Performance Comparison of Face Recognition using Transform Domain Techniques
基于人脸跟踪与识别的行人闯红灯取证系统的设计与实现
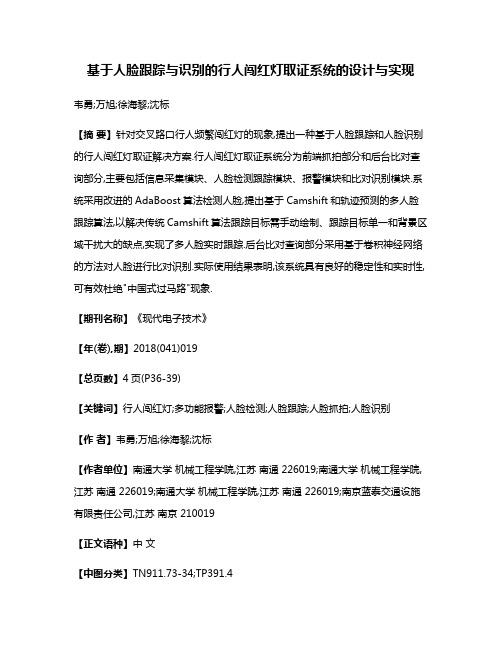
基于人脸跟踪与识别的行人闯红灯取证系统的设计与实现韦勇;万旭;徐海黎;沈标【摘要】针对交叉路口行人频繁闯红灯的现象,提出一种基于人脸跟踪和人脸识别的行人闯红灯取证解决方案.行人闯红灯取证系统分为前端抓拍部分和后台比对查询部分,主要包括信息采集模块、人脸检测跟踪模块、报警模块和比对识别模块.系统采用改进的AdaBoost算法检测人脸,提出基于Camshift和轨迹预测的多人脸跟踪算法,以解决传统Camshift算法跟踪目标需手动绘制、跟踪目标单一和背景区域干扰大的缺点,实现了多人脸实时跟踪.后台比对查询部分采用基于卷积神经网络的方法对人脸进行比对识别.实际使用结果表明,该系统具有良好的稳定性和实时性,可有效杜绝"中国式过马路"现象.【期刊名称】《现代电子技术》【年(卷),期】2018(041)019【总页数】4页(P36-39)【关键词】行人闯红灯;多功能报警;人脸检测;人脸跟踪;人脸抓拍;人脸识别【作者】韦勇;万旭;徐海黎;沈标【作者单位】南通大学机械工程学院,江苏南通 226019;南通大学机械工程学院,江苏南通 226019;南通大学机械工程学院,江苏南通 226019;南京蓝泰交通设施有限责任公司,江苏南京 210019【正文语种】中文【中图分类】TN911.73-34;TP391.40 引言近年来,随着城市道路交通的迅速发展,机动车的数量日益增多。
在我国城市交叉路口,行人过马路闯红灯的现象较为常见,尤其当群体行人数量达到4人时,最容易激发人群的集体闯红灯行为,这种行为被称为“中国式过马路”[1⁃4]。
行人闯红灯的行为不仅会威胁人身安全,还会诱发交通事故,影响交通秩序。
为了提高城市交通秩序、保障人民群众的生命安全、倡导文明出行,需要对行人闯红灯行为进行检测识别,并做出一些相应的提醒和处罚措施,提高行人在道路交通中的自觉意识[5⁃6]。
本文提出了基于人脸跟踪和识别技术的行人闯红灯取证系统的解决方案,用信息化、智能化的手段加强对城市道路交通的管理,推动智慧交通、智慧城市的建设。
英语作文他的脸很圆滑

His face is remarkably round and smooth,a feature that often draws attention and can be described in various ways depending on the context of the essay.Here are some points to consider when writing an essay about someone with a round and smooth face:1.Physical Description:Begin by describing the persons facial features.Mention the roundness of the face,the smoothness of the skin,and how these features contribute to their overall appearance.2.First Impressions:Discuss the impact of his round and smooth face on first impressions.People might perceive him as friendly,approachable,or youthful due to the softness of his features.3.Personality Traits:Explore the possible correlation between his facial features and his personality.For example,if he is known for his diplomatic skills or for being a peacemaker,you could suggest that his round and smooth face might symbolize these qualities.4.Cultural Significance:Depending on the cultural context,round and smooth faces might have different meanings.In some cultures,a round face is associated with good fortune or wealth,while in others,it might be seen as a sign of health and happiness.5.Daily Life:Describe how his round and smooth face might affect his daily interactions. For instance,it could make him more memorable to others,or it might influence the way people approach him in social or professional settings.6.Personal Care:Mention how he might take care of his skin to maintain its smoothness. This could include his skincare routine,diet,or lifestyle habits that contribute to his facial appearance.7.Challenges and Advantages:Address any challenges he might face due to his round and smooth face,such as stereotypes or misconceptions.Conversely,discuss the advantages,such as a perceived trustworthiness or attractiveness.8.Personal Narrative:If the essay is more personal,include anecdotes or stories that illustrate the significance of his facial features in his life.This could be a funny incident, a moment of recognition,or a personal triumph.9.Conclusion:End the essay by reflecting on the significance of his round and smooth face in shaping his identity and how it has influenced his life experiences.Remember to use descriptive language and vivid imagery to bring the persons face to life in your writing.This will help the reader to visualize the subject and understand the impact of his facial features on his life and the lives of those around him.。
特征脸算法人脸识别系统

第3"卷第2期2018年6月金陵科技学院学报JOURNAL OF JINLING INSTITUTE OF TECHNOLOGYVol. 3"! No.2June,2018D O I: 10.16515#.cnki.32-1722/n.2018.02.0001特征脸算法人脸识别系统胡勇,朱莹莹(金陵科技学院软件工程学院,江苏南京211169)摘要:人脸识别技术,是指对给定的一个人脸图像,从存储的已知身份的人脸图像库中识别出该人身份的一种技术。
其主要应用于证件验证、刑侦破案、门禁系统、视频监控等领域。
实现了基于特征脸算法的人脸识别系统,在实验中采用的人脸图像数据库为E SSE X大学的F A C E S9"人脸图像数据库。
该系统的优点是识别速度快、准确率高,具有一定的实际应用价值。
关键词:人脸识别(特征脸;F A C E S9"人脸图像数据库;算法中图分类号:T P391.1文献标识码:A文章编号"672 - 755X(2018)02 - 0001 - 0"Face Recognition System Based on Eigenface AlgorithmHU Yong,ZHU Ying-ying(Jingling Institute of Technology,N anjing211169, China)Abstract:Face recognition is a technique that identifies a person!s identity from a given face image in a face image database.It is widely used in the fields of certificate veri investigation,access control system,video surveillance and so on.A face recognition systembased on the eigenface algorithm is implemented in this paper.The database used in the experiment is the FACES94 face image database of ESSEX University.The proposed system has theadvantages of high recognition rate,high accuracy rate and practical application value.Key words:face recognition;eigenface;FACES9" face image database;algorithm树林里没有两片一模一样的树叶,人世间也没有两张一模一样的面孔。
基于AdaBoost的人脸识别
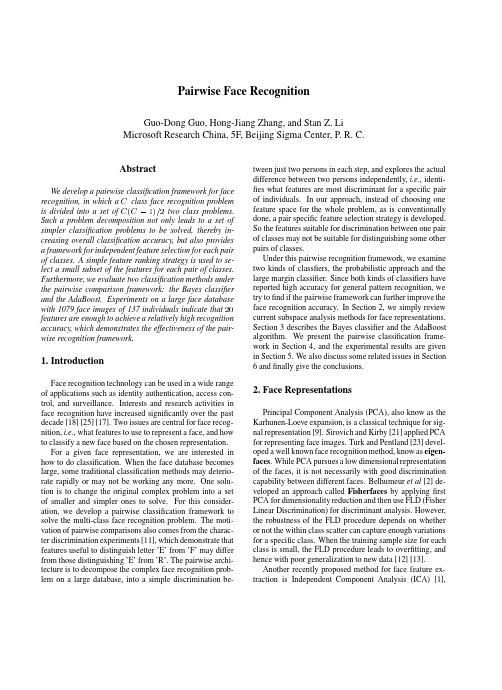
人脸识别外文文献

Method of Face Recognition Based on Red-BlackWavelet Transform and PCAYuqing He, Huan He, and Hongying YangDepartment of Opto-Electronic Engineering,Beijing Institute of Technology, Beijing, P.R. China, 10008120701170@。
cnAbstract。
With the development of the man—machine interface and the recogni—tion technology, face recognition has became one of the most important research aspects in the biological features recognition domain. Nowadays, PCA(Principal Components Analysis) has applied in recognition based on many face database and achieved good results. However, PCA has its limitations: the large volume of computing and the low distinction ability。
