Multi-factor volatility and stock returns
Volatility and Commodity Price Dynamics
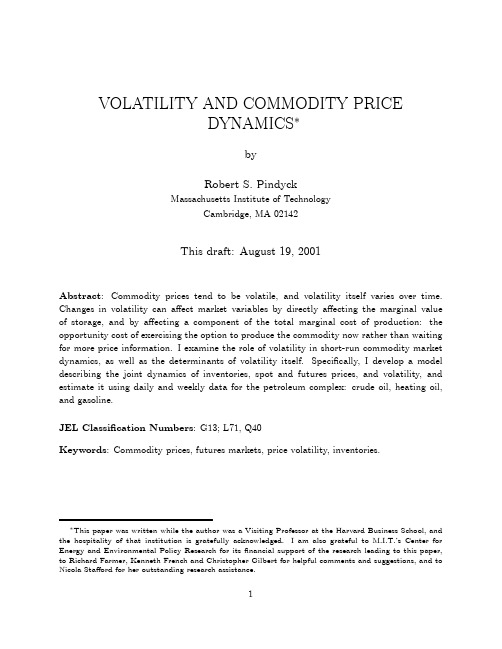
VOLATILITY AND COMMODITY PRICEDYNAMICS∗byRobert S.PindyckMassachusetts Institute of TechnologyCambridge,MA02142This draft:August19,2001Abstract:Commodity prices tend to be volatile,and volatility itself varies over time. Changes in volatility can affect market variables by directly affecting the marginal value of storage,and by affecting a component of the total marginal cost of production:the opportunity cost of exercising the option to produce the commodity now rather than waiting for more price information.I examine the role of volatility in short-run commodity market dynamics,as well as the determinants of volatility itself.Specifically,I develop a model describing the joint dynamics of inventories,spot and futures prices,and volatility,and estimate it using daily and weekly data for the petroleum complex:crude oil,heating oil, and gasoline.JEL Classification Numbers:G13;L71,Q40Keywords:Commodity prices,futures markets,price volatility,inventories.∗This paper was written while the author was a Visiting Professor at the Harvard Business School,and the hospitality of that institution is gratefully acknowledged.I am also grateful to M.I.T.’s Center for Energy and Environmental Policy Research for itsfinancial support of the research leading to this paper, to Richard Farmer,Kenneth French and Christopher Gilbert for helpful comments and suggestions,and to Nicola Stafford for her outstanding research assistance.1Introduction.Most commodity markets are characterized by periods of sharp changes in prices and inven-tory levels.In addition,the level of volatility itselffluctuates over time.This paper examines the short-run dynamics of commodity prices and inventories,with a particular focus on the role of volatility.A goal is to determine how changes in spot prices,futures prices,and inventories are affected by changes in volatility,and to elucidate the channels through which such effects occur.Another objective is to examine the behavior of volatility itself.Understanding the behavior and role of volatility is important for several reasons.First, as I show here,including volatility as a market variable can help us better understand short-run commodity market dynamics.Also,price volatility is a key determinant of the value of commodity-based contingent claims,including opportunities to invest in production facilities.Thus understanding its behavior is important for derivative valuation,hedging decisions,and decisions to invest in production facilities.Changes in volatility affect prices,production,and inventories in two main ways.First, volatility directly affects the marginal value of storage,or,as it is commonly called,marginal convenience yield,i.e.,theflow of benefits from an extra unit of inventory held by producers and/or consumers of the commodity.When prices–and hence production and demand–are more volatile,consumers and producers have a greater demand for inventories,which are needed to smooth production and deliveries,and reduce marketing costs.Thus an increase in volatility can lead to inventory build-ups and thereby raise prices in the short run.Second,volatility affects the total marginal cost of production by affecting the size of the“option premium.”Commodity producers(like producers of most goods)hold operating options,with an exercise price equal to direct marginal production and a payoffequal to the market price of the commodity.The total cost of producing a marginal unit of the commodity equals the direct marginal cost of production plus the opportunity cost of exercising thefirm’s operating option now rather than waiting for new price information.The greater is the volatility of price,the greater is the value of this option,and the greater is the opportunity cost of producing now.Thus an increase in volatility can result in a decrease in production.Using a two-period model,Litzenberger and Rabinowitz(1995)have shown that this option value leads to backwardation in futures markets.Using data for crude oil,Litzenberger and Rabinowitz(1995)showed that consistent with the theory,production is negatively correlated with price volatility,and the extent of futures market backwardation is positively correlated with price volatility.Also,Schwartz (1997)and Schwartz and Smith(2000)have shown how futures and spot prices can be used to estimate the parameters of a mean-reverting price process and derive values of contingent claims on the commodity.I go further and show how volatility and option value can be incorporated in a complete equilibrium model of a commodity market.In this paper,I develop a weekly model that relates the dynamics of inventories,spot and futures prices,and the level of volatility.I estimate the model using data for the three commodities that make up the petroleum complex:crude oil,heating oil,and gasoline.To estimate volatility,I use sample standard deviations of adjusted daily log changes in spot and futures prices.In addition to its simplicity,this approach has the advantage that it does not require a parametric model describing the evolution of volatility over time.1 As shown in this paper,at least for the petroleum complex,changes in price volatility are not predicted by market variables such as spot prices,inventory levels,or convenience yields,and can be viewed as largely exogenous.The volatility of,say,crude oil prices can be forecasted by past levels of volatility,but the marginal forecasting power of market variables is very low.However,changes in volatility directly affect market variables,by affecting the marginal value of storage,and by affecting price and production through the option premium.In addition,changes in the value of storage affect production,inventory holdings, and spot prices,so these variables are indirectly affected by changes in volatility.This paper also provides evidence on how inventory holdings affect short-run price move-ments.In a competitive commodity market,inventories can be used to reduce costs of varying production(when marginal cost is increasing),and to reduce marketing costs by facilitating production and delivery scheduling and avoiding stockouts.These latter factors 1This approach was also used by Campbell et.al.