Four-legged walking gait control using a neuromorphic chip interfaced to a support vector l
高中英语冀教版选择性必修第四册Unit5Self-Control选择性必修第四册课后练习、课时练习

一、根据首字母填写单词(单词拼写)1. She is o________ and easy to get along with, so her classmates elected her monitor. (根据首字母单词拼写)2. It’s e________ that he managed to sleep through the party. (根据首字母单词拼写)3. This course is l________ to 12 people and costs only 50 yuan, which attracts more consumers. (根据首字母单词拼写)二、根据汉语意思填写单词(单词拼写)4. The grain was running out and we were getting ________ (绝望的). (根据汉语提示单词拼写)5. He knew I was ______(精力充沛的)and dynamic and would get things done. (根据汉语提示单词拼写)6. I wanted to tell her, but the words were _______(卡住) in my throat. (根据汉语提示单词拼写)三、根据中英文提示填写单词(单词拼写)7. As a child that has just arrived in such a new environment, he is c__________ (好奇的) about everything because they are different from what he used to see.(根据中英文提示单词拼写)8. When I was a________(意识到) of all the people staring at me, I felt nervous that I was sweating all over. (根据中英文提示单词拼写)四、完成句子9. 综上所述,我们无法否认的事实是:留学有其弊端。
本文介绍了一种应用两个电机驱动的六足式步行机器人-大学学士学位论文.doc

摘要本文介绍了一种应用两个电机驱动的六足式步行机器人,并对该机器人的运动机理与步态进行了分析,经样机实验,所设计的机器人可实现前进、后退、遇障转弯等功能,具有结构简单,控制便捷,行走稳定的特点。
基于仿生学原理,应用连杆机构学中的Robert原理,设计出一连杆轨迹能较好地近似于机器人理想足部轨迹的四杆机构,选择足部运动曲线并在图谱上找到该曲线,以确定四杆机构的各个参数。
由参数和电动机的输出转矩就能确定足部的线速度和加速度。
并通过PRO/E软件,对用这一连杆机构作为腿部机构的六足机器人进行了前进和转弯步态建模,并对它进行了稳定裕量分析,包括静力学分析和动力学分析。
针对这种腿部结构设计了六足的行走方式,通过对12个步进电机的控制,采用三角步态,实现了六足机器人的直行功能。
仿真及试验证明,这种结构能较好地维持六足机器人自身的平衡,并且对今后更深入地研究六足机器人抬腿行走姿态及可行性,具有较高的参考价值。
关键词:六足机器人;行走步态;运动原理;稳定性;四杆机构abstractThis paper introduced a six-legged walking robot propelled by two electromotors,and analyzed the robot's kinetic mechanism and walking style.Proved by the model test,the robot is capable of actions such as forwarding,backwarding and veering in the case of obstruction.The robot demonstrates such advantages as simple structure,comfortable control and stable performance of pacing.Based on the principle of bionics,this paper designs a four-linked mechanism using Robert principle,which can approximate the ideal trace of robot's leg ,choose the sport curve of the foot department, then check to find out that curve on the diagram, the old ability but locations can make sure four each parameters of the pole structure, can make sure the line speed and accelerations of the foot department from the exportation dint of the parameter and electric motor. Some simulations about the hexapod robot which uses the six-linked mechanism as its leg are made,and carried on the stability to it analysis, include the quiet mechanics analysis and dynamics analysis.A hexapod walking mode was designed according to this structure.By controlling 12 step motors straight walking function of the hexapod robot has been implemented with tripod gait movement.Simulation and experiment show that this structure can keep the hexapod robot body's balance better,providing high reference value to research the advantage and feasibility of leg-raising walking gesture.Keyword: six foot robot; Tread the appearance of walk; The sport principle; Stability; Four pole organizations目录摘要 (I)abstract .............................................................................................................. I I 1 绪论. (1)1.1国内外机器人的研究现状 (1)1.2机器人的主要研究问题 (3)1.3机器人的发展趋势 (5)1.4本课题所研究的主要内容 (6)2 机械机构设计 (6)2.1机构分析 (6)2.2 设计方法 (12)2.3四连杆机构的设计 (13)2.4四个钣金零件设计 (28)2.5 躯体部分机构设计 (33)2.6 机构设计总结 (34)参考文献 (35)致谢 (37)附录一 (50)附录二 (61)1 绪论1.1国内外机器人的研究现状1.1.1机器人的定义机器人是上个世纪人类最伟大的发明之一,而从机器人的角度来讲,21世纪将是一个自治机器人的世纪。
搜救犬犬英语作文
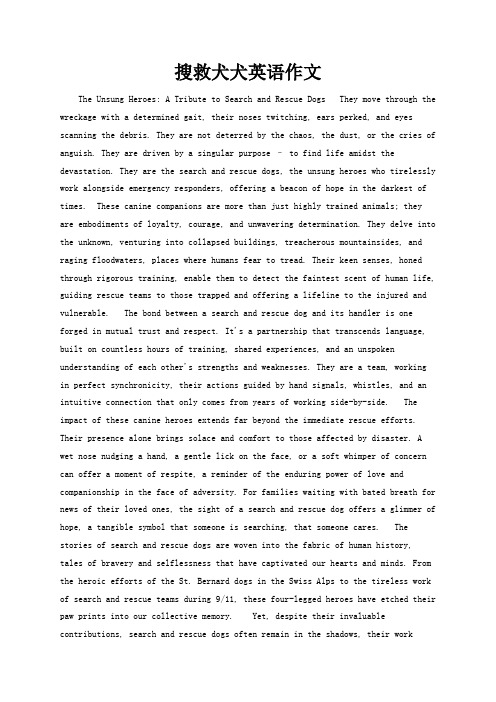
搜救犬犬英语作文The Unsung Heroes: A Tribute to Search and Rescue Dogs They move through the wreckage with a determined gait, their noses twitching, ears perked, and eyes scanning the debris. They are not deterred by the chaos, the dust, or the cries of anguish. They are driven by a singular purpose – to find life amidst the devastation. They are the search and rescue dogs, the unsung heroes who tirelessly work alongside emergency responders, offering a beacon of hope in the darkest of times. These canine companions are more than just highly trained animals; they are embodiments of loyalty, courage, and unwavering determination. They delve into the unknown, venturing into collapsed buildings, treacherous mountainsides, and raging floodwaters, places where humans fear to tread. Their keen senses, honed through rigorous training, enable them to detect the faintest scent of human life, guiding rescue teams to those trapped and offering a lifeline to the injured and vulnerable. The bond between a search and rescue dog and its handler is one forged in mutual trust and respect. It's a partnership that transcends language, built on countless hours of training, shared experiences, and an unspoken understanding of each other's strengths and weaknesses. They are a team, working in perfect synchronicity, their actions guided by hand signals, whistles, and an intuitive connection that only comes from years of working side-by-side. The impact of these canine heroes extends far beyond the immediate rescue efforts. Their presence alone brings solace and comfort to those affected by disaster. A wet nose nudging a hand, a gentle lick on the face, or a soft whimper of concern can offer a moment of respite, a reminder of the enduring power of love and companionship in the face of adversity. For families waiting with bated breath for news of their loved ones, the sight of a search and rescue dog offers a glimmer of hope, a tangible symbol that someone is searching, that someone cares. The stories of search and rescue dogs are woven into the fabric of human history,tales of bravery and selflessness that have captivated our hearts and minds. From the heroic efforts of the St. Bernard dogs in the Swiss Alps to the tireless work of search and rescue teams during 9/11, these four-legged heroes have etched their paw prints into our collective memory. Yet, despite their invaluable contributions, search and rescue dogs often remain in the shadows, their workunrecognized and their sacrifices unacknowledged. They are the silent partners, the unsung heroes who ask for nothing in return but the opportunity to serve. Today, let us take a moment to appreciate these remarkable creatures, to celebrate their unwavering dedication, and to recognize their invaluable role in saving countless lives. Let us honor their courage and their spirit, a testament to the enduring bond between humans and animals and the extraordinary things we can achieve together.。
关于学骑马克服困难的英语作文八百字

