基于小波包分解的苍白球的神经尖峰特征提取(IJEM-V1-N5-6)
小波分解与PCA方法的掌纹特征提取方法
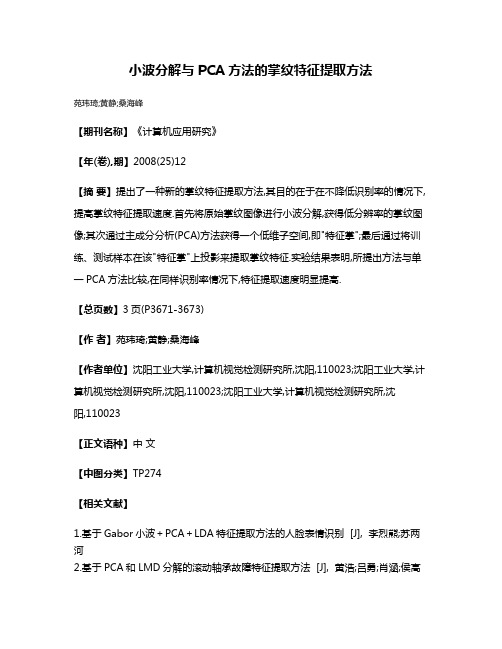
小波分解与PCA方法的掌纹特征提取方法
苑玮琦;黄静;桑海峰
【期刊名称】《计算机应用研究》
【年(卷),期】2008(25)12
【摘要】提出了一种新的掌纹特征提取方法,其目的在于在不降低识别率的情况下,提高掌纹特征提取速度.首先将原始掌纹图像进行小波分解,获得低分辨率的掌纹图像;其次通过主成分分析(PCA)方法获得一个低维子空间,即"特征掌";最后通过将训练、测试样本在该"特征掌"上投影来提取掌纹特征.实验结果表明,所提出方法与单一PCA方法比较,在同样识别率情况下,特征提取速度明显提高.
【总页数】3页(P3671-3673)
【作者】苑玮琦;黄静;桑海峰
【作者单位】沈阳工业大学,计算机视觉检测研究所,沈阳,110023;沈阳工业大学,计算机视觉检测研究所,沈阳,110023;沈阳工业大学,计算机视觉检测研究所,沈
阳,110023
【正文语种】中文
【中图分类】TP274
【相关文献】
1.基于Gabor小波+PCA+LDA特征提取方法的人脸表情识别 [J], 李烈熊;苏两河
2.基于PCA和LMD分解的滚动轴承故障特征提取方法 [J], 黄浩;吕勇;肖涵;侯高
雁
3.一种基于形态小波的在线掌纹的线特征提取方法 [J], 戴青云;余英林
4.基于小波变换和信息熵的掌纹特征提取方法 [J], 谢平;周志丰
5.基于小波包分解法的超声信号特征提取方法研究 [J], 江文鸾
因版权原因,仅展示原文概要,查看原文内容请购买。
基于小波分解的光谱特征提取算法研究
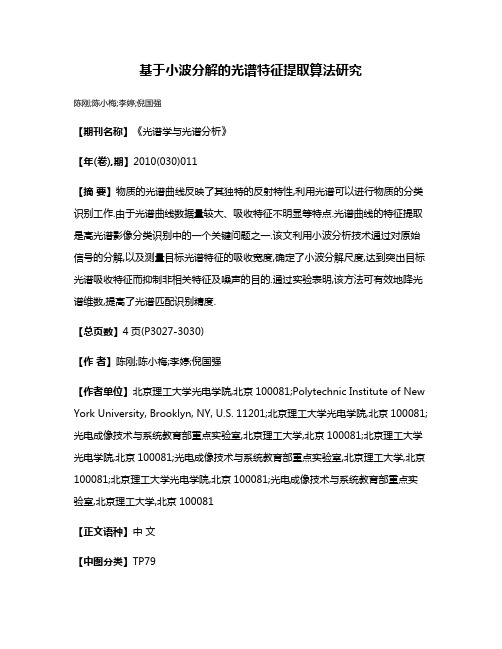
基于小波分解的光谱特征提取算法研究陈刚;陈小梅;李婷;倪国强【期刊名称】《光谱学与光谱分析》【年(卷),期】2010(030)011【摘要】物质的光谱曲线反映了其独特的反射特性,利用光谱可以进行物质的分类识别工作.由于光谱曲线数据量较大、吸收特征不明显等特点.光谱曲线的特征提取是高光谱影像分类识别中的一个关键问题之一.该文利用小波分析技术通过对原始信号的分解,以及测量目标光谱特征的吸收宽度,确定了小波分解尺度,达到突出目标光谱吸收特征而抑制非相关特征及噪声的目的.通过实验表明,该方法可有效地降光谱维数,提高了光谱匹配识别精度.【总页数】4页(P3027-3030)【作者】陈刚;陈小梅;李婷;倪国强【作者单位】北京理工大学光电学院,北京100081;Polytechnic Institute of New York University, Brooklyn, NY, U.S. 11201;北京理工大学光电学院,北京100081;光电成像技术与系统教育部重点实验室,北京理工大学,北京100081;北京理工大学光电学院,北京100081;光电成像技术与系统教育部重点实验室,北京理工大学,北京100081;北京理工大学光电学院,北京100081;光电成像技术与系统教育部重点实验室,北京理工大学,北京100081【正文语种】中文【中图分类】TP79【相关文献】1.