Face Recognition using Symbolic KDA in the framework of Symbolic Data Analysis
人脸识别 面部 数字图像处理相关 中英对照 外文文献翻译 毕业设计论文 高质量人工翻译 原文带出处

人脸识别相关文献翻译,纯手工翻译,带原文出处(原文及译文)如下翻译原文来自Thomas David Heseltine BSc. Hons. The University of YorkDepartment of Computer ScienceFor the Qualification of PhD. — September 2005 -《Face Recognition: Two-Dimensional and Three-Dimensional Techniques》4 Two-dimensional Face Recognition4.1 Feature LocalizationBefore discussing the methods of comparing two facial images we now take a brief look at some at the preliminary processes of facial feature alignment. This process typically consists of two stages: face detection and eye localisation. Depending on the application, if the position of the face within the image is known beforehand (fbr a cooperative subject in a door access system fbr example) then the face detection stage can often be skipped, as the region of interest is already known. Therefore, we discuss eye localisation here, with a brief discussion of face detection in the literature review(section 3.1.1).The eye localisation method is used to align the 2D face images of the various test sets used throughout this section. However, to ensure that all results presented are representative of the face recognition accuracy and not a product of the performance of the eye localisation routine, all image alignments are manually checked and any errors corrected, prior to testing and evaluation.We detect the position of the eyes within an image using a simple template based method. A training set of manually pre-aligned images of feces is taken, and each image cropped to an area around both eyes. The average image is calculated and used as a template.Figure 4-1 - The average eyes. Used as a template for eye detection.Both eyes are included in a single template, rather than individually searching for each eye in turn, as the characteristic symmetry of the eyes either side of the nose, provides a useful feature that helps distinguish between the eyes and other false positives that may be picked up in the background. Although this method is highly susceptible to scale(i.e. subject distance from the camera) and also introduces the assumption that eyes in the image appear near horizontal. Some preliminary experimentation also reveals that it is advantageous to include the area of skin justbeneath the eyes. The reason being that in some cases the eyebrows can closely match the template, particularly if there are shadows in the eye-sockets, but the area of skin below the eyes helps to distinguish the eyes from eyebrows (the area just below the eyebrows contain eyes, whereas the area below the eyes contains only plain skin).A window is passed over the test images and the absolute difference taken to that of the average eye image shown above. The area of the image with the lowest difference is taken as the region of interest containing the eyes. Applying the same procedure using a smaller template of the individual left and right eyes then refines each eye position.This basic template-based method of eye localisation, although providing fairly preciselocalisations, often fails to locate the eyes completely. However, we are able to improve performance by including a weighting scheme.Eye localisation is performed on the set of training images, which is then separated into two sets: those in which eye detection was successful; and those in which eye detection failed. Taking the set of successful localisations we compute the average distance from the eye template (Figure 4-2 top). Note that the image is quite dark, indicating that the detected eyes correlate closely to the eye template, as we would expect. However, bright points do occur near the whites of the eye, suggesting that this area is often inconsistent, varying greatly from the average eye template.Figure 4-2 一Distance to the eye template for successful detections (top) indicating variance due to noise and failed detections (bottom) showing credible variance due to miss-detected features.In the lower image (Figure 4-2 bottom), we have taken the set of failed localisations(images of the forehead, nose, cheeks, background etc. falsely detected by the localisation routine) and once again computed the average distance from the eye template. The bright pupils surrounded by darker areas indicate that a failed match is often due to the high correlation of the nose and cheekbone regions overwhelming the poorly correlated pupils. Wanting to emphasise the difference of the pupil regions for these failed matches and minimise the variance of the whites of the eyes for successful matches, we divide the lower image values by the upper image to produce a weights vector as shown in Figure 4-3. When applied to the difference image before summing a total error, this weighting scheme provides a much improved detection rate.Figure 4-3 - Eye template weights used to give higher priority to those pixels that best represent the eyes.4.2 The Direct Correlation ApproachWe begin our investigation into face recognition with perhaps the simplest approach,known as the direct correlation method (also referred to as template matching by Brunelli and Poggio [29 ]) involving the direct comparison of pixel intensity values taken from facial images. We use the term "Direct Conelation, to encompass all techniques in which face images are compared directly, without any form of image space analysis, weighting schemes or feature extraction, regardless of the distance metric used. Therefore, we do not infer that Pearson's correlation is applied as the similarity function (although such an approach would obviously come under our definition of direct correlation). We typically use the Euclidean distance as our metric in these investigations (inversely related to Pearson's correlation and can be considered as a scale and translation sensitive form of image correlation), as this persists with the contrast made between image space and subspace approaches in later sections.Firstly, all facial images must be aligned such that the eye centres are located at two specified pixel coordinates and the image cropped to remove any background information. These images are stored as greyscale bitmaps of 65 by 82 pixels and prior to recognition converted into a vector of 5330 elements (each element containing the corresponding pixel intensity value). Each corresponding vector can be thought of as describing a point within a 5330 dimensional image space. This simple principle can easily be extended to much larger images: a 256 by 256 pixel image occupies a single point in 65,536-dimensional image space and again, similar images occupy close points within that space. Likewise, similar faces are located close together within the image space, while dissimilar faces are spaced far apart. Calculating the Euclidean distance d, between two facial image vectors (often referred to as the query image q, and gallery image g), we get an indication of similarity. A threshold is then applied to make the final verification decision.d . q - g ( threshold accept ) (d threshold ⇒ reject ). Equ. 4-14.2.1 Verification TestsThe primary concern in any face recognition system is its ability to correctly verify a claimed identity or determine a person's most likely identity from a set of potential matches in a database. In order to assess a given system's ability to perform these tasks, a variety of evaluation methodologies have arisen. Some of these analysis methods simulate a specific mode of operation (i.e. secure site access or surveillance), while others provide a more mathematicaldescription of data distribution in some classification space. In addition, the results generated from each analysis method may be presented in a variety of formats. Throughout the experimentations in this thesis, we primarily use the verification test as our method of analysis and comparison, although we also use Fisher's Linear Discriminant to analyse individual subspace components in section 7 and the identification test for the final evaluations described in section 8. The verification test measures a system's ability to correctly accept or reject the proposed identity of an individual. At a functional level, this reduces to two images being presented for comparison, fbr which the system must return either an acceptance (the two images are of the same person) or rejection (the two images are of different people). The test is designed to simulate the application area of secure site access. In this scenario, a subject will present some form of identification at a point of entry, perhaps as a swipe card, proximity chip or PIN number. This number is then used to retrieve a stored image from a database of known subjects (often referred to as the target or gallery image) and compared with a live image captured at the point of entry (the query image). Access is then granted depending on the acceptance/rej ection decision.The results of the test are calculated according to how many times the accept/reject decision is made correctly. In order to execute this test we must first define our test set of face images. Although the number of images in the test set does not affect the results produced (as the error rates are specified as percentages of image comparisons), it is important to ensure that the test set is sufficiently large such that statistical anomalies become insignificant (fbr example, a couple of badly aligned images matching well). Also, the type of images (high variation in lighting, partial occlusions etc.) will significantly alter the results of the test. Therefore, in order to compare multiple face recognition systems, they must be applied to the same test set.However, it should also be noted that if the results are to be representative of system performance in a real world situation, then the test data should be captured under precisely the same circumstances as in the application environment.On the other hand, if the purpose of the experimentation is to evaluate and improve a method of face recognition, which may be applied to a range of application environments, then the test data should present the range of difficulties that are to be overcome. This may mean including a greater percentage of6difficult9 images than would be expected in the perceived operating conditions and hence higher error rates in the results produced. Below we provide the algorithm for executing the verification test. The algorithm is applied to a single test set of face images, using a single function call to the face recognition algorithm: CompareF aces(F ace A, FaceB). This call is used to compare two facial images, returning a distance score indicating how dissimilar the two face images are: the lower the score the more similar the two face images. Ideally, images of the same face should produce low scores, while images of different faces should produce high scores.Every image is compared with every other image, no image is compared with itself and nopair is compared more than once (we assume that the relationship is symmetrical). Once two images have been compared, producing a similarity score, the ground-truth is used to determine if the images are of the same person or different people. In practical tests this information is often encapsulated as part of the image filename (by means of a unique person identifier). Scores are then stored in one of two lists: a list containing scores produced by comparing images of different people and a list containing scores produced by comparing images of the same person. The final acceptance/rejection decision is made by application of a threshold. Any incorrect decision is recorded as either a false acceptance or false rejection. The false rejection rate (FRR) is calculated as the percentage