关于学骑马克服困难的英语作文八百字全文共3篇示例,供读者参考篇1Learning to Ride Horses: A Journey of Perseverance and GrowthAs a student with a deep fascination for horses, I embarked on a journey to learn horseback riding, little did I know the challenges that lay ahead. The path was filled with obstacles, but each stumbling block served as a stepping stone towards growth and self-discovery.When I first stepped into the stables, the aroma of hay and the gentle whinnies of the horses filled me with excitement and trepidation. I was eager to forge a connection with these magnificent creatures, but the reality of their size and strength was daunting. My first few lessons were a whirlwind of unfamiliar sensations – the rhythmic sway of the horse's gait, the strain of maintaining balance, and the overwhelming fear of falling.Despite the initial setbacks, I remained undeterred. With each session, I learned to trust the guidance of my instructor and the gentle demeanor of the horses. Slowly but surely, I began tounderstand the nuances of communication between rider and steed, a language spoken through subtle shifts in weight and gentle tugs of the reins.Yet, the road was not without its challenges. There were days when frustration threatened to overwhelm me, when my muscles ached from the strain, and when the fear of failure loomed like a dark cloud. But it was in those moments that I discovered the true depths of my resilience.I vividly recall one particular lesson when my mount, a spirited chestnut mare, decided to test my mettle. As we trotted around the arena, she suddenly veered off course, her powerful strides carrying us towards the fence. In that split second, I had a choice – to surrender to panic or to trust my training. With a deep breath and a firm hand, I guided her back onto the path, my heart pounding with a newfound sense of accomplishment.Each triumph, no matter how small, fueled my determination to push forward. I learned to embrace the falls, both literal and figurative, as opportunities to dust myself off and try again. The bruises and scrapes became badges of honor, reminders of the battles fought and won against self-doubt and fear.As the weeks turned into months, I noticed a profound shift within myself. The timid, uncertain rider I had been at the starthad given way to a more confident and capable individual. The lessons I learned on the back of a horse transcended the boundaries of the stable, seeping into every aspect of my life.I learned the value of patience, of taking each challenge one step at a time, and of celebrating the small victories along the way. I discovered the power of perseverance, of refusing to let setbacks define my journey, and of pushing through even when the path seemed insurmountable.Most importantly, I gained a deeper appreciation for the beauty of vulnerability. To truly connect with a horse, one must shed the armor of pride and embrace a state of openness and trust. It was in those moments of vulnerability that I found the courage to face my fears head-on and to trust in my own abilities.As I reflect on my journey, I am filled with a sense of gratitude for the lessons horseback riding has taught me. The stables have become more than just a place to ride; they are a sanctuary where篇2Learning to Ride Horses: A Journey of Perseverance and GrowthAs a student, one of the most rewarding experiences I've had is learning to ride horses. It's a challenging yet exhilarating journey that has taught me invaluable lessons about perseverance, resilience, and personal growth.When I first decided to take up horseback riding, I was equal parts excited and apprehensive. The idea of climbing atop a powerful animal that weighed several times my own weight was both thrilling and daunting. However, I was determined to conquer my fears and embrace this new adventure.My first few lessons were a humbling experience. The horses seemed to sense my inexperience and trepidation, and they responded accordingly. I found myself struggling to maintain control and balance, often feeling like a mere passenger along for the ride. Frustration and self-doubt crept in, and I began to question whether I had what it took to become a proficient rider.But then, something shifted. With the patient guidance of my instructors and the unwavering support of my fellow students, I began to understand the nuances of horseback riding.I learned the importance of body language, subtle cues, and the art of communicating with these majestic creatures through my movements and demeanor.Gradually, I started to build confidence in the saddle. Each lesson brought new challenges, but I approached them with a renewed sense of determination. I embraced the setbacks as opportunities for growth, recognizing that every misstep or stumble was a chance to learn and refine my skills.One of the most profound lessons I learned was the value of trust and partnership. Riding a horse is not just about asserting dominance or exerting control; it's about fostering a mutual understanding and respect between rider and animal. As I developed a deeper connection with the horses, they seemed to respond in kind, becoming more responsive to my commands and more attuned to my emotional state.Through this journey, I also discovered the importance of patience and perseverance. Mastering horseback riding is a lifelong pursuit, and there were moments when progress felt agonizingly slow. But I learned to celebrate the small victories, to revel in the incremental improvements, and to find joy in the process itself.As I advanced in my riding skills, I found myself tackling increasingly complex challenges. From navigating intricate obstacle courses to attempting daring jumps, each new featpushed me beyond my perceived limits and forced me to confront fears and doubts head-on.But with each obstacle overcome, I felt a profound sense of accomplishment and personal growth. I realized that the lessons I was learning extended far beyond the confines of the riding arena. They were lessons about resilience, adaptability, and the power of commitment – lessons that would serve me well in all aspects of my life.Today, as I look back on my journey as a rider, I am filled with a deep sense of gratitude. Horseback riding has not only introduced me to a thrilling and rewarding pastime but has also shaped me into a more confident, disciplined, and resilient individual.The challenges I've faced and the triumphs I've experienced have taught me that growth often comes through adversity, and that the most rewarding experiences in life are those that push us beyond our comfort zones and challenge us to confront our fears head-on.As I continue to hone my skills and explore new horizons in the world of horseback riding, I carry with me the invaluable lessons I've learned along the way. And I know that no matter what obstacles lie ahead, I have within me the strength, resilience,and determination to overcome them – one step, one stride, at a time.篇3Learning to Ride Horses: A Journey of PerseveranceAs a city kid, I never imagined that one day I would find myself in the saddle, learning the art of horseback riding. It all began when my family decided to spend a summer in the countryside, and I stumbled upon a local riding school. Intrigued by the majestic creatures, I signed up for lessons, not knowing the challenges that awaited me.On my first day, I met my instructor, a seasoned equestrian named Emily. She introduced me to a gentle mare named Bella, and I was instantly captivated by her gentle demeanor. However, as soon as I mounted the saddle, I realized that this was no easy feat. My legs ached from the unfamiliar position, and my hands struggled to maintain control of the reins.Despite the initial discomfort, I was determined to master this skill. Emily guided me through the basic techniques, teaching me how to communicate with Bella through subtle leg and rein cues. It was like learning a new language, and I foundmyself fumbling with the vocabulary, often eliciting unintended responses from my four-legged companion.Frustration crept in as I watched more experienced riders gracefully maneuver their horses around the ring. I felt clumsy and out of place, wondering if I had bitten off more than I could chew. Emily sensed my discouragement and offered words of wisdom that would forever shape my perspective."Riding is not just about controlling the horse," she said. "It's about forming a partnership, a bond built on trust and understanding. The more you connect with Bella, the smoother your journey will become."Those words resonated deeply within me, and I realized that my struggles were not unique. Every rider, no matter their level of expertise, had faced similar challenges. It was a humbling realization that reignited my determination.With