cis-regulatory modules in sets
a-box cis-acting regulatory element -回复

a-box cis-acting regulatory element -回复什么是Cis-Acting Regulatory Element?在基因组学中,核糖核酸(DNA和RNA)中的特定序列可以调控基因的表达。
这些序列被称为调控元件,它们处于基因所在的同一染色体上,因此被称为Cis-Acting Regulatory Element(cis-作用调控元件)。
Cis-作用调控元件能够通过与转录因子等调控蛋白相互作用,影响基因的转录和表达。
这一过程对于生物体正常功能的维持和发展至关重要。
Cis-作用调控元件的研究在揭示基因调控机制和开发基因治疗方面具有重要意义。
Cis-作用调控元件和基因调控过程之间的关系:在基因组中,与Cis-作用调控元件相对应的是转录因子结合位点(Trans-Acting Regulatory Element)。
Cis-作用调控元件位于基因的上游或下游区域,并且可以在基因的调控区域内。
其所在的位置在整个基因组内可能是特异性或普遍性的,它们对于控制基因的启动、沉默、重编程和调控等过程起着重要作用。
Cis-作用调控元件的分析方法:研究Cis-作用调控元件的最常用的方法是计算分析和实验验证相结合的策略。
在计算分析方面,研究人员可以使用生物信息学工具来预测和鉴定Cis-作用调控元件。
这些工具可以分析DNA序列的特定标记,如启动子序列、转录因子结合位点以及DNA甲基化位点等。
实验验证方面,研究人员可以利用CRISPR-Cas9技术来进行Cis-作用调控元件的编辑和功能验证。
Cis-作用调控元件的功能:Cis-作用调控元件可以具有多种功能。
首先,它们可以作为启动子(promoter)来驱动基因的转录。
在转录过程中,启动子通过结合转录因子和RNA聚合酶来实现基因的转录。
其次,Cis-作用调控元件还可以作为增强子(enhancer)和沉默子(silencer)。
增强子可以增强基因的转录活性,而沉默子则可以抑制基因的转录活性。
PlantHormoneSignaltransductionpathway植物激素信号转导通路
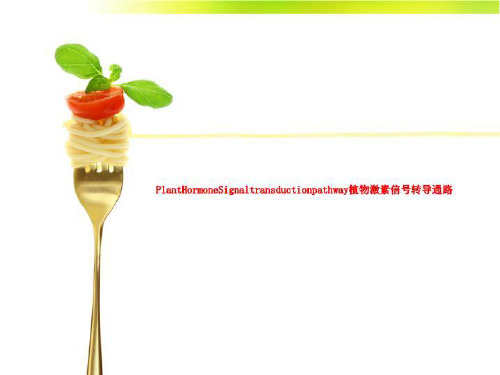
(c) the sensitivity of that tissue to the hormone.
(d) the condition of the plant itself is critical: what is the condition of the plant? its age?
4. E1 (1); E2 (1); UEV (8); E3 (mast abundant): HECT (7); Ring (450); U-domain (61); cullin (11); Fbox (700) BTB (80)
Comparison between auxin and gibberellin signaling pathway
Various signaling transduction pathways in plants
Calcium (Ca+2) signaling (regulatory network) Lipid signaling transduction pathway Reactive oxygen species (ROS) signaling Nitric oxide (NO) signaling transduction pathway Sugar sensing responsive pathway Wounding-signal transduction pathway (plant-pathogen interaction) Light signaling responsive pathway Biological o’clock (circadian rhythm) regulatory pathway
封装相关的英语术语
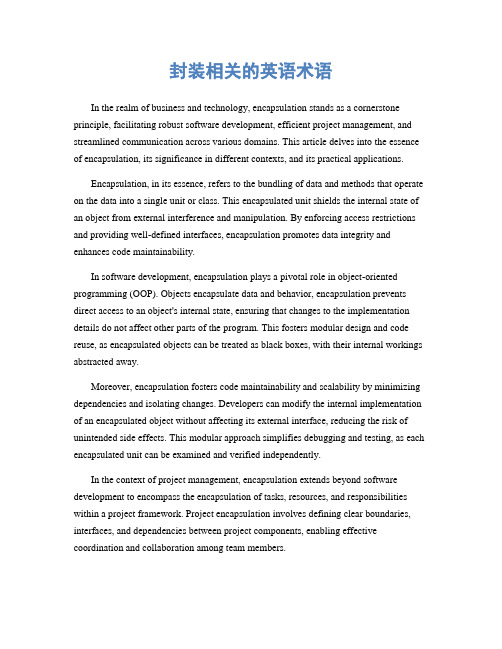
封装相关的英语术语In the realm of business and technology, encapsulation stands as a cornerstone principle, facilitating robust software development, efficient project management, and streamlined communication across various domains. This article delves into the essence of encapsulation, its significance in different contexts, and its practical applications.Encapsulation, in its essence, refers to the bundling of data and methods that operate on the data into a single unit or class. This encapsulated unit shields the internal state of an object from external interference and manipulation. By enforcing access restrictions and providing well-defined interfaces, encapsulation promotes data integrity and enhances code maintainability.In software development, encapsulation plays a pivotal role in object-oriented programming (OOP). Objects encapsulate data and behavior, encapsulation prevents direct access to an object's internal state, ensuring that changes to the implementation details do not affect other parts of the program. This fosters modular design and code reuse, as encapsulated objects can be treated as black boxes, with their internal workings abstracted away.Moreover, encapsulation fosters code maintainability and scalability by minimizing dependencies and isolating changes. Developers can modify the internal implementation of an encapsulated object without affecting its external interface, reducing the risk of unintended side effects. This modular approach simplifies debugging and testing, as each encapsulated unit can be examined and verified independently.In the context of project management, encapsulation extends beyond software development to encompass the encapsulation of tasks, resources, and responsibilities within a project framework. Project encapsulation involves defining clear boundaries, interfaces, and dependencies between project components, enabling effective coordination and collaboration among team members.By encapsulating tasks and resources within well-defined modules or phases, project managers can mitigate risks, allocate resources efficiently, and monitor progress effectively. Encapsulation facilitates agile project management methodologies, allowing teams to adapt to changing requirements and priorities without disrupting the overall project workflow.Furthermore, encapsulation enhances communication and transparency within project teams and stakeholders. By encapsulating project-related information within accessible artifacts such as project plans, status reports, and documentation, project managers can ensure that relevant stakeholders are informed and engaged throughout the project lifecycle.In the domain of data management and information security, encapsulation plays a crucial role in safeguarding sensitive information and controlling access to data resources. Encapsulating data within secure containers or databases, with well-defined access controls and encryption mechanisms, helps prevent unauthorized access and data breaches.Additionally, encapsulation facilitates compliance with regulatory requirements such as GDPR, HIPAA, and PCI DSS by ensuring that data handling practices adhere to