贝叶斯优化算法全面解析-图文
朴素贝叶斯参数调优-概述说明以及解释
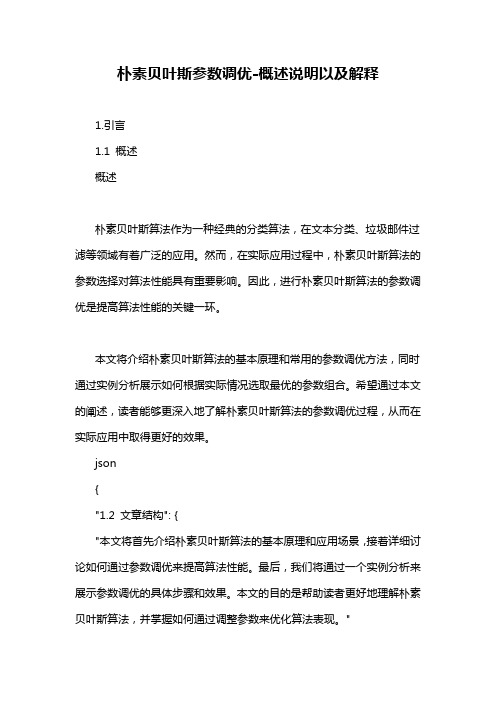
朴素贝叶斯参数调优-概述说明以及解释1.引言1.1 概述概述朴素贝叶斯算法作为一种经典的分类算法,在文本分类、垃圾邮件过滤等领域有着广泛的应用。
然而,在实际应用过程中,朴素贝叶斯算法的参数选择对算法性能具有重要影响。
因此,进行朴素贝叶斯算法的参数调优是提高算法性能的关键一环。
本文将介绍朴素贝叶斯算法的基本原理和常用的参数调优方法,同时通过实例分析展示如何根据实际情况选取最优的参数组合。
希望通过本文的阐述,读者能够更深入地了解朴素贝叶斯算法的参数调优过程,从而在实际应用中取得更好的效果。
json{"1.2 文章结构": {"本文将首先介绍朴素贝叶斯算法的基本原理和应用场景,接着详细讨论如何通过参数调优来提高算法性能。
最后,我们将通过一个实例分析来展示参数调优的具体步骤和效果。
本文的目的是帮助读者更好地理解朴素贝叶斯算法,并掌握如何通过调整参数来优化算法表现。
"}1.3 目的在本文中,我们的主要目的是探讨朴素贝叶斯算法中参数调优的方法和技巧。
通过深入研究朴素贝叶斯算法的原理和常见问题,我们希望能够为读者提供一些实用的调优指南,帮助他们更好地应用朴素贝叶斯算法解决实际问题。
通过对朴素贝叶斯算法中参数的调整和优化,我们可以提高模型的准确性和泛化能力,从而更好地满足现实世界中各种复杂的数据分析需求。
同时,本文也将通过实例分析的方式,演示如何在实际的数据集上进行参数调优,帮助读者更直观地理解调优过程和效果。
通过本文的研究和分析,我们希望读者能够更深入地了解朴素贝叶斯算法的优化方法,为他们在数据分析和机器学习领域的工作提供一定的帮助和启发。
同时,我们也希望促进学术界对朴素贝叶斯算法参数调优方面的研究,推动该领域的发展和进步。
2.正文2.1 朴素贝叶斯算法简介朴素贝叶斯算法是一种基于贝叶斯定理和特征条件独立假设的分类算法。
它的基本思想是通过计算给定特征值的类别的概率来进行分类。
(完整版)贝叶斯算法原理分析
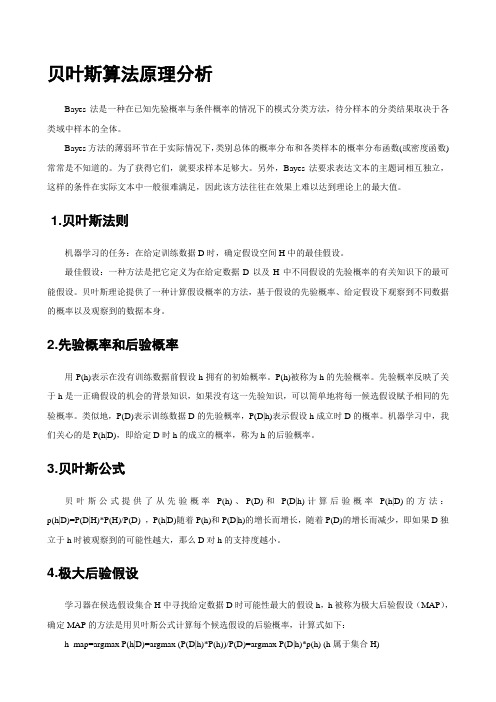
贝叶斯算法原理分析Bayes法是一种在已知先验概率与条件概率的情况下的模式分类方法,待分样本的分类结果取决于各类域中样本的全体。
Bayes方法的薄弱环节在于实际情况下,类别总体的概率分布和各类样本的概率分布函数(或密度函数)常常是不知道的。
为了获得它们,就要求样本足够大。
另外,Bayes法要求表达文本的主题词相互独立,这样的条件在实际文本中一般很难满足,因此该方法往往在效果上难以达到理论上的最大值。
