Gatsby Computational Neuroscience Unit
人工智能领域中英文专有名词汇总
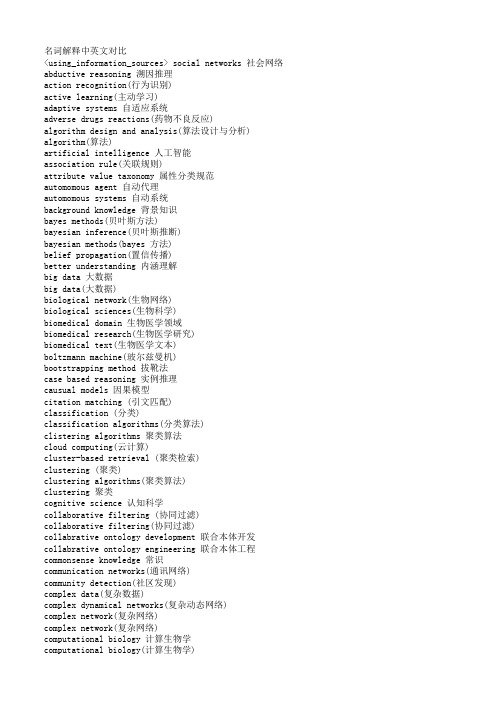
名词解释中英文对比<using_information_sources> social networks 社会网络abductive reasoning 溯因推理action recognition(行为识别)active learning(主动学习)adaptive systems 自适应系统adverse drugs reactions(药物不良反应)algorithm design and analysis(算法设计与分析) algorithm(算法)artificial intelligence 人工智能association rule(关联规则)attribute value taxonomy 属性分类规范automomous agent 自动代理automomous systems 自动系统background knowledge 背景知识bayes methods(贝叶斯方法)bayesian inference(贝叶斯推断)bayesian methods(bayes 方法)belief propagation(置信传播)better understanding 内涵理解big data 大数据big data(大数据)biological network(生物网络)biological sciences(生物科学)biomedical domain 生物医学领域biomedical research(生物医学研究)biomedical text(生物医学文本)boltzmann machine(玻尔兹曼机)bootstrapping method 拔靴法case based reasoning 实例推理causual models 因果模型citation matching (引文匹配)classification (分类)classification algorithms(分类算法)clistering algorithms 聚类算法cloud computing(云计算)cluster-based retrieval (聚类检索)clustering (聚类)clustering algorithms(聚类算法)clustering 聚类cognitive science 认知科学collaborative filtering (协同过滤)collaborative filtering(协同过滤)collabrative ontology development 联合本体开发collabrative ontology engineering 联合本体工程commonsense knowledge 常识communication networks(通讯网络)community detection(社区发现)complex data(复杂数据)complex dynamical networks(复杂动态网络)complex network(复杂网络)complex network(复杂网络)computational biology 计算生物学computational biology(计算生物学)computational complexity(计算复杂性) computational intelligence 智能计算computational modeling(计算模型)computer animation(计算机动画)computer networks(计算机网络)computer science 计算机科学concept clustering 概念聚类concept formation 概念形成concept learning 概念学习concept map 概念图concept model 概念模型concept modelling 概念模型conceptual model 概念模型conditional random field(条件随机场模型) conjunctive quries 合取查询constrained least squares (约束最小二乘) convex programming(凸规划)convolutional neural networks(卷积神经网络) customer relationship management(客户关系管理) data analysis(数据分析)data analysis(数据分析)data center(数据中心)data clustering (数据聚类)data compression(数据压缩)data envelopment analysis (数据包络分析)data fusion 数据融合data generation(数据生成)data handling(数据处理)data hierarchy (数据层次)data integration(数据整合)data integrity 数据完整性data intensive computing(数据密集型计算)data management 数据管理data management(数据管理)data management(数据管理)data miningdata mining 数据挖掘data model 数据模型data models(数据模型)data partitioning 数据划分data point(数据点)data privacy(数据隐私)data security(数据安全)data stream(数据流)data streams(数据流)data structure( 数据结构)data structure(数据结构)data visualisation(数据可视化)data visualization 数据可视化data visualization(数据可视化)data warehouse(数据仓库)data warehouses(数据仓库)data warehousing(数据仓库)database management systems(数据库管理系统)database management(数据库管理)date interlinking 日期互联date linking 日期链接Decision analysis(决策分析)decision maker 决策者decision making (决策)decision models 决策模型decision models 决策模型decision rule 决策规则decision support system 决策支持系统decision support systems (决策支持系统) decision tree(决策树)decission tree 决策树deep belief network(深度信念网络)deep learning(深度学习)defult reasoning 默认推理density estimation(密度估计)design methodology 设计方法论dimension reduction(降维) dimensionality reduction(降维)directed graph(有向图)disaster management 灾害管理disastrous event(灾难性事件)discovery(知识发现)dissimilarity (相异性)distributed databases 分布式数据库distributed databases(分布式数据库) distributed query 分布式查询document clustering (文档聚类)domain experts 领域专家domain knowledge 领域知识domain specific language 领域专用语言dynamic databases(动态数据库)dynamic logic 动态逻辑dynamic network(动态网络)dynamic system(动态系统)earth mover's distance(EMD 距离) education 教育efficient algorithm(有效算法)electric commerce 电子商务electronic health records(电子健康档案) entity disambiguation 实体消歧entity recognition 实体识别entity recognition(实体识别)entity resolution 实体解析event detection 事件检测event detection(事件检测)event extraction 事件抽取event identificaton 事件识别exhaustive indexing 完整索引expert system 专家系统expert systems(专家系统)explanation based learning 解释学习factor graph(因子图)feature extraction 特征提取feature extraction(特征提取)feature extraction(特征提取)feature selection (特征选择)feature selection 特征选择feature selection(特征选择)feature space 特征空间first order logic 一阶逻辑formal logic 形式逻辑formal meaning prepresentation 形式意义表示formal semantics 形式语义formal specification 形式描述frame based system 框为本的系统frequent itemsets(频繁项目集)frequent pattern(频繁模式)fuzzy clustering (模糊聚类)fuzzy clustering (模糊聚类)fuzzy clustering (模糊聚类)fuzzy data mining(模糊数据挖掘)fuzzy logic 模糊逻辑fuzzy set theory(模糊集合论)fuzzy set(模糊集)fuzzy sets 模糊集合fuzzy systems 模糊系统gaussian processes(高斯过程)gene expression data 基因表达数据gene expression(基因表达)generative model(生成模型)generative model(生成模型)genetic algorithm 遗传算法genome wide association study(全基因组关联分析) graph classification(图分类)graph classification(图分类)graph clustering(图聚类)graph data(图数据)graph data(图形数据)graph database 图数据库graph database(图数据库)graph mining(图挖掘)graph mining(图挖掘)graph partitioning 图划分graph query 图查询graph structure(图结构)graph theory(图论)graph theory(图论)graph theory(图论)graph theroy 图论graph visualization(图形可视化)graphical user interface 图形用户界面graphical user interfaces(图形用户界面)health care 卫生保健health care(卫生保健)heterogeneous data source 异构数据源heterogeneous data(异构数据)heterogeneous database 异构数据库heterogeneous information network(异构信息网络) heterogeneous network(异构网络)heterogenous ontology 异构本体heuristic rule 启发式规则hidden markov model(隐马尔可夫模型)hidden markov model(隐马尔可夫模型)hidden markov models(隐马尔可夫模型) hierarchical clustering (层次聚类) homogeneous network(同构网络)human centered computing 人机交互技术human computer interaction 人机交互human interaction 人机交互human robot interaction 人机交互image classification(图像分类)image clustering (图像聚类)image mining( 图像挖掘)image reconstruction(图像重建)image retrieval (图像检索)image segmentation(图像分割)inconsistent ontology 本体不一致incremental learning(增量学习)inductive learning (归纳学习)inference mechanisms 推理机制inference mechanisms(推理机制)inference rule 推理规则information cascades(信息追随)information diffusion(信息扩散)information extraction 信息提取information filtering(信息过滤)information filtering(信息过滤)information integration(信息集成)information network analysis(信息网络分析) information network mining(信息网络挖掘) information network(信息网络)information processing 信息处理information processing 信息处理information resource management (信息资源管理) information retrieval models(信息检索模型) information retrieval 信息检索information retrieval(信息检索)information retrieval(信息检索)information science 情报科学information sources 信息源information system( 信息系统)information system(信息系统)information technology(信息技术)information visualization(信息可视化)instance matching 实例匹配intelligent assistant 智能辅助intelligent systems 智能系统interaction network(交互网络)interactive visualization(交互式可视化)kernel function(核函数)kernel operator (核算子)keyword search(关键字检索)knowledege reuse 知识再利用knowledgeknowledgeknowledge acquisitionknowledge base 知识库knowledge based system 知识系统knowledge building 知识建构knowledge capture 知识获取knowledge construction 知识建构knowledge discovery(知识发现)knowledge extraction 知识提取knowledge fusion 知识融合knowledge integrationknowledge management systems 知识管理系统knowledge management 知识管理knowledge management(知识管理)knowledge model 知识模型knowledge reasoningknowledge representationknowledge representation(知识表达) knowledge sharing 知识共享knowledge storageknowledge technology 知识技术knowledge verification 知识验证language model(语言模型)language modeling approach(语言模型方法) large graph(大图)large graph(大图)learning(无监督学习)life science 生命科学linear programming(线性规划)link analysis (链接分析)link prediction(链接预测)link prediction(链接预测)link prediction(链接预测)linked data(关联数据)location based service(基于位置的服务) loclation based services(基于位置的服务) logic programming 逻辑编程logical implication 逻辑蕴涵logistic regression(logistic 回归)machine learning 机器学习machine translation(机器翻译)management system(管理系统)management( 知识管理)manifold learning(流形学习)markov chains 马尔可夫链markov processes(马尔可夫过程)matching function 匹配函数matrix decomposition(矩阵分解)matrix decomposition(矩阵分解)maximum likelihood estimation(最大似然估计)medical research(医学研究)mixture of gaussians(混合高斯模型)mobile computing(移动计算)multi agnet systems 多智能体系统multiagent systems 多智能体系统multimedia 多媒体natural language processing 自然语言处理natural language processing(自然语言处理) nearest neighbor (近邻)network analysis( 网络分析)network analysis(网络分析)network analysis(网络分析)network formation(组网)network structure(网络结构)network theory(网络理论)network topology(网络拓扑)network visualization(网络可视化)neural network(神经网络)neural networks (神经网络)neural networks(神经网络)nonlinear dynamics(非线性动力学)nonmonotonic reasoning 非单调推理nonnegative matrix factorization (非负矩阵分解) nonnegative matrix factorization(非负矩阵分解) object detection(目标检测)object oriented 面向对象object recognition(目标识别)object recognition(目标识别)online community(网络社区)online social network(在线社交网络)online social