(2001)in their recent study of the behavior of stock price volatility.make it costly forfirms to reduce inventories beyond some minimal level,even if marginal production cost is constant.The extent to which price will move in the short run depends on the cost of varying production as well as the cost of drawing down inventories.Equilibrium inventory behavior is the solution to a stochastic dynamic optimization prob-lem.Early studies of manufacturing inventories(as well as Eckstein and Eichenbaum’s(1985) study of crude oil inventories)rely on a linear-quadratic specification to obtain an analyt-ical solution to this problem.This is unrealistic for commodity markets because the cost of drawing down inventory is highly convex in the stock of inventory,rising rapidly as the stock falls toward zero,and remaining very small as the stock varies across moderate to high levels.Therefore,as in my earlier study of commodity inventories,I adopt a more general specification and estimate the Euler equations that follow from intertemporal optimization.2 In addition,I use futures market data to obtain a direct measure of the marginal value of storage(i.e.,the convenience yield).This paper differs from my earlier study in several respects.First,I explicitly account for price volatility as a determinant of the marginal value of storage,and as a factor affecting the value offirms’operating options,and hence the full marginal cost of production.I can thereby estimate the extent to which changes in volatility will affect the levels of prices and inventories,and I obtain evidence on the channels through which these effects occur.In addition,I examine the determinants of price volatility itself.Finally,by earlier work was based on monthly data,but commodity marketfluctuations occur on a shorter time scale. By estimating a weekly model,I obtain a clearer picture of market dynamics.In the next section,I lay out a model of short-run commodity market dynamics that links prices,inventories,convenience yield,and volatility.The model includes a set of Euler equations(first-order conditions)and cannot be solved analytically.However,in Section3I use phase diagrams to trace through the(theoretical)effects of various shocks.In Section4, I discuss the data set,and examine the behavior of price volatility and market variables2See Pindyck(1994).This approach has also been used in studies of manufacturing inventories;see,e.g., Miron and Zeldes(1988)and Ramey(1991).Considine(1997)and Considine and Heo(2000)have estimated Euler equation models of inventory behavior for various petroleum products,focusing on the joint production characteristics of petroleum refining.for each commodity.I show that these volatilities are rapidly mean reverting,but can be viewed as largely exogenous with respect to market variables such as inventory changes and price.Section4also discusses the results of estimating the full model using General Method of Moments(GMM).In Section5,I use the model to examine the impact of shocks to volatility on price,inventories,and convenience yield.Section6concludes.2A Model of Prices,Inventories,and Volatility.In this section I lay out a structural model that describes equilibrium in two competitive markets:the cash market for spot purchase and sale of the commodity,and the market for storage,in which an equilibrium level of inventories is held at a“price”equal to marginal value,i.e.,marginal convenience yield.Together,these markets determine the spot price, the inventory level,and the convenience yield(and hence,implicitly,the futures price).The model accounts for the role of volatility in both of these markets.2.1Cash Markets and Storage Markets.In a competitive commodity market subject to stochasticfluctuations in production and/or consumption,producers(and to a lesser extent,consumers and third parties)will hold in-ventories.Producers hold them to reduce costs of adjusting production over time,and also to reduce marketing costs by facilitating production and delivery scheduling and avoiding stockouts.If marginal production costs are increasing with the rate of output and if de-mand isfluctuating,producers can reduce costs over time by selling out of inventory during high-demand periods,and replenishing inventories during low-demand periods.Inventories also serve as a“lubricant”to facilitate scheduling and reduce marketing costs.Industrial consumers of a commodity also hold inventories,to facilitate their own production processes.To the extent that inventories can reduce production and marketing costs in the face of changing demand conditions,they will reduce the magnitude of short-run pricefluctuations. Also,because it is costly forfirms to reduce inventory holdings beyond some minimal level, price volatility tends to be greater during periods when inventories are low.When inventory holdings can change,the market-clearing price is determined not only by current production and consumption,but also by inventories.Thus,we must account for equilibrium in both the cash and storage markets.In the cash market,purchases and sales of the commodity for immediate delivery occur at a price that I will refer to as the“spot price.”Equilibrium in this market defines a relationship between the spot price and net demand,i.e.,the difference between production and consumption.To see this,write consumption demand as Q=Q(P,z1),where P is the spot price and z1is a vector of demand-shifting variables.Likewise,write the supply function as x=x(P,z2),where z2is a vector of supply-shifting variables.Letting N t denote the inventory level,the change in inventories at time t is:∆N t=x(P,z2)−Q(P,z1).This just says that the cash market is in equilibrium when net demand(the demand for production in excess of consumption)equals net supply.We can rewrite this in terms of the following inverse net demand function:P t=f(∆N t;z1,z2).(1)Market clearing in the cash market therefore implies a relationship between the spot price and the change in inventories.Now consider the market for storage.At any instant of time,the supply of storage is the total quantity of inventories,N t.In equilibrium,this must equal the quantity demanded, which is a function of price.The price of storage is the“payment”by inventory holders for the privilege of holding a unit of inventory,and has three components:the cost of physical storage(e.g.,tanks to hold heating oil);the opportunity cost of forgone interest;and any expected depreciation or appreciation in the spot price.The price of storage will equal the value of theflow of services from the marginal unit of inventory,and is usually referred to as marginal convenience yield.Denoting the price of storage byψt,the demand for storage function can be written as N(ψt,z3),where z3is a vector of demand-shifting variables,such as temperature.One important component of z3is the volatility of price,which is a goodproxy for market volatility in general.3Writing this as an inverse demand function,we have:ψt=g(N,z3).(2)Thus market clearing in the storage market implies a relationship between marginal conve-nience yield(the price of storage)and the demand for storage.Market equilibrium is determined from eqns.(1)and(2),and an additional equation (to be derived shortly)describing the dynamic tradeoffbetween producing and selling out of inventory.Given values for the exogenous variables z1,z2,and z3,these three equations determine the values at each point in time of the three endogenous variables P t,N t,andψt.2.2Operating Options and Convenience Yield.Consider the incremental production decision for afirm that produces a commodity from a fixed quantity of reserves or other raw material,has a constant marginal production cost c, and faces a market price thatfluctuates stochastically.Thefirm has an option to produce a unit now(and receive incremental net revenue P−c),or wait and possibly produce the unit in the future.At any point in the future,the net payofffrom exercising this option is V=max[0,P t−c].The greater the volatility of price,the greater is the expected value ofthis future payoff,and thus the greater is the opportunity cost of exercising the option now rather than waiting.Thus price will exceed marginal cost by a premium,which I denote by ωt.