established standards and protocols. By encapsulating data processing operations within auditable frameworks and documenting data flows and access controls, organizations can demonstrate accountability and transparency in their data management practices.In conclusion, encapsulation serves as a fundamental principle in business and technology, enabling modular design, code reuse, project management, and data security. By encapsulating data and functionality within well-defined units or modules, organizations can enhance agility, maintainability, and security across various domains. Embracing encapsulation empowers businesses to navigate complexity, mitigate risks, and achieve sustainable growth in an ever-evolving landscape of challenges and opportunities.。
microRNA相关问题的计算分析- 附件1

附件2论文中英文摘要作者姓名:汪小我论文题目:microRNA相关问题的计算分析作者简介:汪小我,男,1980年6月出生,2003年9月师从于清华大学李衍达教授,2008年7月获博士学位。
中文摘要生物信息学是生命科学与信息科学、控制科学等多学科交叉的新兴学科。
近年来,人类基因组的测序完成和各类高通量生物实验技术的发展,使得生物学数据呈指数级增长,如何用生物信息技术挖掘和分析这些海量的信息成为研究的焦点。
同时,随着生物信息学研究的不断深入,它在解决重要的生物学问题、阐明新的生物学规律等方面发挥出巨大作用。
microRNA(miRNA,微小RNA)是近年来新发现的一类非编码RNA,它在诸多重要生命过程中起着关键的调控作用,人们对其在疾病的诊断和治疗等方面的应用前景寄予厚望,关于miRNA的研究是当前生命科学领域最前沿的方向之一。
生物信息学在miRNA的研究中起到了关键作用,极大地推动了该领域的迅速发展。
本论文的工作围绕miRNA这一重要生物学问题展开,运用统计、机器学习和模式识别等多种生物信息学方法,对miRNA的识别、转录调控、进化机制等重要问题进行了多方面的探索,取得了一些创新成果。
主要有以下四方面内容:(1)提出了高效的同源miRNA识别算法,可用于预测远同源的miRNA基因。
要研究miRNA的功能,首先必须找到miRNA。
在提出本课题时,用于发现新miRNA 基因的实验技术费时又费钱,而且很难找到那些表达量较低或者只在特定组织或发育阶段中表达的miRNA。
因此通过高通量的计算方法从基因组中筛选出可能的miRNA基因候选集合,可以对生物学实验提供指导和参考,对推动miRNA研究具有重要意义。
同源基因预测是一类重要的基因识别方法,其出发点是利用基因在物种间的保守性,寻找已知基因的同源基因。
这类方法可以将一个物种中找到的基因及其注释推广到其它物种中。
包括人类基因组在内的多个物种的基因组测序完成为开展同源基因预测创造了条件。
cadence仿真器的参数配置
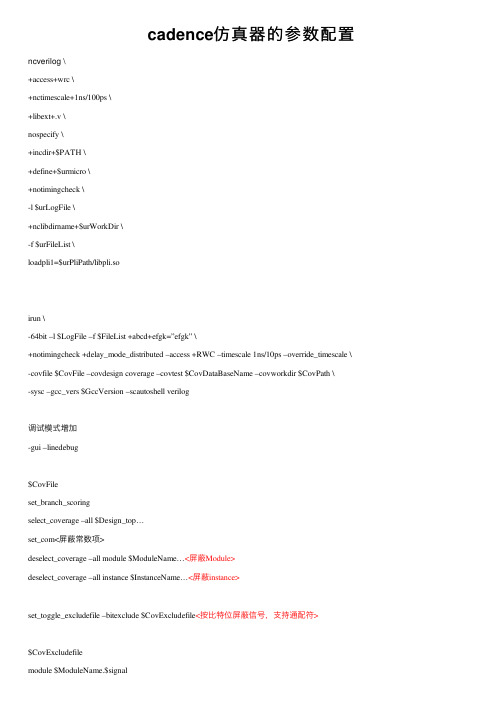
cadence仿真器的参数配置ncverilog \+access+wrc \+nctimescale+1ns/100ps \+libext+.v \nospecify \+incdir+$PATH \+define+$urmicro \+notimingcheck \-l $urLogFile \+nclibdirname+$urWorkDir \-f $urFileList \loadpli1=$urPliPath/libpli.soirun \-64bit –l $LogFile –f $FileList +abcd+efgk=”efgk” \+notimingcheck +delay_mode_distributed –access +RWC –timescale 1ns/10ps –override_timescale \ -covfile $CovFile –covdesign coverage –covtest $CovDataBaseName –covworkdir $CovPath \-sysc –gcc_vers $GccVersion –scautoshell verilog调试模式增加-gui –linedebug$CovFileset_branch_scoringselect_coverage –all $Design_top…set_com<屏蔽常数项>deselect_coverage –all module $ModuleName…<屏蔽Module>deselect_coverage –all instance $InstanceName…<屏蔽instance>set_toggle_excludefile –bitexclude $CovExcludefile<按⽐特位屏蔽信号,⽀持通配符>$CovExcludefilemodule $ModuleName.$signalinstance $InstanceName.$signal我们知道,由于NC-Verilog使⽤了Native Compile Code的技术来加强电路模拟的效率,因此在进⾏模拟时必须经过compile(ncvlog命令)以及elaborate(ncelab命令)的步骤。
SegHMC
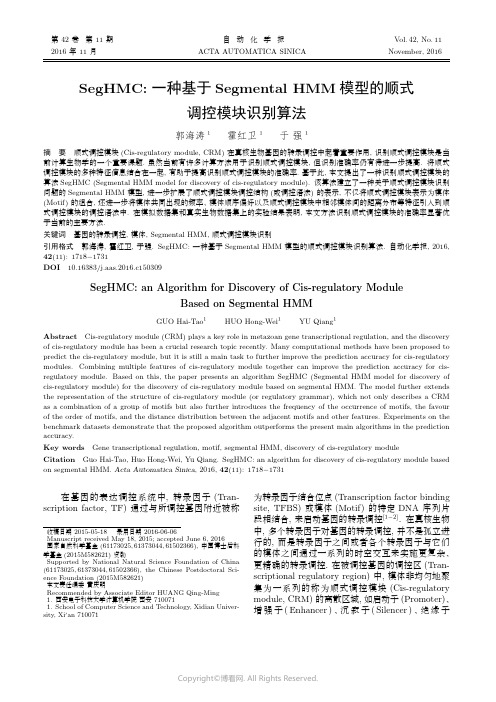
第42卷第11期自动化学报Vol.42,No.11 2016年11月ACTA AUTOMATICA SINICA November,2016SegHMC:一种基于Segmental HMM模型的顺式调控模块识别算法郭海涛1霍红卫1于强1摘要顺式调控模块(Cis-regulatory module,CRM)在真核生物基因的转录调控中起着重要作用,识别顺式调控模块是当前计算生物学的一个重要课题.虽然当前有许多计算方法用于识别顺式调控模块,但识别准确率仍有待进一步提高.将顺式调控模块的多种特征信息结合在一起,有助于提高识别顺式调控模块的准确率.基于此,本文提出了一种识别顺式调控模块的算法SegHMC(Segmental HMM model for discovery of cis-regulatory module).该算法建立了一种关于顺式调控模块识别问题的Segmental HMM模型,进一步扩展了顺式调控模块调控结构(或调控语法)的表示,不仅将顺式调控模块表示为模体(Motif)的组合,还进一步将模体共同出现的频率、模体顺序偏好以及顺式调控模块中相邻模体间的距离分布等特征引入到顺式调控模块的调控语法中.在模拟数据集和真实生物数据集上的实验结果表明,本文方法识别顺式调控模块的准确率显著优于当前的主要方法.关键词基因的转录调控,模体,Segmental HMM,顺式调控模块识别引用格式郭海涛,霍红卫,于强.SegHMC:一种基于Segmental HMM模型的顺式调控模块识别算法.自动化学报,2016, 42(11):1718−1731DOI10.16383/j.aas.2016.c150309SegHMC:an Algorithm for Discovery of Cis-regulatory ModuleBased on Segmental HMMGUO Hai-Tao1HUO Hong-Wei1YU Qiang1Abstract Cis-regulatory module(CRM)plays a key role in metazoan gene transcriptional regulation,and the discovery of cis-regulatory module has been a crucial research topic recently.Many computational methods have been proposed to predict the cis-regulatory module,but it is still a main task to further improve the prediction accuracy for cis-regulatory bining multiple features of cis-regulatory module together can improve the prediction accuracy for cis-regulatory module.Based on this,the paper presents an algorithm SegHMC(Segmental HMM model for discovery of cis-regulatory module)for the discovery of cis-regulatory module based on segmental HMM.The model further extends the representation of the structure of cis-regulatory module(or regulatory grammar),which not only describes a CRM as a combination of a group of motifs but also further introduces the frequency of the occurrence of motifs,the favour of the order of motifs,and the distance distribution between the adjacent motifs and other features.Experiments on the benchmark datasets demonstrate that the proposed algorithm outperforms the present main algorithms in the prediction accuracy.Key words Gene transcriptional regulation,motif,segmental HMM,discovery of cis-regulatory moduleCitation Guo Hai-Tao,Huo Hong-Wei,Yu Qiang.SegHMC:an algorithm for discovery of cis-regulatory module based on segmental HMM.Acta Automatica Sinica,2016,42(11):1718−1731在基因的表达调控系统中,转录因子(Tran-scription factor,TF)通过与所调控基因附近被称收稿日期2015-05-18录用日期2016-06-06Manuscript received May18,2015;accepted June6,2016国家自然科学基金(61173025,61373044,61502366),中国博士后科学基金(2015M582621)资助Supported by National Natural Science Foundation of China (61173025,61373044,61502366),the Chinese Postdoctoral Sci-ence Foundation(2015M582621)本文责任编委黄庆明Recommended by Associate Editor HUANG Qing-Ming1.