networks(在线社交网络)ontology alignment 本体映射ontology development 本体开发ontology engineering 本体工程ontology evolution 本体演化ontology extraction 本体抽取ontology interoperablity 互用性本体ontology language 本体语言ontology mapping 本体映射ontology matching 本体匹配ontology versioning 本体版本ontology 本体论open government data 政府公开数据opinion analysis(舆情分析)opinion mining(意见挖掘)opinion mining(意见挖掘)outlier detection(孤立点检测)parallel processing(并行处理)patient care(病人医疗护理)pattern classification(模式分类)pattern matching(模式匹配)pattern mining(模式挖掘)pattern recognition 模式识别pattern recognition(模式识别)pattern recognition(模式识别)personal data(个人数据)prediction algorithms(预测算法)predictive model 预测模型predictive models(预测模型)privacy preservation(隐私保护)probabilistic logic(概率逻辑)probabilistic logic(概率逻辑)probabilistic model(概率模型)probabilistic model(概率模型)probability distribution(概率分布)probability distribution(概率分布)project management(项目管理)pruning technique(修剪技术)quality management 质量管理query expansion(查询扩展)query language 查询语言query language(查询语言)query processing(查询处理)query rewrite 查询重写question answering system 问答系统random forest(随机森林)random graph(随机图)random processes(随机过程)random walk(随机游走)range query(范围查询)RDF database 资源描述框架数据库RDF query 资源描述框架查询RDF repository 资源描述框架存储库RDF storge 资源描述框架存储real time(实时)recommender system(推荐系统)recommender system(推荐系统)recommender systems 推荐系统recommender systems(推荐系统)record linkage 记录链接recurrent neural network(递归神经网络) regression(回归)reinforcement learning 强化学习reinforcement learning(强化学习)relation extraction 关系抽取relational database 关系数据库relational learning 关系学习relevance feedback (相关反馈)resource description framework 资源描述框架restricted boltzmann machines(受限玻尔兹曼机) retrieval models(检索模型)rough set theroy 粗糙集理论rough set 粗糙集rule based system 基于规则系统rule based 基于规则rule induction (规则归纳)rule learning (规则学习)rule learning 规则学习schema mapping 模式映射schema matching 模式匹配scientific domain 科学域search problems(搜索问题)semantic (web) technology 语义技术semantic analysis 语义分析semantic annotation 语义标注semantic computing 语义计算semantic integration 语义集成semantic interpretation 语义解释semantic model 语义模型semantic network 语义网络semantic relatedness 语义相关性semantic relation learning 语义关系学习semantic search 语义检索semantic similarity 语义相似度semantic similarity(语义相似度)semantic web rule language 语义网规则语言semantic web 语义网semantic web(语义网)semantic workflow 语义工作流semi supervised learning(半监督学习)sensor data(传感器数据)sensor networks(传感器网络)sentiment analysis(情感分析)sentiment analysis(情感分析)sequential pattern(序列模式)service oriented architecture 面向服务的体系结构shortest path(最短路径)similar kernel function(相似核函数)similarity measure(相似性度量)similarity relationship (相似关系)similarity search(相似搜索)similarity(相似性)situation aware 情境感知social behavior(社交行为)social influence(社会影响)social interaction(社交互动)social interaction(社交互动)social learning(社会学习)social life networks(社交生活网络)social machine 社交机器social media(社交媒体)social media(社交媒体)social media(社交媒体)social network analysis 社会网络分析social network analysis(社交网络分析)social network(社交网络)social network(社交网络)social science(社会科学)social tagging system(社交标签系统)social tagging(社交标签)social web(社交网页)sparse coding(稀疏编码)sparse matrices(稀疏矩阵)sparse representation(稀疏表示)spatial database(空间数据库)spatial reasoning 空间推理statistical analysis(统计分析)statistical model 统计模型string matching(串匹配)structural risk minimization (结构风险最小化) structured data 结构化数据subgraph matching 子图匹配subspace clustering(子空间聚类)supervised learning( 有support vector machine 支持向量机support vector machines(支持向量机)system dynamics(系统动力学)tag recommendation(标签推荐)taxonmy induction 感应规范temporal logic 时态逻辑temporal reasoning 时序推理text analysis(文本分析)text anaylsis 文本分析text classification (文本分类)text data(文本数据)text mining technique(文本挖掘技术)text mining 文本挖掘text mining(文本挖掘)text summarization(文本摘要)thesaurus alignment 同义对齐time frequency analysis(时频分析)time series analysis( 时time series data(时间序列数据)time series data(时间序列数据)time series(时间序列)topic model(主题模型)topic modeling(主题模型)transfer learning 迁移学习triple store 三元组存储uncertainty reasoning 不精确推理undirected graph(无向图)unified modeling language 统一建模语言unsupervisedupper bound(上界)user behavior(用户行为)user generated content(用户生成内容)utility mining(效用挖掘)visual analytics(可视化分析)visual content(视觉内容)visual representation(视觉表征)visualisation(可视化)visualization technique(可视化技术) visualization tool(可视化工具)web 2.0(网络2.0)web forum(web 论坛)web mining(网络挖掘)web of data 数据网web ontology lanuage 网络本体语言web pages(web 页面)web resource 网络资源web science 万维科学web search (网络检索)web usage mining(web 使用挖掘)wireless networks 无线网络world knowledge 世界知识world wide web 万维网world wide web(万维网)xml database 可扩展标志语言数据库附录 2 Data Mining 知识图谱(共包含二级节点15 个,三级节点93 个)间序列分析)监督学习)领域 二级分类 三级分类。
Semi-Supervised Learning Using Gaussian Fields and Harmonic Functions

Xiaojin Zhu ZHUXJ@ Zoubin Ghahramani ZOUBIN@ John Lafferty LAFFERTY@ School of Computer Science,Carnegie Mellon University,Pittsburgh PA15213,USAGatsby Computational Neuroscience Unit,University College London,London WC1N3AR,UKAbstractAn approach to semi-supervised learning is pro-posed that is based on a Gaussian randomfieldbeled and unlabeled data are rep-resented as vertices in a weighted graph,withedge weights encoding the similarity between in-stances.The learning problem is then formulatedin terms of a Gaussian randomfield on this graph,where the mean of thefield is characterized interms of harmonic functions,and is efficientlyobtained using matrix methods or belief propa-gation.The resulting learning algorithms haveintimate connections with random walks,elec-tric networks,and spectral graph theory.We dis-cuss methods to incorporate class priors and thepredictions of classifiers obtained by supervisedlearning.We also propose a method of parameterlearning by entropy minimization,and show thealgorithm’s ability to perform feature selection.Promising experimental results are presented forsynthetic data,digit classification,and text clas-sification tasks.1.IntroductionIn many traditional approaches to machine learning,a tar-get function is estimated using labeled data,which can be thought of as examples given by a“teacher”to a“student.”Labeled examples are often,however,very time consum-ing and expensive to obtain,as they require the efforts of human annotators,who must often be quite skilled.For in-stance,obtaining a single labeled example for protein shape classification,which is one of the grand challenges of bio-logical and computational science,requires months of ex-pensive analysis by expert crystallographers.The problem of effectively combining unlabeled data with labeled data is therefore of central importance in machine learning.The semi-supervised learning problem has attracted an in-creasing amount of interest recently,and several novel ap-proaches have been proposed;we refer to(Seeger,2001) for an overview.Among these methods is a promising fam-ily of techniques that exploit the“manifold structure”of the data;such methods are generally based upon an assumption that similar unlabeled examples should be given the same classification.In this paper we introduce a new approach to semi-supervised learning that is based on a randomfield model defined on a weighted graph over the unlabeled and labeled data,where the weights are given in terms of a sim-ilarity function between instances.Unlike other recent work based on energy minimization and randomfields in machine learning(Blum&Chawla, 2001)and image processing(Boykov et al.,2001),we adopt Gaussianfields over a continuous state space rather than randomfields over the discrete label set.This“re-laxation”to a continuous rather than discrete sample space results in many attractive properties.In particular,the most probable configuration of thefield is unique,is character-ized in terms of harmonic functions,and has a closed form solution that can be computed using matrix methods or loopy belief propagation(Weiss et al.,2001).In contrast, for multi-label discrete randomfields,computing the low-est energy configuration is typically NP-hard,and approxi-mation algorithms or other heuristics must be used(Boykov et al.,2001).The resulting classification algorithms for Gaussianfields can be viewed as a form of nearest neigh-bor approach,where the nearest labeled examples are com-puted in terms of a random walk on the graph.The learning methods introduced here have intimate connections with random walks,electric networks,and spectral graph the-ory,in particular heat kernels and normalized cuts.In our basic approach the solution is solely based on the structure of the data manifold,which is derived from data features.In practice,however,this derived manifold struc-ture may be insufficient