In view of these limitations, this paper puts forward a face recognition method based on red—black wavelet transform and PCA. The improved histogram equalization is used to realize image pre-processing in order to compensate the illumination. Then, appling the red—black wavelet sub—band which contains the information of the original image to extract the feature and do matching。
任意光照下人脸图像的低维光照空间表示

Vol.33,No.1ACTA AUTOMATICA SINICA January,2007 A Low-dimensional Illumination Space Representation ofHuman Faces for Arbitrary Lighting ConditionsHU Yuan-Kui1WANG Zeng-Fu1Abstract The proposed method for low-dimensional illumination space representation(LDISR)of human faces can not only synthesize a virtual face image when given lighting conditions but also estimate lighting conditions when given a face image.The LDISR is based on the observation that9basis point light sources can represent almost arbitrary lighting conditions for face recognition application and different human faces have a similar LDISR.The principal component analysis(PCA)and the nearest neighbor clustering method are adopted to obtain the9basis point light sources.The9basis images under the9basis point light sources are then used to construct an LDISR which can represent almost all face images under arbitrary lighting conditions. Illumination ratio image(IRI)is employed to generate virtual face images under different illuminations.The LDISR obtained from face images of one person can be used for other people.Experimental results on image reconstruction and face recognition indicate the efficiency of LDISR.Key words LDISR,basis image,illumination ratio image,face recognition1IntroductionIllumination variation is one of the most important fac-tors which reduce significantly the performance of face recognition system.It has been proved that the variations between images of the same face due to illumination are almost always larger than image variations due to change in face identity[1].So eliminating the effects due to illumi-nation variations relates directly to the performance and practicality of face recognition system.To handle face image variations due to changes in ligh-ting conditions,many methods have been proposed thus far.Generally,the approaches to cope with variation in appearance due to illumination fall into three kinds[2]: invariant features,such as edge maps,imagesfiltered with2D Gabor-like functions,derivatives of the gray-level image,images with Log transformations and the re-cently reported quotient image[3]and self-quotient image[4]; variation-modeling,such as subspace methods[5∼7],illumi-nation cone[8∼10];and canonical forms,such as methods in [11,12].This paper investigates the subspace methods for illumi-nation representation.Hallinan et al.[5,6]proposed an eigen subspace method for face representation.This method firstly collected frontal face images of the same person un-der different illuminations as training set,and then used principal component analysis(PCA)method to get the eigenvalues and eigenvectors of the training set.They concluded that5±2eigenvectors would suffice to model frontal face images under arbitrary illuminations.The ex-perimental results indicated that this method can recon-struct frontal face images with variant lightings using a few eigenvectors.Different from Hallinan,Shashua[7]pro-posed that under the assumption of Lambertian surface, three basis images shot under three linearly independent light sources could reconstruct frontal face images under arbitrary lightings.This method was proposed to discount the lighting effects but not to explain lighting conditions. Belhumeur et al.[8,9]proved that face images with the same pose under different illumination conditions form a convex cone,called illumination cone,and the cone can be repre-sented in a9dimensional space[10].This method performs well but it needs no less than seven face images for each Received January11,2006;in revised form March28,2006 Supported by Open Foundation of National Laboratory of Pattern Recognition,P.R.China.1.Department of Automation,University of Science and Technol-ogy of China,Hefei230027,P.R.ChinaDOI:10.1360/aas-007-0009person to estimate the3D face shape and the irradiance map.Basri&Jacobs[13]and Ramamoorthi[14,15]indepen-dently applied the spherical harmonic representation and explained the low dimensionality of differently illuminated face images.They theoretically proved that the images of a convex Lambertian object obtained under a wide variety of lighting conditions can be approximated accurately with a 9D linear subspace,explaining prior empirical results[5∼7]. However,both of them assumed that the3D surface normal and albedo(or unit albedo)were known.This assumption limits the application of this algorithm.The above research results theoretically and empirically indicate that frontal face images obtained under a wide variety of lighting conditions can be approximated accu-rately with a low-dimensional linear subspace.However, all the above subspace methods construct a subspace from training images for each human face,which is not only cor-responding to the illumination conditions but also to the face identity.The subspaces,in which the intrinsic infor-mation(shape and albedo)and the extrinsic information (lightings)are mixed,are not corresponding to the lighting conditions distinctly.Otherwise,a large training image set would be needed in the learning stage and3d face model might be needed.In this paper,a low-dimensional illumination space rep-resentation(LDISR)of human faces for arbitrary lighting conditions is proposed,which can handle the problems that can not be solved well in the existing methods to a certain extent.The key idea underlying our model is that any lighting condition can be represented by9basis point light sources.The9basis images under the9basis point light sources construct an LDISR,which separates the intrinsic and the extrinsic information and can both estimate ligh-ting conditions when given a face image and synthesize a virtual face image when given lighting condition combin-ing with the illumination ratio image(IRI)method.The method in[10]and the proposed method in this paper have some similarities,but they have some essential differences also.The former needs to build one subspace for each per-son,and the latter only needs to build one subspace for one selected person.Furthermore,the9D illumination space built in the former case is not corresponding to the lighting conditions distinctly,and in our case once the correspon-ding illumination space is built,it can be used to generate virtual frontal face images of anybody under arbitrary illu-minations by using the warping technology and IRI method developed.These virtual images are then used for the pur-pose of both training and recognition.The experiments onc 2007by Acta Automatica Sinica.All rights reserved.10ACTA AUTOMATICA SINICA Vol.33 Fig.1The positions corresponding to the dominant pointlight sourcesYale Face Database B indicate that the proposed methodcan improve the performance of face recognition efficiently.2Constructing the LDISRSince any given set of lighting conditions can be exactlyexpressed as a sum of point light sources,a surface patch sradiance illuminated by two light sources is the sum of thecorresponding radiances when the two light sources are ap-plied separately.More detail was discussed in[5].In thissection,PCA and clustering based method are adopted tofind the basis point light sources,which are able to repre-sent arbitrary lighting conditions.The needed3D face model was obtained using a3D ima-ging machine3DMetrics TM.Then the3D face model ob-tained was used to generate the training images.Moveafloodlight by increments of10degrees to each position(θi,ϕj)to generate image p(θi,ϕj),whereθis the eleva-tion andϕis the azimuth.Typicallyϕ∈[−120◦,120◦]andθ∈[−90◦,90◦].Totally,427images were generated,denoted as{pk ,k=1,···,427}.We use PCA tofind the dominant components for the finite set of images.Since the PCA is used on the images of the same human face with different lighting conditions, the dominant eigenvectors do not reflect the facial shape but the lighting conditions.So the above eigenvectors can be used to represent lighting conditions.In this paper,the lighting subspace is constructed not using the eigenvectors directly but the light sources corresponding to the eigen-vectors.According to the ratio of the corresponding eigen-value to the sum of all the eigenvalues,thefirst60 eigenvalues containing the99.9%energy were selected. And the60corresponding eigenvectors were selected as the principal components.Denote thefirst60eigenvectors as{u i,i=1,···,60}.For the i th eigenvector u i,thecorresponding training image is pj ,where u i and pjsatisfyu T i pj =maxk∈{1, (427){u T i pk}(1)The positions of the60dominant point light sources are shown in Fig.1.By investigating the positions of the dominant point light sources,it can be found that the dominant point light sources are distributed by certain rules.They are distributed almost symmetrically and cluster together in regions such as the frontal,the side,the below,and the above of head.The nearest neighbor clustering method is adopted here to get the basis light positions.Considering the effects of point light sources in different elevation and azimuth,some rules are employed for clustering:1.When the elevation is below−60◦or above60◦,clus-tering is done based on the differences of values in elevation.2.When the elevation is in range[−60◦,60◦],clusteringis donebased on the Euclidian distances in space.Fig.2The clustering result of thefirst60eigenvectors.By adopting the nearest nerghbor clustering method,the 60dominant light sources can be classified into9classes. The clustering result is shown in Fig.2.When the geometric center of each class is regarded as the basis position,the9 basis light positions are shown in Table1.From the above procedure,it is known that point light sources in the9basis positions are dominant and princi-pal components in the lighting space,and they can express arbitrary lighting conditions.The9basis images obtained under the9basis point light sources respectively construct a low-dimensional illumination space representation(LD-ISR)of human face,which can express frontal face images under arbitrary illuminations.Because different human faces have similar3D shapes[3,16],the LDISR of different faces is also similar.As an approximation,it can be as-sumed that different persons have the same LDISR,which has been discussed in[17].Denote the9basis images obtained under9basis