西安电子科技大学计算机学院西安7100711.School of Computer Science and Technology,Xidian Univer-sity,Xi an710071为转录因子结合位点(Transcription factor binding site,TFBS)或模体(Motif)的特定DNA序列片段相结合,来启动基因的转录调控[1−2].在真核生物中,多个转录因子对基因的转录调控,并不是孤立进行的,而是转录因子之间或者各个转录因子与它们的模体之间通过一系列的时空交互来实施更复杂、更精确的转录调控.在被调控基因的调控区(Tran-scriptional regulatory region)中,模体非均匀地聚集为一系列的称为顺式调控模块(Cis-regulatory module,CRM)的离散区域,如启动子(Promoter)、增强子(Enhancer)、沉寂子(Silencer)、绝缘子11期郭海涛等:SegHMC:一种基于Segmental HMM模型的顺式调控模块识别算法1719(Insulator)等.结合这些顺式调控模块的转录因子通过相互协作、相互竞争,激活或抑制所调控基因的转录表达.一个顺式调控模块包含单个或多个转录因子的多个模体实例,其长度通常约为几百到几千个碱基对(Base pair,bp).一个真核生物基因调控区序列可能的调控结构的简单例子如图1所示.识别顺式调控模块是理解基因转录调控分子机制的基础,同时也是构建基因调控网络[3−4]的关键步骤.此外,识别具有特定调控功能的顺式调控模块对疾病机理的研究也有重要的意义.许多疾病的发生都与基因的异常表达有关,调控基因表达的顺式调控模块发生变异是造成基因异常表达的主因.有证据表明特定顺式调控模块中协作调控元素的破坏,可以导致畸形和疾病;例如,Kleinjan等[5]发现PAX6的任何远端调控元素的缺失都会改变其表达水平,从而造成先天性眼球畸形、无虹膜以及大脑缺陷等疾病.通过生物实验,例如高通量检测与顺式调控模块相关的表观遗传标记特征[6],可以识别顺式调控模块,但这种方法费时费力代价较大,并且许多时候受限于实验条件而很难实施.因此,使用计算方法直接从DNA序列中识别顺式调控模块已成为一个非常有吸引力的手段.然而,使用计算方法识别顺式调控模块也存在着许多挑战:1)同一个顺式调控模块不同实例内的模体排列顺序并不完全相同,但也并非完全无序的.此外,模块内的模体之间的距离也不确定,即同一模块不同实例内相同的两相邻模体间的距离也都不相同.因此,很难确定性地刻画这种结构.2)真核生物的调控区通常很长,构成顺式调控模块的模体通常较短且存在退化,根据已知的模体或者借助于现有的模体库(如TRANSFAC[7]、JASPAR[8])直接搜索,会找出大量的假阳性匹配,所以很难通过直接搜索相关模体的方式来识别包含这些模体的顺式调控模块.至今,已有多种用于识别顺式调控模块的模型和方法[6,9−27].为了识别真核生物基因的顺式调控模块,不同方法利用顺式调控模块的不同特征(如模体的聚集和物种间的保守性),使用不同搜索策略.其中一类方法基于窗聚集,利用模体倾向于聚集的特性来搜索顺式调控模块.这类方法用最简单的方式表示顺式调控模块,通过概率统计度量给定长度窗口内的模体组合的统计显著性,相应方法如MSCAN[22]和MCAST[28]等,或者使用组合方法,在指定窗口大小范围内搜索在多个序列共同出现一组模体实例的最小区域,将其作为候选顺式调控模块,如CMStalker[29]等.这类方法本质上假定了序列窗内的模体之间独立同分布.这类方法虽然简单直接,但需要合理确定窗大小以及度量统计显著性的打分阈值,而这些参数在实际应用中通常很难确定;此外,这类方法也忽略了顺式调控模块可能的调控结构(或调控语法),如模体间的顺序和距离.另一类方法基于概率模型,通过对序列或顺式调控模块建立概率模型,进而找出待搜索的目标序列中的顺式调控模块.基于概率模型的方法,除了少数采用判别模型的方法,如HexDiff[30]、Regulatory Potential[31]等外,大部分的方法使用生成模型,主要是隐马尔科夫模型(Hidder Markov model, HMM).HMM模型的主要优势在于,它可以对顺式调控模块的出现进行可靠的统计度量,并能刻画顺式调控模块的调控语法.此外,HMM模型所使用的期望最大化的参数估计算法,可以自动调节大量的参数,避免了手动设置的麻烦.基于HMM的顺式调控模块识别方法通常将顺式调控模块看作由一组过表达的模体和背景组合生成的序列片段.与窗聚集方法相比,它们不仅考虑了构成顺式调控模块的模体组合,也同时考虑了构成顺式调控模块的模体之间的距离.最初的一些方法,如CisModule[20],图1顺式调控模块结构示意图(顺式调控模块是包含多个转录因子相应模体的序列区;模体的方向、模体间的间隔距离、模体间的相互关系可能包含了给定顺式调控模块的重要性质.)Fig.1The structure discription of cis-regulatory modules(A cis-regulatory module is a sequence region that contains multiple motifs of multiple transcription factors;motif orientation,the interval distance between motifs and their cooperation relationship may imply the important regulatory properties of the cis-regulatory module.)1720自动化学报42卷使用HMM间接捕捉顺式调控模块内部以及顺式调控模块之间背景的概率分布.但这种方法仅使用了一般的顺式调控模块内部背景,并未推断顺式调控模块内的模体之间的任何顺序.后续的方法,进一步扩展这种模型的表示,如Stubb[32]方法,创建了一个仅包含模体和背景两个状态的HMM模型,使用统计方法度量模体对间共同出现的显著性,进而决定是否引入相应的转移概率.通过使用定义在指定长度窗口内序列上的打分函数度量窗口内模体聚集显著性来预测顺式调控模块.该方法仅利用了HMM模型的转移概率,没有使用任何其他HMM模型特性.后来的方法CORECLUST[33]和TSHAS[14]建立了更复杂的HMM模型,引入了顺式调控模块内部背景状态,加入了模体到模体的概率转移,更细致地刻画了顺式调控模块的调控结构.训练和解码算法使用标准的Baum-Welch算法[34]和Veterbi算法[34].这类方法使用了HMM的特性,但局限于HMM的表达能力,建立的调控模型并不直观,添加了大量的辅助状态.另一类HMM相关的顺式调控模块识别方法,使用加强的HMM来刻画顺式调控模块的调控结构;如BayCis算法[35],使用贝叶斯层次HMM模型,对顺式调控模块和包含顺式调控模块调控序列进行建模.利用了层次HMM的特性建立了模体之间的概率转移.该模型将HMM状态转移参数看作随机变量,并引入贝叶斯先验.该模型虽然结构直观,表达能力强,但模型的训练和解码需要大量的计算.Lemnian等提出的基于Extended sunflower HMM的顺式调控模块识别方法[19],使用Extended sunflower HMM,对模型的刻画深入到模体内部,不仅刻画了模体间的依赖,还刻画了模体内部碱基之间的依赖关系,但该方法仅用于同型顺式调控模块的识别.此外,还有一类方法利用相近物种的进化保守性来识别顺式调控模块,如Mor-phMS[26]、MultiModule[36]和ReLA[27]等.这类方法首先通过双序列或多序列比对同源基因的调控区找出其中的保守区域,然后使用其他方法在保守区域中搜索顺式调控模块.由于大多数基因的调控区中存在着大量重复(Duplication)和改组(Shuf-fling)的序列片段,很难进行序列比对,所以这类方法并不总是有效.将顺式调控模块的多种特征信息结合在一起,有助于提高识别顺式调控模块的准确率.顺式调控模块虽然结构具有不确定性,很难刻画,但作为频繁出现在多个被调控基因调控区中的调控功能单位,很可能包含某些保守成分.MOPAT[37]从一组同源基因的调控区中搜索保守模体模块(这里的保守模体模块被定义为在一组同源基因调控区中频繁出现且具有一定距离约束的相邻模体对,不考虑两个模体的相对顺序)出发,查找包含多个这种保守模体模块的区域,将其作为候选顺式调控模块,找出了一些保守的顺式调控模块.这一事实间接说明了顺式调控模块中存在保守成分.这里,我们同样利用保守模体模块这种顺式调控模块的保守成分,但限定保守模体模块内的模体具有特定的次序.然后,进一步将顺式调控模块保守性假设从同源基因(不同物种)推广到共调控基因(同一物种).最终,我们将顺式调控模块表示为由单模体和保守模体模块混合组成的,具有部分保守特征的调控结构(也称调控语法),从而将顺式调控模块结构保守性和其内部模体倾向于聚集的特征结合起来.为了刻画这种复杂的顺式调控模块调控结构,我们使用一种被称为Segmental HMM[38]的增强HMM模型来表达.基于此,本文提出了一种识别顺式调控模块的概率模型方法SegHMC(Segmental HMM model for discovery of cis-regulatory module).该方法使用Segmental HMM在给定候选模体集上构建同源或共调控基因调控区序列和顺式调控模块的调控语法结构.同一般的识别顺式调控模块的HMM模型相比,我们不仅将顺式调控模块表示为模体的组合,还将模体共同出现的频率、模体顺序偏好以及顺式调控模块中的相邻模体之间距离分布等特征引入到顺式调控模块的调控语法当中,这些特征可以有效提高顺式调控模块的识别精度.此外,为了处理真核生物基因长的调控区,我们对模型进行了降低搜索空间的优化.这种优化通过提前进行片段分割,显式建立Segmental HMM状态转换图,去除了大量不必要的搜索路径,降低了搜索空间,同时又不失精度.得到的模型可用于待搜索目标基因调控区中甚至整个基因组中的相似顺式调控模块识别.我们分别在一个模拟数据集和两个真实生物数据集: Muscle数据集和果蝇早期发育数据集上对我们的方法进行测试,并选取当前主要方法进行比较,所有方法识别顺式调控模块的准确率使用通用评价指标相关系数(Correlation coefficient,CC)和F1-score 来度量.实验结果表明,我们的方法识别顺式调控模块的准确率显著优于当前的主要方法.1SegHMC算法1.1SegHMC的Segmental HMM模型Segmental HMM[38]是HMM的一个扩展,也称Generalized HMM.与一般HMM的每个状态仅能发射一个碱基相比,Segmental HMM的每个状态可以发射可变长度的碱基序列片段;状态所发射的碱基序列可由一个片段模型来表示.