of scores from the same people that were classified as rejections. The false acceptance rate (FAR) is calculated as the percentage of scores from different people that were classified as acceptances.For IndexA = 0 to length(TestSet) For IndexB = IndexA+l to length(TestSet) Score = CompareFaces(TestSet[IndexA], TestSet[IndexB]) If IndexA and IndexB are the same person Append Score to AcceptScoresListElseAppend Score to RejectScoresListFor Threshold = Minimum Score to Maximum Score:FalseAcceptCount, FalseRejectCount = 0For each Score in RejectScoresListIf Score <= ThresholdIncrease FalseAcceptCountFor each Score in AcceptScoresListIf Score > ThresholdIncrease FalseRejectCountF alse AcceptRate = FalseAcceptCount / Length(AcceptScoresList)FalseRej ectRate = FalseRejectCount / length(RejectScoresList)Add plot to error curve at (FalseRejectRate, FalseAcceptRate)These two error rates express the inadequacies of the system when operating at aspecific threshold value. Ideally, both these figures should be zero, but in reality reducing either the FAR or FRR (by altering the threshold value) will inevitably resultin increasing the other. Therefore, in order to describe the full operating range of a particular system, we vary the threshold value through the entire range of scores produced. The application of each threshold value produces an additional FAR, FRR pair, which when plotted on a graph produces the error rate curve shown below.False Acceptance Rate / %Figure 4-5 - Example Error Rate Curve produced by the verification test.The equal error rate (EER) can be seen as the point at which FAR is equal to FRR. This EER value is often used as a single figure representing the general recognition performance of a biometric system and allows for easy visual comparison of multiple methods. However, it is important to note that the EER does not indicate the level of error that would be expected in a real world application. It is unlikely that any real system would use a threshold value such that the percentage of false acceptances were equal to the percentage of false rejections. Secure site access systems would typically set the threshold such that false acceptances were significantly lower than false rejections: unwilling to tolerate intruders at the cost of inconvenient access denials.Surveillance systems on the other hand would require low false rejection rates to successfully identify people in a less controlled environment. Therefore we should bear in mind that a system with a lower EER might not necessarily be the better performer towards the extremes of its operating capability.There is a strong connection between the above graph and the receiver operating characteristic (ROC) curves, also used in such experiments. Both graphs are simply two visualisations of the same results, in that the ROC format uses the True Acceptance Rate(TAR), where TAR = 1.0 - FRR in place of the FRR, effectively flipping the graph vertically. Another visualisation of the verification test results is to display both the FRR and FAR as functions of the threshold value. This presentation format provides a reference to determine the threshold value necessary to achieve a specific FRR and FAR. The EER can be seen as the point where the two curves intersect.Figure 4-6 - Example error rate curve as a function of the score threshold The fluctuation of these error curves due to noise and other errors is dependant on the number of face image comparisons made to generate the data. A small dataset that only allows fbr a small number of comparisons will results in a jagged curve, in which large steps correspond to the influence of a single image on a high proportion of the comparisons made. A typical dataset of 720 images (as used in section 4.2.2) provides 258,840 verification operations, hence a drop of 1% EER represents an additional 2588 correct decisions, whereas the quality of a single image could cause the EER to fluctuate by up to 0.28.422 ResultsAs a simple experiment to test the direct correlation method, we apply the technique described above to a test set of 720 images of 60 different people, taken from the AR Face Database [ 39 ]. Every image is compared with every other image in the test set to produce a likeness score, providing 258,840 verification operations from which to calculate false acceptance rates and false rejection rates. The error curve produced is shown in Figure 4-7.Figure 4-7 - Error rate curve produced by the direct correlation method using no image preprocessing.We see that an EER of 25.1% is produced, meaning that at the EER threshold approximately one quarter of all verification operations carried out resulted in an incorrect classification. Thereare a number of well-known reasons for this poor level of accuracy. Tiny changes in lighting, expression or head orientation cause the location in image space to change dramatically. Images in face space are moved far apart due to these image capture conditions, despite being of the same person's face. The distance between images of different people becomes smaller than the area of face space covered by images of the same person and hence false acceptances and false rejections occur frequently. Other disadvantages include the large amount of storage necessaryfor holding many face images and the intensive processing required for each comparison, making this method unsuitable fbr applications applied to a large database. In section 4.3 we explore the eigenface method, which attempts to address some of these issues.4二维人脸识别4.1功能定位在讨论比较两个人脸图像,我们现在就简要介绍的方法一些在人脸特征的初步调整过程。
关于人脸识别的英语阅读理解
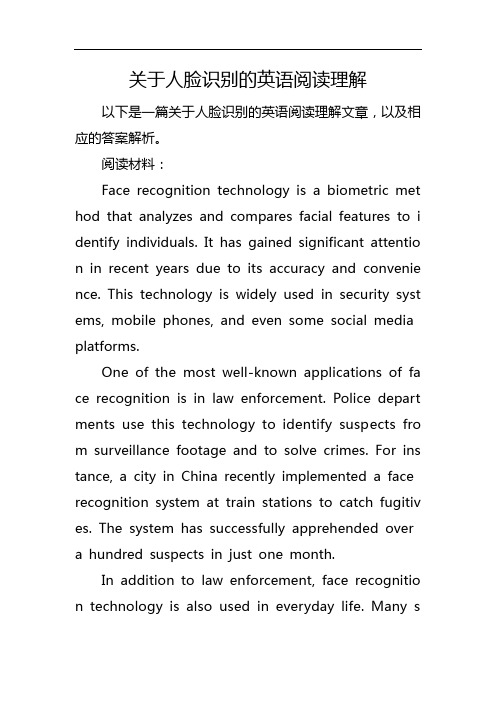
关于人脸识别的英语阅读理解以下是一篇关于人脸识别的英语阅读理解文章,以及相应的答案解析。
阅读材料:Face recognition technology is a biometric met hod that analyzes and compares facial features to i dentify individuals. It has gained significant attentio n in recent years due to its accuracy and convenie nce. This technology is widely used in security syst ems, mobile phones, and even some social media platforms.One of the most well-known applications of fa ce recognition is in law enforcement. Police depart ments use this technology to identify suspects fro m surveillance footage and to solve crimes. For ins tance, a city in China recently implemented a face recognition system at train stations to catch fugitiv es. The system has successfully apprehended over a hundred suspects in just one month.In addition to law enforcement, face recognitio n technology is also used in everyday life. Many smartphones now come with facial recognition soft ware, allowing users to unlock their phones simply by looking at them. This feature adds an extra lay er of security to the device.However, face recognition technology is not wi thout its challenges. Privacy concerns have been ra ised, as people worry about their personal informa tion being stored and used without their consent. There are also concerns about the accuracy of the technology, as it can sometimes mistake one perso n for another.Despite these challenges, face recognition tech nology continues to improve and expand its applic ations. It is now being used in airports, hotels, and even some retail stores. As the technology becom es more advanced, it is likely to play an even grea ter role in our lives.问题与答案解析:1. What is face recognition technology?Answer: Face recognition technology is a biom etric method that analyzes and compares facial fea tures to identify individuals.2. How is face recognition technology used in law enforcement?Answer: Police departments use face recognitio n technology to identify suspects from surveillance footage and to solve crimes.3. What are some everyday applications of face recognition technology?Answer: Everyday applications of face recogniti on technology include using it to unlock smartpho nes and improve security.4. What are some concerns about face recogni tion technology?Answer: Privacy concerns and accuracy issues a re two main concerns about face recognition techn ology.5. Despite the challenges, what is the future of face recognition technology likely to be?Answer: The future of face recognition technol ogy is expected to see continued improvement an d expansion of its applications.通过阅读这篇文章,读者可以了解到人脸识别技术的定义、应用领域、面临的挑战以及未来的发展趋势。
人脸表情识别英文参考资料
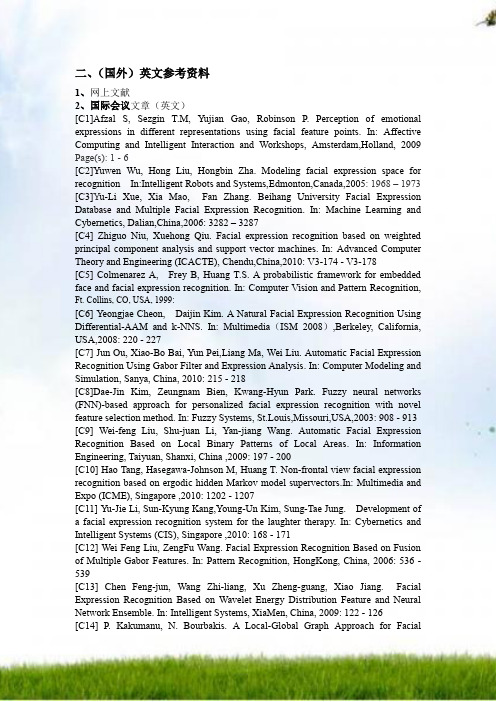
二、(国外)英文参考资料1、网上文献2、国际会议文章(英文)[C1]Afzal S, Sezgin T.M, Yujian Gao, Robinson P. Perception of emotional expressions in different representations using facial feature points. In: Affective Computing and Intelligent Interaction and Workshops, Amsterdam,Holland, 2009 Page(s): 1 - 6[C2]Yuwen Wu, Hong Liu, Hongbin Zha. Modeling facial expression space for recognition In:Intelligent Robots and Systems,Edmonton,Canada,2005: 1968 – 1973 [C3]Y u-Li Xue, Xia Mao, Fan Zhang. Beihang University Facial Expression Database and Multiple Facial Expression Recognition. In: Machine Learning and Cybernetics, Dalian,China,2006: 3282 – 3287[C4] Zhiguo Niu, Xuehong Qiu. Facial expression recognition based on weighted principal component analysis and support vector machines. In: Advanced Computer Theory and Engineering (ICACTE), Chendu,China,2010: V3-174 - V3-178[C5] Colmenarez A, Frey B, Huang T.S. A probabilistic framework for embedded face and facial expression recognition. In: Computer Vision and Pattern Recognition, Ft. Collins, CO, USA, 1999:[C6] Yeongjae Cheon, Daijin Kim. A Natural Facial Expression Recognition Using Differential-AAM and k-NNS. In: Multimedia(ISM 2008),Berkeley, California, USA,2008: 220 - 227[C7]Jun Ou, Xiao-Bo Bai, Yun Pei,Liang Ma, Wei Liu. Automatic Facial Expression Recognition Using Gabor Filter and Expression Analysis. In: Computer Modeling and Simulation, Sanya, China, 2010: 215 - 218[C8]Dae-Jin Kim, Zeungnam Bien, Kwang-Hyun Park. Fuzzy neural networks (FNN)-based approach for personalized facial expression recognition with novel feature selection method. In: Fuzzy Systems, St.Louis,Missouri,USA,2003: 908 - 913 [C9] Wei-feng Liu, Shu-juan Li, Yan-jiang Wang. Automatic Facial Expression Recognition Based on Local Binary Patterns of Local Areas. In: Information Engineering, Taiyuan, Shanxi, China ,2009: 197 - 200[C10] Hao Tang, Hasegawa-Johnson M, Huang T. Non-frontal view facial expression recognition based on ergodic hidden Markov model supervectors.In: Multimedia and Expo (ICME), Singapore ,2010: 1202 - 1207[C11] Yu-Jie Li, Sun-Kyung Kang,Young-Un Kim, Sung-Tae Jung. Development of a facial expression recognition system for the laughter therapy. In: Cybernetics and Intelligent Systems (CIS), Singapore ,2010: 168 - 171[C12] Wei Feng Liu, ZengFu Wang. Facial Expression Recognition Based on Fusion of Multiple Gabor Features. In: Pattern Recognition, HongKong, China, 2006: 536 - 539[C13] Chen Feng-jun, Wang Zhi-liang, Xu Zheng-guang, Xiao Jiang. Facial Expression Recognition Based on Wavelet Energy Distribution Feature and Neural Network Ensemble. In: Intelligent Systems, XiaMen, China, 2009: 122 - 126[C14] P. Kakumanu, N. Bourbakis. A Local-Global Graph Approach for FacialExpression Recognition. In: Tools with Artificial Intelligence, Arlington, Virginia, USA,2006: 685 - 692[C15] Mingwei Huang, Zhen Wang, Zilu Ying. Facial expression recognition using Stochastic Neighbor Embedding and SVMs. In: System Science and Engineering (ICSSE), Macao, China, 2011: 671 - 674[C16] Junhua Li, Li Peng. Feature difference matrix and QNNs for facial expression recognition. In: Control and Decision Conference, Yantai, China, 2008: 3445 - 3449 [C17] Yuxiao Hu, Zhihong Zeng, Lijun Yin,Xiaozhou Wei, Jilin Tu, Huang, T.S. A study of non-frontal-view facial expressions recognition. In: Pattern Recognition, Tampa, FL, USA,2008: 1 - 4[C18] Balasubramani A, Kalaivanan K, Karpagalakshmi R.C, Monikandan R. Automatic facial expression recognition system. In: Computing, Communication and Networking, St. Thomas,USA, 2008: 1 - 5[C19] Hui Zhao, Zhiliang Wang, Jihui Men. Facial Complex Expression Recognition Based on Fuzzy Kernel Clustering and Support Vector Machines. In: Natural Computation, Haikou,Hainan,China,2007: 562 - 566[C20] Khanam A, Shafiq M.Z, Akram M.U. Fuzzy Based Facial Expression Recognition. In: Image and Signal Processing, Sanya, Hainan, China,2008: 598 - 602 [C21] Sako H, Smith A.V.W. Real-time facial expression recognition based on features' positions and dimensions. In: Pattern Recognition, Vienna,Austria, 1996: 643 - 648 vol.3[C22] Huang M.W, Wang Z.W, Ying Z.L. A novel method of facial expression recognition based on GPLVM Plus SVM. In: Signal Processing (ICSP), Beijing, China, 2010: 916 - 919[C23] Xianxing Wu; Jieyu Zhao; Curvelet feature extraction for face recognition and facial expression recognition. In: Natural Computation (ICNC), Yantai, China, 2010: 1212 - 1216[C24]Xu Q Z, Zhang P Z, Yang L X, et al.A facial expression recognition approach based on novel support vector machine tree. In Proceedings of the 4th International Symposium on Neural Networks, Nanjing, China, 2007: 374-381.