Robust adaptive control of nonholonomic mobile robot with parameter and nonparameter uncertainties
机器人运动控制方法综述
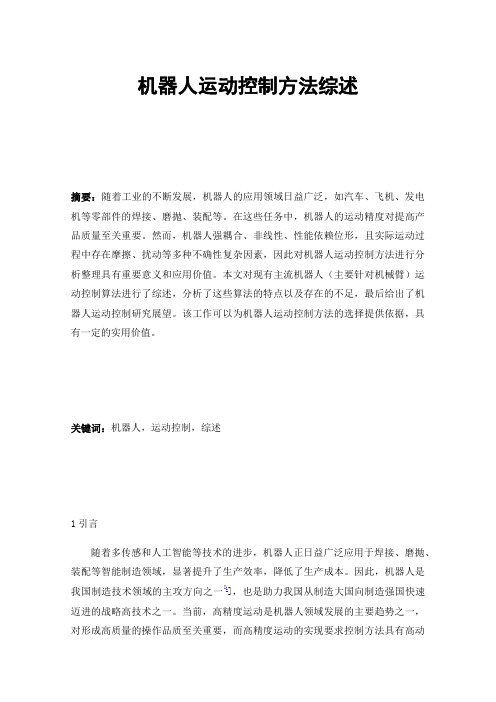
机器人运动控制方法综述摘要:随着工业的不断发展,机器人的应用领域日益广泛,如汽车、飞机、发电机等零部件的焊接、磨抛、装配等。
在这些任务中,机器人的运动精度对提高产品质量至关重要。
然而,机器人强耦合、非线性、性能依赖位形,且实际运动过程中存在摩擦、扰动等多种不确性复杂因素,因此对机器人运动控制方法进行分析整理具有重要意义和应用价值。
本文对现有主流机器人(主要针对机械臂)运动控制算法进行了综述,分析了这些算法的特点以及存在的不足,最后给出了机器人运动控制研究展望。
该工作可以为机器人运动控制方法的选择提供依据,具有一定的实用价值。
关键词:机器人,运动控制,综述1引言随着多传感和人工智能等技术的进步,机器人正日益广泛应用于焊接、磨抛、装配等智能制造领域,显著提升了生产效率,降低了生产成本。
因此,机器人是我国制造技术领域的主攻方向之一[1],也是助力我国从制造大国向制造强国快速迈进的战略高技术之一。
当前,高精度运动是机器人领域发展的主要趋势之一,对形成高质量的操作品质至关重要,而高精度运动的实现要求控制方法具有高动态响应和强鲁棒性。
然而,机器人齿轮和连杆结构受载后均存在不同程度的变形,且整个系统本身强耦合、非线性、动态特性时变、操作工况不确定性复杂多样,导致高动态响应和强鲁棒性的运动控制方法设计尤为困难,从而产生较大控制偏差,影响机器人运动精度,造成操作质量下降。
基于上述分析,本文对机器人(主要针对机械臂)的主流运动控制方法进行了综述,分析了这些方法中存在的不足,并给出了机器人运动控制方法未来发展趋势的一些思考。
2机器人运动控制方法作为一种复杂自动化系统,机器人具有非线性、强耦合、多变量时变特性。
高速运动时各关节惯量变化较大,耦合强烈,低速时摩擦、饱和等非线性效应明显,这些极大地增加了控制难度。
为了使机器人系统稳定运行,要求控制系统能实时提供与机器人动力学特征和多源扰动特征相匹配的控制特性,这给予了运动控制方法极大的挑战。
机械臂自适应非奇异快速终端滑模控制
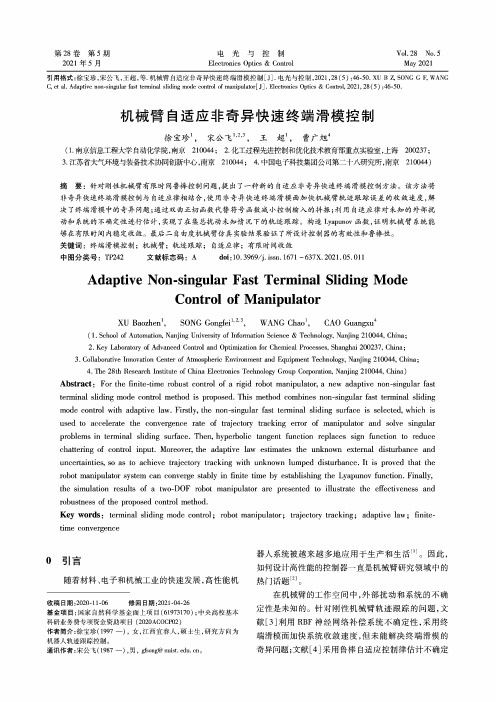
电光与控制Electronics Optics&Control Vol.28No.5 May2021第28卷第5期2021年5月引用格式:徐宝珍,宋公飞,王超,等•机械臂自适应非奇异快速终端滑模控制[J]•电光与控制,2021,28(5):46-50.XU B Z,SONG G F,WANG C,et al.Adaptive non-singular fast terminal sliding mode control of manipulator[J].Electronics Optics&Control,2021,28(5):46-50.机械臂自适应非奇异快速终端滑模控制徐宝珍1,宋公飞33,王超1,曹广旭"(1.南京信息工程大学自动化学院,南京210044;2.化工a程先进控制和优化技术教育部重点实验室,上海200237;3.江苏省大气环境与装备技术协同创新中心,南京210044;4.中国电子科技集团公司第二十八研究所,南京210044)摘要:针对刚性机械臂有限时间鲁棒控制问题,提出了一种新的自适应非奇异快速终端滑模控制方法。
该方法将非奇异快速终端滑模控制与自适应律相结合,使用非奇异快速终端滑模面加快机械臂轨迹跟踪误差的收敛速度,解决了终端滑模中的奇异问题;通过双曲正切函数代替符号函数减小控制输入的抖振;利用自适应律对未知的外部扰动和系统的不确定性进行估计,实现了在集总扰动未知情况下的轨迹跟踪。
构造Lyapunov函数,证明机械臂系统能够在有限时间内稳定收敛。
最后二自由度机械臂仿真实验结果验证了所设计控制器的有效性和鲁棒性。
关键词:终端滑模控制;机械臂;轨迹跟踪;自适应律;有限时间收敛中图分类号:TP242文献标志码:A doi:10.3969/j.issn.1671-637X.2021.05.011Adaptive Non-或ngular Fast Terminal Sliding ModeControl of ManipulatorXU Baozhen1,SONG Gongfei1'2,3,WANG Chao1,CAO Guangxu4(1.School of Automation,Nanjing University of Information Science&Technology,Nanjing210044,China;2.Key Laboratory of Advanced Control and Optimization for Chemical Processes,Shanghai200237,China;3.Collaborative Innovation Center of Atmospheric Environment and Equipment Technology,Nanjing210044,China;4.The28th Research Institute of China Electronics Technology Group Corporation,Nanjing210044,China)Abstract:For the finite-time robust control of a rigid robot manipulator,a new adaptive non-singular fast terminal sliding mode control method is proposed.This method combines non-singular fast terminal sliding mode control with adaptive law.Firstly,the non-singular fast terminal sliding surface is selected,which is used to accelerate the convergence rate of trajectory tracking error of manipulator and solve singular problems in terminal sliding surface.Then,hyperbolic tangent function replaces sign function to reduce chaHeiing of control input.Moreover,the adaptive law estimates the unknown external disturbance and uncertainties,so as to achieve trajectory tracking with unknown lumped disturbance・It is proved that the robot manipulator system can converge stably in finite time by establishing the Lyapunov function.Finally, the simulation results of a two-DOF robot manipulator are presented to illustrate the effectiveness and robustness of the proposed control method.Key words:terminal sliding mode control;robot manipulator;trajectory tracking;adaptive law;finitetime convergence0引言随着材料、电子和机械工业的快速发展,高性能机收稿日期:2020-11-06修回日期:2021-04-26基金项目:国家自然科学基金面上项目(61973170);中央高校基本科研业务费专项资金资助项目(2020ACOCP02)作者简介:徐宝珍(1997-),女,江西宜春人,硕士生,研究方向为机器人轨迹跟踪控制。
国家自然基金申请成功的申请书样本2 new!

第4页
版本 1.029.231
国家自然科学基金申请书
报告正文
(一) 立论依据和研究内容
1. 项目的立项依据
对系统的某一平衡点而言,系统的镇定问题是研究如何设计一个控制器使得闭环系 统对包含该平衡点的某一区域中的任何初值,其运动轨迹都能渐近收敛到该平衡点。这 是研究系统从一个状态精确地运动到另一状态以及系统跟踪控制问题的基础。自上世纪 八十年代以来,随着机器人和自动驾驶技术的发展,迫切需要考虑受控对象与环境接触 的非完整约束下的镇定控制问题。在随后的二十多年内,这一问题成了控制理论界研究 的热点之一。国际上上世纪 80 年代至 90 年代中期,对非完整系统镇定控制的研究主要 是针对由非完整约束方程导出的非完整运动学系统进行的,并取得了如非光滑控制器、 时变控制器以及混合控制器等一大批理论结果[1]。由于实际系统是动力学系统, 在对 系统性能要求较高的情况下通常不能忽略系统的动力学部分。因此自 90 年代后期国际 上更加注重非完整动力学系统镇定的控制研究。通常采用速度跟踪的思想将对非完整运 动学系统设计的控制律推广到非完整动力学系统,这种研究一般依赖于非完整系统的准 确动力学模型[2,3,4]。考虑到非完整动力学系统控制研究具有很强的实际应用背景, 而对实际系统一般无法建立精确模型,且不可避免地受到各种干扰的影响,必须研究不 确定非完整动力学系统的有效控制方法。目前国内外在这方面的研究还刚刚起步,值得 一提的是我国学者对参数不确定非完整动力学控制系统,在镇定控制方面也做出了一定 的贡献[5,6,7]。此外,近两年来关于有外界扰动和传感器噪声情况下的非完整运动学 鲁棒镇定控制问题也有所论及[8]。
本项目就是试图利用视觉量测信息,从控制理论的角度,将量测和控制结合起来, 研究在视觉坐标系(指摄象机坐标系或图象坐标系)下非完整控制系统镇定问题的理论 和方法,为进一步从理论和实际的结合上研究非完整控制系统开拓新的思路。
Adaptive Trajectory Tracking Control of Skid-Steered Mobile Robots
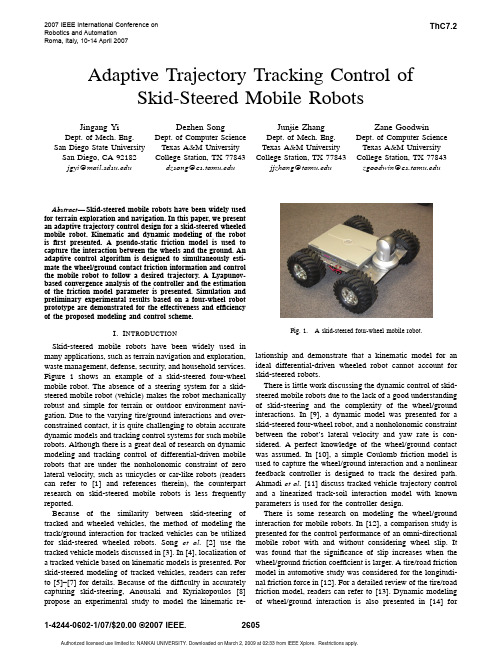
1-4244-0602-1/07/$20.00 ©2007 IEEE.