基于离散小波变换的高光谱特征提取中分解尺度的确定方法 [J], 李军;李培军;郭建聪2.基于改进阈值的小波分解和经验模态分解的人体脉搏信号滤波算法研究 [J], 麻芙阳;谢锐3.面向混合像元分解的光谱维小波特征提取 [J], 吴波;周小成;高海燕4.基于二维Gabor小波与AR-LGC的人脸特征提取算法研究 [J], 倪永婧;孙袆;岳莹;郭志萍;高丽慧;刘微5.基于改进小波变换的QRS特征提取算法研究 [J], 侯晓晴;仝泽友;刘晓文因版权原因,仅展示原文概要,查看原文内容请购买。
基于小波包能量的脑电信号特征提取方法_徐宝国

特征提取是脑机接口中的关键技术之一. 由于 脑电信号十分微弱且本身非常复杂, 要从非平稳的 脑电信号中有效、快速地区分不同意识活动的成分 是对信号处理理论的严峻挑战. 常用的特征提取方 法有 功 率 谱 法 [ 4] 、FFT 法 [ 5] 、SFT 法、AR 法 [ 6] 、 A AR 法 [ 7] 、ICA 法、双谱估计法 [ 8] 、小波分析法 [ 9 ] 等. 功率谱法利用有限长度信号来估计脑电信号的 功率谱密度, 采用该方 法获得的特征 参数意义明 确, 在人们最初认识脑电、分析脑电信号与大脑活 动关系中起到了重要作用; 但是, 在脑电信号数据 长度较短时, 该方法得到的信号统计特征不明显. FFT 法在分析突变信号的频谱时具有许多局限性. SFT 法虽然在一定程度上克服了标准 FFT 法不具 备局部分析能力的缺点, 但也存在自身不可克服的 缺陷: SFT 法是在时间窗内对信号特征取平均值, 时间窗内的信号越短, 获得的时间分辨率就越高; 根据信号测不准原理, 要得到高的频率分辨率, 则 需要长的时间窗. 由此可知, 时间局域化性质和频 率局域化性质是矛盾的. AR 模型反应了信号的时 变特性, 数据长度决定了参数估计的分辨率. AAR 模型参数随每一样本点的输入而改变, 因而能够更 好地反映大脑的状态, 但是该方法更适合分析平稳 信号. 小波分析法在低频时时间分辨率较低, 频率 分辨率较高; 在高频时时间分辨率较高, 频率分辨 率较低. 这正符合低频信号变化缓慢而高频信号变 化较快的特点, 因而更适合分析非平稳的脑电 信号.
基于小波包分析的虹膜特征提取方法

基于小波包分析的虹膜特征提取方法
王琪;费耀平
【期刊名称】《计算机工程与应用》
【年(卷),期】2006(042)013
【摘要】提出了一种新的虹膜特征提取方法.针对虹膜纹理图像的特征表示主要集中在中高频能量部分,对图像进行二级小波包分解,并提取出第一级和第二级的中高频部分的能量分量作为特征向量,并采用欧几里德距离作为模式识别方法.克服了用小波分解只提取虹膜的低频部分,从而不能充分地反映纹理的特征的缺陷.试验结果和数据表明了此算法的合理性和有效性.
