Statistical Physics and Dynamical Systems Models of Phase Transitions
4.MIT教材《Introduction to Statistical Physics》评介研究
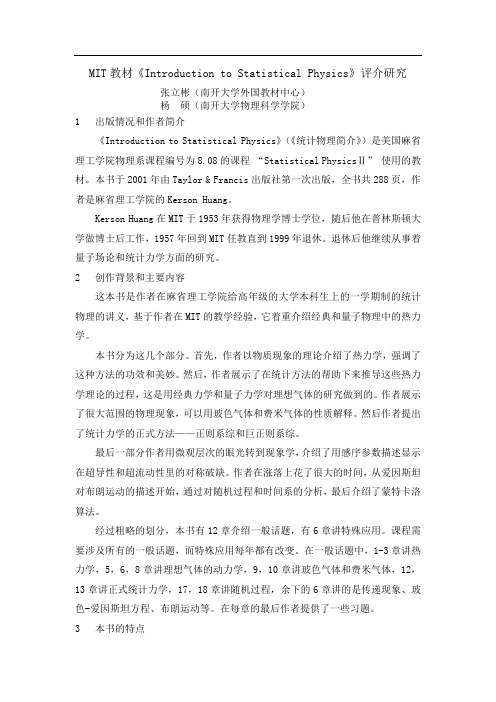
MIT教材《Introduction to Statistical Physics》评介研究张立彬(南开大学外国教材中心)杨硕(南开大学物理科学学院)1 出版情况和作者简介《Introduction to Statistical Physics》(《统计物理简介》)是美国麻省理工学院物理系课程编号为8.08的课程“Statistical PhysicsⅡ”使用的教材。
本书于2001年由Taylor & Francis出版社第一次出版,全书共288页,作者是麻省理工学院的Kerson Huang。
Kerson Huang在MIT于1953年获得物理学博士学位,随后他在普林斯顿大学做博士后工作,1957年回到MIT任教直到1999年退休。
退休后他继续从事着量子场论和统计力学方面的研究。
2 创作背景和主要内容这本书是作者在麻省理工学院给高年级的大学本科生上的一学期制的统计物理的讲义,基于作者在MIT的教学经验,它着重介绍经典和量子物理中的热力学。
本书分为这几个部分。
首先,作者以物质现象的理论介绍了热力学,强调了这种方法的功效和美妙。
然后,作者展示了在统计方法的帮助下来推导这些热力学理论的过程,这是用经典力学和量子力学对理想气体的研究做到的。
作者展示了很大范围的物理现象,可以用玻色气体和费米气体的性质解释。
然后作者提出了统计力学的正式方法——正则系综和巨正则系综。
最后一部分作者用微观层次的眼光转到现象学,介绍了用感序参数描述显示在超导性和超流动性里的对称破缺。
作者在涨落上花了很大的时间,从爱因斯坦对布朗运动的描述开始,通过对随机过程和时间系的分析,最后介绍了蒙特卡洛算法。
经过粗略的划分,本书有12章介绍一般话题,有6章讲特殊应用。
课程需要涉及所有的一般话题,而特殊应用每年都有改变。
在一般话题中,1-3章讲热力学,5,6,8章讲理想气体的动力学,9,10章讲玻色气体和费米气体,12,13章讲正式统计力学,17,18章讲随机过程,余下的6章讲的是传递现象、玻色-爱因斯坦方程、布朗运动等。
自旋玻璃与消息传递算法Spin Glass and Message-Passing 概要
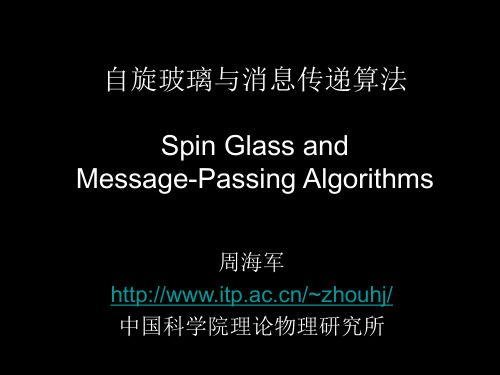
New vertex partially covered
New vertex always covered
39
Mean-field theory result is lower than experimental values for c > e=2.7183
2.7183
40
假定的相空间结构
41
14
distribution of equilibrium free-energies (contl.)
