t s are in a finite set of cardinality G þ1.4.3Motion ModelWe chose a very simple and unconstrained motion model:P ðL n t ¼k j L nt À1¼ Þ¼1=Z Áe À k k À k if k k À k c 0otherwise ;&ð12Þwhere the constant tunes the average human walkingspeed,and c limits the maximum allowable speed.This probability is isotropic,decreases with the distance from location k ,and is zero for k k À k greater than a constantmaximum distance.We use a very loose maximum distance cof one square of the grid per frame,which corresponds to a speed of almost 12mph.We also define explicitly the probabilities of transitions to the parts of the scene that are connected to the hidden location H .This is a single door in the indoor sequences and all the contours of the visible area in the outdoor sequences in Fig.1.Thus,entrance and departure of individuals are taken care of naturally by the estimation of the maximum a posteriori trajectories.If there are enough evidence from the images that somebody enters or leaves the room,then this procedure will estimate that the optimal trajectory does so,and a person will be added to or removed from the visible area.4.4Appearance ModelFrom the input images I t ,we use background subtraction to produce binary masks B t such as those in Fig.4.We denote as T t the colors of the pixels inside the blobs and treat the rest of the images as background,which is ignored.Let X tk be a Boolean random variable standing for the presence of an individual at location k of the grid at time t .In Appendix B,we show thatP ðI t j L n t ¼k Þzfflfflfflfflfflfflfflfflffl}|fflfflfflfflfflfflfflfflffl{Appearance model/P ðL n t ¼k j X kt ¼1;T t Þ|fflfflfflfflfflfflfflfflfflfflfflfflfflfflfflfflfflffl{zfflfflfflfflfflfflfflfflfflfflfflfflfflfflfflfflfflffl}Color modelP ðX kt ¼1j B t Þ|fflfflfflfflfflfflfflfflfflfflffl{zfflfflfflfflfflfflfflfflfflfflffl}Ground plane occupancy:ð13ÞThe ground plane occupancy term will be discussed in Section 5,and the color model term is computed as follows.4.5Color ModelWe assume that if someone is present at a certain location k ,then his presence influences the color of the pixels located at the intersection of the moving blobs and the rectangle A c k corresponding to the location k .We model that dependency as if the pixels were independent and identically distributed and followed a density in the red,green,and blue (RGB)space associated to the individual.This is far simpler than the color models used in either [18]or [13],which split the body area in several subparts with dedicated color distributions,but has proved sufficient in practice.If an individual n was present in the frames preceding the current batch,then we have an estimation for any camera c of his color distribution c n ,since we have previously collected the pixels in all frames at the locations272IEEE TRANSACTIONS ON PATTERN ANALYSIS AND MACHINE INTELLIGENCE,VOL.30,NO.2,FEBRUARY2008Fig.4.The color model relies on a stochastic modeling of the color of the pixels T c t ðk Þsampled in the intersection of the binary image B c t produced bythe background subtraction and the rectangle A ck corresponding to the location k .。
FormabilityofSn-...
