英文文献加翻译(基于神经网络和遗传算法的模糊系统的自动设计)
人工智能英文文献原文及译文
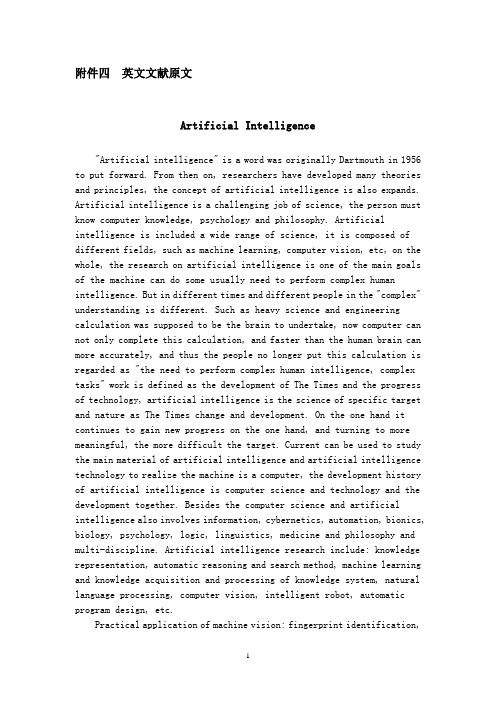
附件四英文文献原文Artificial Intelligence"Artificial intelligence" is a word was originally Dartmouth in 1956 to put forward. From then on, researchers have developed many theories and principles, the concept of artificial intelligence is also expands. Artificial intelligence is a challenging job of science, the person must know computer knowledge, psychology and philosophy. Artificial intelligence is included a wide range of science, it is composed of different fields, such as machine learning, computer vision, etc, on the whole, the research on artificial intelligence is one of the main goals of the machine can do some usually need to perform complex human intelligence. But in different times and different people in the "complex" understanding is different. Such as heavy science and engineering calculation was supposed to be the brain to undertake, now computer can not only complete this calculation, and faster than the human brain can more accurately, and thus the people no longer put this calculation is regarded as "the need to perform complex human intelligence, complex tasks" work is defined as the development of The Times and the progress of technology, artificial intelligence is the science of specific target and nature as The Times change and development. On the one hand it continues to gain new progress on the one hand, and turning to more meaningful, the more difficult the target. Current can be used to study the main material of artificial intelligence and artificial intelligence technology to realize the machine is a computer, the development history of artificial intelligence is computer science and technology and the development together. Besides the computer science and artificial intelligence also involves information, cybernetics, automation, bionics, biology, psychology, logic, linguistics, medicine and philosophy and multi-discipline. Artificial intelligence research include: knowledge representation, automatic reasoning and search method, machine learning and knowledge acquisition and processing of knowledge system, natural language processing, computer vision, intelligent robot, automatic program design, etc.Practical application of machine vision: fingerprint identification,face recognition, retina identification, iris identification, palm, expert system, intelligent identification, search, theorem proving game, automatic programming, and aerospace applications.Artificial intelligence is a subject categories, belong to the door edge discipline of natural science and social science.Involving scientific philosophy and cognitive science, mathematics, neurophysiological, psychology, computer science, information theory, cybernetics, not qualitative theory, bionics.The research category of natural language processing, knowledge representation, intelligent search, reasoning, planning, machine learning, knowledge acquisition, combined scheduling problem, perception, pattern recognition, logic design program, soft calculation, inaccurate and uncertainty, the management of artificial life, neural network, and complex system, human thinking mode of genetic algorithm.Applications of intelligent control, robotics, language and image understanding, genetic programming robot factory.Safety problemsArtificial intelligence is currently in the study, but some scholars think that letting computers have IQ is very dangerous, it may be against humanity. The hidden danger in many movie happened.The definition of artificial intelligenceDefinition of artificial intelligence can be divided into two parts, namely "artificial" or "intelligent". "Artificial" better understanding, also is controversial. Sometimes we will consider what people can make, or people have high degree of intelligence to create artificial intelligence, etc. But generally speaking, "artificial system" is usually significance of artificial system.What is the "smart", with many problems. This involves other such as consciousness, ego, thinking (including the unconscious thoughts etc. People only know of intelligence is one intelligent, this is the universal view of our own. But we are very limited understanding of the intelligence of the intelligent people constitute elements are necessary to find, so it is difficult to define what is "artificial" manufacturing "intelligent". So the artificial intelligence research often involved in the study of intelligent itself. Other about animal or other artificial intelligence system is widely considered to be related to the study of artificial intelligence.Artificial intelligence is currently in the computer field, the moreextensive attention. And in the robot, economic and political decisions, control system, simulation system application. In other areas, it also played an indispensable role.The famous American Stanford university professor nelson artificial intelligence research center of artificial intelligence under such a definition: "artificial intelligence about the knowledge of the subject is and how to represent knowledge -- how to gain knowledge and use of scientific knowledge. But another American MIT professor Winston thought: "artificial intelligence is how to make the computer to do what only can do intelligent work." These comments reflect the artificial intelligence discipline basic ideas and basic content. Namely artificial intelligence is the study of human intelligence activities, has certain law, research of artificial intelligence system, how to make the computer to complete before the intelligence needs to do work, also is to study how the application of computer hardware and software to simulate human some intelligent behavior of the basic theory, methods and techniques.Artificial intelligence is a branch of computer science, since the 1970s, known as one of the three technologies (space technology, energy technology, artificial intelligence). Also considered the 21st century (genetic engineering, nano science, artificial intelligence) is one of the three technologies. It is nearly three years it has been developed rapidly, and in many fields are