该片段模型,给出了生成长度为u的观察序列o=o1o2···o u的11期郭海涛等:SegHMC:一种基于Segmental HMM模型的顺式调控模块识别算法1721联合概率,可由下式表示.P(o,u|s)=P(o|s)P(u|s)=e s(o)d s(u)(1)因此,片段模型由两个分布组成,一个是描述片段长度似然的片段长度分布d s(u),另一个为表示不同长度观察序列发射概率的发射模型e s(o).因此,在顺式调控模块的识别模型中,可以根据对顺式调控模块和调控序列结构的抽象,对这两个分布给出具体的定义.本文使用Segmental HMM,在片段层次上对顺式调控模块和调控序列的调控结构进行建模,具有更强的表达能力;例如,可以对片段之间的依赖进行建模.本节将详细阐述Segmental HMM模型,该模型主要包括:模型的构建、状态转移概率、片段长度分布和生成状态的发射模型.1.1.1Segmental HMM模型构建我们将转录调控序列的调控结构定义如下.转录调控序列由一系列的顺式调控模块和顺式调控模块之间的背景(称为全局背景)构成,而每个顺式调控模块又由一组具有特定次序的模体和模体之间的背景(称为局部背景)构成,这种抽象具有明显的层次性.基于这种结构定义,给定的转录调控序列可由下列过程生成:1)定位给定候选模体集中的模体在目标转录调控序列中所有可能出现实例;2)以这些被定位的模体实例为锚点,使用两模体实例之间的背景(全局背景或局部背景,具体类别待定)序列连接这些模体实例,从而生成整个调控序列.上述过程中,我们允许模体实例在空间上存在重叠,模体之间可能通过多种类型的背景序列相连;因此,存在许多平行的生成路径.从这些路径中,找出最可能的生成路径,即可得出该转录调控序列最可能的调控结构,从而找出相应的顺式调控模块.将每个具体片段(模体、全局背景和局部背景)表示为Segmental HMM的一个状态,片段之间的连接对应了两个状态之间的转移,根据上述生成过程,我们显式构造Segmental HMM的状态转换图.显式构建状态转换图一方面移除了不必要的状态路径,减小算法的搜索空间;另一方面,更便于构建模体的二元语法,模型顺式调控模块内的相邻模体间的一阶依赖关系.为了标识顺式调控模块,我们增加相应的辅助状态:顺式调控模块开始状态和顺式调控模块结束状态.图2给出了表示一个调控序列调控语法结构的Segmental HMM状态转换图的具体例子,整个模型所包含的状态如下:1)模型的初始状态S和终止状态E;2)模体状态M={m1,m2,···,m K};3)全局背景状态B g={b(0)g ,b(1)g,···,b(N+1)g};4)顺式调控模块状态C,又由顺式调控模块开始状态C s和顺式调控模块结束状态C e构成,即C=C s∪C e={c(1)s,c(2)s,···,c(N)s,c(1)e,c(2)e,···,c(N)e};5)局部背景状态B c={b(1,1)c,···,b(1,K)c,···,b(2,1)c,···,b(2,K)c,···,b(K,1)c,···,b(K,K)c}.因此,整个模型的状态空间Q={S,E}∪M∪B g∪C∪B c.Segmental HMM状态转换图的具体构造过程如算法1所示.算法1.Segmental HMM状态转换图的构造输入:一组Motif的PWM集PWMS,用于搜索Motif的p-value阈值和一个调控序列输出:状态转换图的状态集Q和这些状态之间的连接集T1)创建该模型的初始状态S和终止状态E2)对PWMS中每个PWM,在所给调控序列中找出小于给定p-value阈值所有Motif匹配3)根据找出的Motif匹配对所给调控序列进行分割,标记状态类型,创建相应的状态集M,B g和C s4)Q←{S,E}∪M∪B g∪C s5)以状态在序列中的位置为关键字对状态集Q进行排序6)C e←∅7)B c←∅8)for每个状态q i∈Q do9)if q i为模型的初始状态S then10)从Q中顺序取出下一状态q i+111)T←T∪{q i→q i+1}12)else if q i为一个全局背景状态then13)从Q中找出q i位置之后的第一个全局背景状态,记为q j14)T←T∪{q i→q j}15)从Q中找出q i位置之后的第一个CRM初始状态,记为q j16)T←T∪{q j→q i}17)for q i的每个前端模体状态m do18)创建一个CRM终止状态c e19)C e←C e∪{c e}20)T←T∪{m→c e}21)T←T∪{c e→q i}22)else if q i是一个CRM初始状态then23)T←T∪{q i→m i}24)else if q i是一个Motif状态then25)从Q中找出q i位置之后且不与它重叠的下一Motif状态,记为q j26)创建一个局部背景状态b c27)B c←B c∪{b c}28)T←T∪{q i→b c}29)T←T∪{b c→q j}30)Q←Q∪C e∪B c31)以状态在序列中的位置为关键字对状态集Q进行重新排序32)return Q和T1722自动化学报42卷图2Segmental HMM 状态转移图Fig.2The state transition diagram of segmental HMM在我们的模型中使用位置权重矩阵(Position weight matrice,PWM)[39]表示相应的模体,在算法中提前给定待搜索顺式调控模块所包含的可能模体集,所对应的PWM 集表示为PWMS.算法所要求的其他输入包括:搜索模体的p -value,以及待建模的转录调控序列.在上述Segmental HMM 状态转换图的构造算法中,第1行创建模型的初始状态和结束状态;第2行,根据所给p -value 找出给定模体集中模体及其反向互补的模体在序列中所有的出现实例.第3行,根据所找出的模体实例,分别构建模体状态、全局背景状态和顺式调控模块开始状态,对应的状态集分别为M 、B g 和C s .第5行对所有状态的集合进行排序.第6∼7行,分别初始化顺式调控模块结束状态集C e 和模体间局部背景状态集B c .第8∼31行,确定各状态间的转移,具体为:对于模型的初始状态(对应于第9∼11行),只需连接下一任意有效状态;对于全局背景状态,需要与下一全局背景状态(对应于第13∼14行)、相邻顺式调控模块初始状态(对应于第15∼16行)和顺式调控模块终止状态(对应于第17∼21行)相连;对于顺式调控模块初始状态,则只需连接到对应的模体状态,这对应于第22∼23行;对于模体状态,对应于第24∼29行,找出后续与当前状态不重叠的模体状态,然后创建相应的局部背景状态,并依次连接这些状态.第30∼31行,将新创建的顺式调控模块结束状态和模体间局部背景状态加入到总状态集Q ,并对Q 重新排序.关于上述构造算法的几点说明:1)在第3行中,对每个模体,分别创建了位于模体前后的两个可能的全局背景状态.所创建的全局背景状态,仅有一端位置是确定的(即模体的前端或模体的后端),在后面第13∼14行的操作中,会进一步将这些半连接的全局背景片段连接起来,形成一个大的全局背景;2)在第5行和第31行中,按照两个关键字(位置、状态的类型)将前面分别生成的、无次序的各种状态按照生成转录调控序列的时空顺序进行排序,以便于后面确定各状态的连接转移的操作;3)在算法中,我们显式地创建顺式调控模块的开始状态和结束状态,一方面,可以使结构更清晰,另一方面,也便于在推断时确定顺式调控模块的边界;4)对于第27行,为了简化顺式调控模块的结构表示模型,我们假设顺式调控模块内相邻的模体间是非重叠的;5)假定所给序列的长度为T ,在整个算法中,耗时的操作主要集中在:模体的查找,最坏时间复杂度为O(KT ),其中K 为PWM 的个数;查找后继状态的操作,最坏时间复杂度为O(T 2);对状态集的排序,最坏时间复杂度为O(T 2).因此,整个算法的时间复杂度为O(T 2).1.1.2状态转移概率在Segmental HMM 状态转换图中,每个状态对应模体、全局背景或局部背景这些类型的一个实例,状态之间的转移概率即为相应状态类型之间转移的概率.对于顺式调控模块内的模体状态m i 和模体状态m j 之间的转移概率,可由下式估计得到:a m i ,m j =A t (m i ),t (m j )Nk =1A t (m i ),t (m k )(2)其中,t (m )表示模体状态(对应于模体实例)m 所对应的模体类型,A 为模体状态间的转移计数.对于全局背景类型状态b g ,其反映了在长的序列区域中出现顺式调控模块的概率.由状态b g 到顺式调控模块状态的转移概率,或顺式调控模块到b g 状态的转移概率,由于即使在很长的调控序列中顺式调控模块的数目也相对较少,所能获得的数据难以训练出可靠的模型参数.为避免过拟合,可由经验估计得出,作为常量参数,在系统运行时设定.1.1.3片段长度分布全局背景长度和局部背景长度分别表示了顺式调控模块之间以及顺式调控模块内的模体之间的空白区域的长度分布.对于全局背景状态b g 和局部背11期郭海涛等:SegHMC:一种基于Segmental HMM 模型的顺式调控模块识别算法1723景状态b c ,我们假定其序列长度分别满足期望为w g 和w c 的几何分布;这种假定一方面反映了我们对顺式调控模块结构的不确定性,另一方面又为模型顺式调控模块内的模体的二元语法特征提供足够的适应性.在该假设下,背景序列长度为d 的概率为:P b (d )=(1−1w i )d −11w i (3)这里,b 表示b g 或b c ,w i 表示w g 或w c .对于模体状态m ,由于模体所对应的位置权重矩阵[39]是直接从数据库中获取的,其长度w m 及其特定位置碱基的概率都是已知的,所以模体状态上的序列长度d 的概率分布是特定的,即:P m (d)=1,d =w m 0,d=w m(4)1.1.4生成状态的发射模型在本文模型中,只有模体状态、全局背景状态和局部背景状态为生成状态.每种生成状态发射长度满足特定分布的碱基序列片段.对于全局背景和局部背景状态,我们分别使用k 阶Markov 模型和m 阶的局部Markov 模型.在局部Markov 模型中,位置t 处碱基的条件概率仅由以位置t 为中心长度为2D 的窗口内的序列片段来估计.这可采用记笔记的方式预先计算出每个位置碱基的条件概率,并存储计算的结果,需要时直接查表即得.对于模体的生成概率,在本文模型中,使用经典的PM 模型[40].假定模体实例为O ,该模体的PWM Θ=[θ1,θ2,···,θL ],其中θi (1≤i ≤L )为碱基频率的列向量,则模体状态所对应的碱基序列片段为O 的概率为:e (O )=L i =1θo i ,i(5)这里o i 为模体实例O 中第i 位置的碱基.1.2解码和训练算法在我们的模型中,将输入的序列分为训练集和测试集.在训练集上训练出模型参数后,使用已训练的模型识别给定测试集中所有序列的顺式调控模块,这一过程表现为解码出模型的最优状态路径过程.在Segmental HMM 模型中,最优状态路径可形式化定义为:给定长度为T 的转录调控序列(即观测序列)O =o 1o 2···o T ,设其对应的状态序列为Π=(π1,···,πT ),则该转录调控序列所对应的最优状态序列可表示为:ˆΠ=max ΠP (O,Π)(6)进一步设状态变量πi (i =1,···,T )的取值为{s 1,s 2,···,s N },s i ∈Q/{S ,E },i =1,···,N .加入模型的初始状态s 0=S 和终止状态s N +1=E ,状态序列Π的取值可表示为Π=s 0,s 1,···,s 1 d 1,s 2,···,s 2 d 2,···,s N ,···,s Nd N,s N +1 ,其中d i 表示状态s i 的序列片段长度,满足 Ni =1d i =T .