(See Dixit and Pindyck(1994)for a detailed discussion.)I discuss the determination ofωt later;here,simply note thatωt is an increasing function of volatility.Hence an increase in volatility increases the opportunity cost of producing today,and raises full marginal cost.Next,consider the net(of storage costs)marginal convenience yield that we can measure by comparing spot and futures prices:ψt−k=(1+r)P t−F1t,(3)3The marginal value of storage is small when the total stock of inventories is large(because one more unit of inventory is of little extra benefit),but can rise sharply when the stock becomes small.Thus the demand for storage function should be downward sloping and convex,i.e.,∂N/∂ψ<0and∂2N/∂ψ2>0.where F1t is the futures price at time t for a contract maturing at time t+1,r is the one-period interest rate,and k is the one-period cost of storage.4As discussed above,ψt is the value of theflow of production-and delivery-facilitating services from the marginal unit of inventory,a value that should be greater the greater is the volatility of price.However,ψt also includes operating options,such as the value of keeping oil in the ground rather than producing it now.To see this,suppose that inventories yield no other services,and k=0. We would still needψt>0for oil production to take place at all.Ifψt were equal to zero, producers would have no incentive to exercise their options to produce(just as a call option on a non-dividend paying stock is optimally exercised only at expiration).Put differently, oil in the ground provides a price-protection service,the value of which is positive and is included inψt.Thus even if inventory levels are large,we should observe at least weak backwardation in the futures market.5This simply reflects the fact that there is some value to delaying production and waiting for more information about prices,even if the expected future spot price is less than the current spot price.Furthermore,this value will be greater the greater is the volatility of price.In summary,volatility should affect convenience yield in two ways,and in both cases positively.First,it should affect the value of theflow of production-and delivery-facilitating services that inventories provide.Second,it should affect the price-protection service that is part of convenience yield.As an empirical matter,it will not be possible to measure these effects separately.Instead,we can only measure the combined effect,and test whether convenience yield depends positively on volatility.4To see why eqn.(3)must hold,note that the(stochastic)return from holding a unit of the commodity for one period isψt+(P t+1−P t)−k.Suppose that one also shorts a futures contract.The return on this futures contract is F1t−F1,t+1=F1t−P t+1,so one would receive a total return equal toψt+(F1t−P t)−k. No outlay is required for the futures contract,and this total return is non-stochastic,so it must equal the risk-free rate times the cash outlay for the commodity,i.e.,rP t,from which eqn.(3)follows.Because futures contracts are marked to market,strictly speaking,F1t should be a forward price.For most commodities, however,the difference between the futures and forward prices is negligible.5For a detailed discussion of this point and derivation ofψt in the context of a two-period model,see Litzenberger and Rabinowitz(1995).McDonald and Shimko(1998)also address this point,and measureψt and its components for the gold market.2.3Costs.I now turn to the specification of the model that will be estimated.The total economic cost of commodity production,marketing,and storage is given by:TC=C(x)+Ω(x;σ,r)+Φ(N,P,σ)+kN,(4)and has four components:•C(x)is direct production cost,which I assume is quadratic in the production level x.•Ω(x;σ,r)is the opportunity cost of producing x now,rather than waiting.As explained below,it depends on the level of price volatility,σ,and the risk-free interest rate r.•Φ(N,P,σ)is total marketing cost,i.e.,the cost of production and delivery scheduling and avoidance of stockouts,and is decreasing in the level of inventories N.•k is the per-unit cost of physical storage,which I assume is constant.Two other variables must be defined.First,ψ=−∂Φ/∂N is the marginal value of storage,i.e.,marginal convenience yield:ψt=(1+r)P t−F1t+k.Second,ω=∂Ω/∂x is the marginal option premium,i.e.,the opportunity cost of exercising the option to produce an incremental unit of the commodity,given a total production level x.These components of cost are modelled as follows.I assume that the direct cost of production is quadratic.For crude oil,direct cost is:C(x)=(c0+ηt)x t+1c1x2t,(5)2whereηt is a random shock.Note that there are no input cost variables(such as wage rates)in(5);such variables cannot be measured–and are unlikely to vary much–on a weekly basis.For heating oil and gasoline,however,the cost of the crude oil input is a large component of direct production cost,and must be accounted for:c1x2t+c2P C,t x t,(6)C(x)=(c0+ηt)x t+12where P C,t is the price of crude oil.Marketing cost should be roughly proportional to the price of the commodity.It should also be increasing in the level of price volatility,which I use as a proxy for market volatility in general.6Higher volatility makes scheduling and stockout avoidance more difficult,and thus should increase the demand for storage.Ideally,the total marketing cost functionΦshould be derived from a dynamic optimizing model that accounts for stockout costs and costs of scheduling and managing production and shipments,but that is beyond the scope of this work.Instead,I assume that this function is isoelastic in price,the variance of log price changes,and the total inventory level:Φ(N,P,σ)=1α3−1exp(b0+11X j=1b j DUM jt)Pα1t(σ2t)α2N1−α3t,(7)where DUM jt are monthly time dummies andα3>1.This implies that the marginal value of storage(marginal convenience yield),−∂Φ/∂N,can be written as:logψt=b0+11X j=1b j DUM jt+α1log P t+α2logσ2t−α3log N t.(8)To model the marginal opportunity costωt=∂Ωt/∂x t,we need an expression for the value of the option to produce a marginal unit of the commodity,and the optimal price P∗at which that option should be exercised.The difference between P∗and the direct marginal cost C0(x)is the opportunity cost of exercising the option to produce the marginal unit. Valuing this option requires assumptions about the stochastic dynamics of price.To account for the fact that prices tend to be strongly mean-reverting,I assume that the price process can be written in continuous time as:dP/P=λ(µ−P)dt+σdz,(9)or equivalently:d log P=λ(µ−12σ2−P)dt+σdz.