lights are I i,i=1,···,9,the LDISR of human face can be de-noted as A=[I1,I2,···,I9].The face image under lighting s x can be expressed asI x=Aλλ(2) whereλ=[λ1,λ2,···,λ9]T,0≤λi≤1is the lighting pa-rameters of image I x and can be calculated by minimizing the energy function E(λ):E(λ)= Aλλ−I x 2(3) So we can getλ=A+I x(4) whereA+=(A T A)−1A T(5)No.1Hu Yuan-Kui and WANG Zeng-Fu:A Low-dimensional Illumination Space Representation of (11)Table1Positions of the9basis light sourceslight123456789Elevationθ(degree)017.525.7364468.6-33.3-35-70Elevationϕ(degree)0-47.544.4-10888-385-9522.5(a)Input image(b)ASM alignment(c)Warped mean shape(d)The virtual images generated under different lightingsFig.3Generating virtual images using the9D illuminationspace and the IRIGiven an image of human face for learning images,thelighting parametersλcan be calculated by(4),and thevirtual face images can be generated by(2)by using thelighting conditionλ.In order to use the LDISR learnedfrom one human face to generate virtual images of other hu-man faces,the illumination ratio image(IRI)based methodis adopted in next section.3Generating virtual images u-sing illumination ratio-image(IRI)Denote the light sources as s i,i=0,1,2,···,respec-tively,where s0is the normal light source,and I ji the imageunder light source s i for the person with index j.The IRIis based on the assumption that a face is a convex surfacewith a Lambertian function.A face image can be describedasI(u,v)=ρ(u,v)n(u,v)·l(6)where,ρ(u,v)is the albedo of point(u,v),n(u,v)is thesurface normal at(u,v),and l is the direction of light.Different from the quotient image[3],illumination ratioimage is defined as follows[11,18,19,20].R i(u,v)=I ij(u,v)I0j(u,v)(7)From(6)and(7),we haveR i(u,v)=ρj(u,v)n T(u,v)·s iρj(u,v)n T(u,v)·s0=n T(u,v)·s in T(u,v)·s0(8)Equation(8)shows that the IRI can be determined only by the surface normal of a face and the light sources,which is independent of specific albedo.Since different human faces have the similar surface normal[3,16],the IRIs of dif-ferent people under the same lighting condition can be con-sidered to be the same.In order to eliminate the effect due to shapes of different faces,the following procedure should be done.Firstly,all faces can be warped to the same shape, and then the IRI is computed.In this paper,an ASM based method is used to perform the face alignment and all faces will then be warped to the predefined mean shape.After the procedure,all faces will have a quite similar3D shape. That is to say,with the same illumination,IRI is the same for different people.The corresponding face image under arbitrary lighting condition can be generated from the IRI. Finally the face image is warped back to its original shape.From(7),we haveI ij(u,v)=I0j(u,v)R i(u,v)(9)Equation(9)means that,given the IRI under s i and the face image under the normal lighting,we can relight the face under s i.The face relighting problem can be defined as follows. Given one image,I a0,of people A under the normal ligh-ting s0,and one image,I bx,of another people B under some specific lighting s x,how to generate the image,I ax, of people A under lighting S x.Unlike[11,18],the IRI under each lighting is unknown in this paper.Given image I bx,the IRI under lighting s x can be calcu-lated using the LDISR described in Section2.Assume the LDISR,A,is learned from images of people M.The ligh-ting parameter,λx,of image I bx is solved by the least-square methodA T Aλλx=A T I bx(10) Aλλx is the image of people M under lighting s x,denoted as I mx.The IRI under lighting s x can be calculated byR x(u,v)=I xm(u,v)/I0m(u,v)(11)where I0m is the image of people M under normal lighting. After the IRI under lighting s x is calculated,the face image of people A can be relit under lighting s x by I xa(u,v)= I0a(u,v)R x(u,v).In general,given face image I0y of arbitrary face Y under lighting s0,face image of Y under arbitrary lighting can be generated by the following procedure:1.Detect face region I0y and align it using ASM;2.Warp I0y to the mean shape T0;3.Relight T0using the IRI under lighting s k:T k(u,v)=T0(u,v)R k(u,v);4.Reverse-warp the texture T k to its original shape toget the relit image I kyFig.3shows some relighting results on Yale Face Database B.In the experiments,the LDISR was con-structed by the nine basis images of people DZF(not in-cluded in Yale Face Database B).For each image under12ACTA AUTOMATICA SINICA Vol.33abec fd gFig.4Results of image reconstruction.a)Original images.b)Images reconstructed by 5-eigenimages.c)Images reconstructed by 3-basis images.d)Images reconstructed by the LDISR.e)The differences corresponding to the images in b).f)The differences corresponding to the images in c).g)The differences corresponding to the images in d).normal lighting in Yale Face Database B,the virtual im-ages under other 63lightings were generated.It should be highlighted that in the original IRI method [11,18],to calculate the IRI,the image under nor-mal lighting and the image under specific lighting must be of the same people.The LDISR based method proposed in this paper breaks this limitation and the face image used in the algorithm can be of different people.In addition,when no face image under normal lighting is available,the virtual image can be generated by using the given λx from (2).And the IRI