基于上述定义,代入具体的模型参数,式(6)最终可表示为:ˆΠ=arg max s 1,···,s N {max d 1,···,d NN +1 i =0[a s i ,s i +1×P s i (d i )e (o t i +1···t i +1|s i )]}(7)这里P s i (d i )表示状态s i 的序列片段长度的概率分布,a s i ,s i +1为状态s i 到状态s i +1的转移概率,e (o t i +1···t i +1|s i )表示状态s i 生成观测序列片段o t i +1···t i +1的概率.由于缺少足够的标注数据,本文模型使用无监督的Baum-Welch 算法[34]直接从训练集中训练系统的模型参数.对于模型的初始状态概率,由于它只确定了输入序列的第一个位置的初始功能状态,在沿序列的后续操作中,其影响完全可以忽略,在本文模型中简单地由均匀分布随机生成.我们已提前标出对应片段的可能状态,创建了Segmental HMM 的状态转换图.因此,在解码时,不再需要通过使用像最大似然之类的方法去推断最可能的片段分割位置,可直接使用解码算法找出最优路径.为了求式(6)所对应的最优状态路径,本文使用基于动态规划的Veterbi 算法[34],记为SegHMC Veterbi,并把它作为模型的缺省设置.此外,为了提供足够的弹性,代替求最优状态路径,本文还给出了类似于MAP (Maximum a posteri-ori probability)算法[34]基于阈值的后验解码算法,该算法给出了最可能的状态路径,记为SegHMC threshold.与MAP 算法输出每个后验概率最大的序列区域相比,SegHMC threshold 输出后验概率大于指定阈值包含顺式调控模块的序列区域.在SegHMC threshold 算法中,本文搜索后验概率大于给定阈值且至少包含两个模体的连续区域作为候选顺式调控模块顺式调控模块.候选顺式调控模块区域的边界定义为首个模体的起始位置,和最后一个模体的结束位置.在本文模型中,选择的阈值范围为[0.45,0.70],在模型的后验推断中该范围内的阈值通常能给出好的性能.相对于完全输出后验概率最大的MAP 输出来讲,能通过合理地选取相应的阈值在精度和召回率之间达到一个平衡.。
特殊岗位培训计划英文翻译
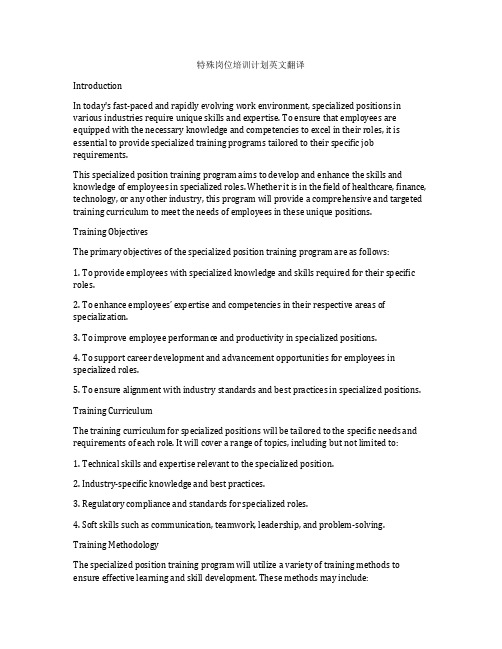
特殊岗位培训计划英文翻译IntroductionIn today's fast-paced and rapidly evolving work environment, specialized positions in various industries require unique skills and expertise. To ensure that employees are equipped with the necessary knowledge and competencies to excel in their roles, it is essential to provide specialized training programs tailored to their specific job requirements.This specialized position training program aims to develop and enhance the skills and knowledge of employees in specialized roles. Whether it is in the field of healthcare, finance, technology, or any other industry, this program will provide a comprehensive and targeted training curriculum to meet the needs of employees in these unique positions.Training ObjectivesThe primary objectives of the specialized position training program are as follows:1. To provide employees with specialized knowledge and skills required for their specific roles.2. To enhance employees’ expertise and competencies in their respective areas of specialization.3. To improve employee performance and productivity in specialized positions.4. To support career development and advancement opportunities for employees in specialized roles.5. To ensure alignment with industry standards and best practices in specialized positions. Training CurriculumThe training curriculum for specialized positions will be tailored to the specific needs and requirements of each role. It will cover a range of topics, including but not limited to:1. Technical skills and expertise relevant to the specialized position.2. Industry-specific knowledge and best practices.3. Regulatory compliance and standards for specialized roles.4. Soft skills such as communication, teamwork, leadership, and problem-solving. Training MethodologyThe specialized position training program will utilize a variety of training methods to ensure effective learning and skill development. These methods may include:1. Classroom-based instruction led by subject matter experts.2. Hands-on training using industry-specific tools and equipment.3. Virtual training sessions for remote employees or those in geographically dispersed locations.4. On-the-job training with mentorship and coaching from experienced professionals in the field.5. Case studies, workshops, and simulations to apply knowledge and skills in real-life scenarios.Training DeliveryTraining for specialized positions will be delivered through a combination of in-person, virtual, and on-the-job methods. This will accommodate the different learning preferences and work arrangements of employees in specialized roles. Training sessions will be scheduled to minimize disruption to regular work activities while ensuring maximum participation and engagement from employees.Training EvaluationEvaluation of the specialized position training program will be conducted to assess the effectiveness and impact of the training on employee performance and job proficiency. Various evaluation methods such as pre and post-training assessments, performance reviews, and feedback from employees and supervisors will be utilized to measure the training outcomes. This will provide insights into the success of the program and identify areas for improvement or additional training needs.Training ResourcesTo support the specialized position training program, a range of resources and materials will be made available to employees. These may include:1. Training manuals, guides, and reference materials specific to the specialized position.2. Access to online learning platforms and resources for self-paced learning and skill development.3. Industry-specific tools, equipment, and software for hands-on training and practice.4. Mentoring and coaching support from experienced professionals in specialized positions. Training ScheduleThe specialized position training program will be structured to accommodate