2
15
16
Which thermodynamic states contribute to the equilibrium properties? • If • If
Excited macrostates matter!
thevertexcoverproblem38alwaysuncoveredalwayscoveredunfrozenweigthartmannprl2000pre200139newvertexuncoverednewvertexpartiallycoverednewvertexalwayscovered40meanfieldtheoryresultislowerthanexperimentalvaluesforce271832718341假定的相空间结构42引入参数y43neighborsvertexiprobabilityvcsizeincreasereweightedprobabilityallunfrozenoralwayscoveredalwaysuncovered0atleastonealwaysuncoveredunfrozenoralwayscovered1444546同样的消息传递的算法可以用于解决神经网络?信息系统?满足性问题?
journal of statistical physics分区
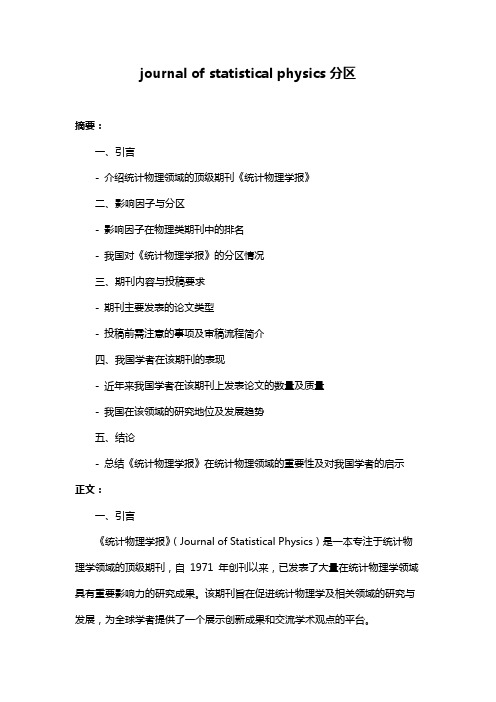
journal of statistical physics分区摘要:一、引言- 介绍统计物理领域的顶级期刊《统计物理学报》二、影响因子与分区- 影响因子在物理类期刊中的排名- 我国对《统计物理学报》的分区情况三、期刊内容与投稿要求- 期刊主要发表的论文类型- 投稿前需注意的事项及审稿流程简介四、我国学者在该期刊的表现- 近年来我国学者在该期刊上发表论文的数量及质量- 我国在该领域的研究地位及发展趋势五、结论- 总结《统计物理学报》在统计物理领域的重要性及对我国学者的启示正文:一、引言《统计物理学报》(Journal of Statistical Physics)是一本专注于统计物理学领域的顶级期刊,自1971 年创刊以来,已发表了大量在统计物理学领域具有重要影响力的研究成果。
该期刊旨在促进统计物理学及相关领域的研究与发展,为全球学者提供了一个展示创新成果和交流学术观点的平台。
二、影响因子与分区《统计物理学报》自创刊以来,影响因子逐年上升,现已成为物理类期刊中的佼佼者。
在我国,该期刊被评定为SCI-E(科学引文索引扩展版)收录期刊,并位于物理类分区的一区,表明其在统计物理学领域具有很高的学术地位。
三、期刊内容与投稿要求《统计物理学报》主要发表关于统计物理学基本理论、方法及其在各个领域的应用等方面的研究论文。
投稿前,作者需确保论文内容符合期刊的范畴,遵循学术道德规范,并且尚未在其他刊物上发表过。
投稿后,论文将经过同行评审,整个审稿流程旨在确保论文质量及学术价值。
四、我国学者在该期刊的表现近年来,我国学者在《统计物理学报》上发表的论文数量逐年增加,论文质量也得到了显著提高。
这表明我国在统计物理学领域的研究地位逐渐上升,为全球科学研究作出了重要贡献。
同时,我国在该领域的发展趋势也呈现出良好的态势,有望在不久的将来取得更多的突破性成果。
五、结论《统计物理学报》作为统计物理学领域的顶级期刊,对我国学者在该领域的研究具有重要意义。
上海交通大学能动培养方案
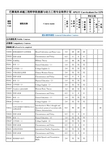
2.0
32
32
32
FR419 人文社科与经管 (2) FR420 人文社科与经管 (3)
2.0 2.0 13.0
32 32 208
32 32 208
32 32 208
通识教育实践 General Education Practice 必修课Compulsory Courses 须修满全部 all need to be completed XP000
MA160 高等数学Ⅱ PH124 大学基础物理 CA041 物质结构导论 FR142 跨学科数值方法实践
Advanced Mathematics II Fundamental physics Introduction to the Structure of Matter Multidisciplinary Project 总
巴黎高科卓越工程师学院能源与动力工程专业培养计划 SPEIT Curriculum for EPE Majo
学时分配
课程 代码 Code
C r e d i t
课程名称
Course name
总 学 分
C 排 T 总 l 课 H学 a 学 r 时 s 时 s
L e c t .