2605
Authorized licensed use limited to: NANKAI UNIVERSITY. Downloaded on March 2, 2009 at 02:33 from IEEE Xplore. Restrictions apply.
I. I NTRODUCTION Skid-steered mobile robots have been widely used in many applications, such as terrain navigation and exploration, waste management, defense, security, and household services. Figure 1 shows an example of a skid-steered four-wheel mobile robot. The absence of a steering system for a skidsteered mobile robot (vehicle) makes the robot mechanically robust and simple for terrain or outdoor environment navigation. Due to the varying tire/ground interactions and overconstrained contact, it is quite challenging to obtain accurate dynamic models and tracking control systems for such mobile robots. Although there is a great deal of research on dynamic modeling and tracking control of differential-driven mobile robots that are under the nonholonomic constraint of zero lateral velocity, such as unicycles or car-like robots (readers can refer to [1] and references therein), the counterpart research on skid-steered mobile robots is less frequently reported. Because of the similarity between skid-steering of tracked and wheeled vehicles, the method of modeling the track/ground interaction for tracked vehicles can be utilized for skid-steered wheeled robots. Song et al. [2] use the tracked vehicle models discussed in [3]. In [4], localization of a tracked vehicle based on kinematic models is presented. For skid-steered modeling of tracked vehicles, readers can refer to [5]–[7] for details. Because of the difficulty in accurately capturing skid-steering, Anousaki and Kyriakopoulos [8] propose an experimental study to model the kinematic re-
含未知信息的轮式移动机器人编队确定学习控制

含未知信息的轮式移动机器人编队确定学习控制彭滔;刘成军【摘要】This paper investigates the formation control of wheeled mobile robots(WMR)with unknown information under nonholonomic constraints.Firstly,based on the leader-follower method and the virtual structure method,the forma-tion control is transformed into the problem that the followers track their virtual leader. Secondly,a radial basis function neural network(RBF NN)is used to learning the unknowninformation(closed-loop system dynamics)of WMR,and a stable adaptive RBF NN controller and the stable adaptive tuning law of RBF NN parameters are derived in the sense of the Lyapunov stabilitytheory.According to deterministic learning,a partial persistentexcitation(PE)condition of some inter-nal signals in the closed-loop system is satisfied in the control process of tracking a recurrent reference trajectory,and an accurate approximation of the unknown closed-loop system dynamics is achieved by the RBF NN parameters convergence to their optimal weights. Finally,a RBF NN learning controller which effectively utilizes the learned knowledge without re-adapting the RBF NN parameters is proposed to achieve the closed-loop stability and improve the control performance, and simulation studies are included to demonstrate the correctness and effectiveness of the proposed approach.%本文研究含未知信息的轮式移动机器人(wheeled mobile robots,WMR)的编队控制问题.首先,基于领航-跟随法和虚拟结构法,将WMR编队控制问题转化为跟随机器人对参考虚拟机器人的跟踪控制问题.然后,利用径向基函数神经网络(radial basis function neural networks,RBF NN)对WMR的未知系统动态进行学习,以及根据李雅普诺夫稳定性理论设计了稳定的自适应RBF NN控制器和RBF NN权值估计的学习率.依据确定学习理论,闭环系统内部信号在对回归轨迹实现跟踪控制的过程中满足部分持续激励(persistent excitation,PE)条件.随着PE条件的满足,RBF NN权值估计收敛到其理想权值,实现了对未知闭环系统动态的准确学习.最后,利用学习结果设计了RBF NN学习控制器,保证了控制系统的稳定与收敛,实现了闭环稳定性和改进了控制性能,并通过仿真验证了所提控制方法的正确性和有效性.【期刊名称】《控制理论与应用》【年(卷),期】2018(035)002【总页数】9页(P239-247)【关键词】未知信息;移动机器人编队;非完整约束;系统动态;学习控制【作者】彭滔;刘成军【作者单位】重庆理工大学电气与电子工程学院,重庆400054;重庆理工大学电气与电子工程学院,重庆400054【正文语种】中文【中图分类】TP2421 引言(Introduction)近30年来,移动机器人编队因具有广泛的应用前景,使其成为机器人领域中的研究热点[1–2].经过多年的研究,现在已经形成了领航–跟随法(leader-follower)[3–4]、基于行为法(behavior based)[5–7]和虚拟结构法(virtual structure)[8–9]3种最常用的方法,其中领航–跟随法具有数学分析简单,机器人编队运动安全高效和易于形成和保持队形等优点,已广泛应用于移动机器人编队控制研究的各领域.现有的研究结果主要是基于机器人的线速度和角速度为控制输入的运动学模型,设计运动学控制器完成编队控制.这使得跟踪速度控制器决定了系统控制的稳定性,为达到编队控制目标需要“完美的”速度跟踪控制[10].这些研究结果大多没有考虑移动机器人的动力学特性,缺乏对高度非线性,不确定性和系统干扰等的鲁棒性;而在实际情况中存在诸多的非线性、扰动和不确定性等因素,例如作用于移动机器人的噪声、扰动、摩擦、负载变化以及未建模动态等.为保证移动机器人跟踪期望速度达到编队控制误差收敛到零,需要考虑机器人的动力学特性.在文献[11]和[12]中,Dierks等通过联合多层神经网络将单移动机器人的轨迹跟踪控制扩展到编队领航跟随控制,该方法用神经网络学习机器人编队的完整动力学和神经网络观测器估计机器人的线速度和角速度,设计了神经网络输出反馈控制器实现了稳定.在文献[13]中,Hou等利用反步技术(backsteping)和模糊逻辑方法为含有不确定动力学和外部扰动的移动机器人提出了自适应控制方法,该方法通过模糊系统在线学习机器人平台的动力学和运动学,使得这些信息不再为必须的先验知识;在文献[14]中利用神经网络对含有不确定动力学和外部扰动的多智能体一致性问题提出了鲁棒自适应控制方法,并将该方法推广到多智能体的编队控制中.在文献[15]中,Defoort等对含有界不确定扰动时变机器人编队问题设计了二阶滑模鲁棒控制器,该方法只需要测量机器人之间的相对构形而不必要测量或估计领航机器人的速度.在文献[16]中,申动斌等对打滑状态下的多机器人编队控制采用领导者–跟随者策略协调各机器人的运动,利用二阶滑模控制方法设计了控制器,使得机器人编队在运动过程中能够形成期望的队形.在文献[17]和[18]中,李艳东等利用神经网络对动力学不确定部分进行了在线估计,设计了自适应控制器和滑模控制器.这些研究结果包含了移动机器人的动力学模型,所提方法中的神经网络单纯地是一个逼近器,每次(即便重复相同的控制任务)都要对神经网络重新训练,对于系统未知动力学的学习问题都未研究.上述文献中所提出的自适应神经网络控制方法都是基于神经网络的通用逼近性展开的,对神经网络是否真的逼近了机器人系统中的未知信息没有进行深入的探讨.本文研究含未知信息轮式移动机器人(WMR)的编队控制问题,基于确定学习方法综合运用移动机器人的运动学和动力学模型,利用径向基函数神经网络(RBF NN)学习WMR的未知闭环系统动态,依据李雅普诺夫稳定性理论设计了稳定的自适应RBF NN控制器和RBF NN权值估计的学习率.闭环系统内部信号在对回归轨迹实现跟踪控制的过程中,满足部分持续激励(PE)条件,使得RBF NN权值估计收敛到其理想权值,实现了对未知闭环系统动态的准确学习.最后,利用学习结果设计了RBF NN学习控制器,保证了控制系统的稳定与收敛,实现了闭环稳定性和改善了控制性能,并用仿真研究验证了所提控制方法的正确性和有效性.2 系统模型(System model)2.1 移动机器人模型(WMR model)如图1中所示的WMR,其运动学和动力学模型为[19]其中:q=[x y θ]T为位姿向量,(x,y)表示后轴中点在全局坐标系中的坐标,θ为方向角;驱动轮间距为2R,半径为r,d为后轴到前端的距离;V=[υ ω]T为广义速度向量,υ和ω分别为线速度和角速度;为惯性矩阵,为向心力和哥氏力矢量,为重力矢量,为表面摩擦力矢量,τd为未知扰动,为控制转换矩阵,τ为控制输入向量.一般地,已知,和难以准确获知.当WMR在水平二维平面内运动时,有矩阵其中:m是WMR的质量,I为WMR的绕轮轴参考点的转动惯量.WMR的动力学方程(1)可改写为其中式(2)中的未知部分可以用一个非线性函数表示为则式(2)可写为图1 领航–跟随机器人编队结构示意图Fig.1 Leader–follower formation sketch 2.2 编队模型(Formation model)如图1所示,对WMR编队用领航–跟随法和虚拟结构法,设队形规划中期望距离为ld,期望角度为φd,用Rl,Rf和Rr分别表示领航WMR和跟随WMR和参考虚拟目标WMR(注下标l代表领航,f代表跟随和r代表参考),则Rf跟踪Rl可转化为跟踪Rr,Rr的姿态可描述为qr=[xryrθr]T,可定义运动学误差系统为由于WMR受非完整约束,每个WMR的方向角在队形变换时不相等,选择θr满足[20]其中βr=θl−θr∈ (−π,π].对式(4)两边微分可得其中:ϕ=φd− β,β =θl− θf.令WMR编队满足下列假设条件:假设1 WMR编队成员间有无延迟的无线通信.假设2Rl的参考线速度和角速度是有界的,并能将测得的Vl通过通讯传递给Rf. 假设 3Rf能测得l,φ和Vf,且Vf有界.3 控制目标(Control objective)本文的研究内容不包括WMR的路径规划,队形规划和避障等问题.根据领航–跟随法可知,Rf的位姿qf=[xfyfθf]T可由Rl的位姿qf=[xlylθl]T及(ld,φd)唯一决定.因此,只要控制(l,φ)使和则可达到期望队形.根据虚拟结构法,控制任务转化为设计一个光滑的速度控制器V使时,WMR编队从当前队形趋近目标队形,即为误差系统(5)渐近稳定时,设计动力学控制器对含未知信息的动力学系统(1)(或(3))稳定.4 自适应神经网络控制(Adaptive neural network control)为了镇定运动学误差系统(5),使Rf到达Rr的位姿,文献[20]中提出了如下速度控制Vfc,可以使得式(5)渐近稳定:其中:定义速度误差Ef为则式(5)可转换为其中对式(7)两边微分,并将式(3)代入可得定义则式(9)可写成由RBF NN的万能逼近性质,对未知闭环系统动态Hf存在一个理想权值向量W∗使得其中:S(Z)=diag{S1(Z),···,Sn(Z)},S(·)为高斯函数,为逼近误差,且‖ϵ(Z)‖≤ ϵ∗(ϵ∗>0);∀Z∈Ω⊂ R5(Ω为紧集).对动力学误差系统(10),设计控制输入和权值估计学习率为其中:控制增益矩阵Kf=diag{kfi},kfix>0;为RBF NN权值估计,是用来逼近的;Γ为常数对角矩阵,σ为较小的正常数.对于式(8)(10)和(11)构成的闭环系统,选取如下李雅普诺夫函数:其中对V1求导得因为对∀θe∈ (−π,π)成立,则有当时,式(14)严格小于零.利用三角不等式可得又因为其中0<k4<2,则其中选择适当的Kf以使得Kf1> 0.让由控制对象(3)(5)和(10),控制器(6)和(11)构成的闭环误差系统为其中定理1 考虑闭环误差系统(16),对给定的从初始条件q(0)∈Ω0(Ω0为紧集)出发的任一回归参考轨迹ψ(q(0)),有初始条件q(0)∈Ω0和可得:1)闭环系统(16)中的所有信号保持最终一致有界;2)适当选择设计参数,存在一个有限时间T,使误差信号Ef收敛到零的小领域内,∀t≥T.证 1)从式(15)可知,当t→∞,有所以Ef和最终一致有界,又因为Z和S(Z)有界,则输入τ有界.由此可得,闭环系统中的所有信号都最终一致有界.2)选取李雅普诺夫函数求导得利用三角不等式有其中s∗为‖S(Z)‖的上界[21].于是式(17)可表示为其中选择适当的Kf以使得Kf2> 0.让显然,选择足够大的k5可使得δ2足够小.由式(18)可得不等式(19)意味着对给定的存在一个有限时间T,使得∀t≥ T,Ef满足‖Ef‖ <ι1,ι1为小的残差集.通过选取大的Kf可使Ef足够小,即通过选取适当的设计参数,可使Ef在有限时间T内收敛到零的小领域内[22]. 