the training needs of employees in various specialized roles. Training sessions may be organized in modules or phases to cover different aspects of the curriculum. The schedule will take intoconsideration the availability and workload of employees to ensure minimal disruption to their regular work responsibilities.ConclusionThe specialized position training program is designed to meet the unique and specific training needs of employees in specialized roles. By providing targeted training and development opportunities, employees will be better equipped to excel in their positions, contribute to the success of their organizations, and advance in their careers. This program will ensure that employees in specialized positions have the knowledge, skills, and competencies necessary to thrive in their roles and make valuable contributions to their respective industries.。
启动子生物信息学分析软件
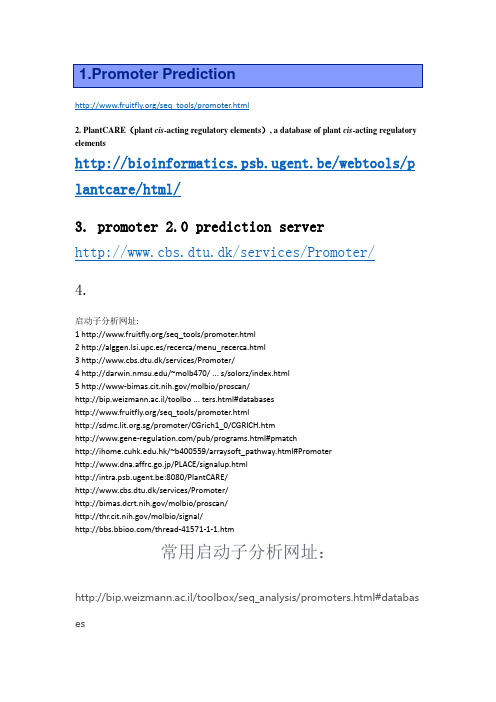
/seq_tools/promoter.html2. PlantCARE(plant cis-acting regulatory elements), a database of plant cis-acting regulatory elementshttp://bioinformatics.psb.ugent.be/webtools/p lantcare/html/3. promoter 2.0 prediction serverhttp://www.cbs.dtu.dk/services/Promoter/4.启动子分析网址:1 /seq_tools/promoter.html2 http://alggen.lsi.upc.es/recerca/menu_recerca.html3 http://www.cbs.dtu.dk/services/Promoter/4 /~molb470/ ... s/solorz/index.html5 /molbio/proscan/http://bip.weizmann.ac.il/toolbo ... ters.html#databases/seq_tools/promoter.html.sg/promoter/CGrich1_0/CGRICH.htm/pub/programs.html#pmatch.hk/~b400559/arraysoft_pathway.html#Promoterhttp://www.dna.affrc.go.jp/PLACE/signalup.htmlhttp://intra.psb.ugent.be:8080/PlantCARE/http://www.cbs.dtu.dk/services/Promoter//molbio/proscan//molbio/signal//thread-41571-1-1.htm常用启动子分析网址:http://bip.weizmann.ac.il/toolbox/seq_analysis/promoters.html#databas es/seq_tools/promoter.html.sg/promoter/CGrich1_0/CGRICH.htm/pub/programs.html#pmatch.hk/~b400559/arraysoft_pathway.html#Promoter http://www.dna.affrc.go.jp/PLACE/signalup.htmlhttp://intra.psb.ugent.be:8080/PlantCARE/http://www.cbs.dtu.dk/services/Promoter//molbio/proscan//molbio/signal/首先就是想直接查找有没有人做过这条基因的启动子,在pubmed中输入genename+promoter接着就想看看有没有数据库可以直接给出启动子序列的,很幸运竟然发现一个极好的启动子搜索讲义网站,如下,.il/workshops/bgu/promoterworkshop.html第一步就是要找到基因确定基因所在基因组区域,其中列出很多网站,不过偶还是习惯genbank,在gene栏中search某个基因,不要搞错基因种属!进入后即可看到该基因的详细条目,别眼花,就点击右侧link栏的Map viewer 链接,进入即可看到该基因在染色体上的形象定位,鼠标悬停在基因的起始位点时,即可在浏览器下方的状态栏中显示该位点在染色体上的明确定位,比如110997788,结合给出的基因跨度,比如110778899-117708899,即可大概确定该启动子在基因组中的大概定位,即110778899-110997788;第二步搞清楚基因组状态,我没搞太清楚,不过其中给的一个链接来查出启动子所在克隆(查出克隆号可以购买)/genome/guide/mouse/该链接中的clonefinder工具可以做到,只要提交你要查找的基因officialname就可以返回一个clonelist;第三步搜索启动子,其中可以用启动子数据库和启动子预测软件,当然如果启动子数据库中有最好,但很失望给出的数据库均不能查到!只好用启动子预测软件,使用了几个在线预测工具后觉得下面这个速度贼快,推荐http://www.cbs.dtu.dk/services/Promoter/我把该基因的dna序列submit之后返回了很多个PolII识别位点,到底哪个是呢?我个人理解启动子应该是翻译起始位点附近,所以在这个dna序列中定位翻译起始位点即可找到最近的Highly likely prediction,那么怎么定位呢?利用blast2这个利器,只要把dna和mrna序列粘贴进去提交就ok,正好在翻译起始位点上游几百bp有个识别位点,ok!启动子序列就是翻译起始位点上游大概1kb长度的序列了!直接用ensemble数据库的话,可以直接知道基因外显子和起始位点的位置,然后直接可以查到之前的序列,再选3k-4k的长度预测就比较方便了。
- 1、下载文档前请自行甄别文档内容的完整性,平台不提供额外的编辑、内容补充、找答案等附加服务。
- 2、"仅部分预览"的文档,不可在线预览部分如存在完整性等问题,可反馈申请退款(可完整预览的文档不适用该条件!)。
- 3、如文档侵犯您的权益,请联系客服反馈,我们会尽快为您处理(人工客服工作时间:9:00-18:30)。
A genetic algorithm for the detection of new cis-regulatory modules in sets of coregulatedgenesStein Aerts*Peter Van Loo Yves Moreau Bart De Moor Department of Electrical Engineering(ESAT-SCD)Katholieke Universiteit Leuven,Kasteelpark Arenberg10,3001Heverlee(Leuven),BelgiumRunning head:”Finding cis-regulatory modules”Web site:http://www.esat.kuleuven.ac.be/˜dna/BioI*Corresponding author:Tel:+32/16321801;Fax:+32/16321970;Email:stein.aerts@esat.kuleuven.ac.be1AbstractSummary:The implementation of a genetic algorithm is described that pro-vides a fast method to search for the optimal combination of transcription factorbinding sites in a set of regulatory sequences.Availability:The algorithm can be used transparently as a web service from within the Toucan software.Toucan can be accessed at http://www.esat.kuleuven.ac.be/∼saerts/software/toucan.php.A standalone version of the software is avail-able upon request.Contact:stein.aerts@esat.kuleuven.ac.beMicroarray and other high-throughput experiments in metazoans often yield sets of coexpressed genes that might share common cis-regulatory modules in their promoters or enhancers.Toucan(Aerts et al.,2003a)can be used to select putative regulatory regions from Ensembl(Hubbard et al.,2002)and to perform so called cis-regulatory analysis.This includes for example the annotation of putative transcription factor bind-ing sites(TFBSs)and the detection of new DNA motifs.Recently we have added a new web service that searches for the optimal combination of TFBSs in a sequence set using an A*tree search algorithm(Aerts et al.,2003b).The score function that is used is essentially the sum of the log-odds scores of the best hit of each individual TF within a window of specified length