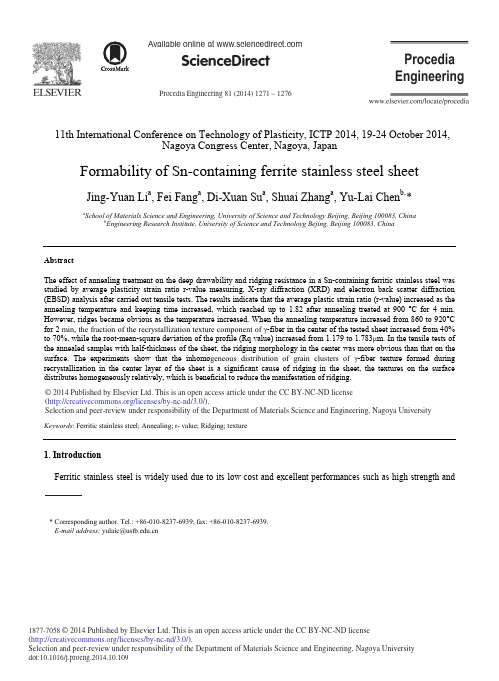
P rocedia Engineering 81 ( 2014 )1271 – 1276Available online at 1877-7058 © 2014 Published by Elsevier Ltd. This is an open access article under the CC BY-NC-ND license(/licenses/by-nc-nd/3.0/).Selection and peer-review under responsibility of the Department of Materials Science and Engineering, Nagoya University doi: 10.1016/j.proeng.2014.10.109ScienceDirect1272J ing-Yuan Li et al. / P rocedia Engineering 81 ( 2014 )1271 – 1276 high resistance to chloride stress corrosion. Hiraide et al. (2013) and Abratis et al. (2013) added Sn to ferritic stainless steel to improve the corrosion resistance of ferritic stainless steel. Han et al. (2013) studied the effect of Sn on the ferritic stainless steel. As a new type Cr-saving stainless steel, the Sn-containing ferritic stainless steel is expect to be employed as stampings such as kitchen equipments, home appliances and automotive assembly parts.So the deep drawing performance plays a decisive role on its application prospects. Thus, to get a product with excellent deep drawability and perfect surface is the objective of deep drawing works (Du et al., 2012).As is well known, plastic strain ratio (r-value) is an important index to reflect the maximum deformation degree during metal sheet forming process. However, even though the annealed sheet has a high r-value and good stamping performance, ridging still appears on the surface after stamping. Since 1960s, researchers have begun to study the mechanism of ridging in ferritic stainless steels during the sheet forming (Takechi et al., 1967). It is now accepted that the ridging phenomenon results from the different plastic flow of different texture components. In this paper we will investigate the influence of annealing process on the formability of the Sn-containing stainless steel and mechanism of the ridging phenomenon.2. Experimental procedureThe tested material was the cold-rolled ferritic stainless steel sheet with 0.6mm thickness, the chemical composition of which is shown in Table 1.Table 1. Chemical compositions of the tested steel (mass%.)C Si Mn S P Cr N Sn Fe0.01 0.58 0.17 0.001 0.019 16.51 0.008 0.09 BalThe tested sheet was annealed at 840, 860, 880, 900 and 920 °C for 2 or 4 min. Dog-bone samples were cut from the annealed sheets at 0º, 45º and 90º with respect to the rolling direction. Two samples were taken for each direction and the average value were calculated to be employed. The samples were drawn to 15% on MTS810 material testing machine. The r-value of each direction was determined as the ratio of the width and thickness plastic strain, and the average plastic strain ratio were calculated by r=(r0o+2×r45o+r90o)/4. The orientation distribution function (ODF) that reflected macro-texture was determined by X-Ray Diffraction, and the Root-mean-square deviation of the profile (Rq value) that reflected the surface roughness of the tensile samples was measured on surface profile Dektak machine.In order to investigate the difference of ridging morphologies, recrystallizations and textures between the surface and center of the sheets, the sheets were annealed at 860 °C for 2 min, 860 °C for 4 min and 920 °C for 2 