renewed vigor, I approached each lesson as an opportunity to strengthen my bond with Bella. I learned to read her body language, anticipate her reactions, and adjust my own movements accordingly. Slowly but surely, we developed a rhythm, a synchronicity that made our rides smoother and more enjoyable.Of course, there were still setbacks along the way. I experienced my fair share of stumbles, near-falls, and even a few unintentional dismounts. But each time, I dusted myself off, remounted, and tried again. The bruises and scrapes were badges of honor, reminders of the perseverance required to conquer this challenging pursuit.As the weeks passed, I noticed a remarkable transformation within myself. Not only had my riding skills improved, but I had also gained a newfound confidence and resilience. The lessons I learned in the saddle transcended the arena, shaping my approach to other aspects of life.I realized that overcoming obstacles is not about brute force or blind determination. It's about patience, adaptability, and a willingness to learn from setbacks. Just as I had to listen to Bella's cues and adjust my actions accordingly, I learned to approach challenges with an open mind, ready to pivot and find alternative solutions.By the end of the summer, I had developed a profound respect for the art of horseback riding and the magnificent creatures that made it possible. Bella had become more than just a riding companion; she was a teacher, a friend, and a constantreminder of the rewards that come from perseverance and resilience.As I bid farewell to the countryside and returned to the city, I carried with me a newfound sense of accomplishment and a deep appreciation for the lessons I had learned. Riding may have started as a mere curiosity, but it had transformed into a lifelong passion, one that had taught me the invaluable art of overcoming obstacles and embracing the journey, no matter how challenging it may seem.。
连续电驱动四足机器人设计与分析
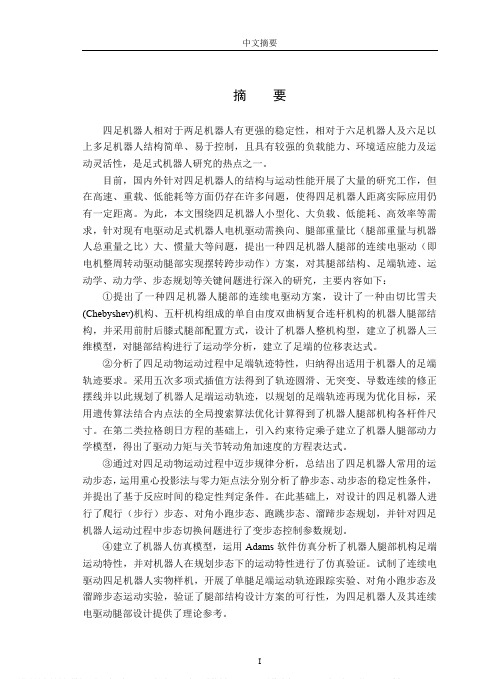
中文摘要摘要四足机器人相对于两足机器人有更强的稳定性,相对于六足机器人及六足以上多足机器人结构简单、易于控制,且具有较强的负载能力、环境适应能力及运动灵活性,是足式机器人研究的热点之一。
目前,国内外针对四足机器人的结构与运动性能开展了大量的研究工作,但在高速、重载、低能耗等方面仍存在许多问题,使得四足机器人距离实际应用仍有一定距离。
为此,本文围绕四足机器人小型化、大负载、低能耗、高效率等需求,针对现有电驱动足式机器人电机驱动需换向、腿部重量比(腿部重量与机器人总重量之比)大、惯量大等问题,提出一种四足机器人腿部的连续电驱动(即电机整周转动驱动腿部实现摆转跨步动作)方案,对其腿部结构、足端轨迹、运动学、动力学、步态规划等关键问题进行深入的研究,主要内容如下:①提出了一种四足机器人腿部的连续电驱动方案,设计了一种由切比雪夫(Chebyshev)机构、五杆机构组成的单自由度双曲柄复合连杆机构的机器人腿部结构,并采用前肘后膝式腿部配置方式,设计了机器人整机构型,建立了机器人三维模型,对腿部结构进行了运动学分析,建立了足端的位移表达式。
②分析了四足动物运动过程中足端轨迹特性,归纳得出适用于机器人的足端轨迹要求。
采用五次多项式插值方法得到了轨迹圆滑、无突变、导数连续的修正摆线并以此规划了机器人足端运动轨迹,以规划的足端轨迹再现为优化目标,采用遗传算法结合内点法的全局搜索算法优化计算得到了机器人腿部机构各杆件尺寸。
在第二类拉格朗日方程的基础上,引入约束待定乘子建立了机器人腿部动力学模型,得出了驱动力矩与关节转动角加速度的方程表达式。
③通过对四足动物运动过程中迈步规律分析,总结出了四足机器人常用的运动步态,运用重心投影法与零力矩点法分别分析了静步态、动步态的稳定性条件,并提出了基于反应时间的稳定性判定条件。
在此基础上,对设计的四足机器人进行了爬行(步行)步态、对角小跑步态、跑跳步态、溜蹄步态规划,并针对四足机器人运动过程中步态切换问题进行了变步态控制参数规划。
Bipedal Walking on Rough Terrain Using Manifold Control
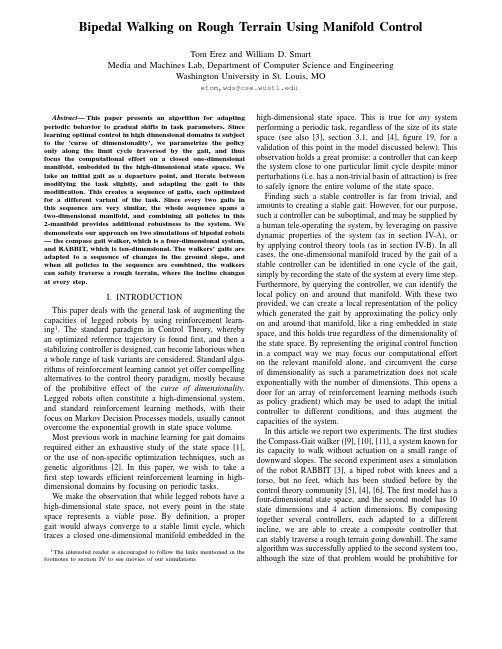
Bipedal Walking on Rough Terrain Using Manifold ControlTom Erez and William D.SmartMedia and Machines Lab,Department of Computer Science and EngineeringWashington University in St.Louis,MOetom,wds@Abstract—This paper presents an algorithm for adapting periodic behavior to gradual shifts in task parameters.Since learning optimal control in high dimensional domains is subject to the’curse of dimensionality’,we parametrize the policy only along the limit cycle traversed by the gait,and thus focus the computational effort on a closed one-dimensional manifold,embedded in the high-dimensional state space.We take an initial gait as a departure point,and iterate between modifying the task slightly,and adapting the gait to this modification.This creates a sequence of gaits,each optimized for a different variant of the task.Since every two gaits in this sequence are very similar,the whole sequence spans a two-dimensional manifold,and combining all policies in this 2-manifold provides additional robustness to the system.We demonstrate our approach on two simulations of bipedal robots —the compass gait walker,which is a four-dimensional system, and RABBIT,which is ten-dimensional.The walkers’gaits are adapted to a sequence of changes in the ground slope,and when all policies in the sequence are combined,the walkers can safely traverse a rough terrain,where the incline changes at every step.I.INTRODUCTIONThis paper deals with the general task of augmenting the capacities of legged robots by using reinforcement learn-ing1.The standard paradigm in Control Theory,whereby an optimized reference trajectory is foundfirst,and then a stabilizing controller is designed,can become laborious when a whole range of task variants are considered.Standard algo-rithms of reinforcement learning cannot yet offer compelling alternatives to the control theory paradigm,mostly because of the prohibitive effect of the curse of dimensionality. Legged robots often constitute a high-dimensional system, and standard reinforcement learning methods,with their focus on Markov Decision Processes models,usually cannot overcome the exponential growth in state space volume. Most previous work in machine learning for gait domains required either an exhaustive study of the state space[1], or the use of non-specific optimization techniques,such as genetic algorithms[2].In this paper,we wish to take a first step towards efficient reinforcement learning in high-dimensional domains by focusing on periodic tasks.We make the observation that while legged robots have a high-dimensional state space,not every point in the state space represents a viable pose.By definition,a proper gait would always converge to a stable limit cycle,which traces a closed one-dimensional manifold embedded in the 1The interested reader is encouraged to follow the links mentioned in the footnotes to section IV to see movies of our simulations high-dimensional state space.This is true for any system performing a periodic task,regardless of the size of its state space(see also[3],section3.1,and[4],figure19,for a validation of this point in the model discussed below).This observation holds a great promise:a controller that can keep the system close to one particular limit cycle despite minor perturbations(i.e.has a non-trivial basin of attraction)is free to safely ignore the entire volume of the state space. Finding such a stable controller is far from trivial,and amounts to creating a stable gait.However,for our purpose, such a controller can be suboptimal,and may be supplied by a human tele-operating the system,by leveraging on passive dynamic properties of the system(as in section IV-A),or by applying control theory tools(as in section IV-B).In all cases,the one-dimensional manifold traced by the gait of a stable controller can be identified in one cycle of the gait, simply by recording the state of the system at every time step. Furthermore,by querying the controller,we can identify the local policy on and around that manifold.With these two provided,we can create a local representation of the policy which generated the gait by approximating the policy only on and around that manifold,like a ring embedded in state space,and this holds true regardless of the dimensionality of the state space.By representing the original control function in a compact way we may focus our computational effort on the relevant manifold alone,and circumvent the curse of dimensionality as such a parametrization does not scale exponentially with the number of dimensions.This opens a door for an array of reinforcement learning methods(such as policy gradient)which may be used to adapt the initial controller to different conditions,and