widely applied, and have made great achievements, artificial intelligence has gradually become an independent branch, both in theory and practice are already becomes a system. Its research results are gradually integrated into people's lives, and create more happiness for mankind.Artificial intelligence is that the computer simulation research of some thinking process and intelligent behavior (such as study, reasoning, thinking, planning, etc.), including computer to realize intelligent principle, make similar to that of human intelligence, computer can achieve higher level of computer application. Artificial intelligence will involve the computer science, philosophy and linguistics, psychology, etc. That was almost natural science and social science disciplines, the scope of all already far beyond the scope of computer science and artificial intelligence and thinking science is the relationship between theory and practice, artificial intelligence is in the mode of thinking science technology application level, is one of its application. From the view of thinking, artificial intelligence is not limited to logicalthinking, want to consider the thinking in image, the inspiration of thought of artificial intelligence can promote the development of the breakthrough, mathematics are often thought of as a variety of basic science, mathematics and language, thought into fields, artificial intelligence subject also must not use mathematical tool, mathematical logic, the fuzzy mathematics in standard etc, mathematics into the scope of artificial intelligence discipline, they will promote each other and develop faster.A brief history of artificial intelligenceArtificial intelligence can be traced back to ancient Egypt's legend, but with 1941, since the development of computer technology has finally can create machine intelligence, "artificial intelligence" is a word in 1956 was first proposed, Dartmouth learned since then, researchers have developed many theories and principles, the concept of artificial intelligence, it expands and not in the long history of the development of artificial intelligence, the slower than expected, but has been in advance, from 40 years ago, now appears to have many AI programs, and they also affected the development of other technologies. The emergence of AI programs, creating immeasurable wealth for the community, promoting the development of human civilization.The computer era1941 an invention that information storage and handling all aspects of the revolution happened. This also appeared in the U.S. and Germany's invention is the first electronic computer. Take a few big pack of air conditioning room, the programmer's nightmare: just run a program for thousands of lines to set the 1949. After improvement can be stored procedure computer programs that make it easier to input, and the development of the theory of computer science, and ultimately computer ai. This in electronic computer processing methods of data, for the invention of artificial intelligence could provide a kind of media.The beginning of AIAlthough the computer AI provides necessary for technical basis, but until the early 1950s, people noticed between machine and human intelligence. Norbert Wiener is the study of the theory of American feedback. Most familiar feedback control example is the thermostat. It will be collected room temperature and hope, and reaction temperature compared to open or close small heater, thus controlling environmental temperature. The importance of the study lies in the feedback loop Wiener:all theoretically the intelligence activities are a result of feedback mechanism and feedback mechanism is. Can use machine. The findings of the simulation of early development of AI.1955, Simon and end Newell called "a logical experts" program. This program is considered by many to be the first AI programs. It will each problem is expressed as a tree, then choose the model may be correct conclusion that a problem to solve. "logic" to the public and the AI expert research field effect makes it AI developing an important milestone in 1956, is considered to be the father of artificial intelligence of John McCarthy organized a society, will be a lot of interest machine intelligence experts and scholars together for a month. He asked them to Vermont Dartmouth in "artificial intelligence research in summer." since then, this area was named "artificial intelligence" although Dartmouth learn not very successful, but it was the founder of the centralized and AI AI research for later laid a foundation.After the meeting of Dartmouth, AI research started seven years. Although the rapid development of field haven't define some of the ideas, meeting has been reconsidered and Carnegie Mellon university. And MIT began to build AI research center is confronted with new challenges. Research needs to establish the: more effective to solve the problem of the system, such as "logic" in reducing search; expert There is the establishment of the system can be self learning.In 1957, "a new program general problem-solving machine" first version was tested. This program is by the same logic "experts" group development. The GPS expanded Wiener feedback principle, can solve many common problem. Two years later, IBM has established a grind investigate group Herbert AI. Gelerneter spent three years to make a geometric theorem of solutions of the program. This achievement was a sensation.When more and more programs, McCarthy busy emerge in the history of an AI. 1958 McCarthy announced his new fruit: LISP until today still LISP language. In. "" mean" LISP list processing ", it quickly adopted for most AI developers.In 1963 MIT from the United States government got a pen is 22millions dollars funding for research funding. The machine auxiliary recognition from the defense advanced research program, have guaranteed in the technological progress on this plan ahead of the Soviet union. Attracted worldwide computer scientists, accelerate the pace of development of AI research.Large programAfter years of program. It appeared a famous called "SHRDLU." SHRDLU "is" the tiny part of the world "project, including the world (for example, only limited quantity of geometrical form of research and programming). In the MIT leadership of Minsky Marvin by researchers found, facing the object, the small computer programs can solve the problem space and logic. Other as in the late 1960's STUDENT", "can solve algebraic problems," SIR "can understand the simple English sentence. These procedures for handling the language understanding and logic.In the 1970s another expert system. An expert system is a intelligent computer program system, and its internal contains a lot of certain areas of experience and knowledge with expert level, can use the human experts' knowledge and methods to solve the problems to deal with this problem domain. That is, the expert system is a specialized knowledge and experience of the program system. Progress is the expert system could predict under certain conditions, the probability of a solution for the computer already has. Great capacity, expert systems possible from the data of expert system. It is widely used in the market. Ten years, expert system used in stock, advance help doctors diagnose diseases, and determine the position of mineral instructions miners. All of this because of expert system of law and information storage capacity and become possible.In the 1970s, a new method was used for many developing, famous as AI Minsky tectonic theory put forward David Marr. Another new theory of machine vision square, for example, how a pair of image by shadow, shape, color, texture and basic information border. Through the analysis of these images distinguish letter, can infer what might be the image in the same period. PROLOGE result is another language, in 1972. In the 1980s, the more rapid progress during the AI, and more to go into business. 1986, the AI related software and hardware sales $4.25 billion dollars. Expert system for its utility, especially by demand. Like digital electric company with such company XCON expert system for the VAX mainframe programming. Dupont, general motors and Boeing has lots of dependence of expert system for computer expert. Some production expert system of manufacture software auxiliary, such as Teknowledge and Intellicorp established. In order to find and correct the mistakes, existing expert system and some other experts system was designed,such as teach users learn TVC expert system of the operating system.From the lab to daily lifePeople began to feel the computer technique and artificial intelligence. No influence of computer technology belong to a group of researchers in the lab. Personal computers and computer technology to numerous technical magazine now before a people. Like the United States artificial intelligence association foundation. Because of the need to develop, AI had a private company researchers into the boom. More than 150 a DEC (it employs more than 700 employees engaged in AI research) that have spent 10 billion dollars in internal AI team.Some other AI areas in the 1980s to enter the market. One is the machine vision Marr and achievements of Minsky. Now use the camera and production, quality control computer. Although still very humble, these systems have been able to distinguish the objects and through the different shape. Until 1985 America has more than 100 companies producing machine vision systems, sales were us $8 million.But the 1980s to AI and industrial all is not a good year for years. 1986-87 AI system requirements, the loss of industry nearly five hundred million dollars. Teknowledge like Intellicorp and two loss of more than $6 million, about one-third of the profits of the huge losses forced many research funding cuts the guide led. Another disappointing is the defense advanced research programme support of so-called "intelligent" this project truck purpose is to develop a can finish the task in many battlefield robot. Since the defects and successful hopeless, Pentagon stopped project funding.Despite these setbacks, AI is still in development of new technology slowly. In Japan were developed in the United States, such as the fuzzy logic, it can never determine the conditions of decision making, And neural network, regarded as the possible approaches to realizing artificial intelligence. Anyhow, the eighties was introduced into the market, the AI and shows the practical value. Sure, it will be the key to the 21st century. "artificial intelligence technology acceptance inspection in desert storm" action of military intelligence test equipment through war. Artificial intelligence technology is used to display the missile system and warning and other advanced weapons. AI technology has also entered family. Intelligent computer increase attracting public interest. The emergence of network game, enriching people's life.Some of the main Macintosh and IBM for application software such as voice and character recognition has can buy, Using fuzzy logic,AI technology to simplify the camera equipment. The artificial intelligence technology related to promote greater demand for new progress appear constantly. In a word ,Artificial intelligence has and will continue to inevitably changed our life.附件三英文文献译文人工智能“人工智能”一词最初是在1956 年Dartmouth在学会上提出来的。
外文翻译---人工神经网络
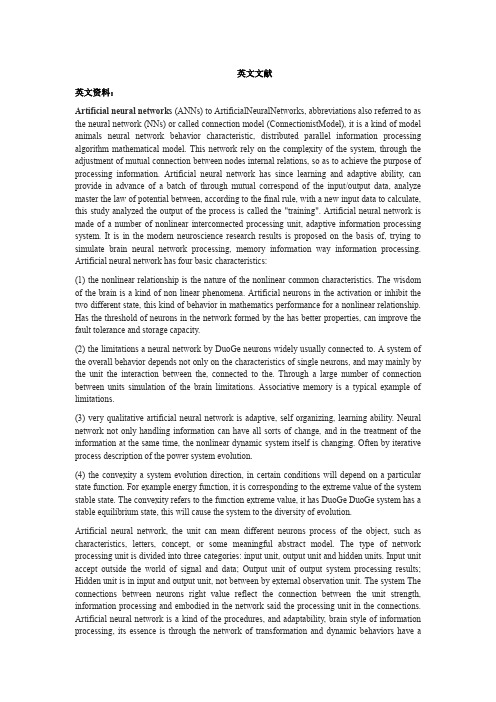