for accurate classification.WeProceedings of the Twentieth International Conference on Machine Learning(ICML-2003),Washington DC,2003.Figure1.The randomfields used in this work are constructed on labeled and unlabeled examples.We form a graph with weighted edges between instances(in this case scanned digits),with labeled data items appearing as special“boundary”points,and unlabeled points as“interior”points.We consider Gaussian randomfields on this graph.show how the extra evidence of class priors can help classi-fication in Section4.Alternatively,we may combine exter-nal classifiers using vertex weights or“assignment costs,”as described in Section5.Encouraging experimental re-sults for synthetic data,digit classification,and text clas-sification tasks are presented in Section7.One difficulty with the randomfield approach is that the right choice of graph is often not entirely clear,and it may be desirable to learn it from data.In Section6we propose a method for learning these weights by entropy minimization,and show the algorithm’s ability to perform feature selection to better characterize the data manifold.2.Basic FrameworkWe suppose there are labeled points, and unlabeled points;typically. Let be the total number of data points.To be-gin,we assume the labels are binary:.Consider a connected graph with nodes correspond-ing to the data points,with nodes corre-sponding to the labeled points with labels,and nodes corresponding to the unla-beled points.Our task is to assign labels to nodes.We assume an symmetric weight matrix on the edges of the graph is given.For example,when,the weight matrix can be(2)To assign a probability distribution on functions,we form the Gaussianfieldfor(3) which is consistent with our prior notion of smoothness of with respect to the graph.Expressed slightly differently, ,where.Because of the maximum principle of harmonic functions(Doyle&Snell,1984),is unique and is either a constant or it satisfiesfor.To compute the harmonic solution explicitly in terms of matrix operations,we split the weight matrix(and sim-ilarly)into4blocks after the th row and column:(4) Letting where denotes the values on the un-labeled data points,the harmonic solution subject to is given by(5)Figure2.Demonstration of harmonic energy minimization on twosynthetic rge symbols indicate labeled data,otherpoints are unlabeled.In this paper we focus on the above harmonic function as abasis for semi-supervised classification.However,we em-phasize that the Gaussian randomfield model from which this function is derived provides the learning frameworkwith a consistent probabilistic semantics.In the following,we refer to the procedure described aboveas harmonic energy minimization,to underscore the har-monic property(3)as well as the objective function being minimized.Figure2demonstrates the use of harmonic en-ergy minimization on two synthetic datasets.The leftfigure shows that the data has three bands,with,, and;the rightfigure shows two spirals,with,,and.Here we see harmonic energy minimization clearly follows the structure of data, while obviously methods such as kNN would fail to do so.3.Interpretation and ConnectionsAs outlined briefly in this section,the basic framework pre-sented in the previous section can be viewed in several fun-damentally different ways,and these different viewpoints provide a rich and complementary set of techniques for rea-soning about this approach to the semi-supervised learning problem.3.1.Random Walks and Electric NetworksImagine a particle walking along the graph.Starting from an unlabeled node,it moves to a node with proba-bility after one step.The walk continues until the par-ticle hits a labeled node.Then is the probability that the particle,starting from node,hits a labeled node with label1.Here the labeled data is viewed as an“absorbing boundary”for the random walk.This view of the harmonic solution indicates that it is closely related to the random walk approach of Szummer and Jaakkola(2001),however there are two major differ-ences.First,wefix the value of on the labeled points, and second,our solution is an equilibrium state,expressed in terms of a hitting time,while in(Szummer&Jaakkola,2001)the walk crucially depends on the time parameter. We will return to this point when discussing heat kernels. An electrical network interpretation is given in(Doyle& Snell,1984).Imagine the edges of to be resistors with conductance.We connect nodes labeled to a positive voltage source,and points labeled to ground.Thenis the voltage in the resulting electric network on each of the unlabeled nodes.Furthermore minimizes the energy dissipation of the electric network for the given.The harmonic property here follows from Kirchoff’s and Ohm’s laws,and the maximum principle then shows that this is precisely the same solution obtained in(5).3.2.Graph KernelsThe solution can be viewed from the viewpoint of spec-tral graph theory.The heat kernel with time parameter on the graph is defined as.Here is the solution to the heat equation on the graph with initial conditions being a point source at at time.Kondor and Lafferty(2002)propose this as an appropriate kernel for machine learning with categorical data.When used in a kernel method such as a support vector machine,the kernel classifier can be viewed as a solution to the heat equation with initial heat sourceson the labeled data.The time parameter must,however, be chosen using an auxiliary technique,for example cross-validation.Our algorithm uses a different approach which is indepen-dent of,the diffusion time.Let be the lower right submatrix of.Since,it is the Laplacian restricted to the unlabeled nodes in.Consider the heat kernel on this submatrix:.Then describes heat diffusion on the unlabeled subgraph with Dirichlet boundary conditions on the labeled nodes.The Green’s function is the inverse operator of the restricted Laplacian,,which can be expressed in terms of the integral over time of the heat kernel:(6) The harmonic solution(5)can then be written asor(7)Expression(7)shows that this approach can be viewed as a kernel classifier with the kernel and a specific form of kernel machine.(See also(Chung&Yau,2000),where a normalized Laplacian is used instead of the combinatorial Laplacian.)From(6)we also see that the spectrum of is ,where is the spectrum of.This indicates a connection to the work of Chapelle et al.(2002),who ma-nipulate the eigenvalues of the Laplacian to create variouskernels.A related approach is given by Belkin and Niyogi (2002),who propose to regularize functions on by select-ing the top normalized eigenvectors of corresponding to the smallest eigenvalues,thus obtaining the bestfit toin the least squares sense.We remark that ourfits the labeled data exactly,while the order approximation may not.3.3.Spectral Clustering and Graph MincutsThe normalized cut approach of Shi and Malik(2000)has as its objective function the minimization of the Raleigh quotient(8)subject to the constraint.The solution is the second smallest eigenvector of the generalized eigenvalue problem .Yu and Shi(2001)add a grouping bias to the normalized cut to specify which points should be in the same group.Since labeled data can be encoded into such pairwise grouping constraints,this technique can be applied to semi-supervised learning as well.In general, when is close to block diagonal,it can be shown that data points are tightly clustered in the eigenspace spanned by thefirst few eigenvectors of(Ng et al.,2001a;Meila &Shi,2001),leading to various spectral clustering algo-rithms.Perhaps the most interesting and substantial connection to the methods we propose here is the graph mincut approach proposed by Blum and Chawla(2001).The starting point for this work is also a weighted graph,but the semi-supervised learning problem is cast as one offinding a minimum-cut,where negative labeled data is connected (with large weight)to a special source node,and positive labeled data is connected to a special sink node.A mini-mum-cut,which is not necessarily unique,minimizes the objective function,and label0other-wise.We call this rule the harmonic threshold(abbreviated “thresh”below).In terms of the random walk interpreta-tion,ifmakes sense.If there is reason to doubt this assumption,it would be reasonable to attach dongles to labeled nodes as well,and to move the labels to these new nodes.6.Learning the Weight MatrixPreviously we assumed that the weight matrix is given andfixed.In this section,we investigate learning weight functions of the form given by equation(1).We will learn the’s from both labeled and unlabeled data;this will be shown to be useful as a feature selection mechanism which better aligns the graph structure with the data.The usual parameter learning criterion is to maximize the likelihood of labeled data.However,the likelihood crite-rion is not appropriate in this case because the values for labeled data arefixed during training,and moreover likeli-hood doesn’t make sense for the unlabeled data because we do not have a generative model.We propose instead to use average label entropy as a heuristic criterion for parameter learning.The average label entropy of thefield is defined as(13) using the fact that.Both and are sub-matrices of.In the above derivation we use as label probabilities di-rectly;that is,class.If we incorpo-rate class prior information,or combine harmonic energy minimization with other classifiers,it makes sense to min-imize entropy on the combined probabilities.For instance, if we incorporate a class prior using CMN,the probability is given bylabeled set size a c c u r a c yFigure 3.Harmonic energy minimization on digits “1”vs.