【总页数】3页(P60-62)
【作者】王琪;费耀平
【作者单位】中南大学信息科学与工程学院,长沙,410075;中南大学信息科学与工程学院,长沙,410075
【正文语种】中文
【中图分类】TP391
【相关文献】
1.基于小波包变换的特征提取方法分析fMRI数据 [J], 支联合;谭素敏;杨建国
2.基于小波包和倒频谱分析的颤振特征提取方法 [J], 王二化;刘忠杰
3.基于奇异值分解和小波包分析的液压泵振动信号特征提取方法 [J], 何庆飞;姚春江;陈桂明;陈小虎;杨庆
4.基于小波包分析的颅颌面纹理特征提取方法 [J], 黄丽雯;庞柯;汪鑫;施帮利;王涛;
炊万年
5.一种基于小波包和主成分分析的超声信号特征提取方法 [J], 肖力伟
因版权原因,仅展示原文概要,查看原文内容请购买。
基于小波能量矩的高分辨距离像特征提取

火 力 与 指 挥 控 制
FieCo to r n r 1& Co mm a d Co to n nr1
第 3 7卷 第 9期 21 0 2年 9月
文 章 编 号 :0 20 4 ( 0 2 0—0 20 10 —6 0 2 1 ) 90 8 —3
c v nton e ho i i p ov d,a a ne m e ho t e t a t l c l n r y a e wa e e e r — on e i alm t d s m r e nd w t d o x r c o a e e g b s d on v l t ne gy mome t s pr s nt d i t i p p r The e n i e e e n h s a e . n w me ho a b u e i f a u e xt a to o h gh r ng — t d c n e s d n e t r e r c i n f i a e r s uton r d r p o ie .Thee r y mo ntf a ur n i a e oton y t e e e gy l v lo a h ba e ol i a a r fl s ne g - me e t e i d c t s n l h n r e e n e c nd,but a s he e r s rbu i he t m p r ld m an a i .Si lo t ne gy dit i ton on t e o a o i x s mul ton r s ls o a ge e o ii ho t a i e u t ft r tr c gn ton s w he f a ur e r c i me h b s d n e t e xt a ton t od a e o wa l t n r y- m e i be t r t n h c nv nton l ve e e e g mo nt s te ha t e o e i a met d n ho i r s c ft e o e pe t o he r c gnii n r t s to a e . Ke y wor ds: v l ta a yss, ne g e e gy mo nt f a u e e r c in wa e e n l i e r y, n r — me ,e t r xt a to
基于小波模极值的虹膜特征提取算法

( p r n f o ue , it nUnv r t o rF re, h n c u 3 0 2 De at t mp t Ava o ies y f oc C a g h n 1 0 2 ) me o C r i i Ai
段一种 常见的小波模极值算法 ,阐述 了算法技 术、解决 方案和效果 。
关键词 : 虹膜识别 ; 特征 提 取 ; 波模 极 值 算 法 小
W a ee o l a i u g rt v l tM du usM x m m Al o ihm fI i a ur t a to o rsFe t e Ex r c i n Pha e s
3 小 波模 极值 技术 和解 决方 案
() 对 预 处 理 后 的 虹 膜 纹 理 进 行 一 种 局 部 支 撑 的 具 任 意 1
M a chng s … l i
( 2 ’ )
次光滑小波变换 。
图 1 粗 匹配 细 化 结 果 直 方 图 :横 轴 表 示 相 似 特 征 点 距离量 度值 ;纵轴 以比例 的方式表示比对次数
oyo l rh g f gi m、teSl n r et n f c. a ot h o igpo c a de et v j
Ke r s rsi e t c t n; h e t r x r ci n; v l t d l sma i m lo t m y wo d :i n i ai i d i f o t e fa u e e t t a o wa e e mo u u x mu a g r h i
极值就是一种常用算 法 ,能够有效完成特征提取功能 。
2 特 征 提取
基于改进小波包与样本熵的表面肌电信号特征提取
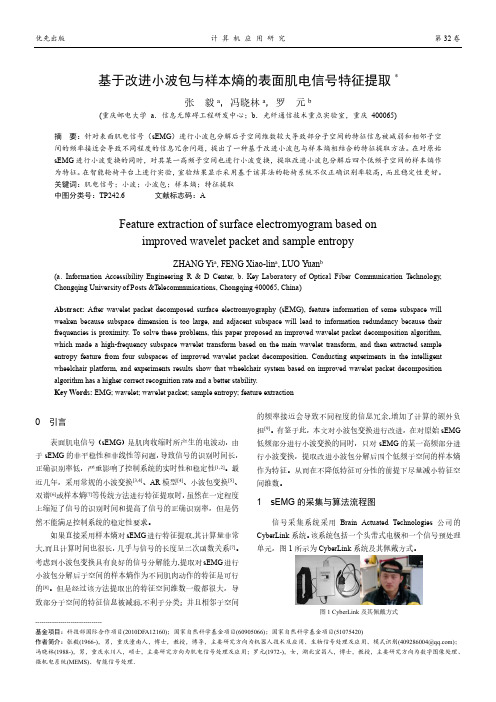
优先出版 计 算 机 应 用 研 究 第32卷--------------------------------基金项目:科技部国际合作项目(2010DFA12160);国家自然科学基金项目(60905066);国家自然科学基金项目(51075420)作者简介:张毅(1966-),男,重庆潼南人,博士,教授,博导,主要研究方向为机器人技术及应用、生物信号处理及应用、模式识别(409286004@);冯晓林(1988-),男,重庆永川人,硕士,主要研究方向为肌电信号处理及应用;罗元(1972-),女,湖北宜昌人,博士,教授,主要研究方向为数字图像处理、微机电系统(MEMS)、智能信号处理.基于改进小波包与样本熵的表面肌电信号特征提取 *张 毅a ,冯晓林a ,罗 元b(重庆邮电大学 a .信息无障碍工程研发中心;b .光纤通信技术重点实验室,重庆 400065)摘 要:针对表面肌电信号(sEMG )进行小波包分解后子空间维数较大导致部分子空间的特征信息被减弱和相邻子空间的频率接近会导致不同程度的信息冗余问题,提出了一种基于改进小波包与样本熵相结合的特征提取方法。
在对原始sEMG 进行小波变换的同时,对其某一高频子空间也进行小波变换,提取改进小波包分解后四个低频子空间的样本熵作为特征。
在智能轮椅平台上进行实验,室验结果显示采用基于该算法的轮椅系统不仅正确识别率较高,而且稳定性更好。
关键词:肌电信号;小波;小波包;样本熵;特征提取 中图分类号:TP242.6 文献标志码:AFeature extraction of surface electromyogram based onimproved wavelet packet and sample entropyZHANG Yi a , FENG Xiao-lin a , LUO Yuan b(a. Information Accessibility Engineering R & D Center, b. Key Laboratory of Optical Fiber Communication Technology, Chongqing University of Posts &Telecommunications, Chongqing 400065, China)Abstract: After wavelet packet decomposed surface electromyography (sEMG), feature information of some subspace will weaken because subspace dimension is too large, and adjacent subspace will lead to information redundancy because their frequencies is proximity. To solve these problems, this paper proposed an improved