[C25] Wang Y B, Ai H Z, Wu B, et al. Real time facial expression recognition with adaboost.In: Proceedings of the 17th International Conference on Pattern Recognition , Cambridge,UK, 2004: 926-929.[C26] Guo G, Dyer C R. Simultaneous feature selection and classifier training via linear programming: a case study for face expression recognition. In: Proceedings of the 2003 IEEE Computer Society Conference on Computer Vision and Pattern Recognition, Madison, W isconsin, USA, 2003,1: 346-352.[C27] Bourel F, Chibelushi C C, Low A A. Robust facial expression recognition using a state-based model of spatially-localised facial dynamics. In: Proceedings of the 5th IEEE International Conference on Automatic Face and Gesture Recognition, Washington, DC, USA, 2002: 113-118·[C28] Buciu I, Kotsia I, Pitas I. Facial expression analysis under partial occlusion. In: Proceedings of the 2005 IEEE International Conference on Acoustics, Speech, and Signal Processing, Philadelphia, PA, USA, 2005,V: 453-456.[C29] ZHAN Yong-zhao,YE Jing-fu,NIU De-jiao,et al.Facial expression recognition based on Gabor wavelet transformation and elastic templates matching. Proc of the 3rd International Conference on Image and Graphics.Washington DC, USA,2004:254-257.[C30] PRASEEDA L V,KUMAR S,VIDYADHARAN D S,et al.Analysis of facial expressions using PCA on half and full faces. Proc of ICALIP2008.2008:1379-1383.[C31] LEE J J,UDDIN M Z,KIM T S.Spatiotemporal human facial expression recognition using Fisher independent component analysis and Hidden Markov model [C]//Proc of the 30th Annual International Conference of IEEE Engineering in Medicine and Biology Society.2008:2546-2549.[C32] LITTLEWORT G,BARTLETT M,FASELL. Dynamics of facial expression extracted automatically from video. In: Proceedings of IEEE Conference on Computer Vision and Pattern Recognition, Workshop on Face Processing inVideo, Washington DC,USA,2006:80-81.[C33] Kotsia I, Nikolaidis N, Pitas I. Facial Expression Recognition in Videos using a Novel Multi-Class Support Vector Machines Variant. In: Acoustics, Speech and Signal Processing, Honolulu, Hawaii, USA, 2007: II-585 - II-588[C34] Ruo Du, Qiang Wu, Xiangjian He, Wenjing Jia, Daming Wei. Facial expression recognition using histogram variances faces. In: Applications of Computer Vision (WACV), Snowbird, Utah, USA, 2009: 1 - 7[C35] Kobayashi H, Tange K, Hara F. Real-time recognition of six basic facial expressions. In: Robot and Human Communication, Tokyo , Japan,1995: 179 - 186 [C36] Hao Tang, Huang T.S. 3D facial expression recognition based on properties of line segments connecting facial feature points. In: Automatic Face & Gesture Recognition, Amsterdam, The Netherlands, 2008: 1 - 6[C37] Fengjun Chen, Zhiliang Wang, Zhengguang Xu, Donglin Wang. Research on a method of facial expression recognition.in: Electronic Measurement & Instruments, Beijing,China, 2009: 1-225 - 1-229[C38] Hui Zhao, Tingting Xue, Linfeng Han. Facial complex expression recognition based on Latent DirichletAllocation. In: Natural Computation (ICNC), Yantai, Shandong, China, 2010: 1958 - 1960[C39] Qinzhen Xu, Pinzheng Zhang, Wenjiang Pei, Luxi Yang, Zhenya He. An Automatic Facial Expression Recognition Approach Based on Confusion-Crossed Support Vector Machine Tree. In: Acoustics, Speech and Signal Processing, Honolulu, Hawaii, USA, 2007: I-625 - I-628[C40] Sung Uk Jung, Do Hyoung Kim, Kwang Ho An, Myung Jin Chung. Efficient rectangle feature extraction for real-time facial expression recognition based on AdaBoost.In: Intelligent Robots and Systems, Edmonton,Canada, 2005: 1941 - 1946[C41] Patil K.K, Giripunje S.D, Bajaj P.R. Facial Expression Recognition and Head Tracking in Video Using Gabor Filter .In: Emerging Trends in Engineering and Technology (ICETET), Goa, India, 2010: 152 - 157[C42] Jun Wang, Lijun Yin, Xiaozhou Wei, Yi Sun. 3D Facial Expression Recognition Based on Primitive Surface Feature Distribution.In: Computer Vision and PatternRecognition, New York, USA,2006: 1399 - 1406[C43] Shi Dongcheng, Jiang Jieqing. The method of facial expression recognition based on DWT-PCA/LDA.IN: Image and Signal Processing (CISP), Yantai,China, 2010: 1970 - 1974[C44] Asthana A, Saragih J, Wagner M, Goecke R. Evaluating AAM fitting methods for facial expression recognition. In: Affective Computing and Intelligent Interaction and Workshops, Amsterdam,Holland, 2009:1-8[C45] Geng Xue, Zhang Youwei. Facial Expression Recognition Based on the Difference of Statistical Features.In: Signal Processing, Guilin, China, 2006[C46] Metaxas D. Facial Features Tracking for Gross Head Movement analysis and Expression Recognition.In: Multimedia Signal Processing, Chania,Crete,GREECE, 2007:2[C47] Xia Mao, YuLi Xue, Zheng Li, Kang Huang, ShanWei Lv. Robust facial expression recognition based on RPCA and AdaBoost.In: Image Analysis for Multimedia Interactive Services, London, UK, 2009: 113 - 116[C48] Kun Lu, Xin Zhang. Facial Expression Recognition from Image Sequences Based on Feature Points and Canonical Correlations.In: Artificial Intelligence and Computational Intelligence (AICI), Sanya,China, 2010: 219 - 223[C49] Peng Zhao-yi, Wen Zhi-qiang, Zhou Yu. Application of Mean Shift Algorithm in Real-Time Facial Expression Recognition.In: Computer Network and Multimedia Technology, Wuhan,China, 2009: 1 - 4[C50] Xu Chao, Feng Zhiyong, Facial Expression Recognition and Synthesis on Affective Emotions Composition.In: Future BioMedical Information Engineering, Wuhan,China, 2008: 144 - 147[C51] Zi-lu Ying, Lin-bo Cai. Facial Expression Recognition with Marginal Fisher Analysis on Local Binary Patterns.In: Information Science and Engineering (ICISE), Nanjing,China, 2009: 1250 - 1253[C52] Chuang Yu, Yuning Hua, Kun Zhao. The Method of Human Facial Expression Recognition Based on Wavelet Transformation Reducing the Dimension and Improved Fisher Discrimination.In: Intelligent Networks and Intelligent Systems (ICINIS), Shenyang,China, 2010: 43 - 47[C53] Stratou G, Ghosh A, Debevec P, Morency L.-P. Effect of illumination on automatic expression recognition: A novel 3D relightable facial database .In: Automatic Face & Gesture Recognition and Workshops (FG 2011), Santa Barbara, California,USA, 2011: 611 - 618[C54] Jung-Wei Hong, Kai-Tai Song. Facial expression recognition under illumination variation.In: Advanced Robotics and Its Social Impacts, Hsinchu, Taiwan,2007: 1 - 6[C55] Ryan A, Cohn J.F, Lucey S, Saragih J, Lucey P, De la Torre F, Rossi A. Automated Facial Expression Recognition System.In: Security Technology, Zurich, Switzerland, 2009: 172 - 177[C56] Gokturk S.B, Bouguet J.-Y, Tomasi C, Girod B. Model-based face tracking for view-independent facial expression recognition.In: Automatic Face and Gesture Recognition, Washington, D.C., USA, 2002: 287 - 293[C57] Guo S.M, Pan Y.A, Liao Y.C, Hsu C.Y, Tsai J.S.H, Chang C.I. A Key Frame Selection-Based Facial Expression Recognition System.In: Innovative Computing, Information and Control, Beijing,China, 2006: 341 - 344[C58] Ying Zilu, Li Jingwen, Zhang Youwei. Facial expression recognition based on two dimensional feature extraction.In: Signal Processing, Leipzig, Germany, 2008: 1440 - 1444[C59] Fengjun Chen, Zhiliang Wang, Zhengguang Xu, Jiang Xiao, Guojiang Wang. Facial Expression Recognition Using Wavelet Transform and Neural Network Ensemble.In: Intelligent Information Technology Application, Shanghai,China,2008: 871 - 875[C60] Chuan-Yu Chang, Yan-Chiang Huang, Chi-Lu Yang. Personalized Facial Expression Recognition in Color Image.In: Innovative Computing, Information and Control (ICICIC), Kaohsiung,Taiwan, 2009: 1164 - 1167[C61] Bourel F, Chibelushi C.C, Low A.A. Robust facial expression recognition using a state-based model of spatially-localised facial dynamics. In: Automatic Face and Gesture Recognition, Washington, D.C., USA, 2002: 106 - 111[C62] Chen Juanjuan, Zhao Zheng, Sun Han, Zhang Gang. Facial expression recognition based on PCA reconstruction.In: Computer Science and Education (ICCSE), Hefei,China, 2010: 195 - 198[C63] Guotai Jiang, Xuemin Song, Fuhui Zheng, Peipei Wang, Omer A.M. Facial Expression Recognition Using Thermal Image.In: Engineering in Medicine and Biology Society, Shanghai,China, 2005: 631 - 633[C64] Zhan Yong-zhao, Ye Jing-fu, Niu De-jiao, Cao Peng. Facial expression recognition based on Gabor wavelet transformation and elastic templates matching.In: Image and Graphics, Hongkong,China, 2004: 254 - 257[C65] Ying Zilu, Zhang Guoyi. Facial Expression Recognition Based on NMF and SVM. In: Information Technology and Applications, Chengdu,China, 2009: 612 - 615 [C66] Xinghua Sun, Hongxia Xu, Chunxia Zhao, Jingyu Yang. Facial expression recognition based on histogram sequence of local Gabor binary patterns. In: Cybernetics and Intelligent Systems, Chengdu,China, 2008: 158 - 163[C67] Zisheng Li, Jun-ichi Imai, Kaneko M. Facial-component-based bag of words and PHOG descriptor for facial expression recognition.In: Systems, Man and Cybernetics, San Antonio,TX,USA,2009: 1353 - 1358[C68] Chuan-Yu Chang, Yan-Chiang Huang. Personalized facial expression recognition in indoor environments.In: Neural Networks (IJCNN), Barcelona, Spain, 2010: 1 - 8[C69] Ying Zilu, Fang Xieyan. Combining LBP and Adaboost for facial expression recognition.In: Signal Processing, Leipzig, Germany, 2008: 1461 - 1464[C70] Peng Yang, Qingshan Liu, Metaxas, D.N. RankBoost with l1 regularization for facial expression recognition and intensity estimation.In: Computer Vision, Kyoto,Japan, 2009: 1018 - 1025[C71] Patil R.A, Sahula V, Mandal A.S. Automatic recognition of facial expressions in image sequences: A review.In: Industrial and Information Systems (ICIIS), Mangalore, India, 2010: 408 - 413[C72] Iraj Hosseini, Nasim Shams, Pooyan Amini, Mohammad S. Sadri, Masih Rahmaty, Sara Rahmaty. Facial Expression Recognition using Wavelet-Based Salient Points and Subspace Analysis Methods.In: Electrical and Computer Engineering, Ottawa, Canada, 2006: 1992 - 1995[C73][C74][C75][C76][C77][C78][C79]3、英文期刊文章[J1] Aleksic P.S., Katsaggelos A.K. Automatic facial expression recognition using facial animation parameters and multistream HMMs.IEEE Transactions on Information Forensics and Security, 2006, 1(1):3-11[J2] Kotsia I,Pitas I. Facial Expression Recognition in Image Sequences Using Geometric Deformation Features and Support Vector Machines. IEEE Transactions on Image Processing, 2007, 16(1):172 – 187[J3] Mpiperis I, Malassiotis S, Strintzis M.G. Bilinear Models for 3-D Face and Facial Expression Recognition. IEEE Transactions on Information Forensics and Security, 2008,3(3) : 498 - 511[J4] Sung J, Kim D. Pose-Robust Facial Expression Recognition Using View-Based 2D+3D AAM. IEEE Transactions on Systems and Humans, 2008 , 38 (4): 852 - 866 [J5]Yeasin M, Bullot B, Sharma R. Recognition of facial expressions and measurement of levels of interest from video. IEEE Transactions on Multimedia, 2006, 8(3): 500 - 508[J6] Wenming Zheng, Xiaoyan Zhou, Cairong Zou, Li Zhao. Facial expression recognition using kernel canonical correlation analysis (KCCA). IEEE Transactions on Neural Networks, 2006, 17(1): 233 - 238[J7]Pantic M, Patras I. Dynamics of facial expression: recognition of facial actions and their temporal segments from face profile image sequences. IEEE Transactions on Systems, Man, and Cybernetics, Part B: Cybernetics, 2006, 36(2): 433 - 449[J8] Mingli Song, Dacheng Tao, Zicheng Liu, Xuelong Li, Mengchu Zhou. Image Ratio Features for Facial Expression Recognition Application. IEEE Transactions on Systems, Man, and Cybernetics, Part B: Cybernetics, 2010, 40(3): 779 - 788[J9] Dae Jin Kim, Zeungnam Bien. Design of “Personalized” Classifier Using Soft Computing Techniques for “Personalized” Facial Expression Recognition. IEEE Transactions on Fuzzy Systems, 2008, 16(4): 874 - 885[J10] Uddin M.Z, Lee J.J, Kim T.