Incorporation of MATLAB into a distributed behavioral robotics architecture
matlab质量管理

matlab质量管理英文回答:Matlab is a powerful tool for quality management, allowing users to analyze data, perform statistical analysis, and create visualizations. It provides a wide range of functions and tools that can be used to ensure the quality of products or processes.One way Matlab can be used for quality management is by analyzing data to identify trends or patterns. For example, let's say I work for a manufacturing company and I want to monitor the quality of our products. I can use Matlab to analyze data from production processes and identify any deviations from the desired specifications. This can help me identify potential issues early on and take corrective actions to improve the quality.Another way Matlab can be used for quality management is by performing statistical analysis. Matlab has built-infunctions for statistical analysis, such as calculating mean, standard deviation, and performing hypothesis testing. These statistical analyses can help me determine if a process is in control or if there are any significant variations that need to be addressed. For example, I canuse Matlab to calculate the process capability index (Cpk)to assess whether a process is capable of meeting customer requirements.In addition to data analysis and statistical analysis, Matlab can also be used to create visualizations that can help in quality management. Visualizations such as histograms, control charts, and scatter plots can provide a clear and intuitive representation of data, making iteasier to identify trends or outliers. For example, I can use Matlab to create a control chart to monitor the quality of a manufacturing process. The control chart will show meif the process is within the control limits or if there are any points that fall outside the limits, indicating a potential quality issue.中文回答:Matlab是一个强大的质量管理工具,可以帮助用户分析数据、进行统计分析并创建可视化图表。
matlab英文字符归一化流程

matlab英文字符归一化流程Normalizing characters in Matlab is an essential process to standardize text data for various natural language processing tasks. Character normalization involves converting text data into a consistent and easy-to-process format by addressing issues like case sensitivity, character encoding, and special characters. In this document, we will discuss the steps involved in character normalization in Matlab.Step 1: Load the text dataThe first step in character normalization is to load the text data into Matlab using the relevant functions like `readtable` or `fileread`. Make sure to specify the correct encoding if the text data is in a different language.Step 2: Convert to lowercaseConvert all characters in the text data to lowercase using the `lower` function. This step helps in treating characters without considering their case, thereby reducing the complexity of the text data.Step 3: Remove special charactersRemove special characters like punctuation marks, symbols, and emojis from the text data using regular expressions or the `strrep` function. This step simplifies the text data and makes it easier to process.Step 4: Remove accents and diacriticsRemove accents and diacritics from characters using the`char` function with 'NormalizationForm' set to 'D' or 'KD'. This step ensures that characters with accents are normalized to their base characters.Step 5: Normalize character encodingNormalize the character encoding in the text data using the `unicode2native` function to convert characters to their Unicode representation. This step ensures consistency in character encoding across the text data.Step 6: TokenizationTokenize the normalized text data using the`tokenizedDocument` function to split the text into individual words or tokens. This step prepares the text data for further processing like word embedding or sentiment analysis.Step 7: Save the normalized text dataSave the normalized text data in a standardized format like a CSV file or a Matlab data file for future analysis and processing.In conclusion, character normalization in Matlab is a critical step in preparing text data for various natural language processing tasks. By following the above steps, you can ensure that the text data is consistent, clean, and ready for further analysis.。
matlab 特征提取

matlab 特征提取英文回答:Feature extraction is a crucial step in data analysis and pattern recognition tasks, including machine learning and computer vision. It involves transforming raw data into a set of representative features that capture the essential characteristics of the data. These features can then be used to train models or make predictions.There are various techniques available for feature extraction in Matlab. One common approach is to use statistical measures such as mean, standard deviation, and skewness to describe the distribution of data. For example, if we have a dataset of images, we can extract features such as the average pixel intensity, the variance of pixel values, and the histogram of pixel intensities.Another popular technique is to use transform-based methods, such as Fourier Transform or Wavelet Transform, toextract frequency or time-domain features. For instance, in speech recognition, we can extract features such as Mel-Frequency Cepstral Coefficients (MFCCs) using the Fourier Transform.In addition to these traditional techniques, Matlab also provides advanced feature extraction methods based on deep learning. Deep learning models, such as Convolutional Neural Networks (CNNs), can automatically learn features from raw data without the need for manual feature engineering. For example, in image classification tasks, a CNN can learn to extract features such as edges, textures, and shapes from images.中文回答:特征提取是数据分析和模式识别任务中的关键步骤,包括机器学习和计算机视觉。
matlab常见错误以及解决办法

matlab常见错误以及解决办法1.Subscript indices must either be real positive integers or logicals 中文解释:下标索引必须是正整数类型或者逻辑类型出错原因:在访问矩阵(包括向量、二维矩阵、多维数组,下同)的过程中,下标索引要么从0 开始,要么出现了负数。
注:matlab 的语法规定矩阵的索引从1 开始,这与C 等编程语言的习惯不一样。
解决办法:自己调试一下程序,把下标为0 或者负数的地方修正。
2.2.Undefined function or variable "U" 中文解释:函数或变量U 没有定义 .出错原因及解决办法:可能变量名输入错误,仔细检查 33..Matrix dimensions must agree 中文解释:矩阵的维数必须一致出错原因:这是由于运算符(= + - / * 等)两边的运算对象维数不匹配造成的,典型的出错原因是错用了矩阵运算符。
matlab 通过“.”来区分矩阵运算和元素运算。
解决办法:自己调试一下程序,保证运算符两边的运算对象维数一致。
4.4.Function definitions are not permitted at the prompt or in scripts 中文解释:不能在命令窗口或者脚本文件中定义函数出错原因:一旦在命令窗口写function c = myPlus(a,b),此错误就会出现,因为函数只能定义在m 文件中。
关于脚本文件和m 文件的区别请查阅matlab 基础书。
简言之: 1) 如果你写成function 的形式,那么必须写在m 文件中,且以function 开头(即function 语句前不能包含其他语句,所有语句必须放在function 中,当然,function 的定义可以有多个,各function 之间是并列关系,不能嵌套);2) 如果你写成脚本的形式,则既可以写在命令窗口中,也可以写在m 文件中,但两者均不能包含function 语句(即不能进行函数的定义)解决办法:新建一个m 文件,然后再进行函数的定义5. 5.One or more output arguments not assigned during call to '...' 中文解释:在调用...函数过程中,一个或多个输出变量没有被赋值出错原因:函数如果带有输出变量,则每个输出在返回的时候都必须被赋值。
matlab英文命令翻译(打印版)
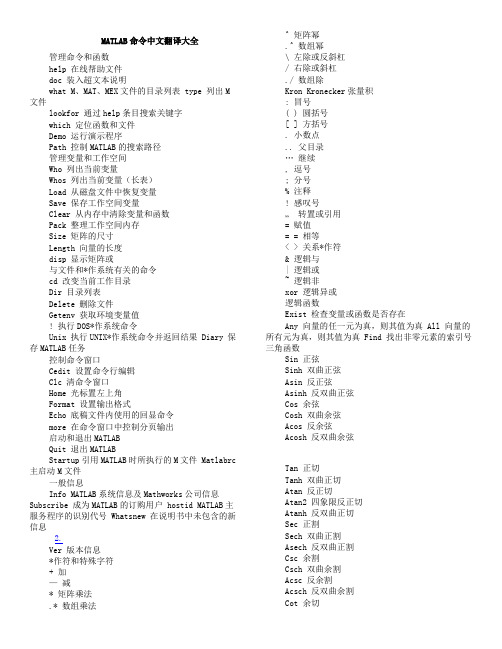
MATLAB命令中文翻译大全管理命令和函数help 在线帮助文件doc 装入超文本说明what M、MAT、MEX文件的目录列表 type 列出M文件lookfor 通过help条目搜索关键字which 定位函数和文件Demo 运行演示程序Path 控制MATLAB的搜索路径管理变量和工作空间Who 列出当前变量Whos 列出当前变量(长表)Load 从磁盘文件中恢复变量Save 保存工作空间变量Clear 从内存中清除变量和函数Pack 整理工作空间内存Size 矩阵的尺寸Length 向量的长度disp 显示矩阵或与文件和*作系统有关的命令cd 改变当前工作目录Dir 目录列表Delete 删除文件Getenv 获取环境变量值! 执行DOS*作系统命令Unix 执行UNIX*作系统命令并返回结果 Diary 保存MATLAB任务控制命令窗口Cedit 设置命令行编辑Clc 清命令窗口Home 光标置左上角Format 设置输出格式Echo 底稿文件内使用的回显命令more 在命令窗口中控制分页输出启动和退出MATLABQuit 退出MATLABStartup 引用MATLAB时所执行的M文件 Matlabrc 主启动M文件一般信息Info MATLAB系统信息及Mathworks公司信息Subscribe 成为MATLAB的订购用户 hostid MATLAB主服务程序的识别代号 Whatsnew 在说明书中未包含的新信息2.Ver 版本信息*作符和特殊字符+ 加—减* 矩阵乘法.* 数组乘法^ 矩阵幂.^ 数组幂\ 左除或反斜杠/ 右除或斜杠./ 数组除Kron Kronecker张量积: 冒号( ) 圆括号[ ] 方括号. 小数点.. 父目录… 继续, 逗号; 分号% 注释! 