1.贝叶斯法则机器学习的任务:在给定训练数据D时,确定假设空间H中的最佳假设。
最佳假设:一种方法是把它定义为在给定数据D以及H中不同假设的先验概率的有关知识下的最可能假设。
贝叶斯理论提供了一种计算假设概率的方法,基于假设的先验概率、给定假设下观察到不同数据的概率以及观察到的数据本身。
2.先验概率和后验概率用P(h)表示在没有训练数据前假设h拥有的初始概率。
P(h)被称为h的先验概率。
先验概率反映了关于h是一正确假设的机会的背景知识,如果没有这一先验知识,可以简单地将每一候选假设赋予相同的先验概率。
类似地,P(D)表示训练数据D的先验概率,P(D|h)表示假设h成立时D的概率。
机器学习中,我们关心的是P(h|D),即给定D时h的成立的概率,称为h的后验概率。
3.贝叶斯公式贝叶斯公式提供了从先验概率P(h)、P(D)和P(D|h)计算后验概率P(h|D)的方法:p(h|D)=P(D|H)*P(H)/P(D) ,P(h|D)随着P(h)和P(D|h)的增长而增长,随着P(D)的增长而减少,即如果D独立于h时被观察到的可能性越大,那么D对h的支持度越小。
4.极大后验假设学习器在候选假设集合H中寻找给定数据D时可能性最大的假设h,h被称为极大后验假设(MAP),确定MAP的方法是用贝叶斯公式计算每个候选假设的后验概率,计算式如下:h_map=argmax P(h|D)=argmax (P(D|h)*P(h))/P(D)=argmax P(D|h)*p(h) (h属于集合H)最后一步,去掉了P(D),因为它是不依赖于h的常量。
数据科学中的贝叶斯多目标优化算法
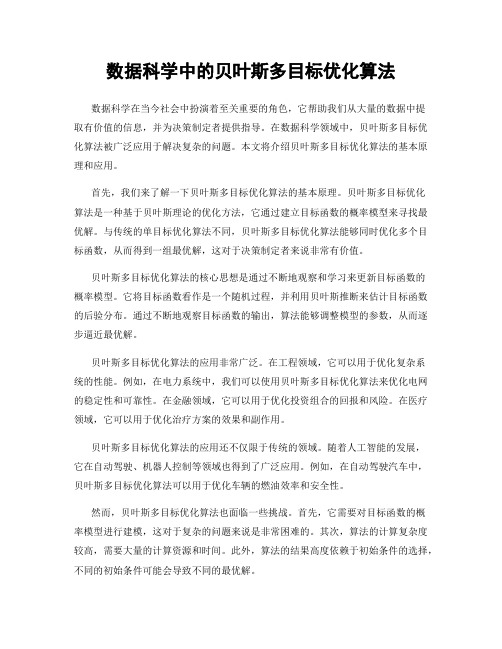
数据科学中的贝叶斯多目标优化算法数据科学在当今社会中扮演着至关重要的角色,它帮助我们从大量的数据中提取有价值的信息,并为决策制定者提供指导。
在数据科学领域中,贝叶斯多目标优化算法被广泛应用于解决复杂的问题。
本文将介绍贝叶斯多目标优化算法的基本原理和应用。
首先,我们来了解一下贝叶斯多目标优化算法的基本原理。
贝叶斯多目标优化算法是一种基于贝叶斯理论的优化方法,它通过建立目标函数的概率模型来寻找最优解。
与传统的单目标优化算法不同,贝叶斯多目标优化算法能够同时优化多个目标函数,从而得到一组最优解,这对于决策制定者来说非常有价值。
贝叶斯多目标优化算法的核心思想是通过不断地观察和学习来更新目标函数的概率模型。
它将目标函数看作是一个随机过程,并利用贝叶斯推断来估计目标函数的后验分布。
通过不断地观察目标函数的输出,算法能够调整模型的参数,从而逐步逼近最优解。
贝叶斯多目标优化算法的应用非常广泛。
在工程领域,它可以用于优化复杂系统的性能。