英文文献英文资料:Artificial neural networks (ANNs) to ArtificialNeuralNetworks, abbreviations also referred to as the neural network (NNs) or called connection model (ConnectionistModel), it is a kind of model animals neural network behavior characteristic, distributed parallel information processing algorithm mathematical model. This network rely on the complexity of the system, through the adjustment of mutual connection between nodes internal relations, so as to achieve the purpose of processing information. Artificial neural network has since learning and adaptive ability, can provide in advance of a batch of through mutual correspond of the input/output data, analyze master the law of potential between, according to the final rule, with a new input data to calculate, this study analyzed the output of the process is called the "training". Artificial neural network is made of a number of nonlinear interconnected processing unit, adaptive information processing system. It is in the modern neuroscience research results is proposed on the basis of, trying to simulate brain neural network processing, memory information way information processing. Artificial neural network has four basic characteristics:(1) the nonlinear relationship is the nature of the nonlinear common characteristics. The wisdom of the brain is a kind of non-linear phenomena. Artificial neurons in the activation or inhibit the two different state, this kind of behavior in mathematics performance for a nonlinear relationship. Has the threshold of neurons in the network formed by the has better properties, can improve the fault tolerance and storage capacity.(2) the limitations a neural network by DuoGe neurons widely usually connected to. A system of the overall behavior depends not only on the characteristics of single neurons, and may mainly by the unit the interaction between the, connected to the. Through a large number of connection between units simulation of the brain limitations. Associative memory is a typical example of limitations.(3) very qualitative artificial neural network is adaptive, self-organizing, learning ability. Neural network not only handling information can have all sorts of change, and in the treatment of the information at the same time, the nonlinear dynamic system itself is changing. Often by iterative process description of the power system evolution.(4) the convexity a system evolution direction, in certain conditions will depend on a particular state function. For example energy function, it is corresponding to the extreme value of the system stable state. The convexity refers to the function extreme value, it has DuoGe DuoGe system has a stable equilibrium state, this will cause the system to the diversity of evolution.Artificial neural network, the unit can mean different neurons process of the object, such as characteristics, letters, concept, or some meaningful abstract model. The type of network processing unit is divided into three categories: input unit, output unit and hidden units. Input unit accept outside the world of signal and data; Output unit of output system processing results; Hidden unit is in input and output unit, not between by external observation unit. The system The connections between neurons right value reflect the connection between the unit strength, information processing and embodied in the network said the processing unit in the connections. Artificial neural network is a kind of the procedures, and adaptability, brain style of information processing, its essence is through the network of transformation and dynamic behaviors have akind of parallel distributed information processing function, and in different levels and imitate people cranial nerve system level of information processing function. It is involved in neuroscience, thinking science, artificial intelligence, computer science, etc DuoGe field cross discipline.Artificial neural network is used the parallel distributed system, with the traditional artificial intelligence and information processing technology completely different mechanism, overcome traditional based on logic of the symbols of the artificial intelligence in the processing of intuition and unstructured information of defects, with the adaptive, self-organization and real-time characteristic of the study.Development historyIn 1943, psychologists W.S.M cCulloch and mathematical logic W.P home its established the neural network and the math model, called MP model. They put forward by MP model of the neuron network structure and formal mathematical description method, and prove the individual neurons can perform the logic function, so as to create artificial neural network research era. In 1949, the psychologist put forward the idea of synaptic contact strength variable. In the s, the artificial neural network to further development, a more perfect neural network model was put forward, including perceptron and adaptive linear elements etc. M.M insky, analyzed carefully to Perceptron as a representative of the neural network system function and limitations in 1969 after the publication of the book "Perceptron, and points out that the sensor can't solve problems high order predicate. Their arguments greatly influenced the research into the neural network, and at that time serial computer and the achievement of the artificial intelligence, covering up development new computer and new ways of artificial intelligence and the necessity and urgency, make artificial neural network of research at a low. During this time, some of the artificial neural network of the researchers remains committed to this study, presented to meet resonance theory (ART nets), self-organizing mapping, cognitive machine network, but the neural network theory study mathematics. The research for neural network of research and development has laid a foundation. In 1982, the California institute of J.J.H physicists opfield Hopfield neural grid model proposed, and introduces "calculation energy" concept, gives the network stability judgment. In 1984, he again put forward the continuous time Hopfield neural network model for the neural computers, the study of the pioneering work, creating a neural network for associative memory and optimization calculation, the new way of a powerful impetus to the research into the neural network, in 1985, and scholars have proposed a wave ears, the study boltzmann model using statistical thermodynamics simulated annealing technology, guaranteed that the whole system tends to the stability of the points. In 1986 the cognitive microstructure study, puts forward the parallel distributed processing theory. Artificial neural network of research by each developed country, the congress of the United States to the attention of the resolution will be on jan. 5, 1990 started ten years as the decade of the brain, the international research organization called on its members will the decade of the brain into global behavior. In Japan's "real world computing (springboks claiming)" project, artificial