感叹号… 转置或引用= 赋值= = 相等< > 关系*作符& 逻辑与| 逻辑或~ 逻辑非xor 逻辑异或逻辑函数Exist 检查变量或函数是否存在Any 向量的任一元为真,则其值为真 All 向量的所有元为真,则其值为真 Find 找出非零元素的索引号三角函数Sin 正弦Sinh 双曲正弦Asin 反正弦Asinh 反双曲正弦Cos 余弦Cosh 双曲余弦Acos 反余弦Acosh 反双曲余弦Tan 正切Tanh 双曲正切Atan 反正切Atan2 四象限反正切Atanh 反双曲正切Sec 正割Sech 双曲正割Asech 反双曲正割Csc 余割Csch 双曲余割Acsc 反余割Acsch 反双曲余割Cot 余切Coth 双曲余切Acot 反余切Acoth 反双曲余切指数函数Exp 指数Log 自然对数Log10 常用对数Sqrt 平方根{{分页}}复数函数Abs 绝对值Argle 相角Conj 复共轭Image 复数虚部Real 复数实部数值函数Fix 朝零方向取整Floor 朝负无穷大方向取整 Ceil 朝正无穷大方向取整 Round 朝最近的整数取整 Rem 除后取余Sign 符号函数基本矩阵Zeros 零矩阵Ones 全“1”矩阵Eye 单位矩阵Rand 均匀分布的随机数矩阵 Randn 正态分布的随机数矩阵 Logspace 对数间隔的向量Meshgrid 三维图形的X和Y数组 : 规则间隔的向量特殊变量和常数MATLAB命令大全Ans 当前的答案Eps 相对浮点精度Realmax 最大浮点数Realmin 最小浮点数Pi 圆周率I,j 虚数单位Inf 无穷大Nan 非数值Flops 浮点运算次数Nargin 函数输入变量数Nargout 函数输出变量数Computer 计算机类型Isieee 当计算机采用IEEE算术标准时,其值为真Why 简明的答案Version MATLAB版本号时间和日期Clock 挂钟Date 日历Etime 计时函数Tic 秒表开始计时Toc 计时函数Cputime CPU时间(以秒为单位)矩阵*作Diag 建立和提取对角阵Fliplr 矩阵作左右翻转Flipud 矩阵作上下翻转Reshape 改变矩阵大小Rot90 矩阵旋转90度Tril 提取矩阵的下三角部分Triu 提取矩阵的上三角部分: 矩阵的索引号,重新排列矩阵Compan 友矩阵Hadamard Hadamard矩阵Hankel Hankel矩阵Hilb Hilbert矩阵Invhilb 逆Hilbert矩阵Kron Kronecker张量积Magic 魔方矩阵Toeplitz Toeplitz矩阵Vander Vandermonde矩阵矩阵分析Cond 计算矩阵条件数Norm 计算矩阵或向量范数Rcond Linpack 逆条件值估计MATLAB命令大全Rank 计算矩阵秩Det 计算矩阵行列式值Trace 计算矩阵的迹Null 零矩阵Orth 正交化线性方程\和/ 线性方程求解Chol Cholesky分解Lu 高斯消元法求系数阵Inv 矩阵求逆Qr 正交三角矩阵分解(QR分解)Pinv 矩阵伪逆特征值和奇异值Eig 求特征值和特征向量Poly 求特征多项式Hess Hessberg形式Qz 广义特征值Cdf2rdf 变复对角矩阵为实分块对角形式 Schur Schur分解Balance 矩阵均衡处理以提高特征值精度 Svde 奇异值分解矩阵函数Expm 矩阵指数Expm1 实现expm的M文件Expm2 通过泰勒级数求矩阵指数Expm3 通过特征值和特征向量求矩阵指数 Logm 矩阵对数Sqrtm 矩阵开平方根Funm 一般矩阵的计算泛函——非线性数值方法Ode23 低阶法求解常微分方程Ode23p 低阶法求解常微分方程并绘出结果图形Ode45 高阶法求解常微分方程Quad 低阶法计算数值积分Quad8 高阶法计算数值积分Fmin 单变量函数的极小变化Fmins 多变量函数的极小化Fzero 找出单变量函数的零点Fplot 函数绘图多项式函数Roots 求多项式根Poly 构造具有指定根的多项式Polyvalm 带矩阵变量的多项式计算Residue 部分分式展开(留数计算)MATLAB命令大全Polyfit 数据的多项式拟合Polyder 微分多项式Conv 多项式乘法Deconv 多项式除法建立和控制图形窗口Figure 建立图形Gcf 获取当前图形的句柄Clf 清除当前图形Close 关闭图形建立和控制坐标系Subplot 在标定位置上建立坐标系Axes 在任意位置上建立坐标系Gca 获取当前坐标系的句柄Cla 清除当前坐标系Axis 控制坐标系的刻度和形式 Caxis 控制伪彩色坐标刻度Hold 保持当前图形句柄图形对象Figure 建立图形窗口Axes 建立坐标系Line 建立曲线Text 建立文本串Patch 建立图形填充块Surface 建立曲面Image 建立图像Uicontrol 建立用户界面控制Uimen 建立用户界面菜单{{分页}} 句柄图形*作Set 设置对象Get 获取对象特征Reset 重置对象特征Delete 删除对象Newplot 预测nextplot性质的M文件 Gco 获取当前对象的句柄Drawnow 填充未完成绘图事件 Findobj 寻找指定特征值的对象打印和存储Print 打印图形或保存图形Printopt 配置本地打印机缺省值 Orient 设置纸张取向Capture 屏幕抓取当前图形基本X—Y图形Plot 线性图形Loglog 对数坐标图形MATLAB命令大全Semilogx 半对数坐标图形(X轴为对数坐标)Semilogy 半对数坐标图形(Y轴为对数坐标)Fill 绘制二维多边形填充图特殊X—Y图形Polar 极坐标图Bar 条形图Stem 离散序列图或杆图Stairs 阶梯图Errorbar 误差条图Hist 直方图Rose 角度直方图Compass 区域图Feather 箭头图Fplot 绘图函数Comet 星点图图形注释Title 图形标题Xlabel X轴标记Ylabel Y轴标记Text 文本注释Gtext 用鼠标放置文本Grid 网格线MATLAB编程语言Function 增加新的函数Eval 执行由MATLAB表达式构成的字串 Feval 执行由字串指定的函数Global 定义全局变量程序控制流If 条件执行语句Else 与if命令配合使用Elseif 与if命令配合使用End For,while和if语句的结束For 重复执行指定次数(循环)While 重复执行不定次数(循环)Break 终止循环的执行Return 返回引用的函数Error 显示信息并终止函数的执行交互输入Input 提示用户输入Keyboard 像底稿文件一样使用键盘输入 Menu 产生由用户输入选择的菜单Pause 等待用户响应Uimenu 建立用户界面菜单Uicontrol 建立用户界面控制MATLAB命令大全一般字符串函数Strings MATLAB中有关字符串函数的说明Abs 变字符串为数值Setstr 变数值为字符串Isstr 当变量为字符串时其值为真MATLAB命令表A aabs 绝对值、模、字符的ASCII码值acos 反余弦acosh 反双曲余弦acot 反余切acoth 反双曲余切acsc 反余割acsch 反双曲余割align 启动图形对象几何位置排列工具all 所有元素非零为真angle 相角ans 表达式计算结果的缺省变量名any 所有元素非全零为真area 面域图argnames 函数M文件宗量名asec 反正割asech 反双曲正割asin 反正弦asinh 反双曲正弦assignin 向变量赋值atan 反正切atan2 四象限反正切atanh 反双曲正切autumn 红黄调秋色图阵axes 创建轴对象的低层指令axis 控制轴刻度和风格的高层指令B bbar 二维直方图bar3 三维直方图bar3h 三维水平直方图barh 二维水平直方图base2dec X进制转换为十进制bin2dec 二进制转换为十进制blanks 创建空格串bone 蓝色调黑白色图阵box 框状坐标轴break while 或for 环中断指令brighten 亮度控制C ccapture (3版以前)捕获当前图形 cart2pol 直角坐标变为极或柱坐标cart2sph 直角坐标变为球坐标cat 串接成高维数组caxis 色标尺刻度cd 指定当前目录cdedit 启动用户菜单、控件回调函数设计工具cdf2rdf 复数特征值对角阵转为实数块对角阵 ceil 向正无穷取整cell 创建元胞数组cell2struct 元胞数组转换为构架数组 celldisp 显示元胞数组内容cellplot 元胞数组内部结构图示char 把数值、符号、内联类转换为字符对象chi2cdf 分布累计概率函数chi2inv 分布逆累计概率函数chi2pdf 分布概率密度函数chi2rnd 分布随机数发生器chol Cholesky分解clabel 等位线标识cla 清除当前轴class 获知对象类别或创建对象clc 清除指令窗clear 清除内存变量和函数clf 清除图对象clock 时钟colorcube 三浓淡多彩交叉色图矩阵 colordef 设置色彩缺省值colormap 色图colspace 列空间的基close 关闭指定窗口colperm 列排序置换向量comet 彗星状轨迹图comet3 三维彗星轨迹图compass 射线图compose 求复合函数cond (逆)条件数condeig 计算特征值、特征向量同时给出条件数condest 范 -1条件数估计conj 复数共轭contour 等位线contourf 填色等位线contour3 三维等位线contourslice 四维切片等位线图conv 多项式乘、卷积cool 青紫调冷色图copper 古铜调色图cos 余弦cosh 双曲余弦cot 余切coth 双曲余切cplxpair 复数共轭成对排列csc 余割csch 双曲余割cumsum 元素累计和cumtrapz 累计梯形积分cylinder 创建圆柱D ddblquad 二重数值积分deal 分配宗量deblank 删去串尾部的空格符dec2base 十进制转换为X进制dec2bin 十进制转换为二进制dec2hex 十进制转换为十六进制deconv 多项式除、解卷delaunay Delaunay 三角剖分del2 离散Laplacian差分demo Matlab演示det 行列式diag 矩阵对角元素提取、创建对角阵 diary Matlab指令窗文本内容记录diff 数值差分、符号微分digits 符号计算中设置符号数值的精度 dir 目录列表disp 显示数组display 显示对象内容的重载函数dlinmod 离散系统的线性化模型dmperm 矩阵Dulmage-Mendelsohn 分解 dos 执行DOS 指令并返回结果double 把其他类型对象转换为双精度数值drawnow 更新事件队列强迫Matlab刷新屏幕 dsolve 符号计算解微分方程E eecho M文件被执行指令的显示edit 启动M文件编辑器eig 求特征值和特征向量eigs 求指定的几个特征值end 控制流FOR等结构体的结尾元素下标 eps 浮点相对精度error 显示出错信息并中断执行errortrap 错误发生后程序是否继续执行的控制erf 误差函数erfc 误差补函数erfcx 刻度误差补函数erfinv 逆误差函数errorbar 带误差限的曲线图etreeplot 画消去树eval 串演算指令evalin 跨空间串演算指令exist 检查变量或函数是否已定义exit 退出Matlab环境exp 指数函数expand 符号计算中的展开操作expint 指数积分函数expm 常用矩阵指数函数expm1 Pade法求矩阵指数expm2 Taylor法求矩阵指数expm3 特征值分解法求矩阵指数eye 单位阵ezcontour 画等位线的简捷指令ezcontourf 画填色等位线的简捷指令 ezgraph3 画表面图的通用简捷指令 ezmesh 画网线图的简捷指令ezmeshc 画带等位线的网线图的简捷指令 ezplot 画二维曲线的简捷指令ezplot3 画三维曲线的简捷指令ezpolar 画极坐标图的简捷指令ezsurf 画表面图的简捷指令ezsurfc 画带等位线的表面图的简捷指令F ffactor 符号计算的因式分解feather 羽毛图feedback 反馈连接feval 执行由串指定的函数fft 离散Fourier变换fft2 二维离散Fourier变换fftn 高维离散Fourier变换fftshift 直流分量对中的谱fieldnames 构架域名figure 创建图形窗fill3 三维多边形填色图find 寻找非零元素下标findobj 寻找具有指定属性的对象图柄 findstr 寻找短串的起始字符下标findsym 机器确定内存中的符号变量 finverse 符号计算中求反函数fix 向零取整flag 红白蓝黑交错色图阵fliplr 矩阵的左右翻转flipud 矩阵的上下翻转flipdim 矩阵沿指定维翻转floor 向负无穷取整flops 浮点运算次数flow Matlab提供的演示数据fmin 求单变量非线性函数极小值点(旧版)fminbnd 求单变量非线性函数极小值点fmins 单纯形法求多变量函数极小值点(旧版)fminunc 拟牛顿法求多变量函数极小值点 fminsearch 单纯形法求多变量函数极小值点 fnder 对样条函数求导fnint 利用样条函数求积分fnval 计算样条函数区间内任意一点的值 fnplt 绘制样条函数图形fopen 打开外部文件for 构成for环用format 设置输出格式fourier Fourier 变换fplot 返函绘图指令fprintf 设置显示格式fread 从文件读二进制数据fsolve 求多元函数的零点full 把稀疏矩阵转换为非稀疏阵funm 计算一般矩阵函数funtool 函数计算器图形用户界面 fzero 求单变量非线性函数的零点G ggamma 函数gammainc 不完全函数gammaln 函数的对数gca 获得当前轴句柄gcbo 获得正执行"回调"的对象句柄 gcf 获得当前图对象句柄gco 获得当前对象句柄geomean 几何平均值get 获知对象属性getfield 获知构架数组的域 getframe 获取影片的帧画面 ginput 从图形窗获取数据global 定义全局变量gplot 依图论法则画图gradient 近似梯度gray 黑白灰度grid 画分格线griddata 规则化数据和曲面拟合 gtext 由鼠标放置注释文字guide 启动图形用户界面交互设计工具H hharmmean 调和平均值help 在线帮助helpwin 交互式在线帮助helpdesk 打开超文本形式用户指南 hex2dec 十六进制转换为十进制 hex2num 十六进制转换为浮点数hidden 透视和消隐开关hilb Hilbert矩阵hist 频数计算或频数直方图 histc 端点定位频数直方图histfit 带正态拟合的频数直方图 hold 当前图上重画的切换开关 horner 分解成嵌套形式hot 黑红黄白色图hsv 饱和色图I iif-else-elseif 条件分支结构 ifft 离散Fourier 反变换ifft2 二维离散Fourier反变换 ifftn 高维离散Fourier反变换ifftshift 直流分量对中的谱的反操作 ifourier Fourier反变换i, j 缺省的"虚单元"变量ilaplace Laplace反变换imag 复数虚部image 显示图象imagesc 显示亮度图象imfinfo 获取图形文件信息 imread 从文件读取图象imwrite 把imwrite 把图象写成文件ind2sub 单下标转变为多下标 inf 无穷大info MathWorks公司网点地址 inline 构造内联函数对象 inmem 列出内存中的函数名 input 提示用户输入inputname 输入宗量名int 符号积分int2str 把整数数组转换为串数组 interp1 一维插值interp2 二维插值interp3 三维插值interpn N维插值interpft 利用FFT插值intro Matlab自带的入门引导 inv 求矩阵逆invhilb Hilbert矩阵的准确逆 ipermute 广义反转置isa 检测是否给定类的对象 ischar 若是字符串则为真 isequal 若两数组相同则为真 isempty 若是空阵则为真isfinite 若全部元素都有限则为真 isfield 若是构架域则为真 isglobal 若是全局变量则为真ishandle 若是图形句柄则为真ishold 若当前图形处于保留状态则为真isieee 若计算机执行IEEE规则则为真 isinf 若是无穷数据则为真isletter 若是英文字母则为真islogical 若是逻辑数组则为真 ismember 检查是否属于指定集 isnan 若是非数则为真isnumeric 若是数值数组则为真 isobject 若是对象则为真isprime 若是质数则为真isreal 若是实数则为真isspace 若是空格则为真issparse 若是稀疏矩阵则为真 isstruct 若是构架则为真isstudent 若是Matlab学生版则为真 iztrans 符号计算Z反变换J j , K kjacobian 符号计算中求Jacobian 矩阵 jet 蓝头红尾饱和色jordan 符号计算中获得 Jordan标准型 keyboard 键盘获得控制权kron Kronecker乘法规则产生的数组L llaplace Laplace变换lasterr 显示最新出错信息lastwarn 显示最新警告信息leastsq 解非线性最小二乘问题(旧版) legend 图形图例lighting 照明模式line 创建线对象lines 采用plot 画线色linmod 获连续系统的线性化模型linmod2 获连续系统的线性化精良模型 linspace 线性等分向量ln 矩阵自然对数load 从MAT文件读取变量log 自然对数log10 常用对数log2 底为2的对数loglog 双对数刻度图形logm 矩阵对数logspace 对数分度向量lookfor 按关键字搜索M文件lower 转换为小写字母lsqnonlin 解非线性最小二乘问题lu LU分解M mmad 平均绝对值偏差magic 魔方阵maple &nb, sp; 运作 Maple格式指令mat2str 把数值数组转换成输入形态串数组material 材料反射模式max 找向量中最大元素mbuild 产生EXE文件编译环境的预设置指令 mcc 创建MEX或EXE文件的编译指令 mean 求向量元素的平均值median 求中位数menuedit 启动设计用户菜单的交互式编辑工具mesh 网线图meshz 垂帘网线图meshgrid 产生"格点"矩阵methods 获知对指定类定义的所有方法函数 mex 产生MEX文件编译环境的预设置指令mfunlis 能被mfun计算的MAPLE经典函数列表mhelp 引出 Maple的在线帮助min 找向量中最小元素mkdir 创建目录mkpp 逐段多项式数据的明晰化mod 模运算more 指令窗中内容的分页显示movie 放映影片动画moviein 影片帧画面的内存预置mtaylor 符号计算多变量Taylor级数展开N nndims 求数组维数NaN 非数(预定义)变量nargchk 输入宗量数验证nargin 函数输入宗量数nargout 函数输出宗量数ndgrid 产生高维格点矩阵newplot 准备新的缺省图、轴nextpow2 取最接近的较大2次幂nnz 矩阵的非零元素总数nonzeros 矩阵的非零元素norm 矩阵或向量范数normcdf 正态分布累计概率密度函数normest 估计矩阵2范数norminv 正态分布逆累计概率密度函数 normpdf 正态分布概率密度函数normrnd 正态随机数发生器notebook 启动Matlab和Word的集成环境 null 零空间num2str 把非整数数组转换为串numden 获取最小公分母和相应的分子表达式nzmax 指定存放非零元素所需内存O oode1 非Stiff 微分方程变步长解算器ode15s Stiff 微分方程变步长解算器ode23t 适度Stiff 微分方程解算器ode23tb Stiff 微分方程解算器ode45 非Stiff 微分方程变步长解算器 odefile ODE 文件模板odeget 获知ODE 选项设置参数odephas2 ODE 输出函数的二维相平面图 