-S. An enhanced independent component-based human facial expression recognition from video. IEEE Transactions on Consumer Electronics, 2009, 55(4): 2216 - 2224[J11] Ruicong Zhi, Flierl M, Ruan Q, Kleijn W.B. Graph-Preserving Sparse Nonnegative Matrix Factorization With Application to Facial Expression Recognition.IEEE Transactions on Systems, Man, and Cybernetics, Part B: Cybernetics, 2011, 41(1): 38 - 52[J12] Chibelushi C.C, Bourel F. Hierarchical multistream recognition of facial expressions. IEE Proceedings - Vision, Image and Signal Processing, 2004, 151(4): 307 - 313[J13] Yongsheng Gao, Leung M.K.H, Siu Cheung Hui, Tananda M.W. Facial expression recognition from line-based caricatures. IEEE Transactions on Systems, Man and Cybernetics, Part A: Systems and Humans, 2003, 33(3): 407 - 412[J14] Ma L, Khorasani K. Facial expression recognition using constructive feedforward neural networks. IEEE Transactions on Systems, Man, and Cybernetics, Part B: Cybernetics, 2004, 34(3): 1588 - 1595[J15] Essa I.A, Pentland A.P. Coding, analysis, interpretation, and recognition of facial expressions. IEEE Transactions on Pattern Analysis and Machine Intelligence, 1997, 19(7): 757 - 763[J16] Anderson K, McOwan P.W. A real-time automated system for the recognition of human facial expressions. IEEE Transactions on Systems, Man, and Cybernetics, Part B: Cybernetics, 2006, 36(1): 96 - 105[J17] Soyel H, Demirel H. Facial expression recognition based on discriminative scale invariant feature transform. Electronics Letters 2010, 46(5): 343 - 345[J18] Fei Cheng, Jiangsheng Yu, Huilin Xiong. Facial Expression Recognition in JAFFE Dataset Based on Gaussian Process Classification. IEEE Transactions on Neural Networks, 2010, 21(10): 1685 – 1690[J19] Shangfei Wang, Zhilei Liu, Siliang Lv, Yanpeng Lv, Guobing Wu, Peng Peng, Fei Chen, Xufa Wang. A Natural Visible and Infrared Facial Expression Database for Expression Recognition and Emotion Inference. IEEE Transactions on Multimedia, 2010, 12(7): 682 - 691[J20] Lajevardi S.M, Hussain Z.M. Novel higher-order local autocorrelation-like feature extraction methodology for facial expression recognition. IET Image Processing, 2010, 4(2): 114 - 119[J21] Yizhen Huang, Ying Li, Na Fan. Robust Symbolic Dual-View Facial Expression Recognition With Skin Wrinkles: Local Versus Global Approach. IEEE Transactions on Multimedia, 2010, 12(6): 536 - 543[J22] Lu H.-C, Huang Y.-J, Chen Y.-W. Real-time facial expression recognition based on pixel-pattern-based texture feature. Electronics Letters 2007, 43(17): 916 - 918[J23]Zhang L, Tjondronegoro D. Facial Expression Recognition Using Facial Movement Features. IEEE Transactions on Affective Computing, 2011, pp(99): 1[J24] Zafeiriou S, Pitas I. Discriminant Graph Structures for Facial Expression Recognition. Multimedia, IEEE Transactions on 2008,10(8): 1528 - 1540[J25]Oliveira L, Mansano M, Koerich A, de Souza Britto Jr. A. Selecting 2DPCA Coefficients for Face and Facial Expression Recognition. Computing in Science & Engineering, 2011, pp(99): 1[J26] Chang K.I, Bowyer W, Flynn P.J. Multiple Nose Region Matching for 3D Face Recognition under Varying Facial Expression. Pattern Analysis and Machine Intelligence, IEEE Transactions on2006, 28(10): 1695 - 1700[J27] Kakadiaris I.A, Passalis G, Toderici G, Murtuza M.N, Yunliang Lu, Karampatziakis N, Theoharis T. Three-Dimensional Face Recognition in the Presence of Facial Expressions: An Annotated Deformable Model Approach.IEEE Transactions on Pattern Analysis and Machine Intelligence, 2007, 29(4): 640 - 649[J28] Guoying Zhao, Pietikainen M. Dynamic Texture Recognition Using Local Binary Patterns with an Application to Facial Expressions. IEEE Transactions on Pattern Analysis and Machine Intelligence, 2007, 29(6): 915 - 928[J29] Chakraborty A, Konar A, Chakraborty U.K, Chatterjee A. Emotion Recognition From Facial Expressions and Its Control Using Fuzzy Logic. IEEE Transactions on Systems, Man and Cybernetics, Part A: Systems and Humans, 2009, 39(4): 726 - 743 [J30] Pantic M, RothkrantzL J.M. Facial action recognition for facial expression analysis from static face images. IEEE Transactions on Systems, Man, and Cybernetics, Part B: Cybernetics, 2004, 34(3): 1449 - 1461[J31] Calix R.A, Mallepudi S.A, Bin Chen, Knapp G.M. Emotion Recognition in Text for 3-D Facial Expression Rendering. IEEE Transactions on Multimedia, 2010, 12(6): 544 - 551[J32]Kotsia I, Pitas I, Zafeiriou S, Zafeiriou S. Novel Multiclass Classifiers Based on the Minimization of the Within-Class Variance. IEEE Transactions on Neural Networks, 2009, 20(1): 14 - 34[J33]Cohen I, Cozman F.G, Sebe N, Cirelo M.C, Huang T.S. Semisupervised learning of classifiers: theory, algorithms, and their application to human-computer interaction. IEEE Transactions on Pattern Analysis and Machine Intelligence, 2004, 26(12): 1553 - 1566[J34] Zafeiriou S. Discriminant Nonnegative Tensor Factorization Algorithms. IEEE Transactions on Neural Networks, 2009, 20(2): 217 - 235[J35] Zafeiriou S, Petrou M. Nonlinear Non-Negative Component Analysis Algorithms. IEEE Transactions on Image Processing, 2010, 19(4): 1050 - 1066[J36] Kotsia I, Zafeiriou S, Pitas I. A Novel Discriminant Non-Negative Matrix Factorization Algorithm With Applications to Facial Image Characterization Problems. IEEE Transactions on Information Forensics and Security, 2007, 2(3): 588 - 595[J37] Irene Kotsia, Stefanos Zafeiriou, Ioannis Pitas. Texture and shape information fusion for facial expression and facial action unit recognition . Pattern Recognition, 2008, 41(3): 833-851[J38]Wenfei Gu, Cheng Xiang, Y.V. Venkatesh, Dong Huang, Hai Lin. Facial expression recognition using radial encoding of local Gabor features and classifier synthesis. Pattern Recognition, In Press, Corrected Proof, Available online 27 May 2011[J39] F Dornaika, E Lazkano, B Sierra. Improving dynamic facial expression recognition with feature subset selection. Pattern Recognition Letters, 2011, 32(5): 740-748[J40] Te-Hsun Wang, Jenn-Jier James Lien. Facial expression recognition system based on rigid and non-rigid motion separation and 3D pose estimation. Pattern Recognition, 2009, 42(5): 962-977[J41] Hyung-Soo Lee, Daijin Kim. Expression-invariant face recognition by facialexpression transformations. Pattern Recognition Letters, 2008, 29(13): 1797-1805[J42] Guoying Zhao, Matti Pietikäinen. Boosted multi-resolution spatiotemporal descriptors for facial expression recognition . Pattern Recognition Letters, 2009, 30(12): 1117-1127[J43] Xudong Xie, Kin-Man Lam. Facial expression recognition based on shape and texture. Pattern Recognition, 2009, 42(5):1003-1011[J44] Peng Yang, Qingshan Liu, Dimitris N. Metaxas Boosting encoded dynamic features for facial expression recognition . Pattern Recognition Letters, 2009,30(2): 132-139[J45] Sungsoo Park, Daijin Kim. Subtle facial expression recognition using motion magnification. Pattern Recognition Letters, 2009, 30(7): 708-716[J46] Chathura R. De Silva, Surendra Ranganath, Liyanage C. De Silva. Cloud basis function neural network: A modified RBF network architecture for holistic facial expression recognition. Pattern Recognition, 2008, 41(4): 1241-1253[J47] Do Hyoung Kim, Sung Uk Jung, Myung Jin Chung. Extension of cascaded simple feature based face detection to facial expression recognition. Pattern Recognition Letters, 2008, 29(11): 1621-1631[J48] Y. Zhu, L.C. De Silva, C.C. Ko. Using moment invariants and HMM in facial expression recognition. Pattern Recognition Letters, 2002, 23(1-3): 83-91[J49] Jun Wang, Lijun Yin. Static topographic modeling for facial expression recognition and analysis. Computer Vision and Image Understanding, 2007, 108(1-2): 19-34[J50] Caifeng Shan, Shaogang Gong, Peter W. McOwan. Facial expression recognition based on Local Binary Patterns: A comprehensive study. Image and Vision Computing, 2009, 27(6): 803-816[J51] Xue-wen Chen, Thomas Huang. Facial expression recognition: A clustering-based approach. Pattern Recognition Letters, 2003, 24(9-10): 1295-1302 [J52] Irene Kotsia, Ioan Buciu, Ioannis Pitas. An analysis of facial expression recognition under partial facial image occlusion. Image and Vision Computing, 2008, 26(7): 1052-1067[J53] Shuai Liu, Qiuqi Ruan. Orthogonal Tensor Neighborhood Preserving Embedding for facial expression recognition. Pattern Recognition, 2011, 44(7):1497-1513[J54] Eszter Székely, Henning Tiemeier, Lidia R. Arends, Vincent W.V. Jaddoe, Albert Hofman, Frank C. Verhulst, Catherine M. Herba. Recognition of Facial Expressions of Emotions by 3-Year-Olds. Emotion, 2011, 11(2): 425-435[J55] Kathleen M. Corcoran, Sheila R. Woody, David F. Tolin. Recognition of facial expressions in obsessive–compulsive disorder. Journal of Anxiety Disorders, 2008, 22(1): 56-66[J56] Bouchra Abboud, Franck Davoine, Mô Dang. Facial expression recognition and synthesis based on an appearance model. Signal Processing: Image Communication, 2004, 19(8): 723-740[J57] Teng Sha, Mingli Song, Jiajun Bu, Chun Chen, Dacheng Tao. Feature level analysis for 3D facial expression recognition. Neurocomputing, 2011,74(12-13) :2135-2141[J58] S. Moore, R. Bowden. Local binary patterns for multi-view facial expression recognition . Computer Vision and Image Understanding, 2011, 15(4):541-558[J59] Rui Xiao, Qijun Zhao, David Zhang, Pengfei Shi. Facial expression recognition on multiple manifolds. Pattern Recognition, 2011, 44(1):107-116[J60] Shyi-Chyi Cheng, Ming-Yao Chen, Hong-Yi Chang, Tzu-Chuan Chou. Semantic-based facial expression recognition using analytical hierarchy process. Expert Systems with Applications, 2007, 33(1): 86-95[J71] Carlos E. Thomaz, Duncan F. Gillies, Raul Q. Feitosa. Using mixture covariance matrices to improve face and facial expression recognitions. Pattern Recognition Letters, 2003, 24(13): 2159-2165[J72]Wen G,Bo C,Shan Shi-guang,et al. The CAS-PEAL large-scale Chinese face database and baseline evaluations.IEEE Transactions on Systems,Man and Cybernetics,part A:Systems and Hu-mans,2008,38(1):149-161.[J73] Yongsheng Gao,Leung ,M.K.H. Face recognition using line edge map.IEEE Transactions on Pattern Analysis and Machine Intelligence,2002,24:764-779. [J74] Hanouz M,Kittler J,Kamarainen J K,et al. Feature-based affine-invariant localization of faces.IEEE Transactions on Pat-tern Analysis and Machine Intelligence,2005,27:1490-1495.[J75] WISKOTT L,FELLOUS J M,KRUGER N,et al.Face recognition by elastic bunch graph matching.IEEE Trans on Pattern Analysis and Machine Intelligence,1997,19(7):775-779.[J76] Belhumeur P.N, Hespanha J.P, Kriegman D.J. Eigenfaces vs. fischerfaces: recognition using class specific linear projection.IEEE Trans on Pattern Analysis and Machine Intelligence,1997,15(7):711-720[J77] MA L,KHORASANI K.Facial Expression Recognition Using Constructive Feedforward Neural Networks. IEEE Transactions on Systems, Man and Cybernetics, Part B,2007,34(3):1588-1595.[J78][J79][J80][J81][J82][J83][J84][J85][J86][J87][J88][J89][J90]4、英文学位论文[D1]Hu Yuxiao. Three-dimensional face processing and its applications in biometrics:[Ph.D dissertation]. USA,Urbana-Champaign: University of Illinois, 2008。