wavelet packet decomposition algorithm, which made a high-frequency subspace wavelet transform based on the main wavelet transform, and then extracted sample entropy feature from four subspaces of improved wavelet packet decomposition. Conducting experiments in the intelligent wheelchair platform, and experiments results show that wheelchair system based on improved wavelet packet decomposition algorithm has a higher correct recognition rate and a better stability.Key Words: EMG; wavelet; wavelet packet; sample entropy; feature extraction0 引言表面肌电信号(sEMG )是肌肉收缩时所产生的电波动,由于sEMG 的非平稳性和非线性等问题,导致信号的识别时间长,正确识别率低,严重影响了控制系统的实时性和稳定性[1,2]。
基于小波熵的弹丸激波信号特征提取方法研究

Abta tDu o ifu n eo ait ffco si h i,t ee p rme tlp 0e t es o k v in l h v o f sr c: et n le c faV r yo a t r n te ar h x e i na rjci h c wa esg as a ea lto e l
中 图 分 类 号 : J l. 3 T 4 0 3 文献 标 志码 : A
The S u y 0 a u e Ex r c i n M e h d f t d n Fe t r t a t0 t 0 s0
P 0 t eS 0 k V in l ae n W a ee n r p rjci h c wa eSg a sd0 V lt t0 y e l B E
t a f m ・ t i na n r y wasc m p r m e a ie i t m a n ofs ae n h a r a d t e w a ee ne gy s e — r nsor he sg le e g o a t nt lz d n hedO i c ls i t e p pe . n h v lte r p c t u nd e r r m a ntopy w e e i r duc d a he fat r st e lc he s a itc c r c e . h xDe i e a e u t h r ntO e s t e u e o r fe tt t ts i ha a t r T e e rm nt lr s ls s ow ha t t
ZH A0 n i n W A NG m i Yi gla g, Li ng, A N n H Ya
( t n lKe a 0 ao y frElcr Ic Me s r r e 1 h oo y, rh UI v ri fChn , y a 3 O 1 C}n ) Nai a 0 y L b r t r o e to l a u en m n l g No t l e S y o ia 1 u n O 0 5 , 1 a i i t i
- 1、下载文档前请自行甄别文档内容的完整性,平台不提供额外的编辑、内容补充、找答案等附加服务。
- 2、"仅部分预览"的文档,不可在线预览部分如存在完整性等问题,可反馈申请退款(可完整预览的文档不适用该条件!)。
- 3、如文档侵犯您的权益,请联系客服反馈,我们会尽快为您处理(人工客服工作时间:9:00-18:30)。
I.J. Engineering and Manufacturing 2011, 5, 46-51Published Online October 2011 in MECS ()DOI: 10.5815/ijem.2011.05.06Available online at /ijemNeural Spike Feature Extraction of Globus Pallidus Based onWavelet Package DecompositionYan HE a, Jue WANG a, Guangjun ZHANG a, Guodong GAO b,*a The Key Laboratory of Biomedical Information Engineering of Ministry of Education, and Institute of Biomedical Engineering, School of Life Science and Technology, Xi’an Jiaotong University, Xi’an 710049,Chinab Department of Neurosurgery, Tangdu Hospital, Xi'an 710049, ChinaAbstractIn this study, features of neural signal recordings with microelectrode injected into the internal globus pallidus of non-human primates before and after 1-methyl-4-phenyl-1, 2, 3, 6-tetrahydropyridine (MPTP) treatment which induces Parkinson’s d isease (PD) were investigated. Neural oscillations were analyzed using the measure of wavelet spectrum and singular value extraction. Differences in the time-frequency analysis were observed between groups. Results showed that the PD states had significantly higher amplitudes of spectrum. Singular values extracted from wavelet coefficients after wavelet package decomposition were used to differentiate pathological changes from normal states, and the singular values of the reconstructed wavelet coefficients of the PD states were statistically smaller than controls. We speculate that the irregular high frequency oscillations and reduced singular values observed in the pathological firings are consistent with the previous view that a loss of information transmission in the neural circuitry underlies PD and these measurements could be of diagnostic and monitoring values of the disease state.Index Terms: Parkinson’s Disease; Neuronal