L,summed over all sequences in the set.Al-though this method guarantees tofind the optimal solution,it can be slow for certain parameter settings,for large sequence sets,or for modules that contain many different transcription factors(e.g.,more thanfive).Therefore we have implemented another search algorithm based on Genetic Algorithms(GA)that is faster and more practical. The algorithm starts with the creation of p random modules.A module is a vector that contains nΘposition specific probability matrices derived from TRANSFAC(Wingen-der et al.,2000)or from other matrix collections that are available on our server.The list of modules is sorted according to the score function mentioned above,and the s highest scoring modules are retained for the reproduction step.In the reproduction step the population grows back to size p by successive paring and mutating of ran-domly selected modules.When two modules are paired,for each position in the vector one element is chosen from either of the two parents,unless this element or a similar element is already present in the child module.Each element of a child module can then be mutated according to a mutation probabilityρ.After g generations the“fittest”module is selected as solution.The complexity of the algorithm is O(g(p−s)nq nΘ)where n is the number of sequences in the set and q is the average number of binding sites of a transcription factor on a sequence.Figure1.A summarizes the genetic algorithm procedure and Figure1.B visually shows a reproduction example.For the technical and biological validation of the algorithm we refer to the vali-dation of the A*algorithm(Aerts et al.,2003b).Since the GA does not guarantee optimality the user can perform multiple runs of the GA and select only those modules that are consistently found among different runs.In order to compare GA with A*in terms of accuracy(i.e.,does GA alsofind the optimal solution that A*finds?)and of speed,we have run the GA and the A*version on the same set of sequences as in(Aerts et al.,2003b).For a set of genes that are co-expressed with cyclin B2according to a time course microarray experiment during cell cycle in human HeLa cells(Whitfield et al.,2002),all human-mouse conserved sequence blocks within10kb upstream of the transcription start site are selected and scored with all position weight matrices of TRANSFAC using the MotifScanner.The CPU time(on a1GHz Pentium III processor running Red Hat Linux)taken by GA,setting L to100bp and g to100iterations,is2Figure1:A.Procedure of the genetic algorithm;g is the number of generations.B. Example of the generation of child modules by pairing(1)and mutations(2).Each geometricalfigure represents a transcription factor.C.The TOUCAN software envi-ronment showing the use of BioJava,Ensj-core and SOAP web services.about7,10,13and18minutes when nΘis set to4,5,6and7respectively.The time required for A*increases more dramatically with nΘ.For nΘ=4,A*takes about30 minutes,and for nΘ=5it takes betweenfive hours and three days depending on the data set and on L.nΘ>5was not feasible for this particular data set,neither in time, nor in memory.The maximum scores of three GA-runs with100iterations is,for nΘ=3,4,5exactly the same(and thus the optimal module is found)as in A*.Although we have no results of A*for nΘ>5,the results of GA for larger nΘ’s show the same scores in multiple runs of GA(e.g.,in two out of three runs),and therefore these can be assumed to be the optimal scores.In conclusion,the GA version of the ModuleSearcher is able tofind the optimal combination of binding sites without a limitation of the number of sites, and within a fraction of the time that A*needs.A newly found module should be validated in silico by screening the full genome of the species that was used.For this purpose several methods have been published re-cently that take the individual matrices of a module as input and that return putative hits with a certain statistical significance:COMET(Frith et al.,2002),MSCAN(Johansson et al.,2003),Stubb(Sinha et al.,2003),CREME(Sharan et al.,2003),MCAST(Bailey &Noble,2003),and ModuleScanner(Aerts et al.,2003b).A module that was found in the“training set”by using the ModuleSearcher(either the A*or the GA version)can be retained for experimental validation in case(1)multiple top-scoring genes found in the genome-scan overlap with the genes of the training set;and(2)the top-scoring genes are functionally coherent and related to the function of the genes in the training set.The latter can be investigated by comparing the over-represented Gene Ontology annotations of both gene sets,using tools like FatiGO(io.es/), GOMiner(Zeeberg et al.,2003),EASE(/david/ease.htm),or GO4G(Coessens et al.,2003).The ModuleSearcher is available within Toucan.This is a Java application that can be launched directly from our web site using Java Web Start.Behind the user interface we have made extensive use of the BioJava library for all sequence and