例如,在电力系统中,我们可以使用贝叶斯多目标优化算法来优化电网的稳定性和可靠性。
在金融领域,它可以用于优化投资组合的回报和风险。
在医疗领域,它可以用于优化治疗方案的效果和副作用。
贝叶斯多目标优化算法的应用还不仅限于传统的领域。
随着人工智能的发展,它在自动驾驶、机器人控制等领域也得到了广泛应用。
例如,在自动驾驶汽车中,贝叶斯多目标优化算法可以用于优化车辆的燃油效率和安全性。
然而,贝叶斯多目标优化算法也面临一些挑战。
首先,它需要对目标函数的概率模型进行建模,这对于复杂的问题来说是非常困难的。
其次,算法的计算复杂度较高,需要大量的计算资源和时间。
此外,算法的结果高度依赖于初始条件的选择,不同的初始条件可能会导致不同的最优解。
为了克服这些挑战,研究人员正在不断改进贝叶斯多目标优化算法。
一种常见的改进方法是使用近似推断技术来简化模型的计算。
例如,人们可以使用变分推断来近似目标函数的后验分布,从而减少计算复杂度。
贝叶斯优化算法
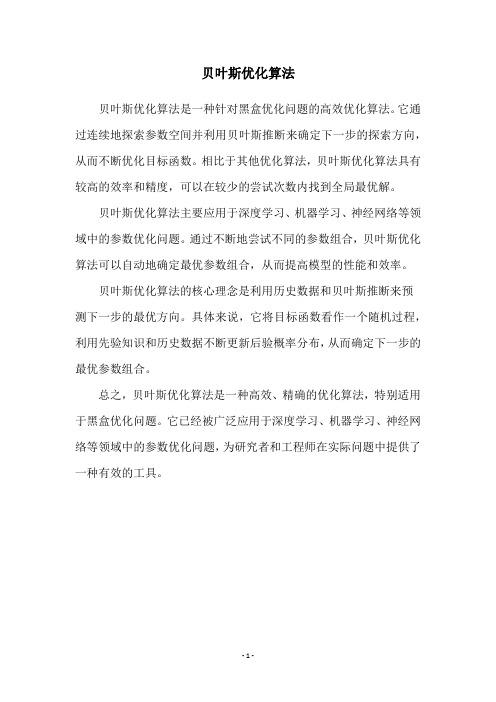
贝叶斯优化算法
贝叶斯优化算法是一种针对黑盒优化问题的高效优化算法。
它通过连续地探索参数空间并利用贝叶斯推断来确定下一步的探索方向,从而不断优化目标函数。
相比于其他优化算法,贝叶斯优化算法具有较高的效率和精度,可以在较少的尝试次数内找到全局最优解。
贝叶斯优化算法主要应用于深度学习、机器学习、神经网络等领域中的参数优化问题。
通过不断地尝试不同的参数组合,贝叶斯优化算法可以自动地确定最优参数组合,从而提高模型的性能和效率。
贝叶斯优化算法的核心理念是利用历史数据和贝叶斯推断来预
测下一步的最优方向。
具体来说,它将目标函数看作一个随机过程,利用先验知识和历史数据不断更新后验概率分布,从而确定下一步的最优参数组合。
总之,贝叶斯优化算法是一种高效、精确的优化算法,特别适用于黑盒优化问题。
它已经被广泛应用于深度学习、机器学习、神经网络等领域中的参数优化问题,为研究者和工程师在实际问题中提供了一种有效的工具。
- 1 -。
十大经典算法朴素贝叶斯讲解PPT
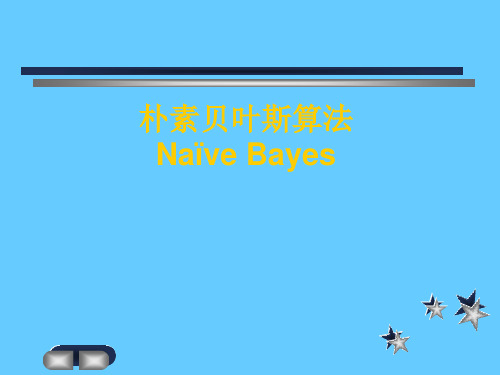
在人工智能领域,贝叶斯方法是一种非常具有 代表性的不确定性知识表示和推理方法。
贝叶斯定理:
P(A)是A的先验概率或边缘概率。之所以称为“先验”是因为它不考 虑任何B方面的因素。 P(A|B)是已知B发生后A的条件概率,也由于得自B的取值而被称 作A的后验概率。 P(B|A)是已知A发生后B的条件概率,也由于得自A的取值而被称 作B的后验概率。 P(B)是B的先验概率或边缘概率,也作标准化常量(normalized constant).