“2”(left)and on all 10digits (middle)and combining voted-perceptron with harmonic energy minimization on odd vs.even digits (right)Figure 4.Harmonic energy minimization on PC vs.MAC (left),baseball vs.hockey (middle),and MS-Windows vs.MAC (right)10trials.In each trial we randomly sample labeled data from the entire dataset,and use the rest of the images as unlabeled data.If any class is absent from the sampled la-beled set,we redo the sampling.For methods that incorpo-rate class priors ,we estimate from the labeled set with Laplace (“add one”)smoothing.We consider the binary problem of classifying digits “1”vs.“2,”with 1100images in each class.We report aver-age accuracy of the following methods on unlabeled data:thresh,CMN,1NN,and a radial basis function classifier (RBF)which classifies to class 1iff .RBF and 1NN are used simply as baselines.The results are shown in Figure 3.Clearly thresh performs poorly,because the values of are generally close to 1,so the major-ity of examples are classified as digit “1”.This shows the inadequacy of the weight function (1)based on pixel-wise Euclidean distance.However the relative rankings ofare useful,and when coupled with class prior information significantly improved accuracy is obtained.The greatest improvement is achieved by the simple method CMN.We could also have adjusted the decision threshold on thresh’s solution ,so that the class proportion fits the prior .This method is inferior to CMN due to the error in estimating ,and it is not shown in the plot.These same observations are also true for the experiments we performed on several other binary digit classification problems.We also consider the 10-way problem of classifying digits “0”through ’9’.We report the results on a dataset with in-tentionally unbalanced class sizes,with 455,213,129,100,754,970,275,585,166,353examples per class,respec-tively (noting that the results on a balanced dataset are sim-ilar).We report the average accuracy of thresh,CMN,RBF,and 1NN.These methods can handle multi-way classifica-tion directly,or with slight modification in a one-against-all fashion.As the results in Figure 3show,CMN again im-proves performance by incorporating class priors.Next we report the results of document categorization ex-periments using the 20newsgroups dataset.We pick three binary problems:PC (number of documents:982)vs.MAC (961),MS-Windows (958)vs.MAC,and base-ball (994)vs.hockey (999).Each document is minimally processed into a “tf.idf”vector,without applying header re-moval,frequency cutoff,stemming,or a stopword list.Two documents are connected by an edge if is among ’s 10nearest neighbors or if is among ’s 10nearest neigh-bors,as measured by cosine similarity.We use the follow-ing weight function on the edges:(16)We use one-nearest neighbor and the voted perceptron al-gorithm (Freund &Schapire,1999)(10epochs with a lin-ear kernel)as baselines–our results with support vector ma-chines are comparable.The results are shown in Figure 4.As before,each point is the average of10random tri-als.For this data,harmonic energy minimization performsmuch better than the baselines.The improvement from the class prior,however,is less significant.An explanation for why this approach to semi-supervised learning is so effec-tive on the newsgroups data may lie in the common use of quotations within a topic thread:document quotes partof document,quotes part of,and so on.Thus, although documents far apart in the thread may be quite different,they are linked by edges in the graphical repre-sentation of the data,and these links are exploited by the learning algorithm.7.1.Incorporating External ClassifiersWe use the voted-perceptron as our external classifier.For each random trial,we train a voted-perceptron on the la-beled set,and apply it to the unlabeled set.We then use the 0/1hard labels for dongle values,and perform harmonic energy minimization with(10).We use.We evaluate on the artificial but difficult binary problem of classifying odd digits vs.even digits;that is,we group “1,3,5,7,9”and“2,4,6,8,0”into two classes.There are400 images per digit.We use second order polynomial kernel in the voted-perceptron,and train for10epochs.Figure3 shows the results.The accuracy of the voted-perceptron on unlabeled data,averaged over trials,is marked VP in the plot.Independently,we run thresh and CMN.Next we combine thresh with the voted-perceptron,and the result is marked thresh+VP.Finally,we perform class mass nor-malization on the combined result and get CMN+VP.The combination results in higher accuracy than either method alone,suggesting there is complementary information used by each.7.2.Learning the Weight MatrixTo demonstrate the effects of estimating,results on a toy dataset are shown in Figure5.The upper grid is slightly tighter than the lower grid,and they are connected by a few data points.There are two labeled examples,marked with large symbols.We learn the optimal length scales for this dataset by minimizing entropy on unlabeled data.To simplify the problem,wefirst tie the length scales in the two dimensions,so there is only a single parameter to learn.As noted earlier,without smoothing,the entropy approaches the minimum at0as.Under such con-ditions,the results of harmonic energy minimization are usually undesirable,and for this dataset the tighter grid “invades”the sparser one as shown in Figure5(a).With smoothing,the“nuisance minimum”at0gradually disap-pears as the smoothing factor grows,as shown in FigureFigure5.The effect of parameter on harmonic energy mini-mization.(a)If unsmoothed,as,and the algorithm performs poorly.(b)Result at optimal,smoothed with(c)Smoothing helps to remove the entropy minimum. 5(c).When we set,the minimum entropy is0.898 bits at.Harmonic energy minimization under this length scale is shown in Figure5(b),which is able to dis-tinguish the structure of the two grids.If we allow a separate for each dimension,parameter learning is more dramatic.With the same smoothing of ,keeps growing towards infinity(we usefor computation)while stabilizes at0.65, and we reach a minimum entropy of0.619bits.In this case is legitimate;it means that the learning al-gorithm has identified the-direction as irrelevant,based on both the labeled and unlabeled data.Harmonic energy minimization under these parameters gives the same clas-sification as shown in Figure5(b).Next we learn’s for all256dimensions on the“1”vs.“2”digits dataset.For this problem we minimize the entropy with CMN probabilities(15).We randomly pick a split of 92labeled and2108unlabeled examples,and start with all dimensions sharing the same as in previous ex-periments.Then we compute the derivatives of for each dimension separately,and perform gradient descent to min-imize the entropy.The result is shown in Table1.As entropy decreases,the accuracy of CMN and thresh both increase.The learned’s shown in the rightmost plot of Figure6range from181(black)to465(white).A small (black)indicates that the weight is more sensitive to varia-tions in that dimension,while the opposite is true for large (white).We can discern the shapes of a black“1”and a white“2”in thisfigure;that is,the learned parametersCMNstart97.250.73%0.654298.020.39%Table1.Entropy of CMN and accuracies before and after learning ’s on the“1”vs.“2”dataset.Figure6.Learned’s for“1”vs.