min and then were grinded and mechanically polished to the mid-thickness of the sheet. The half-thickness samples were drawn to 20% elongation and the surface roughnesses were detected by Dektak machine. The micro-orientation distribution function sections on the original surface and the new surface (the original center of the sheet) were investigated by electron back scatter diffraction (EBSD) analysis, respectively. The measurement were carried out in a scanning electron microscopy (SEM) equipped with HKL-channel-5 system.3. Results and discussion3.1. r-value and Rq valueTable 2 shows the average plastic strain ratio, r-value and the surface roughness, Rq value of the annealed Sn-containing stainless steel sheets after 15% tensile. It can be seen that for both keeping time of 2 min and 4 min, the r-value exhibits a rising trend basically when the annealing temperature was below 900 °C. When the annealing temperature exceeded 900°C, r-value changed to decrease oppositely. The highest r-value appeared at 900 °C for 4 min, which was 1.82. The variety of Rq value exhibits approximately the same tend as that of r-value. It means that increase of r-value caused the increase of Rq value with the temperature rising and keeping time extension.1273J ing-Yuan Li et al. / P rocedia Engineering 81 ( 2014 ) 1271 – 1276 Table 2.r and Rq values of tested sheet after different annealing treatments.Fig. 1. XRD-determined orientation distribution function VHFWLRQV ZLWK FRQVWDQW ij VKRZLQJ WH[WXUH HYROXWLRQ DIWHU WUHDWHG DW (a) cold rolled state and (b) 840°C, (c) 860°C, (d) 880°C, (e) 900°C, (f) 920°C for 2min.For metals and alloys with bcc crystal structure, the r-value that can evaluate deep drawability has a close UHODWLRQ ZLWK GHQVLW\ RI Ȗ-fiber texture. The higher the intensity of {111} texture is, the greater the r-value is. The UHVXOW LQGLFDWHV WKDW WKH Ȗ-fiber texture strengthened and r-value increased as the recrystallization proceeds further. 0e 45e 90eØ Ø1 (a)(b) (c) (d)(e) (f) 7.97.8 4.0 4.06.5 3.0 8.5 8.88.41274 J ing-Yuan Li et al. / P rocedia Engineering 81 ( 2014 ) 1271 – 1276Fig. 2. Distribution of differently-recrystallized grains (a), (c) and (e) on surface and (b), (d) and (f) in center of the tested sheet after annealed at (a), (b) 860°C for 2 min, (c), (d) 860°C for 4 min and (e), (f) 920°C for 2 min.3.4. Micro-textureGLVWULEXWLRQ RI Į-ILEHU DQG Ȗ-fiber texture in the Sn-containing stainless steel sheet, which annealed at 860°C for 2min and 4min and 920°C for 2min, are shown in Fig.3, in which the dark red grains and blue grains represent the grains with Į-fiber and Ȗ-fiber texture, respectively, and the white ones are the grains with other orientations. The orientation was highlighted with a 15º tolerance. It can be seen that the blue Ȗ-fiber texture components increase with the increase of annealing temperature and extension of keeping time, especially in the center of sheet. In addition, the JUDLQV ZLWK WKH RULHQWDWLRQ RI Ȗ-fiber texture gathered together to form clustersRecrystallized Deformed Substructured1275J ing-Yuan Li et al. / P rocedia Engineering 81 ( 2014 ) 1271 – 1276LVWULEXWLRQ RI Į-fiber DQG Ȗ-fiber texture (a), (c) and (e) on surface