thus augment the capacities of the system.In this article we report two experiments.Thefirst studies the Compass-Gait walker([9],[10],[11],a system known for its capacity to walk without actuation on a small range of downward slopes.The second experiment uses a simulation of the robot RABBIT[3],a biped robot with knees and a torso,but no feet,which has been studied before by the control theory community[5],[4],[6].Thefirst model has a four-dimensional state space,and the second model has10 state dimensions and4action dimensions.By composing together several controllers,each adapted to a different incline,we are able to create a composite controller that can stably traverse a rough terrain going downhill.The same algorithm was successfully applied to the second system too, although the size of that problem would be prohibitive formost reinforcement learning algorithms.In the following wefirst give a short review of previous work in machine learning,and then explain the technical aspects of constructing a manifold controller,as well as the learning algorithms used.We then demonstrate the effec-tiveness of Manifold Control by showing how it is used to augment the capacities of existing controllers in two different models of bipedal walk.We conclude by discussing the potential of our approach,and offer directions for future work.II.P REVIOUS W ORKThe generalfield of gait design has been at the focus of mechanical engineering for many years,and recent years saw an increase in the contribution from the domain of machine learning.For example,Stilman et al.[7]studied an eight-dimensional system of a biped robot with knees, similar to the one studied below.They showed that in their particular case the dimensionality can be reduced through some specific approximations during different phases.Then, they partitioned the entire volume of the reduced state space into a grid,and performed Q-learning using a simulation model of the system’s dynamics.The result was a robot walker that can handle a range of slopes around the level horizontal plane.In addition,there is a growing interest in recent years in gaits that can effectively take advantage of the passive dynamics(see the review by Collins et al.[8]for a thorough coverage).Tedrake[9]discusses several versions the com-pass gait walker which were built and analyzed.Controllers for the compass gait based on an analytical treatment of the system equations wasfirst suggested by Goswami et al.[10],who used both hip and ankle actuation.Further results were described by Spong and Bhatia[11],where the case of uneven terrain was also discussed.Ramamoorthy and Kuipers[12]suggested hybrid control of walking over irregular terrain by seeking inspiration from human walking.III.M ANIFOLD C ONTROLA.The Basic Control SchemeThe basic idea in manifold control is to focus the com-putational effort on the limit cycle.Therefore,the policy is approximated using locally activated processing elements (PEs),positioned along the manifold spanned by the limit cycle.Each PE defines a local policy,linear in the position of the state relative to that PE.When the policy is queried with a given state x,the local policy of each PE is calculated as:µi(x)=[1(x−c i)T M T]G i(1) where c i is the location of element i,M is a diagonal matrix which determines the scale of each dimension,and G i is an (n+1)-by-m matrix,where m is the action dimension and n is the number of state space dimensions.G i is made of m columns,one for each action dimension,and each column is an(n+1)-sized gain vector.Thefinal policy u(x)is calculated by mixing the local policies of each PEaccordingFig.1.Illustrations of the models used.On the left,the compass-gait walker:the system’s state is defined by the two legs’angles from the vertical direction and the associated angular velocities,for a total of four dimensions. Thisfigure also depicts the incline of the sloped ground.On the right, RABBIT:the system’s state is defined bt the angle of the torso form the vertical direction,the angles between the thighs and the torso,and the knee angles between the shank and the thigh.This model of RABBIT has ten state dimensions,where at every moment one leg isfixed to the ground, and the other leg is free to swing.to a normalized Gaussian activation function,usingσas a scalar bandwidth term:w i=exp(−(x−c i)T M TσM(x−c i)),(2)u(x)= n i=1w iµitraverse a path of higher value(i.e.collect more rewards,or less cost)along its modified limit cycle.1)Defining the Value Function:In the present work we consider a standard nondiscounted reinforcement learning formulation with afinite time horizon and no terminal costs. More accurately,we define the Value Vπ(x0)of a given state x0under afixed policyπ(x)as:Vπ(x0)= T0r(x t,π(x t))dt(4) where r(x,u)is the reward determined by the current state and the selected action,T is the time horizon,and x t is the solution of the time invariant ordinary differential equation ˙x=f(x,π(x))with the initial condition x=x0,so thatx t= t0f(xτ,π(xτ))dτ.(5) 2)Approximating the Policy Gradient:With C being the locations of the processing elements,and G being the set of their local gains,we make use of a method,due to[14],of piecewise estimation of the gradient of the value function at a given initial state x0with respect to the parameter set G. As Munos showed in[14],from(4)we can write∂V∂G,(6) and for the general form r=r(x,u)we can decompose∂r/∂G as∂r∂u ∂u∂x∂xFig.2.The path used to test the compass gait walker,and an overlay of the walker traversing this path.Note how the step length adapts to the changing incline.the forward swing,but will undergo an inelastic collision with thefloor during the backward swing.At this point it will become the stance leg,and the other leg will be set free to swing.The entire system is placed on a plane that is inclined at a variable angleφfrom the horizon.In the interests of brevity,we omit a complete description of the system dynamics here,referring the interested reader to the relevant literature[15],[10],[11].Although previous work considered actuation both at the hip and the ankle,we chose to study the case of actuation at the hip only.The learning phase in this system was done using simple stochastic gradient ascent,rather than the elaborate policy gradient estimation described in section III-B.2.The initial manifold was sampled at an incline ofφ=−0.05(the initial policy is the zero policy,so there were no approximation errors involved).One shaping iteration consisted of the following:first,G was modified to G tent=G+ηδG, withη=0.1andδG drawn at random from a multinormal distribution with unit covariance.The new policy’s perfor-mance was measured as a sum of all the rewards along20 steps.If the value of this new policy was bigger than the present one,it was adopted,otherwise it was rejected.Then, a newδG was drawn,and the process repeated itself.After 3successful adoptions,the shaping iterations step concluded with a resampling of the new controller,and the incline was decreased by0.01.After10shaping iteration steps,we had controllers that could handle inclines up toφ=−0.14.After another10 iteration steps with the incline increasing by0.005,we had controllers that could handle inclines up toφ=0.0025(a slight uphill).This range is approxmately double the limit of the passive walker[15].Finally,we combined the various controllers into one composite controller.This new controller used1500charts to span a two-dimensional manifold embedded in the four-dimensional state space.The performance of the composite controller was tested on an uneven terrain where the incline was gradually changed fromφ=0toφ=0.15radians, made