Computation of the E_3-term of the Adams spectral sequence
20140219_Analytical_Procedures_and_Methods_Validation_for_Drugs_and_Biologics
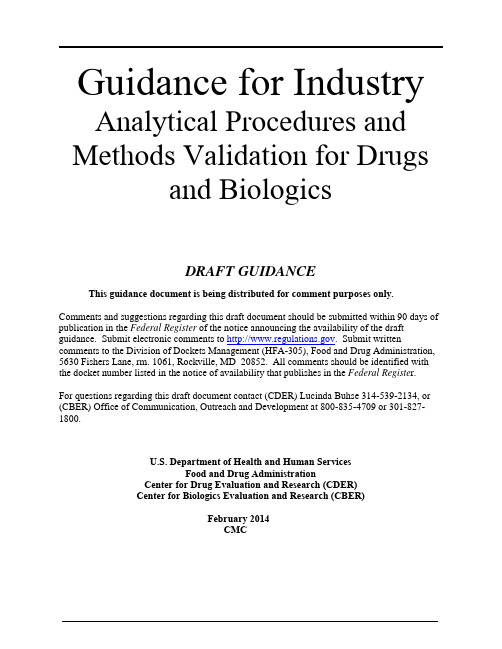
Analytical Procedures and Methods Validation for Drugsand BiologicsDRAFT GUIDANCEThis guidance document is being distributed for comment purposes only. Comments and suggestions regarding this draft document should be submitted within 90 days of publication in the Federal Register of the notice announcing the availability of the draft guidance. Submit electronic comments to . Submit written comments to the Division of Dockets Management (HFA-305), Food and Drug Administration, 5630 Fishers Lane, rm. 1061, Rockville, MD 20852. All comments should be identified with the docket number listed in the notice of availability that publishes in the Federal Registe r.For questions regarding this draft document contact (CDER) Lucinda Buhse 314-539-2134, or (CBER) Office of Communication, Outreach and Development at 800-835-4709 or 301-827-1800.U.S. Department of Health and Human ServicesFood and Drug AdministrationCenter for Drug Evaluation and Research (CDER)Center for Biologics Evaluation and Research (CBER)February 2014CMCAnalytical Procedures and Methods Validation for Drugsand BiologicsAdditional copies are available from:Office of CommunicationsDivision of Drug Information, WO51, Room 2201Center for Drug Evaluation and ResearchFood and Drug Administration10903 New Hampshire Ave., Silver Spring, MD 20993Phone: 301-796-3400; Fax: 301-847-8714druginfo@/Drugs/GuidanceComplianceRegulatoryInformation/Guidances/default.htmand/orOffice of Communication, Outreach andDevelopment, HFM-40Center for Biologics Evaluation and ResearchFood and Drug Administration1401 Rockville Pike, Rockville, MD 20852-1448ocod@/BiologicsBloodVaccines/GuidanceComplianceRegulatoryInformation/Guidances/default.htm(Tel) 800-835-4709 or 301-827-1800U.S. Department of Health and Human ServicesFood and Drug AdministrationCenter for Drug Evaluation and Research (CDER)Center for Biologics Evaluation and Research (CBER)Febr uary 2014CMCTABLE OF CONTENTSI.INTRODUCTION (1)II.BACKGROUND (2)III.ANALYTICAL METHODS DEVELOPMENT (3)IV.CONTENT OF ANALYTICAL PROCEDURES (3)A.Principle/Scope (4)B.Apparatus/Equipment (4)C.Operating Parameters (4)D.Reagents/Standards (4)E.Sample Preparation (4)F.Standards Control Solution Preparation (5)G.Procedure (5)H.System Suitability (5)I.Calculations (5)J.Data Reporting (5)V.REFERENCE STANDARDS AND MATERIALS (6)VI.ANALYTICAL METHOD VALIDATION FOR NDA, ANDAs, BLAs, AND DMFs (6)A.Noncompendial Analytical Procedures (6)B.Validation Characteristics (7)pendial Analytical Procedures (8)VII.STATISTICAL ANALYSIS AND MODELS (8)A.Statistics (8)B.Models (8)VIII.LIFE CYCLE MANAGEMENT OF ANALYTICAL PROCEDURES (9)A.Revalidation (9)B.Analytical Method Comparability Studies (10)1.Alternative Analytical Procedures (10)2.Analytical Methods Transfer Studies (11)C.Reporting Postmarketing Changes to an Approved NDA, ANDA, or BLA (11)IX.FDA METHODS VERIFICATION (12)X.REFERENCES (12)Guidance for Industry11Analytical Procedures and Methods Validation for Drugs and2Biologics345This draft guidance, when finalized, will represent the Food and Drug Administration’s (FDA’s) current 6thinking on this topic. It does not create or confer any rights for or on any person and does not operate to 7bind FDA or the public. You can use an alternative approach if the approach satisfies the requirements of 8the applicable statutes and regulations. If you want to discuss an alternative approach, contact the FDA9staff responsible for implementing this guidance. If you cannot identify the appropriate FDA staff, call 10the appropriate number listed on the title page of this guidance.11121314I. INTRODUCTION1516This revised draft guidance supersedes the 2000 draft guidance for industry on Analytical17Procedures and Methods Validation2,3 and, when finalized, will also replace the 1987 FDA18guidance for industry on Submitting Samples and Analytical Data for Methods Validation. It19provides recommendations on how you, the applicant, can submit analytical procedures4 and20methods validation data to support the documentation of the identity, strength, quality, purity,21and potency of drug substances and drug products.5It will help you assemble information and 22present data to support your analytical methodologies. The recommendations apply to drug23substances and drug products covered in new drug applications (NDAs), abbreviated new drug 24applications (ANDAs), biologics license applications (BLAs), and supplements to these25applications. The principles in this revised draft guidance also apply to drug substances and drug 26products covered in Type II drug master files (DMFs).2728This revised draft guidance complements the International Conference on Harmonisation (ICH) 29guidance Q2(R1)Validation of Analytical Procedures: Text and Methodology(Q2(R1)) for30developing and validating analytical methods.3132This revised draft guidance does not address investigational new drug application (IND) methods 33validation, but sponsors preparing INDs should consider the recommendations in this guidance.34For INDs, sufficient information is required at each phase of an investigation to ensure proper35identity, quality, purity, strength, and/or potency. The amount of information on analytical36procedures and methods validation will vary with the phase of the investigation.6 For general371 This guidance has been prepared by the Office of Pharmaceutical Science, in the Center for Drug Evaluation andResearch (CDER) and the Center for Biologics Evaluation and Research (CBER) at the Food and DrugAdministration.2 Sample submission is described in section IX, FDA Methods Verification.3 We update guidances periodically. To make sure you have the most recent version of a guidance, check the FDADrugs guidance Web page at/Drugs/GuidanceComplianceRegulatoryInformation/Guidances/default.htm.4Analytical procedure is interchangeable with a method or test procedure.5The terms drug substance and drug product, as used in this guidance, refer to human drugs and biologics.6 See 21 CFR 312.23(a)(7).guidance on analytical procedures and methods validation information to be submitted for phase 38one studies, sponsors should refer to the FDA guidance for industry on Content and Format of39Investigational New Drug Applications (INDs) for Phase 1 Studies of Drugs, Including40Well-Characterized, Therapeutic, Biotechnology-Derived Products. General considerations for 41analytical procedures and method validation (e.g., bioassay) before conduct of phase three42studies are discussed in the FDA guidance for industry on IND Meetings for Human Drugs and 43Biologics, Chemistry, Manufacturing, and Controls Information.4445This revised draft guidance does not address specific method validation recommendations for46biological and immunochemical assays for characterization and quality control of many drug47substances and drug products. For example, some bioassays are based on animal challenge48models, and immunogenicity assessments or other immunoassays have unique features that49should be considered during development and validation.5051In addition, the need for revalidation of existing analytical methods may need to be considered 52when the manufacturing process changes during the product’s life cycle. For questions on53appropriate validation approaches for analytical procedures or submission of information not54addressed in this guidance, you should consult with the appropriate FDA product quality review 55staff.5657If you choose a different approach than those recommended in this revised draft guidance, we58encourage you to discuss the matter with the appropriate FDA product quality review staff before 59you submit your application.6061FDA’s guidance documents, including this guidance, do not establish legally enforceable62responsibilities. Instead, guidances describe the Agency’s current thinking on a topic and should 63be viewed only as recommendations, unless specific regulatory or statutory requirements are64cited. The use of the word should in Agency guidances means that something is suggested or65recommended, but not required.666768II.BACKGROUND6970Each NDA and ANDA must include the analytical procedures necessary to ensure the identity, 71strength, quality, purity, and potency of the drug substance and drug product.7 Each BLA must 72include a full description of the manufacturing methods, including analytical procedures that73demonstrate the manufactured product meets prescribed standards of identity, quality, safety,74purity, and potency.8 Data must be available to establish that the analytical procedures used in 75testing meet proper standards of accuracy and reliability and are suitable for their intended76purpose.9 For BLAs and their supplements, the analytical procedures and their validation are77submitted as part of license applications or supplements and are evaluated by FDA quality78review groups.79807 See 21 CFR 314.50(d)(1) and 314.94(a)(9)(i).8 See 21 CFR 601.2(a) and 601.2(c).9 See 21 CFR 211.165(e) and 211.194(a)(2).Analytical procedures and validation data should be submitted in the corresponding sections of 81the application in the ICH M2 eCTD: Electronic Common Technical Document Specification.108283When an analytical procedure is approved/licensed as part of the NDA, ANDA, or BLA, it84becomes the FDA approved analytical procedure for the approved product. This analytical85procedure may originate from FDA recognized sources (e.g., a compendial procedure from the 86United States Pharmacopeia/National Formulary (USP/NF)) or a validated procedure you87submitted that was determined to be acceptable by FDA. To apply an analytical method to a88different product, appropriate validation studies with the matrix of the new product should be89considered.909192III.ANALYTICAL METHODS DEVELOPMENT9394An analytical procedure is developed to test a defined characteristic of the drug substance or95drug product against established acceptance criteria for that characteristic. Early in the96development of a new analytical procedure, the choice of analytical instrumentation and97methodology should be selected based on the intended purpose and scope of the analytical98method. Parameters that may be evaluated during method development are specificity, linearity, 99limits of detection (LOD) and quantitation limits (LOQ), range, accuracy, and precision.100101During early stages of method development, the robustness of methods should be evaluated102because this characteristic can help you decide which method you will submit for approval.103Analytical procedures in the early stages of development are initially developed based on a104combination of mechanistic understanding of the basic methodology and prior experience.105Experimental data from early procedures can be used to guide further development. You should 106submit development data within the method validation section if they support the validation of 107the method.108109To fully understand the effect of changes in method parameters on an analytical procedure, you 110should adopt a systematic approach for method robustness study (e.g., a design of experiments 111with method parameters). You should begin with an initial risk assessment and follow with112multivariate experiments. Such approaches allow you to understand factorial parameter effects 113on method performance. Evaluation of a method’s performance may include analyses of114samples obtained from in-process manufacturing stages to the finished product. Knowledge115gained during these studies on the sources of method variation can help you assess the method 116performance.117118119IV.CONTENT OF ANALYTICAL PROCEDURES120121You should describe analytical procedures in sufficient detail to allow a competent analyst to 122reproduce the necessary conditions and obtain results within the proposed acceptance criteria. 123You should also describe aspects of the analytical procedures that require special attention. An 124analytical procedure may be referenced from FDA recognized sources (e.g., USP/NF,12510 See sections 3.2.S.4 Control of Drug Substance, 3.2.P.4 Control of Excipients, and 3.2.P.5 Control of DrugProduct.Association of Analytical Communities (AOAC) International)11 if the referenced analytical126procedure is not modified beyond what is allowed in the published method. You should provide 127in detail the procedures from other published sources. The following is a list of essential128information you should include for an analytical procedure:129130A.Principle/Scope131132A description of the basic principles of the analytical test/technology (separation, detection, etc.); 133target analyte(s) and sample(s) type (e.g., drug substance, drug product, impurities or compounds 134in biological fluids, etc.).135136B.Apparatus/Equipment137138All required qualified equipment and components (e.g., instrument type, detector, column type, 139dimensions, and alternative column, filter type, etc.).140141C.Operating Parameters142143Qualified optimal settings and ranges (allowed adjustments) critical to the analysis (e.g., flow144rate, components temperatures, run time, detector settings, gradient, head space sampler). A145drawing with experimental configuration and integration parameters may be used, as applicable. 