“2”dataset.From left to right: average“1”,average“2”,initial’s,learned’s.exaggerate variations within class“1”while suppressing variations within class“2”.We have observed that with the default parameters,class“1”has much less variation than class“2”;thus,the learned parameters are,in effect, compensating for the relative tightness of the two classes in feature space.8.ConclusionWe have introduced an approach to semi-supervised learn-ing based on a Gaussian randomfield model defined with respect to a weighted graph representing labeled and unla-beled data.Promising experimental results have been pre-sented for text and digit classification,demonstrating that the framework has the potential to effectively exploit the structure of unlabeled data to improve classification accu-racy.The underlying randomfield gives a coherent proba-bilistic semantics to our approach,but this paper has con-centrated on the use of only the mean of thefield,which is characterized in terms of harmonic functions and spectral graph theory.The fully probabilistic framework is closely related to Gaussian process classification,and this connec-tion suggests principled ways of incorporating class priors and learning hyperparameters;in particular,it is natural to apply evidence maximization or the generalization er-ror bounds that have been studied for Gaussian processes (Seeger,2002).Our work in this direction will be reported in a future publication.ReferencesBelkin,M.,&Niyogi,P.(2002).Using manifold structure for partially labelled classification.Advances in Neural Information Processing Systems,15.Blum,A.,&Chawla,S.(2001).Learning from labeled and unlabeled data using graph mincuts.Proc.18th Interna-tional Conf.on Machine Learning.Boykov,Y.,Veksler,O.,&Zabih,R.(2001).Fast approx-imate energy minimization via graph cuts.IEEE Trans. on Pattern Analysis and Machine Intelligence,23. Chapelle,O.,Weston,J.,&Sch¨o lkopf,B.(2002).Cluster kernels for semi-supervised learning.Advances in Neu-ral Information Processing Systems,15.Chung,F.,&Yau,S.(2000).Discrete Green’s functions. Journal of Combinatorial Theory(A)(pp.191–214). Doyle,P.,&Snell,J.(1984).Random walks and electric networks.Mathematical Assoc.of America. Freund,Y.,&Schapire,R.E.(1999).Large margin classi-fication using the perceptron algorithm.Machine Learn-ing,37(3),277–296.Hull,J.J.(1994).A database for handwritten text recog-nition research.IEEE Transactions on Pattern Analysis and Machine Intelligence,16.Kondor,R.I.,&Lafferty,J.(2002).Diffusion kernels on graphs and other discrete input spaces.Proc.19th Inter-national Conf.on Machine Learning.Le Cun,Y.,Boser, B.,Denker,J.S.,Henderson, D., Howard,R.E.,Howard,W.,&Jackel,L.D.(1990). Handwritten digit recognition with a back-propagation network.Advances in Neural Information Processing Systems,2.Meila,M.,&Shi,J.(2001).A random walks view of spec-tral segmentation.AISTATS.Ng,A.,Jordan,M.,&Weiss,Y.(2001a).On spectral clus-tering:Analysis and an algorithm.Advances in Neural Information Processing Systems,14.Ng,A.Y.,Zheng,A.X.,&Jordan,M.I.(2001b).Link analysis,eigenvectors and stability.International Joint Conference on Artificial Intelligence(IJCAI). Seeger,M.(2001).Learning with labeled and unlabeled data(Technical Report).University of Edinburgh. Seeger,M.(2002).PAC-Bayesian generalization error bounds for Gaussian process classification.Journal of Machine Learning Research,3,233–269.Shi,J.,&Malik,J.(2000).Normalized cuts and image segmentation.IEEE Transactions on Pattern Analysis and Machine Intelligence,22,888–905.Szummer,M.,&Jaakkola,T.(2001).Partially labeled clas-sification with Markov random walks.Advances in Neu-ral Information Processing Systems,14.Weiss,Y.,,&Freeman,W.T.(2001).Correctness of belief propagation in Gaussian graphical models of arbitrary topology.Neural Computation,13,2173–2200.Yu,S.X.,&Shi,J.(2001).Grouping with bias.Advances in Neural Information Processing Systems,14.。
有限单元法基础
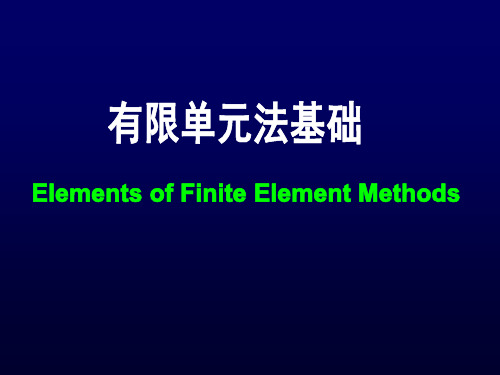
性体在各节点处的位移解。
3、单元分析---三角形单元
y
3.1 单元的结点位移和结点力向量
从离散化的网格中任取一个单元。三个结点 按反时针方向的顺序编号为:i, j, m。
结点坐标: (xi,yi) , (xj,yj) , (xm,ym) 结点位移: (ui,vi) , (uj,yj) , (um,vm) 共有6个自由度
单元位移插值函数: u(x, y) a1 a2 x a3 y
(3.1)
v(x, y) a4 a5x a6 y
插值函数的系数: a1 aiui a ju j amum / 2 A, a4 aivi a jv j amvm / 2 A,
a2 biui bju j bmum / 2 A, a5 bivi bjv j bmvm / 2 A,
um a1 a2 xm a3 ym , vm a4 a5 xm a6 ym ,
求解以上方程组得到以节点位移和节点坐标表示的6个参数:
a1 aiui a ju j amum / 2 A, a4 aivi a jv j amvm / 2 A, a2 biui bju j bmum / 2 A, a5 bivi bjv j bmvm / 2 A, a3 ciui c ju j cmum / 2 A, a6 civi c jv j cmvm / 2 A,
研究方法
从数学上讲它是微分方程边值问题(椭圆型微分方程、抛物型微分方程和双曲型微 分方程)的一种的数值解法,是一种将数学物理问题化为等价的变分问题的解法,并作 为一种通用的数值解法成为应用数学的一个重要分支。从物理上讲是将连续介质物理 场进行离散化,将无限自由度问题化为有限自由度问题的一种解方法。从固体力学上 认识,是瑞利-里兹法的推广。
信息管理与信息系统专业英语Unit1~6 TextB 课文翻译

管理的角色和技能管理角色亨利·明茨伯格对执行者行为的研究让他得出这样的结论:经理都需要承担大量的角色。
一个角色是一组预期的行为对一个特定的位置。
明茨伯格的角色可以分为三大类如图1.1所示:信息角色(管理信息);人际角色(管理通过人)和决策角色(管理行动)。
每个角色代表活动经理承担最终完成的功能规划、组织、领导、控制。
重要的是要记住,真正的工作的管理不能练习作为一组独立的部分;所有的角色交互的方式在现实世界的管理。
图1.1 管理角色信息角色描述活动用来维持和发展一个信息网络。
这三个信息角色监督者、传播者和发言人。
监督者涉及从许多来源寻求当前的信息。
经理获得信息来自他人和扫描书面材料来保持消息灵通。
传播者和发言人的角色是正好相反。
经理把当前信息传递给他人,内部和外部的组织,才能使用它。
与授权趋势的低级别员工,很多经理都共享尽可能丰富的信息。
由于人际角色让经理们被叫去与众多组织和个人交互。
这三个人际角色是挂名首脑、领袖和交流与合作者。
这个挂名首脑角色专注于管理正式的和象征性的活动的部门或组织。
经理代表本组织在他或她作为单位的负责人的正式管理能力。
领导的作用是指经理的工作在激励下属,以满足单位的目标。
交流与合作者的作用来自于经理的责任与各种团体在组织内外交流。
一个例子是一个面对面讨论控制器和计划主管之间解决关于预算的一种误解。
决策角色指管理的决策过程。
这些角色通常需要概念以及人类的技能。
这四种管理角色都属于这一类企业家,障碍处理者,资源分配者,谈判代表。
一个管理者承担一个企业家的角色当他或她启动项目来提高部门或工作单位时。
当问题比如错过了交付关键客户的出现,经理必须采用一个障碍处理的角色。
决定如何分配单位的金钱、时间、材料和其他资源,称为经理的资源分配角色。
最后,谈判者角色指的是这种情况,经理必须代表单位和其他人的利益,如供应商、客户和政府。
根据一篇经典文章由罗伯特·l·卡茨,管理上的成功主要取决于性能而不是人格特质。
神经科学核心辞汇翻译

精要速览系列(影印版)Instant Notes Neuroscience神经科学……………核心词汇翻译参考手册……………………按章节顺序:Section A Brain cellsA1 Neuron structureNeuron 神经细胞,神经元Subcellular organelles 亚细胞器Nissl body 尼氏体神经元中的粗面内质网形成的聚合物。
Neurite 神经突Axon 轴突Dendrite 树突Mitochondria 线粒体dendritic spines 树突脊树突在神经元上分化成几百个微小投射。
axon hillock 轴丘myelin sheath 髓鞘axon collalterals 轴突分枝terminals 突触末稍varicosities 曲张体microtubules 微管cytoskeleton 细胞骨架A2 Classes and numbers of neuronsMorphology 形态学Neurotranmitters 神经递质Unipolar 单极神经元仅有一个树突的神经元。
Bipolar 双极神经元Multipolar 多极神经元Pseudounipolar 假单极神经元生长出两个神经突,但随后融合Pyramidal cell 锥体细胞Purkinje cell 浦肯野氏细胞Projection neuron 投射神经元拥有长轴突的神经元Interneurons 中间神经元拥有短的轴突的神经元Afferent 传入Efferent 传出Sensory neuron 感觉神经元Motor neuron 运动神经元A3 Morphology of chemical synapseselectrical synapse 电突触chemical synapse 化学突触synaptic cleft 突触间隙axodendritic synapse 轴树突触轴突与树突之间的突触axosomatic synapse 轴体突触axoaxonal synapse 轴轴突触small clear synaptic vesicles(SSVs) 小清楚突触囊泡在突触前神经元存在的贮存递质的囊泡dense projections 致密突起active zone 活性区域postsynaptic density 突触致密物质large dense-core vesicles 庞大致密度中心囊泡A4 glial cells and myelinationGlial cells 胶质细胞是神经细胞的辅助细胞Astrocytes 星状细胞Oligodendrocytes 寡突细胞Schwann cells 许旺氏细胞围绕在神经细胞外的一种胶质细胞,形成髓鞘。
常用学术网址与链接(用于收集资料熟悉研究方向)(常更新)(发给研究生)

常用学术网址链接(用于收集资料熟悉研究方向)论坛部落类:1、小木虫论坛:/bbs/2、研学论坛/index.jsp3、子午学术论坛/bbs/index.php4、零点花园(内有大量基金报告)/bbs/5、科研基金网/6、5Q部落: 7、博士部落(包括求职、职务描绘、创业、科研资料、课题、论文、外语、计算机等,网页上推荐了不少好网站)8天下论坛9、清华BBS:10、科苑星空BBS:11、博研联盟/index.html(博士、博士后信息)12、源代码下载与搜索网站/(天空软件)13、软件性能分析程序VTune:分析在Intel芯片上运行的C或Fortran程序(高永华推荐)14、高校课件:15、FFTW: /用C语言编写的快速傅立叶变换程序(Fastest Fourier Transform in the West), 库文件及头文件放在E:\fftw. 使用时需将之拷贝至C:\Windows\system32研究机构与学者主页类:1.加州大学佰克利分校计算机系:2.北京邮电大学主页:有一些关于通信会议的信息及动态3.SVM用于语音识别[Mississippi state university institute for signal and information processing] Aravind ganapathiraju,Jonathan4.在北大bbs 上的语音处理:/学术讨论/语音语言处理5.Boosting 机器学习技术6.核ICA (另有机器学习的一些资源)7.