(10) Here,µis the“normal”price to which P t tends to revert andλis the speed of reversion.I treatσas a constant because allowing for stochastic volatility precludes a closed-form6Price volatility is highly correlated with the volatility of consumption and production.Also,price fluctuations themselves(whether caused byfluctuations in net demand,or something else,such as speculative buying and selling)cause consumption and production tofluctuate.solution for the option value.Furthermore,it should not affect the way in which the option value depends on volatility,although it will affect its magnitude(overstating it).To account for this,I include a scaling coefficient that is estimated as part of the model.7 In the Appendix,I show that if the price process follows eqn.(10)and direct marginal cost is non-stochastic,a series solution can be found for the value of the option to produce. For estimation purposes,I use a quadratic approximation to this solution.As shown in the Appendix,letting r denote the risk-free interest rate andρthe risk-adjusted expected return on the commodity,the opportunity costωt can be written as:ωt=1√γ1γ2−µ,(11)whereγ1=λθλµ+θσ2,γ2=λ(θ+1)2λµ+(2θ+1)σ2,(12)andθ=12+(ρ−r−λµ)σ2+v u u t"(r−ρ+λµ)σ2−12#2+2rσ2.(13)I include a scaling coefficient,so that c2ωt is the marginal opportunity cost.Note that the estimated value of c2should be close to1.2.4Euler Equations.With expressions for the components of cost,we can solve the intertemporal profit max-imization problem,making use of the fact that in the U.S.markets for crude oil and oil products are reasonably competitive,so that producers can be treated as price takers.Of course much of the crude oil and some of the gasoline consumed in the U.S.is imported, but the presence of imports will simply make the domestic net demand function(which I estimate)more elastic than it would be otherwise.(If the supply of imports is highly elastic, the spot price will have little or no dependence on the change in domestic inventories.) 7The numerical analyses of Hull and White(1987)suggest that treatingσas non-stochastic makes little quantitative difference in any case.See,also,Franks and Schwartz(1991).Taking prices as given,firms choose production and inventory levels to maximize the present value of the expectedflow of profits:max E t∞Xτ=0Rτ,t(P t+τQ t+τ−TC t+τ),(14) where Rτ,t is theτ-period discount factor,Q is sales,TC is given by eqn.(4),and the maximization is subject to the accounting identity∆N t=x t−Q t.(15) (The maximization is subject to the additional constraint that N t+τ≥0for allτ,but because Φ→∞as N→0,this constraint will never be binding.)To obtainfirst-order conditions,first maximize with respect to x t,holding N tfixed so that∆x t=∆Q t:P t=c00+c1x t+c2P C,t+c3ωt+ηt.(16) (I have included the term for the crude oil input price,c2P C,t,so this equation would apply to heating oil and gasoline;for crude oil this term is dropped.)It will be convenient to eliminate production and write the model in terms of prices and inventories.In the short run(a period of one week),consumption should be very inelastic with respect to price,so I model it as:Q t=Q+11X j=1d j DUM jt+c4HDD t+c5CDD t+c6T t+²t,(17)where the DUM jt are monthly dummies,HDD and CDD are,respectively,heating and cool-ing degree days,and T is a time trend.Thus I assume that consumptionfluctuates seasonally and in response to changes in temperature,is subject to(possibly serially correlated)random shocks(²t),but is insensitive to price.Substituting for Q t in eqn.(15)and rearranging:x t=∆N t+Q+11X j=1d j DUM jt+c4HDD t+c5CDD t+c6T t+²t(18)Thus eqn.(16)can be rewritten as:P t=c0+c1∆N t+c2P C,t+c3ωt+c4HDD t+c5CDD t+c6T t+11X j=1d j DUM jt+c1²t+ηt,(19)where c0=c00+c1Q.Eqn.(19)describes market clearing in the cash market.8 Next,maximize eqn.(14)with respect to N t,holding Q t and N t+1fixed:0=E t[c0(1−R1t)+ψt−k+ηt−R1tηt+1+c1(x t−R1t x t+1)+c2(P C,t−R1t P C,t+1)+c3(ωt−R1tωt+1)].(20) Over a one-week time period,R1t≈1.Making this substitution and also substituting eqn.(18)for x t,yields the secondfirst-order condition:0=E t[c1∆2N t+1+ψt−k+c2∆P C,t+1+c3∆ωt+1+11X j=1d j∆DUM j,t+1+c4∆HDD t+1+c5∆CDD t+1+c6+∆ηt+1+∆²t+1],(21)where∆2N t+1≡∆N t+1−∆N t.Eqn.(19)simply equates price with full marginal cost,where the latter includes the opportunity cost of exercising the marginal operating option.It contains error terms repre-senting the unexplained part of marginal cost(ηt)and unanticipated shocks to demand(²t). Eqn.(21)describes the tradeoffbetween selling out of inventory versus producing.To see this,rearrange the equation so thatψt−k is on the left-hand side.The equation then says that net marginal convenience yield(the savings in marketing costs over the coming period from having another unit of inventory,net of storage costs)should equal the expected change in production cost(the increase this period minus the decrease next period)from producing a unit now rather than selling it from inventory and then replenishing inventory by producing it next period.The expected change in production cost can come from expected changes in input prices,expected changes in opportunity costs,and expected increases in cost due to convexity of the cost function.To estimate the model,I substitute eqn.(8)forψt in eqn.(21).Also,because estimation is by GMM,I drop the expectation operator and use actual values of variables dated at t+1:0=c1∆2N t+1+exp(b0+11X j=1b j DUM jt)Pα1t(σ2t)α2N−α3t−k+c2∆P C,t+1+c3∆ωt+1+8Eqn.(19)is an expanded version of a model that has been used by a number of other authors.See,for example,Williams and Wright(1991),Routledge,Seppi,and Spatt(2000),and Schwartz and Smith(2000).11X j=1d j∆DUM j,t+1+c4∆HDD t+1+c5∆CDD t+1+c6+∆ηt+1+∆²t+1.(22) The model is closed by including eqn.(8)for the marginal convenience yield.Together, eqns.(19),(22),and(8)describe the evolution of the state variables P t,N t,andψt.As I show later,it is reasonable to treat the fourth state variable,the volatilityσt,as exogenous. 3Market Dynamics.Before discussing the estimation of this model,it is useful to examine its theoretical impli-cations for market dynamics and the effects of volatility and other shocks.Although the model contains a complete empirical description of the market,I have not actually solved thefirm’s stochastic dynamic optimization problem(beyond deriving first-order conditions).Thus I cannot calculate optimal trajectories for market variables that correspond to particular stochastic processes for demand,cost,and volatility shocks. However,I can analyze deterministically optimal trajectories for market variables,consistent withfirms choosing output and inventory levels that are solutions to the corresponding deterministic optimization problem.I examine such trajectories qualitatively as a way of characterizing the market behavior implied by the ter I use this approach to quantitatively estimate the response of prices and inventories to various shocks.First,consider the(deterministic)steady-state equilibrium in which there are no seasonal variations in cost or demand,σis constant,and there are no other shocks so that∆N=∆2N=∆P=0.Replacing expectations with actual values in eqn.(21),treating HDD and CDD as constants and setting the time dummies and time trend parameter c6to zero,and using overbars to denote equilibrium values,we then haveψ=k.Also,P=c0+c3ω,9andN=bPα1/α3σ2α2/α3k−1/α3,where b=e b0/α3.We can now draw a phase diagram for the two state variables N and∆N.When esti-mating the model,Ifind that for both crude oil and heating oil,the parametersα1andα3 9This applies to crude oil.For heating oil or gasoline,P=c0+c2P C+c3ω.。