and (b), (d) and (f) in center of the tested sheet after annealed at (a), (b) 860°C for 2 min, (c), (d) 860°C for 4 min and (e), (f) 920°C for 2 min.Fig. 4. Misorientation distribution in Ȗ-fiber texture cluster marked in Fig. 3.Similar to the conclusion of Du et al. (2012) and Gao et al. (2012), the result indicates that the proceeding of recrystallization is beneficial to WKH GHYHORSPHQW RI Ȗ-fiber texture, but it will lead to the increase in the number of coarse grains and inhomogeneous distribution of grains with different sizes, which disturbs the random distribution of grain orientations. Harase et al. (1991) and Raabe et al. (1995) have indicated that the strong Ȗ-fiber texture in the steel product can result in high surface quality during the sheet metal forming. Szpunar et al. (1994) also Į-fiber texture Ȗ-fiber texture AB CD EF1276J ing-Yuan Li et al. / P rocedia Engineering 81 ( 2014 )1271 – 1276 UHSRUWHG WKDW VWURQJ Ȗ-fiber texture component {111}<112> may not reduce the ridging morphology of the steel product. Furthermore, Vlad et al. (1988)found that ridging occurred in the ferritic stainless steel with only {111}<112> texture and fine grain structure. Similar to the result of Verbeken et al. (2003) and Chen et al. (2010), the samples annealed at 920°C for 2 minutes showed high r-value resulting from the coarse Ȗ-fiber grain clusters.The result in this study proves that there is a nearly opposite relationship between the deep drawability and the ridging resistance of the tested sheets. In other words, a strong Ȗ-fiber texture can lead to an excellent deep drawability while it does not certainly lead to high ridging resistance ability.4. Conclusion(1) In the Sn-containing ferritic stainless steel, the r-value increases with the proceeding of recrystallization andthe highest r-value appears after annealing at 900 °C for 4 min.(2) The inhomogeneously-GLVWULEXWHG VWURQJ Ȗ-fiber texture in the center of the sheet leads to more severe ridgingmorphology than on the surface. The homogeneous texture components on the surface are beneficial to reduce the ridging.(3) There is a nearly opposite relationship between the plastic stain ratio r-value and the ridging resistance in Sn-containing ferritic stainless steel sheet. A strong Ȗ-fiber texture can lead to an excellent deep drawability while it does not certainly lead to high ridging resistance abilityAcknowledgementsThe authors acknowledge the financial support from the National Natural Science Foundation of China (Grant 51174026) and the National Key Technology Research and Development Program of China (No.2012BAE04B02).ReferencesHiraide, N., Kajimura, H., Kimura, K.,2013. Stainless steel excellent in corrosion resistance, ferritic stainless steel excellent in resistance to crevice corrosion and formability, and ferritic stainless steel excellent in resistance to crevice corrosion: U.S. Patent 8,470,237.Abratis C., Hamburg D.E.; Lutz E., Krefeld D.E.; Wilfried K., Grevenbroich D.E., et al.,2013. Stainless steel, cold strip produced from this steel, and method for producing a flat steel product from this steel: U.S. Patent 8,608,873.Han, J.P., Li Y, Jiang Z.H., Yang Y CH., Wang X X., Wang L., et al., 2013. Summary of the Function of Sn in Iron and Steel. Advanced Materials Research, 773: 406-411.Du, W.,Jiang, L.Z.,Yu, H.F., Sun Q.S., 2012. 