介绍人脸识别在中国的应用英语作文

介绍人脸识别在中国的应用英语作文Title: An Overview of Facial Recognition Applications in ChinaIntroductionFacial recognition technology has gained significant momentum in China in recent years, propelled by advancements in artificial intelligence and extensive data collection. This technology is being widely used for various purposes, including security, payment systems, and even social credit scoring. In this essay, we will delve into the applications of facial recognition in China and explore its implications for society.Facial Recognition in SecurityOne of the most prominent applications of facial recognition in China is in the realm of security. The Chinese government has implemented widespread surveillance systems using facial recognition technology to monitor public spaces and track individuals. This has raised concerns about privacy and civil liberties, as the government has been accused of using the technology to suppress dissent and control the population.Facial Recognition in Payment SystemsFacial recognition is also being integrated into payment systems in China, allowing individuals to make purchases and transactions using their face as identification. This technology has been adopted by major tech companies such as Alibaba and Tencent, who are leading the way in developing seamless and secure payment solutions. This has revolutionized the way people in China conduct transactions, making cashless payments more convenient and efficient.Facial Recognition in Social Credit ScoringIn addition to security and payment systems, facial recognition technology is being used for social credit scoring in China. Individuals are assigned a social credit score based on their behavior and activities, which can impact their access to services and opportunities. Facial recognition technology is used to track individuals and monitor their actions, contributing to the data used to calculate their social credit score. This has led to concerns about surveillance and discrimination, as individuals may be penalized for minor infractions or perceived deviations from societal norms.Implications for SocietyThe widespread adoption of facial recognition technology in China has raised important questions about privacy, security, andindividual rights. While the technology offers convenience and efficiency in various applications, it also poses risks and challenges in terms of data protection and surveillance. There is a need for robust regulations and safeguards to ensure that facial recognition technology is used ethically and responsibly, without infringing on the rights of individuals.ConclusionFacial recognition technology is reshaping society in China, with applications ranging from security to payment systems and social credit scoring. While this technology offers numerous benefits in terms of efficiency and convenience, it also raises significant concerns about privacy and surveillance. It is crucial for policymakers, tech companies, and society at large to address these challenges and ensure that facial recognition technology is used in a manner that respects individual rights and promotes social good.。
人脸识别英语作文
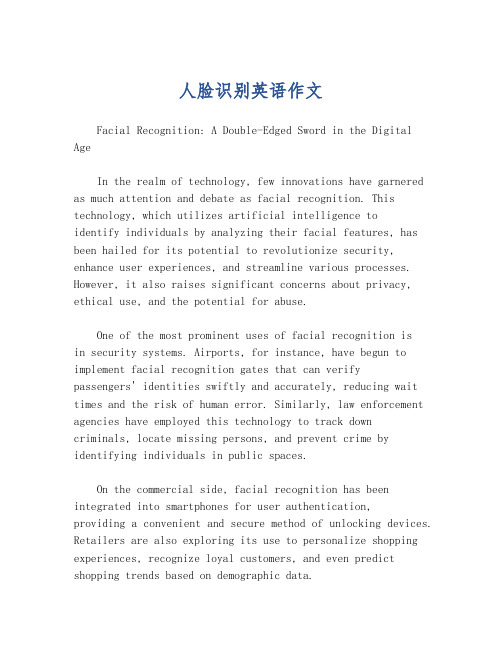
人脸识别英语作文Facial Recognition: A Double-Edged Sword in the Digital AgeIn the realm of technology, few innovations have garnered as much attention and debate as facial recognition. This technology, which utilizes artificial intelligence toidentify individuals by analyzing their facial features, has been hailed for its potential to revolutionize security, enhance user experiences, and streamline various processes. However, it also raises significant concerns about privacy, ethical use, and the potential for abuse.One of the most prominent uses of facial recognition isin security systems. Airports, for instance, have begun to implement facial recognition gates that can verifypassengers' identities swiftly and accurately, reducing wait times and the risk of human error. Similarly, law enforcement agencies have employed this technology to track down criminals, locate missing persons, and prevent crime by identifying individuals in public spaces.On the commercial side, facial recognition has been integrated into smartphones for user authentication,providing a convenient and secure method of unlocking devices. Retailers are also exploring its use to personalize shopping experiences, recognize loyal customers, and even predict shopping trends based on demographic data.Despite these benefits, the technology is not without its critics. Privacy advocates argue that the widespread use of facial recognition could lead to a surveillance state where individuals' movements and behaviors are constantly monitored without their consent. There are also concerns about the accuracy of the technology, with studies showing that it can be less effective for certain demographics, potentially leading to false identifications and unjust consequences.Moreover, the ethical implications of facial recognition are profound. Who has the right to access this technology and how it is used? What safeguards are in place to prevent misuse? These questions become even more critical when considering the potential for facial recognition to be usedin more invasive ways, such as monitoring political dissent or suppressing minority groups.Regulation is a key component in addressing these concerns. Governments and international bodies must work together to establish clear guidelines on the use of facial recognition technology. This includes ensuring transparencyin how the data is collected, stored, and used, as well as implementing strict penalties for misuse.In conclusion, facial recognition represents asignificant leap forward in technological capability. It has the potential to greatly enhance security, efficiency, and personalization in various sectors. However, it also presents a profound challenge to our understanding of privacy and personal freedom. As this technology continues to evolve, itis imperative that we approach its use with caution, guided by a robust ethical framework and stringent regulation to ensure that it serves as a tool for societal benefit rather than a weapon against individual liberty.。
面部识别在中国的应用英语作文
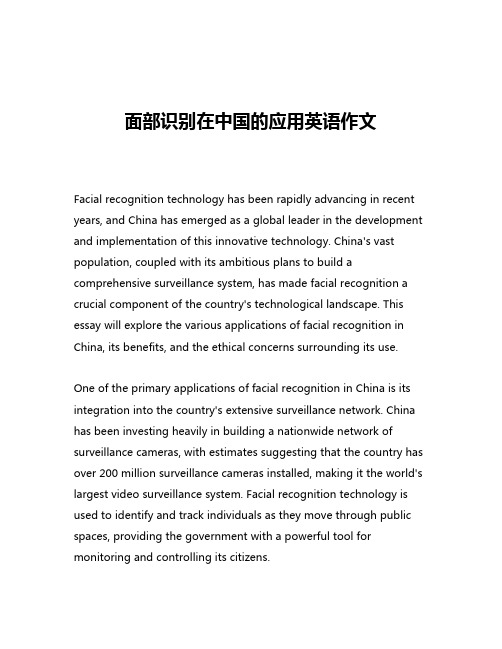
面部识别在中国的应用英语作文Facial recognition technology has been rapidly advancing in recent years, and China has emerged as a global leader in the development and implementation of this innovative technology. China's vast population, coupled with its ambitious plans to build a comprehensive surveillance system, has made facial recognition a crucial component of the country's technological landscape. This essay will explore the various applications of facial recognition in China, its benefits, and the ethical concerns surrounding its use.One of the primary applications of facial recognition in China is its integration into the country's extensive surveillance network. China has been investing heavily in building a nationwide network of surveillance cameras, with estimates suggesting that the country has over 200 million surveillance cameras installed, making it the world's largest video surveillance system. Facial recognition technology is used to identify and track individuals as they move through public spaces, providing the government with a powerful tool for monitoring and controlling its citizens.The Chinese government has justified the use of facial recognition by claiming that it enhances public safety and security. The technology has been employed to identify and apprehend criminals, as well as to monitor the movements of individuals deemed to be potential threats to social stability. For example, the government has used facial recognition to track and monitor the Uyghur minority population in the Xinjiang region, a practice that has been widely criticized by human rights organizations as a violation of individual privacy and a form of ethnic discrimination.In addition to its use in surveillance, facial recognition technology has also been integrated into various other aspects of daily life in China. The technology is widely used in mobile payment systems, allowing users to authenticate their identity and make payments using their facial features. This has led to a significant increase in the adoption of mobile payment platforms, such as Alipay and WeChat Pay, which have become ubiquitous in the country.Furthermore, facial recognition has been implemented in various public services, such as accessing public transportation, entering office buildings, and even checking into hotels. This has led to increased efficiency and convenience for users, but it has also raised concerns about the potential for abuse and the erosion of personal privacy.One of the most controversial applications of facial recognition in China is its use in the country's social credit system. The social credit system is a government-run initiative that aims to monitor and assess the behavior of Chinese citizens, with the goal of incentivizing "good" behavior and punishing "bad" behavior. Facial recognition is used to identify individuals and track their activities, which can then be used to assign them a social credit score. This score can have significant consequences, affecting an individual's access to various public services and opportunities.The use of facial recognition in China's social credit system has been widely criticized by human rights organizations and international observers. They argue that the system represents a significant threat to individual privacy and civil liberties, as it gives the government unprecedented power to monitor and control its citizens.Despite these concerns, the Chinese government has continued to invest heavily in the development and deployment of facial recognition technology. The country has become a global leader in this field, with Chinese companies such as Hikvision, Dahua, and SenseTime emerging as major players in the global facial recognition market.The rapid advancement of facial recognition technology in China has also raised concerns about the potential for abuse and the erosion ofindividual privacy. There are fears that the technology could be used to suppress dissent, target minority groups, and create a highly invasive surveillance state. Moreover, the lack of robust privacy protections and oversight mechanisms in China has exacerbated these concerns.In response to these concerns, the Chinese government has attempted to address some of the ethical issues surrounding the use of facial recognition. For example, the government has introduced regulations that require companies to obtain user consent before collecting and using facial recognition data. Additionally, the government has established guidelines for the ethical use of facial recognition technology, which include measures to protect individual privacy and prevent discrimination.However, critics argue that these measures are largely inadequate and that the Chinese government's commitment to protecting individual privacy is questionable. They point to the government's continued use of facial recognition for surveillance and social control purposes as evidence of its prioritization of national security over individual rights.In conclusion, the application of facial recognition technology in China is a complex and multifaceted issue. While the technology has brought about increased efficiency and convenience in variousaspects of daily life, it has also raised significant ethical concerns about the potential for abuse and the erosion of individual privacy. As China continues to push the boundaries of this technology, it will be crucial for the government to strike a delicate balance between national security and individual rights, and to implement robust safeguards and oversight mechanisms to ensure the ethical and responsible use of facial recognition technology.。