理 论 教 学
实 验
E X P T
96 320 64 64 32 32 32 48 32 72 304 32 64 64 16 16 48 120 16 16 16 16 16 16 0 0
FR126 初级法语语法(2) FR129 初级综合法语(2) FR134 科技法语 (2) MA159 高等数学Ⅰ
Elementary French Grammar (2) Elementary Comprehensive French 2 French for Sciences (2) Advanced Mathematics I
journal of statistical physics分区 -回复

journal of statistical physics分区-回复Journal of Statistical Physics的分区情况。
首先,让我们先了解一下Journal of Statistical Physics(简称JSP)的概况。
JSP是由Springer Science+Business Media公司出版的学术期刊,于1969年首次发行。
它是一本国际性的学术期刊,涵盖了统计物理学领域的研究成果。
JSP旨在将统计物理学的理论和实证研究发表为一流的学术论文,并为研究人员提供学术交流的平台。
那么JSP的分区情况是如何的呢?JSP的分区情况是按照期刊影响因子(Journal Impact Factor)进行划分的。
期刊影响因子是一种衡量期刊影响力的指标,它反映了一定时间内论文被引用的频次。
根据InCites Journal Citation Reports的数据,JSP的最新期刊影响因子为1.662(2021年数据),处于物理学类期刊的中上水平。
根据期刊影响因子的大小,JSP一般被划分为不同的分区。
目前,根据InCites Journal Citation Reports的数据,JSP被分区为物理学类期刊的Q2(二分区)。
JSP被分区为Q2意味着它在物理学领域中的影响力较高,但仍然有一定的提升空间。
处于Q2的期刊通常被认为是具有较高学术水平并受到广泛关注的期刊,但并不是顶级期刊。
在该分区的期刊中,每篇论文的平均被引用次数可能在10到20次之间。
除了影响因子外,JSP还有其他指标用于衡量其学术影响力。
例如,在Google学术(Google Scholar)中,JSP的h指数(h-index)为112,这意味着至少有112篇JSP的论文被引用了112次以上。
总结起来,Journal of Statistical Physics(JSP)是一本国际性的学术期刊,以其在统计物理学领域的研究成果而闻名。
热力学统计物理的教与学
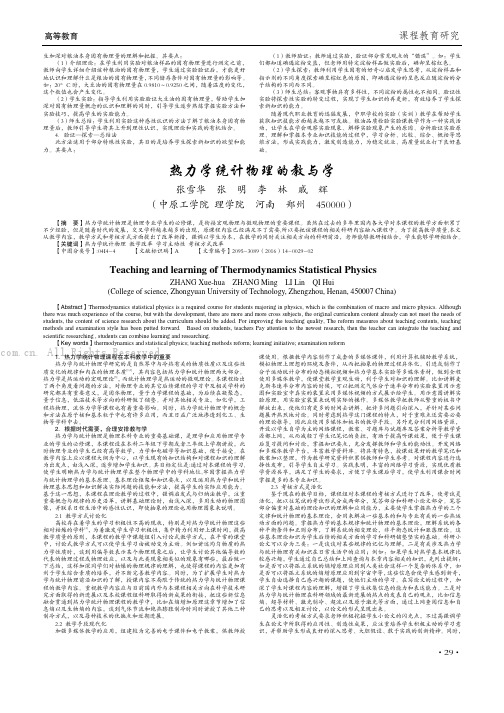
高等教育 课程教育研究 ·29·生加深对粮油本身固有物理量的理解和把握。
其要点:(1)介绍理论:在学生利用实验对粮油样品的固有物理量进行测定之前,教师向学生详细介绍该种粮油的固有物理量,学生通过实验验证后,才能更好地认识和理解什么是粮油的固有物理量,不同储存条件对固有物理量的影响等。
如:20°C 时,大豆油的固有物理量在0.9810~0.9250之间,随着温度的变化,这个数值也会产生变化。
(2)学生实验:指导学生利用实验验证大豆油的固有物理量,帮助学生加深对固有物理量概念的认识和理解的同时,引导学生逐步熟练掌握实验方法和实验技巧,提高学生的实验能力。
(3)师生总结:学生利用实验这种感性认识的方法了解了粮油本身固有物理量后,教师引导学生将其上升到理性认识,实现理论和实践的有机结合。
4.验证—探索—总结法此方法适用于部分特殊性实验,其目的是培养学生探索新知识的欲望和能力。
其要点:(1)教师验证:教师通过实验,验证部分常见观点的“错误”。
如:学生们都知道碘遇淀粉变蓝,但老师用特定淀粉样品做实验后,碘却呈棕红色。
(2)学生探索:教师利用学生固有的好奇心启发学生思考,从淀粉样品和指示剂的不同角度探索碘呈棕红色的原因,即碘遇淀粉的呈色反应随淀粉的分子结构的不同而不同。
(3)师生总结:客观事物具有多样性,不同淀粉的属性也不相同。
验证性实验将探索性实验的转变过程,实现了学生知识的再更新,有效培养了学生探索新知识的能力。
随着现代职业教育的迅猛发展,中职学校的实验(实训)教学在帮助学生获取知识技能方面越来越不可或缺。
粮油品质检验实验课教学作为一种实践活动,让学生在学会观察实验现象、解释实验现象产生的原因、分析验证实验原理、理解和掌握本专业知识技能的过程中,学习分析、比较、综合、概括等思维方法,形成实践能力,激发创造能力,为稳定就业、高质量就业打下良好基础。
热力学统计物理的教与学张雪华 张 明 李 林 戚 辉(中原工学院 理学院 河南 郑州 450000)【摘 要】热力学统计物理是物理专业学生的必修课,是衔接宏观物理与微观物理的重要课程。
Stochastics and Dynamics c ○ World Scientific Publishing Company OPTIMAL PERTURBATION OF U

Stochastics and Dynamicsc World Scientific Publishing CompanyOPTIMAL PERTURBATION OF UNCERTAIN SYSTEMSBRIAN F.FARRELL∗Division of Engineering and Applied Sciences,Harvard UniversityPierce Hall,29Oxford Street,Cambridge,MA02138,U.S.A.PETROS J.IOANNOU†Physics Department,Capodistrian and National University of AthensPanepistimiopolis,15784Zografos,GreeceReceived(received date)Revised(revised date)In studies of perturbation dynamics in physical systems certain specification of the gov-erning perturbation dynamical system is generally lacking,either because the perturba-tion system is imperfectly known or because its specification is intrinsically uncertain,while a statistical characterization of the perturbation dynamical system is often avail-able.In this report exact and asymptotically valid equations are derived for the ensemblemean and moment dynamics of uncertain systems.These results are used to extend theconcept of optimal deterministic perturbation of certain systems to uncertain systems.Remarkably,the optimal perturbation problem has a simple solution:in uncertain sys-tems there is a sure initial condition producing the greatest expected second momentperturbation growth.Keywords:List four to six.1.IntroductionLinear stability theory has been extensively studied because of its role in advancing understanding of a wide range of physical phenomena related to the structure and growth of