证毕.5 确定学习(Deterministic learning)根据确定学习理论[23],系统(16)在时间T后利用RBF NN的局部特性,沿着跟踪轨迹ψ(q(t))|t≥T可表示为其中:Sξ(Z)为S(Z)的子向量,为相应权值子向量,下标ξ和分别代表靠近和远离轨迹ψ(q(t))|t≥T的区域,ϵξ为局部逼近误差,且‖ϵξ‖是很小的值.定理2 考虑闭环误差系统(20),对任意给定的从初始条件q(0)∈Ω0(Ω0为紧集)出发的任一回归参考轨迹ψ(q(0)),有初始条件q(0)∈Ω0和合理地选取控制参数,则沿着跟踪轨迹ψ(q(t))|t≥T,RBF NN权值收敛到理想权值的小领域内,未知闭环系统动态Hf可由和来局部准确逼近,其中证令则式(20)可表示为将上式写成矩阵形式为由定理1知,RBF NN的输入Z(t)时间T后为回归轨迹,回归子向量Sξ(Z)满足PE条件[23],根据文献[24]中的引理1可知系统(21)的标称系统一致指数稳定.对于系统(21)根据文献[25]的引理4.6,权值误差在有限时间T1(T1>T)内指数收敛到零的小领域内,领域的大小由NN逼近能力和状态跟踪误差决定.未知闭环系统动态可由整个RBF神经网络和准确逼近,即其中‖ϵ′‖和‖ϵ′′‖是很小的值,表明整个RBF神经网络和沿着轨迹ψ(q(t))|t≥T能够逼近未知闭环系统动态到任意准确度.从式(22)可知,对于经历的回归轨迹ψ(q(t)),存在小的正常数ι2,沿着ψ(q(0))存在一个局部区域Ωψ满足[23]其中ϵ∗2接近ϵ∗. 证毕.6 学习控制(Learning control)运用学习结果,对动力学误差系统(10),可用RBF NN学习控制器(23)替代自适应RBF NN控制器(11)实现学习控制其中:为未知闭环系统动态的局部准确逼近,是学习过程中存储的RBF NN常数权值. 定理3 由控制对象(3),控制器(6)和(23)构成的闭环系统,对任意给定的从初始条件q(0)∈Ω0(Ω0为紧集)出发的任一回归参考轨迹ψ(q(0)),有初始条件q(0)∈ Ω0和可得:1)闭环系统中的所有信号保持最终一致有界;2)适当选择设计参数,存在一个有限时间T2,使误差信号Ef收敛到零的小领域内,∀t≥T2.证将式(23)代入式(10)得选取李雅普诺夫函数为对V3求导化简可得由三角不等式可得其中k6>0,由此可得其中选择适当的Kf以使得Kf3> 0.取令由于学习阶段的准确逼近使ϵ∗2很小,因此ι3和δ3也很小.从式(25)可得则有类似定理1的证明,可得闭环系统中所有信号保持最终一致有界.从式(26)可知,不用选择大的设计参数k6,Ef能指数收敛到零的一个小领域内,即存在一个有限时间T2>0,对任意的t>T2,误差Ef收敛到零的一个小领域内. 证毕.7 系统控制性能分析((The analysis of system control performance)综合上述分析,对比自适应RBF NN控制器(11)和RBF NN学习控制器(23)的控制性能有:i)使用自适应RBF NN控制器(11)时不满足PE条件,与许多自适应NN控制的文献获得的结果一样,能保证有界,但不能保证它收敛到零的一个小区域内.为保证误差Ef 收敛到零的一个小领域内,须选择足够大的k5(即足够大的Kf),以使δ2足够小.然而,较大的k5容易引起高增益控制,应在实际应用中避免.ii)使用RBF NN学习控制器(23),由于学习阶段的准确逼近,不需选择大的控制参数k6(即Kf)就能保证ι3和δ3足够小,从而保证误差Ef收敛到零的一个小领域内.同时,RBF NN学习控制器(23)采用了无需在控制过程中更新的常数权值,比自适应RBF NN控制器(11)能在软硬件实现时节约时间和能量,这在实际应用中有优势.8 仿真研究((Simulation studies)为了验证所提控制方法的正确性和有效性,本部分运用MATLAB进行仿真研究.选取WMR参数为控制目标为ld=1m,选取领航机器人Rl的轨迹为初值为跟随机器人Rf的初值设为参考虚拟目标机器人Rr的轨迹可由如下方程组计算得到选择控制参数为k1=1,k2=0.5,kv=5;RBF NN节点数N=1600,初值W=zeros(N,1),Γ=2∗eye(N),σ=0.0001;RBF NN中心点均匀分布在[−2:1:2],[−2:1:2],[−1:1:2],[−1.5:1.5:3],[−4:1:1]网格点上.设置=[0.06m(v2f+2ω2f)|θf|,0.06m(2v2f+ω2f)|θf|]T,=0.在自适应RBF NN控制阶段设干扰为τd=0.1∗[sint cost]T,在RBF NN学习控制阶段τd=1∗[sint cost]T[26].仿真研究结果如图2–13所示.图2–6展示了自适应RBF NN控制的控制效果,其中图2–3是线速度和角速度跟踪曲线.图2 自适应神经网络控制线速度跟踪Fig.2 Adaptive NN control linear velocity tracking图3 自适应神经网络控制角速度跟踪Fig.3 Adaptive NN control angular velocity tracking图4–6是轨迹跟踪和姿态误差曲线.从图2–6中可以看出,在前25s误差相对较大,从图2–5可以看出25s后两条曲线几乎重合,从图6中可以看出特别是前10s的最大误差超过了0.5,在10∼25s的最大误差也接近0.5,25s过后误差一直保持在0.15以内,这表明Rf稳定的跟踪上Rr.分析原因是前25s RBF NN权值处于在线调整中,没有收敛到稳定值,这导致误差相对较大,这吻合图7中的RBF NN权值收敛过程.图4 自适应神经网络控制轨迹跟踪(2维)Fig.4 Adaptive NN control trajectory图5 自适应神经网络控制轨迹跟踪(3维)Fig.5 Adaptive NN control trajectory tracking(three dimensional)图6 自适应神经网络控制姿态跟踪误差Fig.6 Adaptive NN control posture tracking error未知闭环系统动态的RBF NN学习效果如图7–8所示,其中图7是部分RBFNN权值收敛,图8是未知闭环系统动态的学习误差曲线.从图7中可以看出,RBF NN络权值经过25s的调整收敛到常值.未知闭环系统动态的RBF NN学习效果如图8所示,在权值调整过程中学习误差偏大,当权值收敛之后学习误差大大减少,这意味着学习效果改善.因此,图7和图8表明本文提出的自适应RBF NN控制方法在Rf稳定跟踪Rr的过程中,实现了对未知闭环系统动态的准确学习.图7 部分神经网络权值收敛Fig.7 The partial NN weights convergence图8 未知闭环系统动态学习误差Fig.8 Unknown closed-loop system dynamics learning error图9–13展示了RBF NN学习控制的控制效果,图9–10是线速度和角速度跟踪曲线,图11–13是轨迹跟踪和姿态误差曲线.从图9–12中可以看出两条曲线没有调整过程始终保持几乎重合,从图13中可以看出姿态跟踪误差几乎一直保持在0.15以内,这表明Rf稳定的跟踪上Rr所需时间较采用自适应RBF NN控制器少了许多,分析原因是RBF NN学习控制利用了已学到的知识使得RBF NN权值无需再调整. 图9 学习控制线速度跟踪Fig.9 Learning control line velocity tracking图10 学习控制角速度跟踪Fig.10 Learning control angular velocity tracking 图11 学习控制轨迹跟踪(2维)Fig.11 Learning control trajectory tracking(two dimensional)图12 学习控制轨迹跟踪(3维)Fig.12 Learning control trajectory图13 学习控制姿态跟踪误差Fig.13 Learning control posture tracking error 通过上述对比分析两种控制效果,使用了学过知识的RBF NN学习控制器,实现了系统的稳定控制,并节约了资源和改善了性能.9 结论(Conclusions)本文针对含未知信息的WMR研究了编队控制,利用RBF NN提出了自适应神经网络控制器和学习控制器,实现了闭环系统的所有信号最终一致有界.由于对回归轨迹满足部分PE条件,在稳定的闭环动态控制过程中RBF NN准确学习到未知闭环系统动态,并且以时不变且空间分布的方式表达和常数权值的方式存储.调用存储的RBF NN常数权值建立的学习控制器中包含已学到的系统未知信息,避免了RBF NN权值的重新训练和更新,实现了系统良好的控制.这在工程实践中能节约软硬件资源,是非常有用的.参考文献(References):【相关文献】[1]DONG X,YU B,SHI Z,et al.Time-varying formation control for unmanned aerial vehicles:theories and applications[J].IEEE Transactions on Control Systems Technology,2015,23(1):340–348.[2]WANG Yintao,YAN Weisheng.Consensus formation tracking control of multiple autonomous underwater vehicle systems[J].Control Theory&Applications,2013,30(3):379–384.(王银涛,严卫生.多自主水下航行器系统一致性编队跟踪控制[J].控制理论与应用,2013,30(3):379–384.)[3]DESAI J P,OSTROWSKI J,KUMAR V.Controlling formations of multiple mobilerobots[C]//Proceedings of IEEE International Conference on Robotics and Automation.Leuven:IEEE,1998:2864–2869.[4]LORIA A,DASDEMIR J,JARQUIN N A.Leader–follower formation and tracking control ofmobile robots along straight paths[J].IEEE Transactions on Control Systems Technology,2016,24(2):727–732.[5]BALCH T,ARKIN R C.Behavior-based formation control for multirobot teams[J].IEEE Transactions on Robotics and Automation,1998,14(6):926–939.[6]KUPPAN CHETTY R M,SINGAPERUMAL M,NAGARAJAN T.Behavior based multi robot formations with active obstacle avoidance based on switching controlstrategy[J].Advanced Materials Research,2012,433(440):6630–635.[7]QIU Huaxin,DUAN Haibin,FAN Yanming.Multiple unmanned aerialvehicleautonomousformationbasedonthebehaviormechanism in pigeonflocks[J].Control Theory&Applications,2015,32(10):1298–1304.(邱华鑫,段海滨,范彦铭.基于鸽群行为机制的多无人机自主编队[J].控制理论与应用,2015,32(10):1298–1304.)[8]BENZERROUK A,ADOUANE L,MARTINET P.Stable navigation in formation for a multi-robot system based on a constrained virtual structure[J].Robotics and Autonomous Systems,2014,62(12):1806–1815.[9]LEWIS M A,TAN K H.High precision formation control of mobile robots using virtual structures[J].Autonomous Robots,1997,4(4):387–403.[10]DAS A K,FIERRO R,KUMAR V.A vision based formation control framework[J].IEEE Transactions on Robotics and Automation,2002,18(5):813–825.[11]DIERKS T,JAQANNATHAN S.Neural network control of mobile robot formations using RISE feedback[J].IEEE Transactions on Systems,Man,and Cybernetics,PartB(Cybernetics),2009,39(2):332–347.[12]DIERKS T,JAQANNATHAN S.Neural network output feedback control of robot formations[J].IEEE Transactions on Systems,Man,and Cybernetics,PartB(Cybernetics),2010,40(2):383–399.[13]HOU Z G,ZOU A M,CHENG L,et al.Adaptive control of an electrically driven nonholonomic mobile robot via backstepping and fuzzy approach[J].IEEE Transactions on Control Systems Technology,2009,17(4):803–815.[14]HOU Z G,CHENG L,TAN M.Decentralized robust adaptive control for the multiagent system consensus problem using neural networks[J].IEEE Transactions onSystems,Man,and Cybernetics,Part B(Cybernetics),2009,39(3):636–647.[15]DEFOORT M,FLOQUET T,KOKOSY A,et al.Sliding-mode formation control for cooperative autonomous mobile robots[J].IEEE Transactions on Industrial Electronics,2008,55(11):3944–3953.[16]SHEN Dongbin,SUN Weijie.Multirobot formation control under slippingcondition[J].Journal of Mechanical Engineering,2012,48(23):30–35.(申动斌,孙伟杰.打滑状态下的多机器人编队控制[J].机械工程学报,2012,48(23):30–35.)[17]LIYandong,ZHULing,SUNMing.Adaptivecontrolofmobilerobot formations includingactuator dynamics[J].Computer Engineering and Applications,2014,50(1):235–239.(李艳东,朱玲,孙明.含驱动器动力学的移动机器人编队自适应控制[J].计算机工程与应用,2014,50(1):235–239.)[18]ZHU Ling,LI Yandong,SUN Ming,et al.Sliding mode control of mobile robot formations based on neural networks[J],Electric Machines and Control,2014,18(3):113–118.