2012. Drawability of ferritic stainless steel, Bao-Steel Technology, (6):66-76.Takechi, H., Kato, H., Sunami, T., Nakayama, T., 1967. The mechanism of ridging formation in 17% chromium stainless steel sheets.Transactions of the Japan Institute of Metals, 8:233-239.Rajib, S., Ray, P K., 2007. Microstructural and textural changes in a severely cold rolled boron-added interstitial-free steel, Scripta Materialia, 57(9):841-844.Gao, F., Liu, Z. Y., Zhang, W. N., Liu, H. D., Sun G. T., Wang G. D., 2012. Textures and precipitates in a 17% ferritic stainless steels. Acta metallurgica sinica, 10(48):1166-1174.Harase, J., Ohta, K., Takeshita, T., Kawamo Y., 1991. Metallurgy for the Production of 17% Chromium Ferritic Stainless Steel Sheet Without Hot Band Annealing. Stainless Steels'9, 2: 856-863.Raabe, D., 1995. Textures of strip cast and hot rolled ferritic and austenitic stainless steel. Materials science and technology, 11(5): 461-468.Szpunar, J. A., Kim, H. M., 1994. Ridging phenomena in textured ferritic stainless steel sheets[C]//Materials Science Forum. 157: 753-760.Vlad, C. M., Dahms, M., Bunge, H. J., 1988. Proc. 8th Int, Conf, on ‘Textures of materials’ (ICOTOM8), 873-879.Verbeken, K., Kestens, L., Jonas, J. J., 2003. Microtextural study of orientation change during nucleation and growth in a cold rolled ULC steel.Scripta materialia, 48(10): 1457-1462.Chen, W. Y., Tong, W. P., Zhang, H., Zuo, L., 2010. Texure evolution and growth of differenty sized grains in IF steel during annealing. Acta metallurgica sinica, 09(46):1055-1060.。
IPCC_Special_Report_on_Carbon_Dioxide_Capture_and_Storage
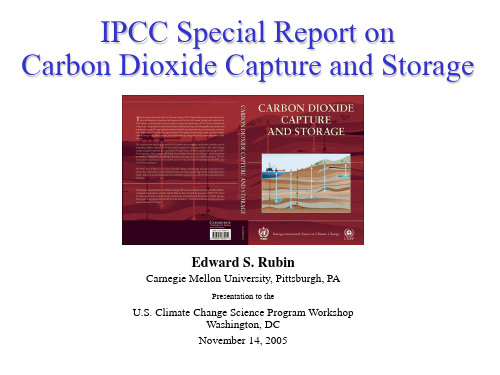
About IPCC Reports
Provide assessments of scientifically and technically sound published information No research, monitoring, or recommendations Authors are best experts available worldwide, reflecting experience from academia, industry, government and NGOs Policy relevant, but NOT policy prescriptive Thoroughly reviewed by other experts and governments Final approval of Summary by governments
IPCC SRCCS
(Source: Dakota Gasification
Pre-Combustion Capture
(coal gasification plant, USA)
E.S.Rubin, Carnegie Mellon
CO2 Pipelines (for EOR Projects)
Source: USDOE/Battelle
Structure of the Intergovernmental Panel on Climate Change (IPCC)
Plenary: All UNEP/WMO Member Countries ( >150 )
Review Editors
Working Groups I, II, III
Storage prospectivity
Manual Dish说明书
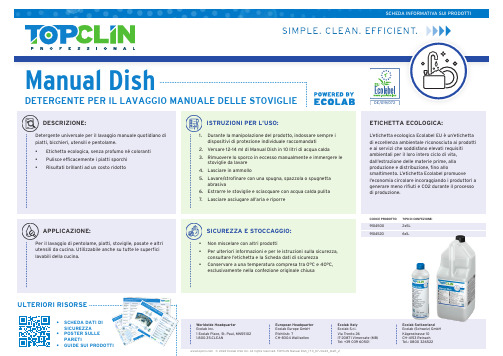
SICUREZZA E STOCCAGGIO:
• Non miscelare con altri prodotti • Per ulteriori informazioni e per le istruzioni sulla sicurezza,
consultare l’etichetta e la Scheda dati di sicurezza • Conservare a una temperatura compresa tra 0°C e 40°C,
APPLICAZIONE:
Per il lavaggio di pentolame, piatti, stoviglie, posate e altri utensili da cucina. Utilizzabile anche su tutte le superfici lavabili della cucina.
European Headquarter Ecolab Europe GmbH Richtistr. 7 CH-8304 Wallisellen
Ecolab Italy Ecolab S.r.l. Via Trento 26 IT-20871 Vimercate (MB) Tel: +39 039 60501
SCHEDA INFORMATIVA SUI PRODOTTI
SIMPLE. CLEAN. EFFICIENT.