perturbations to dynamical systems.Historically,linear stability theory was developed using the method of modes(Rayleigh[13]).However,the method of modes is incomplete for understanding perturbation growth even for autonomous systems because the non-normality of the linear operator in physical problems often produces transient development of a subset of perturbations that dominates the physically relevant growth processes(Farrell[4]).Recognition of the role of non-normality in linear stability led to the development of Generalized Stability Theory (Farrell and Ioannou[6]).Compared to the methods of modes,the methods of Generalized Stability Theory,which are based on the non-normality of the linear operator,allow a far wider class of stability problems to be addressed including ∗farrell@†pji@cc.uoa.grB.F.Farrell,P.J.Ioannouperturbation growth associated with aperiodic time dependent certain operators to which the method of modes does not apply(Farrell and Ioannou[7]).The problems to which Generalized Stability Theory has been applied hereto-fore involve growth of perturbations in a system with no time dependence or a system with known time dependence;perturbations to these systems may be sure or stochastically distributed and may be imposed at the initial time,or distributed continuously in time,but the operator to which the perturbation is applied is con-sidered to be certain and the problem is that of the stability of a certain operator. However,it may happen that we either do not have complete knowledge of the system that is being perturbed or that exact specification may be inappropriate to the physical system in which case the problem is to determine the stability of an uncertain operator.In Generalized Stability Theory the perturbation produc-ing greatest growth plays a central role in quantifying the stability of the system. In this report we obtain exact dynamical equations for the perturbation ensemble mean and covariance and use these results to obtain the optimal perturbation to uncertain systems.2.Exact equations for the ensemble meanConsider the uncertain linear system for the vector stateψ:dψ=Aψ+ η(t)Bψ(2.1)dtwhere A is the ensemble mean matrix,η(t)is a stochastic process with zero mean, B is the matrix of thefluctuation structure and is an amplitude parameter.Equa-tions for the evolution of the ensemble meanfield,<ψ(t)>,and for the ensemble mean covariance,<ψi(t)ψ∗j(t)>,can be readily obtained ifηis a white noise pro-cess(Arnold[1]).Although it is a great advantage for analysis to assume thatηis a white noise process,this assumption is often hard to justify in physical contexts because the uncertainties in physical operators are often red and because white noise processes producing non-vanishing variance in(2.1)have unboundedfluctua-tions that in a physical context would imply,for example,infinite wind speeds or unbounded negative damping rates.Approximate dynamical equations for the ensemble meanfield evolving under uncertain dynamics and not restricted to white noise stochastic processes have been obtained by Bourret[2],Keller[8],Papanicolaou and Keller[11]and Van Kampen [14].These approximations are accurate for small Kubo number K= t c,where t c is the autocorrelation time of the stochastic processη(t).In addition,exact evolution equations for the ensemble mean can be obtained whenη(t)is a telegraph process;results which are discussed in Brissaud&Frisch[3].Finally,making use of the properties of cumulant expansions it is possible to obtain an exact expression for the evolution of the ensemble mean covariance for the case in whichη(t)is a Gaussian process as follows:Theorem2.1.Ifη(t)is a Gaussian stochastic process with zero mean,unit vari-ance,and autocorrelation time t c=1/νso that<η(t1)η(t2)>=exp(−ν|t1−t2|)Optimal Perturbation of Uncertain Systems then the ensemble mean of the stochastic equation(2.1)obeys the deterministic equa-tion:d<ψ>dt =A+ 2B D(t)<ψ>,(2.2)whereD(t)=te A s B e−A s e−νs ds.