Oscillation; Wavelet Packet Decomposition; Singular Value© 2011 Published by MECS Publisher. Selection and/or peer review under responsibility of the Research Association of Modern Education and Computer Science.1.IntroductionThe pathophysiology of the PD disease is related with the loss of pigmented dopaminergic neurons which leads to alterations in the activity of the neural circuits within the basal ganglia that regulate movement [1-2]. The role of dopamine within the basal ganglia appears to be complex [3] while PD is an age-related neurological disorder viewed as a dysfunction of information processing or disruption of the dynamics of neural information processing in the neural circuits in the basal ganglia. The information processing in the Parkinsonism basal ganglia involves not only activities of individual rhythms, but also interactions between rhythms.* Corresponding author:E-mail address: taohuacun@Many previous studies have reported changes in the neural activity of globus pallidus (GP) neurons in MPTP-treated monkeys compared with healthy ones while firing rates of the internal globus pallidus (GPi) cells are increased, and the external globus pallidus (GPe) cells display decreased firing rates in PD subjects.Another example is the low frequency modulation of firing activity that arises in PD[4]. The cross-correlation analysis of simultaneously recorded pallidal cells in MPTP-treated monkeys revealed that the spiking activities of many of these neurons are synchronized [5]. Mean discharge rates, instantaneous discharge rates, interspike interval (ISI) histograms, and autocorrelation have all been used to assess spontaneous neuronal activities. However, due to the complexity and large amounts of data, the previous studies are unable to uncover more dynamical changes hidden in the spike train, more effective neuronal spike train decoding and feature extraction methods are needed for delineation and characterization of these changes in discharge neural signal. In this paper, we will mainly focus on the research of feature extraction of neuronal oscillatory activity of Gpi before and after incidence of the disease determined by the MPTP intervention in the animal model and try to capture the dominant dynamic changes of the neuronal firing patterns under PD state.2.Material and MethodsWavelet decomposition can be realized by the Mallat Algorithm [6-7]. Wavelet packet decomposition (WPD) [8-9], sometimes known as just wavelet packets is a wavelet transform where the signal is passed through more filters than the digital wavelet transform, and it’s an advantageous and favorable method in digital signal processing especially on the non-stationary signal such as neuronal spike trains which composed of different frequencies simultaneously. The capability of this mathematical microscope to analyze different scales of neural rhythms is proved to be a powerful tool for investigating multi-scale oscillations of the brain signals. Wavelet spectrum is adopted for the spectral representation of the neural signal analysis at first after signal de-noising by wavelet transform. The coefficients derived from the temporal spike profiles could be taken as feature extraction parameters [10-11]. For n levels of decomposition the WPD produces 2n (n=1, 2, 3…) different sets of coefficients as opposed to 3n + 1 set for the discrete wavelet transform. In the theory of Singular value decomposition, any m×n real valued matrix A of rank r, where r ≦min (m, n), can be decomposed as A = UΣVT, where U is an m × n matrix with UTU = I, V is an orthogonal n × n matrix, and is an n× n diagonal matrix. The diagonal elements α1, α2…αn of Σ can be arranged in a non-increasing order and are called the singular values of A (SV).The energy of special sub-bands and corresponding coefficients of wavelet packetdecomposition especially the largest singular values are selected as features which have maximal discriminability according to the Fisher distance criterion and can describe the main features of the processing signals based on the quantification of energy found in specific frequency bands at specific time locations during each spike profile.The objective of feature extraction is to produce a suitable representation of neural signals for pathology recognition. We adopt one-dimensional case with a 3 level decomposition for feature extraction. The daubechies 4 (db4) wavelet [12] is chosen as the wavelet basis for neuron spike train analysis because of its adaptability to the spike shape by comparing the decomposition level required while keeping the energy as much as possible and the optimization criteria is set as entropy. Since that energy distribution of the original signal is related with the reconstructed decomposed wavelet coefficients, the projection coefficients have close biological relevance and would demonstrate statistical significant difference between signals.3.ResultsThe MPTP induced monkey model of PD are produced the same way as it was described in [13]. Recording began as the stable neuronal firing came out and it would last for 300s or longer with the electrode injected in the GPi [14].We select two dominant kinds of representative neural spiking trains recorded from the primate model as follows: 1) rest spontaneous spike trains of GPi neuron of normal healthy monkey; 2) rest spontaneous spike trains of GPi neurons under pathological condition after MPTP intervention.Fig. 1 and Fig. 2 demonstrate the normalized wavelet spectrum of the dominant neural signals. At one time spike trains are chosen according to their representation of the main characteristic of the data, and then the statistical result is taken as final measurement for further discussion.As for the spectral analysis, the pathological neuron spike demonstrates significant irregular higher frequency oscillation compared with the normal ones. The decomposition of neural signals into transient oscillations could yield new knowledge about real signal components and shed light on neuronal mechanisms of information processing. As for WPD, its coefficients could has been taken as the measure to describe neural signals, which has an advantage that demonstrates signal's character by several coefficients, and the singular value of the matrix has two dominant features while one is its strong stability and the other is its inheritance of in the matrix reflecting underlying information.Eight wavelet coefficients are selected as the basis matrix and Fig. 3 and Fig. 4 illustrates the largest singular values of WPD coefficients under the pathological and normal conditions, and the pathological ones are lower than that of the normal ones. More details are illustrated in Table 1. These irregular higher frequency oscillations are closely related with the loss of energy and the miscarriage of information which induce the symptoms of PD.Fig. 1. Wavelet spectrum of neural signal under normal stateFig. 