英文翻译
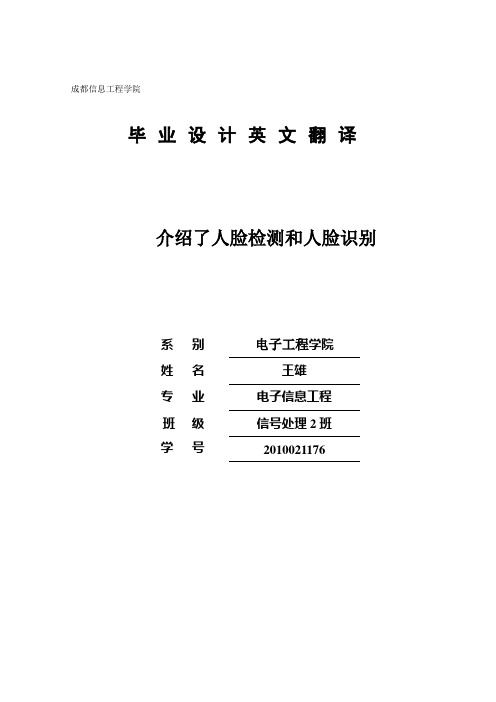
成都信息工程学院毕业设计英文翻译介绍了人脸检测和人脸识别系别电子工程学院姓名王雄专业电子信息工程班级信号处理2班学号2010021176Introduction to Face Detection and Face RecognitionLast updated on 4th Feb, 2012 by Shervin Emami. Posted originally on 2nd June, 2010."Face Recognition" is a very active area in the Computer Vision and Biometrics fields, as it has been studied vigorously for 25 years and is finally producing applications in security, robotics, human-computer-interfaces, digital cameras, games and entertainment."Face Recognition" generally involves two stages:Face Detection, where a photo is searched to find any face (shown here as a green rectangle), then image processing cleans up the facial image for easier recognition. Face Recognition, where that detected and processed face is compared to a database of known faces, to decide who that person is (shown here as red text).Since 2002, Face Detection can be performed fairly reliably such as with OpenCV's Face Detector, working in roughly 90-95% of clear photos of a person looking forward at the camera. It is usually harder to detect a person's face when they are viewed from the side or at an angle, and sometimes this requires 3D Head Pose Estimation. It can also be very difficult to detect a person's face if the photo is not very bright, or if part of the face is brighter than another or has shadows or is blurry or wearing glasses, etc. However, Face Recognition is much less reliable than Face Detection, generally 30-70% accurate. Face Recognition has been a strong field of research since the 1990s, but is still far from reliable, and more techniques are being invented each year such as the ones listed at the bottom of this page (Alternatives to Eigenfaces such as 3D face recognition or recognition from video).I will show you how to use Eigenfaces (also called "Principal Component Analysis" or PCA), a simple and popular method of 2D Face Recognition from a photo, as opposed to other common methods such as Neural Networks orFisher Faces.To learn the theory of how Eigenface works, you should read Face Recognition With Eigenface from Servo Magazine (April 2007), and perhaps the mathematical algorithm. First I will explain how to implement Eigenfaces for offline training from the command-line, based on the Servo Magazine tutorial and source-code (May 2007). Once I have explained to you how offline training and offline face recognition works from the command-line, I will explain how this can be extended to online training directly from a webcam in realtime :-)How to detect a face using OpenCV's Face Detector:As mentioned above, the first stage in Face Recognition is Face Detection. The OpenCV library makes it fairly easy to detect a frontal face in an image using its Haar Cascade Face Detector (also known as the Viola-Jones method).The function "cvHaarDetectObjects" in OpenCV performs the actual face detection, but the function is a bit tedious to use directly, so it is easiest to use this wrapper function: // Perform face detection on the input image, using the given Haar Cascade. // Returns a rectangle for the detected region in the given image.CvRect detectFaceInImage(IplImage *inputImg, CvHaarClassifierCascade* cascade) {// Smallest face size.CvSize minFeatureSize = cvSize(20, 20);// Only search for 1 face.int flags = CV_HAAR_FIND_BIGGEST_OBJECT | CV_HAAR_DO_ROUGH_SEARCH;// How detailed should the search be.float search_scale_factor = 1.1f;IplImage *detectImg;IplImage *greyImg = 0;CvMemStorage* storage;CvRect rc;double t;CvSeq* rects;CvSize size;int i, ms, nFaces;storage = cvCreateMemStorage(0);cvClearMemStorage( storage );// If the image is color, use a greyscale copy of the image.detectImg = (IplImage*)inputImg;if (inputImg->nChannels > 1) {size = cvSize(inputImg->width, inputImg->height);greyImg = cvCreateImage(size, IPL_DEPTH_8U, 1 );cvCvtColor( inputImg, greyImg, CV_BGR2GRAY );detectImg = greyImg; // Use the greyscale image.}// Detect all the faces in the greyscale image.t = (double)cvGetTickCount();rects = cvHaarDetectObjects( detectImg, cascade, storage,search_scale_factor, 3, flags, minFeatureSize);t = (double)cvGetTickCount() - t;ms = cvRound( t / ((double)cvGetTickFrequency() * 1000.0) );nFaces = rects->total;printf("Face Detection took %d ms and found %d objects\n", ms, nFaces);// Get the first detected face (the biggest).if (nFaces > 0)rc = *(CvRect*)cvGetSeqElem( rects, 0 );elserc = cvRect(-1,-1,-1,-1); // Couldn't find the face.if (greyImg)cvReleaseImage( &greyImg );cvReleaseMemStorage( &storage );//cvReleaseHaarClassifierCascade( &cascade );return rc; // Return the biggest face found, or (-1,-1,-1,-1).}Now you can simply call "detectFaceInImage" whenever you want to find a face in an image. You also need to specify the face classifier that OpenCV should use to detect the face. For example, OpenCV comes with several different classifiers for frontal face detection, as well as some profile faces (side view), eye detection, nose detection, mouth detection, whole body detection, etc. You can actually use this function with any of these other detectors if you want, or even create your own custom detector such as for car or person detection (read here), but since frontal face detection is the only one that is very reliable, it is the only one I will discuss.For frontal face detection, you can chose one of these Haar Cascade Classifiers that come with OpenCV (in the "data\haarcascades\" folder):"haarcascade_frontalface_default.xml""haarcascade_frontalface_alt.xml""haarcascade_frontalface_alt2.xml""haarcascade_frontalface_alt_tree.xml"Each one will give slightly different results depending on your environment, so you could even use all of them and combine the results together (if you want the most detections). There are also some more eye, head, mouth and nose detectors in the downloads section of Modesto's page.So you could do this in your program for face detection:// Haar Cascade file, used for Face Detection.char *faceCascadeFilename ="haarcascade_frontalface_alt.xml";// Load the HaarCascade classifier for face detection.CvHaarClassifierCascade* faceCascade;faceCascade = (CvHaarClassifierCascade*)cvLoad(faceCascadeFilename, 0, 0, 0);if( !faceCascade ) {printf("Couldnt load Face detector '%s'\n", faceCascadeFilename);exit(1);}// Grab the next frame from the camera.IplImage *inputImg = cvQueryFrame(camera);// Perform face detection on the input image, using the given Haar classifierCvRect faceRect = detectFaceInImage(inputImg, faceCascade);// Make sure a valid face was detected.if (faceRect.width > 0) {printf("Detected a face at (%d,%d)!\n", faceRect.x, faceRect.y);}.... Use 'faceRect' and 'inputImg' ....// Free the Face Detector resources when the program is finished cvReleaseHaarClassifierCascade( &cascade );How to preprocess facial images for Face Recognition:Now that you have detected a face, you can use that face image for Face Recognition. However, if you tried to simply perform face recognition directly on a normal photo image, you will probably get less than 10% accuracy!It is extremely important to apply various image pre-processing techniques to standardize the images that you supply to a face recognition system. Most face recognition algorithms are extremely sensitive to lighting conditions, so that if it was trained to recognize a person when they are in a dark room, it probably wont recognize them in a bright room, etc. This problem is referred to as "lumination dependent", and there are also many other issues, such as the face should also be in a very consistent position within the images (such as the eyes being in the same pixel coordinates), consistent size, rotation angle, hair and makeup, emotion (smiling, angry, etc), position of lights (to the left or above, etc). This is why it is so important to use a good image preprocessing filters before applying face recognition. You should also do things like removing the pixels around the face that aren't used, such as with an elliptical mask to only show the inner face region, not the hair and image background, since they change more than the face does.For simplicity, the face recognition system I will show you is Eigenfaces using greyscale images. So I will show you how to easily convert color images to greyscale (also called 'grayscale'), and then easily apply Histogram Equalization as a very simplemethod of automatically standardizing the brightness and contrast of your facial images. For better results, you could use color face recognition (ideally with color histogram fitting in HSV or another color space instead of RGB), or apply more processing stages such as edge enhancement, contour detection, motion detection, etc. Also, this code is resizing images to a standard size, but this might change the aspect ratio of the face. You can read my tutorial HERE on how to resize an image while keeping its aspect ratio the same.Here you can see an example of this preprocessing stage:Here is some basic code to convert from a RGB or greyscale input image to a greyscale image, resize to a consistent dimension, then apply Histogram Equalization for consistent brightness and contrast:// Either convert the image to greyscale, or use the existing greyscale image. IplImage *imageGrey;if (imageSrc->nChannels == 3) {imageGrey = cvCreateImage( cvGetSize(imageSrc), IPL_DEPTH_8U, 1 );// Convert from RGB (actually it is BGR) to Greyscale.cvCvtColor( imageSrc, imageGrey, CV_BGR2GRAY );}else {// Just use the input image, since it is already Greyscale.imageGrey = imageSrc;}// Resize the image to be a consistent size, even if the aspect ratio changes.IplImage *imageProcessed;imageProcessed = cvCreateImage(cvSize(width, height), IPL_DEPTH_8U, 1);// Make the image a fixed size. // CV_INTER_CUBIC or CV_INTER_LINEAR is good for enlarging, and // CV_INTER_AREA is good for shrinking / decimation, but bad at enlarging.cvResize(imageGrey, imageProcessed, CV_INTER_LINEAR);// Give the image a standard brightness and contrast.cvEqualizeHist(imageProcessed, imageProcessed);..... Use 'imageProcessed' for Face Recognition ....if (imageGrey)cvReleaseImage(&imageGrey);if (imageProcessed)cvReleaseImage(&imageProcessed);How Eigenfaces can be used for Face Recognition:Now that you have a pre-processed facial image, you can perform Eigenfaces (PCA) for Face Recognition. OpenCV comes with the function "cvEigenDecomposite()", which performs the PCA operation, however you need a database (training set) of images for it to know how to recognize each of your people.So you should collect a group of preprocessed facial images of each person you want to recognize. For example, if you want to recognize someone from a class of 10 students, then you could store 20 photos of each person, for a total of 200 preprocessed facial images of the same size (say 100x100 pixels).The theory of Eigenfaces is explained in the two Face Recognition with Eigenface articles in Servo Magazine, but I will also attempt to explain it here.Use "Principal Component Analysis" to convert all your 200 training images into a set of "Eigenfaces" that represent