(2.3) Proof.Consider the interaction perturbationφ(t)defined byψ(t)≡e A tφ(t),(2.4) in which Eq.(2.1)becomes:dφdt= η(t)e−A t B e A tφ= η(t)H(t)φ,(2.5) whereH(t)≡e−A t B e A t.(2.6) For a sure initial perturbation we haveφ(0)=ψ(0)and the interaction perturbation at time t is:φ(t)=G(t)ψ(0),(2.7) with the fundamental matrix G(t)given byG(t)=I+t0η(t1)H(t1)dt1+ 2tdt1t1dt2η(t1)H(t1)η(t2)H(t2)+···(2.8)This expression for the fundamental matrix defines the time-ordered exponential:G(t)=exp otη(s)H(s)ds.(2.9)The subscript o denotes time ordering and the term exponential is used because Eq.(2.8)is obtained if the argument of Eq.(2.9)is expanded as an exponential with the convention that the terms are grouped in ascending time order.Note that time ordered exponentials of matrices satisfy the familiar properties of exponen-tials of scalars even for matrices that do not commute;for example,we can write exp o(H(t1)+H(t2))=exp o(H(t1))exp o(H(t2))even if the matrices H(t1)and H(t2)do not commute if time ordering is enforced meaning that in all products H(t1)is placed to the left of H(t2).Kubo[9]has shown that the average of the time ordered exponential in Eq.(2.9)can be expanded in the cumulants ofη(t)in the same way that the exponents of ordinary stochastic processes are expanded in cumulants of their arguments:<G(t)>=exp o(F(t)),(2.10)B.F.Farrell,P.J.IoannouwhereF (t )=22! t 0 t 0dt 1dt 2<<η(t 1)η(t 2)>>H (t 1)H (t 2)+···+ 2n n ! t 0··· t 0dt 1···dt n <<η(t 1)···η(t n )>>H (t 1)···H (t n )+···,(2.11)and in which <<·>>denote the cumulants of η.Note that exp o (F (t ))in Eq.(2.10)is an abbreviation in the sense that in order to evaluate this expression we must first expand it in powers of its argument observing the time ordering.For a Gaussian process all cumulants of order higher than 2vanish and because <<η(t 1)η(t 2)>>=<η(t 1)η(t 2)>we obtain for this case:<G (t )>=exp o 22! t 0 t 0dt 1dt 2H (t 1)H (t 2)<η(t 1)η(t 2)> =exp o 22! t 0 t 0dt 1dt 2H (t 1)H (t 2)e −ν(t 1−t 2) .(2.12)Consequently,the ensemble average of the interaction perturbation obeys the equa-tion:d <φ>dt = 2H (t ) t 0ds H (s )e ν(t −s ) <φ>,(2.13)from which we obtain,d <ψ>dt=A <ψ>+e A t d<φ>dt =A <ψ>++ 2e A t H (t ) t 0ds H (s )e ν(t −s )e −A t <ψ>,(2.14)giving the exact evolution equation for the ensemble average perturbation:d <ψ>dt = A + 2B t 0e A s B e −A s e −νs ds <ψ>.(2.15)3.Approximate equations for the ensemble meanWe seek approximations to the ensemble mean equation (2.2)that are valid for short autocorrelation time,t c =1/ν<<1.The integrand defining the matrix D (t )given by Eq.(2.3)can be expanded ase A s B e −A s e −νs =e −νs B +s [A ,B ]+s 22![A ,[A ,B ]]+··· ,(3.16)where [A ,B ]≡AB −BA is the commutator.The ensemble mean Eq.(2.2)then takes the exact form:d <ψ>dt = A + 2B I 0B +I 1[A ,B ]+I 22![A ,[A ,B ]]+··· <ψ>,(3.17)Optimal Perturbation of Uncertain Systemswhere I n= ts n e−νs ds.Because I n+1=O(1/νn+1),for1/ν<<0the ensemblemean accurately evolves by retaining only thefirst power of1/νin Eq.(3.17).In that limit the ensemble mean equation becomes:d<ψ>dt =A+2νB2<ψ>.