146147D.Reagents/Standards148149The following should be listed:150151•Grade of chemical (e.g., USP/NF, American Chemical Society, High152Performance or Pressure Liquid Chromatography, or Gas153Chromatography and preservative free).154•Source (e.g., USP reference standard or qualified in-house reference material). 155•State (e.g., dried, undried, etc.) and concentration.156•Standard potencies (purity correction factors).157•Storage controls.158•Directions for safe use (as per current Safety Data Sheet).159•Validated or useable shelf life.160161New batches of biological reagents, such as monoclonal antibodies, polyclonal antisera, or cells, 162may need extensive qualification procedures included as part of the analytical procedure.163164E.Sample Preparation165166Procedures (e.g., extraction method, dilution or concentration, desalting procedures and mixing 167by sonication, shaking or sonication time, etc.) for the preparations for individual sample tests. 168A single preparation for qualitative and replicate preparations for quantitative tests with16911 See 21 CFR 211.194(a)(2).appropriate units of concentrations for working solutions (e.g., µg/ml or mg/ml) and information 170on stability of solutions and storage conditions.171172F.Standards Control Solution Preparation173174Procedures for the preparation and use of all standard and control solutions with appropriate175units of concentration and information on stability of standards and storage conditions,176including calibration standards, internal standards, system suitability standards, etc.177178G.Procedure179180A step-by-step description of the method (e.g., equilibration times, and scan/injection sequence 181with blanks, placeboes, samples, controls, sensitivity solution (for impurity method) and182standards to maintain validity of the system suitability during the span of analysis) and allowable 183operating ranges and adjustments if applicable.184185H.System Suitability186187Confirmatory test(s) procedures and parameters to ensure that the system (equipment,188electronics, and analytical operations and controls to be analyzed) will function correctly as an 189integrated system at the time of use. The system suitability acceptance criteria applied to190standards and controls, such as peak tailing, precision and resolution acceptance criteria, may be 191required as applicable. For system suitability of chromatographic systems, refer to CDER192reviewer guidance on Validation of Chromatographic Methods and USP General Chapter <621> 193Chromatography.194195I.Calculations196197The integration method and representative calculation formulas for data analysis (standards,198controls, samples) for tests based on label claim and specification (e.g., assay, specified and199unspecified impurities and relative response factors). This includes a description of any200mathematical transformations or formulas used in data analysis, along with a scientific201justification for any correction factors used.202203J.Data Reporting204205A presentation of numeric data that is consistent with instrumental capabilities and acceptance 206criteria. The method should indicate what format to use to report results (e.g., percentage label 207claim, weight/weight, and weight/volume etc.) with the specific number of significant figures 208needed. The American Society for Testing and Materials (ASTM) E29 describes a standard209practice for using significant digits in test data to determine conformance with specifications. For 210chromatographic methods, you should include retention times (RTs) for identification with211reference standard comparison basis, relative retention times (RRTs) (known and unknown212impurities) acceptable ranges and sample results reporting criteria.213214215V.REFERENCE STANDARDS AND MATERIALS216217Primary and secondary reference standards and materials are defined and discussed in the218following ICH guidances: Q6A Specifications: Test Procedures and Acceptance Criteria for 219New Drug Substances and New Drug Products: Chemical Substances (ICH Q6A), Q6B220Specifications: Test Procedures and Acceptance Criteria for Biotechnological/Biological221Products, and Q7 Good Manufacturing Practice Guidance for Active Pharmaceutical222Ingredients. For all standards, you should ensure the suitability for use. Reference standards for 223drug substances are particularly critical in validating specificity for an identity test. You should 224strictly follow storage, usage conditions, and handling instructions for reference standards to225avoid added impurities and inaccurate analysis. For biological products, you should include226information supporting any reference standards and materials that you intend to use in the BLA 227and in subsequent annual reports for subsequent reference standard qualifications. Information 228supporting reference standards and materials include qualification test protocols, reports, and 229certificates of analysis (including stability protocols and relevant known impurity profile230information, as applicable).231232Reference standards can often be obtained from USP and may also be available through the233European Pharmacopoeia, Japanese Pharmacopoeia, World Health Organization, or National 234Institute of Standards and Technology. Reference standards for a number of biological products 235are also available from CBER. For certain biological products marketed in the U.S., reference 236standards authorized by CBER must be used before the product can be released to the market.12 237Reference materials from other sources should be characterized by procedures including routine 238and beyond routine release testing as described in ICH Q6A. You should consider orthogonal 239methods. Additional testing could include attributes to determine the suitability of the reference 240material not necessarily captured by the drug substance or product release tests (e.g., more241extensive structural identity and orthogonal techniques for purity and impurities, biological242activity).243244For biological reference standards and materials, we recommend that you follow a two-tiered 245approach when qualifying new reference standards to help prevent drift in the quality attributes 246and provide a long-term link to clinical trial material. A two-tiered approach involves a247comparison of each new working reference standard with a primary reference standard so that it 248is linked to clinical trial material and the current manufacturing process.249250251VI.ANALYTICAL METHOD VALIDATION FOR NDA, ANDAs, BLAs, AND 252DMFs253254A.Noncompendial Analytical Procedures255256Analytical method validation is the process of demonstrating that an analytical procedure is257suitable for its intended purpose. The methodology and objective of the analytical procedures 258should be clearly defined and understood before initiating validation studies. This understanding 25912 See 21 CFR 610.20.is obtained from scientifically-based method development and optimization studies. Validation 260data must be generated under an protocol approved by the sponsor following current good261manufacturing practices with the description of methodology of each characteristic test and262predetermined and justified acceptance criteria, using qualified instrumentation operated under 263current good manufacturing practices conditions.13 Protocols for both drug substance and264product analytes or mixture of analytes in respective matrices should be developed and executed. 265266ICH Q2(R1) is considered the primary reference for recommendations and definitions on267validation characteristics for analytical procedures. The FDA Reviewer Guidance: Validation of 268Chromatographic Methods is available as well.269270B.Validation Characteristics271272Although not all of the validation characteristics are applicable for all types of tests, typical273validation characteristics are:274275•Specificity276•Linearity277•Accuracy278•Precision (repeatability, intermediate precision, and reproducibility)279•Range280•Quantitation limit281•Detection limit282283If a procedure is a validated quantitative analytical procedure that can detect changes in a quality 284attribute(s) of the drug substance and drug product during storage, it is considered a stability285indicating assay. To demonstrate specificity of a stability-indicating assay, a combination of286challenges should be performed. Some challenges include the use of samples spiked with target 287analytes and all known interferences; samples that have undergone various laboratory stress288conditions; and actual product samples (produced by the final manufacturing process) that are289either aged or have been stored under accelerated temperature and humidity conditions.290291As the holder of the NDA, ANDA, or BLA, you must:14 (1) submit the data used to establish292that the analytical procedures used in testing meet proper standards of accuracy and reliability, 293and (2) notify the FDA about each change in each condition established in an approved294application beyond the variations already provided for in the application, including changes to 295analytical procedures and other established controls.296297The submitted data should include the results from the robustness evaluation of the method,298which is typically conducted during method development or as part of a planned validation299study.1530013 See 21 CFR 211.165(e); 21 CFR 314.50 (d), and for biologics see 21 CFR 601.2(a), 601.2(c), and 601.12(a).14 For drugs see 21 CFR 314.50 (d), 314.70(d), and for biologics see 21 CFR 601.2(a), 601.2(c), and 601.12(a). For aBLA, as discussed below, you must obtain prior approval from FDA before implementing a change in analyticalmethods if those methods are specified in FDA regulations15 See section III and ICH Q2(R1).pendial Analytical Procedures302303The suitability of an analytical procedure (e.g., USP/NF, the AOAC International Book of304Methods, or other recognized standard references) should be verified under actual conditions of 305use.16 Compendial general chapters, which are complex and mention multiple steps and/or306address multiple techniques, should be rationalized for the intended use and verified. Information 307to demonstrate that USP/NF analytical procedures are suitable for the drug product or drug308substance should be included in the submission and generated under a verification protocol.309310The verification protocol should include, but is not limited to: (1) compendial methodology to 311be verified with predetermined acceptance criteria, and (2) details of the methodology (e.g.,312suitability of reagent(s), equipment, component(s), chromatographic conditions, column, detector 313type(s), sensitivity of detector signal response, system suitability, sample preparation and314stability). The procedure and extent of verification should dictate which validation characteristic 315tests should be included in the protocol (e.g., specificity, LOD, LOQ, precision, accuracy, etc.). 316Considerations that may influence what characteristic tests should be in the protocol may depend 317on situations such as whether specification limits are set tighter than compendial acceptance318criteria, or RT or RRT profiles are changing in chromatographic methods because of the319synthetic route of drug substance or differences in manufacturing process or matrix of drug320product. Robustness studies of compendial assays do not need to be included, if methods are 321followed without deviations.322323324VII.STATISTICAL ANALYSIS AND MODELS325326A.Statistics327328Statistical analysis of validation data can be used to evaluate validation characteristics against 329predetermined acceptance criteria. All statistical procedures and parameters used in the analysis 330of the data should be based on sound principles and appropriate for the intended evaluation.331Reportable statistics of linear regression analysis R (correlation coefficient), R square332(coefficient of determination), slope, least square, analysis of variance (ANOVA), confidence 333intervals, etc., should be provided with justification.For information on statistical techniques 334used in making comparisons, as well as other general information on the interpretation and335treatment of analytical data, appropriate literature or texts should be consulted.17336337B.Models338339Some analytical methods might use chemometric and/or multivariate models. When developing 340these models, you should include a statistically adequate number and range of samples for model 341development and comparable samples for model validation. Suitable software should be used for 342data analysis. Model parameters should be deliberately varied to test model robustness.34334416 See 21 CFR 211.194(a)(2) and USP General Chapter <1226> Verification of Compendial Procedures.17 See References section for examples including USP <1010> Analytical Data – Interpretation and Treatment.。