volatility surface
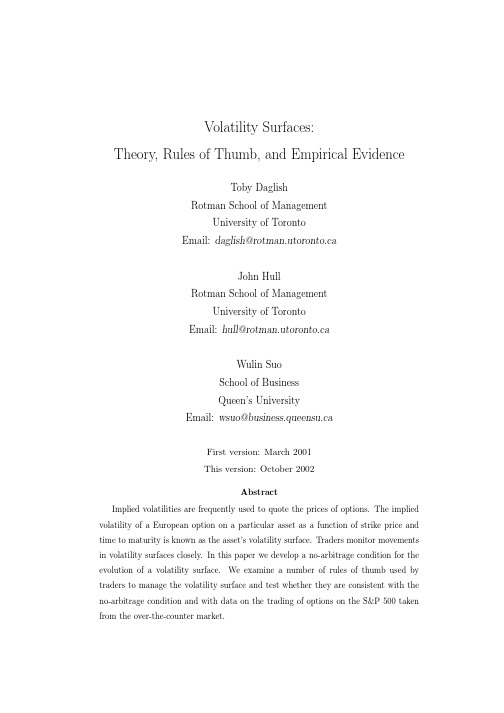
1
risk when these options are priced. Traders also use the volatility surface in an ad hoc way for hedging. They attempt to hedge against potential changes in the volatility surface as well as against changes in the asset price. As described in Derman (1999) one popular approach to hedging against asset price movements is the “volatility-by-strike” or “sticky strike” rule. This assumes that the implied volatility for an option with a given strike price and maturity will be unaffected by changes in the underlying asset price. Another popular approach is the “volatility-bymoneyness” or “sticky delta” rule. This assumes that the volatility for a particular maturity depends only on the moneyness (that is, the ratio of the price of an asset to the strike price). The first attempts to model the volatility surface were by Rubinstein (1994), Derman and Kani (1994), and Dupire (1994). These authors show how a one-factor model for an asset price, known as the implied volatility function (IVF) model, can be developed so that it is exactly consistent with the current volatility surface. Unfortunately, the evolution of the volatility surface under the IVF model can be unrealistic. The volatility surface given by the model at a future time is liable to be quite different from the initial volatility surface. For example, in the case of a foreign currency the initial U-shaped relationship between implied volatility and strike price is liable to evolve to one where the volatility is a monotonic increasing or decreasing function of strike price. Dumas, Fleming, and Whaley (1997) have shown that the IVF model does not capture the dynamics of market prices well. Hull and Suo (2002) have shown that it can be dangerous to use the model for the relative pricing of barrier options and plain vanilla options. In the first part of this paper we develop a general diffusion model for the evolution of a volatility surface and derive a restriction on the specification of the model necessary for it to be a no-arbitrage model. Other researchers that have independently followed a similar approach are Ledoit and Santa Clara (1998), Sch¨ onbucher (1999), and Brace et al (2001). In addition, Britten–Jones and Neuberger (2000) produce some interesting results characterizing the set of all continuous price processes that are consistent with a given set of option prices. Our work is different from that of other researchers in that we a) investigate the implications of the no-arbitrage condition for the shapes of the volatility surfaces likely to be observed in different situations and b) examine whether the various rules of thumb that have been put forward by traders are consistent with the no-arbitrage condition. We also extend the work of Derman (1999) to examine whether the rules of thumb are supported 2
基于多因子模型的量化投资研究
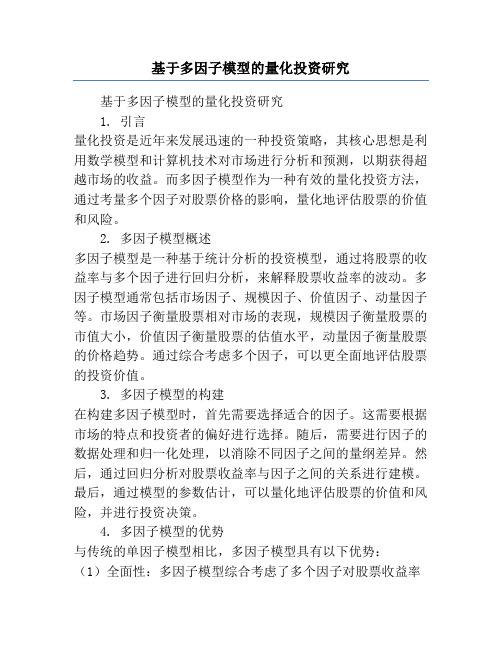
基于多因子模型的量化投资研究基于多因子模型的量化投资研究1. 引言量化投资是近年来发展迅速的一种投资策略,其核心思想是利用数学模型和计算机技术对市场进行分析和预测,以期获得超越市场的收益。
而多因子模型作为一种有效的量化投资方法,通过考量多个因子对股票价格的影响,量化地评估股票的价值和风险。
2. 多因子模型概述多因子模型是一种基于统计分析的投资模型,通过将股票的收益率与多个因子进行回归分析,来解释股票收益率的波动。
多因子模型通常包括市场因子、规模因子、价值因子、动量因子等。
市场因子衡量股票相对市场的表现,规模因子衡量股票的市值大小,价值因子衡量股票的估值水平,动量因子衡量股票的价格趋势。
通过综合考虑多个因子,可以更全面地评估股票的投资价值。
3. 多因子模型的构建在构建多因子模型时,首先需要选择适合的因子。
这需要根据市场的特点和投资者的偏好进行选择。
随后,需要进行因子的数据处理和归一化处理,以消除不同因子之间的量纲差异。
然后,通过回归分析对股票收益率与因子之间的关系进行建模。
最后,通过模型的参数估计,可以量化地评估股票的价值和风险,并进行投资决策。
4. 多因子模型的优势与传统的单因子模型相比,多因子模型具有以下优势:(1)全面性:多因子模型综合考虑了多个因子对股票收益率的影响,可以更全面地评估股票的价值和风险。
(2)稳定性:多因子模型通过考虑多个因子,可以降低单个因子的不确定性对投资组合的影响,提高投资策略的稳定性。
(3)有效性:多因子模型通过统计分析和回归分析,可以对不同因子的权重进行优化调整,从而提高投资组合的收益率。
5. 多因子模型的应用多因子模型在量化投资中有广泛的应用。
一方面,多因子模型可以用于股票的选择和投资组合的构建。
通过量化评估股票的价值和风险,可以选择具有良好投资价值的股票,并构建具有较高收益率和较低风险的投资组合。
另一方面,多因子模型还可以用于市场的预测和交易信号的生成。
通过对多个因子的综合分析,可以预测市场的走势,并基于此生成交易信号。
Multifactor Explanations of Asset Pricing Anomalies
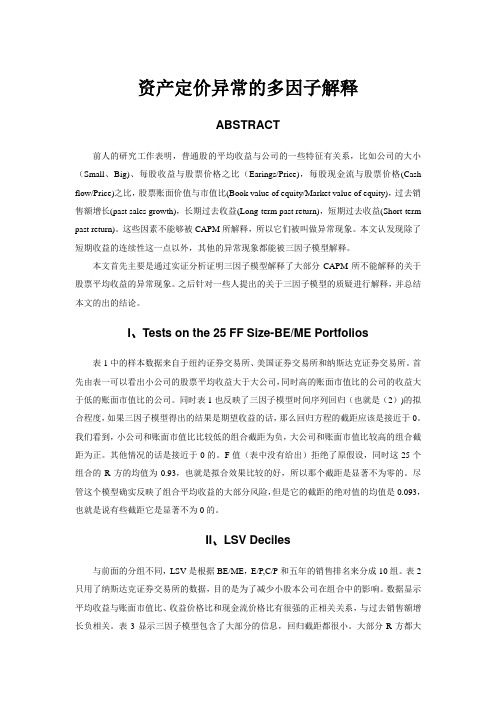
资产定价异常的多因子解释ABSTRACT前人的研究工作表明,普通股的平均收益与公司的一些特征有关系,比如公司的大小(Small、Big)、每股收益与股票价格之比(Earings/Price),每股现金流与股票价格(Cash flow/Price)之比,股票账面价值与市值比(Book value of equity/Market value of equity),过去销售额增长(past sales growth),长期过去收益(Long-term past return),短期过去收益(Short-term past return)。
这些因素不能够被CAPM所解释,所以它们被叫做异常现象。
本文认发现除了短期收益的连续性这一点以外,其他的异常现象都能被三因子模型解释。
本文首先主要是通过实证分析证明三因子模型解释了大部分CAPM所不能解释的关于股票平均收益的异常现象。
之后针对一些人提出的关于三因子模型的质疑进行解释,并总结本文的出的结论。
I、Tests on the 25 FF Size-BE/ME Portfolios表1中的样本数据来自于纽约证券交易所、美国证券交易所和纳斯达克证券交易所。
首先由表一可以看出小公司的股票平均收益大于大公司,同时高的账面市值比的公司的收益大于低的账面市值比的公司。
同时表1也反映了三因子模型时间序列回归(也就是(2))的拟合程度,如果三因子模型得出的结果是期望收益的话,那么回归方程的截距应该是接近于0。
我们看到,小公司和账面市值比比较低的组合截距为负,大公司和账面市值比较高的组合截距为正。
其他情况的话是接近于0的。
F值(表中没有给出)拒绝了原假设,同时这25个组合的R方的均值为0.93,也就是拟合效果比较的好,所以那个截距是显著不为零的。
尽管这个模型确实反映了组合平均收益的大部分风险,但是它的截距的绝对值的均值是0.093,也就是说有些截距它是显著不为0的。
II、LSV Deciles与前面的分组不同,LSV是根据BE/ME,E/P,C/P和五年的销售排名来分成10组。
计量经济学(重要名词解释)

——名词解释将因变量与一组解释变量和未观测到的扰动联系起来的方程,方程中未知的总体参数决定了各解释变量在其他条件不变下的效应。
与经济分析不同,在进行计量经济分析之前,要明确变量之间的函数形式。
经验分析(Empirical Analysis):在规范的计量分析中,用数据检验理论、估计关系式或评价政策有效性的研究。
确定遗漏变量、测量误差、联立性或其他某种模型误设所导致的可能偏误的过程线性概率模型(LPM)(Linear Probability Model, LPM):响应概率对参数为线性的二值响应模型。
没有一个模型可以通过对参数施加限制条件而被表示成另一个模型的特例的两个(或更多)模型。
有限分布滞后(FDL)模型(Finite Distributed Lag (FDL) Model):允许一个或多个解释变量对因变量有滞后效应的动态模型。
布罗施-戈弗雷检验(Breusch-Godfrey Test):渐近正确的AR(p)序列相关检验,以AR(1)最为流行;该检验考虑到滞后因变量和其他不是严格外生的回归元。
布罗施-帕甘检验(Breusch-Pagan Test)/(BP Test):将OLS 残差的平方对模型中的解释变量做回归的异方差性检验。
若一个模型正确,则另一个非嵌套模型得到的拟合值在该模型是不显著的。
因此,这是相对于非嵌套对立假设而对一个模型的检验。
在模型中包含对立模型的拟合值,并使用对拟合值的t 检验来实现。
回归误差设定检验(RESET)(Regression Specification Error Test, RESET):在多元回归模型中,检验函数形式的一般性方法。
它是对原OLS 估计拟合值的平方、三次方以及可能更高次幂的联合显著性的F 检验。
怀特检验(White Test):异方差的一种检验方法,涉及到做OLS 残差的平方对OLS 拟合值和拟合值的平方的回归。
这种检验方法的最一般的形式是,将OLS 残差的平方对解释变量、解释变量的平方和解释变量之间所有非多余的交互项进行回归。
第6章多因子定价模型
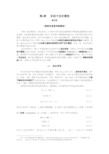
第6章 多因子定价模型黄万阳(根据肖俊喜译稿整理)在第5章结束部分,我们总结了CAPM 贝塔不能完全解释资产期望收益截面部分的经验证据。
该证据意味着可能需要1或多个其它因子刻画期望收益行为,自然考虑多因子定价模型。
理论争论也表明:由于仅在强假设下CAPM 才被逐期应用,需要多因子定价模型。
有两个主要的理论方法:罗斯(Ross,1976)提出的以套利为基础的套利定价理论(APT )。
默顿(Merton,1973a )提出的以均衡为基础的跨期资本资产定价模型。
在这一章,我们考虑多因子模型计量经济分析。
这章安排如下。
第6.1节简短地讨论多因子方法理论背景。
在第6.2节中我们考虑已知因子模型的估计与检验。
而在第6.3节中我们给出风险溢价(PREMIA )与期望收益的估计量。
既然因子不总是由理论提供,那么在第6.4节我们讨论构造因子的方法。
第6.5节给出了实证结论。
由于缺乏模型设定,离差总能被其余因子解释。
因此,这就产生了解释违背模型问题。
在第6.6节我们将讨论这个问题。
6.1 理论背景作为资本资产定价模型可供选择的模型,罗斯(Ross,1976)引入了套利定价理论。
APT 比CAPM 更一般,由于它考虑多个风险因子。
不像CAPM ,APT 也不要求识别市场投资组合。
然而,这种一般性不是无成本的。
在其一般形式中,APT 给出了资产期望收益与个数不确定的未识别因子之间近似关系。
在这种情况下,否定该理论是不可能的(除非套利机会存在)。
因此,模型可检验性依赖于额外假设的引入1。
套利定价理论假设市场是竞争的、无摩擦的;所考虑的资产收益生成过程为i i i i a R ε+'+=f b (6.1.1)0][=f i E ε (6.1.2)∞<≤=222][σσεi i E (6.1.3)其中i R 是资产i 的收益,i a 是因子模型截距,i b 是资产i 因子敏感度)1(⨯K 向量,f 是共同因子实现(realization ))1(⨯K 向量,i ε是扰动项。
idiosyncratic volatility
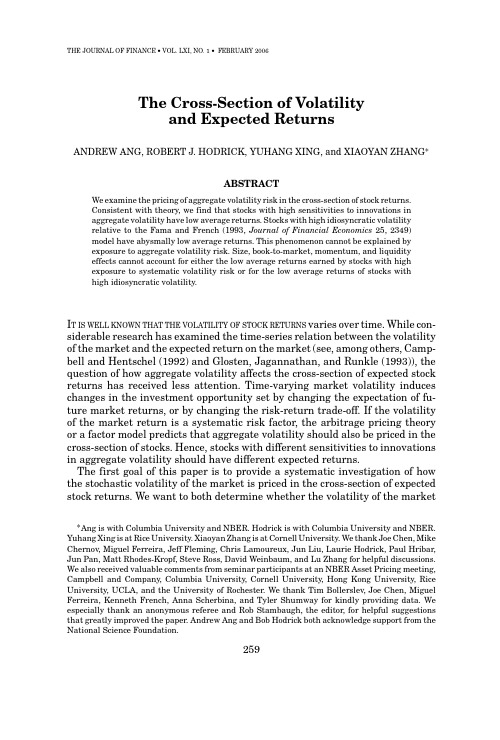