will then be calculated according to the virtual image.4Experimental results4.12D image reconstructionThe experiment was based on the 427frontal face images under different lightings described in Section 2.In this experiment,three image reconstruction methods were im-plemented:5-eigenimages representation method proposed by Hallinan [5],a linear combination of 3-basis images pro-posed by Shashua [7],and the LDISR based method.The face images under different lightings were reconstructed and the performances were evaluated by the differences between the original and the reconstructed images.According to [5],PCA was adopted to train the 427images and the eigenvectors corresponding to the first 5eigenvalues were selected to construct face illumination sub-space I.According to [7],the selected 3basis images under three point light sources respectively were used to construct face illumination subspace II.The LDISR constructed by the nine basis images was the face illumination subspace III.The total 427face images were reconstructed by the three face illumination subspace,respectively.Some original images are shown in Fig.4a),and the images reconstructed using face illumination I,II,III are shown in Fig.4b),c),and d),respectively.The corre-sponding differences are shown in Fig.4e),f),and g),respectively.It can be concluded from Fig.4that the performances of the 5-eigenimages representation method and the LDISR are comparative,and they are both better than that of the 3-basis images representation method.When the variation due to lighting condition is large (Fig.4c),columns 2,3,and 4),the differences between the original and the recon-structed images are very large (Fig.4f),columns 2,3,and 4),especially when there are shadows in face images.To evaluate more rigorously,the fit function defined in [5]was adopted.The quality of the reconstruction can be measured by the goodness of the fit function:ε=1−I rec −I in 2I in 2(12)where I rec is the reconstructed image,and I in is the original image.The values of the fit function corresponding to all the 427reconstructions by three methods are shown in Fig.5.From Fig.5,it can be seen that the fitness of images reconstructed by the 5-eigenimages representation method and the LDISR to the original image is very good,while the 3-basis images representation method is not so good.When the variation in lighting is larger (corresponding to the abscissas are 50and 280in Fig.5)the performance of the LDISR is better than that of the 5-eigenimages repre-sentation method.Besides,the 5-eigenimages and the 3-basis images rep-resentation methods need multiple images of each person,and train one model for each person.However,the LDISR trains one model using 9basis images of one person,and can be used for other person by warping technique.4.2Face recognition with variant lightings based on virtual imagesIn this experiment,the LDISR and the IRI method were combined to generate virtual face images,which were used for face recognition with variant lightings.The experiments were based on the Yale Face Database B [10].64frontal face images of each person under 64different lightings were selected,and there were 640images of 10persons.TheNo.1Hu Yuan-Kui and WANG Zeng-Fu:A Low-dimensional Illumination Space Representation of ···13Fig.5The values of fit function corresponding to thereconstruction by three methods.images have been divided into five subsets according to the angles the light source direction makes with the camera axis [10]:Subset 1(up to 12◦),subset 2(up to 25◦),subset 3(up to 50◦),subset 4(up to 77◦),and subset 5(others).Correlation,PCA,and LDA methods were adopted for face recognition.For correlation method,the image under normal lighting of each person was the template image and the rest 63images of each person were test images.For PCA and LDA methods,three images of each person (of which the angles the light source direction makes with the camera axis are the smallest)were training images,and the rest were test images.The LDISR was constructed by the nine basis images of people DZF (not included in Yale Face Database B).For each frontal face image in Yale Face Database B,the virtual images corresponding to the other 63lightings were gener-ated using the LDISR and IRI.In order to decrease the effect of illumination,we used gamma intensity correction (GIC).Here γ=4.The three recognition methods were performed on the original images,images with GIC and virtual images with GIC.The results are shown in Fig.6,where correlation,PCA and LDA correspond to the results for the original images,GIC correlation,GIC PCA,and GIC LDA correspond to the results for the images with GIC,and GIC virtual correlation,GIC virtual PCA,and GIC virtual LDA correspond to the results for the virtual images with GIC.Fig.6illustrates that the recognition accuracy for the virtual images is improved greatly.When the variations due to illumination are larger,the improvement is greater.The recognition rates of correlation,PCA,and LDA on the virtual images are 87.24%,87.99%,and 90.5%,respec-tively.For subset 1,subset 2,and subset 3,in which the variations due to illumination are small,the performance of three recognition methods are comparable,while in sub-set 4and subset 5,LDA performs better.This indicates that the classifying ability of LDA is better