机器学习专业词汇中英文对照
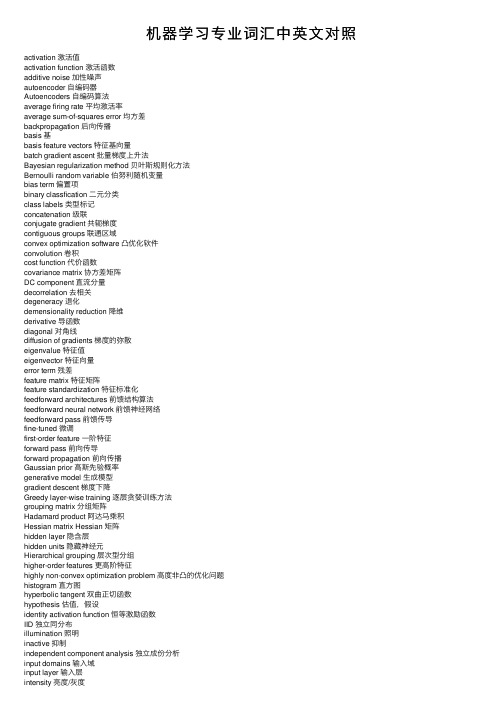
机器学习专业词汇中英⽂对照activation 激活值activation function 激活函数additive noise 加性噪声autoencoder ⾃编码器Autoencoders ⾃编码算法average firing rate 平均激活率average sum-of-squares error 均⽅差backpropagation 后向传播basis 基basis feature vectors 特征基向量batch gradient ascent 批量梯度上升法Bayesian regularization method 贝叶斯规则化⽅法Bernoulli random variable 伯努利随机变量bias term 偏置项binary classfication ⼆元分类class labels 类型标记concatenation 级联conjugate gradient 共轭梯度contiguous groups 联通区域convex optimization software 凸优化软件convolution 卷积cost function 代价函数covariance matrix 协⽅差矩阵DC component 直流分量decorrelation 去相关degeneracy 退化demensionality reduction 降维derivative 导函数diagonal 对⾓线diffusion of gradients 梯度的弥散eigenvalue 特征值eigenvector 特征向量error term 残差feature matrix 特征矩阵feature standardization 特征标准化feedforward architectures 前馈结构算法feedforward neural network 前馈神经⽹络feedforward pass 前馈传导fine-tuned 微调first-order feature ⼀阶特征forward pass 前向传导forward propagation 前向传播Gaussian prior ⾼斯先验概率generative model ⽣成模型gradient descent 梯度下降Greedy layer-wise training 逐层贪婪训练⽅法grouping matrix 分组矩阵Hadamard product 阿达马乘积Hessian matrix Hessian 矩阵hidden layer 隐含层hidden units 隐藏神经元Hierarchical grouping 层次型分组higher-order features 更⾼阶特征highly non-convex optimization problem ⾼度⾮凸的优化问题histogram 直⽅图hyperbolic tangent 双曲正切函数hypothesis 估值,假设identity activation function 恒等激励函数IID 独⽴同分布illumination 照明inactive 抑制independent component analysis 独⽴成份分析input domains 输⼊域input layer 输⼊层intensity 亮度/灰度intercept term 截距KL divergence 相对熵KL divergence KL分散度k-Means K-均值learning rate 学习速率least squares 最⼩⼆乘法linear correspondence 线性响应linear superposition 线性叠加line-search algorithm 线搜索算法local mean subtraction 局部均值消减local optima 局部最优解logistic regression 逻辑回归loss function 损失函数low-pass filtering 低通滤波magnitude 幅值MAP 极⼤后验估计maximum likelihood estimation 极⼤似然估计mean 平均值MFCC Mel 倒频系数multi-class classification 多元分类neural networks 神经⽹络neuron 神经元Newton’s method ⽜顿法non-convex function ⾮凸函数non-linear feature ⾮线性特征norm 范式norm bounded 有界范数norm constrained 范数约束normalization 归⼀化numerical roundoff errors 数值舍⼊误差numerically checking 数值检验numerically reliable 数值计算上稳定object detection 物体检测objective function ⽬标函数off-by-one error 缺位错误orthogonalization 正交化output layer 输出层overall cost function 总体代价函数over-complete basis 超完备基over-fitting 过拟合parts of objects ⽬标的部件part-whole decompostion 部分-整体分解PCA 主元分析penalty term 惩罚因⼦per-example mean subtraction 逐样本均值消减pooling 池化pretrain 预训练principal components analysis 主成份分析quadratic constraints ⼆次约束RBMs 受限Boltzman机reconstruction based models 基于重构的模型reconstruction cost 重建代价reconstruction term 重构项redundant 冗余reflection matrix 反射矩阵regularization 正则化regularization term 正则化项rescaling 缩放robust 鲁棒性run ⾏程second-order feature ⼆阶特征sigmoid activation function S型激励函数significant digits 有效数字singular value 奇异值singular vector 奇异向量smoothed L1 penalty 平滑的L1范数惩罚Smoothed topographic L1 sparsity penalty 平滑地形L1稀疏惩罚函数smoothing 平滑Softmax Regresson Softmax回归sorted in decreasing order 降序排列source features 源特征sparse autoencoder 消减归⼀化Sparsity 稀疏性sparsity parameter 稀疏性参数sparsity penalty 稀疏惩罚square function 平⽅函数squared-error ⽅差stationary 平稳性(不变性)stationary stochastic process 平稳随机过程step-size 步长值supervised learning 监督学习symmetric positive semi-definite matrix 对称半正定矩阵symmetry breaking 对称失效tanh function 双曲正切函数the average activation 平均活跃度the derivative checking method 梯度验证⽅法the empirical distribution 经验分布函数the energy function 能量函数the Lagrange dual 拉格朗⽇对偶函数the log likelihood 对数似然函数the pixel intensity value 像素灰度值the rate of convergence 收敛速度topographic cost term 拓扑代价项topographic ordered 拓扑秩序transformation 变换translation invariant 平移不变性trivial answer 平凡解under-complete basis 不完备基unrolling 组合扩展unsupervised learning ⽆监督学习variance ⽅差vecotrized implementation 向量化实现vectorization ⽮量化visual cortex 视觉⽪层weight decay 权重衰减weighted average 加权平均值whitening ⽩化zero-mean 均值为零Letter AAccumulated error backpropagation 累积误差逆传播Activation Function 激活函数Adaptive Resonance Theory/ART ⾃适应谐振理论Addictive model 加性学习Adversarial Networks 对抗⽹络Affine Layer 仿射层Affinity matrix 亲和矩阵Agent 代理 / 智能体Algorithm 算法Alpha-beta pruning α-β剪枝Anomaly detection 异常检测Approximation 近似Area Under ROC Curve/AUC Roc 曲线下⾯积Artificial General Intelligence/AGI 通⽤⼈⼯智能Artificial Intelligence/AI ⼈⼯智能Association analysis 关联分析Attention mechanism 注意⼒机制Attribute conditional independence assumption 属性条件独⽴性假设Attribute space 属性空间Attribute value 属性值Autoencoder ⾃编码器Automatic speech recognition ⾃动语⾳识别Automatic summarization ⾃动摘要Average gradient 平均梯度Average-Pooling 平均池化Letter BBackpropagation Through Time 通过时间的反向传播Backpropagation/BP 反向传播Base learner 基学习器Base learning algorithm 基学习算法Batch Normalization/BN 批量归⼀化Bayes decision rule 贝叶斯判定准则Bayes Model Averaging/BMA 贝叶斯模型平均Bayes optimal classifier 贝叶斯最优分类器Bayesian decision theory 贝叶斯决策论Bayesian network 贝叶斯⽹络Between-class scatter matrix 类间散度矩阵Bias 偏置 / 偏差Bias-variance decomposition 偏差-⽅差分解Bias-Variance Dilemma 偏差 – ⽅差困境Bi-directional Long-Short Term Memory/Bi-LSTM 双向长短期记忆Binary classification ⼆分类Binomial test ⼆项检验Bi-partition ⼆分法Boltzmann machine 玻尔兹曼机Bootstrap sampling ⾃助采样法/可重复采样/有放回采样Bootstrapping ⾃助法Break-Event Point/BEP 平衡点Letter CCalibration 校准Cascade-Correlation 级联相关Categorical attribute 离散属性Class-conditional probability 类条件概率Classification and regression tree/CART 分类与回归树Classifier 分类器Class-imbalance 类别不平衡Closed -form 闭式Cluster 簇/类/集群Cluster analysis 聚类分析Clustering 聚类Clustering ensemble 聚类集成Co-adapting 共适应Coding matrix 编码矩阵COLT 国际学习理论会议Committee-based learning 基于委员会的学习Competitive learning 竞争型学习Component learner 组件学习器Comprehensibility 可解释性Computation Cost 计算成本Computational Linguistics 计算语⾔学Computer vision 计算机视觉Concept drift 概念漂移Concept Learning System /CLS 概念学习系统Conditional entropy 条件熵Conditional mutual information 条件互信息Conditional Probability Table/CPT 条件概率表Conditional random field/CRF 条件随机场Conditional risk 条件风险Confidence 置信度Confusion matrix 混淆矩阵Connection weight 连接权Connectionism 连结主义Consistency ⼀致性/相合性Contingency table 列联表Continuous attribute 连续属性Convergence 收敛Conversational agent 会话智能体Convex quadratic programming 凸⼆次规划Convexity 凸性Convolutional neural network/CNN 卷积神经⽹络Co-occurrence 同现Correlation coefficient 相关系数Cosine similarity 余弦相似度Cost curve 成本曲线Cost Function 成本函数Cost matrix 成本矩阵Cost-sensitive 成本敏感Cross entropy 交叉熵Cross validation 交叉验证Crowdsourcing 众包Curse of dimensionality 维数灾难Cut point 截断点Cutting plane algorithm 割平⾯法Letter DData mining 数据挖掘Data set 数据集Decision Boundary 决策边界Decision stump 决策树桩Decision tree 决策树/判定树Deduction 演绎Deep Belief Network 深度信念⽹络Deep Convolutional Generative Adversarial Network/DCGAN 深度卷积⽣成对抗⽹络Deep learning 深度学习Deep neural network/DNN 深度神经⽹络Deep Q-Learning 深度 Q 学习Deep Q-Network 深度 Q ⽹络Density estimation 密度估计Density-based clustering 密度聚类Differentiable neural computer 可微分神经计算机Dimensionality reduction algorithm 降维算法Directed edge 有向边Disagreement measure 不合度量Discriminative model 判别模型Discriminator 判别器Distance measure 距离度量Distance metric learning 距离度量学习Distribution 分布Divergence 散度Diversity measure 多样性度量/差异性度量Domain adaption 领域⾃适应Downsampling 下采样D-separation (Directed separation)有向分离Dual problem 对偶问题Dummy node 哑结点Dynamic Fusion 动态融合Dynamic programming 动态规划Letter EEigenvalue decomposition 特征值分解Embedding 嵌⼊Emotional analysis 情绪分析Empirical conditional entropy 经验条件熵Empirical entropy 经验熵Empirical error 经验误差Empirical risk 经验风险End-to-End 端到端Energy-based model 基于能量的模型Ensemble learning 集成学习Ensemble pruning 集成修剪Error Correcting Output Codes/ECOC 纠错输出码Error rate 错误率Error-ambiguity decomposition 误差-分歧分解Euclidean distance 欧⽒距离Evolutionary computation 演化计算Expectation-Maximization 期望最⼤化Expected loss 期望损失Exploding Gradient Problem 梯度爆炸问题Exponential loss function 指数损失函数Extreme Learning Machine/ELM 超限学习机Letter FFactorization 因⼦分解False negative 假负类False positive 假正类False Positive Rate/FPR 假正例率Feature engineering 特征⼯程Feature selection 特征选择Feature vector 特征向量Featured Learning 特征学习Feedforward Neural Networks/FNN 前馈神经⽹络Fine-tuning 微调Flipping output 翻转法Fluctuation 震荡Forward stagewise algorithm 前向分步算法Frequentist 频率主义学派Full-rank matrix 满秩矩阵Functional neuron 功能神经元Letter GGain ratio 增益率Game theory 博弈论Gaussian kernel function ⾼斯核函数Gaussian Mixture Model ⾼斯混合模型General Problem Solving 通⽤问题求解Generalization 泛化Generalization error 泛化误差Generalization error bound 泛化误差上界Generalized Lagrange function ⼴义拉格朗⽇函数Generalized linear model ⼴义线性模型Generalized Rayleigh quotient ⼴义瑞利商Generative Adversarial Networks/GAN ⽣成对抗⽹络Generative Model ⽣成模型Generator ⽣成器Genetic Algorithm/GA 遗传算法Gibbs sampling 吉布斯采样Gini index 基尼指数Global minimum 全局最⼩Global Optimization 全局优化Gradient boosting 梯度提升Gradient Descent 梯度下降Graph theory 图论Ground-truth 真相/真实Letter HHard margin 硬间隔Hard voting 硬投票Harmonic mean 调和平均Hesse matrix 海塞矩阵Hidden dynamic model 隐动态模型Hidden layer 隐藏层Hidden Markov Model/HMM 隐马尔可夫模型Hierarchical clustering 层次聚类Hilbert space 希尔伯特空间Hinge loss function 合页损失函数Hold-out 留出法Homogeneous 同质Hybrid computing 混合计算Hyperparameter 超参数Hypothesis 假设Hypothesis test 假设验证Letter IICML 国际机器学习会议Improved iterative scaling/IIS 改进的迭代尺度法Incremental learning 增量学习Independent and identically distributed/i.i.d. 独⽴同分布Independent Component Analysis/ICA 独⽴成分分析Indicator function 指⽰函数Individual learner 个体学习器Induction 归纳Inductive bias 归纳偏好Inductive learning 归纳学习Inductive Logic Programming/ILP 归纳逻辑程序设计Information entropy 信息熵Information gain 信息增益Input layer 输⼊层Insensitive loss 不敏感损失Inter-cluster similarity 簇间相似度International Conference for Machine Learning/ICML 国际机器学习⼤会Intra-cluster similarity 簇内相似度Intrinsic value 固有值Isometric Mapping/Isomap 等度量映射Isotonic regression 等分回归Iterative Dichotomiser 迭代⼆分器Letter KKernel method 核⽅法Kernel trick 核技巧Kernelized Linear Discriminant Analysis/KLDA 核线性判别分析K-fold cross validation k 折交叉验证/k 倍交叉验证K-Means Clustering K – 均值聚类K-Nearest Neighbours Algorithm/KNN K近邻算法Knowledge base 知识库Knowledge Representation 知识表征Letter LLabel space 标记空间Lagrange duality 拉格朗⽇对偶性Lagrange multiplier 拉格朗⽇乘⼦Laplace smoothing 拉普拉斯平滑Laplacian correction 拉普拉斯修正Latent Dirichlet Allocation 隐狄利克雷分布Latent semantic analysis 潜在语义分析Latent variable 隐变量Lazy learning 懒惰学习Learner 学习器Learning by analogy 类⽐学习Learning rate 学习率Learning Vector Quantization/LVQ 学习向量量化Least squares regression tree 最⼩⼆乘回归树Leave-One-Out/LOO 留⼀法linear chain conditional random field 线性链条件随机场Linear Discriminant Analysis/LDA 线性判别分析Linear model 线性模型Linear Regression 线性回归Link function 联系函数Local Markov property 局部马尔可夫性Local minimum 局部最⼩Log likelihood 对数似然Log odds/logit 对数⼏率Logistic Regression Logistic 回归Log-likelihood 对数似然Log-linear regression 对数线性回归Long-Short Term Memory/LSTM 长短期记忆Loss function 损失函数Letter MMachine translation/MT 机器翻译Macron-P 宏查准率Macron-R 宏查全率Majority voting 绝对多数投票法Manifold assumption 流形假设Manifold learning 流形学习Margin theory 间隔理论Marginal distribution 边际分布Marginal independence 边际独⽴性Marginalization 边际化Markov Chain Monte Carlo/MCMC 马尔可夫链蒙特卡罗⽅法Markov Random Field 马尔可夫随机场Maximal clique 最⼤团Maximum Likelihood Estimation/MLE 极⼤似然估计/极⼤似然法Maximum margin 最⼤间隔Maximum weighted spanning tree 最⼤带权⽣成树Max-Pooling 最⼤池化Mean squared error 均⽅误差Meta-learner 元学习器Metric learning 度量学习Micro-P 微查准率Micro-R 微查全率Minimal Description Length/MDL 最⼩描述长度Minimax game 极⼩极⼤博弈Misclassification cost 误分类成本Mixture of experts 混合专家Momentum 动量Moral graph 道德图/端正图Multi-class classification 多分类Multi-document summarization 多⽂档摘要Multi-layer feedforward neural networks 多层前馈神经⽹络Multilayer Perceptron/MLP 多层感知器Multimodal learning 多模态学习Multiple Dimensional Scaling 多维缩放Multiple linear regression 多元线性回归Multi-response Linear Regression /MLR 多响应线性回归Mutual information 互信息Letter NNaive bayes 朴素贝叶斯Naive Bayes Classifier 朴素贝叶斯分类器Named entity recognition 命名实体识别Nash equilibrium 纳什均衡Natural language generation/NLG ⾃然语⾔⽣成Natural language processing ⾃然语⾔处理Negative class 负类Negative correlation 负相关法Negative Log Likelihood 负对数似然Neighbourhood Component Analysis/NCA 近邻成分分析Neural Machine Translation 神经机器翻译Neural Turing Machine 神经图灵机Newton method ⽜顿法NIPS 国际神经信息处理系统会议No Free Lunch Theorem/NFL 没有免费的午餐定理Noise-contrastive estimation 噪⾳对⽐估计Nominal attribute 列名属性Non-convex optimization ⾮凸优化Nonlinear model ⾮线性模型Non-metric distance ⾮度量距离Non-negative matrix factorization ⾮负矩阵分解Non-ordinal attribute ⽆序属性Non-Saturating Game ⾮饱和博弈Norm 范数Normalization 归⼀化Nuclear norm 核范数Numerical attribute 数值属性Letter OObjective function ⽬标函数Oblique decision tree 斜决策树Occam’s razor 奥卡姆剃⼑Odds ⼏率Off-Policy 离策略One shot learning ⼀次性学习One-Dependent Estimator/ODE 独依赖估计On-Policy 在策略Ordinal attribute 有序属性Out-of-bag estimate 包外估计Output layer 输出层Output smearing 输出调制法Overfitting 过拟合/过配Oversampling 过采样Letter PPaired t-test 成对 t 检验Pairwise 成对型Pairwise Markov property 成对马尔可夫性Parameter 参数Parameter estimation 参数估计Parameter tuning 调参Parse tree 解析树Particle Swarm Optimization/PSO 粒⼦群优化算法Part-of-speech tagging 词性标注Perceptron 感知机Performance measure 性能度量Plug and Play Generative Network 即插即⽤⽣成⽹络Plurality voting 相对多数投票法Polarity detection 极性检测Polynomial kernel function 多项式核函数Pooling 池化Positive class 正类Positive definite matrix 正定矩阵Post-hoc test 后续检验Post-pruning 后剪枝potential function 