odephas3 ODE 输出函数的三维相空间图 odeplot ODE 输出函数的时间轨迹图odeprint 在Matlab指令窗显示结果odeset 创建或改写 ODE选项构架参数值 ones 全1数组optimset 创建或改写优化泛函指令的选项参数值orient 设定图形的排放方式orth 值空间正交化P ppack 收集Matlab内存碎块扩大内存pagedlg 调出图形排版对话框patch 创建块对象path 设置Matlab搜索路径的指令pathtool 搜索路径管理器pause 暂停pcode 创建预解译P码文件pcolor 伪彩图peaks Matlab提供的典型三维曲面permute 广义转置pi (预定义变量)圆周率pie 二维饼图pie3 三维饼图pink 粉红色图矩阵pinv 伪逆plot 平面线图plot3 三维线图plotmatrix 矩阵的散点图plotyy 双纵坐标图poissinv 泊松分布逆累计概率分布函数poissrnd 泊松分布随机数发生器pol2cart 极或柱坐标变为直角坐标polar 极坐标图poly 矩阵的特征多项式、根集对应的多项式poly2str 以习惯方式显示多项式poly2sym 双精度多项式系数转变为向量符号多项式 polyder 多项式导数polyfit 数据的多项式拟合polyval 计算多项式的值polyvalm 计算矩阵多项式pow2 2的幂ppval 计算分段多项式pretty 以习惯方式显示符号表达式print 打印图形或SIMULINK模型printsys 以习惯方式显示有理分式prism 光谱色图矩阵procread 向MAPLE输送计算程序profile 函数文件性能评估器propedit 图形对象属性编辑器pwd 显示当前工作目录Q qquad 低阶法计算数值积分quad8 高阶法计算数值积分(QUADL)quit 推出Matlab 环境quiver 二维方向箭头图quiver3 三维方向箭头图R rrand 产生均匀分布随机数randn 产生正态分布随机数randperm 随机置换向量range 样本极差rank 矩阵的秩rats 有理输出rcond 矩阵倒条件数估计real 复数的实部reallog 在实数域内计算自然对数 realpow 在实数域内计算乘方realsqrt 在实数域内计算平方根realmax 最大正浮点数realmin 最小正浮点数rectangle 画"长方框"rem 求余数repmat 铺放模块数组reshape 改变数组维数、大小residue 部分分式展开return 返回ribbon 把二维曲线画成三维彩带图 rmfield 删去构架的域roots 求多项式的根rose 数扇形图rot90 矩阵旋转90度rotate 指定的原点和方向旋转rotate3d 启动三维图形视角的交互设置功能round 向最近整数圆整rref 简化矩阵为梯形形式rsf2csf 实数块对角阵转为复数特征值对角阵rsums Riemann和S ssave 把内存变量保存为文件scatter 散点图scatter3 三维散点图sec 正割sech 双曲正割semilogx X轴对数刻度坐标图semilogy Y轴对数刻度坐标图series 串联连接set 设置图形对象属性setfield 设置构架数组的域setstr 将ASCII码转换为字符的旧版指令 sign 根据符号取值函数signum 符号计算中的符号取值函数 sim 运行SIMULINK模型simget 获取SIMULINK模型设置的仿真参数simple 寻找最短形式的符号解simplify 符号计算中进行简化操作simset 对SIMULINK模型的仿真参数进行设置simulink 启动SIMULINK模块库浏览器 sin 正弦sinh 双曲正弦size 矩阵的大小slice 立体切片图solve 求代数方程的符号解spalloc 为非零元素配置内存sparse 创建稀疏矩阵spconvert 把外部数据转换为稀疏矩阵 spdiags 稀疏对角阵spfun 求非零元素的函数值sph2cart 球坐标变为直角坐标sphere 产生球面spinmap 色图彩色的周期变化spline 样条插值spones 用1置换非零元素sprandsym 稀疏随机对称阵sprank 结构秩spring 紫黄调春色图sprintf 把格式数据写成串spy 画稀疏结构图sqrt 平方根sqrtm 方根矩阵squeeze 删去大小为1的"孤维"sscanf 按指定格式读串stairs 阶梯图std 标准差stem 二维杆图step 阶跃响应指令str2double 串转换为双精度值str2mat 创建多行串数组str2num 串转换为数strcat 接成长串strcmp 串比较strjust 串对齐strmatch 搜索指定串strncmp 串中前若干字符比较strrep 串替换strtok 寻找第一间隔符前的内容struct 创建构架数组struct2cell 把构架转换为元胞数组strvcat 创建多行串数组sub2ind 多下标转换为单下标subexpr 通过子表达式重写符号对象subplot 创建子图subs 符号计算中的符号变量置换subspace 两子空间夹角sum 元素和summer 绿黄调夏色图superiorto 设定优先级surf 三维着色表面图surface 创建面对象surfc 带等位线的表面图surfl 带光照的三维表面图surfnorm 空间表面的法线svd 奇异值分解svds 求指定的若干奇异值switch-case-otherwise 多分支结构sym2poly 符号多项式转变为双精度多项式系数向量symmmd 对称最小度排序symrcm 反向Cuthill-McKee排序syms 创建多个符号对象T ttan 正切tanh 双曲正切taylortool 进行Taylor逼近分析的交互界面text 文字注释tf 创建传递函数对象tic 启动计时器title 图名toc 关闭计时器trapz 梯形法数值积分treelayout 展开树、林treeplot 画树图tril 下三角阵trim 求系统平衡点trimesh 不规则格点网线图trisurf 不规则格点表面图 triu 上三角阵try-catch 控制流中的Try-catch结构 type 显示M文件 U uuicontextmenu 创建现场菜单uicontrol 创建用户控件uimenu 创建用户菜单unmkpp 逐段多项式数据的反明晰化 unwrap 自然态相角upper 转换为大写字母V vvar 方差varargin 变长度输入宗量varargout 变长度输出宗量vectorize 使串表达式或内联函数适于数组运算ver 版本信息的获取view 三维图形的视角控制voronoi Voronoi多边形vpa 任意精度(符号类)数值W wwarning 显示警告信息what 列出当前目录上的文件whatsnew 显示Matlab中 Readme文件的内容which 确定函数、文件的位置while 控制流中的While环结构white 全白色图矩阵whitebg 指定轴的背景色who 列出内存中的变量名whos 列出内存中变量的详细信息winter 蓝绿调冬色图workspace 启动内存浏览器xlabel X轴名xor 或非逻辑yesinput 智能输入指令ylabel Y轴名zeros 全零数组zlabel Z轴名zoom 图形的变焦放大和缩小ztrans 符号计算Z变换SUB 矩阵abbr 缩写active 当前add 增加adius 心align 定位ALP 热膨胀系数amplitude 振幅angular 角度animate 动画annotation 注释文字apply 应用arccosine 反余弦archive 合并arcsine 反正弦area 面积areas 面array 矩阵arrow 箭头assembly 组件back up 恢复behavior 特性block 块Booleans 布尔box框by 通过calc 运算case 情况cent 中心centr 中心circumscr外接圆cntrls 控制comp 构件complexvariable 复数变量component 构件concrete 混凝土cone 圆锥consistent 固定contact 接触contours 等值线contraction 收缩因子CONVERGENCE INDICATOR 收敛精度CONVERGENCEVALUE 收敛值coord 坐标corner 对角cornr 对角count 总数couple 耦合coupled 耦合coupling 耦合create 创建creep 蠕变criteria 准则cross product 向量积cross-sectional 截面CS 坐标系ctr 中点ctrls 控制cupl 耦合curvature 圆弧custom 定制cylinder 圆柱damping 阻尼系数DB DB dependent 相关derivative 导数design opt 优化设计device 设备differentiate 微分dimensions 尺寸discipline 练习displacement 变形display 显示does not 没DOF 自由度Dot product 点积edge 边缘electr 电磁electromag 电磁electromagnetic 电磁elem 单元element 单元end 端energy 能量EPPL COMP 塑性应变分量EPTO COMP 总应变eq 方程eqn 方程equation 方程式erase 删除everything 所有EX 弹性模量EX exclude 排除expansion 膨胀因子exponentiate 幂指数extra 附加extreme 极值EY 弹性模量EYEZ 弹性模量EZface 面fact 因子factor 系数factr 因子failure 破坏fatigue 疲劳fill 填充fillet 倒角fluid 流体force 力function 函数gap 间隙general 通用generator 生成器geom 单元global 全局gradient 梯度graphics 图形GUI 图形用户界面GXY 剪切模量GXY GXZ 剪切模量GXZ GYZ 剪切模量GYZ hard 硬hardening 强化hex 六面体hidden 隐藏higher-order 高阶Hill 希尔h-method 网格细分法hollow 空心hp-method 混合并行法I-J I-J imaginary 虚部immediate 即时independent 非相关individual 单个inscribed 内切圆int 强度integral 积分integrat 积分integrate 积分interactive 交互式intermed 中间interpolate 插入invert 转成is done 完成isotropic 各向同性iteration 叠代jobname 文件名KABS KABS keypoint 关键点kinematic 随动kps 关键点layout 布局legend 图例load 加载lower-order 低阶LS-DYNA 显示动力分析magnification 放大倍数main 主manager 管理器manualsize 手动尺寸map 图mapped 映射master 主mat 材料max 最大maximum 最大member 构件memory 内存menuctrls 菜单控制mesh 网格meshing 网格划分meshtool 网格工具metafile 图元文件moment 力矩multi 多multilinear 多线性multiplied 乘natural log 自然对数nds 节点no 无nodal 节点NONE 无norm 法向Normal 法向normals 没num 编号NUMB NUMBnumber 编号NUXY 泊松比offset 偏移ok 确定only 仅OPT 优化opts 选项order 顺序origin 原点Orthotropic 正交各向异性p 高次单元法pan 移动parall 平行PDS 概率设计系统phase 相位pick 选取piping 管plasticity 塑性plot 绘图plotctrls 绘图控制poisson 泊松POST1 通用后处理器POST26 时间历程后处理器postpro 后处理器postproc 后处理器potential 势preferences 首选项PREP7 前处理器preprocessor 前处理器pretension 先张prism 棱柱Pro Pro profiles 档案资料props 属性PRXY 泊松比PRXY PRXZ 泊松比PRXZ PRYZ 泊松比PRYZPT 点pts Pts 点query 查询quit 退出R 圆rad 半径radiation 辐射矩阵radius 半径range of variable 变量范围rate 率real 实redirect 重定向refine 细化reflection 镜像release 版本remove 删除rename 重命名replot 重新绘图reset 取消response 响应RESUM_DB 恢复_DB resume 恢复rigid 刚性rotational 旋转RUNSTAR 估计分析模块SAT SATSAVE_DB 保存_DB scaling 比例sect 截面section 截面segmented 分段septagon 七边形septagonal 七边形的shell 壳show 显示sine 正弦sint 应力强度size 尺寸skinning 2线smartsize 智能尺寸solid 实体SOLUTION 求解器sort 排序source 源specified 指定specs spectrm 响应谱spline 样条square 平方state 状态stats 状态status 信息step 步store 存贮stress 应力struct 结构structural 结构submodeling 子模型superelement 超单元surf 表面switch 转换sys 系table 表tan 相切tangent 相切temp 温度tet 四面体texturing 材质thermal 热thickness func 函数定义变化的厚度timehist 时间历程title 标题topological 拓扑torus 环行圆柱ttribs 属性uniform 均布unpick 排除user 用户utility 应用value 值vector 矢量view 视图visco 粘volume 体wall 壁warp 翘曲win 窗口wish 希望with 通过workplane 工作平面WP 工作平面write 写zero 零zoom 缩放。
MATLAB与电路分析中英文翻译

MATLAB与电路分析在当今世界,计算机技术,网络技术和通信技术正在迅猛的发展,人民的生活水平逐步提高,电子产品变得越来越重要。
对于电子专业的学生来说电路分析是一门必要的课程和基础的知识技能,它是电路维护和电路应用的基础。
对于那些专业为电子信息工程、职业能力为电路分析的本科学生来说,电路分析是最为重要的专业课程之一。
随着电路分析领域的发展,电路变得越来越复杂,于是计算机仿真技术应运而生。
动态电路的分析是最困难的,它不但耗费时间而且要花费很多的人工,尤其是当电路很复杂的时候。
因此,我们采用了基于Matlab / Simulink仿真工具及电力系统块模型库,来帮助我们分析几个简单的电路,比如交流稳态电路,直流稳态电路和一阶动态电路。
这个电路通过Matlab语言进行编程,并由Simulink仿真工具进行仿真。
虽然通过计算机仿真软件还不能取代传统的方式,但它能帮助人们更加直接和有效的理解电路分析。
通过这些仿真工具,人们可以验证电路的性能,降低计算的复杂程度。
它使我们节省了大量的工作(时间)。
在整个电路仿真分析的过程中,我们知道了Matlab / Simulink软件包是如何工作的,并且展示了 Matlab / Simulink 仿真工具在电路分析中的优越性,它被证明是简单的、方便的、有效率的和灵活的。
MATLAB是一种用于工程计算的高性能的计算机编程语言。
它集数值计算、图形显示和编程于一体,构成了一个方便的、界面友好的用户环境。
在这个环境下,所要求解的问题及其答案都采用我们熟悉的数学符号来表示。
其典型应用包括:1.数值计算2.算法设计3.建模、仿真和造型4.数据分析、探测和显示5.科技和工程图形6. 应用开发(包括图形用户界面的建立)MATLAB 是一个交互式系统,它的基本数据元素是一个不要求预先定义维数的数组。
这样我们就可以花费很少的时间去解决许多工程计算问题,特别是那些带有矩阵和矢量公式的问题,同样的问题如果要用非交互式语言比如C或Fortran来编程的话要花费很多时间。
matlab自带优化工具箱遗传算法中文解释
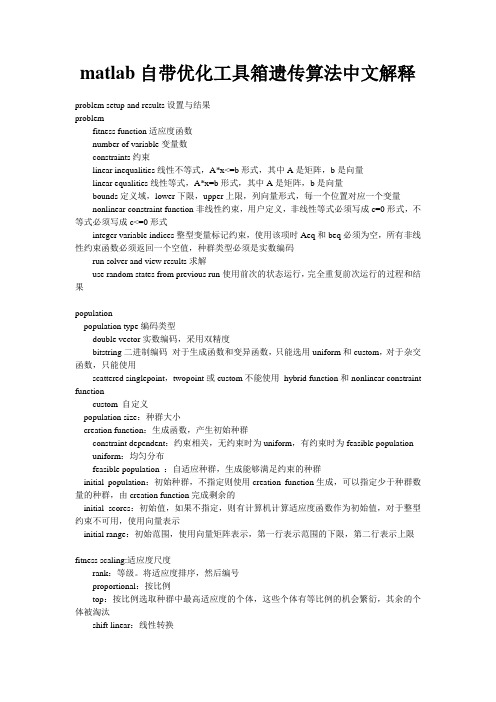
matlab自带优化工具箱遗传算法中文解释problem setup and results设置与结果problemfitness function适应度函数number of variable变量数constraints约束linear inequalities线性不等式,A*x<=b形式,其中A是矩阵,b是向量linear equalities线性等式,A*x=b形式,其中A是矩阵,b是向量bounds定义域,lower下限,upper上限,列向量形式,每一个位置对应一个变量nonlinear constraint function非线性约束,用户定义,非线性等式必须写成c=0形式,不等式必须写成c<=0形式integer variable indices整型变量标记约束,使用该项时Aeq和beq必须为空,所有非线性约束函数必须返回一个空值,种群类型必须是实数编码run solver and view results求解use random states from previous run使用前次的状态运行,完全重复前次运行的过程和结果populationpopulation type编码类型double vector实数编码,采用双精度bitstring二进制编码对于生成函数和变异函数,只能选用uniform和custom,对于杂交函数,只能使用scattered singlepoint,twopoint或custom不能使用hybrid function和nonlinear constraint functioncustom 自定义population size:种群大小creation function:生成函数,产生初始种群constraint dependent:约束相关,无约束时为uniform,有约束时为feasible population uniform:均匀分布feasible population :自适应种群,生成能够满足约束的种群initial population:初始种群,不指定则使用creation function生成,可以指定少于种群数量的种群,由creation function完成剩余的initial scores:初始值,如果不指定,则有计算机计算适应度函数作为初始值,对于整型约束不可用,使用向量表示initial range:初始范围,使用向量矩阵表示,第一行表示范围的下限,第二行表示上限fitness scaling:适应度尺度rank:等级。