of“tiles”of variable length whose inclines were0.01 radians apart.figure III-B.2shows an overlay of the walker’s downhill path.A movie of this march is available online.2B.The Uphill-Walking RABBIT RobotWe applied manifold control also to simulations of the legged robot RABBIT,using code from Prof.Jessy Grizzle that is freely available online[16].RABBIT is a biped robot with a torso,two knees and no feet(seefigure1b.),and is actuated at four places:both hip joins(where thighs are actuated against the torso),and both knees(where shanks are actuated against the thighs).The simulation assumes a stance leg with no slippage,and a swing leg that is free to move at all directions until it collides inelastically with the floor,and becomes the stance leg,freeing the other leg to swing.This robot too is modeled as a nonlinear system with impulse effects.Again,we are forced to omit a complete reconstruction of the model’s details,and refer the interested reader to[4],equation8.This model was studied extensively by the control theory community.In particular,an optimal desired signal was derived in[6],and a controller that successfully realizes this signal was presented in[4].However,all the efforts were focused on RABBIT walking on even terrain.We sought a way to augment the capacities of the RABBIT model,and allow it to traverse a rough,uneven terrain.We found that the controller suggested by[4]can easily handle negative (downhill)incline of0.2radiand and more,but cannot handle positive(uphill)inclines.3.Learning started by approximating the policy from[4]as a manifold controller,using400processing elements with a mean distance of about0.03state space length units.The performance of the manifold controller was indistinguishable to the naked eye from the original controller,and perfor-mance,as measured by the performance criterion C3in[6] (the same used by[4]),was only1%worse,probably due to minor approximation errors.The policy gradient was estimated using(6),according to a simple reward model:r(x,u)=10v x hip−1Fig.3.The rough terrain traversed by RABBIT.Since this model has knees,it can walk both uphill and downhill.Note how the step length adapts to the changing terrain.The movie of this parade can be seen at tt http:/2b8sdm,which is a shortcut to the YouTube website.where v xhip is the velocity of the hip joint(where the thighand the torso meet)in the positive direction of the X-axis, and u max is a scalar parameter(in our case,chosen to be 120)that tunes the nonlinear action penalty and promotes energetic efficiency.After the initial manifold controller was created,the system followed a fully automated shaping protocol for 20iterations:at every iteration,∂V/∂G was estimated, andηwasfixed to0.1%of|G|.This small learning rate ensured that we don’t modify the policy too much and lose stability.The modified policy,assumed to be slightly better, was then tested on a slightly bigger incline(the veryfirst manifold controller was tried on an incline of0rad.,and in every iteration we increased the incline in0.003rad.).This small modification to the model parameters ensured that the controller can still walk stably on the incline.If stability was not lost(as was the case in all our iterations),we resampled u(·;C,G new)so that C adj overlapped the limit cycle of the modified system(with the new policy and new incline),and the whole process repeated.This procedure allowed a gradual increase in the system’s maximal stable incline.Figure4depicts the evolution of the stability margins of every ring along the shaping iteration:for every iteration we present an upper(and lower)bound on the incline for which the controller can maintain stability.Thiswas tested by setting a test incline,and allowing the system to run for10 seconds.If no collapse happened by this time,the test incline was raised(lowered),until an incline was found for which the system can no longer maintain stability.As this picture shows,our automated shaping protocol does not maintain a tight control on the stability margins-for most iterations,a modest improvement is recorded.The system’s nonlinearity is well illustrated by the curious case of iteration9,where the same magnitude ofδG causes a massive improvement, despite the fact that the control manifold itself didn’t change dramatically(seefigure5).The converse is also true for some iterations(such as17and18)there is a decrease in the stability margins,but this is not harming the overall effectiveness,since these iterations are using training data obtained at an incline that is very far from the stability Fig.4.Thisfigure shows the inclines for which each iteration could maintain a stable gait on the RABBIT model.The diagonal line shows the incline for which each iteration was trained.Iteration0is the original controller.The initial manifold control approximation degrades most of the stability margin of the original control,but this is quickly regained through adaptation.Note that both the learning rate and the incline change rate were held constant through the entire process.The big jump in iteration 9exemplifies the nonlinearity of the system,as small changes may have unpredictable results,in this case,for the best.margin.Finally,three iterations were composed together,and the resulting controller successfully traversed a rough terrain that included inclines from−0.05to0.15radians.Figure3 shows an overlay image of the rough path.V.C ONCLUSION AND F UTURE W ORKIn this paper we present a compact representation of the policy for periodic tasks,and apply a trajectory-based policy gradient algorithm to it.Most importantly,the methods we present do not scale exponentially with the number of dimensions,and hence allow us to circumvent the curse of dimensionality in the particular case of periodic tasks.By following a gradual shaping process,we are able to create robust controllers that augment the capacities of existing systems in a consistent way.33.54−1.5−0.2anglea n g . v e l.anglea n g . v el.anglea n g . v e l.anglea n g . v el.anglea n g . v e l.anglea n g . v el.anglea n g . v e l.anglea n g . v el.anglea n g . v e l.anglea n g . v el.anglea n g . v e l.anglea n g . v el.anglea n g . v e l.anglea n g . v e l.Fig.5.A projection of the manifold of several stages of the shaping process for the RABBIT model.The top row shows the angle and angular velocity between the torso and the stance thigh,and the bottom row shows the angle and angular velocity of the knee of the swing leg.Every two consecutive iterations are only slightly different from each other.Throughout the entire shaping process,changes accumulate,and new solutions emerge.Manifold control may also be used when the initial con-troller is profoundly suboptimal 4.It is also important to note that the rough terrain was traversed without informing the walker of the current terrain.We may say that the walkers walked blindly on their rough path.This demonstrates how stable a composite manifold controller can be.However,in some practical applications it could be beneficial to represent this important piece of information explicitly,and select the most appropriate ring at every step.We believe that the combination of local learning and careful shaping holds a great promise to many applications of periodic tasks,and hope to demonstrate it through future work on even higher-dimensional systems.Future research directions could include methods that allow second-order convergence,and learning a model of the plant.R EFERENCES[1]M.Stilman,C.G.Atkeson,J.J.Kuffner,and G.Zeglin,“Dynamicprogramming in reduced dimensional spaces:Dynamic planning for robust biped locomotion,”in Proceedings of the 2005IEEE Interna-tional Conference on Robotics and Automation (ICRA 2005),2005,pp.2399–2404.