势函数Precision 查准率/准确率Prepruning 预剪枝Principal component analysis/PCA 主成分分析Principle of multiple explanations 多释原则Prior 先验Probability Graphical Model 概率图模型Proximal Gradient Descent/PGD 近端梯度下降Pruning 剪枝Pseudo-label 伪标记Letter QQuantized Neural Network 量⼦化神经⽹络Quantum computer 量⼦计算机Quantum Computing 量⼦计算Quasi Newton method 拟⽜顿法Letter RRadial Basis Function/RBF 径向基函数Random Forest Algorithm 随机森林算法Random walk 随机漫步Recall 查全率/召回率Receiver Operating Characteristic/ROC 受试者⼯作特征Rectified Linear Unit/ReLU 线性修正单元Recurrent Neural Network 循环神经⽹络Recursive neural network 递归神经⽹络Reference model 参考模型Regression 回归Regularization 正则化Reinforcement learning/RL 强化学习Representation learning 表征学习Representer theorem 表⽰定理reproducing kernel Hilbert space/RKHS 再⽣核希尔伯特空间Re-sampling 重采样法Rescaling 再缩放Residual Mapping 残差映射Residual Network 残差⽹络Restricted Boltzmann Machine/RBM 受限玻尔兹曼机Restricted Isometry Property/RIP 限定等距性Re-weighting 重赋权法Robustness 稳健性/鲁棒性Root node 根结点Rule Engine 规则引擎Rule learning 规则学习Letter SSaddle point 鞍点Sample space 样本空间Sampling 采样Score function 评分函数Self-Driving ⾃动驾驶Self-Organizing Map/SOM ⾃组织映射Semi-naive Bayes classifiers 半朴素贝叶斯分类器Semi-Supervised Learning 半监督学习semi-Supervised Support Vector Machine 半监督⽀持向量机Sentiment analysis 情感分析Separating hyperplane 分离超平⾯Sigmoid function Sigmoid 函数Similarity measure 相似度度量Simulated annealing 模拟退⽕Simultaneous localization and mapping 同步定位与地图构建Singular Value Decomposition 奇异值分解Slack variables 松弛变量Smoothing 平滑Soft margin 软间隔Soft margin maximization 软间隔最⼤化Soft voting 软投票Sparse representation 稀疏表征Sparsity 稀疏性Specialization 特化Spectral Clustering 谱聚类Speech Recognition 语⾳识别Splitting variable 切分变量Squashing function 挤压函数Stability-plasticity dilemma 可塑性-稳定性困境Statistical learning 统计学习Status feature function 状态特征函Stochastic gradient descent 随机梯度下降Stratified sampling 分层采样Structural risk 结构风险Structural risk minimization/SRM 结构风险最⼩化Subspace ⼦空间Supervised learning 监督学习/有导师学习support vector expansion ⽀持向量展式Support Vector Machine/SVM ⽀持向量机Surrogat loss 替代损失Surrogate function 替代函数Symbolic learning 符号学习Symbolism 符号主义Synset 同义词集Letter TT-Distribution Stochastic Neighbour Embedding/t-SNE T – 分布随机近邻嵌⼊Tensor 张量Tensor Processing Units/TPU 张量处理单元The least square method 最⼩⼆乘法Threshold 阈值Threshold logic unit 阈值逻辑单元Threshold-moving 阈值移动Time Step 时间步骤Tokenization 标记化Training error 训练误差Training instance 训练⽰例/训练例Transductive learning 直推学习Transfer learning 迁移学习Treebank 树库Tria-by-error 试错法True negative 真负类True positive 真正类True Positive Rate/TPR 真正例率Turing Machine 图灵机Twice-learning ⼆次学习Letter UUnderfitting ⽋拟合/⽋配Undersampling ⽋采样Understandability 可理解性Unequal cost ⾮均等代价Unit-step function 单位阶跃函数Univariate decision tree 单变量决策树Unsupervised learning ⽆监督学习/⽆导师学习Unsupervised layer-wise training ⽆监督逐层训练Upsampling 上采样Letter VVanishing Gradient Problem 梯度消失问题Variational inference 变分推断VC Theory VC维理论Version space 版本空间Viterbi algorithm 维特⽐算法Von Neumann architecture 冯 · 诺伊曼架构Letter WWasserstein GAN/WGAN Wasserstein⽣成对抗⽹络Weak learner 弱学习器Weight 权重Weight sharing 权共享Weighted voting 加权投票法Within-class scatter matrix 类内散度矩阵Word embedding 词嵌⼊Word sense disambiguation 词义消歧Letter ZZero-data learning 零数据学习Zero-shot learning 零次学习Aapproximations近似值arbitrary随意的affine仿射的arbitrary任意的amino acid氨基酸amenable经得起检验的axiom公理,原则abstract提取architecture架构,体系结构;建造业absolute绝对的arsenal军⽕库assignment分配algebra线性代数asymptotically⽆症状的appropriate恰当的Bbias偏差brevity简短,简洁;短暂broader⼴泛briefly简短的batch批量Cconvergence 收敛,集中到⼀点convex凸的contours轮廓constraint约束constant常理commercial商务的complementarity补充coordinate ascent同等级上升clipping剪下物;剪报;修剪component分量;部件continuous连续的covariance协⽅差canonical正规的,正则的concave⾮凸的corresponds相符合;相当;通信corollary推论concrete具体的事物,实在的东西cross validation交叉验证correlation相互关系convention约定cluster⼀簇centroids 质⼼,形⼼converge收敛computationally计算(机)的calculus计算Dderive获得,取得dual⼆元的duality⼆元性;⼆象性;对偶性derivation求导;得到;起源denote预⽰,表⽰,是…的标志;意味着,[逻]指称divergence 散度;发散性dimension尺度,规格;维数dot⼩圆点distortion变形density概率密度函数discrete离散的discriminative有识别能⼒的diagonal对⾓dispersion分散,散开determinant决定因素disjoint不相交的Eencounter遇到ellipses椭圆equality等式extra额外的empirical经验;观察ennmerate例举,计数exceed超过,越出expectation期望efficient⽣效的endow赋予explicitly清楚的exponential family指数家族equivalently等价的Ffeasible可⾏的forary初次尝试finite有限的,限定的forgo摒弃,放弃fliter过滤frequentist最常发⽣的forward search前向式搜索formalize使定形Ggeneralized归纳的generalization概括,归纳;普遍化;判断(根据不⾜)guarantee保证;抵押品generate形成,产⽣geometric margins⼏何边界gap裂⼝generative⽣产的;有⽣产⼒的Hheuristic启发式的;启发法;启发程序hone怀恋;磨hyperplane超平⾯Linitial最初的implement执⾏intuitive凭直觉获知的incremental增加的intercept截距intuitious直觉instantiation例⼦indicator指⽰物,指⽰器interative重复的,迭代的integral积分identical相等的;完全相同的indicate表⽰,指出invariance不变性,恒定性impose把…强加于intermediate中间的interpretation解释,翻译Jjoint distribution联合概率Llieu替代logarithmic对数的,⽤对数表⽰的latent潜在的Leave-one-out cross validation留⼀法交叉验证Mmagnitude巨⼤mapping绘图,制图;映射matrix矩阵mutual相互的,共同的monotonically单调的minor较⼩的,次要的multinomial多项的multi-class classification⼆分类问题Nnasty讨厌的notation标志,注释naïve朴素的Oobtain得到oscillate摆动optimization problem最优化问题objective function⽬标函数optimal最理想的orthogonal(⽮量,矩阵等)正交的orientation⽅向ordinary普通的occasionally偶然的Ppartial derivative偏导数property性质proportional成⽐例的primal原始的,最初的permit允许pseudocode伪代码permissible可允许的polynomial多项式preliminary预备precision精度perturbation 不安,扰乱poist假定,设想positive semi-definite半正定的parentheses圆括号posterior probability后验概率plementarity补充pictorially图像的parameterize确定…的参数poisson distribution柏松分布pertinent相关的Qquadratic⼆次的quantity量,数量;分量query疑问的Rregularization使系统化;调整reoptimize重新优化restrict限制;限定;约束reminiscent回忆往事的;提醒的;使⼈联想…的(of)remark注意random variable随机变量respect考虑respectively各⾃的;分别的redundant过多的;冗余的Ssusceptible敏感的stochastic可能的;随机的symmetric对称的sophisticated复杂的spurious假的;伪造的subtract减去;减法器simultaneously同时发⽣地;同步地suffice满⾜scarce稀有的,难得的split分解,分离subset⼦集statistic统计量successive iteratious连续的迭代scale标度sort of有⼏分的squares平⽅Ttrajectory轨迹temporarily暂时的terminology专⽤名词tolerance容忍;公差thumb翻阅threshold阈,临界theorem定理tangent正弦Uunit-length vector单位向量Vvalid有效的,正确的variance⽅差variable变量;变元vocabulary词汇valued经估价的;宝贵的Wwrapper包装分类:。
glossaryofterms

Administrative Services Planning Council (ASPC): The shared governance council for the Finance and Administrative Services Division.Americans with Disabilities Act (ADA): Federal regulation that insures that all facilities are constructed with equal access for persons with disabilities.Annual Implementation Plan (AIP): Annual review process for the goals and objectives as defined within a Strategic Plan time period.Apportionment: Federal, state or local monies distributed to college districts or other governmental units according to certain formulas.Assigned Square Feet (ASP): A term used to identify facility footage assigned to classrooms, labs, and non-instructional spaces.Association of Physical Plant Administrators (APPA): This is an international group that deals with the maintenance and operational standards for all levels of colleges and universities. Budgeting and Accounting Manual (BAM): The BAM has the authority of regulation in accordance with Title 5 Section 59011 of the California Code of Regulations (CCR), is distributed as part of the Board of Governors' responsibility to define, establish, and maintain the budgeting and accounting structure and procedures for the California Community Colleges as defined in California Education Code (EC) Section 70901.California Community College Chancellor's Office (CCCCO): The CCCCO is currently comprised of 72 districts, 109 campuses, 64 approved educational centers, and 20 separately reported district offices. These assets include 58.4 million gross square feet of space housed in 4,629 buildings atop more than 20,489 acres of land. Website: California Environmental Quality Act (CEQA): This is a document that provides statutes and guidelines for construction projects in the State of California.California Field Act (Field Act): A set of construction standards for school buildings to be earthquake resistive.Capacity Load Ratio (CAP Load Ratio): The ratio is a calculation based upon the number of Weekly Student Contact hours and the amount of instructional space based upon ASF. The ratio indicates how well the district utilizes its space and is used to determine eligibility for new space. Capital Outlay Budget Change Proposal (COBCP): This document is prepared by the Chancellor's Office staff for submittal to the Department of Finance (DOF) for inclusion in the Governor's spending plan that funds State construction projects.Capital Outlay: The acquisition of or additions to fixed assets, including land or existing buildings, improvements of grounds, construction of buildings, additions to buildings, remodeling of buildings, or equipment.Capital Projects: Funds that are used for the acquisition or construction of capital outlay items, e.g. buildings, major equipment.Certificates of Participation (COPs): Are used to finance the lease/purchase of capital projects. Essentially, they are the issuance of shares in the lease for a specified term.Change Order (CO): A term used to describe additional work or duties required during a construction project.Change Order Request (COR): A term used to describe a request by the contractor for a change order.Community College Facilities Coalition (CCFC): Was formed to provide advocacy for facilities’ issues and critical information and services to California's community college districts and their business partners on facility matters. Website: Community College League of California (CCLC): Is a nonprofit public benefit corporation whose voluntary membership consists of the 72 local community college districts in California. Website: Design Development Package (DD Package): This is a term used to identity the first phase in the development of construction plans and specifications.Division of the State Architect (DSA): This is the state organization that approves the plans and specifications for community college construction projects.Education Code: The body of law that regulates education in California. Other laws that affect colleges are found in the Government Code, Public Contracts Code, Penal Code and others.Encumbered Funds: Obligations in the form of purchase orders, contracts, salaries, and other commitments for which part of an appropriation is reserved.Environmental Impact Report (EIR): A document that is prepared to address any environmental impacts associated with construction project(s).Escondido Center (EC or EC CNTR): Palomar College's Education Center. (Note: The District has outreach sites in addition to this center.)Expenditures: Amounts actually dispersed for the expenses associated with operations of a fund. (Note: Accounts kept on an accrual basis include all charges whether paid or not. Accounts kept on a cash basis include only actual cash disbursements.)Facilities Condition Index (FCI): A rating used to determine the condition of a building or area. This standard has been adopted by the Building Owners & Managers Association (BOMA), the Council on Educational Facilities, and the American University Planners Association.Facilities Review Committee (FRC): A district shared governance committee that reviews and comments on requests for changes in the District's buildings and grounds which reports to ASPC. Final Project Proposal (FPP): Term used to describe the final proposal that is sent to the State for approval and funding for state capital outlay projects. (Note: The State must approve the IPP before an FPP can be submitted.)Financial and Compliance Audit: An examination leading to the expression of an opinion on (1) the fairness of presentation of the audited entity's basic financial statements in conformity with GAAP, and (2) the audited entity's compliance with the various finance-related legal and contractual provisions used to assure acceptable governmental organizational performance and effective management stewardship. Public sector oversight bodies typically require independent auditors to include responses to standardized legal compliance audit questionnaires in financial and compliance audit reports.Fiscal Year: Twelve calendar months; for governmental agencies in California, it is the period beginning July 1 and ending June 30. Some special projects use a fiscal year beginning in October 1 and ending September 30, which is consistent with the federal government's fiscal year.Foundation for California Community Colleges (FCCC): Assists community colleges and other partner schools to operate more effectively and efficiently through donations, grants, programs and services. FCCC is the sole auxiliary for the CCC Board of Governors, Chancellor and Systems Office. Website: Full-Time Equivalent Students (FTES): A measurement used to convert part-time and full-time student headcount into a full-time load equivalent. An FTES represents 525 class (contact) hours of student instruction/activity in credit and noncredit courses. FTES is one of the workload measures used in the computation of state aid for California Community Colleges.Fusion: The integrated web-based software system that all community colleges use to manage space, capital construction plans, facilities condition assessments. All project proposals for State