Matlab的第三方工具箱大全(强烈推荐)

Matlab ToolboxesADCPtools - acoustic doppler current profiler data processingAFDesign - designing analog and digital filtersAIRES - automatic integration of reusable embedded softwareAir-Sea - air-sea flux estimates in oceanographyAnimation - developing scientific animationsARfit - estimation of parameters and eigenmodes of multivariate autoregressive methods ARMASA - power spectrum estimationAR-Toolkit - computer vision trackingAuditory - auditory modelsb4m - interval arithmeticBayes Net - inference and learning for directed graphical modelsBinaural Modeling - calculating binaural cross-correlograms of soundBode Step - design of control systems withmaximized feedbackBootstrap - for resampling, hypothesis testing and confidence interval estimationBrainStorm- MEG and EEG data visualization and processingBSTEX - equation viewerCALFEM - interactive program for teaching the finite element methodCalibr - for calibrating CCD camerasCamera CalibrationCaptain - non-stationary time series analysis and forecastingCHMMBOX - for coupled hidden Markov modeling using maximum likelihood EMClassification - supervised and unsupervised classification algorithmsCLOSIDCluster - for analysis of Gaussian mixture models for data set clusteringClustering - cluster analysisClusterPack - cluster analysisCOLEA - speech analysisCompEcon - solving problems in economics and financeComplex - for estimating temporal and spatial signal complexitiesComputational StatisticsCoral - seismic waveform analysisDACE - kriging approximations to computer modelsDAIHM - data assimilation in hydrological and hydrodynamic modelsData VisualizationDBT - radar array processingDDE-BIFTOOL- bifurcation analysis of delay differential equationsDenoise - for removing noise from signalsDiffMan- solving differential equations on manifoldsDimensional Analysis -DIPimage - scientific image processingDirect - Laplace transform inversion via the direct integration methodDirectSD - analysis and design of computer controlled systems with process-oriented modelsDMsuite - differentiation matrix suiteDMTTEQ - design and test time domain equalizer design methodsDrawFilt - drawing digital and analog filtersDSFWAV - spline interpolation with Dean wave solutionsDWT - discrete wavelet transformsEasyKrigEconometricsEEGLABEigTool - graphical tool for nonsymmetric eigenproblemsEMSC - separating light scattering and absorbance by extended multiplicative signal correctionEngineering VibrationFastICA- fixed-point algorithm for ICA and projection pursuitFDC - flight dynamics and controlFDtools - fractional delay filter designFlexICA - for independent components analysisFMBPC - fuzzy model-based predictive controlForWaRD - Fourier-wavelet regularized deconvolutionFracLab - fractal analysis for signal processingFSBOX - stepwise forward and backward selection of features using linear regressionGABLE - geometric algebra tutorialGAOT - genetic algorithm optimizationGarch - estimating and diagnosing heteroskedasticity in time series modelsGCE Data - managing, analyzing and displaying data and metadata stored using the GCE data structure specificationGCSV- growing cell structure visualizationGEMANOVA- fitting multilinear ANOVA modelsGenetic AlgorithmGeodetic - geodetic calculationsGHSOM - growing hierarchicalself-organizing mapglmlab - general linear modelsGPIB - wrapper for GPIB library from National InstrumentGTM - generative topographic mapping, a model for density modeling and data visualization GVF - gradient vector flow for finding 3-D object boundariesHFRadarmap - converts HF radar data fromradial current vectors to total vectorsHFRC - importing, processing andmanipulating HF radar dataHilbert- Hilbert transform by the rationaleigenfunction expansion methodHMM - hidden Markov modelsHMMBOX - for hidden Markov modeling usingmaximum likelihood EMHUTear - auditory modelingICALAB - signal and image processing usingICA and higher order statisticsImputation - analysis of incompletedatasetsIPEM - perception based musical analysis2007-8-29 15:04#1littleboy助理工程师精华 0JMatLink - Matlab Java classesKalman - Bayesian Kalman filterKalman Filter - filtering, smoothing and parameter estimation (using EM) for linear dynamical systemsKALMTOOL - state estimation of nonlinear systemsKautz - Kautz filter designKrigingLDestimate - estimation of scaling exponentsLDPC - low density parity check codes积分 49 帖子 76 水位 164技术分 0LISQ - wavelet lifting scheme on quincunx gridsLKER - Laguerre kernel estimation toolLMAM-OLMAM - Levenberg Marquardt with Adaptive Momentum algorithm for training feedforward neural networksLow-Field NMR - for exponential fitting, phase correction of quadrature data and slicingLPSVM- Newton method for LP support vector machine for machine learning problemsLSDPTOOL- robust control system design using the loop shaping design procedureLS-SVMlabLSVM - Lagrangian support vector machine for machine learning problemsLyngby - functional neuroimagingMARBOX - for multivariate autogressive modeling andcross-spectral estimationMatArray - analysis of microarray dataMatrix Computation - constructing test matrices, computing matrix factorizations, visualizing matrices, and direct search optimizationMCAT - Monte Carlo analysisMDP - Markov decision processesMESHPART - graph and mesh partioning methodsMILES- maximum likelihood fitting using ordinary least squares algorithmsMIMO - multidimensional code synthesisMissing - functions for handling missing data valuesM_Map - geographic mapping toolsMODCONS - multi-objective control system designMOEA - multi-objective evolutionary algorithmsMS - estimation of multiscaling exponentsMultiblock - analysis and regression on several data blocks simultaneouslyMultiscale Shape AnalysisMusic Analysis- feature extraction from raw audio signals for content-based music retrievalMWM - multifractal wavelet modelNetCDFNetlab - neural network algorithmsNiDAQ - data acquisition using the NiDAQ libraryNEDM - nonlinear economic dynamic modelsNMM - numerical methods in Matlab textNNCTRL - design and simulation of control systems based on neural networksNNSYSID- neural net based identification of nonlinear dynamic systemsNSVM - newton support vector machine for solving machine learning problemsNURBS - non-uniform rational B-splinesN-way - analysis of multiway data with multilinear models OpenFEM - finite element developmentPCNN - pulse coupled neural networksPeruna - signal processing and analysisPhiVis - probabilistic hierarchical interactive visualization, . functions for visual analysis of multivariate continuous dataPlanar Manipulator - simulation of n-DOF planar manipulators PRTools - pattern recognitionpsignifit - testing hyptheses about psychometric functions PSVM - proximal support vector machine for solving machine learning problemsPsychophysics - vision researchPyrTools - multi-scale image processingRBF - radial basis function neural networksRBN- simulation of synchronous and asynchronous random boolean networksReBEL - sigma-point Kalman filtersRegression - basic multivariate data analysis and regression Regularization ToolsRegularization Tools XPRestore ToolsRobot - robotics functions, . kinematics, dynamics and trajectory generationRobust Calibration - robust calibration in statsRRMT - rainfall-runoff modellingSAM - structure and motionSchwarz-Christoffel - computation of conformal maps to polygonally bounded regionsSDH - smoothed data histogramSeaGrid - orthogonal grid makerSEA-MAT - oceanographic analysisSLS - sparse least squaresSolvOpt - solver for local optimization problemsSOM - self-organizing mapSOSTOOLS- solving sums of squares (SOS) optimization problems Spatial and Geometric AnalysisSpatial RegressionSpatial StatisticsSpectral MethodsSPM - statistical parametric mappingSSVM - smooth support vector machine for solving machine learning problemsSTATBAG- for linear regression, feature selection, generation of data, and significance testingStatBox - statistical routinesStatistical Pattern Recognition- pattern recognition methods Stixbox - statisticsSVM - implements support vector machinesSVM ClassifierSymbolic Robot DynamicsTEMPLAR - wavelet-based template learning and pattern classificationTextClust - model-based document clusteringTextureSynth - analyzing and synthesizing visual textures TfMin- continous 3-D minimum time orbit transfer around Earth Time-Frequency - analyzing non-stationary signals usingtime-frequency distributionsTree-Ring - tasks in tree-ring analysisTSA- uni- and multivariate, stationary and non-stationary time series analysisTSTOOL - nonlinear time series analysisT_Tide - harmonic analysis of tidesUTVtools - computing and modifying rank-revealing URV and UTV decompositionsUvi_Wave - wavelet analysisvarimax - orthogonal rotation of EOFsVBHMM - variation Bayesian hidden Markov modelsVBMFA - variational Bayesian mixtures of factor analyzers VMT - VRML Molecule Toolbox, for animating results from molecular dynamics experimentsVOICEBOXVRMLplot - generates interactive VRML graphs and animations VSVtools - computing and modifying symmetric rank-revealing decompositionsWAFO - wave analysis for fatique and oceanographyWarpTB - frequency-warped signal processingWAVEKIT - wavelet analysisWaveLab - wavelet analysisWeeks - Laplace transform inversion via the Weeks methodWetCDF - NetCDF interfaceWHMT - wavelet-domain hidden Markov tree modelsWInHD - Wavelet-based inverse halftoning via deconvolution。
- 1、下载文档前请自行甄别文档内容的完整性,平台不提供额外的编辑、内容补充、找答案等附加服务。
- 2、"仅部分预览"的文档,不可在线预览部分如存在完整性等问题,可反馈申请退款(可完整预览的文档不适用该条件!)。
- 3、如文档侵犯您的权益,请联系客服反馈,我们会尽快为您处理(人工客服工作时间:9:00-18:30)。
Incorporation of MATLAB into a Distributed BehavioralRobotics ArchitectureA. L. Nelson, L. Doitsidis, M. T. Long, K. P. Valavanis, and R. R. MurphyCenter for Robot Assisted Search and Rescue (CRASAR)Dept Computer Science and EngineeringUniversity of South FloridaTampa, Florida 33620Email: aanelson@Abstract—This paper presents a method that integrates MATLAB into a distributed behavioral robotics architecture. The architecture is written in Java and uses the Jini platform for distributed object registration, lookup and remote method invocation. The method described here can be used to integrate MATLAB into any Java-based behavioral architecture. The form of the integration allows a running MATLAB workspace to be accessed as a distributed object within the larger Java/Jini-based architecture. This is beneficial because MATLAB scripts and functions may be called in interpreted form and can make full use of MATLAB tool boxes and have access to the MATLAB workspace environment. This is not possible when MATLAB scripts are compiled into stand-alone C++, Java or p-code. The use of the architecture is demonstrated on an iRobot ATRV-JR robot and remote computer workstation. Experiments have been conducted to quantify GPS and odometry errors in outdoor environments using automated methods supported by the distributed architecture.Keywords-mobile Distributed architecture; autonomous robot control; control architecture; MATLAB; Jini; GPS-based navigation;I.I NTRODUCTIONThis paper is motivated by the challenge to provide a platform to support advanced robot control research both at the AI level [1] and at the control-theoretic level [17]. The architecture, referred to in this paper as Distributed SFX, supports the implementation of large scale complex behavior-based robot control but still provides tools for low level experimental research within a single structured paradigm [5][10].The overall distributed behavioral architecture is implemented in Java and uses Jini to manage distributed objects and services between robots and computers. Using the JMatLink library [2], MATLAB is supported within the larger Java/Jini based system to allow for mathematical control-theoretic research and experimentation and for rapid prototyping of both behavioral and control modules and services. Wrapping the MATLAB workspace environment with JMatLink, in conjunction with the Jini distributed object platform allows modules and services implemented as native interpreted MATLAB code to be accessed as remote and distributed objects. Although it is the case that many behavioral architectures and languages support implementation of modules in different languages, the work described here allows MATLAB, a standard tool for developing control-theoretic concepts offline, to be directly incorporated into behavioral architectures. This provides advantages such as speed up of development, added flexibility of implementation.The architecture is currently implemented on iRobot ATRV-JR robots and computer workstations. The robots have been fitted with a versatile array of sensors including GPS, planar laser range sensors, heat imaging video (FLIR) standard video, and inertial gyroscopes. To demonstrate the flexibility of the architecture, and to lay the groundwork for outdoor robot navigation, a series of tests have been performed. These tests included automated GPS and odometry error quantification experiments (reported on in the results section of this paper). During the tests, the distributed architecture ran both on a robot in the field and on a computer workstation in the lab. This demonstrated distributed object sharing and allowed for flexible remote invocation of robot tests from the workstation.The paper is organized as follows. Section II reviews the state of the art in current robot control architectures. Section III presents the architecture used in this research and describes the integration of MATLAB into the architecture. Section IV discusses the robots and the hardware used in this work, and section V presents results.II.R OBOT C ONTROL A RCHITECTURES Robot control architectures are systems that provide structured methodologies and constraints for designing and implementing robot control systems. There are many mobile robot control architectures described in the literature. For a recent review of autonomous robot control architectures see [3]. This work primarily focuses on architectures as described in [3], rather than simple programming environments. We concentrate on process architectures that provide tools and paradigms for the design of robot control systems. Software or implementation architectures, on the other hand, may be used to provide the underlying support for systems, such as communications and access to the robot sensors and actuators, but are not the direct focus of this work.Many of the large-scale architectures are organized around structured paradigms. For example, in [4] an architecture designed to facilitate the fault tolerant control of teams of heterogeneous robots using (among other things) a mathematical model of motivation is discussed.In [5] a distributed fault handling and robot task management architecture using a distributed Java framework is presented. These architectures are designedto support robot control within methodological and conceptual constraints.Behavioral robotics architectures support the implementation of control within the behavior-based robotics paradigm [6]. Behavior-based robotics incorporates elements from artificial intelligence, engineering, and cognitive sciences. Conceptually, behavior-based robot control provides methods for the parallel integration of simple behaviors to generate more complex emergent robot behaviors [1]. The Distributed SFX architecture used in this paper falls into this category as well as being a hybrid deliberative-reactive system.There are several examples of general-purpose robot control architectures designed to allow programmers as much design flexibility as easily as possible. These are not meant to support any particular control paradigm and are designed to provide the greatest possible flexibility in robot control implementation and experimentation. For example,in [7][8] a multi-robot control architecture using MATLABas the primary computing environment is used for various intelligent robotics control experimentation. That system is designed to allow access to all sensors and actuators (on both local and remote robots) in the form of functions that appear a standard MATLAB function calls. Unicast and broadcast communications are also supported between robots and can be used to transmit anything from a