intelligence research into an important component.Network modelArtificial neural network model of the main consideration network connection topological structure, the characteristics, the learning rule neurons. At present, nearly 40 kinds of neural network model, with back propagation network, sensor, self-organizing mapping, the Hopfieldnetwork.the computer, wave boltzmann machine, adapt to the ear resonance theory. According to the topology of the connection, the neural network model can be divided into:(1) prior to the network before each neuron accept input and output level to the next level, the network without feedback, can use a loop to no graph. This network realization from the input space to the output signal of the space transformation, it information processing power comes from simple nonlinear function of DuoCi compound. The network structure is simple, easy to realize. Against the network is a kind of typical prior to the network.(2) the feedback network between neurons in the network has feedback, can use a no to complete the graph. This neural network information processing is state of transformations, can use the dynamics system theory processing. The stability of the system with associative memory function has close relationship. The Hopfield network.the computer, wave ear boltzmann machine all belong to this type.Learning typeNeural network learning is an important content, it is through the adaptability of the realization of learning. According to the change of environment, adjust to weights, improve the behavior of the system. The proposed by the Hebb Hebb learning rules for neural network learning algorithm to lay the foundation. Hebb rules say that learning process finally happened between neurons in the synapse, the contact strength synapses parts with before and after the activity and synaptic neuron changes. Based on this, people put forward various learning rules and algorithm, in order to adapt to the needs of different network model. Effective learning algorithm, and makes the godThe network can through the weights between adjustment, the structure of the objective world, said the formation of inner characteristics of information processing method, information storage and processing reflected in the network connection. According to the learning environment is different, the study method of the neural network can be divided into learning supervision and unsupervised learning. In the supervision and study, will the training sample data added to the network input, and the corresponding expected output and network output, in comparison to get error signal control value connection strength adjustment, the DuoCi after training to a certain convergence weights. While the sample conditions change, the study can modify weights to adapt to the new environment. Use of neural network learning supervision model is the network, the sensor etc. The learning supervision, in a given sample, in the environment of the network directly, learning and working stages become one. At this time, the change of the rules of learning to obey the weights between evolution equation of. Unsupervised learning the most simple example is Hebb learning rules. Competition rules is a learning more complex than learning supervision example, it is according to established clustering on weights adjustment. Self-organizing mapping, adapt to the resonance theory is the network and competitive learning about the typical model.Analysis methodStudy of the neural network nonlinear dynamic properties, mainly USES the dynamics system theory and nonlinear programming theory and statistical theory to analysis of the evolution process of the neural network and the nature of the attractor, explore the synergy of neural network behavior and collective computing functions, understand neural information processing mechanism. In order to discuss the neural network and fuzzy comprehensive deal of information may, the concept of chaos theory and method will play a role. The chaos is a rather difficult toprecise definition of the math concepts. In general, "chaos" it is to point to by the dynamic system of equations describe deterministic performance of the uncertain behavior, or call it sure the randomness. "Authenticity" because it by the intrinsic reason and not outside noise or interference produced, and "random" refers to the irregular, unpredictable behavior, can only use statistics method description. Chaotic dynamics of the main features of the system is the state of the sensitive dependence on the initial conditions, the chaos reflected its inherent randomness. Chaos theory is to point to describe the nonlinear dynamic behavior with chaos theory, the system of basic concept, methods, it dynamics system complex behavior understanding for his own with the outside world and for material, energy and information exchange process of the internal structure of behavior, not foreign and accidental behavior, chaos is a stationary. Chaotic dynamics system of stationary including: still, stable quantity, the periodicity, with sex and chaos of accurate solution... Chaos rail line is overall stability and local unstable combination of results, call it strange attractor.A strange attractor has the following features: (1) some strange attractor is a attractor, but it is not a fixed point, also not periodic solution; (2) strange attractor is indivisible, and that is not divided into two and two or more to attract children. (3) it to the initial value is very sensitive, different initial value can lead to very different behavior.superiorityThe artificial neural network of characteristics and advantages, mainly in three aspects: first, self-learning. For example, only to realize image recognition that the many different image model and the corresponding should be the result of identification input artificial neural network, the network will through the self-learning function, slowly to learn to distinguish similar images. The self-learning function for the forecast has special meaning. The prospect of artificial neural network computer will provide mankind economic forecasts, market