funded projects are submitted through FUSION.General Fund: The fund used to account for the ordinary operations of the district. It is available for any legally authorized purpose not specified for payment by other funds.General Obligation Bond, Proposition 39 (GO39): An initiative that reduced the percent of voters required for passage of local bonds for K-12 and community colleges to 55% of electorate. (Note: GO39 bond propositions may only be presented to electorate in even year elections.)Generally Accepted Accounting Principles (GAAP) & Generally Accepted Accounting Standards (GAAS): Uniform minimum standards and guidelines for financial accounting and reporting.Governing Board Resolution (GBR): Resolution passed by Palomar College's Governing Board.Governmental Accounting Standards Board (GASB): The authoritative accounting and financial reporting standard-setting body for governmental entities.Gross Square Feet (GSF): A term used to identify the total facility space in a building. Heating, Ventilation and Air Conditioning (HVAC)Human Resources Planning Council (HRPC): The shared governance council for Human Resources.Independent Citizens Oversight Committee (ICOC)Indoor Air Quality (IAQ)Initial Project Proposal (IPP): Term used to describe the initial proposal sent to the State for approval and funding for state capital outlay projects.Instructional Services Planning Council (IPC): The shared governance council for the Instruction Division.Intermediate Distribution Frame (IDF): This identifies a cable rack in a room that interconnects and manages the telecommunications wiring between itself and any number of IDFs and manages the telecommunications wiring between a MDF and the workstations.JCAF 31 Analysis of Building Space Use & WSCH: Required form for State funded projects. JCAF 32 Cost Estimate Summary and Project Time Schedule: Required form for State funded projects.JCAF 33 Guideline based Group 2 Equipment Cost Estimate: Required form for State Funded projects.Leadership in Energy and Environmental Design (LEED): A "green building" rating system developed and administered by the US Green Building Council, Washington D.C.Lease Revenue Bonds: Bonds secured by a lease agreement and rental payments. Community colleges use lease revenue bonds to finance construction or purchase of facilities.Main Distribution Frame (MDF): This identifies a cable rack in a room that interconnects and manages the telecommunications wiring between itself and any number of IDFs.Palomar Community College District (PCCD)Palomar Community College District Master Plan 2022 (MP2022)PeopleSoft: The integrated database system used by Palomar College.Preliminary Plans Package (PP Package): A term used to identify the early phase in the development of construction plans and specifications.Preventive Maintenance (PM): Program that provides preventive maintenance services to every building and grounds area owned by the District.Resource Allocation Committee (RAC): Palomar College's budget committee which reports to SPC. (Note: The name has been changed effective December 2007 to Budget Committee.)San Diego Association of Governments (SANDAG): The eighteen cities and San Diego county government serve as a forum for regional decision-making. SANDAG builds consensus, makes strategic plans, obtains and allocates resources, plans, engineers, and builds public transportation, and provides information on a broad range of topics pertinent to the region's quality of life.Scheduled Maintenance: Major repairs of buildings and equipment. Matching state funds are available to districts to establish a scheduled maintenance program as approved in the State's Annual Budget Act.Solar Panels (SOLAR): A term is used to describe solar panels that are used in the heating of hot water systems.Solar Photo Voltaic (SOLAR PV): A type of solar system that converts the sun's energy into electrical energy.State Administrative Manual (SAM)State Apportionment: An allocation of state money to a district, determined by multiplying the district's total FTES times its base revenue per FTES.State Scheduled Maintenance (SSM): Program that provides a 50-50 match for specific scheduled maintenance projects. The District is required to submit a 5-year Scheduled Maintenance Plan to the State annually.Strategic Planning Council (SPC): Palomar College's primary shared governance council. Reporting to SPC are IPC, SSPC, ASPC and HRPC, which represent the four primary divisions of the college.Student Services Planning Council (SSPC); The shared governance council for the Student Services Division.Title 5 California Code of Regulations: The section of the California Administrative Code that regulates community colleges. The Board of Governors adopts Title 5 regulations.Total Quality Management (TQM)Value Engineering (VE)Voice Over Internet Protocol (VOiP): Telephone system that uses the internet and computer for communications.Weekly Student Contact Hours (WSCH): The number of hours a student has face-to-face contact with an instructor.Working Drawing Package (WD Package): A term used to identify the later phase in the development of construction plans and specifications.。
计算机专业英语多选题

1.A user interface we said here is __ABC________A.a text-based user interface or GUIB.an interface between a computer and its peripheral deviceC.an interaction between an operating system and a userD.an interaction between an application program and a user2.___A___provides transparent transfer of data between end users, providing reliable data transfer services to the upper layers.A.The Transport LayerB. Session LayerC. Network LayerE.Application Layer E. Presentation Layer3.Many viruses do harmful things such as (ABCD ).A.deleting filesB. slowing your PC downB.simulating typos D. changing random data on your disk4.We can classify programming languages under two types:(AB ) languages and ( )languages.A.high-levelB. low-levelC. advanced-levelD. basic-level5.With an Internet connection you can get some of the basic services available are:___ABCD_______A.E-mailB. TelnetC. FTPD. Usenet news6. A general purpose computer has four main sections: ( ABCE).A.the control unitB. the memoryC. the input and output devicesD. the cpuE. the arithmetic and logic unit (ALU),7.Windows 2000 has the key technologies, they are (ABCD ).A.securityB. active directoryC. flat directoryD. enterprise management8.The register file is___ACD_______A.addressed by much shorter addressesB. physically largeC.physically smallD. one the same chip as the CPU9. A stack protocol can be used for (A ).A.removing the latest element ins( )ertedB. removing the earliest element ins( )ertedC. subroutine callsD. operation of arithmetic expressions10.The end equipment in a communication system includes (ABCD ).A.printersB. computersC. CRTsD. keyboards11.Microsoft Office Professional 2000 include____ABCD______.A.Excel 2000B. PowerPoint 2000C. Word 2000D. Outlook 200012. A general purpose computer has four main sections: ______ABCE______A.the input and output devicesB. the memoryC. the arithmetic and logic unit (ALU),D. the cpuE. the control unit13.The two most common types of scanners are (BC ) and ( )A. hand-held scannersB. flatbed scannersC. auto scannersD. handler scanners14.Some viruses use (CD ) and ( ) techniques to hide their existence.A.quickly spreadB. replace a part of system softwareC. stealthD. polymorphic15.The Windows 2000 product line includes____ABCD______.A.Windows 2000 Datacenter ServerB. Windows 2000 ProfessionalC. Windows 2000 ServerD. Windows 2000 Advanced Server16.Similar to viruses, you can also find malicious code in (ABC ).A.Trojan HorsesB. logic bombsC. wormsD. Microsoft Word Documents17.Viruses all have two phases to their execution, the ( ) and the ( BD).A.create phaseB. attack phaseC. del( )ete phaseD. infection phase18.Active Directory can help you (ACD ).A.get off the limits of down level networksB. deliver complete enterprise security by itselfC. build a complex international networkD. manage every resource with a single logon19.High-level languages are commonly classified as (ACDE ).A.object-orientedB. automaticC. functionalD. logic languagesE. procedure-oriented20.(CD )is a type of executable file .A.TXT fileB. JPG fileC. EXE fileD. COM file21.( ABCD) maybe a certain cause that some viruses infect upon.A.an external event on your PCB. a dayC. a counter within the virusD. a time22.(BC )is a type of executable file .A.TXT fileB. EXE fileC. COM fileD. JPG file23.The web browsers which is normal used, such as(ABCE ).A.FirefoxB. Internet ExplorerC. OpraD. ICQE. Apple Safari24.Newer ideas in computing such as(ABDE ) have radically altered the traditional concepts that once determined program form and functionA.artificial intelligenceB. distributed computingC. software engineeringD. parallel computingE. data mining25.Microsoft Windows currently supports __AC___and _____file systemsA.NTFSB. OCFSC. FATD. ext2E. NILFS26.Modem is ____ACD______.A.a modulator/demodulatorB. a data setC. a demodulatorD. a modulator27.The equipment _AB_____.A.transfers the number of bits in serial formB.manipulates digital information internally in word unitsC.transfers the number of bits in parallelD. manipulates digital information internally in serial form28.Electronic commerce that is conducted between businesses is referred to as business-to-business or DA. C2CB. C2BC. e-commerceD. B2B29.The World Wide Web also subsumes previous Internet information systems such as (AC ).A.GopherB. FtpC. FTPD. Telnet relies on the services of .NET data providers.There are ABCDA.ConnectionB. Data AdapterC. DataReaderD. Command31.The development process in the software life cycle involves four phases: analysis, design, implementation, and ___ACDE_____.A.analysisB. auditC. implementationD. designE. testing32.The end equipment in a communication system includes __ABCD____.A.printersB. CRTsC. computersD. keyboards33.In electronic commerce ,information search and discovery services include (ABCDE ).A.search enginesB. information filtersC. software agentsD. directoriesE. electronic catalogs34.GIS work with two fundamentally different types of geographic models.They are the (BD ).A.geography modelB. vector modelC. mathematic modelD. raster modelE. data model35.The two most common types of scanners are ____AC___and _____A.flatbed scannersB. hand-held scannersC. auto scannersD. handler scanners36.Windows 2000 has the key technologies, they are ( ABCD).A.active directoryB. flat directoryC. enterprise managementD. securityputer software, or just software is a general term used to describe a collection of computer programs, procedures and documentation that perform some tasks on a computer system.The term includes: ABCA.Firmware which is software programmed resident to electrically programmable memorydevices on board mainboards or other types of integrated hardware carriers.B.Application software such as word processors which perform productive tasks for users.C.Middleware which controls and co-ordinates distributed systems.38.Software engineering is related to the disciplines of ___ADE_____A.project managementB. natural languageC. netural networkD. systems engineeringE. computer science39.What make it is difficult to agree on how to count viruses? ABCDA.some viruses can create different versions when they infect other programsB.just a trivial change may create a new virusC.some viruses can use polymorphic techniqueD.new virus arise from an existing virus40. A virus is a program that reproduces its own code by (ABC ).A.ins_erting into the middle of a fileB. simply placing a pointerC. adding to the end of a fileD. replacing another program41.Input devices include: ____ABCD______.A.the keyboardB. microphoneC. touch screenD. the mouse42.Viruses all have two phases to their execution, the ( ) and the ( AD).A.attack phaseB. create phaseC. del_ete phaseD. infection phase43.The equipment (BC ).A.manipulates digital information internally in serial formB.manipulates digital information internally in word unitsC.transfers the number of bits in serial formD.transfers the number of bits in parallel44.Office automation is___CD_______.A.. the computerB. communications technologyC. the application of computerD. used to improve the productivity of people45.The types (classes, structs, enums, and so on) associated with each .NET data provider are located in their own namespaces are: ABCDEA.System.Data.SqlClient. Contains the SQL Server .NET Data Provider types.B.System.Data.Odbc. Contains the ODBC .NET Data Provider types.C.System.Data. Contains provider-independent types such as the DataSet and DataTable.D.System.Data.OracleClient. Contains the Oracle .NET Data ProviderE.System.Data.OleDb. Contains the OLE DB .NET Data Provider types.46.C++ is __ACD________.A.extended from CB. a superset of CC. object-orientedD. procedure-oriented47.Some viruses, with no attack phase, often damage the programs or disks they infect because they (AD ).A.have bugs in themB. show messages on your screenC. steal storageD. contain poor