THE JOURNAL OF FINANCE•VOL.LXI,NO.1•FEBRUARY2006The Cross-Section of Volatilityand Expected ReturnsANDREW ANG,ROBERT J.HODRICK,YUHANG XING,and XIAOYAN ZHANG∗ABSTRACTWe examine the pricing of aggregate volatility risk in the cross-section of stock returns.Consistent with theory,we find that stocks with high sensitivities to innovations inaggregate volatility have low average returns.Stocks with high idiosyncratic volatilityrelative to the Fama and French(1993,Journal of Financial Economics25,2349)model have abysmally low average returns.This phenomenon cannot be explained byexposure to aggregate volatility risk.Size,book-to-market,momentum,and liquidityeffects cannot account for either the low average returns earned by stocks with highexposure to systematic volatility risk or for the low average returns of stocks withhigh idiosyncratic volatility.I T IS WELL KNOWN THAT THE VOLATILITY OF STOCK RETURNS varies over time.While con-siderable research has examined the time-series relation between the volatility of the market and the expected return on the market(see,among others,Camp-bell and Hentschel(1992)and Glosten,Jagannathan,and Runkle(1993)),the question of how aggregate volatility affects the cross-section of expected stock returns has received less attention.Time-varying market volatility induces changes in the investment opportunity set by changing the expectation of fu-ture market returns,or by changing the risk-return trade-off.If the volatility of the market return is a systematic risk factor,the arbitrage pricing theory or a factor model predicts that aggregate volatility should also be priced in the cross-section of stocks.Hence,stocks with different sensitivities to innovations in aggregate volatility should have different expected returns.The first goal of this paper is to provide a systematic investigation of how the stochastic volatility of the market is priced in the cross-section of expected stock returns.We want to both determine whether the volatility of the market ∗Ang is with Columbia University and NBER.Hodrick is with Columbia University and NBER. Yuhang Xing is at Rice University.Xiaoyan Zhang is at Cornell University.We thank Joe Chen,Mike Chernov,Miguel Ferreira,Jeff Fleming,Chris Lamoureux,Jun Liu,Laurie Hodrick,Paul Hribar, Jun Pan,Matt Rhodes-Kropf,Steve Ross,David Weinbaum,and Lu Zhang for helpful discussions. We also received valuable comments from seminar participants at an NBER Asset Pricing meeting, Campbell and Company,Columbia University,Cornell University,Hong Kong University,Rice University,UCLA,and the University of Rochester.We thank Tim Bollerslev,Joe Chen,Miguel Ferreira,Kenneth French,Anna Scherbina,and Tyler Shumway for kindly providing data.We especially thank an anonymous referee and Rob Stambaugh,the editor,for helpful suggestions that greatly improved the paper.Andrew Ang and Bob Hodrick both acknowledge support from the National Science Foundation.259260The Journal of Financeis a priced risk factor and estimate the price of aggregate volatility risk.Many option studies have estimated a negative price of risk for market volatility using options on an aggregate market index or options on individual stocks.1Using the cross-section of stock returns,rather than options on the market,allows us to create portfolios of stocks that have different sensitivities to innovations in market volatility.If the price of aggregate volatility risk is negative,stocks with large,positive sensitivities to volatility risk should have low average returns. Using the cross-section of stock returns also allows us to easily control for a battery of cross-sectional effects,such as the size and value factors of Fama and French(1993),the momentum effect of Jegadeesh and Titman(1993),and the effect of liquidity risk documented by P´astor and Stambaugh(2003).Option pricing studies do not control for these cross-sectional risk factors.We find that innovations in aggregate volatility carry a statistically signif-icant negative price of risk of approximately−1%per annum.Economic the-ory provides several reasons why the price of risk of innovations in market volatility should be negative.For example,Campbell(1993,1996)and Chen (2002)show that investors want to hedge against changes in market volatility, because increasing volatility represents a deterioration in investment opportu-nities.Risk-averse agents demand stocks that hedge against this risk.Periods of high volatility also tend to coincide with downward market movements(see French,Schwert,and Stambaugh(1987)and Campbell and Hentschel(1992)). As Bakshi and Kapadia(2003)comment,assets with high sensitivities to mar-ket volatility risk provide hedges against market downside risk.The higher demand for assets with high systematic volatility loadings increases their price and lowers their average return.Finally,stocks that do badly when volatility increases tend to have negatively skewed returns over intermediate horizons, while stocks that do well when volatility rises tend to have positively skewed re-turns.If investors have preferences over coskewness(see Harvey and Siddique (2000)),stocks that have high sensitivities to innovations in market volatility are attractive and have low returns.2The second goal of the paper is to examine the cross-sectional relationship be-tween idiosyncratic volatility and expected returns,where idiosyncratic volatil-ity is defined relative to the standard Fama and French(1993)model.3If the Fama–French model is correct,forming portfolios by sorting on idiosyncratic volatility will obviously provide no difference in average returns.Nevertheless, if the Fama–French model is false,sorting in this way potentially provides a set 1See,among others,Jackwerth and Rubinstein(1996),Bakshi,Cao and Chen(2000),Chernov and Ghysels(2000),Burashi and Jackwerth(2001),Coval and Shumway(2001),Benzoni(2002), Pan(2002),Bakshi and Kapadia(2003),Eraker,Johannes and Polson(2003),Jones(2003),and Carr and Wu(2003).2Bates(2001)and Vayanos(2004)provide recent structural models whose reduced form factor structures have a negative risk premium for volatility risk.3Recent studies examining total or idiosyncratic volatility focus on the average level of firm-level volatility.For example,Campbell et al.(2001)and Xu and Malkiel(2003)document that idiosyncratic volatility has increased over time.Brown and Ferreira(2003)and Goyal and Santa-Clara(2003)argue that idiosyncratic volatility has positive predictive power for excess market returns,but this is disputed by Bali et al.