Manual Dish
DETERGENTE PER IL LAVAGGIO MANUALE DELLE STOVIGLIE
DESCRIZIONE:
Detergente universale per il lavaggio manuale quotidiano di piatti, bicchieri, utensili e pentolame. • Etichetta ecologica, senza profumo né coloranti • Pulisce efficacemente i piatti sporchi • Risultati brillanti ad un costo ridotto
含有酯季铵盐和高碘酸盐的织物处理组合物[发明专利]
![含有酯季铵盐和高碘酸盐的织物处理组合物[发明专利]](https://img.taocdn.com/s3/m/e145a19e168884868662d641.png)
专利名称:含有酯季铵盐和高碘酸盐的织物处理组合物专利类型:发明专利
发明人:J·-P·格朗迈尔,L·莱特姆,A·埃尔莫西拉
申请号:CN200780016290.7
申请日:20070504
公开号:CN101437930A
公开日:
20090520
专利内容由知识产权出版社提供
摘要:可分散的含水织物处理组合物,例如织物柔软剂,其包括生物可降解的季铵织物柔软剂活性组分,其显示了优越的长期存储稳定性,甚至在高温长时间存储的情况下。
该组合物包括生物可降解的脂肪酯季铵化合物和高碘酸盐,例如高碘酸钾,所述季铵化合物来自烷醇胺和脂肪酸衍生物的反应,随后产物进行季铵化反应。
申请人:高露洁-棕榄公司
地址:美国纽约州
国籍:US
代理机构:中国专利代理(香港)有限公司
更多信息请下载全文后查看。
法国Carbios公司生物回收技术取得进展
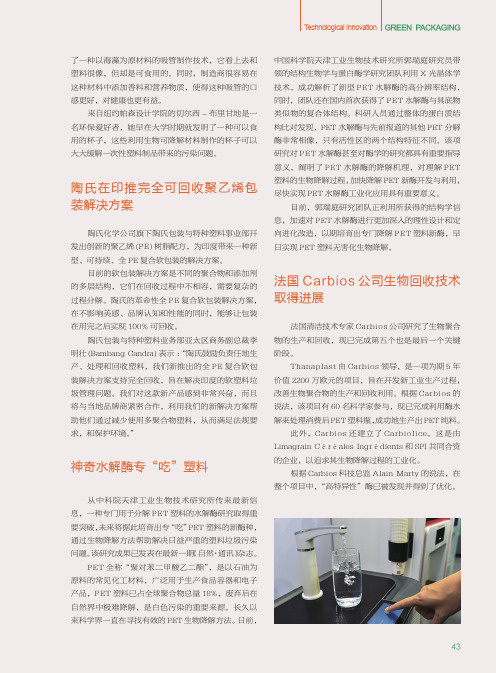
43T echnological Innovation了一种以海藻为原材料的吸管制作技术,它看上去和塑料很像,但却是可食用的。
同时,制造商很容易在这种材料中添加香料和营养物质,使得这种吸管的口感更好,对健康也更有益。
来自纽约帕森设计学院的切尔西-布里甘地是一名环保爱好者,她早在大学时期就发明了一种可以食用的杯子,这些利用生物可降解材料制作的杯子可以大大缓解一次性塑料制品带来的污染问题。
陶氏在印推完全可回收聚乙烯包装解决方案陶氏化学公司旗下陶氏包装与特种塑料事业部开发出创新的聚乙烯(PE)树脂配方,为印度带来一种新型、可持续、全PE 复合软包装的解决方案。
目前的软包装解决方案是不同的聚合物和添加剂的多层结构,它们在回收过程中不相容,需要复杂的过程分解。
陶氏的革命性全PE 复合软包装解决方案,在不影响美感、品牌认知和性能的同时,能够让包装在用完之后实现100%可回收。
陶氏包装与特种塑料业务部亚太区商务副总裁李明壮(Bambang Candra)表示:“陶氏鼓励负责任地生产、处理和回收塑料,我们新推出的全PE 复合软包装解决方案支持完全回收,旨在解决印度的软塑料垃圾管理问题。
我们对这款新产品感到非常兴奋,而且将与当地品牌商紧密合作,利用我们的新解决方案帮助他们通过减少使用多聚合物塑料,从而满足法规要求,和保护环境。
”神奇水解酶专“吃”塑料从中科院天津工业生物技术研究所传来最新信息,一种专门用于分解PET 塑料的水解酶研究取得重要突破,未来将据此培育出专“吃”PET 塑料的新酶种,通过生物降解方法帮助解决日益严重的塑料垃圾污染问题。
该研究成果已发表在最新一期《自然·通讯》杂志。
PET 全称“聚对苯二甲酸乙二酯”,是以石油为原料的常见化工材料,广泛用于生产食品容器和电子产品,PET 塑料已占全球聚合物总量18%,废弃后在自然界中极难降解,是白色污染的重要来源。
长久以来科学界一直在寻找有效的PET 生物降解方法。
- 1、下载文档前请自行甄别文档内容的完整性,平台不提供额外的编辑、内容补充、找答案等附加服务。
- 2、"仅部分预览"的文档,不可在线预览部分如存在完整性等问题,可反馈申请退款(可完整预览的文档不适用该条件!)。
- 3、如文档侵犯您的权益,请联系客服反馈,我们会尽快为您处理(人工客服工作时间:9:00-18:30)。
the absolute advantage and comparative advantage
the merits and demerits of international trade various instruments and reasons for protectionism policies
Quotas
•
•
•
Quota is a legal limit on the number of units of a particular commodity that can be imported into the country. Example : Malaysian government decides to limit import of television sets from Japan to 10,000 units. Quota can reduce the supply of a commodity and raise the price of the import for domestic consumers.