2. Wavelet spectrum of neural signal under pathological stateFig. 3. The largest singular value of the eight level wavelet coefficients (d1-d8) of one sampleTable 1. Paired-sample test for siingular values under pd state and normal state4.ConclusionClarifying the relationship between intrinsic oscillations of neural signals and the pathological states seemsto be efficacious by way of WPD, which is simple and easier for implementation although the mathematicalprinciple is complex. It helps us effectively explore the progressive but fatal changes not only in the frequency domain but also in temporal aspect; these evidences might be a strong support for the prediction, diagnosis and the evaluation of clinical treatment.The objective of feature extraction is to produce a suitable representation of neural signals for PD recognition; we deduce the singular value is the signature of the information loss and incidence of the disease which could be used as the parameter for further diagnosis and surgery evaluation.AcknowledgementsWe thank Prof. Guodong GAO and his group for their cooperation in the experiment.This work was supported in part by the grant from the National Natural Science Foundation (Grant No.30670660, Grant No.10872156).References[1]McCormick D.A, Bal T, Sleep and arousal: thalamocortical mechanisms, Annual Review of Neuroscience20 (1997) 185–215.[2]Bar-Gad, H Bergman H, Stepping out of the box: information processing in the neural networks of thebasal ganglia, CURRENT OPINION IN NEUROBIOLOGY 11 (2001) 689-695.[3]David Williams, Marina TIjssen, Gerard van Bruggen, Dopamine-dependent changes in the functionalconnectivity between basal ganlia and cerebral cortex in humans, Brain 125 (2002) 1558-1569.[4]S. Morrison, G. Kerr, K.M. Newell, P.A. Silburn, Differential time- and frequency-dependent structure ofpostural sway and finger tremor in Parkinson’s disease, Neuroscience Letters 443 (2008) 123–128.[5]Aoki F, Changes in power and coherence of brain activity in human sensorimotor cortex duringperformance of visuomotor tasks, Biosystems 63 (2001) 89–99.[6]Mallat S, A theory for multiresolution signal decomposition: the wavelet representation, IEEE PatternAnalysis and Machine Intelligence 11 (1989) 674-693.[7]Mallat S, A Wavelet Tour of Signal Processing, Academic Press; 2nd edition, 1999.[8]Daubechies I, Ten lectures on wavelets, CBMS-NSF conference series in applied mathematics. SIAMEd.1992[9]Daubechies I, The wavelet transform of time-frequency localization and signal analysis, IEEE transactionson Information Theory 36 (1990) 961-1005.[10]Donoghue J. P, Neural discharge and local field potential oscillations in primate motor cortex duringvoluntary movements, Journal of Neurophysiology 79 (1998) 159–173.[11]Simona Mrakic-Sposta , Sara Marceglia, Marcello Egidi, Giorgio Carrabba, Extracellular spikemicrorecordings from the subthalamic area in Parkinson’s disease,Journal of Clinical Neuroscience 15 (2008) 559–567.[12]Zenadic Z, J.W. Burdick, Spike detection using continuous wavelet transform, IEEE Transactions onBiomedical Engineering 52 (2005) 74-87.[13]J M. Hurtado, Charles M. Gray, Laszlo B. Tamas, Karen A. Sigvardt, Dynamics of tremor-relatedoscillations in the human globus pallidus: A single case study, PNAS 96 (1999) 1674-1679.[14]Yan He, Jue Wang, Globus pallidus neuron spike time series prediction based on local-region multi-stepforecasting model, 3rd International Conference on Intelligent System and Knowledge Engineering (2008) 224 – 229.。