forecast, benefit forecast, the application outlook is very great. The second, with lenovo storage function. With the artificial neural network of feedback network can implement this association. Third, with high-speed looking for the optimal solution ability. Looking for a complex problem of the optimal solution, often require a lot of calculation, the use of a problem in some of the design of feedback type and artificial neural network, use the computer high-speed operation ability, may soon find the optimal solution.Research directionThe research into the neural network can be divided into the theory research and application of the two aspects of research. Theory study can be divided into the following two categories:1, neural physiological and cognitive science research on human thinking and intelligent mechanism.2, by using the neural basis theory of research results, with mathematical method to explore more functional perfect, performance more superior neural network model, the thorough research network algorithm and performance, such as: stability and convergence, fault tolerance, robustness, etc.; The development of new network mathematical theory, such as: neural network dynamics, nonlinear neural field, etc.Application study can be divided into the following two categories:1, neural network software simulation and hardware realization of research.2, the neural network in various applications in the field of research. These areas include: pattern recognition, signal processing, knowledge engineering, expert system, optimize the combination, robot control, etc. Along with the neural network theory itself and related theory, related to the development of technology, the application of neural network will further.Development trend and research hot spotArtificial neural network characteristic of nonlinear adaptive information processing power, overcome traditional artificial intelligence method for intuitive, such as mode, speech recognition, unstructured information processing of the defects in the nerve of expert system, pattern recognition and intelligent control, combinatorial optimization, and forecast areas to be successful application. Artificial neural network and other traditional method unifies, will promote the artificial intelligence and information processing technology development. In recent years, the artificial neural network is on the path of human cognitive simulation further development, and fuzzy system, genetic algorithm, evolution mechanism combined to form a computational intelligence, artificial intelligence is an important direction in practical application, will be developed. Information geometry will used in artificial neural network of research, to the study of the theory of the artificial neural network opens a new way. The development of the study neural computers soon, existing product to enter the market. With electronics neural computers for the development of artificial neural network to provide good conditions.Neural network in many fields has got a very good application, but the need to research is a lot. Among them, are distributed storage, parallel processing, since learning, the organization and nonlinear mapping the advantages of neural network and other technology and the integration of it follows that the hybrid method and hybrid systems, has become a hotspot. Since the other way have their respective advantages, so will the neural network with other method, and the combination of strong points, and then can get better application effect. At present this in a neural network and fuzzy logic, expert system, genetic algorithm, wavelet analysis, chaos, the rough set theory, fractal theory, theory of evidence and grey system and fusion.汉语翻译人工神经网络(ArtificialNeuralNetworks,简写为ANNs)也简称为神经网络(NNs)或称作连接模型(ConnectionistModel),它是一种模范动物神经网络行为特征,进行分布式并行信息处理的算法数学模型。
外文文献翻译译稿和原文
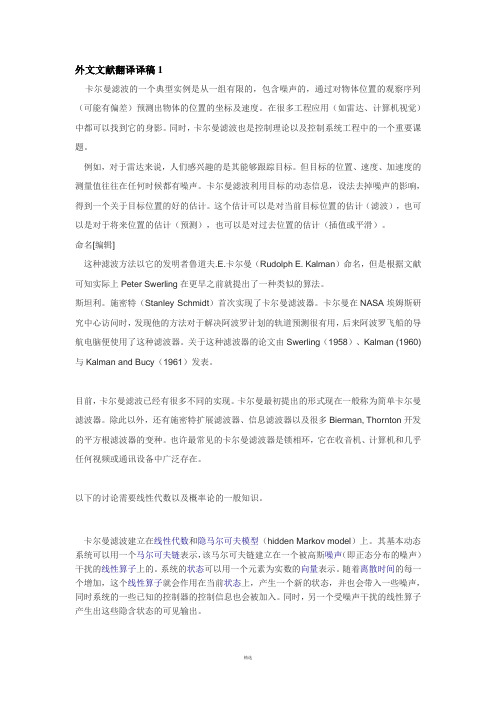
外文文献翻译译稿1卡尔曼滤波的一个典型实例是从一组有限的,包含噪声的,通过对物体位置的观察序列(可能有偏差)预测出物体的位置的坐标及速度。
在很多工程应用(如雷达、计算机视觉)中都可以找到它的身影。
同时,卡尔曼滤波也是控制理论以及控制系统工程中的一个重要课题。
例如,对于雷达来说,人们感兴趣的是其能够跟踪目标。
但目标的位置、速度、加速度的测量值往往在任何时候都有噪声。
卡尔曼滤波利用目标的动态信息,设法去掉噪声的影响,得到一个关于目标位置的好的估计。
这个估计可以是对当前目标位置的估计(滤波),也可以是对于将来位置的估计(预测),也可以是对过去位置的估计(插值或平滑)。
命名[编辑]这种滤波方法以它的发明者鲁道夫.E.卡尔曼(Rudolph E. Kalman)命名,但是根据文献可知实际上Peter Swerling在更早之前就提出了一种类似的算法。
斯坦利。
施密特(Stanley Schmidt)首次实现了卡尔曼滤波器。
卡尔曼在NASA埃姆斯研究中心访问时,发现他的方法对于解决阿波罗计划的轨道预测很有用,后来阿波罗飞船的导航电脑便使用了这种滤波器。
关于这种滤波器的论文由Swerling(1958)、Kalman (1960)与Kalman and Bucy(1961)发表。
目前,卡尔曼滤波已经有很多不同的实现。
卡尔曼最初提出的形式现在一般称为简单卡尔曼滤波器。
除此以外,还有施密特扩展滤波器、信息滤波器以及很多Bierman, Thornton开发的平方根滤波器的变种。
也许最常见的卡尔曼滤波器是锁相环,它在收音机、计算机和几乎任何视频或通讯设备中广泛存在。
以下的讨论需要线性代数以及概率论的一般知识。
卡尔曼滤波建立在线性代数和隐马尔可夫模型(hidden Markov model)上。
其基本动态系统可以用一个马尔可夫链表示,该马尔可夫链建立在一个被高斯噪声(即正态分布的噪声)干扰的线性算子上的。
系统的状态可以用一个元素为实数的向量表示。
基于神经网络和遗传算法的采油控制系统
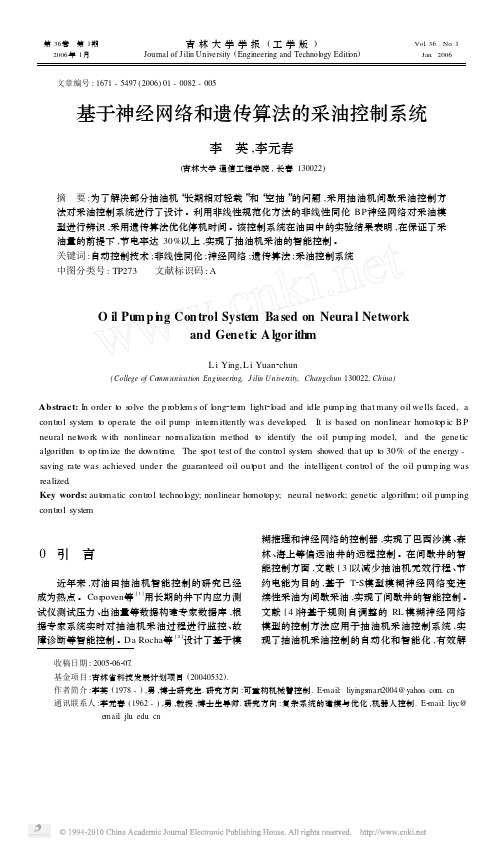
第36卷 第1期吉林大学学报(工学版) Vol .36 No .12006年1月Journal of J ilin University (Engineering and Technol ogy Editi on ) Jan .2006文章编号:1671-5497(2006)01-0082-005收稿日期:2005206207.基金项目:吉林省科技发展计划项目(20040532).作者简介:李英(1978-),男,博士研究生.研究方向:可重构机械臂控制.E 2mail:liyings mart2004@yahoo 通讯联系人:李元春(1962-),男,教授,博士生导师.研究方向:复杂系统的建模与优化,机器人控制.E 2mail:liyc@e mail .jlu .edu .cn基于神经网络和遗传算法的采油控制系统李 英,李元春(吉林大学通信工程学院,长春130022)摘 要:为了解决部分抽油机“长期相对轻载”和“空抽”的问题,采用抽油机间歇采油控制方法对采油控制系统进行了设计。
利用非线性规范化方法的非线性同伦BP 神经网络对采油模型进行辨识,采用遗传算法优化停机时间。
该控制系统在油田中的实验结果表明,在保证了采油量的前提下,节电率达30%以上,实现了抽油机采油的智能控制。
关键词:自动控制技术;非线性同伦;神经网络;遗传算法;采油控制系统中图分类号:TP273 文献标识码:AO il Pu m p i n g Con trol System Ba sed on Neura l Networkand Geneti c A lgor ithmL i Ying,L i Yuan 2chun(College of Co mm unication Engineering,J ilin U niversity,Changchun 130022,China )Abstract:I n order t o s olve the p r oble m s of l ong 2ter m light 2l oad and idle pump ing that many oil wells faced,a contr ol syste m t o operate the oil pump inter m ittently was devel oped .It is based on nonlinear homot op ic BPneural net w ork with nonlinear nor malizati on method t o identify the oil pump ing model,and the genetic algorith m t o op ti m ize the downti m e .The s pot test of the contr ol syste m showed that up t o 30%of the energy -saving rate was achieved under the guaranteed oil out put and the intelligent contr ol of the oil pu mp ing was realized .Key words:aut omatic contr ol technol ogy;nonlinear homot opy;neural net w ork;genetic algorithm;oil pu mp ing contr ol syste m0 引 言近年来,对油田抽油机智能控制的研究已经成为热点。