[2]J.Buchli, F.Iida,and A.Ijspeert,“Finding resonance:Adaptivefrequency oscillators for dynamic legged locomotion,”in Proceedings of the IEEE/RSJ International Conference on Intelligent Robots and Systems (IROS).IEEE,2006,pp.3903–3909.[3] C.Chevallereau and P.Sardain,“Design and actuation optimization ofa 4-axes biped robot for walking and running,”in Proceedings of the IEEE International Conference on Robotics and Automation (ICRA),2000.[4] F.Plestan,J.W.Grizzle,E.Westervelt,and G.Abba,“Stable walkingof a 7-dof biped robot,”IEEE Trans.Robot.Automat.,vol.19,no.4,pp.653–668,Aug.2003.4theinterested reader is welcome to see other results of manifold learning on a 14-dimensional system at /2h3qny and /2462j7.[5] C.Sabourin,O.Bruneau,and G.Buche,“Experimental validation ofa robust control strategy for the robot rabbit,”in Proceedings of the IEEE International Conference on Robotics and Automation (ICRA),2005.[6] C.Chevallereau and Y .Aoustin,“Optimal reference trajectories forwalking and running of a biped robot,”Robotica ,vol.19,no.5,pp.557–569,2001.[7]M.Stilman,C.G.Atkeson,J.J.Kuffner,and G.Zeglin,“Dynamicprogramming in reduced dimensional spaces:Dynamic planning for robust biped locomotion,”in Proceedings of the 2005IEEE Interna-tional Conference on Robotics and Automation (ICRA 2005),2005,pp.2399–2404.[8]S.H.Collins,A.Ruina,R.Tedrake,,and M.Wisse,“Efficient bipedalrobots based on passive-dynamic walkers,”Science ,pp.307:1082–1085,February 2005.[9]R.L.Tedrake,“Applied optimal control for dynamically stable leggedlocomotion,”Ph.D.dissertation,Massachusetts Institute of Technol-ogy,August 2004.[10] A.Goswami, B.Espiau,and A.Keramane,“Limit cycles in apassive compass gait biped and passivity-mimicking control laws,”Autonomous Robots ,vol.4,no.3,pp.273–286,1997.[11]M.W.Spong and G.Bhatia,“Further results on the control of the com-pass gait biped,”in Proceedings of the 2003IEEE/RSJ International Conference on Intelligent Robots and Systems (IROS 2003),vol.2,2003,pp.1933–1938.[12]S.Ramamoorthy and B.Kuipers,“Qualitative hybrid control ofdynamic bipedal walking,”in Robotics :Science and Systems II ,G.S.Sukhatme,S.Schaal,W.Burgard,and D.Fox,Eds.MIT Press,2007.[13]S.Schaal and C.Atkeson,“constructive incremental learning fromonly local information,”neural computation ,no.8,pp.2047–2084,1998.[14]R.Munos,“Policy gradient in continuous time,”Journal of MachineLearning Research ,vol.7,pp.771–791,2006.[15] A.Goswami,B.Thuilot,and B.Espiau,“Compass-like biped robotpart i:Stability and bifurcation of passive gaits,”INRIA,Tech.Rep.2996,October 1996.[16] E.Westervelt, B.Morris,and J.Grizzle.(2003)Five link walker.IEEE-CDC Workshop:Feedback Control of Biped Walking Robots.[Online].Available:/2znlz2。
2024年中考英语(江苏省盐城地区)教材考点梳理专练课件九年级下册Unit3
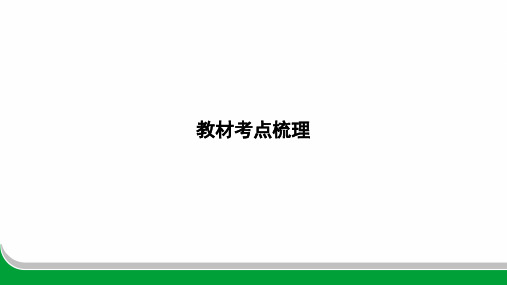
一
二
三
四
五
A powerful robot named ChatGPT has been made by an American company OpenAI. It is developed to make up human-like texts. It can translate languages,talk with people and write songs,poems and even jokes.
一
二
三
四
五
ChatGPT appears at a time when AI is becoming better at doing creative tasks. The system can produce something similar to human’s own works. 4. D But the creation of art should be a lot more personal. Sopeople have many worries about that. However,ChatGPT is a completely new thing. It is only natural for it to cause some problems. 5. C
五
三、 (2023·凉山)阅读下面短文,从所给选项中选出能填入空白处的 最佳选项,使短文通顺、意思完整,其中有一项为多余选项。(词数: 238 建议用时:5分钟) A. People around the world tried ChatGPT. B. It has the writing skills of a real person. C. We expect the problems to be solved over time. D. People start depending on ChatGPT for artwork. E. Nowadays few people use ChatGPT to write or draw. F. But ChatGPT can produce a well-written article at once.
四足仿生机器人论文关节运动控制器论文
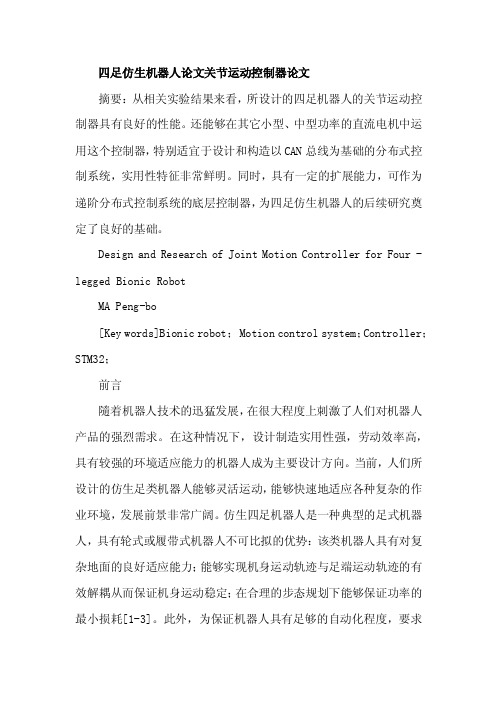
四足仿生机器人论文关节运动控制器论文摘要:从相关实验结果来看,所设计的四足机器人的关节运动控制器具有良好的性能。
还能够在其它小型、中型功率的直流电机中运用这个控制器,特别适宜于设计和构造以CAN总线为基础的分布式控制系统,实用性特征非常鲜明。
同时,具有一定的扩展能力,可作为递阶分布式控制系统的底层控制器,为四足仿生机器人的后续研究奠定了良好的基础。
Design and Research of Joint Motion Controller for Four - legged Bionic RobotMA Peng-bo[Key words]Bionic robot; Motion control system;Controller;STM32;前言隨着机器人技术的迅猛发展,在很大程度上刺激了人们对机器人产品的强烈需求。
在这种情况下,设计制造实用性强,劳动效率高,具有较强的环境适应能力的机器人成为主要设计方向。
当前,人们所设计的仿生足类机器人能够灵活运动,能够快速地适应各种复杂的作业环境,发展前景非常广阔。
仿生四足机器人是一种典型的足式机器人,具有轮式或履带式机器人不可比拟的优势:该类机器人具有对复杂地面的良好适应能力;能够实现机身运动轨迹与足端运动轨迹的有效解耦从而保证机身运动稳定;在合理的步态规划下能够保证功率的最小损耗[1-3]。
此外,为保证机器人具有足够的自动化程度,要求机载控制系统能够实时地处理各种复杂环境反馈信息,并能准确地发出控制指令,为了保证机器人达到良好的运动特性,本文分别提出结构类似的分层式的控制体系结构,对控制任务进行分担,提高系统实时性。
此外,双足机器人步入四足机器人的承载能力强,后者的稳定性更好。
而且四足机器人比六足机器人相比,前者的机构更加简单、能够有效地适应作业环境,具有良好的灵活性[4]。
所以,本文以四足类机器人作为自己的研究对象,设计和规划四足机器人的运动控制器。
- 1、下载文档前请自行甄别文档内容的完整性,平台不提供额外的编辑、内容补充、找答案等附加服务。
- 2、"仅部分预览"的文档,不可在线预览部分如存在完整性等问题,可反馈申请退款(可完整预览的文档不适用该条件!)。
- 3、如文档侵犯您的权益,请联系客服反馈,我们会尽快为您处理(人工客服工作时间:9:00-18:30)。
Susanne StillNEC Research Institute4Independence Way,Princeton NJ08540,USA sasa@Bernhard Sch¨o lkopfMicrosoft Research Institute1Guildhall Street,Cambridge,UK bsc@Klaus Hepp Institute of Theoretical Physics ETH Z¨u rich,SwitzerlandRodney J.Douglas Institute of Neuroinformatics ETH/UNI Z¨u rich,SwitzerlandAbstractTo control the walking gaits of a four-legged robot we present a novelneuromorphic VLSI chip that coordinates the relative phasing of therobot’s legs similar to how spinal Central Pattern Generators are believedto control vertebrate locomotion[3].The chip controls the leg move-ments by driving motors with time varying voltages which are the out-puts of a small network of coupled oscillators.The characteristics of thechip’s output voltages depend on a set of input parameters.The rela-tionship between input parameters and output voltages can be computedanalytically for an idealized system.In practice,however,this ideal re-lationship is only approximately true due to transistor mismatch and off-sets.Fine tuning of the chip’s input parameters is done automatically bythe robotic system,using an unsupervised Support Vector(SV)learningalgorithm introduced recently[7].The learning requires only that thedescription of the desired output is given.The machine learns from(un-labeled)examples how to set the parameters to the chip in order to obtaina desired motor behavior.1IntroductionModern robots still lag far behind animals in their capability for legged locomotion.Four-legged animals use distinct walking gaits[1],resulting for example in reduction of energy consumption at high speeds[5].Similarly,the use of different gaits can allow legged robots to adjust their walking behavior not only for speed but also to the terrain they encounter. Coordinating the rhythmic movement patterns necessary for locomotion is a difficult task involving a large number of mechanical degrees of freedom(DOF)and input from many sensors,and considerable advantages may be gained by emulating control architectures found in animals.Neuroscientists have found increasingly strong evidence during the past century to support the hypothesis that centers in the nervous system,called Central Pattern Generators(CPGs),generate rhythmic output responsible for coordinating the large number of muscles needed for locomotion[2].CPGs are influenced by signals from the brain stem and the cerebellum,brain structures in which locomotive adaptation is believed to take place[11].This architecture greatly simplifies the control problem for the brain.The brain only needs to set the general level of activity,leaving it to the CPG to coordinateMuscles SensorsBrainBAFigure 1:A:Sketch of the control architecture of the robot.Thick arrows indicate the learning loop.B:Sketch