(朱玲,李艳东,孙明,等.移动机器人编队的神经网络滑模控制[J].电机与控制学,2014,18(3):113–118.)[19]FIERRO R,LEWIS F L.Control of a nonholonomic mobile robot using neuralnetworks[J].IEEE Transactions on Neural Network,1998,9(4):589–600.[20]WU K Y.Multiple mobile robots formation control and obstacleavoidance[C]//Proceedings of International Conference on Advanced Computer Control.Harbin:IEEE,2011:639–643.[21]AJ K,JD W,FJ N.Persistency of excitation in identification using radial basis function approximants[J].SIAM Journal on Control&Optimization,1995,33(2):625–642.[22]SLOTINE J E,LI W P.Applied Nonlinear Control[M].New Jersey,American:Prentice Hall,1991.[23]WANG C,HILL D J.Deterministic Learning Theory for Identification,Recognition,and Control[M].Boca Raton,American:CRC Press,2009.[24]LIU T F,WANG C,HILL D J.Learning from neural control of nonlinear systems in normal form[J].Systems&Control Letters,2009,58(9):633–638.[25]KHALIL H K.Nonlinear Systems[M].3rd edition.Englewood Cliffs:Prentice Hall,2002.[26]ZHOU Yong,WANG Cong,GU Wujun,et al.Deterministic learning and control of mobile robots[J].Control Theory&Applications 2012,29(1):119–124.(周勇,王聪,顾武军,等.移动机器人的确定学习与控制[J].控制理论与应用,2012,29(1):119–124.)。
驱动约束下直线电机自适应鲁棒优化控制
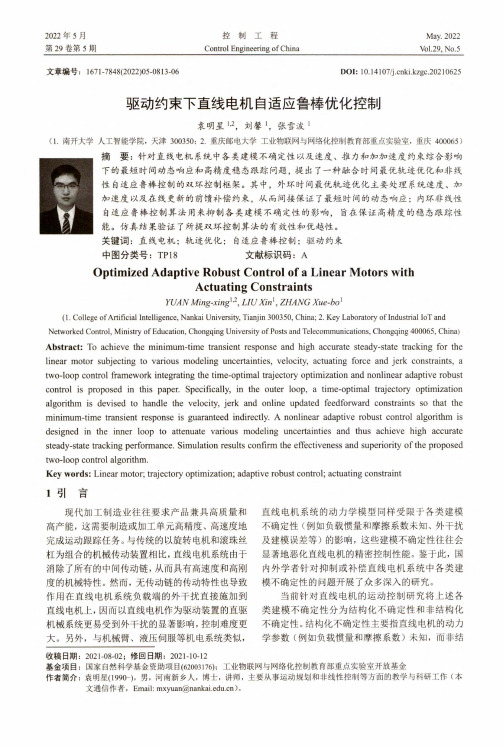
May. 2022Vbl.29, No.52022年5月 第29卷第5期控制工程Control Engineering of China文章编号:1671-7848(2022)05-0813-06DOI: 10.14107/j .cnki.kzgc.20210625驱动约束下直线电机自适应鲁棒优化控制袁明星",刘馨1,张雪波I(1.南开大学人工智能学院,天津300350; 2.重庆邮电大学工业物联网与网络化控制教育部重点实验室,重庆400065).摘 要:针对直线电机系统中各类建模不确定性以及速度、推力和加加速度约束综合影响 下的最短时间动态响应和高精度稳态跟踪问题,提出了一种融合时间最优轨迹优化和非线 性自适应鲁棒控制的双环控制框架。
其中,外环时间最优轨迹优化主要处理系统速度、加 加速度以及在线更新的前馈补偿约束,从而间接保证了最短时间的动态响应;内环非线性自适应鲁棒控制算法用来抑制各类建模不确定性的影响,旨在保证高精度的稳态跟踪性 能。
仿真结果验证了所提双环控制算法的有效性和优越性。
关键词:直线电机;轨迹优化;自适应鲁棒控制;驱动约束中图分类号:TP18 文献标识码:AOptimized Adaptive Robust Control of a Linear Motors withActuating ConstraintsYUANMing-xing 1-2, LIUXin x , ZHANG Xue-bo'(1. College of A rtificial Intelligence, Nankai University, Tianjin 300350, China; 2. Key Laboratory of Industrial IoT andNetworked Control, Ministry of Education, Chongqing University of Posts and Telecommunications, Chongqing 400065, China)Abstract: To achieve the minimum-time transient response and high accurate steady-state tracking for the linear motor subjecting to various modeling uncertainties, velocity, actuating force and jerk constraints, a two-loop control framework integrating the time-optimal trajectory optimization and nonlinear adaptive robustcontrol is proposed in this paper. Specifically, in the outer loop, a time-optimal trajectory optimizationalgorithm is devised to handle the velocity, jerk and online updated feedforward constraints so that the minimum-time transient response is guaranteed indirectly. A nonlinear adaptive robust control algorithm isdesigned in the inner loop to attenuate various modeling uncertainties and thus achieve high accuratesteady ・state tracking performance. Simulation results confirm the effectiveness and superiority of the proposedtwo-loop control algorithm.Key words: Linear motor; trajectory optimization; adaptive robust control; actuating constraint1引言现代加工制造业往往要求产品兼具高质量和 高产能,这需要制造或加工单元高精度、高速度地完成运动跟踪任务。
基于事件触发的多智能体分布式编队控制
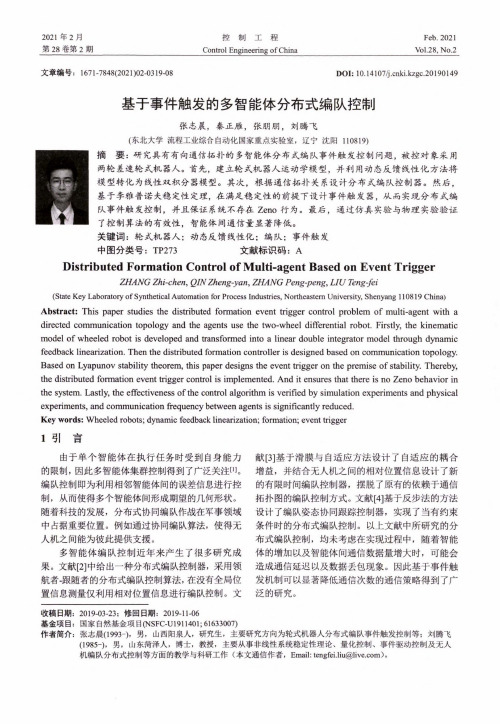
Feb. 2021Vdl.2& No.22021年2月 第28卷第2期控制工程Control Engineering of China文章编号:1671 -7848(2021 )02-0319-08DOI: 10.14107/ki.kzgc.20190149基于事件触发的多智能体分布式编队控制张志晨,秦正雁,张朋朋,刘腾飞(东北大学流程工业综合自动化国家重点实验室,辽宁沈阳110819)摘 要:研究具有有向通信拓扑的多智能体分布式编队事件触发控制问题,被控对象采用两轮差速轮式机器人。
首先,建立轮式机器人运动学模型,并利用动态反馈线性化方法将 模型转化为线性双积分器模型。
其次,根据通信拓扑关系设计分布式编队控制器。
然后,基于李雅普诺夫稳定性定理,在满足稳定性的前提下设计事件触发器,从而实现分布式编队事件触发控制,并且保证系统不存在Zeno 行为。
最后,通过仿真实验与物理实验验证 了控制昇法的有效性,智能体间通信量显著降低。
关键词:轮式机器人;动态反馈线性化;编队;事件触发中图分类号:TP273 文献标识码:ADistributed Formation Control of Multi-agent Based on Event TriggerZHANG Zhi-chen, QIN Zheng-yan, ZHANG Peng-peng, LIU Teng-fei(State Key Laboratory of Synthetical Automation for Process Industries, Northeastern University, Shenyang 110819 China)Abstract: This paper studies the distributed formation event trigger control problem of multi-agent with adirected communication topology and the agents use the two-wheel differential robot. Firstly, the kinematic model of wheeled robot is developed and transformed into a linear double integrator model through dynamic feedback linearization. Then the distributed formation controller is designed based on communication topology.Based on Lyapunov stability theorem, this paper designs the event trigger on the premise of stability. Thereby, the distributed formation event trigger control is implemented. And it ensures that there is no Zeno behavior in the system. Lastly, the effectiveness of the control algorithm is verified by simulation experiments and physicalexperiments, and communication frequency between agents is significantly reduced.Key words: Wheeled robots; dynamic feedback linearization; formation; event trigger1引言由于单个智能体在执行任务时受到自身能力 的限制,因此多智能体集群控制得到了广泛关注⑴。
基于扰动观测器的轮式移动机器人滚动时域路径跟踪控制
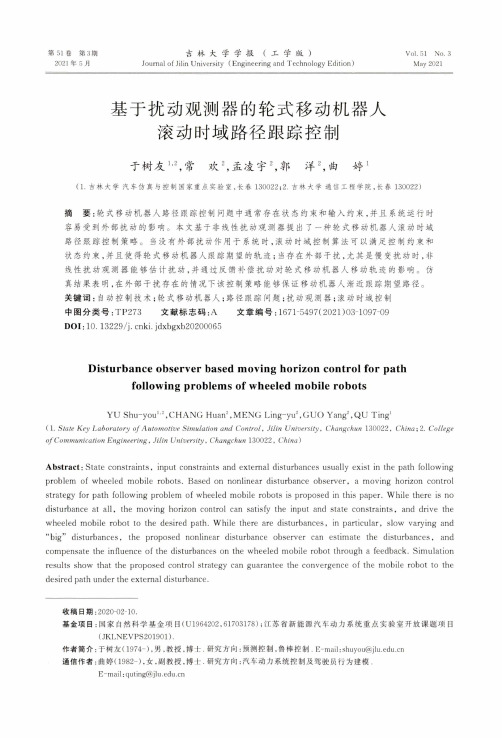
第51卷第3期2021年5月吉林大学学报(工学版)Journal of Jilin University (Engineering and Technology Edition)V o l. 51 N o. 3M a y 2021基于扰动观测器的轮式移动机器人滚动时域路径跟踪控制于树友“2,常欢2,孟凌宇2,郭洋2,曲婷1(1.吉林大学汽车仿真与控制国家重点实验室,长春130022;2.吉林大学通信工程学院,长春130022)摘要:轮式移动机器人路径跟踪控制问题中通常存在状态约束和输入约束,并且系统运行时 容易受到外部扰动的影响。
本文基于非线性扰动观测器提出了一种轮式移动机器人滚动时域 路径跟踪控制策略。
当没有外部扰动作用于系统时,滚动时域控制算法可以满足控制约束和 状态约束,并且使得轮式移动机器人跟踪期望的轨迹;当存在外部干扰,尤其是慢变扰动时,非线性扰动观测器能够估计扰动,并通过反馈补偿扰动对轮式移动机器人移动轨迹的影响。
仿 真结果表明,在外部干扰存在的情况下该控制策略能够保证移动机器人渐近跟踪期望路径。
关键词:自动控制技术;轮式移动机器人;路径跟踪问题;扰动观测器;滚动时域控制中图分类号:T P273 文献标志码:A文章编号:1671-5497(2021)03-1097-09D O I:10. 13229/ki.j d x b g x b20200065Disturbance observer based moving horizon control for pathfollowing problems of wheeled mobile robotsY U S h u-y o u12,C H A N G H u a n2,M E N G L i n g-y u2,G U O Y a n g z,Q U T i n g1(1. S ta te K e y L a b o r a to r y o f A u to m o tiv e S im u la tio n a n d C o n tro l ^J ilin U n i v e r s i ty C h a n g c h u n130022, C h in a;2. C o lle g e o f C o m m u n ic a tio n E n g in e e r in g, J ilin U n iv e r sity y C h a n g ch u n130022, C h in a)Abstract:State constraints,input constraints and external disturbances usually exist in the path following problem of w h e e l e d mobile robots.Ba s e d o n nonlinear disturbance observer,a m o v i n g horizon control strategy for path following p r o blem of wheeled mobile robots is proposed in this paper.W h i l e there is n o disturbance at all,the m o v i n g horizon control can satisfy the input and state constraints,and drive the w h e eled mobile robot to the desired path.W h i l e there are disturbances,in particular,slow varying and “big”disturbances,the proposed nonlinear disturbance observer can estimate the disturbances,and c o m p ensate the influence of the disturbances o n the w h e e l e d mobile robot through a feedback.Simulation results s h o w that the proposed control strategy can guarantee the convergence of the mobile robot to the desired path under the external disturbance.收稿日期:2020-02-10.基金项目:国家自然科学基金项目(U1964202,61703178);江苏省新能源汽车动力系统重点实验室开放课题项目(JKLNEVPS201901).作者简介:于树友(1974-),男,教授,博士 .研究方向:预测控制,鲁棒控制.********************.cn通信作者:曲婷(1982-),女,副教授,博士 .研究方向:汽车动力系统控制及驾驶员行为建模.E-mail :**************.cn•1098 .吉林大学学报(工学版)第51卷Key words:automatic control technology;w h e eled mobile robot;path following p r o b l e m;disturbance observer;m o d e l predictive control〇引言轮式移动机器人(W h e e l e d mobile robot,W M R>是典型的非完整约束系统由于Brockett 条件11的存在,不能获得连续可微、线性时不变的 反馈控制律镇定轮式移动机器人系统。