(2004).Cross-Section of Volatility and Expected Returns261 of assets that may have different exposures to aggregate volatility and hence different average returns.Our logic is the following.If aggregate volatility is a risk factor that is orthogonal to existing risk factors,the sensitivity of stocks to aggregate volatility times the movement in aggregate volatility will show up in the residuals of the Fama–French model.Firms with greater sensitivities to aggregate volatility should therefore have larger idiosyncratic volatilities rela-tive to the Fama–French model,everything else being equal.Differences in the volatilities of firms’true idiosyncratic errors,which are not priced,will make this relation noisy.We should be able to average out this noise by constructing portfolios of stocks to reveal that larger idiosyncratic volatilities relative to the Fama–French model correspond to greater sensitivities to movements in aggre-gate volatility and thus different average returns,if aggregate volatility risk is priced.While high exposure to aggregate volatility risk tends to produce low ex-pected returns,some economic theories suggest that idiosyncratic volatility should be positively related to expected returns.If investors demand compen-sation for not being able to diversify risk(see Malkiel and Xu(2002)and Jones and Rhodes-Kropf(2003)),then agents will demand a premium for holding stocks with high idiosyncratic volatility.Merton(1987)suggests that in an information-segmented market,firms with larger firm-specific variances re-quire higher average returns to compensate investors for holding imperfectly diversified portfolios.Some behavioral models,like Barberis and Huang(2001), also predict that higher idiosyncratic volatility stocks should earn higher ex-pected returns.Our results are directly opposite to these theories.We find that stocks with high idiosyncratic volatility have low average returns.There is a strongly significant difference of−1.06%per month between the average re-turns of the quintile portfolio with the highest idiosyncratic volatility stocks and the quintile portfolio with the lowest idiosyncratic volatility stocks.In contrast to our results,earlier researchers either find a significantly pos-itive relation between idiosyncratic volatility and average returns,or they fail to find any statistically significant relation between idiosyncratic volatility and average returns.For example,Lintner(1965)shows that idiosyncratic volatil-ity carries a positive coefficient in cross-sectional regressions.Lehmann(1990) also finds a statistically significant,positive coefficient on idiosyncratic volatil-ity over his full sample period.Similarly,Tinic and West(1986)and Malkiel and Xu(2002)unambiguously find that portfolios with higher idiosyncratic volatility have higher average returns,but they do not report any significance levels for their idiosyncratic volatility premiums.On the other hand,Longstaff (1989)finds that a cross-sectional regression coefficient on total variance for size-sorted portfolios carries an insignificant negative sign.The difference between our results and the results of past studies is that the past literature either does not examine idiosyncratic volatility at the firm level, or does not directly sort stocks into portfolios ranked on this measure of inter-est.For example,Tinic and West(1986)work only with20portfolios sorted on market beta,while Malkiel and Xu(2002)work only with100portfolios sorted on market beta and size.Malkiel and Xu(2002)only use the idiosyncratic262The Journal of Financevolatility of one of the100beta/size portfolios to which a stock belongs to proxy for that stock’s idiosyncratic risk and,thus,do not examine firm-level idiosyn-cratic volatility.Hence,by not directly computing differences in average returns between stocks with low and high idiosyncratic volatilities,previous studies miss the strong negative relation between idiosyncratic volatility and average returns that we find.The low average returns to stocks with high idiosyncratic volatilities could arise because stocks with high idiosyncratic volatilities may have high exposure to aggregate volatility risk,which lowers their average returns.We investigate this conjecture and find that this is not a complete explanation.Our idiosyn-cratic volatility results are also robust to controlling for value,size,liquidity, volume,dispersion of analysts’forecasts,and momentum effects.We find the effect robust to different formation periods for computing idiosyncratic volatil-ity and for different holding periods.The effect also persists in bull and bear markets,recessions and expansions,and volatile and stable periods.Hence,our results on idiosyncratic volatility represent a substantive puzzle.The rest of this paper is organized as follows.In Section I,we examine how aggregate volatility is priced in the cross-section of stock returns.Section II documents that firms with high idiosyncratic volatility have very low average returns.Finally,Section III concludes.I.Pricing Systematic Volatility in the Cross-SectionA.Theoretical MotivationWhen investment opportunities vary over time,the multifactor models of Merton(1973)and Ross(1976)show that risk premia are associated with the conditional covariances between asset returns and innovations in state vari-ables that describe the time-variation of the investment opportunities.Camp-bell’s(1993,1996)version of the Intertemporal Capital Asset Pricing Model (I-CAPM)shows that investors care about risks both from the market return and from changes in forecasts of future market returns.When the represen-tative agent is more risk averse than log utility,assets that covary positively with good news about future expected returns on the market have higher av-erage returns.These assets command a risk premium because they reduce a consumer’s ability to hedge against a deterioration in investment opportuni-ties.The intuition from Campbell’s model is that risk-averse investors want to hedge against changes in aggregate volatility because volatility positively affects future expected market returns,as in Merton(1973).However,in Campbell’s setup,there is no direct role for fluctuations in mar-ket volatility to affect the expected returns of assets because Campbell’s model is premised on homoskedasticity.Chen(2002)extends Campbell’s model to a heteroskedastic environment which allows for both time-varying covariances and stochastic market volatility.Chen shows that risk-averse investors also want to directly hedge against changes in future market volatility.In Chen’s model,an asset’s expected return depends on risk from the market return,Cross-Section of Volatility and Expected Returns263 changes in forecasts of future market returns,and changes in forecasts of fu-ture market volatilities.For an investor more risk averse than log utility,Chen shows that an asset that has a positive covariance between its return and a variable that positively forecasts future market volatilities causes that asset to have a lower expected return.This effect arises because risk-averse investors reduce current consumption to increase precautionary savings in the presence of increased uncertainty about market returns.Motivated by these multifactor models,we study how exposure to market volatility risk is priced in the cross-section of stock returns.A true conditional multifactor representation of expected returns in the cross-section would take the following form:r i t+1=a i t+βi m,tr mt+1−γm,t+βi v,t(v t+1−γv,t)+Kk=1βi k,t(f k,t+1−γk,t),(1)where r it+1is the excess return on stock i,βi m,t is the loading on the excess mar-ket return,βi v,t is the asset’s sensitivity to volatility risk,and theβik,t coefficientsfor k=1,...,K represent loadings on other risk factors.In the full conditional setting in equation(1),factor loadings,conditional means of factors,and fac-tor premiums potentially vary over time.The model in equation(1)is writtenin terms of factor innovations,so