of simplified control architecture for locomotion of vertebrates.the complex pattern of muscle activity required to generate locomotion [3].We make use of these biological findings by implementing a similar control architecture to control a walking machine.A neuromorphic Gait Controller (GC)chip produces time varying output voltages that control the movement of the robot’s legs.Their frequency,phase relationships and duty cycles determine the resulting walking behavior (step frequency,walking gait and direction of motion)and depend on a small set of control parameters.For an ideal system,the relationship between control parameters and output voltages can be determined analytically,but deviations of the chip from ideal have to be compensated for by other means.Since the goal here is that the resulting machine works autonomously,we propose a learning procedure to solve this problem.The robot is given the specifications of the desired movement sequence and explores parameter combinations in the input parameter space of its GC chip,keeping only those leading to a movement that is correct within some tolerance.It then locates the region in input parameter space that contains most of these parameter combinations using an algorithm [7]that extends SV learning to unlabelled data.2The robotic systemThe robotic system consists of (i)a body with one degree of freedom per leg 1and a po-tentiometer attached to each motor that serves as a sensor providing information about the angular displacement of the leg,(ii)the neuromorphic Gait Controller (GC)chip and (iii)a PC on which algorithms are run to (a)acquire data from chip and sensors,(b)set the chip’s input parameters and (c)implement the learning algorithm.The control architecture is inspired by the architecture which is used for locomotion in vertebrates (see Fig.1and Sec.1).Like in biology,the existence of the GC chip considerably simplifies the control task.The computer only needs to set the input parameters to the GC chip,leaving the chip to coordinate the pattern of motor movements necessary to generate locomotion of the robot.The circuitry on the GC chip is based on an analysis [8]of circuits originating from M.W.Tilden (e.g.[4]).The GC chip contains five oscillators which can be inter-connected in different ways.The chip can be used in three reasonable configurations,one of which,a chain of four oscillators (Fig.2),is used in the present work.Each oscillator (see Fig.3)consists of two similar sub-circuits.In the following,subscriptswill denote the oscillator identity and subscripts will denote one of the two sub-circuits within an oscillator.Here stands for the left side of the oscillator circuit and for the right side.Each sub-circuit has a capacitor connecting an input node to a node ,to which theinput node of an inverter is connected.The output node of the inverter is called.Left Front LegRight Front LegRight Front LegLeft Hind LegFigure 2:Sketch of the configuration in which the chip is used:a chain of four coupled oscillators.Each of the thin lines is connected to a pad.The round symbols stand for oscillators numbered corresponding to the text.The thick arrows stand for the transmission gates which couple the oscillators (see circuit diagram in Fig.3).The arrows that lead to the four legs represent the outputs of the oscillators.Finally,a n-FET transistor with gate voltage is connected between and ground.An oscillator is obtained by connecting the input node of one sub-circuit to the output node of the other and vice versa.The output voltages of a single oscillator are two mirror-imagestep functions atand .These voltages control the stepping movements of one leg.Two oscillators,and (),are coupled with two transmissiongates.One is connected betweenand .The current that flows through it de-pends on the bias voltage.Likewise,the other transmission gate connects and and has the bias voltage .Note that the coupling is asymmetric,af-fecting only oscillator.The voltages at the input nodes to the inverters of oscillator are not affected by the coupling since the inverters act as impedance buffers.The chip’s output is characterized by the frequency (common to all oscillators),the four duty cycles of the oscillators and three phase lags between oscillators.The phase lags determine which gait the robot adopts.The duty cycles of the oscillators set the ratio be-tween stance and swing phase of the legs.Certain combinations of duty cycles differingfrom 50%make the robot turn [8].For a set of constant input parameters,,,,a rhythmic output is produced with oscillation period ,duty cyclesand phase shifts ,where and .Analysis of the resulting cir-cuit reveals [8]how the output characteristics of the chip depend on the input parameters.Assume that all transistors on the chip are identical and that the peak voltages at node and at node are identical and equal to .For a certain range of input parameters,the period of the oscillators is given by the period of the first oscillator in the chain (called the master oscillator)(1)where F is the capacitance and is the drain source leakage currentof the n-FET.The threshold voltage of the inverter,V ,is calculated from the process parameters [9].V ,A and are estimated with a least squares fit to the data (Fig.4a).is the temperature,the Boltzmann constant and the electron charge.Let the duty cycle be defined as the fractionof the period during whichis high.The master oscillator’s duty cycle isFigure3:Two oscillators are coupled through a transmission gate.The gate voltage on the n-FET of each transmission gate is set to be the complementary voltage of the p-FET of the same transmission gate by the circuits which are drawn next to the transmission gates. These circuits are controlled by the bias voltages and and copy the voltages and,to nodes2and4,respectively,while the voltages at nodes 1and3are and,respectively.The symbols on the right correspond to the symbols in Fig.2.where V and3LearningIn theory,all duty cycles should be1/2for the symmetric circuit.In the real system,the duty cycle changes with the phase lag(see Fig.4c)due to transistor mismatch.Thus,to obtain a duty cycle of1/2,might have to differ from.Parameter points which lead to both a desired phase lag and a duty cycle of1/2lie in a two dimensional space spanned by and.These parameters are learned2.First,a subset in this input parameter space is chosen according to the estimate given by(3).is scanned and at each point,the output characteristics of the GC chip are determined.If they match the desired output characteristics(within specified tolerances),this point is added to the training data set.After the scan is completed,the training data is transformed by a feature map,into a feature space such that if,the dot(a)0.30.40.50.624681012P / s 4.22 4.24 4.26 4.280.50.60.70.80.91V b,12 / VV b / V0.50.60.70.80.9100.10.20.30.40.5D 2 φ1φ1(b)(c)Figure 4:(a):Oscillation period(points =data,3%error)as a function of the bias voltage .implies that the duty cycle of the oscillation is 1/2(see (2)).The oscillation period follows (1)(solid line).(b):Phase lag between the first two oscillators in a chain of 4oscillators.Function given in (3)(solid line)and data points.and are as determined in (a),,as estimated by data fitting is A.