- 1、下载文档前请自行甄别文档内容的完整性,平台不提供额外的编辑、内容补充、找答案等附加服务。
- 2、"仅部分预览"的文档,不可在线预览部分如存在完整性等问题,可反馈申请退款(可完整预览的文档不适用该条件!)。
- 3、如文档侵犯您的权益,请联系客服反馈,我们会尽快为您处理(人工客服工作时间:9:00-18:30)。
IEEE TRANSACTIONS ON ROBOTICS,VOL.21,NO.2,APRIL2005261[24]P.Coelho and U.Nunes,“Lie algebra application to mobile robot con-trol:A tutorial,”Robotica,vol.21,no.5,pp.483–493,2003.[25]P.Coelho,“Path following control of wheeled mobile robots in presenceof uncertainties,”Ph.D.dissertation(in Portuguese),Univ.Coimbra, Coimbra,Portugal,2004.[26]G.Campion et al.,“Controllability and state feedback stabilization ofnonholonomic mechanical systems,”in Lecture Notes in Control and In-formation Science,C.C.de Wit et al.,Ed.New York:Springer-Verlag, 1991,vol.162,pp.106–124.[27]X.Yun and Y.Yamamoto,“Stability analysis of the internal dynamicsof a wheeled mobile robot,”J.Robot.Syst.,vol.14,no.10,pp.697–709, 1997.[28]L.E.Dubins,“On curves of minimal lenght with a constraint on av-erage curvature,and with prescribed initial and terminal positions and tangents,”Amer.J.Math.,vol.79,pp.497–516,1957.[29]H.Nijmeijer and A.Schaft,Nonlinear Dynamic Control Systems.NewYork:Springer-Verlag,1990.[30]K.J.Åström and B.Wittenmark,Computer Controlled Systems:Theoryand Design.Englewood Cliffs,NJ:Prentice-Hall,1997.[31]G.Pires and U.Nunes,“A wheelchair steered through voice commandsand assisted by a reactive fuzzy-logic controller,”J.Intell.Robot.Syst., vol.34,no.3,pp.301–314,2002.Robust Adaptive Control of Nonholonomic Mobile Robot With Parameter and Nonparameter UncertaintiesWenjie Dong and K.-D.KuhnertAbstract—This paper considers the tracking-control problem of a nonholonomic wheeled mobile robot with both parameter and nonpa-rameter uncertainties.A robust adaptive controller is proposed with the aid of the adaptive backstepping technique and the learning ability of neural networks.The proposed controller guarantees that the tracking error converges to a small ball containing the origin.The ball’s radius can be adjusted by control parameters.The proposed controller is successfully implemented in our simulator.Index Terms—Adaptive control,nonholonomic system,robust control, tracking control,wheeled mobile robot.I.I NTRODUCTIONIn recent years,there has been a growing interest in the design of feedback-control laws for mechanical systems subjected to non-holonomic constraints.Due to Brockett’s necessary condition for asymptotic feedback stabilization[1],a nonholonomic system cannot be asymptotically stabilized to a equilibrium by any smooth or con-tinuous pure-state feedback law.To overcome this limitation,several approaches have been proposed in last decade(see[18],[20],and the references therein).Tracking control is another control problem of nonholonomic sys-tems.Based on whether the system is described by a kinematic model or a dynamic model,the tracking-control problem is classified as ei-ther a kinematic or a dynamic tracking-control problem.The kine-matic tracking-control problem has been studied in recent years.With Manuscript received January1,2004;revised May11,2004.This paper was recommended for publication by Associate Editor G.Oriolo and Editor H.Arai upon evaluation of the reviewers’comments.W.Dong was with the Department of Electrical and Computer Engineering, University of Siegen,Siegen D-57068,Germany.K.-D.Kuhnert is with the Department of Electrical and Computer Engi-neering,University of Siegen,Siegen D-57068,Germany.Digital Object Identifier10.1109/TRO.2004.837236Fig.1.Configuration of a wheeled mobile robot.the aid of the linearization technique,local controllers are proposedin[9],[17],and[25].Based on the dynamic feedback linearizationand the differentialflatness concept,the dynamic controllers with sin-gular points are proposed in[4]and[10].In[15],global tracking con-trollers are proposed for nonholonomic wheeled mobile robots.Forchained systems,tracking controllers are proposed in[8]and[16].The dynamic tracking-control problem of the nonholonomic systemhas received attention recently,because most practical nonholonomicmechanical systems are dynamic systems and have uncertainties.In[23],Suand Stepanenko stu dy the tracking-control problem of the dy-namic nonholonomic systems with unknown inertia parameters,andpropose an adaptive controller.Chen et al.discuss the dynamic trackingproblem of the uncertain nonholonomic systems,and propose a ro-bust H-infinite controller in[2].In[9]and[11],the dynamic trackingproblem of a wheeled mobile robot is studied,and neural-network-based controllers are proposed.In[5],[6],[7],and[24],the dynamictracking-control problem of nonholonomic systems with uncertainty isdiscussed.Robust and adaptive controllers are proposed.Most of the results on the dynamic tracking problem of nonholo-nomic systems are proposed based on the assumption that the kine-matics of the system is exactly known,and there are only uncertaintiesin the dynamics of the system.However,in practice,there are uncer-tainties in both kinematics and dynamics.In this paper,we study thetracking-control problem of a wheeled mobile robot with uncertain-ties in both kinematics and dynamics.It is assumed that in the kine-matics,there are parameter uncertainties,and in the dynamics,there areparameter uncertainties and nonparameter uncertainties.To solve thistracking-control problem,a robust adaptive neural-network controlleris proposed with the aid of backstepping techniques and the approxi-mation property of neural networks.II.P ROBLEM S TATEMENTConsider a wheeled mobile robot moving on a horizontal plane(Fig.1).The robot is composed of a rigid body,twofixed rear wheels,and one steering wheel.Two torque motors are equipped in the frontwheel for driving and steering.Given a differentiable simple curve(C)defined by one of its points,the unitary tangent vector at this point,andits curvature curv(s),with s being the curvilinear coordinate alongthe curve,for a point Q in the curve(C),assume that the curvilinearcoordinate at Q is s.Let f Q;T(s);N(s)g be the Frenet frame onthe curve at point Q,and assume that j curv(s)j<1=R(8s)whereR(>0)is a constant.If the distance between P and the curve(C)issmaller than R,the position of P is parameterized by(s;d),whered is the coordinate of P along N(s).The robot’s configuration isparameterized by q=[q1;q2;q3;q4]T=[s;d; ; ]T,where is theangle between P F and T(s)and is the steering angle of the front 1552-3098/$20.00©2005IEEEAuthorized licensed use limited to: SOUTH CHINA UNIVERSITY OF TECHNOLOGY. Downloaded on July 26,2010 at 12:04:06 UTC from IEEE Xplore. Restrictions apply.262IEEE TRANSACTIONS ON ROBOTICS,VOL.21,NO.2,APRIL2005 wheel with respect to the robot body.By the classic law of mechanics,and also the results