郭天佑Tin-you KWOKt.hk/~jamesk朱海龙(博士)http://202.117.29.24/grzhy/zhuhailong/links.htm8.wiley出版社Springer出版社http://springer.de9.Christopher M.Bishop的主页/~cmbishop关于统计模式识别,写了一本书《Neural Networks for Pattern Recognition》10 Netlab的网址(一个机器学习与统计工具箱)/netlab/index.htmlncrg:神经计算研究组aston: aston大学(有博后职位)11高斯过程(Mackay Williams)/~carl.html(由Carl建立,也见105)12正则化网络(MITCBCL--Poggio)/projects/cbcl/13apnik的主页(提出了SVM)/info/vlad14ahba的主页(研究样条插值ANOV A 、RKHS等, 有博后职位) /~wahba/15Kernel Fisher discriminant [KFD]http://ida.first.gmd.de/hompages/mika/Fisher.html16SMO for LS-SVMS (贝叶斯与SVM).sg/~mpessk/publications.html17支持向量机、核方法(Cristianini)18Keerthi的主页(新加坡国立大学).sg/~mpessk19搜索国外FTP以及专业资料的网页20郭天佑的主页上有许多关于机器学习的链接有关于各种学术杂志的网站链接(统计神经网络方面)有研究神经网络、机器学习、统计方法、信息检索、文本分类、智能代理、手写体识别、计算机视觉及模式识别的机构及个人网址,有香港本地研究机构网址t.hk/~jamesk/others.html21数学资源22神经网络,神经计算研究资源/resource.html23Plivis: Probabilistic Hierachical interactive visualization 潜在变量分析软件包/Phivis24TM: generative topographic mapping 自组织映射(SOM)的概率统计方法/GTM25关于人工智能的参考书、学者、公司、研究组大全/ai.html26关于贝叶斯网的各种资源http://www-2.cs.cmu.2du/~stefann/Bayesian-learning.htm27R.Herbrich的主页(研究机器学习、贝叶斯点机器学习,目前在微软研究组http://stat.cs.tu-berlin.de/~ralfh28David J.C Mackay的主页(提出显著度框架,也研究GP和变分法等)/mackay/网站在加拿大的镜象:http://www//~mackay/README.html29Radford.M.Neal的主页(Monte Carlo模拟, 主页上有贝叶斯方法的程序) /~radford30一些书籍的pdf格式文件下载/theses/available31关于LS-SVMhttp://www.esat.kuleuven.ac.be/sista/lssvmlab/home.html32. IEEE主页:IEEE数据库:IEICE主页:(IEICE: The Institute of Electronics, Information and Communication Engineers) Spie主页:32SI公司主页(SCI是其主要产品)33中科院主页:中国科技信息网:34一个有许多数字书籍的ftp ftp://202.38.85.7535Chu Wei的主页(提出了SVM分段损失函数,主页上有源程序).sg/~chuweihttp://www.ai.univie.ac.at/~brain/pthesis/~chuwei36Beal的主页(关于Bayesian learning的变分法,主页上有源程序)/~bealE-mail: beal@37Nando的主页(MCMC, 变分推理,主页上有源程序)www.cs.ubc.ca/~nando/publications.html38Gatsby Computational Neuroscience Unit39Schölkopf 的最新主页(核方法的鼻祖)www.kyb.tuebingen.mpg.de/~bs40Tom Mitchell的主页(machine learning一书的作者,卡内基梅隆大学教授)/~tom/41卡内基梅隆大学CALD中心(机器学习,人机智能,属于计算机科学学院,center for Automated Learning and Discovery,同时有一些聚类、分类软件)42Mallick和Veerabhadram的主页(关于Bayesian+Spline)/~bmallick (教授)/~veera(研究生)43Denison的主页(关于Bayesian+Spline,写了一本书) /~dgtd44Holmes的主页(Bayesian+Spline,博士已毕业)/~ccholmes (提出MLS)45David Ruppert的主页(关于Bayesian+Spline,写了一本书Semiparametric regression, 主要研究方向:Penalized splines, MCMC, Semiparametric Modeling, Local Polynomial; Additive models; Spatial model; Interaction models)(资料已下载放在E盘)/~davidr46Zhou Ding-Xuan的主页(提出了RKHS的覆盖数、容量等).hk/~mazhou47(香港)大学教育资助委员会.hk48美国数学协会(AMS), 出版proceedings of AMS和Trans of AMS等49Grudic的主页(关于Machine learning,有博后职位)/~grudic50美国计算机协会中国电子学会51Thomas Strohmann的主页(关于Minimax Probability Machine)/~strohmanLanckriet的主页(关于Minimax Probability Machine)/~gert52关于贝叶斯和统计的网站,网站上有软件可下载的软件有:Belief Networks; Poly-Splines; MC Inference; Poly-mars53 Association for uncertainty in AI,在其resource中有一些链接,主要有Bayes net; Decision analysis; machine learning; PR等54 机器学习(ML)资源大全ML 软件; ML Benchmarks; ML papers, Bibliographies, Journals, Organization,研究ML公司,出版社, ML Conferences等网站上有相关专题:Inductive logic programming; Data mining; Conceptual clustering; Reinforcement learning; Genetic algorithm; NN; Computational learning http://www.ai.univie.ac.at/oefai/ml/ml-resources.html55 达夫特大学模式识别资源大全研究领域、期刊、书、文章、研究小组等介绍,Job announcement栏目里有大量博后职位http://www.ph.tn.tudelft.nl/PRInfo/index.html56 核方法主页由Shawe-Taylor建立,Kernel methods for pattern analysis一书的主页57一个机器学习资源更丰富的站点,Jobs栏目里有一些博后职位/~aha/research/machine-learning.html58 博后职位在线Current listings of post-docs online,另外在google中可直接链入“pos tdoctoral position”进行搜索,在北大、清华的BBS中也有博后版59 Michael I. Jordan的主页(徐雷的老师,有博后职位)/~jordan60徐雷的主页.hk/~lxu/61Arnaud Doucet的主页(研究Sequential Monte Carlo和Particle Filtering)Nando的老师,有博士后职位/~ad2/arnaud_doucet.html62统计多媒体学习组,Statistical Multimedia Learning Groupwww.cs.ubc.ca/nest/lci/sml63剑桥大学统计实验室(数学学院/数学统计系)64CMC资料大全/~mcmc65研究贝叶斯统计的学者主页,Bayesian Statistics Personal Web Pages /~madigan/bayes-people.html66新语丝(学术打假) 67中国科技在线科技咨询、科技成果(863计划,火炬计划等)、科研机构、科技资料68 新加坡高性能计算中心Institute of High Performance Computing, Singapore,有博后职位,由“a comparison of PCA,FPCA and ICA...”一文发现69芬兰HUT,Helsinki University of Technology,Neural Networks Research center,Laboratory of computer and information,有博后职位www.cis.hut.fi/jobs70 ICA研究主页关于ICA的程序,研究人员,论文等(ICA for communication)http://www.cis.hut.fi/projects/ica71 SOM研究主页http://www.cis.hut.fi/projects/somtoolbox72tefan Harmeling的主页(研究基于核的盲源分离)http://www.first.fhg.de/~harmeli/index.html73.Muller的主页(研究SVM)http://www.first.fhg.de/persons/mueller.klaus-robert.html73iehe的主页(提出了关于盲源分离的一种新方法TDSEP)http://www.first.fhg.de/~ziehe/74王力波的主页(南洋理工大学博士,人工神经网络,软计算).sg/home/elpwang75Roman Rosipal的主页(研究核偏最小二乘KPLS)http://aiolos.um.savba.sk/rosi76IEEE北京地区分会/relations/IEEE%20BJ/index.htm77.周志华的主页(南京大学计算机系教授,研究机器学习)/people/zhouzh78.C. K .I. Williams的主页(研究高斯过程)/homes/ckiw79.P. Sollich的主页(研究贝叶斯学习)/~psollich80.Carl Edward Rasmussen的主页(研究高斯过程,建立了一个高斯过程网站)/~edward81 Santa Fe 时间序列预测分析竞赛(由Andreas主持)/~andreas/Time-Series/SantaFe.html82 Andreas的主页/~andreas83张志华t.hk/~zhzhangResearch interests(1)Bayesian Statistics (mixture model\graphical models(2)Machine learning (KM\spectral-graph)(3)Applications84 Dit-Yan-Yeung 的主页(Kwok的老师)t.hk/faculty/dyyeung/index.html85I Group at UST (Yeung是其中一员)t.hk/aigroup86 NIPS (neural information processing systems)/web/groups/NIPS(可下载NIPS会议集全文)87 JMLR(Journal of machine learning research) 杂志的主页/projects/jmlr能下载全文88 Neural Computation杂志的主页89 David Dowe的主页(研究混合模型)有各种混合模型的介绍与软件,比如GMM、Gamma分布的混合、对数分布的混合、Poisson分布的混合、Weibull分布的混合等.au/~dld/cluster.html90 Nell. D. Lawrence的主页(Bishop的学生,提出高斯过程潜变量模型GPLVM)/neil/ (老主页)/~neil(新主页)91 F. R. Bach的主页(提出KICA,将核方法与图模型结合)/~fbach92 Avrim Blum的主页(卡内基梅隆大学教授,研究机器学习)/~avrim93 学习理论大全,包括各种兴趣组、参考书、邮件列表,资源,COLT链接等94R.Schapire的主页(研究boosting)/~schapire95 T.Hastie的主页(主曲线的提出者,《统计学习基础》一书的作者)/~hastie/96.J.Friedman的主页(MARS、投影寻踪等方法的提出者)/people/faculty/friedman.html97最小最大概率机研究者主页nckriet: /~gertT.Strohmann: /~strohman98中国人工智能网99Kevin Murphy 的主页(研究概率图模型和贝叶斯网,并将之应用于计算机视觉有一个matlab工具箱BNT)/~murphyk或www.cs.ubc.ca/~murphyk100人脸识别学术网站/databases101 Sam Roweis的主页(多伦多大学助教,研究统计机器学习,主页上有NSPS,MNITS等手写体库和人脸库)/~roweis/102中国学术会议在线(网站内有很多国际会议消息)/index.jsp103史忠值的主页(中科院计算所信息处理实验室)104中科院数学与系统科学研究院105 A.Ronjyakotomam的主页(提出了小波核)http://asi.insa-rouen.fr/~arakotom106 Elad Yom-Tov的主页(与Duda和Strok开发了一个分类工具箱,《Computer Manual in Matlab to Accompany Pattern Classification》书的作者,该书是Duda模式分类一书的配套)/index.html107 G.Stork的主页(Pattern Classification一书的作者)/~stork108 Colin Fyfe的主页(研究SOM及其核版本、主曲线等)/fyfe-ci0/109 Dominique Mantinez的主页(提出基于核的盲源分离KBSS) http://www.loria.fr/~dmartine110 Andreas Ziehc的主页(KBSS 关于盲源分离的资料链接) http://idafirst.gmd.de/~Ziehe/research.html111 盲源分离欧洲项目(BLISS: Blind source separation and application) http://www.cis.inpg.fr/pages-paperso/bliss/index.php112 Gao Junbin 的主页(用贝叶斯方法实现SVM,有博后职位)http://athene.riv.csu.au/~jbgao/jbgao@.au113南安普敦大学电子与计算机科学系信号、图像、系统中心(有博后职位)/people114 David Zhang (张大鹏,香港理工大学教授,研究生物统计学, 有博后职位).hk/~csdzhang115自动生成计算机领域内的论文:/scigen116周志华的“机器学习与数据挖掘”研究组, 有机器学习领域内一些研究杂志与研究机构的链接/index_cn.htm117英文学术论文润色,检查可读性、语法、拼写、清晰度118黄德双的主页(中科大教授,中科院合肥智能机械研究所智能计算实验室)/119一个关于通信的ftp: 162.105.75.232有程序代码/书籍资料/通信文献/协议标准120一个关于DSP之类的ftp: http://202.38.73.175121合众达电子(关于DSP的入门网站)122、微波技术网/出国留学类:1、国外留学信息:国家留学网:/(国家留学基金委)中国留学网:/publish/portal0/tab171/2、欧洲中国留学生之家:3、我爱英语网:/tl/4、飞跃重洋:/5、英语学习太傻网:地球物理类:1、SEP: Stanford exploration project 斯坦福大学地震勘探工程以Claerbout为首的研究小组,网页内有源代码,人员介绍等。
普林斯顿 结构生物 类脑计算 经费

《普林斯顿:结构生物与类脑计算的探索与发展》一、引言在当今科技飞速发展的时代,结构生物学和类脑计算作为两大前沿交叉学科,对人类社会和科学技术发展产生了深远的影响。
作为这一领域的先锋,普林斯顿大学一直致力于对结构生物与类脑计算进行探索与发展。
本文将从普林斯顿大学的视角出发,全面评估和解析普林斯顿在这一领域的最新研究成果,并共享对结构生物与类脑计算的个人理解和观点。
二、普林斯顿:结构生物的前沿研究1. 普林斯顿在结构生物领域的权威地位作为一所拥有丰富研究资源和顶尖科研团队的顶尖大学,普林斯顿在结构生物领域拥有显著的研究优势。
在高分辨率结构生物学、蛋白质折叠与组装、大分子相互作用等方面,普林斯顿都有着卓越的研究成果和学术地位。
2. 普林斯顿的结构生物研究成果通过对普林斯顿在结构生物学领域的研究成果进行深入探讨,我们可以发现,普林斯顿在解析高分辨率蛋白质结构、研究生命大分子的结构与功能、探索生命活动的分子机制等方面取得了突破性进展。
这些成果不仅为生命科学领域的发展提供了重要的理论和实践支持,同时也为人类社会的生物医药、食品安全等方面带来了巨大的影响和推动力。
三、普林斯顿:类脑计算的前沿研究1. 普林斯顿在类脑计算领域的研究方向作为类脑计算领域的佼佼者,普林斯顿在神经科学、人工智能、认知科学等领域的研究成果备受关注。
通过对普林斯顿在类脑计算领域的研究方向进行全面评估,我们可以发现,普林斯顿在神经元信号传导模型、神经网络结构与功能的模拟、认知计算理论等方面取得了令人瞩目的成就。
2. 普林斯顿的类脑计算研究成果普林斯顿在类脑计算领域的研究成果不仅涉及基础理论研究,还包括脑-机器接口技术、人工智能系统的优化与应用、大规模脑网络行为建模等领域。
这些成果为人工智能、神经科学、认知计算等领域的交叉研究提供了重要的理论和技术支持,推动了类脑计算技术的迅速发展和应用。
四、结构生物与类脑计算的交叉研究1. 普林斯顿在结构生物与类脑计算交叉研究的探索普林斯顿在结构生物与类脑计算交叉研究的探索成果丰硕,涉及蛋白质与神经元的相互作用、大脑认知功能的生物结构基础、类脑计算技术在生物医学领域的应用等诸多领域。
脑部神经元格子的计算模型研究
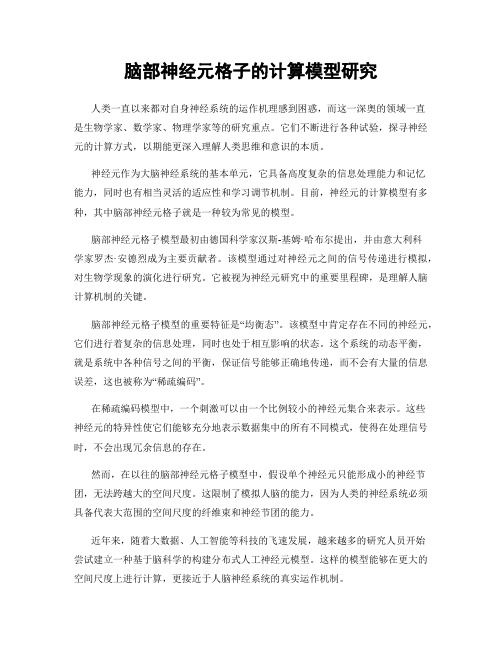
脑部神经元格子的计算模型研究人类一直以来都对自身神经系统的运作机理感到困惑,而这一深奥的领域一直是生物学家、数学家、物理学家等的研究重点。
它们不断进行各种试验,探寻神经元的计算方式,以期能更深入理解人类思维和意识的本质。
神经元作为大脑神经系统的基本单元,它具备高度复杂的信息处理能力和记忆能力,同时也有相当灵活的适应性和学习调节机制。
目前,神经元的计算模型有多种,其中脑部神经元格子就是一种较为常见的模型。
脑部神经元格子模型最初由德国科学家汉斯-基姆·哈布尔提出,并由意大利科学家罗杰·安德烈成为主要贡献者。
该模型通过对神经元之间的信号传递进行模拟,对生物学现象的演化进行研究。
它被视为神经元研究中的重要里程碑,是理解人脑计算机制的关键。
脑部神经元格子模型的重要特征是“均衡态”。
该模型中肯定存在不同的神经元,它们进行着复杂的信息处理,同时也处于相互影响的状态。
这个系统的动态平衡,就是系统中各种信号之间的平衡,保证信号能够正确地传递,而不会有大量的信息误差,这也被称为“稀疏编码”。
在稀疏编码模型中,一个刺激可以由一个比例较小的神经元集合来表示。
这些神经元的特异性使它们能够充分地表示数据集中的所有不同模式,使得在处理信号时,不会出现冗余信息的存在。
然而,在以往的脑部神经元格子模型中,假设单个神经元只能形成小的神经节团,无法跨越大的空间尺度。
这限制了模拟人脑的能力,因为人类的神经系统必须具备代表大范围的空间尺度的纤维束和神经节团的能力。
近年来,随着大数据、人工智能等科技的飞速发展,越来越多的研究人员开始尝试建立一种基于脑科学的构建分布式人工神经元模型。
这样的模型能够在更大的空间尺度上进行计算,更接近于人脑神经系统的真实运作机制。
为此,科学家们不断在脑部神经元格子模型的架构上进行创新,引入新的机器学习算法和数据分析方法。
他们同样也在自然智能计算中,引入神经科学的理论和计算模型,以提高机器学习和主流人工智能算法的性能。
- 1、下载文档前请自行甄别文档内容的完整性,平台不提供额外的编辑、内容补充、找答案等附加服务。
- 2、"仅部分预览"的文档,不可在线预览部分如存在完整性等问题,可反馈申请退款(可完整预览的文档不适用该条件!)。
- 3、如文档侵犯您的权益,请联系客服反馈,我们会尽快为您处理(人工客服工作时间:9:00-18:30)。