(c):Duty cycle of the second oscillator in a chain of 4oscillators as a function of the phase lag between oscillators 1and 2.product can be computed by evaluation of the Gaussian kernel (which fulfills Mercer’s condition [6])(4)In feature space,a hyperplaneseparating most of the data from the origin with large margin is found by solving the constrained optimization problem (see [7])(5)subject to(6)A decision function,,is computed,which is +1on a region in input space capturing most of the training data points and -1elsewhere.The approximate geometrical center of this region is used as the input to the GC chip.The algorithmic implementation of the learning procedure uses the quadratic optimizer LOQO implementing a primal-dual interior-point method [10].The parameter upper bounds the fraction of outliers (see [7],Proposition 4),which is related to the noise that the training data is subject to.In our experiments,is chosen such that the algorithm disregards approximately as many points as canbe expected to be falsely included in the training data given the noise of the data acquisition.4ResultsAs an example,the input parameters are learned for a forward walk,requiring phase shiftsofand duty cycles of .The oscillation periods and the duty cycle are set according to (1)and (2).The value of takes the mechanics of the robot into account [8].The scanning step size is2mV and the tolerances are chosen to befor the phase lags and for the duty cycles.The parametersand are learned in sequence,first for (see Fig.5).The result is applied to the GC chip.Then and are learned andthe result is also applied to the GC chip.Finally,and are learned.All input parameters of the GC chip are set to the learned values and the robot moves forward using a walk gait (see Fig.6).The phase relationships of the robot’s leg movements are measured.Simultaneously,the robot’s trajectory is tracked using a video camera monitoring the robot from above,and two Light Emitting Diodes (LEDs)attached to the robot’s front and rear.The robot has learned to move in the forward direction,using a walk gait,as desired,despite the inability to theoretically predict the exact values of the GC chip’s bias voltages.4.284.2954.31 4.325 4.344.244.2554.274.2854.3Vb,12,rV b ,12,lFigure 5:Result of learning values of the bias voltages and which lead toand .Correctly classified (stars)and misclassified (crosses)trainingdata (left)and test data (right).Outlined regions:learned by algorithm from training data.The training data is obtained from one scan of the displayed rectangular region .The test data is a set of points obtained from three scans.5DiscussionWe have introduced a novel neuromorphic chip for inter-leg coordination of a walking machine.This chip successfully controls a four-legged robot.A Support Vector algorithm enables the robotic system to learn a desired movement sequence.We have demonstrated this here using the walk gait as an example.Other gaits have also been learned [8].The architecture we used reduced the learning of a complex motor behavior to a classification task.The classifier we used requires only a few examples,making the learning efficient,and it can handle noisy data,making the learning robust.The chip need not be interfaced to a computer;it can control the robot without any need of software once the input parameters of the chip are known.Note,that the chips bias voltages can also be changed by simple sensors in a direct way,enabling the robot to adapt its behavior according to sensory information.This point is elaborated in [8].However,the chip-computer interface creates a hybrid system in which the complex movement pattern required to make a four legged machine locomote is controlled by the chip,while algorithms run on the computer can focus on more demanding tasks.This architecture enables the robotic system to exploit the motor abilities it has due to the GC chip –independent of the particular physical shape of the robot.The hybrid system could also be useful for the development of a second generation of neu-romorphic motor control chips,able to solve more complex tasks.Furthermore,the control circuit could easily be extended to the control of six (or more)legs simply by addition of two (or more)oscillators,without increasing drastically in complexity,as the number of control parameters is small and scales linearly with the number of oscillators.Similarly,the circuit could be expanded to control -jointed legs if each of the four oscillators becomes itself the master of a chain of oscillators.Finally,the learning procedure intro-duced here could be used as a general method for fine tuning of neuromorphic aVLSI chips.AcknowledgmentsS.S.is grateful to the late Misha Mahowald for inspiring discussions and indebted to Mark W.Tilden for discussing his circuits.We thank Adrian M.Whatley for useful comments and technical assistance.For helpful discussions we thank William Bialek,Gert Cauwenberghs,Giacomo Indiveri,Shih-Chii Liu,John C.Platt,Alex J.Smola,John Shawe-Taylor and Robert C.Williamson.S.S.was supported by CSEM,Neuchˆa tel,the Physics Department of ETH Z¨u rich and the SPP of the Swiss National Science Foundation.a n g u l a r d i s p l a c e m e n t Figure 6:Left:Control voltages (upper plot)and angular displacements of the legs as measured by potentiometers that are attached to the motors (lower plot)as a function of time shown for a cycle of the rhythmic movement.The four legs are distinguished by the abbreviations:left front (LF;dots),right front (RF;circles),left hind (LH;crosses)and right hind (RH;stars).The legs move in succession with a phase shift of :LF,RH,RF,and finally LH,a typical walk sequence [1].Note that the data is acquired with a limited sampling rate.Thus the duty cycles of the control voltages appear to deviate from 50%.However,the data on the right proves that the duty cycles are sufficiently close to 50%to cause the robot to walk forward in a straight line,as desired.Right:Position of the robot’s center of gravity as a function of time.Upper plot:x-coordinate,lower plot:y-coordinate.Errors are due mainly to the extension of the images of the LEDs in the image frames obtained from the CCD camera.The y-coordinate is constant within the error.This shows that the robot moves forward on a straight line.The robot moves at roughly 4.7cm s .References[1]R.McN.Alexander,The Gaits of Bipedal and Quadrupedal Animals.Intl.J.Robotics Research ,1984,3,pp.49-59[2]F.Delcomyn,Neural Basis of Rhythmic Behaviour in Animals.Science ,1980,210,pp.492-498[3]S.Grillner,1981,Control of locomotion in bipeds,tetrapods and fish.In:Handbook of Physiology II ,M.D.Bethesda (ed.),Am.Physiol.Soc.,pp.1179-1236;S.Grillner,1998,Vertebrate Locomotion -A Lamprey Perspective.In:Neuronal Mechanisms for Generating Locomotor Activity ,O.Kiehn et.al.(eds.),New York Academy of Science[4]B.Hasslacher &M.W.Tilden,Living Machines.Robotics and Autonomous Systems:The Biology and Technology of Intelligent Autonomous Agents ,1995,L.Steels (ed.),Elsevier;S.Still &M.W.Tilden Controller for a four legged walking machine.In:Neuromorphic Systems ,1998,L.S.Smith &A.Hamilton (eds.),World Scientific[5]D.F.Hoyt &R.C.Taylor,Gait and the energetics of locomotion in horses.Nature ,1981,292,pp.239-240[6]J.Mercer,Functions of positive and negative type and their connection with the theory of integral equations.Phil.Trans.Roy.Soc.London A ,1909,209,pp.415-446[7]B.Sch¨o lkopf,J.C.Platt,J.Shawe-Taylor,A.J.Smola and R.C.Williamson,Estimating the Support of a High-Dimensional Distribution.Technical Report,Microsoft Research,1999,MSR-TR-99-87,Redmond,WA,To appear in Neural Computation .[8]S.Still,Walking Gait Control for Four-legged Robots ,PhD Thesis,ETH Z¨u rich,2000[9]N.H.E.Weste &K.Eshraghian,Principles of CMOS VLSI Design ,1993,Addison Wesley[10]R.J.Vanderbei,LOQO User’s Manual –Version 3.10,Technical Report,SOR-97-08,Princeton University,Statistics and Operations Research,1997[11]D.Yanagihara,M.Udo,I.Kondo and T.Yoshida,Neuroscience Research ,1993,18,pp.241-244。