the main differences between the training images. First it will find the "average face image" of your images by getting the mean value of each pixel. Then the eigenfaces are calculated in comparison to this average face, where the first eigenface is the most dominant face differences, and the second eigenface is the second most dominant face differences, and so on, until you have about 50 eigenfaces that represent most of the differences in all the training set images.In these example images above you can see the average face and the first and last eigenfaces that were generated from a collection of 30 images each of 4 people. Notice that the average face will show the smooth face structure of a generic person, the first few eigenfaces will show some dominant features of faces, and the last eigenfaces (eg: Eigenface 119) are mainly image noise. You can see the first 32 eigenfaces in the image below.Explanation of Face Recognition using Principal Component Analysis:To explain Eigenfaces (Principal Component Analysis) in simple terms, Eigenfaces figures out the main differences between all the training images, and then how to represent each training image using a combination of those differences.So for example, one of the training images might be made up of:(averageFace) + (13.5% of eigenface0) - (34.3% of eigenface1) + (4.7% of eigenface2) + ... + (0.0% of eigenface199).Once it has figured this out, it can think of that training image as the 200 ratios: {13.5, -34.3, 4.7, ..., 0.0}.It is indeed possible to generate the training image back from the 200 ratios by multiplying the ratios with the eigenface images, and adding the average face. But since many of the last eigenfaces will be image noise or wont contribute much to the image, this list of ratios can be reduced to just the most dominant ones, such as the first 30 numbers, without effecting the image quality much. So now its possible to represent all 200 training images using just 30 eigenface images, the average face image, and a list of 30 ratios for each of the 200 training images.Interestingly, this means that we have found a way to compress the 200 images into just 31 images plus a bit of extra data, without loosing much image quality. But this tutorial is about face recognition, not image compression, so we will ignore that :-)To recognize a person in a new image, it can apply the same PCA calculations to find 200 ratios for representing the input image using the same 200 eigenfaces. And once again it can just keep the first 30 ratios and ignore the rest as they are less important. It can then search through its list of ratios for each of its 20 known people in its database, to see who has their top 30 ratios that are most similar to the 30 ratios for the input image. This is basically a method of checking which training image is most similar to the input image, out of the whole 200 training images that were supplied. Implementing Offline Training:For implementation of offline training, where files are used as input and output through the command-line, I am using a similar method as the Face Recognition with Eigenface implementation in Servo Magazine, so you should read that article first, but I have made a few slight changes.Basically, to create a facerec database from training images, you create a text file that lists the image files and which person each image file represents.For example, you could put this into a text file called "4_images_of_2_people.txt":1 Shervindata\Shervin\Shervin1.bmp1 Shervindata\Shervin\Shervin2.bmp1 Shervindata\Shervin\Shervin3.bmp1 Shervindata\Shervin\Shervin4.bmp2 Chandandata\Chandan\Chandan1.bmp2 Chandandata\Chandan\Chandan2.bmp2 Chandandata\Chandan\Chandan3.bmp2 Chandandata\Chandan\Chandan4.bmpThis will tell the program that person 1 is named "Shervin", and the 4 preprocessedfacial photos of Shervin are in the "data\Shervin" folder, and person 2 is called "Chandan" with 4 images in the "data\Chandan" folder. The program can then loaded them all into an array of images using the function "loadFaceImgArray()". Note that for simplicity, it doesn't allow spaces or special characters in the person's name, so you might want to enable this, or replace spaces in a person's name with underscores (such as Shervin_Emami).To create the database from these loaded images, you use OpenCV's "cvCalcEigenObjects()" and "cvEigenDecomposite()" functions, eg:// Tell PCA to quit when it has enough eigenfaces.CvTermCriteria calcLimit = cvTermCriteria( CV_TERMCRIT_ITER, nEigens, 1);// Compute average image, eigenvectors (eigenfaces) and eigenvalues (ratios).cvCalcEigenObjects(nTrainFaces, (void*)faceImgArr, (void*)eigenVectArr, CV_EIGOBJ_NO_CALLBACK, 0, 0, &calcLimit,pAvgTrainImg, eigenValMat->data.fl);// Normalize the matrix of eigenvalues.cvNormalize(eigenValMat, eigenValMat, 1, 0, CV_L1, 0);// Project each training image onto the PCA subspace.CvMat projectedTrainFaceMat = cvCreateMat( nTrainFaces, nEigens, CV_32FC1 );int offset = projectedTrainFaceMat->step / sizeof(float);for(int i=0; i<nTrainFaces; i++) {cvEigenDecomposite(faceImgArr[i], nEigens, eigenVectArr, 0, 0,pAvgTrainImg, projectedTrainFaceMat->data.fl + i*offset);}You now have:the average image "pAvgTrainImg",the array of eigenface images "eigenVectArr[]" (eg: 200 eigenfaces if you used nEigens=200 training images),the matrix of eigenvalues (eigenface ratios) "projectedTrainFaceMat" of each training image.These can now be stored into a file, which will be the face recognition database. The function "storeTrainingData()" in the code will store this data into the file "facedata.xml", which can be reloaded anytime to recognize people that it has beentrained for. There is also a function "storeEigenfaceImages()" in the code, to generate the images shown earlier, of the average face image to "out_averageImage.bmp" and eigenfaces to "out_eigenfaces.bmp".Implementing Offline Recognition:For implementation of the offline recognition stage, where the face recognition system will try to recognize who is the face in several photos from a list in a text file, I am also using an extension of the Face Recognition with Eigenface implementation in Servo Magazine.The same sort of text file that is used for offline training can also be used for offline recognition. The text file lists the images that should be tested, as well as the correct person in that image. The program can then try to recognize who is in each photo, and check the correct value in the input file to see whether it was correct or not, for generating statistics of its own accuracy.The implementation of the offline face recognition is almost the same as offline training:The list of image files (preprocessed faces) and names are loaded into an array of images, from the text file that is now used for recognition testing (instead of training). This is performed in code by "loadFaceImgArray()".The average face, eigenfaces and eigenvalues (ratios) are loaded from the face recognition database file "facedata.xml", by the function "loadTrainingData()".Each input image is projected onto the PCA subspace using the OpenCV function "cvEigenDecomposite()", to see what ratio of eigenfaces is best for representing this input image.But now that it has the eigenvalues (ratios of eigenface images) to represent the input image, it looks for the original training image that had the most similar ratios. This is done mathematically in the function "findNearestNeighbor()" using the "Euclidean Distance", but basically it checks how similar the input image is to each training image, and finds the most similar one: the one with the least distance in Euclidean Space. As mentioned in the Servo Magazine article, you might get better results if you use the Mahalanobis space (define USE_MAHALANOBIS_DISTANCE in the code).The distance between the input image and most similar training image is used to determine the "confidence" value, to be used as a guide of whether someone wasactually recognized or not. A confidence of 1.0 would mean a good match, and a confidence of 0.0 or negative would mean a bad match. But beware that the confidence formula I use in the code is just a very basic confidence metric that isn't necessarily too reliable, but I figured that most people would like to see a rough confidence value. You may find that it gives misleading values for your images and so you can disable it if you want (eg: set the confidence always to 1.0).Once it knows which training image is most similar to the input image, and assuming the confidence value is not too low (it should be atleast 0.6 or higher), then it has figured out who that person is, in other words, it has recognized that person! Implementing Realtime Recognition from a Camera:It is very easy to use a webcam stream as input to the face recognition system instead of a file list. Basically you just need to grab frames from a camera instead of from a file, and you run forever until the user wants to quit, instead of just running until the file list has run out. OpenCV provides the 'cvCreateCameraCapture()' function (also known as 'cvCaptureFromCAM()') for this.Grabbing frames from a webcam can be implemented easily using this function:// Grab the next camera frame. Waits until the next frame is ready, and // provides direct access to it, so do NOT modify or free the returned image! // Will automatically initialize the camera on the first frame.IplImage* getCameraFrame(CvCapture* &camera){IplImage *frame;int w, h;// If the camera hasn't been initialized, then open it.if (!camera) {printf("Acessing the camera ...\n");camera = cvCreateCameraCapture( 0 );if (!camera) {printf("Couldn't access the camera.\n");exit(1);}// Try to set the camera resolution to 320 x 240.cvSetCaptureProperty(camera, CV_CAP_PROP_FRAME_WIDTH, 320);cvSetCaptureProperty(camera, CV_CAP_PROP_FRAME_HEIGHT, 240);// Get the first frame, to make sure the camera is initialized.frame = cvQueryFrame( camera );if (frame) {w = frame->width;h = frame->height;printf("Got the camera at %dx%d resolution.\n", w, h);}// Wait a little, so that the camera can auto-adjust its brightness.Sleep(1000); // (in milliseconds)}// Wait until the next camera frame is ready, then grab it.frame = cvQueryFrame( camera );if (!frame) {printf("Couldn't grab a camera frame.\n");exit(1);}return frame;}This function can be used like this:CvCapture* camera = 0; // The camera device.while ( cvWaitKey(10) != 27 ) { // Quit on "Escape" key.IplImage *frame = getCameraFrame(camera);...