single parsable set of characters to files containing interpreted code that can be added to a currently operating robot control structure. In [9], a system designed to support distributed control in robots is presented. That system is explicitly indented to provide as few restrictions on robot control programming as possible while allowing transparent platform and language independent access to services such as actuators and sensors.A potential drawback of very flexible robot control research architectures is that they often produce single-purpose control programs that are difficult to maintain or expand. For this reason, many structured paradigm base architectures restrict the fashion in which control can be structured. On the other hand, a potential drawback to relying on structured paradigm base architectures for primary research is that they may be too restrictive to easily support some aspects of the research design and experimentation process.There are very few robotics research architectures that explicitly enable both paradigm-based and flexible experimental research design methodologies. One reason that such systems are not well represented is that the methodologies have a number of apparent incompatibilities. For instance autonomous robot control research based in control theoretic and engineering backgrounds tends to take a very unstructured approach to software implementation of research. Groups from Computer Science on the other hand, often focus their research on the software architecture itself, and place much more emphasis on the development of paradigms, and enforcement of good programming practices. This often excludes or limits the agile programming and unstructured rapid prototyping design methods used in engineering research.The goal is to support general robot control research and experimentation while providing a pathway to incorporate results from such work into a larger structured distributed robotics control architecture. The architecture discussed in this paper provides for a less structured flexible form of use of the system as a development phase and research tool, while the overall system provides a method for incorporation of the research products of the development phase into the production phase structured architecture.The architecture is being used to control real robots in outdoor environments. As a case study, experimental results quantifying GPS and odometer errors under various test conditions are reported.III.T HE R ESEARCH A RCHITECTUREThe robot control architecture is designed to accommodate autonomous distributed control of robots in outdoor environments. It is designed to support proper implementation (in the software development sense) and at the same time be useful as a rapid experimentation research tool and programming environment (common in engineering research). An overview of the architecture is presented below, along with the details of communication and integration between Java and MATLAB, followed by a discussion of the implementation on the ATRV-JR robots. A. Distributed SFXDistributed SFX is a service-based distributed robot architecture designed to support behavior based control. It is a distributed Java based descendant of the Sensor Fusion Effects architecture (SFX) [10]. The system makes use of modules to implement robot sensing and control related services. The architecture also supplies high-level service managers for the coordination and functional integration of low level modular services. Modules are exported to a distributed run-time system as services with certain attributes and types. Service may then be searched for (using a distributed-object lookup service) based on functional attributes rather than details of actual implementation or physical location. This type of architecture allows a decoupling of client and server. Clients, in an abstract sense, are interested in services with particular attributes that provide or consume certain types of data. For example, processes running on one robot might need to request perceptual data from a remote source such as another robot. In this case, a service able to supply the correct type of data would be searched for using its functional attributes (type of data, location of data source, ext.). If a service is found, an interface (proxy) can be provided to the requesting process in a modular fashion regardless of where the requested services physically resides or how it is implemented at the local level.Fig. 1. Distributed SFX horizontal class hierarchy diagram.MATLAB is shown as a driver implementation module.Heterogeneity of robot platforms is specifically supported by the architecture. This is done by representingservices and components as distributed objects with consistent interfaces. Different classes of robots, such as unmanned aerial vehicles (UAV) and unmanned ground vehicles (UGV) can use a common architecture and request remote sensor and other services based on features of the required service, regardless of the actual sensor or robot providing the information. Distributed SFX is written in Java and uses the Jini distributed service platform. Each module is roughly divided into three components- the proxy, server and driver. The proxy is the representation of the service that is transported around the network. The proxy provides the ability to move code and data, and is not merely a localrepresentation of a remote object. The server is the representation of the service. The server deals with the distributed system and mediates between the implementation of the service (the driver) and the remote clients. The driver is the actual implementation of the service.In a distributed and changing environment the health of the overall network can change dramatically due to communication loss, hardware failure or other causes. The use of a flexible service-oriented architecture allows for a more resilient system.In Fig. 1 a horizontal class hierarchy diagram is shown. The figure shows the hierarchical class relationship between the distributed and native local elements of a generic behavior within the system.B. MATLAB SupportThere are a few examples of the integration of MATLAB and Java in engineering systems (see [11], [12]). However, the combination of Java and MATLAB for use in autonomous robot control is not well represented in theliterature. No major Java-based distributed robotics architectures that make use of MATLAB in its interpreted form have been reported on.Within the larger Java/Jini based Distributed SFX framework, MATLAB is currently supported for control module design and testing and research experimentation. The JMatLink class library [2] has been used to connect Java to MATLAB. JMatLink includes methods and objects that allow Java to be used to initialize a workspace, write data members of any format to the workspace, read from the work space, and execute command line functions. The MATLAB workspace engine is accessed by delivering a formatted string to MATLAB and its behavior is identical to that of a user entering command via the MATLAB workspace command line.A full MATLAB engine and workspace is supported.This means that MATLAB scripts and functions can be runlocally on the robots as interpreted code without the need to be compiled into stand-alone executables. In addition, afull range of GUI and function based toolboxes areavailable for direct use on the robots. The system allows ofany format of testing that is possible within MATLAB,including onboard modeling and numerical processing, but supports the integration of these developments into thelarger framework in a way that is compatible with the distributed service oriented architecture. The actual interaction between Java and MATLAB is summarized as follows: First, a thread for MATLAB is activated by its Java service implementation using the JMatLink class library method engOpen . This is outlined in the abbreviated Java code segment shown in Fig. 2. JMatLink is the constructor for the library and MatlabImpl inherits from the standard service implementation class within the Distributed SFX Java-based implementation.Fig. 2. Abbreviated Java code initiating a new instance of aMATLAB engine