than others.In the future,we will validate the proposed method on larger face database.5ConclusionThis paper proposes a method to construct an LDISR u-sing the 9basis images under the 9basis point light sources.The LDISR can represent almost all face images underar-Fig.6The results of Face recognition on Yale face Database Bbitrary lighting conditions.The LDISR combined with the IRI is corresponding to the lighting conditions distinctly,and can estimate lighting conditions when given a face im-age and synthesize a virtual face image when given lighting conditions.The experiments of reconstruction illustrate that the representation ability of LDISR is better than the 5-eigenimages and 3-basis images representation methods.The experiments on Yale Face Database B confirm the abi-lity of LDISR in synthesizing a virtual face image and in-dicate that the virtual face images can improve greatly the accuracy of face recognition under variant lightings.The main advantage of the proposed model is that it can be used to generate virtual images of anybody only from 9basis face images of one person.And at the same time,the method need not know the lighting conditions or pre-calculate the IRI.References1Moses Y,Adini Y,Ullman S.Face recognition:the problem of compensating for changes in illumination direction.In:Pro-ceedings of the Third European Conference on Computer Vision.Stockholm,Sweden.Springer-Verlag,1994,286∼2962Sim T,Kanade T,Combining models and exemplars for face recognition:An illuminating example,In:Proceedings of CVPR 2001Workshop on Models versus Exemplars in Computer Vi-sion,Hawaii,USA.IEEE,2001,1∼103Shashua A,Riklin-Raviv T,The quotient image:Class-based re-rendering and recognition with varying illuminations.IEEE Transactions on Pattern Analysis and Machine Intelligence ,2001,23(2):129∼1394Wang H,Li Stan Z,Wang Y.Face recognition under varying lighting conditions using self quotient image.In:Proceedings of the 6th International Conference on Automatic Face and Gesture Recognition,Seoul,Korea.IEEE,2004,819∼8245Hallinan P W.A low-dimensional representation of human faces for arbitrary lighting conditions.In:Proceedings of IEEE Com-puter Society Conference on Computer Vision and Pattern Recognition,Seattle,USA.IEEE,1994,995∼9996Epstein R,Hallinan P W,Yuille A L.5±2eigenimages suffice:an empirical investigation of low dimensional lighting models.In:Proceedings of IEEE Workshop on Physics-Based Vision,Boston,USA.IEEE,1995,108∼1167Shashua A.On photometric issues in 3D visual recognition from a single 2D image.International Journal of Computer Vision ,1997,21(1):99∼1228Belhumeur P,Kriegman D.What is the set of images of an object under all possible illumination conditions.International Journal of Computer Vision ,1998,28(3):245∼2609Georghiades A S,Belhumeur P N,Kriegman D J.From few to many:Illumination cone models for face recognition under variable lighting and pose.IEEE Transactions on Pattern Analysis and Machine Intelligence ,2001,23(6):643∼66014ACTA AUTOMATICA SINICA Vol.3310Lee K,Jeffrey Ho,Kriegman D.Nine points of light:Acquiring subspaces for face recognition under variable lighting.In:Pro-ceedings of IEEE Computer Society Conference on Computer Vision and Pattern Recognition,Hawaii,USA.IEEE,2001(1), 519∼52611Gao W,Shan S,Chai X,Fu X.Virtual face image generation for illumination and pose insensitive face recognition.In:Proceed-ings of IEEE International Conference on Acoustics,Speech,and Signal Processing,Hong Kong.IEEE,2003,776∼77912Zhao W,Chellappa R.Robust face recognition using symmetric shape-from-shading.Technical Report CARTR-919,1999,Cen-ter for Automation Research,University of Maryland,College Park,MD.13Basri R,Jacobs mbertian reflectance and linear subspaces, In:Proceedings of the8th IEEE Computer Society International Conference On Computer Vision,Vancouver,Canada.IEEE, 2001,2:383∼39014Ramamoorthi R,Hanrahan P.An efficient representation for ir-radiance environment maps.In:Proceedings of the28th Annual Conference on Computer Graphics and Interactive Techniques, Los Angeles,USA:ACM Press,2001,497∼50015Ramamoorthi R.Analytic PCA construction for theoretical analysis of lighting variability in images of a Lambertian object, IEEE Transactions on Pattern Analysis and Machine Intelligence, 2002,24(10):1322∼133316Chen H F,Belhumeur P N,Jacobs D W.In search of illumi-nation invariants.In:Proceedings of IEEE Computer Society Conference on Computer Vision and Pattern Recognition,South Carolina,USA.IEEE,2000,1:54∼26117Wang H,Li Stan Z,Wang Y,Zhang W,Illumination modeling and normalization for face recognition,In:Proceedings of IEEE International Workshop on Analysis and Modeling of Faces and Gestures,Nice,France.IEEE,2003,104∼11118Zhao J,Su Y,Wang D,Luo S.Illumination ratio image:Synthe-sizing and recognition with varying illuminations.Pattern Recog-nition Letters,2003,24(15):2703∼271019Wen Z,Liu Z,Huang T S.Face relighting with radiance envi-ronment maps.In:Proceedings of IEEE Computer Society Con-ference on Computer Vision and Pattern Recognition,Madison, USA.IEEE,2003,2:158∼16520Qing L,Shan S,Chen X.Face relighting for face recognition under generic illumination.In:Proceedings of IEEE Interna-tional Conference on Acoustics,Speech,and Signal Processing, Montreal,Canada.IEEE,2004,5:733∼736HU Yuan-Kui Ph.D.candidate in the De-partment of Automation at University of Sci-ence and Technology of China.His researchinterests include face recognition,image pro-cessing,and pattern recognition.W ANG Zeng-Fu Professor of the Depart-ment of Automation at University of Scienceand Technology of China.His current re-search interests include audio&vision in-formation processing,intelligent robots,andpattern recognition.Corresponding author ofthis paper.E-mail:zfwang@。