}// Free the camera.cvReleaseCapture( &camera );Note that if you are developing for MS Windows, you can grab camera frames twice as fast as this code by using the videoInput Library v0.1995 by Theo Watson. It uses hardware-accelerated DirectShow, whereas OpenCV uses VFW that hasn't changed in 15 years!Putting together all the parts that I have explained so far, the face recognition systemruns as follows:Grab a frame from the camera (as I mentioned here).Convert the color frame to greyscale (as I mentioned here).Detect a face within the greyscale camera frame (as I mentioned here).Crop the frame to just show the face region (using cvSetImageROI() and cvCopyImage()).Preprocess the face image (as I mentioned here).Recognize the person in the image (as I mentioned here).Implementing Online Training from a Camera:Now you have a way to recognize people in realtime using a camera, but to learn new faces you would have to shutdown the program, save the camera images as image files, update the training images list, use the offline training method from the command-line, and then run the program again in realtime camera mode. So in fact, this is exactly what you can do programmatically to perform online training from a camera in realtime!So here is the easiest way to add a new person to the face recognition database from the camera stream without shutting down the program:Collect a bunch of photos from the camera (preprocessed facial images), possibly while you are performing face recognition also.Save the collected face images as image files onto the hard-disk using cvSaveImage().Add the filename of each face image onto the end of the training images list file (the text file that is used for offline training).Once you are ready for online training the new images (such as once you have 20 faces, or when the user says that they are ready), you "retrain" the database from all the image files. The text file listing the training image files has the new images added to it, and the images are stored as image files on the computer, so online training works just like it did in offline training.But before retraining, it is important to free any resources that were being used, and re-initialize the variables, so that it behaves as if you shutdown the program and restarted. For example, after the images are stored as files and added to the training list text file, you should free the arrays of eigenfaces, before doing the equivalent of offline training (which involves loading all the images from the training list file, then findingthe eigenfaces and ratios of the new training set using PCA).This method of online training is fairly inefficient, because if there was 50 people in the training set and you add one more person, then it will train again for all 51 people, which is bad because the amount of time for training is exponential with more users or training images. But if you are just dealing with a few hundred training images in total then it shouldn't take more than a few seconds.Download OnlineFaceRec:The software and source-code is available here (open-source freeware), to use on Windows, Mac, Linux, iPhone, etc as you wish for educational or personal purposes, but NOT for commercial, criminal-detection, or military purposes (because this code is way too simple & unreliable for critical applications such as criminal detection, and also I no longer support any military).Click here to download "OnlineFaceRec" for Windows: onlineFaceRec.zip(0.07MB file including C/C++ source code, VS2008 project files and the compiled Win32 program, created 4th Feb 2012).Click here to download "OnlineFaceRec" for Linux: onlineFaceRec_Linux.zip(0.003MB file including C/C++ source code and a compiled Linux program, created 30th Dec 2011).If you dont have the OpenCV 2.0 SDK then you can just get the Win32 DLLs and HaarCascade for running this program (including 'cvaux200.dll' and 'haarcascade_frontalface_alt.xml'): onlineFaceRec_OpenCVbinaries.7z (1.7MB 7-Zip file).And if you want to run the program but dont have the Visual Studio 2008 runtime installed then you can just get the Win32 DLLs ('msvcr90.dll', etc): MS_VC90_CRT.7z (0.4MB 7-Zip file).To open Zip or 7z files you can use the freeware 7-Zip program (better than WinZip and WinRar in my opinion) from HERE.The code was tested with MS Visual Studio 2008 using OpenCV v2.0 and on Linux with GCC 4.2 using OpenCV v2.3.1, but I assume it works with other versions & compilers fairly easily, and it should work the same in all versions of OpenCV before v2.2. Students also ported this code to Dev-C++ athttps:///projects/facerec/.There are two different ways you can use this system:As a realtime program that performs face detection and online face recognition from a web camera.As a command-line program to perform offline face recognition using text files, just like the eigenface program in Servo Magazine.How to use the realtime webcam FaceRec system:If you have a webcam plugged in, then you should be able to test this program by just double-clicking the EXE file in Windows (or compile the code and run it if you are using Linux or Mac). Hit the Escape key on the GUI window when you want to quit the program.After a few seconds it should show the camera image, with the detected face highlighted. But at first it wont have anyone in its face rec database, so you will need to create it by entering a few keys. Beware that to use the keyboard, you have to click on the DOS console window before typing anything (because if the OpenCV window is highlighted then the code wont know what you typed).In the console window, hit the 'n' key on your keyboard when a person is ready for training. This will add a new person to the facerec database. Type in the person's name (without any spaces) and hit Enter.It will begin to automatically store all the processed frontal faces that it sees. Get a person to move their head around a bit until it has stored about 20 faces of them. (The facial images are stored as PGM files in the "data" folder, and their names are appended to the text file "train.txt").Get the person in front of the camera to move around a little and move their face a little, so that there will be some variance in the training images.Then when you have enough detected faces for that person, ideally more than 30 for each person, hit the 't' key in the console window to begin training on the images that were just collected. It will then pause for about 5-30 seconds (depending on how many faces and people are in the database), and finally continue once it has retrained with the extra person. The database file is called "facedata.xml".It should print the person's name in the console whenever it recognizes them. Repeat this again from step 1 whenever you want to add a new person, even after you have shutdown the program.。
基于深度卷积神经网络的人脸表情识别方法
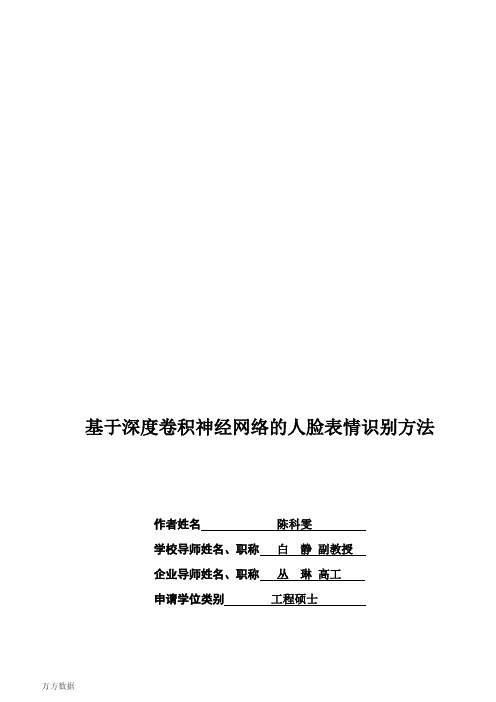
作者姓名
陈科雯
学校导师姓名、职称
丛 琳 高工
申请学位类别
万方数据
工程硕士
万方数据
学校代码
10701
学 号
分 类 号
TP39
密 级
1402121191
公开
西安电子科技大学
硕士学位论文
基于深度卷积神经网络的人脸表情识别方法
作者姓名:陈科雯
performance of various applications for the field has brought new breakthroughs and
development prospects. Different from the traditional method of robot learning, the depth
与深度卷积神经网络表情特征的表情识别网路(LBP-DCNN),将 LBP 算子进行与表
情特征区域相匹配的运算规则改进,并通过对比实验得到最优性能的卷积神经网络,
然后对表情图像提取改进的 LBP 特征,然后在训练一个 8 层的深度卷积神经网络提
取表情深度抽象特征,对抽象特征与改进的 LBP 特征进行融合,训练一个七分类的
expression recognition and to improve the separability of the extracted facial features, a
fusion of improved LBP expression features and deep convolution (LBP-DCNN), the LBP
convolution neural network is applied to facial expression recognition to study the feature.
- 1、下载文档前请自行甄别文档内容的完整性,平台不提供额外的编辑、内容补充、找答案等附加服务。
- 2、"仅部分预览"的文档,不可在线预览部分如存在完整性等问题,可反馈申请退款(可完整预览的文档不适用该条件!)。
- 3、如文档侵犯您的权益,请联系客服反馈,我们会尽快为您处理(人工客服工作时间:9:00-18:30)。
1. Introቤተ መጻሕፍቲ ባይዱuction
Of the appearance based face recognition methods [4][5][12][16][26][29], those utilizing LDA techniques [11][10][28][35] have shown promising results. However, statistical learning methods including the LDA based ones often suffer from the so called small sample size (SSS) problem encountered in high dimensional pattern recognition tasks where the number of training samples available for each subject is smaller than the dimensionality of the sample space. Therefore numerous modified versions of the LDA were proposed. These modified versions have shown promising results as it is demonstrated in [3][34][6] [30][20][23][33]. There are two ways to address the problem. One option is to apply linear algebra techniques to solve the numerical problem of inverting the singular within class scatter matrix. For example, Tian et al., utilize the pseudo inverse to complete this task. Also, some researchers [15][34] recommended the addition of a small perturbation to the within class scatter matrix so that it becomes nonsingular. However, the above methods typically computationally expensive since the scatter matrices are very large. The second option is a subspace approach, such as the one followed in the development of the Fisherfaces method [3], where PCA is firstly used as a preprocessing step to remove the null space of within class scatter matrix and then LDA is performed in the lower dimensional PCA subspace. However, it has been shown that the discarded null spaces may contain significant discriminatory information [19] to prevent this from happening solutions without a separate PCA step, called direct LDA methods have been proposed recently in [6][30][23]. Although successful in many cases, linear methods fail to deliver good performance when face patterns are subject to large variations in view points, which result in a highly non convex and complex distribution. The limited success of these methods should be attributed to their linear nature. As a result, it is reasonable to assume that better solution to this non linear problem could be achieved using non linear methods, such as the so called kernel machine techniques [17][25]. Among them, kernel principal component analysis (KPCA)[27] and kernel Fisher discriminant analysis (KFD)[24] have aroused considerable interest in the fields of pattern recognition and machine learning. KPCA was originally developed by Scholkopf et al., in 1998, while KFD was first proposed by Mika et al., in 1999 [24]. Subsequent research saw the development of series of KFD algorithms [24][2][31][35][32]. The defining characteristic of KFD based algorithms is that they directly use the pixel intensity values in a face image as the features on which to base the recognition decision. The pixel intensities that are used as features are represented using single valued variables. However, in many situations same face is captured in different orientation, lighting, expression and background, which lead to image variations. The pixel intensities do change because of image variations. The use of single valued variables may not be able to capture the variation of feature values of the images of the same subject. In such a case, we need to consider the symbolic data analysis (SDA) [1][7[8][9][18], in which the interval-valued data are analyzed.
In this paper, new appearance based method is proposed in the framework of Symbolic Data Analysis (SDA) [1][9], namely, symbolic KDA for face recognition, which are generalization of the classical KDA to symbolic objects. In the first step, we represent the face images as symbolic objects (symbolic faces) of interval type variables. The representation of face images as symbolic faces accounts for image variations of human faces under different lighting conditions, orientation and facial expression. It also drastically reduces the dimension of the image space without losing a significant amount of information. E ach symb olic face summarizing the variation of feature values through the different images of the same subject. In the second step, we applied symbolic KDA algorithm to extract interval type non-linear discriminating features. According to this algorithm, In the first phase, we applied kernel function to symbolic faces, as a result a pattern in the original input space is mapped into a potentially much higher dimensional feature vector in the feature space, and then perform in the feature space to choose subspace dimension carefully. In the second phase, Symbolic KDA is applied to obtain interval type non-linear discriminating features, which are robust to variations due to illumination, orientation and facial expression. Finally, minimum distance classifier with symbolic dissimilarity measure [1] is employed for classification. Proposed method has been successfully tested using two standard databases ORL and Yale face database. The remainder of this paper is organized as follows: In section 2, the idea of constructing the symbolic faces is given. Symbolic KDA is developed in section 3. In section 4, the experiments are performed on the ORL and Yale face database whereby the proposed algorithm is evaluated and compared to other methods. Finally, a conclusion and dis cussion are offered in section 5.