and workspace.Fig. 3. Abbreviated Java code to write data (in the form of a struct) to the MATLAB workspace and then call an interpretedMATLAB function using the data struct.Data are written to the MATLAB workspace and a formatted field struct is constructed using the engPutVariable and engEvalString methods from the JMatLink class library.Abbreviated Java code that builds a MATLAB data struct (sensorData) and then calls an interpreted MATLAB function (mFunction) with the struct as an argument is shown in Fig. 3.The code in Fig. 3 would generally be used within a thread runner method of the MatlabRunner class of Fig. 2. Note that mFunction will be some function written in MATLAB script and sensorData and result are both MATLAB variables existing within the workspace. Results are read from the MATLAB workspace back into Java variables using the JMatLink method engPutVariable.Fig. 4 shows the forms of support for MATLAB within the larger architecture. Development phase and production phase support are provided.At the production level, for fully developed services, MATLAB is supported at the driver module implementation level discussed in the previous section. It can be used as the native server implementation of a service. The associated server and proxy handle the remote overhead and interaction with other services. On the left of Fig. 4 within the Distributed SFX block a native service implemented in MATLAB is shown. Note that many languages are supported for implementation of services and most services are currently implemented in Java. The purpose of the figure is to illustrate the two forms in which MATLAB is used in the system.The MATLAB workspace on the right of Fig. 4 shows MATLAB’s relationship to the larger architecture when itis being used for research and module development. Thisis considered the development phase. In this form, MATLAB is used as a research tool in addition to being used for standard service module development and is not strictly constrained by the overall Distributed SFX architectural paradigm.n d P ro d u ctio n P h a se D e ve lo p m e n t P h a seFig. 4. Data flow diagram illustrating the relationship between MATLAB and the larger distributed Java/Jini based architecture. For research and development, a full MATLAB workspace is supported in the development phase (Development Phase). Inthe production phase, fully developed modules are seen as local service implementations within Distributed SFX (ProductionPhase).In summary, the architecture supports research from its primary synthesis, to its mature implementation within a structured paradigm. The architecture supports uniformity, compatibility and integration of high level AI elements and low level mathematical control-theoretic elements. In addition, the architecture supports low level research experimentation without sacrificing the integrity of the larger structured distributed robotics behavior based paradigm.IV.R OBOTS AND H ARDWAREThe Distributed SFX architecture including integrated MATLAB support has been implemented on mobile robots and computer workstations. The architecture’s functionality is demonstrated using a system consisting of one robot operating outdoors and one computer workstation located inside the laboratory.Distributed SFX has been implemented on iRobot ATRV-JR ground robots. These robots have been equipped with a versatile array of sensors including scanning planar lasers, FLIR heat imaging video, standard video, GPS and inertial gyroscopes. In addition, the robots have wheel odometers supplied by the manufacturer and managed by iRobot’s Mobility software.(a)(b)Fig. 5. An ATRV-JR robot (a) in the CRASAR robotics lab and (b) outside on the USF campus near the CRASAR robotics lab.The ATRV-JR ground robots have PCs with 1GHz PIII processors and 2Gb ram. The onboard computers include auxiliary 10 serial port cards, firewire and UBS to accommodate sensors and other equipment. The robots were originally supplied with Linux RedHat 7.x from iRobot but have been upgraded to Linux 9.x. The robots are capable of running locally any software that will run on a standard PC and are accessible via wireless Ethernet (802.11g). Photos of the CRASAR ATRV-JR robots are shown in Fig. 5.The ATRV-JR in Fig. 5 (a) is shown with a SICK scanning planar laser mounted on the front of the vehicle, a pan/tilt unit with FLIR and standard video cameras attached to the front-top equipment rack, and inertial gyroscope and GPS mounted on the center-top equipment rack.The experiments present in this paper rely on GPS and odometry. The GPS system used is a Synergy Systems LLC M12+ with evaluation board and a HAWK GPS antenna connected via serial link to the robot’s main computer. The device uses proprietary filters and error estimation algorithms. Both filtered and unfiltered readings are available to the robots.V.R ESULTSHere we report on testing of robots in an outdoor environment using the architecture described above. Experiments to test and quantify GPS and odometer accuracy were performed in outdoor conditions. GPS, and odometer errors were measured over the course of several benchmark test driving patterns, and under varying conditions.The tests were conducted by setting up and executing automated test sequences. The distributed architecture operated on a system including a robot in the field and a computer workstation in the lab. The experiments made use of MATLAB as supported in development mode as illustrated in Fig. 4 in section III above. The Distributed SFX architecture managed all the sensor and actuator services as distributed objects. MATLAB, was used to implement the automated test services on the robot, and to process and correlate test data within the larger Java/Jini based distributed architecture. The larger Distributed SFX architecture also managed the tests at a high level including maintenance of distributed objects and services between the lab computer workstation and the robot.A.GPS based navigationThe example tests conducted here serve both to demonstrate the functionality of the Distributed SFX architecture and to lay the groundwork for outdoor navigation with mobile robots using GPS. In order to provide a context and motivation for the experiments, a short review of the use of GPS and odometry for positioning and navigation in mobile robots follows.Several references can be found in the literature that discuss testing and performance of GPS receivers in mobile robots. In [13], issues concerning the use of GPS for controlling and navigating an autonomous vehicle are addressed. In that work, several experiments were conducted to measure the accuracy of GPS and the availability of satellite fix for 3D GPS. In [14] the use of a differential GPS (DGPS) is proposed for positioning in a single mobile robot. In that work, limitations and variability in accuracy as functions of number and location of satellites are studied, as well as the affects of choice of the satellite to be used and the offset of the receiver’s location.In unstructured outdoor environments, Fused GPS and wheel odometry data have been proposed for use in absolute positioning of mobile robots. For example, in [15] the authors propose a method for reducing errors in differential GPS measurements by incorporating information from robot position odometry. In that work, the authors present experimental results validating their approach. In [16] another approach for outdoor navigation using GPS data and odometry is presented. Their methodology for vehicle navigating is based on the construction of covariance matrices for automatic localization computations that include the number, and location of, visible satellites as factors. The matrices are built and maintained by sampling GPS data along a route and continuously updating the matrices to accommodate drift in GPS data quality.In order to provide a baseline level of performance for the methods described above, and to improve the use of GPS and odometry for navigation it is beneficial to accurately measure relative and absolute GPS and odometry position error. The experiments reported on in this section provide a quantification of such errors.B.Test PatternsDuring