基于受试者工作特征pAUC_优化的人脸识别系统

第 21 卷 第 9 期2023 年 9 月太赫兹科学与电子信息学报Journal of Terahertz Science and Electronic Information TechnologyVol.21,No.9Sept.,2023基于受试者工作特征pAUC优化的人脸识别系统唐林瑞泽,白仲鑫,张晓雷*(西北工业大学航海学院,陕西西安710072)摘要:基于深度学习的人脸识别技术在大量应用场景中表现出优于传统方法的性能,它们的损失函数大致可分为2类:基于验证的和基于辨识的。
验证型损失函数符合开集人脸识别的流程,但实施过程比较困难。
因此目前性能较优的人脸识别算法都是基于辨识型损失而设计的,通常由softmax输出单元和交叉熵损失构成,但辨识型损失并没有将训练过程与评估过程统一起来。
本文针对开集人脸识别任务提出一种新的验证型损失函数,即最大化受试者工作特征(ROC)曲线下的部分面积(pAUC);同时还提出一种类中心学习策略提高训练效率,使提出的验证型损失和辨识型损失有较强的可比性。
在5个大规模非限定环境下的人脸数据集上的实验结果表明,提出的方法和目前性能最优的人脸识别方法相比,具有很强的竞争性。
关键词:人脸识别;部分面积优化;损失函数;类中心中图分类号:TP391.4 文献标志码:A doi:10.11805/TKYDA2021258Partial Area Under Curve optimization for face recognition systemTANG Linruize,BAI Zhongxin,ZHANG Xiaolei*(School of Marine Science and Technology,Northwestern Polytechnical University,Xi'an Shaanxi 710072,China)AbstractAbstract::Deep learning based face recognition has outperformed traditional methods in many application scenarios. There are two main lines of research to design loss functions for face recognition, i.e., verification and identification. The verification loss functions match the pipeline of open-set facerecognition, but it is hard to implement. Therefore, most state-of-the-art deep learning methods for facerecognition take the identification loss functions with softmax output units and cross-entropy loss.Nevertheless, identification loss function dose not match the training process with evaluation procedure.A verification loss function is proposed for open-set face recognition to maximize partial area under theReceiver-Operating-Characteristic(ROC) curve, partial Area Under Curve(pAUC). A class-centerlearning method is also proposed to improve training efficiency, which is critical for the proposed lossfunction to be comparable to the identification loss in performance. Experimental results on five largescale unconstrained face recognition benchmarks show that the proposed method is highly competitivewith state-of-the-art face recognition methods.KeywordsKeywords::face recognition;partial Area Under Curve optimization;loss function;class centers 目前使用深层卷积神经网络(Deep Convolution Neural Networks,DCNNs)的嵌入进行人脸表征是人脸识别的首选方法[1-4]。
gaussianface高斯脸 人脸识别
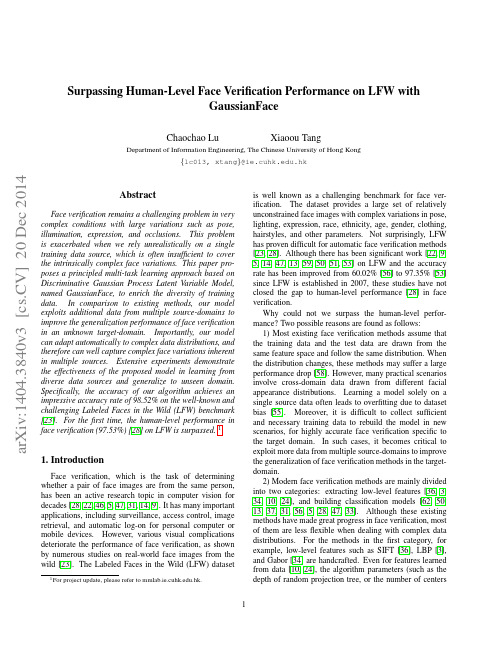
{lc013, xtang}@.hk
arXiv:1404.3840v3 [cs.CV] 20 Dec 2014
Abstract
Face verification remains a challenging problem in very complex conditions with large variations such as pose, illumination, expression, and occlusions. This problem is exacerbated when we rely unrealistically on a single training data source, which is often insufficient to cover the intrinsically complex face variations. This paper proposes a principled multi-task learning approach based on Discriminative Gaussian Process Latent Variable Model, named GaussianFace, to enrich the diversity of training data. In comparison to existing methods, our model exploits additional data from multiple source-domains to improve the generalization performance of face verification in an unknown target-domain. Importantly, our model can adapt automatically to complex data distributions, and therefore can well capture complex face variations inherent in multiple sources. Extensive experiments demonstrate the effectiveness of the proposed model in learning from diverse data sources and generalize to unseen domain. Specifically, the accuracy of our algorithm achieves an impressive accuracy rate of 98.52% on the well-known and challenging Labeled Faces in the Wild (LFW) benchmark [23]. For the first time, the human-level performance in face verification (97.53%) [28] on LFW is surpassed. 1
- 1、下载文档前请自行甄别文档内容的完整性,平台不提供额外的编辑、内容补充、找答案等附加服务。
- 2、"仅部分预览"的文档,不可在线预览部分如存在完整性等问题,可反馈申请退款(可完整预览的文档不适用该条件!)。
- 3、如文档侵犯您的权益,请联系客服反馈,我们会尽快为您处理(人工客服工作时间:9:00-18:30)。
82
WCSIT 2 (3), 82 -89, 2012 Contribution: In this paper, PCFTD model is proposed. The features of face images are generated using wavelet families and FFT. The features of test image are compared with database images using ED. Organization: The Introduction is given in section I, the existing research papers are discussed in section II, the proposed model is explained in section III, the algorithm is described in section IV, the performance analysis is discussed in section V and finally, conclusion is given in section VI. II. LITERATURE SURVEY other facial features like eyes, mouth etc., separately. Satiyan et. al., [14] investigated the performance of a Daubechies Wavelet family in recognizing facial expressions. A set of luminance stickers were fixed on subject’s face and the subject is instructed to perform required facial expressions. Also the subject’s expressions are recorded in video. A set of 2D coordinate values are obtained by tracking the movements of the stickers in video using tracking software. Standard deviation is derived from wavelet approximation coefficients for each daubechies wavelet orders. Hengliang Tang et