in[22],onehas_q1=v1cos q310curv(q1)q2 _q2=v1sin q3_q3=v1tan q4l 0v1curv(q1)cos q3 10curv(q1)q2_q4=v2(1)M(q)_v+C(q;_q)v+G(q)+U(q;_q)=B(q) (2) where l is the distance between the two points P and F;v1is the ve-locity of the point P;v2is the angular velocity of the steering wheel, M(q)is a bounded positive definite symmetric inertia matrix,C(q;_q)_q is centripetal and Coriolis torques,G(q)is the gravitational torque, U(q;_q)includes the unmodeled dynamics of the system and bounded continuous external disturbance,B(q)is the input matrix, is the con-trol input,and the superscript T denotes the transpose.For(1)–(2),it is assumed that:1)in(1),l is not exactly known,i.e.,l2[l min;l max]wherel max(>0)and l min(>0)are known;2)in(2),the matrices M(q);C(q;_q);G(q),and U(q;_q)areunknown;3)in(2),the expression of B(q)is known.In fact,it can beeasily derived that B(q)=diag[1=(r cos q4);1].In B(q),itis assumed that r is not exactly known,i.e.,r2[r min;r max]where r min(>0)and r max(>0)are known.Control Problem:Given a desired simple curve(C)and a desired velocity v31(t)of the robot,for(1)–(2),the control problem in this paper is defined asfinding a controller ,such thatlim t!1q2(t)=0limt!1q3(t)=0limt!1(v1(t)0v31(t))=0:Remark1:Noting that the assumption j curv(q1)j<1=R,(1)is well-defined if j q2j<R and j q4j< =2.In the controller design, these conditions will be guaranteed if j q2(0)j<R and j q4(0)j< =2. In(2),the following well-known property is satisfied[23]. Property1:For a suitably defined C(q;_q);(_M02C)is skew-symmetric.III.B ACKSTEPPING D ESIGN P ROCEDURETo deal with parameter uncertainties and nonparameter uncertainties in(1)–(2),the adaptive backstepping technique[19]and the learning ability of neural networks[3],[14]are used to design the controller. Assuming j q2(0)j<R;j q3(0)j< =2,and j q4(0)j< =2, let u1=v1cos q3=(10curv(q1)q2);u2=v2;b=1=l;g2= [0;0;0;1]T;g1=[1;(10curv(q1)q2)tan q3;0curv(q1);0]T;g3= [0;0;(10curv(q1)q2)tan q4=cos q3;0]T,(1)–(2)can be written as_q=g1u1+g2u2+bg3u1(3)M1(q)_u+C1(q;_q)u+G1(q)+U1(q;_q)=B1(q) (4) whereM1(q)=9T(q)M(q)9(q)C1(q;_q)=9T(q)(M(q)_9(q)+C(p;_p)9(q))G1(q)=9T(q)G(q)U1(q;_q)=9T(q)U(q;_q)B1(q)=diag[(10curv(q1)q2)=(r cos q3cos q4);1]9(q)=diag[(10curv(q1)q2)=cos q3;1]:Step1:Introducing~u1=u10v31if u1were the actual control input,one had~u1 0and u1 v31.Letz2=h(q2)where h(q2)is a smooth monotonic function which maps(0R;R) onto(01;+1),with thefirst derivative(with respect to q2)strictly larger than a positive real number,and such that h(0)=0,then _z2=v31L g z2+u2L g z2+bv31L g z2=v31L g z2(5) where L g z j is the Lie derivative of z j along g i.Hereafter,L means Lie derivative in this paper.Introducingz3=L g z20 3if L g z2were the actual control input,one had z3 0and L g z2 3.Let Lyapunov functionV2=12z22to make_V2=0k2z22v31we choose3=0k2z2(6) where constant k2(>0)is a design parameter.Since L g z2is not the control,z3 0.So_V2=0k2z22v31+z2z3v31:The second term z2z3v31will be cancelled at the next step.The closed-loop system(5)with(6)is_z2=0k2z2v31+z3v31(7)_z3=v31L2g z20k22z2v31+k2z3v31+bv31L g L g z2:(8) Step2:Introducingz4=L2g z20 4let Lyapunov functionV3=12z22+z23+12011(^b0b)2where constant 1(>0)is a design parameter,then_V3=0k2z22v31+z3(z4+z20k22z2+k2z3+^bLg L g z2+ 4)v31+ 011(^b0b)(_^b0 1v31z3L g L g z2): If L2g z2were the actual control input,one had z4 0.To make_V3=0k2z22v310k3z23v31we would choose_^b=14=0(k2+k3)z30(10k22)z20^bL g L g z2(9) where1= 1v31z3L g L g z2constant k3(>0)is a design parameter.Authorized licensed use limited to: SOUTH CHINA UNIVERSITY OF TECHNOLOGY. Downloaded on July 26,2010 at 12:04:06 UTC from IEEE Xplore. Restrictions apply.IEEE TRANSACTIONS ON ROBOTICS,VOL.21,NO.2,APRIL 2005263Since L 2g z 2is not the control,z 4 0and we do not use _^b = 1asan update law in the control.Then_V 3=0k 2z 22v 310k 3z 23v 31+z 3z 4v 31+ 011(^b 0b )(_^b 0 1):The third term z 3z 4v 31will be cancelled at the next step.The closed-loop system (8)with (9)is_z 3=0k 3z 3v 31+z 4v 310z 2v 31+(b 0^b )v 31L g L g z 2_z 4=v 31L 3g z 2+(k 2+k 3)z 4v 310(k 2k 3+k 2301+k 22)z 3v 310(2k 20k 32+k 3)z 2v 31+_^bL g L g z 2+^bv 31L g L g L g z 2+(k 2+k 3)(b 0^b )v 31L g L g z 2+^bbv 31L 2g L g z 2+bv 31L g L 2gz 2+^bu 2L g L g L g z 2:(10)Step 3:Introducing~u 2=u 20 5let Lyapunov functionV 4=12z 22+z 23+z 24+12011(^b 0b )2then _V4=0k 2z 22v 310k 3z 23v 31+z 4[z 3v 31+v 31L 3g z 2+(k 2+k 3)z 4v 310(k 2k 3+k 2301+k 22)z 3v 310(2k 20k 32+k 3)z 2v 31+_^bL g L g z 2+^bv 31L g L g L g z 2+^b 2v 31L 2g L g z 2+^bv 31L g L 2gz 2+^b (~u 2+ 5)L g L g L g z 2]+ 011(^b 0b )(_^b 0 1(z 3v 31L g L g z 2+z 4[(k 2+k 3)2v 31L g L g z 2+^bv 31L 2g L g z 2+v 31L g L 2g z 2])):If u 2were the actual control input,one had ~u 2 0.The update law of^b is chosen as_^b= 20 1(^b 0b 0);if ^b 2(b l ;b u );or ^b =b l ; 2 0;or ^b =b u 2 00 1(^b 0b 0);if ^b =b l ; 2<0or ^b =b u 2>0(11)where b l =1=l max and b u =1=l min ,constants 1(>0)and b 0(2(b l ;b u ))are design parameters,and 2is defined by 2= 1+1z 4v 31[(k 2+k 3)L g L g z 2+^bL 2g L g z 2+L g L 2g z 2]:The virtual control 5is chosen as5=[0k 4z 4v 310z 3v 310v 31L 3g z 20(k 2+k 3)z 4v 31+(k 2k 3+k 2301+k 22)z 3v 31+(2k 20k 32+k 3)z 2v 31+50^bv 31L g L g L g z 20^b 2v 31L 2g L g z 20^bv 31L g L 2gz 2]=(^bL g L g L g z 2)where 5=1+ 22+ 11+(^b 0b 0)2L g L g z 22tanh z41+ 22+ 11+(^b 0b 0)2L g L g z 2)= 2)constants k 4(>0)and 2(>0)are design parameters,and 5is thethird stabilizing function.Then_V4 0k 2z 22v 310k 3z 23v 310k 4z 24v 310 011 12(^b 0b )2011 12(^b 0b 0)2+ 011 12(b 0b 0)2+ 2where satisfies =e0( +1)(i.e., =0:2785)[21].Remark 2:By the relation between z and q ,boundedness of z 2;z 3,and z 4guarantees that q 22(0R;R );q 32(0 =2; =2),and q 42(0 =2; =2).The update law (11)guarantees ^b 2[b l ;b u ]all the time.Therefore, 5is well defined.Since u 2is not the control input,~u 2 0.However,we will choose(11)as the final update law of ^b in the control.Then_V 4 0k 2z 22v 310k 3z 23v 310k 4z 24v 31+z 4^bL g L g L g z 2~u20 011 12(^b 0b )20 011 12(^b 0b 0)2+ 011 12(b 0b 0)2+ 2:The closed-loop system (10)is_z 4=0k 4z 4v 310z 3v 31+~u 2^bL g L g L g z 2+L g L g z 2_^b +51+(b 0^b )[^bL 2gL g z 2+L g L 2g z 2+(k 2+k 3)L g L g z 2]v 31:Step 4:Since u 1is not the control input,~u 1 0.Let ~u =[~u 1;~u 2]Tand =[v 31; 5]T(12)thenM 1_~u =B 1 0C 1~u 0(M 1_ +C 1 +G 1+U 1):(13)In (13),M 1;C 1;G 1,and U 1are unknown.Also in B 1;r is un-known.To deal with the uncertainty of (M 1_ +C 1 +G 1+U 1),a two-layer neural network with sigmoid base functions will be usedto approximate it.Since (M 1_+C 1 +G 1+U 1)is continuous,let s (q;_q;;_ )be an m -vector of continuous sigmoidal functions,ac-cording to the approximation property of neural networks [3]M 1(q )_ +C 1(q;_q ) +G 1(q )+U 1(q;_q )=w T s (q;_q;;_ )+ (14)where w 2R m 22is an unknown optimal constant weight vector,andis defined by w :=argmin 2Rfsup (q;_q; ;_ )2k M 1(q )_ +C 1(q;_q )+G 1(q )+U 1(q;_q )0 T s (q;_q;;_ )kg where is a compact region. =[ 1; 2]T represents the networkreconstruction error corresponding to the optimal weight vector.Gen-erally,increasing the number of adjustable