Ruslan Salakhutdinov,Sam Roweis CS Department,University of Toronto rsalakhu,roweis@Zoubin GhahramaniGatsby Computational Neuroscience Unit zoubin@AbstractWe show a close relationship between bound optimization(BO)algo-rithms such as Expectation-Maximization and direct optimization(DO)algorithms such as gradient-based methods for parameter learning.Weidentify analytic conditions under which BO algorithms exhibit Quasi-Newton convergence behavior,and conditions under which these algo-rithms possess poor,first-order convergence.In particular,for the EMalgorithm we show that if a certain measure of the proportion of missinginformation is small,then EM exhibits Quasi-Newton behavior;when itis large,EM converges slowly.Based on this analysis,we present a newExpectation-Conjugate-Gradient(ECG)algorithm for maximum likeli-hood estimation,and report empirical results,showing that,as predictedby the theory,ECG outperforms EM in certain cases.1IntroductionMany problems in machine learning and pattern recognition ultimately reduce to the op-timization of a scalar valued function of a free parameter vector.For example, in(supervised or)unsupervised probabilistic modeling the objective function may be the (conditional)data likelihood or the posterior over parameters.In discriminative learning we may use a classification or regression score;in reinforcement learning we may use av-erage discounted reward.Optimization may also arise during inference;for example we may want to reduce the cross entropy between two distributions or minimize a function such as the Bethe free energy.A variety of general techniques exist for optimizing such objective functions.Broadly, they can be placed into one of two categories:direct optimization(DO)algorithms and what we will refer to as bound optimization(BO)algorithms.Direct optimization works directly with the objective and its derivatives(or estimates thereof),trying to maximize or minimize it by adjusting the free parameters in a local search.This category of algorithms includes random search,standard gradient-based algorithms,line search methods such as conjugate gradient(CG),and more computationally intensive second-order methods,such as Newton-Raphson.They can be applied,in principle,to any deterministic function of the parameters.Bound optimization,on the other hand,takes advantage of the fact that many objective functions arising in practice have a special structure.We can often exploit this structure to obtain a bound on the objective function and proceed by optimizing this bound. Ideally,we seek a bound that is valid everywhere in parameter space,easily optimized,and equal to the true objective function at one(or more)point(s).A general form of a bound maximizer which iteratively lower bounds the objective function is given below:General form of Bound Optimization for maximizing: Assume:functions and such that:1.for any,and any.2.can be found easily for anyIterate:Guarantee:Many popular iterative algorithms are bound optimizers,including the EM algorithm for maximum likelihood learning in latent variable models[3],iterative scaling(IS)algorithms for parameter estimation in maximum entropy models[2]and the recent CCCP algorithm for minimizing the Bethe free energy in approximate inference problems[13].Bound opti-mization algorithms enjoy a strong guarantee;they never worsen the objective function. In this paper we study the relationship between direct and bound optimizers and determine conditions under which one technique can be expected to outperform another.Our general results apply to any model for which a bound optimizer can be constructed,although in later sections we focus on the case of probabilistic models with latent variables.2Gradient and Newton behaviors of bound optimizationFor most objective functions,the BO step in parameter space and true gradi-ent can be trivially related by a projection matrix,which changes at each iteration:(1) (We define1Note that,where,we have.We can further study the structure of the projection matrix by considering the map-ping defined by one step of BO:.Taking derivatives of both sides of(1) with respect to,we have(3) whereis the input-output derivative matrix for the BO mapping and(6)which can be interpreted as the ratio of missing information to the complete information near the local optimum.Thus,in the neighbourhood of a solution(for sufficiently large),(7)This formulation of the EM algorithm has a very interesting interpretation which is appli-cable to any latent variable model:When the missing information is small compared to the complete information,EM exhibits a Quasi-Newton behavior and enjoys fast,typically superlinear convergence in the neighborhood of.If fraction of missing information ap-proaches unity,the eigenvalues of thefirst term above approach zero and EM will exhibitFigure1:Contour plots of the likelihood function for MoG examples using well-separated (upper panels)and not-well-separated(lower panels)one-dimensional datasets(see left panels offigure3).Axes correspond to the two means.The dashdot line shows the direction of the truegradient,the solid line shows the direction of and the dashed line shows the direction of.Right panels are blowups of dashed regions on the left.The numbers indicate the log of the norm of the gradient.Note that for the”well-separated”case,in the vicinityof the maximum vectors and become identical.extremely slow convergence.Figure1illustrates these results in the simple case offitting a mixture of Gaussians model to well-clustered and not-well-clustered data.This analysis motivates the use of alternative optimization techniques in the regime wheremissing information is high and EM is likely to perform poorly.In the following sec-tion,we present exactly such an alternative,the Expectation-Conjugate Gradient(ECG) algorithm,a novel and simple direct optimization method for optimizing the parameters of latent variables models.We go on to show experimentally that ECG can in fact outperform EM under the conditions described above.4Expectation Conjugate Gradient(ECG)AlgorithmThe key idea of the ECG algorithm is to note that if we can easily compute the derivative.This exact gradient can then be utilized in any stan-dard manner,for example to do gradient(as)descent or to control a line search technique. As an example,we describe a conjugate gradient algorithm:Expectation-Conjugate-Gradient(ECG)algorithm:Apply a conjugate gradient optimizer to,performing an“EG”stepwhenever the value or gradient of is requested(e.g.during a line search).The gradient computation is given byE-Step:Compute posterior and log-likelihood as normal.G-Step:5Experimental ResultsWe now present empirical results comparing the performance of EM and ECG for learning the parameters2of three well know latent variable models:Mixtures of Gaussians(MoG), Probabilistic PCA(PPCA),and Hidden Markov Models(HMM).The models were trained on different data sets and with different initial conditions to illustrate both the regime in which ECG is superior to EM and in which it is inferior.Figure2summarizes our results: for“well-separated”,“low-rank”,or“structured”data in which the fraction of missing in-formation is small,EM converges quickly;for“overlapping”,”ill-conditioned”or“aliased”data where the latent variables are poorly determined,ECG significantly outperforms EM. First,consider a mixture of Gaussians(MoG)model.For visualization purposes,we have plotted and learned only the values of the means,fixing the mixing proportions, and variances.We considered two types of datasets,one in which the data is“well-separated”into distinct clusters and another“not-well-separated”case in which the data overlaps in one contiguous region.Figure2shows that ECG outperforms EM in the poorly separated cases.For the well-separated cases,in the vicinity of the local optima the di-rections of the vectors and become identical(fig.1),suggest-ing EM will have a Quasi-Newton type convergence behavior.For the not well-separated case,this is generally not true., for covariance matrices to be symmetric positive definite,we use the Choleski decomposition.To keep diagonal entries of the noise models in FA/PPCA positive,we set,and in HMMs,we reparameterize probabilities via softmax functions as well.Figure2:Learning curves for ECG(dots)and EM(solid lines)algorithms,showing superior(upper panels)and inferior(lower panels)performance of ECG under different conditions for three models: MoG(left),PPCA(middle),and HMM(right).The number of E-steps taken by either algorithm is shown on the horizontal axis,and log likelihood is shown on the vertical axis.For ECG,diamonds indicate the maximum of each line search.The zero-level for likelihood corresponds tofitting a single Gaussian density for MoG and