购买电脑实例:
购买电脑实例:
P(X | buys_computer = “no”) P(buys_computer = “no”) = 0.019×0.357 = 0.007
因此,对于样本X,朴素贝叶斯分类预测 buys_computer =”yes” 特别要注意的是:朴素贝叶斯的核心在于它假设向量 的所有分量之间是独立的。
扩展:
该算法就是将特征相关的属性分成一组,然后假设不 同组中的属性是相互独立的,同一组中的属性是相互 关联的。 (3)还有一种具有树结构的TAN(tree augmented naï ve Bayes)分类器,它放松了朴素贝叶斯中的独 立性假设条件,允许每个属性结点最多可以依赖一个 非类结点。TAN具有较好的综合性能。算是一种受限 制的贝叶斯网络算法。
Thank you!
贝叶斯算法处理流程:
第二阶段——分类器训练阶段: 主要工作是计算每个类别在训练样本中出现 频率以及每个特征属性划分对每个类别的条件 概率估计。输入是特征属性和训练样本,输出 是分类器。 第三阶段——应用阶段:
Hale Waihona Puke 这个阶段的任务是使用分类器对待分类项进行分类 ,其输入是分类器和待分类项,输出是待分类项与类 别的映射关系。
贝叶斯超参数优化-图文
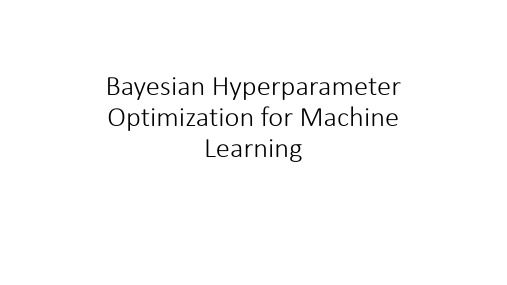
Learning
什么是超参数优化
• 机器学习中超参数优化的目的是找到给定机器学习算法的超参数,以返回在 验证集上测量的最佳性能。
• 比如:随机森林中树的个数
• 简单地说,我们希望找到在验证集指标上获得最佳分数的模型超参数。
• 在随机搜索和网格搜索的情况下,我们搜索的超参数域是一个网 格。上面是一个随机森林的例子
Objective Function
• 目标函数就是问题的评估。 • 计算起来较为昂贵
代理模型
代理函数有几种不同的形式,包括
高斯过程和随机森林回归,树
结构Parzen估计器。
这些方法在构造代理函数的方式上 有所不同,稍后我们将对此进行解 释。首先我们需要讨论选择函数。
其它超参数优化方法
• 《Population Based Training of Neural Networks》
• 思想朴素,但是应用广泛。
• Multi-Agent
• 比如第一视角多人游戏(Quake III Arena Capture the Flag)的超人表现,NeurIPS2018首届多智能 体竞赛(The NeurIPS 2018 Pommerman Competition)的冠军算法,DeepMind团队ICLR 2019 conference paper的2V2足球,甚至星际争霸II里的AlphaStar,都运用了类似方法。
超参数 • 由算法用于更新代理模型的(分数、超参数)对组成的历史记录
Domain
• hyperparameter_grid = { • 'n_estimators': [100, 200, 300, 400, 500, 600], • 'max_depth': [2, 5, 10, 15, 20, 25, 30, 35, 40], • 'min_samples_leaf': [1, 2, 3, 4中的序列优化过程只有一个模型在不断优化,消耗大量时间。(b)中的并行搜索可以节省 时间,但是相互之间没有任何交互,不利于信息利用。(c)中的PBT算法结合了二者的优点 。
贝叶斯分类器经典讲解图文
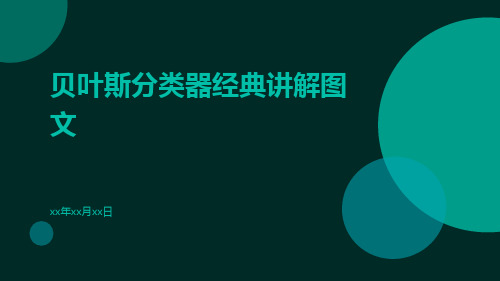
xx年xx月xx日
贝叶斯分类器经典讲解图文
CATALOGUE
目录
贝叶斯分类器概述贝叶斯分类器原理与技术贝叶斯分类器优化方法贝叶斯分类器实践技巧贝叶斯分类器与其他机器学习算法的比较贝叶斯分类器经典案例分析
贝叶斯分类器概述
01
定义与特点
适用性强:适用于文本、图像、声音等多种类型数据。
简单高效:算法逻辑简单,训练和分类过程高效。
高斯贝叶斯分类器
基于多项式分布假设,对特征进行建模并完成分类。
原理
特征符合多项式分布或存在交叉项,数据存在噪声。
适用场景
对特征交叉项有较好的处理能力,对噪声有一定的鲁棒性。
优势
多项式贝叶斯分类器
将贝叶斯分类器与决策树算法相结合,通过树结构对特征进行选择和组合。
原理
适用场景
优势
特征之间存在依赖关系,需要特征选择和组合。
图像分类概述:图像分类是将图像按照不同的类别进行划分的一种计算机视觉技术。
图像分类流程:图像预处理、特征提取、模型训练、分类和评估。
贝叶斯分类器在图像分类中的应用:人脸识别、物体检测、场景分类等。
贝叶斯分类器原理:对于每一个像素,利用贝叶斯定理来计算其属于某一类别的概率,并以此作为该像素的标签。
利用贝叶斯分类器进行图像分类
超参数优化
通过交叉验证和网格搜索等方式寻找最优超参数组合
参数优化
先验概率优化
根据数据分布情况调整先验概率,提高分类器性能
噪声处理
通过引入噪声模型对数据进行预处理,提高分类器鲁棒性
通过集成多个贝叶斯分类器,提高分类准确率和泛化性能
多个分类器融合
将贝叶斯算法与其他机器学习算法进行融合,实现优势互补
(完整版)贝叶斯算法原理分析
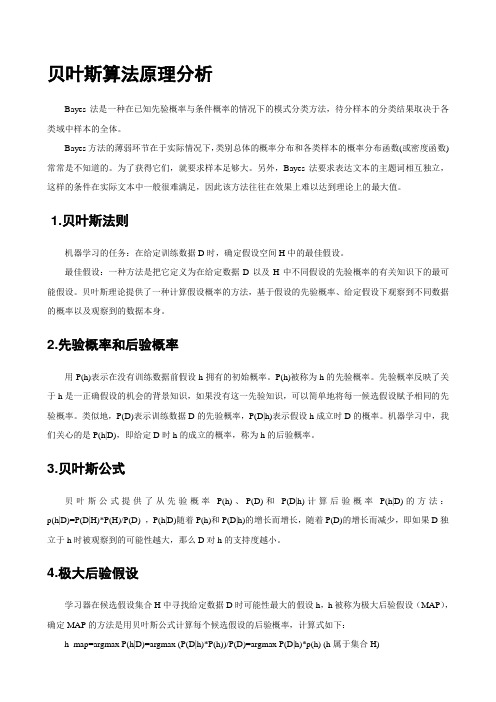
贝叶斯算法原理分析Bayes法是一种在已知先验概率与条件概率的情况下的模式分类方法,待分样本的分类结果取决于各类域中样本的全体。
Bayes方法的薄弱环节在于实际情况下,类别总体的概率分布和各类样本的概率分布函数(或密度函数)常常是不知道的。
为了获得它们,就要求样本足够大。
另外,Bayes法要求表达文本的主题词相互独立,这样的条件在实际文本中一般很难满足,因此该方法往往在效果上难以达到理论上的最大值。
1.贝叶斯法则机器学习的任务:在给定训练数据D时,确定假设空间H中的最佳假设。
最佳假设:一种方法是把它定义为在给定数据D以及H中不同假设的先验概率的有关知识下的最可能假设。
贝叶斯理论提供了一种计算假设概率的方法,基于假设的先验概率、给定假设下观察到不同数据的概率以及观察到的数据本身。
2.先验概率和后验概率用P(h)表示在没有训练数据前假设h拥有的初始概率。
P(h)被称为h的先验概率。
先验概率反映了关于h是一正确假设的机会的背景知识,如果没有这一先验知识,可以简单地将每一候选假设赋予相同的先验概率。
类似地,P(D)表示训练数据D的先验概率,P(D|h)表示假设h成立时D的概率。
机器学习中,我们关心的是P(h|D),即给定D时h的成立的概率,称为h的后验概率。
3.贝叶斯公式贝叶斯公式提供了从先验概率P(h)、P(D)和P(D|h)计算后验概率P(h|D)的方法:p(h|D)=P(D|H)*P(H)/P(D) ,P(h|D)随着P(h)和P(D|h)的增长而增长,随着P(D)的增长而减少,即如果D独立于h时被观察到的可能性越大,那么D对h的支持度越小。
4.极大后验假设学习器在候选假设集合H中寻找给定数据D时可能性最大的假设h,h被称为极大后验假设(MAP),确定MAP的方法是用贝叶斯公式计算每个候选假设的后验概率,计算式如下:h_map=argmax P(h|D)=argmax (P(D|h)*P(h))/P(D)=argmax P(D|h)*p(h) (h属于集合H)最后一步,去掉了P(D),因为它是不依赖于h的常量。
- 1、下载文档前请自行甄别文档内容的完整性,平台不提供额外的编辑、内容补充、找答案等附加服务。
- 2、"仅部分预览"的文档,不可在线预览部分如存在完整性等问题,可反馈申请退款(可完整预览的文档不适用该条件!)。