weights (i.e.,m )reduces the network reconstruction error.The approximation results for neural networks indicate that if m is sufficiently large,then can be made bounded on a compact region [3],[12].Given a compact region ,we assume thatk k ;8(q;_q; ;_ )2(15)where is an unknown bound.It is worth noting that the unknown bound is not unique,since any constant which is greater than satisfies (15).To avoid confusion,we define to be the smallest constant,such that (15)is satisfied.With (14),(13)can be written asM 1(q )_~u =B 1(q ) 0C 1(q;_q )~u 0w T s 0 :Defining c =1=r ,and letting ^c ;^w ,and ^be the estimates of c;w ,and ,respectively,we choose the control law=^B 011[0K p ~u +^w T s (q;u )0^tanh ~u2+3](16)Authorized licensed use limited to: SOUTH CHINA UNIVERSITY OF TECHNOLOGY. Downloaded on July 26,2010 at 12:04:06 UTC from IEEE Xplore. Restrictions apply.264IEEE TRANSACTIONS ON ROBOTICS,VOL.21,NO.2,APRIL2005where K p is a positive definite matrix,and^B1is the value ofB1corresponding to the estimate^c(i.e.,^B1=diag[^c(10curv(q1)q2)=cos q3cos q4;1]).3and the update laws of^w and^will be determined next.ThenM1_~u=0K p~u0C1~u+(^w0w)T s0^tanh~u20 +3+(B10^B1) :LetV5=12z22+z23+z24+~u T M1~u+ 011(^b0b)2+ 012tr2((^w0w)T(^w0w))+ 013(^0)2+ 014(^c0c)2 then_V5=0k2z22v310k3z23v310k4z24v31+z4L g L g z2_^b+z45+ 011(^b0b)_b0z3u1L g L g z20z4u 1^bL2g L g z2 +L g L2g z2+(k2+k3)L g L g z20~u T K p~u+tr(^w0w)T 012_^w+s~uT+0~u1tanh~u120~u1 10~u2tanh~u220~u22+ 013(^0)2_^0 3~u Ttanh~u2+~u T30k2z22+k3z23+k4z24~u1+z 4^bL g L g L g z2+L g L2g z2~u2+ 014(^c0c )_^c0 4~u1 1(10curv(q1)q2)cos q3cos q4where 1is thefirst element of .If we choose the update law^b as in(11),the update laws of^w;^, and^c are as follows:_^w=02s~u T0 1(^w0w0)(17)_^=3~u Ttanh~u20 1(^00)(18)_^c=4~u1 1(10curv(q1)q2)cos q3cos q40 1(^c0c0);if^c2(c l;c u)or^c=c l~u1 1(10curv(q1)q2)cos q3cos q4>0or^c=c u~u1 1(10curv(q1)q2)cos q3cos q4<00 1(^c0c0);if^c=c l;~u1 1(10curv(q1)q2)cos q3cos q40 or^c=c u;~u1 1(10curv(q1)q2)cos q3cos q4(19)3=k2z22+k3z23+k4z24 0z4^bL g L g L g z2where 2(>0); 3(>0); 4(>0);w0;0,and c0(2(c l;c u))are design parameters,c l=1=r max,and c u=1=r min,then_V5 0k2z22v310k3z23v310k4z24v310 011 12(^b0b)20~u T K p~u0 01221tr((^w0w)T(^w0w))0 0132 1(^0)20 01421(^c0c)2+01112(b0b0)2+ 01221tr((w0w0)T(w0w0))+01321(00)2+ 01421(c0c0)2+2 2+ 2:(20)IV.M AIN R ESULTS AND D ISCUSSIONSA.Main ResultsWith the aid of the preceding design procedure,one has the followingresult.Theorem1:With the controller(16)and the update laws of^b;^w;^,and^c defined in(11),(17),(18),and(19),respectively,if v31(t)v>0,then z i(2 i 4);~u;(^b0b);(^c0c);(^w0w),and(^0)are uniformly bounded,and exponentially converge to a smallball containing the origin.The radius of the ball can be adjusted by thedesign parameters.Proof:It can be proved that the modified projection algorithm(11)guarantees that^b2[b l;b u],therefore 5is well defined all thetime.With the update law(19),it can be proved that^c2[c l;c u].So the control law(16)is well defined.Therefore,all variables in thesystem are well defined.Differentiating V5with respect to time alongthe closed-loop system,one has(20).Therefore_V5 0 1V5+ 2(21)where 1is a positive constant which depends on the control parame-ters,and2=01112(b0b0)2+01221tr((w0w0)T(w0w0))+01321(00)2+01421(c0c0)2+2 2+ 2:SoV5(t) V5(0)0 21e0 t+ 21:z i(2 i 4);~u;^b;^c;^w,and^are uniformly bounded and expo-nentially converge to a small ball.The radius of the ball can be adjustedby the design parameters i(1 i 4); 1;b0;c0;w0;0,and 2.With the aid of the state transformation and Theorem1,one has thefollowing result.Theorem2:With the controller(16)and the update laws^b;^w;^,and^c defined in(11),(17),(18),and(19),respectively,if j q2(0)j<R;j q3(0)j= =2;j q4(0)j= =2,and v31(t) v>0,then q i(2i 4);(v10v31);(^b0b);(^c0c);(^w0w),and(^0)are uniformlybounded and converge to a small ball containing the origin.The radiusof the ball can be adjusted by the design parameters.Proof:By Theorem1,z i(2 i 4);~u;(^b0b);(^c0c);(^w0w),and(^0)are uniformly bounded and exponentially converge toa small ball.By calculation,it can be proved that q i(2 i 4)and(v10v31)are uniformly bounded and converge to a small ball.B.DiscussionsIf j q2(0)j<R,in order to make q22(0R;R)all the time,z2=h(q2)is introduced in Step1.With the condition imposed on h(q2),ifz2is bounded,q22(0R;R).Therefore,the definition of point Q isunique,and d is well defined in the control.If R<1,one choice ofh(q2)ish(q2)=2Rtan q22R:Specially,if curv(s)=0,one can choose h(q2)=q2.If j q3(0)j<=2and j q4(0)j< =2,the proposed controller will make j q3j<=2and j q4j< =2all the time.If q2(0)j R,j q3(0)j= =2,orj q4(0)j= =2,one canfirst use an open-loop control law to makethe robot move into the region where the proposed controller can beapplied,then apply the proposed controller.Unknown parameters b(=1=l)and c(=1=r)are updated by theadaptive laws(11)and(19),respectively.They guarantee that^b2[b l;b u]and^c2[c l;c u].The neural network is used to approximateAuthorized licensed use limited to: SOUTH CHINA UNIVERSITY OF TECHNOLOGY. Downloaded on July 26,2010 at 12:04:06 UTC from IEEE Xplore. Restrictions apply.IEEE TRANSACTIONS ON ROBOTICS,VOL.21,NO.2,APRIL 2005265Fig.2.Response of d.Fig.3.Response of .the unknown dynamics of the system.The weight matrix is updated bythe adaptive law (17).The reconstruction error of the neural network is estimated by the adaptive law (18).The control parameters are k i (2 i 4);K p ; i (1 i 4); 1; 2;b 0;c 0; 0,and w rge values of k i (2 i 4)and K p make q i (2 i 4)and (v 10v 31)converge quickly to the small ball.Small values of 01i 1(1 i 4)and 1make the radius of the ball small.Parameters b 0;c 0; 0,and w 0also affect the radius of the small ball.If b 0;c 0; 0,and w 0are close to b;c; ,and w ,respectively,the tracking error will be small.Therefore,in order tomake q i (2 i 4)and (v 10v 31)converge quickly to the origin,one can make k i (1 i 4);K p ; i (1 i 4)large and 1small.V .S IMULATIONIn order to verify the validity of the proposed controller,we imple-ment the controller in the simulator available in our lab.The setup of the simulator is comprised of two interconnected computers.One com-puter,the so-called System-PC,processes the video streams and calcu-lates the control commands for the robot to follow the path.These con-trol commands are then passed to the second computer,the so-called Simulator-PC,which is responsible for the robot and environment sim-ulation.In the experiment,we choose one type of wheeledmobileFig.4.Response of (v 0v ).Fig.5.Response of ^b.Fig.6.Response of ^c .robots and a rocky path to test the controller.Figs.2–4show the re-sponses of d; ,and (v 10v 31)with a group of control parameters.From the results,it is shown that d; ,and (v 10v 31)converge to zero during the control.Figs.5and 6show the responses of ^b and ^c .It is Authorized licensed use limited to: SOUTH CHINA UNIVERSITY OF TECHNOLOGY. Downloaded on July 26,2010 at 12:04:06 UTC from IEEE Xplore. Restrictions apply.266IEEE TRANSACTIONS ON ROBOTICS,VOL.21,NO.2,APRIL 2005shown that they are bounded.Especially,^b and ^c do not go throughzero.Responses of ^ and ^w also show the boundedness of ^ and ^w .The control inputs calculated from the control law are bounded and not large.They can be realized by typical mobile actuators.These exper-imental results show that the proposed controller is effective.To test the proposed controller further,we implement it in different robots and different paths.All results show that the controller is effective.VI.C ONCLUSIONIn this paper,the tracking-control problem of a nonholonomic wheeled mobile robot with parameter uncertainties and nonparameter uncertainties is considered.A robust adaptive controller is proposed.The idea in this paper can be applied to control other uncertain nonholonomic dynamics systems.R EFERENCES[1]R.W.Brockett,“Asymptotic stability and feedback stabilization,”in Dif-ferential Geometric Control Theory ,R.W.Brockett,Ed.Boston,MA:Birkhauser,1983,pp.181–208.