(3.18)This equation is identical to the ensemble mean equation obtained forη(t)a white noise process,in which case as the limitν→∞is taken thefluctuation amplitude also increases so that the ratio 2/νapproaches a constant;and Eq.(3.18)becomes the equation appropriate for a white noise processη(t)with variance2 2/νin thephysically relevant Stratonovich limit(Arnold,[1]).4.Obtaining the optimal perturbations of uncertain systemsDefinition4.1.The optimal perturbation of an uncertain linear dynamical system for time t is the unit magnitude initial(t=0)perturbation that maximizes the expected perturbation magnitude in the chosen norm at time t.The expected growth in magnitude of the optimal perturbation is called the optimal growth.For deterministic dynamical systems governed by non-normal operators the op-timal growth in the L2norm is the2-norm of the fundamental matrix evolved to time t and the optimal perturbations can be readily found by a Schmidt decom-position(singular value decomposition)of the fundamental matrix at time t.We extend this result for obtaining the optimal perturbations to take account of the uncertainty in the dynamics as follows:Theorem4.1.The optimal perturbation at time t of the uncertain dynamical sys-tem(2.1)is the eigenfunction associated with the largest eigenvalue of the Hermitian matrix<S(t)>that is obtained by integrating to time t the differential equation:d<S>dt =A+ 2E(t)B†<S>+<S>A+ 2E(t)B++ 2E(t)†<S>B+B†<S>E(t)(4.19)where,E(t)=te−A t B e A t e−νt dt ,(4.20)with initial condition<S(0)>=I.Proof.LetΦ(t,0)be the fundamental matrix associated with each realization of the operator A+ η(t)B.We seek the initial perturbation leading to greatest expected perturbation magnitude at future time,t.At time t the perturbation square amplitude for each realization of thefluctuations is:ψ†(t)ψ(t)=ψ†(0)Φ†(t,0)Φ(t,0)ψ(0),(4.21)B.F.Farrell,P.J.Ioannouwhereψ(0)the initial state.It is apparent from this expression that the eigenvector of the hermitian matrix:S(t)=Φ†(t,0)Φ(t,0)(4.22) with largest eigenvalue is the initial condition leading to greatest perturbation mag-nitude at time t for that realization of thefluctuations.The other eigenvectors of S(t)complete the set of mutually orthogonal initial conditions ordered according to their growth at time t.The hermitian matrix S(t)can be determined by integrating forward the equa-tion:d Sdt=(A+ η(t)B)†S+S(A+ η(t)B).(4.23) The initial perturbation resulting in maximum mean square perturbation mag-nitude at time t is the eigenfunction corresponding to the largest eigenvalue of the mean of S(t).We need therefore to obtain the mean evolution equation correspond-ing to the matrix Eq.(4.23).This can be readily achieved using the results of the previous section byfirst expressing Eq.(4.23)as a vector equation using tensor products.This is done by associating with the n×n matrix S(t)the n2column vector s(t)formed by consecutively stacking the columns of S(t).In tensor form Eq.(4.23)becomes:dsdt=A s+ η(t)B s,(4.24) in which:A=I⊗A†+A T⊗I,B=I⊗B†+B T⊗I,(4.25) where T denotes the transposed matrix and†the Hermitian conjugate matrix. The ensemble average evolution equation for s over Gaussian realizations ofη(t), indicated by<s>,is obtained by applying Theorem(2.1)to(4.24)with result:d<s>dt =A+ 2BD(t)<s>,(4.26)whereD(t)= te A t B e−A t e−νt dt .(4.27)Because e A t=e A T t⊗e A†t repeated application of the tensor product properties givesD(t)=I⊗E(t)†+E(t)T⊗I,(4.28) whereE(t)=te−A t B e A t e−νt dt .(4.29) The ensemble average evolution equation can then be written as:d<s>dt=L<s>(4.30)Optimal Perturbation of Uncertain Systems in which L is given by:L=I⊗A†+A T⊗I+ 2I⊗B†+B T⊗II⊗E(t)†+E(t)T⊗I.