these tests, the robot automatically followed predetermined test patterns. These included forward and backward motion along a straight line, tracing a rectangle, and tracing a circle. The controller service modules for each of these test patterns produced predetermined timed sequences of movements and recorded all sensor and actuator related data over the course of each test.C. Data Collection and Coordinate framesData were recorded at each controller time step during each test-pattern driving session. Data included GPS readings, actuator commands, odometry generated position, time stamp and other sensor readings (such as Laser range values). For the test conditions used here, full control loop cycles required about 0.2 seconds to complete so data were collected at a rate of approximately 5 Hz.In order to relate the data collected during robot driving, several coordinate frames of reference must be kept track of. These include the robot body-attached frame of reference, the robot’s internal world frame of reference, and the real world frame or reference. Although these frames are technically 3 dimensional, we consider only the x and y-axis of each frame since these tests were conducted under very nearly planar conditions (mixed dirt and grass flat terrain).The real world coordinate frame of reference is defined here to have its origin at the initial position of the robot at the start of a driving session, and to be oriented so that the positive x-axis points toward the East and the positive y-axis points toward the North. GPS data are converted to meters and related to this coordinate frame.The robot’s internal world frame is used to record and maintain position and orientation calculated from odometry. The robot internal reference frame deviates from the real world by the cumulative error generated by the odometry system.The robot body-attached frame of reference is defined with its origin at the center of the robot and its x-axis pointed in the forward facing direction of the robot. The robot is initializes at the beginning of each driving session so that its body-attached frame is coincident with its internal world frame. Fig. 6. GPS points and points calculated from odometry for anexample linear test pattern.Fig. 7. GPS points and points calculated from odometry for arectangular test pattern.Fig. 8. GPS points and points calculated from odometry and for an example of the circular test pattern.West --- East (m)South---North(m)Linear Test PatternWest --- East (m)South---North(m)Rectangular Test PatternWest --- East (m)South---North(m)Circular Test PatternD.GPS and Odometry ResultsFor each of the test patterns, the robots were driven 5 times (for a total of 15 runs). Each test was initiated remotely from a computer workstation in the lab using the distributed architecture.Within the MATLAB-based test service, data were collected, stored, and processed. At each iteration of control (time step), the following data were collected: GPS lock status, filtered GPS latitude and longitude coordinates, unfiltered GPS latitude and longitude coordinates, incremental x and y positions based on odometry, linear and rotational velocity based on odometry and all calculated actuator control commands. These data were used to reconstruct the robot’s sensor history and internal record of position history.Figures 6, 7 and 8 show GPS and wheel odometry position data collected on the linear, rectangular, and circular test patterns respectively. Each panel shows data collected over a single example run of each test pattern.The paths taken by the robots during tests (as judged by GPS and odometry position measurement methods) are shown as sequences of points. All data have been converted to meters (m) and oriented as they would be in a standard map. During each run, the origin was set to be the starting point of the robot. In all three cases the positions calculate from odometry and positions measured from GPS deviate from one another over the courses of the tests. In the rectangular and circular tests, odometry positions and GPS positions deviate by approximately 2.0 meters and 6.0 meters on average respectively. This is to be expected because odometry errors are cumulative and thus influenced by the size and duration of the test patterns. The rectangular test used a square with 10 meter sides as compared to the much lager circular test patter which used a circle with a 25 meter radius. Below, we consider absolute GPS and odometry errors.In order to quantify GPS and odometry errors, with respect to the real world coordinate frame of reference, the robots were driven along a linear course with known intermediate points.The cumulative linear odometry error was found to be 0.4% (1/5 of one percent) per meter traveled. Errors were averaged over 6 runs of traveling forward and back along a course of 14.8 meters over dirt and grass.Filtered GPS position error was calculated as the average difference between each GPS reading and the robot’s true position at the time of reading. The robot was driven along a known linear path similar to the one shown in Fig. 6. A total of 5 tests were conducted on 3 different days. The average GPS position error was found to be 0.91 (m) with a standard deviation of error of 0.52 (m) over all of the test runs. Note that a total of approximately 2400 data points were used to generate the average error. This actual position error is somewhat lower than, but consistent with estimated position errors reported in [13].VI.C ONCLUSIONIn this paper a novel form of integration of MATLAB into a distributed behavioral robotics control architecture was presented. The architecture supports advanced robot control research both at the AI level and at the control-theoretic level. It is implemented in Java and uses Jini to manage distributed objects and services between robots and computers. The JMatLink Java library was used to incorporate MATLAB into the Distributed SFX architecture. MATLAB within the architecture and servers the dual purposes of providing a versatile robot experimentation tool, and as a rapid module and service implementation tool. Although modules and services can be implemented in many native languages, the ability to use interpreted MATLAB code is beneficial because it allows the efficient and direct transfer of research and development results generated in MATLAB into functional service implementations within the paradigm of the distributed behavioral architecture.The architecture was used to conduct automated experiments to quantify GPS and odometry position errors in mobile robots in an outdoor environment. During the experiments all data collection, robot sensor and actuator services and higher level components were managed by the architecture as distributed objects existing on a system consisting of one robot in the field and an additional computer workstation in the lab. These results show the versatility of the architecture and lay the ground work for GPS and odometry based positioning control for the ATRV-JR mobile robot platforms used here.A CKNOWLEDGMENTSThis work was partially supported by a grant form ONR, N 000 14-03-1-786 (2132-033-LO). L. Doitsidis was partially supported by “IRAKLITOS fellowships for research from the Technical University of Crete, EPEAEK II – 88727R EFERENCES[1] R. R. Murphy, Introduction to AI Robotics, The MIT Press,Massachusetts, 2000.[2] S. Müller, H. Waller, “Efficient Integration of Real-TimeHardware and Web Based Services Into MATLAB,” ESS'99 11th European Simulation Symposium and Exhibition,Erlangen-Nuremberg, 1999, ESS'99, Oct. 26-28, 1999.JMatLink download site: http://www.held-mueller.de/JMatLink/[3] A. D. Mali, “On the Behavior-based Architectures ofAutonomous Agency,” IEEE transactions on Systems, Man and Cybernetics, Part C: Applications and Reviews, vol. 32, no. 3, August 2002, pp. 231-242.[4] L. E. Parker, “ALLIANCE: An Architecture for FaultTolerant Multi-Robot Cooperation,” IEEE Transactions on Robotics and Automation, vol. 14, no. 2, pp. 220-240, 1998. [5] M. T. Long, R. R. Murphy, L. E. Parker, “Distributed multi-agent diagnosis and recovery from sensor failures,”Intelligent Robots and Systems, 2003 (IROS 2003),。