al., [15] proposed a novel face representation approach known as Haar Local Binary Pattern histogram (HLBPH). The face image is decomposed into fourchannel sub images in frequency domain by Haar wavelet transform, and then the LBP operator is applied on each sub image to extract the face features. Hafiz Imtiaz and Shaikh Anowarul Fattah [16] proposed a multi-resolution feature extraction algorithm for face recognition based on 2D-DWT. For feature extraction, an entropy-based local band selection criterion is developed. A very high degree of recognition accuracy is achieved by the proposed method. Ramesh and Raja [17] proposed a performance evaluation of face recognition based on DWT and DT-CWT using Multimatching Classifiers. The face images are resized to required size for DT-CWT. The two level DWT is applied on face images to generate four sub bands. Euclidian Distance, Random Forest and Support Vector Machine matching algorithms are used for matching. III. MODEL
The reliable identification systems are required to verify and confirm the identity of an individual requesting their service. Secure access to the buildings, laptops, cellular phones, ATM etc., are an example of some of the applications. In the absence of robust verification systems, these systems are vulnerable to the wiles of an impostor. The traditional methods of authentications are passwords (knowledge – based security) and the ID Cards (token – based security). These methods can be easily breached due to the chance of stolen, lost or forget. The development and progress of biometrics technology, the fear of stolen, lost or forget can be eliminated. Biometrics refers to the automatic identification (or verification) of an individual (or a claimed identity) by using certain physiological or behavioral traits associated with the person [1]. The biometrics identifies the person based on features vector derived from physiological or behavioral characteristics such as uniqueness, permanence, accessibility, collectability and the minimum cost. The physiological biometrics are Fingerprint, Hand Scan, Iris Scan, Facial Scan and Retina Scan etc. and behavioral bio-metric are Voice, Keystroke, Gait, Signature etc. The physiological biometrics measures the specific part of the structure or shape of a portion of a subject’s body. But the behavioral biometric are more concerned with mood and environment.
Jossy P. George
Department of Computer Science Christ University Bangalore, dia
Saleem S Tevaramani and K B Raja
Electronics and Communication Engineering Department University Visvesvaraya College of Engineering Bangalore, India
Abstract— The biometrics is a powerful tool to authenticate a person for multiple applications. The face recognition is better biometrics compared to other biometric traits as the image can be captured without the knowledge and cooperation of a person. In this paper, we propose Performance Comparison of Face Recognition using Transform Domain Techniques (PCFTD). The face databases L – Spacek, JAFFE and NIR are considered. The features of face are generated using wavelet families such as Haar, Symelt and DB1 by considering approximation band only. The face features are also generated using magnitudes of FFTs. The test image features are compared with database features using Euclidian Distance (ED). The performance parameters such as FAR, FRR, TSR and EER computed using wavelet families and FFT. It is observed that the performance of FFT is better compared to wavelet families. The success rate of recognition is 100% for L – Spacek and JAFFE face databases as compared to 95% for NIR face databases Keywords- Face Recognition; DWT; FFT; ED; Biometrics. I. INTRODUCTION The face recognition is a challenging and fast growing area in real time applications of the several Physiological characteristics used in biometrics. Face recognition is one of the sought after areas in automatic face recognition, vision communication and human computer interaction. Every face recognition system generally consists of (i) Face image acquisition and storage: The face database consisting of face images of different persons with certain degree of orientation and large variations in the facial expressions. (ii) Preprocessing: The images of different size are computed in to uniform scale by resizing, color image is converted to gray scale, gray image is converted to binary image and filters may be used to remove unwanted noise. (iii) Features extractions: The image features are extracted in the spatial domain itself or transform domain of an image. The extracted features in spatial domain are counting the pixel density, distance between lips and nose, distance between lips and line joining two eyes, width of the lips, pixel mean, variance and standard deviation etc. The features in transformation domain are Fast Fourier Transformation (FFT) [2], Discrete Cosine Transform (DCT) [3], Short-Time Fourier Transform (STFT) [4], Discrete Wavelet Transform (DWT) [5] and Dual-Tree Complex Wavelet Transform (DT-CWT) [6] transformation domain coefficients. (iv) The matching features of a test image with the data base image: In this the features of the test image is compared with stored data base image features using Euclidean Distance (ED), Hamming Distance (HD) Chi-square, Support Vector Machine (SVM) [7] etc.