DeepLearning论文翻译(NatureDeepReview)

DeepLearning论⽂翻译(NatureDeepReview)原论⽂出处:by Yann LeCun, Yoshua Bengio & Geoffrey HintonNature volume521, pages436–444 (28 May 2015)译者:零楚L()这篇论⽂性质为深度学习的综述,原本只是想做做笔记,但找到的翻译都不怎么通顺。
既然要啃原⽂献,索性就做个翻译,尽⼒准确通畅。
转载使⽤请注明本⽂出处,当然实在不注明我也并没有什么办法。
论⽂中⼤量使⽤貌似作者默认术语但⼜难以赋予合适中⽂意义或会造成歧义的词语及其在本⽂中将采⽤的固定翻译有:representation-“特征描述”objective function/objective-“误差函数/误差” 本意该是⽬标函数/评价函数但实际应⽤中都是使⽤的cost function+正则化项作为⽬标函数(参考链接:)所以本⽂直接将其意为误差函数便于理解的同时并不会影响理解的正确性这种量的翻译写下⾯这句话会不会太⾃⼤了点,不过应该也没关系吧。
看过这么多书⾥接受批评指正的谦辞,张宇⽼师版本的最为印象深刻:我⽆意以“⽔平有限”为遁词,诚⼼接受批评指正。
那么,让我们开始这⼀切吧。
Nature Deep Review摘要:深度学习能让那些具有多个处理层的计算模型学习如何表⽰⾼度抽象的数据。
这些⽅法显著地促进了⼀些领域最前沿技术的发展,包括语⾳识别,视觉对象识别,对象检测和如药物鉴定、基因组学等其它很多领域。
深度学习使⽤⼀种后向传播算法(BackPropagation algorithm,通常称为BP算法)来指导机器如何让每个处理层根据它们⾃⾝前⾯的处理层的“特征描述”改变⽤于计算本层“特征描述”的参数,由此深度学习可以发现所给数据集的复杂结构。
深度卷积⽹络为图像、视频、语⾳和⾳频的处理领域都带来了突破,⽽递归⽹络(或更多译为循环神经⽹络)则在处理⽂本、语⾳等序列数据⽅⾯展现出潜⼒。
fpga英文文献翻译
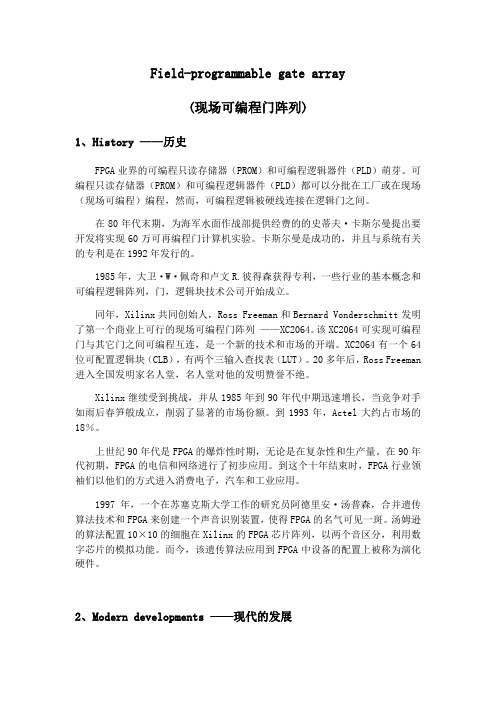
Field-programmable gate array(现场可编程门阵列)1、History ——历史FPGA业界的可编程只读存储器(PROM)和可编程逻辑器件(PLD)萌芽。
可编程只读存储器(PROM)和可编程逻辑器件(PLD)都可以分批在工厂或在现场(现场可编程)编程,然而,可编程逻辑被硬线连接在逻辑门之间。
在80年代末期,为海军水面作战部提供经费的的史蒂夫·卡斯尔曼提出要开发将实现60万可再编程门计算机实验。
卡斯尔曼是成功的,并且与系统有关的专利是在1992年发行的。
1985年,大卫·W·佩奇和卢文R.彼得森获得专利,一些行业的基本概念和可编程逻辑阵列,门,逻辑块技术公司开始成立。
同年,Xilinx共同创始人,Ross Freeman和Bernard Vonderschmitt发明了第一个商业上可行的现场可编程门阵列——XC2064。
该XC2064可实现可编程门与其它门之间可编程互连,是一个新的技术和市场的开端。
XC2064有一个64位可配置逻辑块(CLB),有两个三输入查找表(LUT)。
20多年后,Ross Freeman 进入全国发明家名人堂,名人堂对他的发明赞誉不绝。
Xilinx继续受到挑战,并从1985年到90年代中期迅速增长,当竞争对手如雨后春笋般成立,削弱了显著的市场份额。
到1993年,Actel大约占市场的18%。
上世纪90年代是FPGA的爆炸性时期,无论是在复杂性和生产量。
在90年代初期,FPGA的电信和网络进行了初步应用。
到这个十年结束时,FPGA行业领袖们以他们的方式进入消费电子,汽车和工业应用。
1997年,一个在苏塞克斯大学工作的研究员阿德里安·汤普森,合并遗传算法技术和FPGA来创建一个声音识别装置,使得FPGA的名气可见一斑。
汤姆逊的算法配置10×10的细胞在Xilinx的FPGA芯片阵列,以两个音区分,利用数字芯片的模拟功能。
电气工程的外文文献(及翻译)

电气工程的外文文献(及翻译)文献一:Electric power consumption prediction model based on grey theory optimized by genetic algorithms本文介绍了一种基于混合灰色理论与遗传算法优化的电力消耗预测模型。
该模型使用时间序列数据来建立模型,并使用灰色理论来解决数据的不确定性问题。
通过遗传算法的优化,模型能够更好地预测电力消耗,并取得了优异的预测结果。
此模型可以在大规模电力网络中使用,并具有较高的可行性和可靠性。
文献二:Intelligent control for energy-efficient operation of electric motors本文研究了一种智能控制方法,用于电动机的节能运行。
该方法提供了一种更高效的控制策略,使电动机能够在不同负载条件下以较低的功率运行。
该智能控制使用模糊逻辑方法来确定最佳的控制参数,并使用遗传算法来优化参数。
实验结果表明,该智能控制方法可以显著降低电动机的能耗,节省电能。
文献三:Fault diagnosis system for power transformers based on dissolved gas analysis本文介绍了一种基于溶解气体分析的电力变压器故障诊断系统。
通过对变压器油中的气体样品进行分析,可以检测和诊断变压器内部存在的故障类型。
该系统使用人工神经网络模型来对气体分析数据进行处理和分类。
实验结果表明,该系统可以准确地检测和诊断变压器的故障,并有助于实现有效的维护和管理。
文献四:Power quality improvement using series active filter based on iterative learning control technique本文研究了一种基于迭代研究控制技术的串联有源滤波器用于电能质量改善的方法。
参考文献(人工智能)
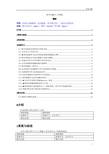
参考文献(人工智能)曹晖目的:对参考文献整理(包括摘要、读书笔记等),方便以后的使用。
分类:粗分为论文(paper)、教程(tutorial)和文摘(digest)。
0介绍 (1)1系统与综述 (1)2神经网络 (2)3机器学习 (2)3.1联合训练的有效性和可用性分析 (2)3.2文本学习工作的引导 (2)3.3★采用机器学习技术来构造受限领域搜索引擎 (3)3.4联合训练来合并标识数据与未标识数据 (5)3.5在超文本学习中应用统计和关系方法 (5)3.6在关系领域发现测试集合规律性 (6)3.7网页挖掘的一阶学习 (6)3.8从多语种文本数据库中学习单语种语言模型 (6)3.9从因特网中学习以构造知识库 (7)3.10未标识数据在有指导学习中的角色 (8)3.11使用增强学习来有效爬行网页 (8)3.12★文本学习和相关智能A GENTS:综述 (9)3.13★新事件检测和跟踪的学习方法 (15)3.14★信息检索中的机器学习——神经网络,符号学习和遗传算法 (15)3.15用NLP来对用户特征进行机器学习 (15)4模式识别 (16)4.1JA VA中的模式处理 (16)0介绍1系统与综述2神经网络3机器学习3.1 联合训练的有效性和可用性分析标题:Analyzing the Effectiveness and Applicability of Co-training链接:Papers 论文集\AI 人工智能\Machine Learning 机器学习\Analyzing the Effectiveness and Applicability of Co-training.ps作者:Kamal Nigam, Rayid Ghani备注:Kamal Nigam (School of Computer Science, Carnegie Mellon University, Pittsburgh, PA 15213, knigam@)Rayid Ghani (School of Computer Science, Carnegie Mellon University, Pittsburgh, PA 15213 rayid@)摘要:Recently there has been significant interest in supervised learning algorithms that combine labeled and unlabeled data for text learning tasks. The co-training setting [1] applies todatasets that have a natural separation of their features into two disjoint sets. We demonstrate that when learning from labeled and unlabeled data, algorithms explicitly leveraging a natural independent split of the features outperform algorithms that do not. When a natural split does not exist, co-training algorithms that manufacture a feature split may out-perform algorithms not using a split. These results help explain why co-training algorithms are both discriminativein nature and robust to the assumptions of their embedded classifiers.3.2 文本学习工作的引导标题:Bootstrapping for Text Learning Tasks链接:Papers 论文集\AI 人工智能\Machine Learning 机器学习\Bootstrap for Text Learning Tasks.ps作者:Rosie Jones, Andrew McCallum, Kamal Nigam, Ellen Riloff备注:Rosie Jones (rosie@, 1 School of Computer Science, Carnegie Mellon University, Pittsburgh, PA 15213)Andrew McCallum (mccallum@, 2 Just Research, 4616 Henry Street, Pittsburgh, PA 15213)Kamal Nigam (knigam@)Ellen Riloff (riloff@, Department of Computer Science, University of Utah, Salt Lake City, UT 84112)摘要:When applying text learning algorithms to complex tasks, it is tedious and expensive to hand-label the large amounts of training data necessary for good performance. This paper presents bootstrapping as an alternative approach to learning from large sets of labeled data. Instead of a large quantity of labeled data, this paper advocates using a small amount of seed information and alarge collection of easily-obtained unlabeled data. Bootstrapping initializes a learner with the seed information; it then iterates, applying the learner to calculate labels for the unlabeled data, and incorporating some of these labels into the training input for the learner. Two case studies of this approach are presented. Bootstrapping for information extraction provides 76% precision for a 250-word dictionary for extracting locations from web pages, when starting with just a few seed locations. Bootstrapping a text classifier from a few keywords per class and a class hierarchy provides accuracy of 66%, a level close to human agreement, when placing computer science research papers into a topic hierarchy. The success of these two examples argues for the strength of the general bootstrapping