[2] B.S.Chen et al.,“A robustH model reference tracking design fornonholonomic mechanical control systems,”Int.J.Control ,vol.63,pp.283–306,1996.[3]G.Cybenko,“Approximation by superpositions of a sigmoidal func-tion,”Math.Control Signals Syst.,vol.2,no.4,pp.303–314,1989.[4] B.d’Andrea-Novel et al.,“Control of nonholonomic wheeled mobilerobots by state feedback linearization,”Int.J.Robot.Res.,vol.14,pp.543–559,1995.[5]W.Dong and W.L.Xu,“Adaptive tracking control of uncertain non-holonomic dynamic system,”IEEE Trans.Autom.Control ,vol.43,pp.450–454,Mar.2001.[6]W.Dong et al.,“Tracking control of dynamic nonholonomic systemsand its application to mobile robots with uncertainty,”IEEE Trans.Robot.Autom.,vol.16,pp.870–874,Dec.2000.[7]W.Dong et al.,“Trajectory tracking control of dynamic nonholonomicsystems with unknown dynamics,”Int.J.Robust,Nonlinear Control ,vol.9,no.13,pp.905–922,1999.[8]W.Dong and W.Huo,“Trajectory tracking control of chained systems,”Zidonghua Xuebao/Acta Automatica Sinica ,vol.26,no.3,pp.310–316,2000.[9]R.Fierro and F.L.Lewis,“Control of a nonholonomic mobile robotusing neural networks,”IEEE Trans.Neural Netw.,vol.9,pp.589–600,Jul.1998.[10]M.Fliess et al.,“Design of trajectory stabilizing feedback for driftlessflat systems,”in Proc.Eur.Control Conf.,1995,pp.1882–1887.[11]T.Fukao,H.Nakagawa,and N.Adachi,“Adaptive tracking control ofa nonholonomic mobile robot,”IEEE Trans.Robot.Autom.,vol.16,pp.609–615,Oct.2000.[12]S.S.Ge et al.,Stable Adaptive Neural Network Control .Boston,MA:Kluwer,2001.[13]S.S.Ge et al.,“Adaptive robust stabilization of dynamic nonholonomicchained systems,”J.Robot.Syst.,vol.18,no.3,pp.119–113,2001.[14]K.Hornik et al.,“Multilayer feedforward networks are universal approx-imators,”Neural Netw.,vol.2,pp.359–366,1989.[15]Z.-P.Jiang and H.Nijmeijer,“Tracking control of mobile robots:A casestudy in backstepping,”Automatica ,vol.33,no.7,pp.1393–1399,1997.[16],“A recursive technique for tracking control of nonholonomicsystems in chained form,”IEEE Trans.Autom.Control ,vol.44,pp.256–279,Feb.1999.[17]Y .Kanayama et al.,“A stable tracking control method for an au-tonomous mobile robot,”in Proc.IEEE Conf.Robot.Autom.,1990,pp.384–389.[18]I.Kolmanovsky and N.H.McClamroch,“Development in nonholo-nomic control problems,”IEEE Control Syst.Mag.,vol.15,pp.20–36,Dec.1995.[19]M.Krstic et al.,Nonlinear and Adaptive Control Design .New York:Wiley,1995.[20]P.Lucibello and G.Oriolo,“Robust stabilization by iterative statesteering with an application to chained-form systems,”Automatica ,vol.37,no.1,pp.71–79,2001.[21]M.M.Polycarpou,“Stable adaptive neural network control scheme fornonlinear systems,”IEEE Trans.Autom.Control ,vol.41,pp.447–451,Mar.1996.[22] C.Samson,“Path following and time-varying feedback stabilization ofwheeled mobile robot,”in Proc.ICARCV ,vol.1,Singapore,1992,pp.RO-13.1.1–RO-13.1.5.[23] C.Y .Suand Y .Stepanenko,“Robu st motion/force control of mechanicalsystems with classical nonholonomic constraints,”IEEE Trans.Autom.Control ,vol.39,pp.609–614,Mar.1994.[24]M.Oya,C.Y .Su,and R.Katoh,“Robust adaptive motion/force trackingcontrol of uncertain nonholonomic mechanical systems,”IEEE Trans.Robot.Autom.,vol.19,pp.175–181,Feb.2003.[25]G.Walsh et al.,“Stabilization of trajectories for systems with nonholo-nomic constraints,”IEEE Trans.Autom.Control ,vol.39,pp.216–222,Jan.1994.A New Formulation of Visual Servoing Based on Cylindrical Coordinate SystemMasami Iwatsuki and Norimitsu OkiyamaAbstract—Image-based visual servoing is a flexible and robust technique to control a robot and guide it to a desired position only by using two-di-mensional visual data.However,it is well known that the classical visual servoing based on the Cartesian coordinate system has one crucial problem,that the camera moves backward at infinity,in case that the camera motion from the initial to desired poses is a pure rotation of 180around the op-tical axis.This paper proposes a new f ormulation of visual servoing,based on a cylindrical coordinate system that can shift the position of the origin.The proposed approach can interpret from a pure rotation around an ar-bitrary axis to the proper camera rotational motion.It is shown that this formulation contains the classical approach based on the Cartesian coor-dinate system as an extreme case with the origin located at infinity.Fur-thermore,we propose a decision method of the origin-shift parameters by estimating a rotational motion from the differences between initial and de-sired image-plane positions of feature points.Index Terms—Camera retreat problem,cylindrical coordinate system,eye-in-hand robot,visual servoing.I.I NTRODUCTIONVisual servoing is a flexible and robust control technique of robots using vision in feedback-control loops.It is classified into position-based approaches and image-based approaches [1],[2].It is known that the image-based visual servoing can derive a robot motion directly from two-dimensional (2-D)visual data [3].One of the chief advantages to the image-based visual servoing is that the positioning accuracy of the robot is less sensitive to robot and camera calibration errors and image measurement errors.In most of the image-based visual servo systems,image features are composed with a set of image points,frequently the centroids of re-gions,thanks to the robustness and implementation ease of feature ex-traction [1].Unfortunately,the conventional approach of monocularManuscript received April 5,2003;revised May 11,2004;June 19,2004;July 19,2004.This paper was recommended for publication by Associate Editor F.Chaumette and Editor S.Hutchinson upon evaluation of the reviewers’com-ments.This paper was presented in part at the IEEE/RSJ International Confer-ence on Intelligent Robots and Systems,Lausanne,Switzerland,October 2002.The authors are with the Faculty of Engineering,Hosei University,Tokyo 184-8584,Japan (e-mail:iwatsuki@k.hosei.ac.jp).Digital Object Identifier 10.1109/TRO.2004.8372421552-3098/$20.00©2005IEEEAuthorized licensed use limited to: SOUTH CHINA UNIVERSITY OF TECHNOLOGY. Downloaded on July 26,2010 at 12:04:06 UTC from IEEE Xplore. Restrictions apply.。