quality code48.Windows 2000 is ( A).A.an inventive technologyB. used for building a scalable networkB.the same as Windows NT D. new lease of Windows49.Some common applications related to electronic commerce are the following:A.TeleconferencingB. Online bankingC. EmailD. Instant messagingE. Enterprise content managementF. NewsgroupsG. Shopping cart software50. A program is a sequence of ( ) that can be executed by a computer.It can either be built into the hardware or exist independently in the form of (BC ).A.hardwareB. softwareC. instructionsD. data51.Electronic payments include ___ABCD______.A.credit card paymentsB. electronic checksC. digital currenciesD. cash payment52.The web browsers which is normal used, such as____ABCD_____.A.OpraB. Internet ExplorerC. FirefoxD. Apple SafariE. ICQputer programs fall into two major classes: __AC____and ____.A.application programsB. application suiteC. operating systemsD. database application54.Database connection ( B) allows an application to reuse an existing connection from a pool instead of repeatedly establishing a new connection with the database.A.pondB. poolingC. linkD. connection55.The development process in the software life cycle involves four phases: analysis, design, implementation, and (ABCE ).A.implementationB. designC. analysisD. auditE. testing56.Hypermedia include ( ABCDEF)A.video clipsB. imagesC. textD. flashE. vidoeF. sounds57.An asleep state is_____ABD_____ed to lessen wear-and tear on the computerB. used for saving energyC. indicated by the indicator light putting outD. a low power standby mode58.Electronic payments include (ABCD ).A.digital currenciesB. electronic checksC. credit card paymentsD. cash payment59.You want to (BCD ) maybe wake up a virus that has residented in memory.A.del( )ete a fileB. access a disketteC. execute a programD. copy a file60.Before you turn the power on with a new computer, you should make sure_____ABCD_____A.the computer system has been set upB. the computer is already out of the boxB.appropriate software have been installed D. appropriate cables are correctly connected61.Security is usually enforced through ___ABE________.A.access controlB. encryptionC. data retrievingD. data storingE. auditingputer programming is the process of ABCD__the source code of computer programs.A. testingB. maintainingC. debuggingD. writing63.Queues that occur everyday life can be seen ( ABCD).A.as automobiles waiting for a traffic lightB. as people waiting for service at a bankC. in certain societies lacking equalityD. in an emergency room of a hospital64.Static graphics include____AB______.A.animatorsB. photographsC. moviesD. pictures66.which is the threat to computer security in the following choices ? ABCDA. Computer CriminalsB. Human ErrorsC. Computer CrimeD. earthquake65.The attributes of the stack are______A____.A.queueB. FIFOC. built into their circuitryD. LIFO66.If a virus simply reproduce and have no cause for an attack phase, but it will still ( ) without your permission. BDA.play musicB. stealing storageC. del( )ete filesD. pilfer CPU cycles67.According to the text,modern digital computers can be divided into four major categories on the basis of cost and performance.They are _______ABDE___________. A.minicomputers B. mainframes C. note book D. workstation E. microcomputers F. lenovo68.The Application layer in TCP/IP Model is correspond to (ABD ) in OSI ModelA.Presentation LayerB. Session LayerC. Transport LayerD. Application LayerE. Network Layer69. A computer system user generally more cares for___ABD_______A.speed of computationB. storage sizeC. physical size of the computerD. efficiency of the computer71.Cache is____ABC______A. slowB. high costC. fastD. relatively small72.We can say a bus is simply_____ABC_____ .A. a wireB. a 16-bit busC. a group of wiresD. a 8-bit bus73.Viruses can delay their attack for (ABCD ).A.yearsB. monthsC. weeksD. days74.In order to increase our computer’s performance we need to____BCD______A.buy a L1 cacheB. have a much larger main memoryC. have a L2 cacheD. buy a L2 cache75.The software that controls the interaction between the input and output hardware is called BIOS,which stands of __A________A.Basic Input Output SystemB. Classic Input Output SystemB.Advanced Input Output System D. Junior Input Output System76.To enhance performance of a computer system we should____ACD______A.improve the pattern of referencing operandB. optimize the simple movement of dataC. optimize the basic sequence control mechanismD. use IF and LOOP instructions as many as possible77.Their company used international lawyers to prosecute a crime ring involving software ____A____in Thailand.A.piracyB. copyingC. duplicationD. cloning78.The software that controls the interaction between the input and output hardware is called BIOS,which stands of (B)A.Advanced Input Output SystemB. Basic Input Output SystemC. Classic Input Output SystemD. Junior Input Output System79.Some viruses use ( AC) and ( ) techniques to hide their existence.A.stealthB. quickly spreadC. polymorphicD. replace a part of system software80.Middleware lies in______ACD____A.the middle of interactions between different application programsB.the top of the layering vertical stackC.the top of an operating systemD.the middle of the layering vertical stack81.Software includes ( ACDE) etcA.video gamesB. all kinds of filesC. programsD. websitesE. mobile application82.The major functional components of an office automation system include: ___ABCD__A.electronic mailB. personal assistance featuresC. information storage and retrievalD. text processing83.The Internet carries various information resources and services, such as (ACDEF ) and the inter-linked hypertext documentsA.online chatB. talkingC. electronic mailD. file transferE. online gamingF. file sharing84. A processor is composed of:____ABCD______.A.an arithmeticB. a control unitC. RegistersD. logic unit85.Functions of the compiler used in RISC are ___ABC_______A.to optimize register usageB.to maximize register usageC.to allocate registers to those variables that will be used the most in a given time periodD.to compile a high level language program86. A digital computer is generally made up of five dstinct elements: a central processing unit,(ABCD).A.a busB. input devicesC. memeory storage devicesD. output devicesE. crt screen87.There are AB (CD)_between the DTEs.A.digital-to-analog converterB. the modemC. communications equipmentD. will be replaced by an upd_ated standard88.What make it is difficult to agree on how to count viruses? ABCDA.just a trivial change may create a new virusB.some viruses can use polymorphic techniqueC.some viruses can create different versions when they infect other programsD.new virus arise from an existing virus89.which aspect have to be considered in the design of a piece of software. ABCDEFGA.Fault-toleranceB. ExtensibilityC. ModularityD. CompatibilityE.MarketabilityF. PackagingG. Maintainability90.Active Directory can help you (ACD ).A.build a complex international networkB. deliver complete enterprise security by itselfC.manage every resource with a single logonD. get off the limits of down level networks91.Early computer solved_____CD_____ problems.A.controlB. engineeringC. mathematicalD. business applications92.The tools which Programming software usually provides include: ABCDEA.debuggersB. text editorsC. linkersD. compilersE. interpreters93.DTE is ( AB).A.data terminal equipmentB.the last piece of equipment that belonged to the subscriber in a data link systemC.satelliteD. Digital T-carrier94.According to the text,modern digital computers can be divided into four major categories on the basis of cost and performance.They are ( BDEF).A.note bookB. microcomputersC. lenovoD. minicomputersE. workstationF. mainframes95.which is the type of electronic commerce in the following choice ACA.B2BB. C2CC. B2C96.The operations of a structured data type might act on (ABCD ).A.a stackB. the values of the data typeC. component elements of the data structureD. a queue97.Types of media include__ACD________.A.textB. animationC. audioD. full-motion video98. A virus is a program that reproduces its own code by (ABC ).A.simply placing a pointerB. adding to the end of a fileC. ins( )erting into the middle of a fileD. replacing another program99.According to the text,the author mentions three of the most commonly used types of printer.They are (BDE ).A.belt printerB. dot-matrix printers;C. array printerD. laser printerE. inkjet printers100.The end equipment in a communication system includes ___ABD_______A.keyboardsB. DCEC. CRTsD. computers101.Software includes _____ACDE________etcA.programsB. all kinds of filesC. video gamesD. websitesE. mobile application102.With .NET, Microsoft is opening up a channel both to ( ) in other programming languages and to ( BC). (developers; components)A.coderB. developersC. componentsD. architecturemon contemporary operating systems include (ABCD ).A.LinuxB. Microsoft WindowsC. SolarisD. Mac OS104.A mechanism for translating Internet hostnames into IP addresses is___BCD_______A.equipped into the general-purpose operating systemB.typically inside of operating system kernelC.as a middleware by author’s definitionD.typically outside of operating system kernel105.RISC is____ABC______ed for many computer manufacturers nowadaysB.guided to be built from studying the execution behavior of high-level language programsC.abbreviation of reduced instruction set computerD.abbreviation of complex instruction set computer106.With .NET, Microsoft is opening up a channel both to _BC_______in other programming languages and to ________. (developers; components)A.coderB. componentsC. developersD. architecture107.The tools which Programming software usually provides include: ABCDEpilersB. interpretersC. text editorsD. linkersE. debuggers108.The following products of software are belong to middleware____BCD______A.OracleB. IBM’s Web Sphere MQC. Java 2 PlatformD. J2EE109.The system manager used by a fast processor can____BCD______A.connect a networkB. monitor processor’s core temperatureC. monitor processor’s supply voltagesD. reset a system110.Queues that occur everyday life can be seen (ABCD ).A.as automobiles waiting for a traffic lightB. as people waiting for service at a bankC. in an emergency room of a hospitalD. in certain societies lacking equality111.C++ include the following pillars: ____ABCD______.A.data hidingB. polymorphismC. encapsulationD. inheritance112.Windows 2000 is____ACD______A.new lease of WindowsB. an inventive technologyC. the same as Windows NTD. used for building a scalable network113.We use paged virtual memory to___ABCD_______A.extend the size of memoryB. reduce latency of the diskC. store large program and data setD. increase bandwidth of the disk114.According to the physical size of computers we can classify the __ABCD____ computers into A. supercomputer B. minicomputer C. microcomputer D. mainframe115.Some common applications related to electronic commerce are the following: ABCDEFGA.EmailB. TeleconferencingC. Instant messagingD. Shopping cart softwareE.NewsgroupsF. Enterprise content managementG. Online banking116.One machine cycle in RISC has _B_________A.two machine instructionsB. one machine instructionC. four machine instructionsD. three machine instructions117.The function of computer hardware is typically divided into three main categories.They are____ADE_____.A.inputB. motherboardC. cpuD. storageE. output118.Active Directory supports ( ABCD).A.granular access controlB. inheritanceC. encapsulationD. delegation of administrative task119.The core of SQL is formed by a command language that allows the (ACDE ) and performing management and administrative functions.A.deletion of dataB. process of dataC. updating of dataD. retrieval of dataE. ins( )ertion of data120.Some commentators say the outcome of the information revolution is likely to be as profound as the shift in (ABCD )A.industrialB. agriculturalC. Service IndustryD. handicraft industry。
Evolution of the Computer

Evolution of the Computer:演化的电脑:The first counting device was the abacus, originally from Asia. It worked on a place-value notion meaning that the place of a bead or rock on the apparatus determined how much it was worth.第一个计数装置是算盘,原来来自亚洲。
它工作在一个值概念意味着代替了珠和岩石上确定仪器它需要多少钱的价值。
1600s: John Napier discovers logarithms. Robert Bissaker invents the slide rule which will remain in popular use until 19??.1600年代纳皮尔的对数约翰发现。
罗伯特Bissaker发明计算尺它将始终在流行使用,直到19 ? ?。
1642: Blaise Pascal, a French mathematician and philosopher, invents the first mechanical digital calculator using gears, called the Pascaline. Although this machine could perform addition and subtraction on whole numbers, it was too expensive and only Pascal himself could repare it.1642:Blaise帕斯卡尔,一个法国数学家和哲学家,发明了第一个机械数字计算器使用齿轮,叫做Pascaline。
尽管这台机器能完成对整数加法和减法,它太贵了,只有自己能返修。
英语--计算机之父冯诺依曼

Along with Edward Teller (爱德华特勒)and Stanislaw Ulam(斯坦尼斯 乌拉姆), von Neumann
worked out key steps in the nuclear physics(核物 理) involved in
thermonuclear reactions(热核反应) and the hydrogen bomb(氢弹).