- 3、如文档侵犯您的权益,请联系客服反馈,我们会尽快为您处理(人工客服工作时间:9:00-18:30)。
Bayesian Optimization CSC2541 - Topics in Machine LearningScalable and Flexible Models of UncertaintyUniversity of Toronto - Fall 2017Overview1.Bayesian Optimization of Machine Learning Algorithms2.Gaussian Process Optimization in the Bandit Setting3.Exploiting Structure for Bayesian OptimizationBayesian Optimization of Machine Learning Algorithms J. Snoek, A. Krause, H. Larochelle, and R.P. Adams (2012)Practical Bayesian Optimization of Machine Learning AlgorithmsJ. Snoek et al. (2015)Scalable Bayesian Optimization Using Deep Neural NetsPresentation by: Franco Lin, Tahmid Mehdi, Jason LiMotivationPerformance of Machine Learning algorithms are usually dependent on the choice of hyperparametersPicking the optimal hyperparameter values are hard-Ex. grid search, random search, etc.-Instead could we use a model to select which hyperparameters will be good next?Bayes Opt. of Machine Learning Algorithms-Bayesian Optimization uses all of the information from previous evaluations and performs some computation to determine the next point to try-If our model takes days to train, it would be beneficial to have a well structured way of selecting the next combination of hyperparameters to try-Bayesian Optimization is much better than a person finding a good combination of hyperparametersBayesian OptimizationIntuition:We want to find the peak of our true function (eg. accuracy as a function of hyperparameters)To find this peak, we will fit a Gaussian Process to our observed points and pick our next best point where we believe the maximum will be.This next point is determined by an acquisition function - that trades of exploration and exploitationBayesian Optimization TutorialBayesian Optimization Tutorialthat maximizes acquisition function Find the next best point xnBayesian Optimization TutorialEvaluate ƒ at the new observation xand update posteriornUpdate acquisition function from new posterior and find the next best pointAcquisition Function Intuition-We will use the acquisition functionProbability of Improvement (PI) asan example.-We want to find the point with thelargest area above our best value-This corresponds to the maximumof our acquisition functionAcquisition Functions-Guides the optimization by determining which point to observe next and is easier to optimize to find the next sample pointProbability of Improvement (PI)Expected Improvement (EI)GP-Upper/Lower Confidence Bound (GP-UCB/LCB)The Prior-Power of Gaussian Process depends on covariance function-For optimization, we don’t want kernels that produce unrealistically smooth sample functions-Automatic Relevance Determination (ARD) Matern 5/2 kernel is a good choiceKernel HyperparametersMarginalize over hyperparameters and compute integrated acquisition functionApproximate integral with Monte Carlo methodsConsiderations for Bayes Opt-Evaluating f may be time-consuming-Modern optimization methods should take advantage of multi-core/parallel programmingExpected Improvement per Second-Evaluating f will take longer in some regions of the parameter space-We want to pick points that are likely to be good and evaluated quickly -Let c(x) be the duration time to evaluate f(x)-Use GP to model ln[c(x)]-we can compute predicted expected inverse duration which allows us to obtain the EI per Second as a function of xParallelizing Bayes Opt-Can we determine which x to evaluate next, while other points are being evaluated?