Country
Malaysia China
Cotton
Rice
0.17 (10/60) 6 (60/10) 0.5 (10/20) 2 (20/10)
Calculation of opportunity cost
• •
From the table, Malaysia has lower opportunity cost (0.17 tons of rice) in the production of cotton.
Sharing of knowledge and technology
•
•
Through international trade,there will also be a sharing of knowledge,information and technology by countries. Example : if Malaysia imports new technology-based machinery from Japan, then both countries can share the technology.
Comparative Advantage
•
•
Refers to ability of one country to produce goods at a lower opportunity cost than another country.Opportunity cost is the cost of the desired goods that have to be forgone to obtain another good. The following table shows the production of cotton and rice by China and Malaysia.
the components in the balance of payment
exchange rate and explain the effects of appreciation and depreciation of exchange rate to export and import
•
• • •
Only two goods are produced
Free trade exists between these two countries
•
No transportation costs are involved.
•
•
•
•
•
The table shows the production of cotton and rice by Malaysia and China. Malaysia uses 50% of the given resources to produce cotton and rice. China uses 50% of the given resources to produce cotton and rice. Malaysia can produce 20 tons of cotton and 60 tons of rice China can produce 40 tons of cotton and 20 tons of rice.
China has lower opportunity cost (2 tons of cotton) in the production of rice.
•
Comparative Advantage
•
•
Malaysia has to sacrifice only 0.17 tons of rice to produce 1 ton of cotton while China has to forgo 0.5 tons of rice.It is better for Malaysia to produce cotton since it has lower opportunity cost than China. In terms of rice, China has to give up 2 tons of cotton.Malaysia has to give up 6 tons of cotton.So, China should produce rice.
Country Malaysia China
Total
Cotton 20 40
60
Rice 60 20
80
•
From the table, Malaysia has an absolute advantage in producing rice and China has absolute advantage in producing cotton.
Absolute advantage
•
the ability to produce a good more efficiently using fewer inputs than another producer
Assumptions:
There are only two countries in the world
Explain the components in the balance of payment
Define exchange rate and explain the effects of appreciation and depreciation of exchange rate to export and import
Protectionism
•
Even though international trade brings a greater increase in world output, most countries have restrictions to protect local products from foreign competition.
Last Updated:1 August 2018
© LMS SEGi education group
3
International Trade
•
•
Exports: goods produced domestically and sold abroad Imports: goods produced abroad and sold domestically
Advantages of international trade
•
Relationship between trading partners
•
•
The relationship between trading partners can be improved. Example : economic co-operation (ASEAN,EU)
Tools of Protectionism
•
Tariffs
•
A tariff is a tax imposed by the government on imports.Tariff refers to taxes on imports.Tariff will raise the price of imports for domestic consumption.
ห้องสมุดไป่ตู้
Advantages of international trade
•
Increased world output
•
World output increases through specialization and trade.
Advantages of international trade
•
Varieties of goods and services • There are varieties of goods and services available for consumption. Consumers can enjoy some of the goods which cannot be produced in their country due to geographical and other reasons. • Example : apples and oranges
Calculation of opportunity cost
Opportunity cost in the production of rice Malaysia : 1 ton of rice cotton/rice = 60/10 =6 China : 1 ton of rice cotton/rice = 20/10 = 2