----
John Von Neumann (约翰.冯.诺依曼)
The eldest of three brothers, von Neumann was born Neumann János Lajos in Budapest, Hungary, to a wealthy Jewish family. His father is a lawyer who worked in a bank.
János, nicknamed “Jancsi” (Johnny), was a child prodigy(奇才) who showed an aptitude for languages, memorization, and mathematics.
Although he attended school at the grade level appropriate to his age, his father hired private tutors (家庭教师) to give him advanced instruction in those areas in which he had displayed an aptitude。 He received his Ph.D. (Philosophiae Doctor) in mathematics from Pázmány Péter University in Budapest at the age of 22.
HETEROGENEOUS COMPUTING
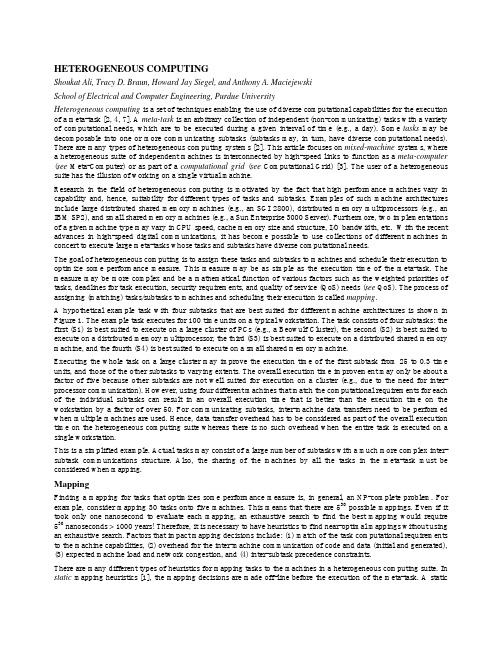
HETEROGENEOUS COMPUTINGShoukat Ali, Tracy D. Braun, Howard Jay Siegel, and Anthony A. MaciejewskiSchool of Electrical and Computer Engineering, Purdue UniversityHeterogeneous computing is a set of techniques enabling the use of diverse computational capabilities for the execution of a meta-task [2, 4, 7]. A meta-task is an arbitrary collection of independent (non-communicating) tasks with a variety of computational needs, which are to be executed during a given interval of time (e.g., a day). Some tasks may be decomposable into one or more communicating subtasks (subtasks may, in turn, have diverse computational needs). There are many types of heterogeneous computing systems [2]. This article focuses on mixed-machine systems, where a heterogeneous suite of independent machines is interconnected by high-speed links to function as a meta-computer (see Meta-Computer) or as part of a computational grid(see Computational Grid) [3]. The user of a heterogeneous suite has the illusion of working on a single virtual machine.Research in the field of heterogeneous computing is motivated by the fact that high performance machines vary in capability and, hence, suitability for different types of tasks and subtasks. Examples of such machine architectures include large distributed shared memory machines (e.g., an SGI 2800), distributed memory multiprocessors (e.g., an IBM SP2), and small shared memory machines (e.g., a Sun Enterprise 3000 Server). Furthermore, two implementations of a given machine type may vary in CPU speed, cache memory size and structure, I/O bandwidth, etc. With the recent advances in high-speed digital communications, it has become possible to use collections of different machines in concert to execute large meta-tasks whose tasks and subtasks have diverse computational needs.The goal of heterogeneous computing is to assign these tasks and subtasks to machines and schedule their execution to optimize some performance measure. This measure may be as simple as the execution time of the meta-task. The measure may be more complex and be a mathematical function of various factors such as the weighted priorities of tasks, deadlines for task execution, security requirements, and quality of service (QoS) needs (see QoS). The process of assigning (matching) tasks/subtasks to machines and scheduling their execution is called mapping.A hypothetical example task with four subtasks that are best suited for different machine architectures is shown in Figure 1. The example task executes for 100 time units on a typical workstation. The task consists of four subtasks: the first (S1) is best suited to execute on a large cluster of PCs (e.g., a Beowulf Cluster), the second (S2) is best suited to execute on a distributed memory multiprocessor, the third (S3) is best suited to execute on a distributed shared memory machine, and the fourth (S4) is best suited to execute on a small shared memory machine.Executing the whole task on a large cluster may improve the execution time of the first subtask from 25 to 0.3 time units, and those of the other subtasks to varying extents. The overall execution time improvement may only be about a factor of five because other subtasks are not well suited for execution on a cluster (e.g., due to the need for inter-processor communication). However, using four different machines that match the computational requirements for each of the individual subtasks can result in an overall execution time that is better than the execution time on the workstation by a factor of over 50. For communicating subtasks, inter-machine data transfers need to be performed when multiple machines are used. Hence, data transfer overhead has to be considered as part of the overall execution time on the heterogeneous computing suite whereas there is no such overhead when the entire task is executed on a single workstation.This is a simplified example. Actual tasks may consist of a large number of subtasks with a much more complex inter-subtask communications structure. Also, the sharing of the machines by all the tasks in the meta-task must be considered when mapping.MappingFinding a mapping for tasks that optimizes some performance measure is, in general, an NP-complete problem. For example, consider mapping 30 tasks onto five machines. This means that there are 530 possible mappings. Even if it took only one nanosecond to evaluate each mapping, an exhaustive search to find the best mapping would require 530 nanoseconds > 1000 years! Therefore, it is necessary to have heuristics to find near-optimal mappings without using an exhaustive search. Factors that impact mapping decisions include: (1) match of the task computational requirements to the machine capabilities, (2) overhead for the inter-machine communication of code and data (initial and generated), (3) expected machine load and network congestion, and (4) inter-subtask precedence constraints.There are many different types of heuristics for mapping tasks to the machines in a heterogeneous computing suite. In static mapping heuristics [1], the mapping decisions are made off-line before the execution of the meta-task. A staticmapping heuristic is employed if (1) the tasks that comprise the meta-task are known a priori , (2) predictions about the available heterogeneous computing resources are likely to be accurate, and (3) the estimated expected execution time of each task on each machine in the suite is known reasonably accurately. Static mapping heuristics can be used for planning the “next day’s work” on a heterogeneous computing system.Figure 1. Hypothetical example (based on [4]) of the advantage of using a heterogeneous computing suite of machines. The number underneath each bar indicates execution time. For the suite, each subtask execution time includes the overhead to receive data. Not drawn to scale.In dynamic mapping heuristics [6], the mapping decisions are made on-line during the execution of the meta-task. Dynamic approaches to mapping are needed if any of the following are unpredictable: (1) arrival times of the tasks, (2) machines available in the heterogeneous computing system (some machines in the suite may go off-line and new machines may come on-line), and (3) expected execution times of the tasks on the machines. While a static mapper considers the entire meta-task to be executed (e.g., the next day) when making decisions, a dynamic mapper has only information about tasks that have already arrived for execution. Furthermore, because a dynamic mapper operates on-line, it must make decisions much faster than an off-line static mapper. Consequently, dynamic mapping heuristics often use feedback from the heterogeneous computing system (while tasks are executing) to improve any “bad” mapping decisions.A semi-static mapping heuristic [8] can be used for an iterative task whose subtask execution times will change from iteration to iteration based on the input data. A semi-static methodology observes, from one iteration to another, the effects of the changing characteristics of the task's input data, called dynamic parameters, on the task's execution time. The off-line phase uses a static mapping algorithm to generate high quality mappings for a sampling of values for the dynamic parameters a priori. During the on-line phase, the actual dynamic parameters are observed and a new mapping for the subtasks may be selected from the precomputed off-line mappings. Automatic Heterogeneous ComputingOne of the long-term goals of heterogeneous computing research is to develop software environments that will automatically map and execute tasks expressed in a machine-independent high-level language. Such an environment will facilitate the use of heterogeneous computing suite by increasing portability, because the programmer need not be concerned with the composition of the heterogeneous computing suite, and increasing the possibility of deriving better mappings than the user can derive with ad hoc methods. Thus, it will improve the performance of and encourage the use of heterogeneous computing. While no such environment exists today, many researchers are working to develop one. A conceptual model for such an environment using a dedicated heterogeneous computing suite of machines is described in Figure 2, and consists of four stages.Stage 1 uses information about the type of tasks in the meta-task and machines in the heterogeneous computing suite to generate a set of parameters relevant to both the computational characteristics of tasks and the architectural features of machines. The system then derives categories for computational requirements and categories for machine capabilities from this set of parameters.Stage 2 consists of two components: task profiling and analytical benchmarking. Task profiling (see Task Profiling) decomposes each task of the meta-task into subtasks, where each subtask is computationally homogeneous. The computational requirements of each subtask are quantified by profiling the code and data. Analytical benchmarkingexecution on a cluster(see Analytical Benchmarking) quantifies how effectively each available machine in the suite performs on each type of computational requirement.The information available from stage 2 is used by stage 3 to derive the estimated execution time of each subtask on each machine in the heterogeneous computing suite, along with the associated inter-machine communication overheads. These statically derived results are then incorporated with initial values for machine ready times, inter-machine network delays, and status parameters (e.g., machine/network faults) to perform the mapping of subtasks to machines based on a given performance metric. The result is an assignment of subtasks to machines and an execution schedule. The process as described corresponds to a static mapping.The subtasks are executed in stage 4. If dynamic mapping is employed, the subtask completion times and loading/status of the machines/network are monitored (shown in dashed lines in Figure 2). The monitoring process is necessary because the actual computation times and data transfer times may be input-data dependent and deviate considerably from the static estimates. This information may be used to re-invoke the mapping of stage 3 to improve the machine assignment and execution schedule.Figure 2. Model for integrating the software support needed for automating the use of heterogeneous computing systems (based on [7]). Ovals indicate information and rectangles indicate action.1 2 3 4Environments and ApplicationsExamples of heterogeneous computing environments are: (1) the Purdue University Network Computing Hubs, a wide area network computing system which can be used to run a selection of software tools via a World Wide Web browser [5]; (2) NetSolve, a client-server system with geographically distributed servers that can be accessed from a variety of interfaces, including MATLAB, shell scripts, C, and FORTRAN [3]; and (3) the Globus meta-computing infrastructure toolkit, a set of low-level mechanisms that can be built upon to develop higher level heterogeneous computing services [3].Example applications that have demonstrated the usefulness of heterogeneous computing include: (1) a three-dimensional simulation of mixing