-Idea: Utilize tractable properties of GP to get Monte Carlo estimates of acquisition function under different results from pending function evaluationsConsider the case where N evaluations have completed, with data {xn ,yn}n=1N, and Jevaluations are pending {xj }j=1JParallelization Example-We’ve evaluated 3 observations and 2are pending {x 1,x 2}-Fit a model for each possible realizationof {f(x 1), f(x 2)}-Calculate acquisition for each model -Integrate all acquisitions over xResults●Branin-Hoo●Logistic Regression MNIST ●Online LDA●M3E●CNN CIFAR-10Logistic Regression - MNISTCIFAR-10●3-layer conv-net●Optimized over○Number of epochs○Learning rate○L2-norm constants ●Achieved state of the art○9.5% test errorGP Bayesian Optimization - Pros and Cons●Advantages○Computes the mean and variance●Disadvantages○Function evaluation is cubic on the number of inputsScalable Bayesian Optimization Using Deep Neural Networks●Replace a Gaussian Process with a Bayesian Neural Network●Use a deterministic neural network with Bayesian linear regression onthe last hidden layer●More accurately, use Bayesian linear regression with basis functions○DNN: R k -> R d○Bayesian linear regression: R d -> R○k is the dimensionality of the input, and d is the number of hidden units in the last layerBayesian Linear Regression●Still requires an inversion●Linear in the number of observations●Cubic in the basis function dimension or number of hidden units, DResultsGaussian Process Optimization in the Bandit SettingN. Srinivas, A. Krause, S. Kakade, and M. Seeger (2010)Gaussian process optimization in the bandit setting: No regret and experimental design Presentation by: Shadi Zabad, Wei Zhen Teoh, Shuja KhalidThe Bandits are Back!-We just learned about some exciting newtechniques for optimizing black box functions.Can we apply them to the classic multi-armedbandit problem?-In this case, we’d like to optimize the unknownreward function.Credit: D. Tolpin at ECAI 2012Cost-bounded Optimization-In the bandit setting, the optimization procedure is cost-sensitive: There’s a cost incurred each time we evaluate the function.-The cost is proportional to how far the point is from the point of maximum reward.-Therefore, we have to optimize the reward function while minimizing the cost incurred along the way.An Infinite Number of Arms-The multi-armed bandit algorithms and analyses we’ve seen so farassumed a discrete decision space (e.g. a decision space wherewe have K slot machines).-However, in Gaussian Process optimization, we’d like to considercontinuous decision spaces.-And in this domain, some of the theoretical analyses that wederived for discrete decision spaces can’t be extended in astraightforward manner.Credit: @Astrid, CrossValidatedMulti-armed Bandit Problem: Recap -The basic setting : We have a decision space that’sassociated with anunknown reward function .-Discrete examples: Slot machines at a casino, drug trials.-Continuous examples : Digging for oil or minerals, robotmotion planning.