annotation ac-tions.The bottom layer of the application serves two goals:data access classes and web service client classes.The Ensj-core library of Ensembl is used to retrieve genes,3transcripts,and annotations either from the public Ensembl database or from a local Ensembl installation.Via the MySQL classes direct queries to the Ensembl MySQL database are also possible.The Apache SOAP(Simple Object Access Protocol)imple-mentation is used to send requests in XML format to services running on our servers. For most services,a fastA formatted string together with some parameters of the al-gorithm is sent to the service(running within Tomcat on Apache),and GFF formatted features are sent back to Toucan after the execution of the algorithm.For reasons of efficiency we do not run the methods on the web server itself,but send RMI(Remote Method Invocation)requests to a dedicated machine that performs all calculations.Fig-ure1.C shows a detailed view of the design of the Toucan platform with its components and its web services.The following web services are currently available:MotifScanner,MotifLocator, MotifSampler,A VID/VISTA(Bray et al.,2003),Footprinter(Blanchette&Tompa, 2002),and ModuleSearcher.Tofind modules,the user runs either the MotifScanner, the MotifLocator,or the MotifSampler to annotate putative TFBSs and then he/she runs the ModuleSearcher on the annotated set.A manual,tutorial,installation instruc-tions,news list,references,and a list of all available web services can be found on the application’s website.AcknowledgementsStein Aerts is research assistant of the K.U.Leuven.Yves Moreau is a postdoctoral researcher of the FWO-Vlaanderen,currently on leave at the Center for Biological Sequence Analysis,Danish Technical University,Lyngby,Denmark.Bart De Moor is Full professor of the K.U.Leuven. Research supported by Research Council KUL:GOA-Mefisto666,IDO;Flemish Government: FWO:projects G.0115.01,G.0240.99,G.0407.02,G.0413.03,G.0388.03,G.0229.03,ICCoS, ANMMM;AWI;IWT;STWW-Genprom,GBOU-McKnow,GBOU-SQUAD,GBOU-ANA;Bel-gian Federal Government:DWTC(IUAP IV-02and IUAP V-22);EU:CAGE;ERNSI;Contract Research/agreements:VIB.ReferencesAerts,S.,Thijs,G.,Coessens,B.,Staes,M.,Moreau,Y.&De Moor,B.(2003a)Toucan:de-ciphering the cis-regulatory logic of coregulated genes.Nucleic Acids Res,31(6),1753–1764.Aerts,S.,Van Loo.,P.,Thijs,G.,Moreau,Y.&De Moor.,B.(2003b)Computational detection of cis-regulatory modules.Bioinformatics,19Suppl2,II5–II14.Bailey,T.&Noble,W.(2003)Searching for statistically significant regulatory modules.Bioin-formatics,19Suppl2,II16–II25.Blanchette,M.&Tompa,M.(2002)Discovery of regulatory elements by a computational method for phylogenetic footprinting.Genome Res,12(5),739–748.Letter.Bray,N.,Dubchak,I.&Pachter,L.(2003)A VID:A Global Alignment Program.Genome Res, 13(1),97–102.Coessens,B.,Thijs,G.,Aerts,S.,Marchal,K.,De Smet.,F.,Engelen,K.,Glenisson,P.,Moreau, Y.,Mathys,J.&De Moor.,B.(2003)INCLUSive:a web portal and service registry for microarray and regulatory sequence analysis.Nucleic Acids Res,31(13),3468–3470.4Frith,M.C.,Spouge,J.L.,Hansen,U.&Weng,Z.(2002)Statistical significance of clusters of motifs represented by position specific scoring matrices in nucleotide sequences.Nucleic Acids Res,30(14),3214–3224.Hubbard,T.,Barker,D.,Birney,E.,Cameron,G.,Chen,Y.,Clark,L.,Cox,T.,Cuff,J.,Curwen, V.,Down,T.,Durbin,R.,Eyras,E.,Gilbert,J.,Hammond,M.,Huminiecki,L.,Kasprzyk,A.,Lehvaslaiho,H.,Lijnzaad,P.,Melsopp,C.,Mongin,E.,Pettett,R.,Pocock,M.,Pot-ter,S.,Rust,A.,Schmidt,E.,Searle,S.,Slater,G.,Smith,J.,Spooner,W.,Stabenau,A.,Stalker,J.,Stupka,E.,Ureta-Vidal,A.,Vastrik,I.&Clamp,M.(2002)The Ensemblgenome database project.Nucleic Acids Res,30(1),38–41.Johansson,O.,Alkema,W.,Wasserman,W.&Lagergren,J.(2003)Identification of functional clusters of transcription factor binding motifs in genome sequences:the MSCAN algo-rithm.Bioinformatics,19(Suppl1),I169–I176.Sharan,R.,Ovcharenko,I.,Ben-Hur,A.&Karp,R.(2003)CREME:a framework for identifying cis-regulatory modules in human-mouse conserved segments.Bioinformatics,19(Suppl1),I283–I291.Sinha,S.,Van Nimwegen,E.&Siggia,E.(2003)A probabilistic method to detect regulatory modules.Bioinformatics,19(Suppl1),I292–I301.Whitfield,M.L.,Sherlock,G.,Saldanha,A.J.,Murray,J.I.,Ball,C.A.,Alexander,K.E., Matese,J.C.,Perou,C.M.,Hurt,M.M.,Brown,P.O.&Botstein,D.(2002)Identification of genes periodically expressed in the human cell cycle and their expression in tumors.Mol Biol Cell,13(6),1977–2000.Wingender,E.,Chen,X.,Hehl,R.,Karas,H.,Liebich,I.,Matys,V.,Meinhardt,T.,Pruss,M., Reuter,I.&Schacherer,F.(2000)TRANSFAC:an integrated system for gene expression regulation.Nucleic Acids Res,28(1),316–319.Zeeberg,B.R.,Feng,W.,Wang,G.,Wang,M.D.,Fojo,A.T.,Sunshine,M.,Narasimhan, S.,Kane,D.W.,Reinhold,W.C.,Lababidi,S.,Bussey,K.J.,Riss,J.,Barrett,J.C.& Weinstein,J.N.(2003)GoMiner:a resource for biological interpretation of genomic and proteomic data.Genome Biol,4(4),R28.5。