PPCA,and tofitting a histogram using empirical symbol counts for HMM.The bottom panels use“well-separated”,“low-rank”,or“structured”data for which EM converges quickly;the upper panels use“overlapping”,”ill-conditioned”or“aliased”data for which ECG performs much better.We also experimented with the Probabilistic Principal Component Analysis(PPCA)latent variable model[9,11],which has continuous rather than discrete hidden variables.Here the concept of missing information is related to the ratios of the leading eigenvalues of the sample covariance,which corresponds to the ellipticity of the distribution.For“low-rank”data with a large ratio,our experiments show that EM performs well;for nearly circular data ECG converges faster.As a confirmation that this behavior is in accordance with our analysis,infigure3we show the evolution of the eigenvalues of the matrix during learning of the same datasets,generated from known parameters for which we can compute this missing information matrix exactly.For the well-separated MoG case the eigenvalues of the matrix approach zero,and the ratio of missing information to the complete information becomes very small,driving toward the negative of the inverse Hessian.Interestingly,in the case of PPCA,even though the rank of the matrix approaches zero,one of its eigenvalues remains nonzero even in the low-rank data case(fig.3).This suggests that the convergence of the EM algorithm for PPCA can still be slow very close to the optimum in certain directions in parameter space,even for“nice”data.3Hence,direct optimization methods may be preferred for thefinal stages of learning,even in these cases.Finally,we applied our algorithm to the training of Hidden Markov Models(HMMs).A simple2-state HMM(see insetfig.2)was trained to model sequences of discrete symbols. Missing information in this model is high when the observed data do not well determine the underlying state sequence(given the parameters).In one case(“aliased”sequences), we used sequences from a two-symbol alphabet consisting of alternating“AB...”of length 600(with probability of alternation95%and probablity of repeating5%).In the other case(“structured sequences”),the training data consisted of41character sequences from the book”Decline and Fall of the Roman Empire”by Gibbon,with an alphabet size of30 characters.(Parameters were initialized to uniform values plus small noise.)Once again,we observe that for the ambiguous or aliased data,ECG outperforms EM substantially.For real,structured data,ECG slightly outperforms EM.6DiscussionIn this paper we have presented comparative analysis of the bound and direct optimiza-tion algorithms,and built up the connection between those two classes of optimizers.We have also analyzed and determined conditions under which BO algorithms can demon-strate local-gradient and Quasi-Newton convergence behaviors.In particular,we gave a new analysis of the EM algorithm by showing that if the fraction of missing information is small,EM is expected to have Quasi-Newton behavior near local optima.Motivated by these analyses,we have proposed a novel direct optimization method(ECG) that can significantly outperform EM in some cases.We tested this algorithm on several basic latent variable models,showing regimes in which it is both superior and inferior to EM and explaining these behaviors with reference to our analysis.Previous studies have considered the convergence properties of the EM algorithm in spe-cific cases.Xu and Jordan[12],Ma,Xu and Jordan[7]studied a relationship between EM and gradient-based methods for ML learning offinite Gaussian mixture models.These au-thors state conditions under which EM can approximate a superlinear method(but only in the MoG setting),and give general preference to EM over gradient-based methods.Red-ner and Walker[8],on the other hand,argued that the speed of EM convergence can be extremely slow,and that second-order methods should generally be favored to EM. Many methods have also been proposed to enhance the convergence speed of the EM al-gorithm,mostly based on the conventional optimization theory.Louis[6]proposed an ap-proximate Newton’s method,known as Turbo EM,that makes use of Aitken’s acceleration method to yield the next iterate.Jamshidian and Jennrich[5]proposed accelerating the EM algorithm by applying generalized conjugate gradient algorithm.Other authors(Red-ner and Walker[8],Atkinson[1])have proposed hybrid approaches for learning,advocating switching to a Newton or Quasi-Newton method after performing several EM iterations. All of the methods,although sometimes successful in terms of convergence,are much more complex than EM,and difficult to analyze;thus they have not been popular in practice. While BO algorithms have played a dominating role in learning with hidden variables and in some approximate inference procedures,our results suggest that it is important not to underestimate the power of DO methods.Our analysis has indicated when one strategy may outperform another;however it is limited by being valid only in the neighbourhood of optima or plateaus and also by requiring the computation of quantities not readily available at runtime.The key to practical speedups will be the ability to design hybrid algorithms which can detect on thefly when to use bound optimizers like EM and when to switch to direct optimizers like ECG via efficiently estimating the local missing information ratio. AcknowledgmentsWe would like to thank Yoshua Bengio,Drew Bagnell,and Max Welling for many useful comments and Carl Rasmussen for providing an initial version of conjugate gradient code. References[1]S.E.Atkinson.The performance of standard and hybrid EM algorithms for ML estimates of thenormal mixture model with censoring.J.of putation and Simulation,44,1992. [2]Stephen Della Pietra,Vincent J.Della Pietra,and John fferty.Inducing features of randomfields.IEEE Transactions on Pattern Analysis and Machine Intelligence,19(4):380–393,1997.[3] A.P.Dempster,ird,and D.B.Rubin.Maximum likelihood from incomplete data viathe EM algorithm(with discussion).J.of the Royal Statistical Society series B,39:1–38,1977.[4]Zoubin Ghahramani and Geoffrey Hinton.The EM algorithm for mixtures of factor analyzers.Technical Report CRG-TR-96-1,Dept.of Computer Science,University of Toronto,May1996.[5]Mortaza Jamshidian and Robert I.Jennrich.Conjugate gradient acceleration of the EM algo-rithm.Journal of the American Statistical Association,88(421):221–228,March1993.[6]T.A.Louis.Finding the observed information matrix when using the EM algorithm.Journalof the Royal Statistical Society series B,44:226–233,1982.[7]Jinwen Ma,Lei Xu,and Michael Jordan.Asymptotic convergence rate of the EM algorithm forgaussian mixtures.Neural Computation,12(12):2881–2907,2000.[8]Richard A.Redner and Homer F.Walker.Mixture densities,maximum likelihood and the EMalgorithm.SIAM Review,26(2):195–239,April1984.[9]S.T.Roweis.EM algorthms for PCA and SPCA.In Advances in neural information processingsystems,volume10,pages626–632,Cambridge,MA,1998.MIT Press.[10]Ruslan Salakhutdinov.Relationship between gradient and em steps for several latent variablemodels./rsalakhu.[11]M.E.Tipping and C.M.Bishop.Mixtures of probabilistic principal component analysers.Neural Computation,11(2):443–482,1999.[12]L.Xu and M.I.Jordan.On convergence properties of the EM algorithm for Gaussian mixtures.Neural Computation,8(1):129–151,1996.[13]Alan Yuille and Anand Rangarajan.The convex-concave computational procedure(CCCP).InAdvances in Neural Information Processing Systems,volume13.MIT Press,2001. Appendix:Explicit relationships between EM step and gradientIn this section,we derive the exact relationship between gradient of log-likelihood and the step EM performs in the parameter space for the Mixture of Factor Analyzers(MFA)model,extending the results of Xu and Jordan[12].The derivation can be easily modified to yield identical result for PPCA,FA,Mixture of PPCA,and HMM models.The log-likelihood function for MFA model with parameters is4.At each iteration of EM algorithm we have(8) The reader can easily verify the validity of this symmetric positive definite projection matrix by multiplying it by the gradient of the log likelihood function.The general form of the projection matrix can also be easily derived for the regular exponential family in terms of its natural parameters[10].The matrix is positive definite with respect to the gradient(by C1and C2)due to the well-known convexity property of.。