approach for text learning tasks.3.3 ★采用机器学习技术来构造受限领域搜索引擎标题:Building Domain-specific Search Engines with Machine Learning Techniques链接:Papers 论文集\AI 人工智能\Machine Learning 机器学习\Building Domain-Specific Search Engines with Machine Learning Techniques.ps作者:Andrew McCallum, Kamal Nigam, Jason Rennie, Kristie Seymore备注:Andrew McCallum (mccallum@ , Just Research, 4616 Henry Street Pittsburgh, PA 15213)Kamal Nigam (knigam@ , School of Computer Science, Carnegie Mellon University Pittsburgh, PA 15213)Jason Rennie (jr6b@)Kristie Seymore (kseymore@)摘要:Domain-specific search engines are growing in popularity because they offer increased accuracy and extra functionality not possible with the general, Web-wide search engines. For example, allows complex queries by age-group, size, location and cost over summer camps. Unfortunately these domain-specific search engines are difficult and time-consuming to maintain. This paper proposes the use of machine learning techniques to greatly automate the creation and maintenance of domain-specific search engines. We describe new research in reinforcement learning, information extraction and text classification that enables efficient spidering, identifying informative text segments, and populating topic hierarchies. Using these techniques, we have built a demonstration system: a search engine forcomputer science research papers. It already contains over 50,000 papers and is publicly available at ....采用多项Naive Bayes 文本分类模型。
- 1、下载文档前请自行甄别文档内容的完整性,平台不提供额外的编辑、内容补充、找答案等附加服务。
- 2、"仅部分预览"的文档,不可在线预览部分如存在完整性等问题,可反馈申请退款(可完整预览的文档不适用该条件!)。
- 3、如文档侵犯您的权益,请联系客服反馈,我们会尽快为您处理(人工客服工作时间:9:00-18:30)。
附录1基于神经网络和遗传算法的模糊系统的自动设计摘要本文介绍了基于神经网络和遗传算法的模糊系统的设计,其目的在于缩短开发时间并提高该系统的性能。
介绍一种利用神经网络来描绘的多维非线性隶属函数和调整隶属函数参数的方法。
还提及了基于遗传算法的集成并自动化三个模糊系统的设计平台。
1 前言模糊系统往往是人工手动设计。
这引起了两个问题:一是由于人工手动设计是费时间的,所以开发费用很高;二是无法保证获得最佳的解决方案。
为了缩短开发时间并提高模糊系统的性能,有两种独立的途径:开发支持工具和自动设计方法。
前者包括辅助模糊系统设计的开发环境。
许多环境已具有商业用途。
后者介绍了自动设计的技术。
尽管自动设计不能保证获得最优解,他们仍是可取的手工技巧,因为设计是引导走向和依某些标准的最优解。
有三种主要的设计决策模糊控制系统设计:(1)确定模糊规则数,(2)确定隶属度函数的形式。
(3)确定变化参数再者,必须作出另外两个决定:(4)确定输入变量的数量(5)确定论证方法(1)和(2)相互协调确定如何覆盖输入空间。
他们之间有高度的相互依赖性。
(3)用以确定TSK(Takagi-Sugeno-Kang)模式【1】中的线性方程式的系数,或确定隶属度函数以及部分的Mamdani模型【2】。
(4)符合决定最低套相关的输入变量,计算所需的目标决策或控制的价值观。
像逆向消除(4)和信息标准的技术在此设计中经常被利用。
(5)相当于决定使用哪一个模糊算子和解模糊化的方法。
虽然由数种算法和模糊推理的方法已被提出,仍没有选择他们标准。
[5]表明动态变化的推理方法,他依据这个推理环境的结果在性能和容错性高于任何固定的推理的方法。
神经网络模型(以更普遍的梯度)和基于遗传算法的神经网络(最常见的梯度的基础)和遗传算法被用于模糊系统的自动设计。
基于神经网络的方法主要是用来设计模糊隶属度函数。
这有两种主要的方法;(一)直接的多维的模糊隶属度函数的设计:该方法首先通过数据库确定规则的数目。
然后通过每个簇的等级的训练来确定隶属函数的形式。
更多细节将在第二章给出。
(二)间接的多维的模糊隶属度函数的设计:这种方法通过结合一维模糊隶属函数构建多维的模糊隶属度函数。
隶属度函数梯度技术被用于调节试图减少模糊系统的期望产量和实际生产所需的产出总量的误差。
第一种方法的优点在于它可以直接产生非线性多维的模糊隶属度函数;没有必要通过结合一维模糊隶属函数构建多维的模糊隶属度函数。
第二种方法的优点在于可通过监测模糊系统的最后性能来调整。
这两种方法都将在第二章介绍。
许多基于遗传算法的方法与方法二在本质上一样;一维隶属函数的形式利用遗传算法自动的调整。
这些方法中很多只考虑了一个或两个前面提及的设计问题。
在第三章中,我们将介绍一种三个设计问题同时考虑的方法。
2 神经网络方法2.1多维输入空间的直接的模糊分区该方法利用神经网络来实现多维的非线性隶属度函数,被称为基于NN的模糊推理。
该方法的优点在于它可以产生非线性多维的模糊隶属度函数。
在传统的模糊系统中,用于前期部分的一维隶属度函数是独立设计的,然后结合起来间接实现多维的模糊隶属度函数。
可以说,神经网络方法在由神经网络吸收的结合操作方面是传统模糊系统的一种更普遍的形式。
当输入变量是独立的时传统的间接设计方法就有问题。
例如,设计一个基于将温度和湿度作为输入的模糊系统的空调控制系统。
在模糊系统的传统设计方法中,隶属函数的温度和湿度是独立设计的。
输入空间所产生的模糊分区如图1(a)。
然而,当输入变量是独立的,如温度、湿度,模糊分区如图1(b)比较合适。
很难构建这样来自一维模糊隶属度函数的非线性分区。
由于NN-driven模糊推理直接构建多维的非线性的模糊隶属度函数,很有可能使线性分区如图1(b)。
NN-driven模糊推理的设计的有三个步骤:聚集给出的训练数据,利用神经网络的模糊分区输入空间,和设计各分区空间的随机部分。
第一步是要聚集培训资料,确定规则的数目。
这一步之前,不恰当的输入变量已经利用信息或淘汰落后指标的方法消除掉了。
逆向消除方法的任意消除n个输入变量和训练神经网络的n - 1个输入变量。
然后比较n个和n-1个变量的神经网络的性能。
如果n-1个变量的神经网络的性能与n个变量的性能相似或者更好,那么消除输入变量就被认为是无关紧要的。
然后这些数据被聚集,得到了数据的分布。
集群数量是规则的数目。
第二步是决定在第一步中得到的集群资料的簇边界;输入空间的划分并确定多维输入的隶属函数。
监督数据是由在第1步中获得的隶属度的输入数据聚类提供的。
第一个带有n输入和c输出的神经网络被准备好,其中n是输入变量的数量,c是在第一步中得到的集群数量。
为了神经网络的数据,图2中NN数量,产生于第一步提供的集群信息。
一般来说,每个输入变量被分配到其中的一个集群。
集群任务就是将输入变量和培训模式相结合。
例如,在属于集群2的四个集群和输入向量的案例中,监督的培训模式将是(0,1,0,0)。
在某些情况下,如果他/她相信一个输入的数据点应按不同的聚类,用户不得非法干预和手动建造部分监督。
举例来说,如果用户认为一个数据点同样属于一个两个班,适当的监管输出模式可能(0.5,0.5,0,0)。
这个神经网络在关于该培训资料的训练结束后,神经网络计算特定输入属于各集群向量。
因此,我们认为该神经网络通过学习获得特征的隶属度函数所有的规则,可以产生与隶属度相适应的任意的输入向量。
利用神经网络如发生器的模糊系统是NN-driven模糊推理。
第三步是随机的设计。
因为我们知道哪个集群能举出一个输入数据,我们可以使用输入数据和期望的结果训练随机的部分。
神经网络的表达可在这里,如[3,4]中所言,但是其他的方法,如数学方程或模糊变量,可以用来代替。
该模型的最关键的是神经网络的输入空间分割模糊聚类。
图2所示的一个例子NN-driven模糊推理系统。
这是一个输出由神经网络或TSK模型计算的一个单独的价值的模型。
在图乘法和加法计算加权平均值。
如果后续的部分输出模糊值,适当的t-conorm和/或解模糊化操作应该被使用。
图1.模糊划分:(a)常规(b)期望图2.NN-driven结构模糊推理实例2.2调整参数的模糊系统这个定义隶属度函数形式的参数来减少模糊系统输出和监督的数据之间的误差。
两种方法用于修改这些参数:摘要现有基于梯度方法和遗传算法。
遗传算法的方法将在下一章节讲述,基于梯度的方法将在这部分解释。
这个基于梯度的方法的程序是:(1)决定如何确定的隶属度函数的形式(2)利用梯度方法调整降低模糊系统的实际输出与期望输出的参数,通常最速下降。
隶属函数的中心的位置和宽度通常用来定义参数的形状。
Ichihashi et al. [6]and Nomura et al. [7, 8], Horikawa et al.[9][10], Ichihashi et al.[ll] and Wang et al. [12], Jang [13][14] 已经分别用三角形,结合sigmoidal、高斯,钟型隶属度函数。
他们利用最速下降法来调整模糊隶属函数参数。
图3. 神经网络调整模糊系统的参数图4. 调整模糊系统的神经网络图3显示了此方法和同构于图4. 图中的u ij在i-th 规则下输入模糊隶属函数的参数x j,而它实际上是代表一个描述隶属度函数的形式的参数向量。
也就是说,这个方法使模糊系统作为神经网络的模糊隶属度函数和通过节点执行重量和规则一样。
任何网络学习算法,例如反向传播算法,可以用来设计这种结构。
3遗传算法方法3.1遗传算法与模糊控制遗传算法是进行优化、生物激励的技术,他的运行用二进制表示,并进行繁殖,交叉和变异。
繁殖后代的权利是通过应用程序提供的一种健身价值。
遗传算法吸引人是因为他们不需要存在的衍生物,他们的同时搜索的鲁棒性很强,并能避免陷入局部最小。
SeverM的论文提出了利用自动遗传算法的模糊系统的设计方法。
大量的工作主要集中在调整的模糊隶属度函数[17]-[25]。
其他的方法使用遗传算法来确定模糊规则数[18,26]。
在[26]中,通过专家制定了一系列规则,并且遗传算法找到他们的最佳的组合。
在[18],卡尔已经开发出一种方法用于测定模糊隶属度函数和模糊规则数。
在这篇文章中,卡尔的方法首先用遗传算法按照预先定义的规则库确定规则的数目。
这个阶段后,利用遗传算法来调整模糊隶属度函数。
虽然这些方法设计的系统表现的比手工设计的系统好,它们仍可能会欠佳,因为他们一次只有一个或两个三大设计阶段。
因为这些设计阶段可能不会是独立的,所以重要的是要考虑它们同时找到全局最优解。
在下一节里,我们提出一个结合的三个主要设计阶段自动设计方法。
3.2基于遗传算法的模糊系统的综合设计这部分提出了一种利用遗传算法的自动模糊系统的设计方法, 并将三个主要设计阶段一体化:隶属函数的形式, 模糊规则数, 和规则后件同一时间内确定[27]。
当将遗传算法应用于程序上时,有两个主要步骤;(a)选择合适的基因表达,(b) 设计一个评价函数的人口排名。
在接下来的段落里,我们讨论我们的模糊系统表现及基因表达。
一个嵌入验前知识的评价函数和方法将会在接下来的章节中提及模糊系统和基因表现我们用TSK模型的模糊系统,它被广泛应用于控制问题,对地图系统的状态来控制的价值。
TSK模型在随之而来的模糊模型中的线性方程与模糊语言表达方面有别于传统的模糊系统。
例如,一个TSK模型规则的形式:如果X1是A,X2是B,那么y=w1X1+w2X2+w3;是常数其中wn最后的控制价值通过每个规则的输出和依据规则的加权的射击力量来计算。
我们利用左基地,右基地,和以往的中心点距离(第一个中心是一个绝对的位置)对三角形隶属度函数进行参数化,。
其他参数化的形状,如双曲形、高斯,钟形,或梯形可以代替。
不同于大多数方法、重叠限制不是放在在我们的系统和完整的重叠存在(见图5)。
图5. (a)隶属度函数表示法(b)可能的隶属函数一般来说,每个输入变量模糊集理论的数量与确定的模糊规则的数目相结合。
例如,一个带有m输入变量的TSK模型,每n个模糊集,将会产生n m模糊规则。
因为规则的数目直接取决于这个数目的隶属函数,消除隶属度函数对消除规则有直接的影响。
每一隶属函数需要三个参数并且每一个模糊规则需要三个参数。
因此,每个变量需要n个模糊输入的m-input-one-output系统要求3(mn+n m)参量。
这个基因表达明确包含三个成员函数的参数及前述的随机参数。
然而,规则的数,通过应用编码含蓄的边界条件和隶属函数的定位。
我们可以隐含的控制规则的数目,消除隶属函数的中心位置的范围之外的相应的包含这些内容的输入变量和规则。
例如,在单摆应用隶属度函数使用θ的中心位置大于90°都是可以避免的。