and turbulent convection at the Minnesota Supercomputer Center [7]; (2) the shipboard anti-air warfare program (HiPer-D) used at the Naval Surface Warfare Center for threat detection, target engagement, and missile guidance; and (3) a simulation of colliding galaxies performed by solving large n-body dynamics problems and large gas dynamics problems at the National Center for Supercomputing Applications [7]. Open Problems in Heterogeneous ComputingHeterogeneous computing is a relatively new research area for the computer field. Interest in such systems continues to grow both in the research community and in the user community. The realization of the automatic heterogeneous computing environment envisioned in Figure 2 requires further research in many areas. Machine-independent languages with user-specified directives are needed to (1) allow compilation of a given task into efficient code for any machine in the suite, (2) aid in decomposing tasks into subtasks, and (3) facilitate determination of subtask computational requirements. Moreover, methods must be refined for measuring the loading and status of the machines in the heterogeneous computing suite and the network, and for estimating the subtask completion times. Also, the uncertainty present in the estimated parameter values, such as subtask completion times, should be taken into consideration in determining the mappings. Other research areas are (1) developing communication protocols for reliable, low overhead data transmission over heterogeneous networks with given QoS requirements, (2) devising debugging tools that can be used transparently across the suite of machines, and (3) formulating algorithms for task migration between heterogeneous machines, using task migration for fault tolerance or load re-balancing. Acknowledgment: This work was supported by the DARPA/ITO Quorum Program through the Office of Naval Research under Grant No. N00014-00-1-0599.References[1] T. D. Braun, H. J. Siegel, N. Beck, L. L. Boloni, M. Maheswaran, A. I. Reuther, J. P. Robertson, M. D. Theys, B. Yao, D. Hensgen, and R. F. Freund, A Comparison of Eleven Static Heuristics for Mapping a Class of Independent Tasks onto Heterogeneous Distributed Computing Systems, Journal of Parallel and Distributed Computing, 61(6), 810-837, 2001.[2] M. M. Eshaghian (ed.), Heterogeneous Computing, Artech House, Norwood, MA, 1996.[3] I. Foster and C. Kesselman (eds.), The Grid: Blueprint for a New Computing Infrastructure, Morgan Kaufmann, San Francisco, CA, 1999.[4] R. F. Freund and H. J. Siegel (guest eds.), Special Issue on Heterogeneous Processing, IEEE Computer, 26(6), 1993.[5] N. H. Kapadia and J. A. B. Fortes, PUNCH: An Architecture for Web-Enabled Wide-Area Network-Computing, Cluster Computing: The Journal of Networks, Software Tools and Applications, Special Issue on High Performance Distributed Computing, 2(2), 153-164, 1999.[6] M. Maheswaran, S. Ali, H. J. Siegel, D. Hensgen, and R. F. Freund, Dynamic Mapping of a Class of Independent Tasks onto Heterogeneous Computing Systems, Journal of Parallel and Distributed Computing, Special Issue on Software Support for Distributed Computing, 59(2), 107-131, 1999.[7] M. Maheswaran, T. D. Braun, and H. J. Siegel, “Heterogeneous Distributed Computing,” in Encyclopedia of Electrical and Electronics Engineering, Vol. 8, J. G. Webster, ed., John Wiley, New York, NY, 1999, pp. 679-690. [8] M. D. Theys, T. D. Braun, Y.-K. Kwok, H. J. Siegel, and A. A. Maciejewski, “Mapping of Tasks onto Distributed Heterogeneous Computing Systems Using a Genetic Algorithm Approach,” in Solutions to Parallel and Distributed Computing Problems: Lessons from Biological Sciences, A. Y. Zomaya, ed., John Wiley & Sons, New York, NY, 2001, pp. 135-178.Cross Reference:Analytical Benchmarking see Heterogeneous Computing.Computational Grid see Heterogeneous Computing.Meta-Computer see Heterogeneous Computing.Meta-Task see Heterogeneous Computing.Task Profiling see Heterogeneous Computing.Dictionary Terms:Analytical BenchmarkingAnalytical benchmarking of a given computing machine provides a measure of performance of the machine on each of the different code types that may be present in a given source program. The performance of a particular code type on a specific kind of resource is a multi-variable function. Some of the variables of such a function may be: the quality of service requirements of the application program (e.g., data precision), the size of the data set to be processed, the algorithm to be applied, programmer and compiler efforts to optimize the program, and the operating system and architecture of the machine that will execute the specific code type. (See Heterogeneous Computing.) Computational GridA developing area of research and technology seeking to connect regional and national computational resources in a transparent fashion, thus transforming any computer connected to the grid into part of a new class of supercomputer. The implied analogy is with an electric power grid. If access to advanced computational capabilities and accessories became as ubiquitous and dependable as an electric power grid, the impact on society would be dramatic. (See Heterogeneous Computing.)Meta-ComputerA system framework that utilizes the resources of many different computers connected via a network to cooperate on solving a problem. In general, this allows the problem to be solved much more quickly than would be possible using a single computer. Meta-computers usually consist of heterogeneous, distributed elements, and operate in a coarse-grained fashion. A meta-computer would be a more localized component of a larger computational grid. (See Heterogeneous Computing.)QoSQoS (Quality of Service) is an aggregate function of many different system characteristics used to represent the overall performance of a system. The components in the function, and the computation of the function itself, vary widely (i.e., QoS means many different things to many different people). Sample components of a QoS measure could include task deadlines, data precision, image color range, video jitter, network bandwidth, bit error rate, and end-to-end latency. (See Heterogeneous Computing.)Task ProfilingTask profiling of a given source program specifies types of computations that are present in the source program by decomposing it into code blocks based on computational requirements of the blocks. The set of computation types defined depends on the architectural features of the machines available for executing the source program or its subprograms, and on both the application task code and the types and sizes of data sets it is to process. (See Heterogeneous Computing.)。
中级会计学 intermediate accounting 赖红宁 chapter 17课后答案

E17-18
Consignor. Preparation of journal entries.
10-15
P17-1
Revenue Recognition Alternatives. Indication of when revenue should be recognized under various situations.
The company advances and defers expenses for which there is a direct "association of cause and effect" so that they are matched against the revenue. It usually recognizes other expenses in the normal manner.
20-30
P17-3
(AICPA adapted).Percentage-of-Completion. Total contract income and amount of cash in first year determined from balance sheet and income statement accounts.
45-60
P17-6
Long-Term Construction Contracts. Percentage-of-completion, completed contract. Journal entries to record transactions.
45-60
P17-7
Proportional Performance. Preparation of income statements. Theoretical question.
- 1、下载文档前请自行甄别文档内容的完整性,平台不提供额外的编辑、内容补充、找答案等附加服务。
- 2、"仅部分预览"的文档,不可在线预览部分如存在完整性等问题,可反馈申请退款(可完整预览的文档不适用该条件!)。
- 3、如文档侵犯您的权益,请联系客服反馈,我们会尽快为您处理(人工客服工作时间:9:00-18:30)。
∂ ( x) y = x∂ ( y) holds in B1 .
COMPUTATION OF THE E3 -TERM OF THE ADAMS SPEto see that there results an exact sequence of graded B0 - B0-bimodules − B0 → π 0 B → 0 0 → π 1 B → B1 → where in fact π0 B is a k-algebra, π1 B is a π0 B-π0 B-bimodule, and B0 → π0 ( B) is a homomorphism of algebras. (1.6) Definition. A (left) module over a pair algebra B is a graded pair M = (∂ : M1 → M0 ) in Mod such that M1 and M0 are left B0 -modules and ∂ is B0 -linear. Moreover a B0 -linear map µ ¯ : B1 ⊗B0 M0 → M1 is given fitting in the commutative diagram B1 ⊗B0 M1 / B1 ⊗B0 M0 p ppp p µ µ p pp µ ¯ xppp / M0 , M1
∂
in Mod. We write π0 (X ) = ker ∂ and π1 (X ) = coker ∂. A morphism f : X → Y of pairs is a commutative diagram X1
∂ f1
/ Y1
∂
X0 π0 , π1 :
f0
Evidently pairs with these morphisms form a category
A pair morphism is called a weak equivalence if it induces isomorphisms on π0 and π1 . Clearly a pair in Mod coincides with a chain complex concentrated in degrees 0 and 1. For two pairs X and Y the tensor product of the complexes corresponding to them is concentrated in degrees in 0, 1 and 2 and is given by X1 ⊗ Y1 − → X1 ⊗ Y0 ⊕ X0 ⊗ Y1 − → X0 ⊗ Y0 with ∂0 = (∂ ⊗ 1, 1 ⊗ ∂) and ∂1 = (−1 ⊗ ∂, ∂ ⊗ 1). Truncating X ⊗ Y we get the pair ¯ Y = (X ⊗ ¯ Y )1 = coker(∂1 ) → ¯ Y )0 X⊗ − X0 ⊗ Y0 = (X ⊗ with ∂ induced by ∂0 .
COMPUTATION OF THE E3 -TERM OF THE ADAMS SPECTRAL SEQUENCE
HANS JOACHIM BAUES AND MAMUKA JIBLADZE
arXiv:math/0407045v1 [math.AT] 3 Jul 2004
The algebra B of secondary cohomology operations is a pair algebra with Σ-structure which as a Hopf algebra was explicitly computed in [1]. In particular the multiplication map A of B was determined by an algorithm. In this paper we introduce algebraically the secondary Ext-groups ExtB and we prove that the E3 -term of the Adams spectral sequence (computing stable maps in {Y, X }∗ p ) is given by E3 (Y, X ) = ExtB (H X, H Y ). Here H X is the secondary cohomology of the spectrum X which is the B -module GΣ if X is the sphere spectrum S 0 . This leads to an algorithm for the computation of the group E3 (S 0 , S 0 ) = ExtB (GΣ , GΣ ) which is a new explicit approximation of stable homotopy groups of spheres improving the Adams approximation E2 (S 0 , S 0 ) = ExtA (F, F). An implementation of our algorithm computed E3 (S 0 , S 0 ) by now up to degree 40. In this range our results confirm the known results in the literature, see for example the book of Ravenel [6]. 1. M We here recall from [1] the notion of pair modules, pair algebras, and pair modules over a pair algebra B. The category B-Mod of pair modules over B is an additive track category in which we consider secondary resolutions as defined in [3]. Using such secondary resolutions we shall obtain the secondary derived functors ExtB in section 3. Let k be a commutative ring with unit and let Mod be the category of k-modules and k-linear maps. This is a symmetric monoidal category via the tensor product A⊗B over k of k-modules A, B. A pair of modules is a morphism (1.1) − X0 X = X1 →
1 ∂ ∂1 ∂0
È Ö (Mod) → Mod.
È Ö (Mod) and one has functors
/ Y0 .
2
HANS JOACHIM BAUES AND MAMUKA JIBLADZE
(1.2) Remark. Note that the full embedding of the category of pairs into the category of chain complexes induced by the above identification has a left adjoint Tr given by truncation: for a chain complex C = ... → C2 − → C1 − → C0 − − → C−1 → ... , one has ¯0 induced by ∂0 . Then clearly one has with ∂ ¯ Y = Tr(X ⊗ Y ). X⊗ (Mod) Using the fact that Tr is a reflection onto a full subcategory, one easily checks that the category ¯ and unit k = (0 → k) is a symmetric monoidal category, and Tr is a together with the tensor product ⊗ monoidal functor. We define the tensor product A ⊗ B of two graded modules in the usual way, i. e. by ( A ⊗ B)n = Ai ⊗ B j .
∂ f1 g1
∂ }H }} f0 / / Y0 , X0 g0
//> Y1 } }}
satisfying f0 − g0 = ∂ H and f1 − g1 = H ∂. A pair algebra B is a monoid in the monoidal category of graded pair modules, with multiplication ¯ B → B. µ : B⊗ We assume that B is concentrated in nonnegative degrees, that is Bn = 0 for n < 0. A left B-module is a graded pair module M together with a left action ¯M → M µ : B⊗ of the monoid B on M . More explicitly pair algebras and modules over them can be described as follows. (1.5) Definition. A pair algebra B is a graded pair ∂ : B1 → B0