-In this setting, a “policy ” is a procedure for exploringthe decision space. An optimal policy is defined as aprocedure which minimizes a cost measure. The mostcommon cost measure is the “regret ”.Credit: Intelligent Motion Lab (Duke U)Credit: Gatis GribustsA Measure of Regret-In general terms, regret is defined as “the loss in reward due to not knowing” the maximum pointsbeforehand.-We can formalize this notion with 2 concepts:-Instantaneous regret (r t): the loss in reward at step t: r t = f(D max) - f(D t)-Cumulative regret (R T): the total loss in reward after T steps:R T = ∑r tMinimizing Regret: A Tradeoff-As we have seen before, we can define policies thatbalance exploration and exploitation. Some of thepolicies we’ve looked at are:-Epsilon-greedy-Thompson sampling-Upper Confidence Bound (UCB)-Some of these policies perform better than othersin minimizing the average regret over time.Average Regret = R T / TCredit: Russo et al., 2017Asymptotic Regret-We can also look at the cumulative or average regret measure as the number of iterations goes toinfinity.-An algorithm is said to be no-regret if its asymptoticcumulative regret rate is sublinear with respect to T (i.e. the number of iterations)sqrt(T) and log(T) are examples of sublinear regret rates w.r.t. T.Why is Asymptotic Regret Important?-In real world applications, we know neitherinstantaneous nor average regret. So, whyare we concerned with characterizing theirasymptotic behavior?-Answer: Bounds on the average regret tellus about the convergence rate (i.e. how fastwe approach the maximum point) of theoptimization algorithm.Credit: N. de Freitas et al., 2012Regret Bounds in Discrete Decision Spaces-In the previous lecture, we discussed asymptotic regret in discrete decision spaces where we have K slot machines or drug trials.-We also looked at theorems by Auer et al. that derive an upper bound on the regret rate for the UCB algorithm in discrete settings.Dani et al. 2008“In the traditional K-arm bandit literature, the regret is often characterized for a particular problem in terms of T, K, and problem dependent constants . In the K-arm bandit results of Auer et al. [2002], this problem dependent constant is the ‘gap’ between the loss of the best arm and the second best arm.”Regret Bounds in Continuous Decision Spaces-Dani et al.** extended Auer et al.’s theoretical results to continuous decision spaces and proved upper and lower regretbounds for the UCB algorithm.-However, their method places restrictions on the types of reward functions considered, primarily: The functions are defined overfinite-dimensional linear spaces.** Dani, V., Hayes, T. P., and Kakade, S. M. Stochastic linear optimization under bandit feedback. In COLT, 2008.Infinite-dimensional Functions-Srinivas et al. propose to relax some of the restrictionsof Dani et al.’s analysis and extend the results torandom, infinite-dimensional functions.-Earlier in the semester, we learned about a method forgenerating such classes of functions: GaussianProcesses.-Idea: Assuming the target reward function is sampledfrom a Gaussian Process, try to optimize it usingGP-UCB.