(4.31)Reverting to matrix notation we obtain that the ensemble mean,<S>,obeys the deterministic equation:d<S>dt =A+ 2E(t)B†<S>+<S>A+ 2E(t)B++ 2E(t)†<S>B+B†<S>E(t).(4.32)For short autocorrelation times for the operatorfluctuations corresponding to 1/ν<<1the ensemble mean matrix<S>satisfies the white noise equation:d<S>dt =A+2νB2†<S>+<S>A+2νB2+2 2νB†<S>B.(4.33)In their appropriate limits these equations can be used to obtain<S(t)>and eigenanalysis of<S(t)>in turn determines the optimal initial condition that leads to the largest expected growth in square magnitude at time t.Determining the optimal in this manner also offers constructive proof of the remarkable fact that the optimal initial covariance matrix has rank1implying that a sure initial condition maximizes expected growth in an uncertain system.5.ConclusionsUncertainty in perturbation dynamical systems can arise from many sources including statistical specification of parameters(Sardeshmukh et al[12];Palmer [10])and incomplete knowledge of the mean state.We have obtained dynamical equations for ensemble mean and second moment quantities in such systems that are generally valid and others that are valid in the limit of short autocorrelation times. Optimal perturbation plays a central role in Generalized Stability Analysis and we have used ensemble mean second moment equations to solve for the perturbation producing the greatest expected second moment growth in an uncertain system; remarkably,this optimal perturbation is sure.AcknowledgmentsThe authors thank Ludwig Arnold for helpful discussions.This work was supported by NSF ATM-0123389and by ONR N00014-99-1-0018.References1.L.Arnold,Stochastic Differential Equations:Theory and Applications(Krieger,1992).2.R.C.Bourret,Stochastically perturbedfields,with application to wave propagation inrandom media,Nuovo Cimento26(1962)1-31.B.F.Farrell,P.J.Ioannou3. A.Brissaud and U.Frisch,Solving linear stochastic differential equations,J.Math.Phys.15(1974)524-534.4. B.F.Farrell,The initial growth of disturbances in a baroclinicflow,J.Atmos.Sci.39(1982)1663-1686.5. B.F.Farrell,Optimal excitation of neutral Rossby waves,J.Atmos.Sci.45(1988)163-172.6. B.F.Farrell and P.J.Ioannou,Generalized Stability.Part I:Autonomous Operators,J.Atmos.Sci.53(1996)2025-2041.7. B.F.Farrell and P.J.Ioannou,Perturbation Growth and Structure in Time DependentFlows,J.Atmos.Sci.56(1996)3622-3639.8.J.B.Keller,Wave propagation in random media,Proc.Symp.Appl.Math.13(1962)227-246.9.R.Kubo,Generalized cumulant expansion method,J.Phys.Soc.Japan17(1962)1100-1120.10.T.N.Palmer,A nonlinear dynamical perspective on model error:a proposal for non-local stochastic-dynamic parameterization in weather and climate prediction models, Quart.J.Roy.Meteor.Soc.127279-304.11.G.Papanicolau and J.B.Keller,Stochastic differential equations with applications torandom harmonic oscillators and wave propagation in random media,SIAM J.App.Math21(1971)287-305.12.P.Sardeshmukh,C.Penland and M.Newman,2001:Rossby waves in a stochasticallyfluctuating medium,Progress in Probability49(2001)369-384.13.Lord Rayleigh,On the stability or instability of certainfluid motions,Proc.LondonMath.Soc.11(1880)57-70.14.N.G.Van Kampen,A cumulant expansion for stochastic differential equations,Physica74(1974)239-247.。
自旋玻璃与消息传递算法Spin Glass and Message-Passing 概要
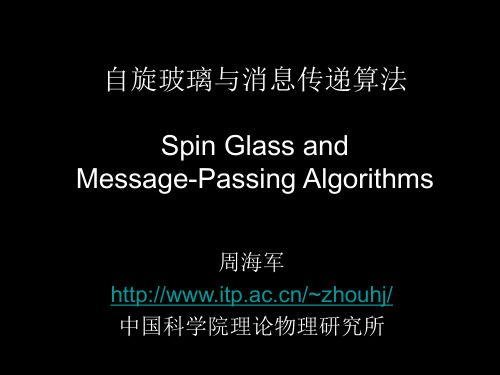
自旋玻璃:无序与阻错系统的简单模型
• 3D regular lattice
(Edwards-Anderson, 1975)
• Complete graph
(Sherrington-Kirkpatrick 1975)
• Random Poisson graph
(Viana-Bray, 1985)
8
11
ergodic vs non-ergodic
?