-How to derive regret bounds for those classes ofCredit: Duvenaud, The Kernel Cookbook functions?Using Information Gain To Derive Regret BoundsInformation GainRecall Mackay (1992) paper: Information gain can be quantified as change in entropy In this context: Information gain =entropy in prior - entropy in posterior after y A sampled = H(f) - H( f | y A )= I(f; y A ), mutual information between f and observed y A=I(y A ; f) = H(y A ) - H( y A |f) = (log| 2I + K A |)/2 - (log| 2I |)/2 = (log|I + -2 K A |)/2Note:information gain depends on kernel of GP prior and input spaceCredit: Srinivas et al. 2010If our goal is just exploration ...Greedy Experimental Design Algorithm:Sequentially, findHowever the worse point weselect, the more penalty we get Credit: Srinivas et al. 2010GP - UCB to the rescueExplore ExploitCredit: Srinivas et al. 2010Maximum information GainDefinition:Maximum information gain after T data points sampled,This term will be used to quantify the regret bound for the algorithmRegret Bounds - Finite Domain Theorem 1:Assumptions:-Finite D- f sample of a GP with mean 0,-k(x, x’) of GP s.t. k(x,x) (variance) not greater than 1Then, by running GP-UCB for f withWe obtain: TAssuming some strictly sublinear T ...(we will verify later that this is achievable by choice of kernels),We can find some sublinear function f(T) bounding above P(R T curve lies below) is at least 1-f(T)Regret Bounds II - General Compact+Convex SpaceTheorem 2:Assumptions:- D compact and convex in [0,r]d,- f sample of a GP with mean 0,-k(x, x’) of GP s.t. k(x,x) (variance) not greater than 1-k(x,x’) s.t. f fulfills smoothness condition -- discussed nextThen, by running GP-UCB for f withWe obtain:Regret Bounds II ContinuedTheorem 2 requires f to fulfill:This holds for stationary kernels k(x,x’) = k(x-x’) which are 4-times differentiable: Squared Exponential Kernel Matern Kernels with v>2Credit: Srinivas et al. 2010Bounding Information Gain-- F is submodular function⇒T⇒ T SubmodularityThis holds if A constructed byGreedy Experiment Design Rule Credit: Krause, https://las.inf.ethz.ch/sfo/Bounding Information Gain ContinuedWe can bound the term by considering the worst allocation of the T samples under some relaxed greedy procedure (see appendix section C).In finite space D, this eventually gives us a bound in terms of the eigenvalues of the covariance matrix for all |D| points:The faster the spectrum decays, the slower the growth of the boundBounding Information Gain ContinuedCredit: Srinivas et al. 2010Bounding Information Gain ContinuedTheorem 5: Assume general compact and convex set D in R d , kernel k(x,x’)≤1:1.d- dimensional bayesian linear regression:2.Squared exponential kernel:3.Matern kernel (v>1) :Now recall the bound obtained for GP-UCB in theorem 2: Combining the two theorems we obtain the following (1-δ) upper confidence bound for the total regret, R T (up to polylog factors):With T = + Credit: Srinivas et al. 2010Results and DiscussionExperimental Setup●Synthetic and real sensor network data (traffic and temperature)used to illustrate the differences●Gaussian Processes - Upper Confidence Bound (GP-UCB) iscompared with various heuristics:○Expected Improvement (EI)○Most Probable Improvement (MPI)○Naive Methods (only mean or only variance)。