12
repeated heating—annealing and the equilibrium Gibbs measure
Complexity (复杂度)
13
distribution of equilibrium free-energies
1
……
6
Equilibrium (static) and dynamical properties of complex systems are both difficult and interesting • Quenched randomness, frustration, nonself averaging, …, broken ergodicity. • NP-complete combinatorial optimizations, message-passing algorithms for information science (CDMA, for example!), econo-physics, …, biological systems.
23
How to calculate the grand free energy? The cavity approabrium dynamics of even a simple system may be difficult to understand
- 1、下载文档前请自行甄别文档内容的完整性,平台不提供额外的编辑、内容补充、找答案等附加服务。
- 2、"仅部分预览"的文档,不可在线预览部分如存在完整性等问题,可反馈申请退款(可完整预览的文档不适用该条件!)。
- 3、如文档侵犯您的权益,请联系客服反馈,我们会尽快为您处理(人工客服工作时间:9:00-18:30)。
freezing. A order parameter and correlations with a time scale dependent on
rate of cooling and heating are present. Any model for the kinetics of melting
and the same matrix acting on xn, yn with (−1, 0.5) deducted on the interval
0.5 < xn < 1.
More generally the Baker like transforms ca; 1 and for xn > α < 1.
iteration and a ’unit cell square’.
A point xn, yn goes to xn+1 , yn+1
using matrix mapping (1, 1),(1, 2) with this ’cat map’ having eigenvalues 0.5(3 + / − √5) = exp(+/ − σ).
flows on Riemann spaces gives a intrinsic definition of geodesic deviation equa-
tion and Lyapunov exponents. This has led to defining the connection between
This paper explores the connection between dynamical system properties and statistical phyics of ensembles of such systems. Simple models are used to give novel phase transitions; particularly for finite N particle systems with many physically interesting examples.
1
the integrable to chaotic transitions in their units. This can be seen in the Toda
and Fermi Pasta Ulam like systems. The description of Hamiltonian systems as
iterations become ’smeared’ all overthe square. A two dimensional crystal , a
snow flake, a liquid crystal, a spin or metallic glass has a kinetics of melting and
Then yn+1 = λayn, xn+1 = xn/α and respectively,
yn+1
=
λbyn
+ 0.5
,xn+1
=
xn −α 1−α
for α, λa, λb all between 0 and 1.
This gives a number of adjustable parameters to model a variety of melting
1. INTRODUCTION The developments in dynamical systems and in Statistical Mechanics have occured for over a century. The ergodic hypothesis of J W Gibbs and Boltzmann’s H theorem were re- investigated as integrable and chaotic dynamical systems were found. Poincare, Birkhoff, Krylov formulated these problems. Kolmogorov, Arnold and Moser theorem gave a detailed description of phase space as ’islands of integrable and sea of chaotic regions’. From chaotic billiards to integrable Toda lattices the range of the systems includes mixed type of systems. Work of Ruelle, Benettin, Galgani, Casati, Galavotti and others clarified some properties of classical and quantum systems. Recent work of E.G.D.Cohen, L Casetti and others has given a rigourous basis for Riemannian space based description of dynamics of Hamiltonian systems and K entropy. However the Statistical mechanics of dynamical systems remains to be formulated. Ensembles with model Hamiltonians showing chaos transitions and topological transitions have become significant as simulation and empirical work has grown. A partition function inclusive of the Kolmogorov entropy and the Euler characteristic has been defined. Nano clusters of particles show properties dependent on the boundaries, number, energy and interaction parameters. Strongly interacting systems in condensed matter , nucleons and quarks are also possible applications. This requires that any dynamical system model such as with maps, or differential equations for the ’units’ in the physical system, will have consequences for the statistical mechanics of their ensemble. It leads to novel phase transitions and new interpretations of thermodynamic phase transitions. This has been shown in this paper for some simple models . The Baker transform is used to model kinetics of melting in two dimensions. The Henon Heiles like models are used to model ergodic channels with correlated charge densities in superconductors. The gas of molecules with Henon Heiles Hamiltonian is shown to have a ’phase’ transition dependent on the chaotic transition in the molecule. Quantum chaos also creates a transition in the ensemble of such systems and the Poisson, GOE, GUE and Husimi distributions are an example of Wigner distributions on phase space. These ideas could be generalised to more complex model maps or Hamiltonians, that are used in physical systems. Hence a statistical physics of finite ( any N ) number of particles is expected to have definite properties dependent on
The folding property of this transform causes mixing and ergodicity and has
a K entropy. Any regular structure of points in the (0, 1) square, will after many
scale
lmax = τ −1/σ ; where τ is the time step and the σ is the K entropy.
A more general model would use different maps on the half intervals.