r mt+1−γm,t represents the innovation in themarket return,v t+1−γv,t represents the innovation in the factor reflecting ag-gregate volatility risk,and innovations to the other factors are represented by f k,t+1−γk,t.The conditional mean of the market and aggregate volatility are denoted byγm,t andγv,t,respectively,while the conditional means of the other factors are denoted byγk,t.In equilibrium,the conditional mean of stock i is given bya i t=E tr it+1=βi m,tλm,t+βi v,tλv,t+Kk=1βi k,tλk,t,(2)whereλm,t is the price of risk of the market factor,λv,t is the price of aggre-gate volatility risk,and theλk,t are the prices of risk of the other factors.Note that only if a factor is traded is the conditional mean of a factor equal to its conditional price of risk.The main prediction from the factor model setting of equation(1)that we examine is that stocks with different loadings on aggregate volatility risk have different average returns.4However,the true model in equation(1)is infeasible 4While an I-CAPM implies joint time-series as well as cross-sectional predictability,we do not examine time-series predictability of asset returns by systematic volatility.Time-varying volatility risk generates intertemporal hedging demands in partial equilibrium asset allocation problems.In a partial equilibrium setting,Liu(2001)and Chacko and Viceira(2003)examine how volatility risk affects the portfolio allocation of stocks and risk-free assets,while Liu and Pan(2003)show how investors can optimally exploit the variation in volatility with options.Guo and Whitelaw(2003) examine the intertemporal components of time-varying systematic volatility in a Campbell(1993, 1996)equilibrium I-CAPM.264The Journal of Financeto examine because the true set of factors is unknown and the true conditionalfactor loadings are unobservable.Hence,we do not attempt to directly use equa-tion(1)in our empirical work.Instead,we simplify the full model of equation(1),which we now detail.B.The Empirical FrameworkTo investigate how aggregate volatility risk is priced in the cross-section ofequity returns we make the following simplifying assumptions to the full spec-ification in equation(1).First,we use observable proxies for the market factorand the factor representing aggregate volatility risk.We use the CRSP value-weighted market index to proxy for the market factor.To proxy innovationsin aggregate volatility,(v t+1−γv,t),we use changes in the VIX index from the Chicago Board Options Exchange(CBOE).5Second,we reduce the number offactors in equation(1)to just the market factor and the proxy for aggregatevolatility risk.Finally,to capture the conditional nature of the true model,weuse short intervals—1month of daily data—to take into account possible timevariation of the factor loadings.We discuss each of these simplifications in turn.B.1.Innovations in the VIX IndexThe VIX index is constructed so that it represents the implied volatility of asynthetic at-the-money option contract on the S&P100index that has a matu-rity of1month.It is constructed from eight S&P100index puts and calls andtakes into account the American features of the option contracts,discrete cashdividends,and microstructure frictions such as bid–ask spreads(see Whaley(2000)for further details).6Figure1plots the VIX index from January1986to December2000.The mean level of the daily VIX series is20.5%,and itsstandard deviation is7.85%.Because the VIX index is highly serially correlated with a first-order au-tocorrelation of0.94,we measure daily innovations in aggregate volatility byusing daily changes in VIX,which we denote as VIX.Daily first differences inVIX have an effective mean of zero(less than0.0001),a standard deviation of5In previous versions of this paper,we also consider:Sample volatility,following French et al.(1987);a range-based estimate,following Alizadeh,Brandt,and Diebold(2002);and a high-frequency estimator of volatility from Andersen,Bollerslev,and Diebold(2003).Using these mea-sures to proxy for innovations in aggregate volatility produces little spread in cross-sectional av-erage returns.These tables are available upon request.6On September22,2003,the CBOE implemented a new formula and methodology to constructits volatility index.The new index is based on the S&P500(rather than the S&P100)and takes into account a broader range of strike prices rather than using only at-the-money option contracts. The CBOE now uses VIX to refer to this new index.We use the old index(denoted by the ticker VXO).We do not use the new index because it has been constructed by backfilling only to1990, whereas the VXO is available in real time from1986.The CBOE continues to make both volatility indices available.The correlation between the new and the old CBOE volatility series is98%from 1990to2000,but the series that we use has a slightly broader range than the new CBOE volatility series.Cross-Section of Volatility and Expected Returns26519861988199019921994199619982000 Figure1.Plot of VIX.The figure shows the VIX index plotted at a daily frequency.The sample period is January1986to December2000.2.65%,and negligible serial correlation(the first-order autocorrelation of VIX is−0.0001).As part of our robustness checks in Section I.C,we also measure innovations in VIX by specifying a stationary time-series model for the con-ditional mean of VIX and find our results to be similar to those using simple first differences.While VIX appears to be an ideal proxy for innovations in volatility risk because the VIX index is representative of traded option secu-rities whose prices directly reflect volatility risk,there are two main caveats with respect to using VIX to represent observable market volatility.The first concern is that the VIX index is the implied volatility from the Black–Scholes(1973)model,and we know that the Black–Scholes model is an approximation.If the true stochastic environment is characterized by stochas-tic volatility and jumps, VIX will reflect total quadratic variation in both diffusion and jump components(see,for example,Pan(2002)).Although Bates (2000)argues that implied volatilities computed taking into account jump risk are very close to original Black–Scholes implied volatilities,jump risk may be priced differently from volatility risk.Our analysis does not separate jump risk from diffusion risk,so our aggregate volatility risk may include jump risk components.266The Journal of FinanceA more serious reservation about the VIX index is that VIX combines both stochastic volatility and the stochastic volatility risk premium.Only if the risk premium is zero or constant would VIX be a pure proxy for the innovation in aggregate volatility.Decomposing VIX into the true innovation in volatility and the volatility risk premium can only be done by writing down a formal model.The form of the risk premium depends on the parameterization of the price of volatility risk,the number of factors,and the evolution of those factors. Each different model specification implies a different risk premium.For exam-ple,many stochastic volatility option pricing models assume that the volatility risk premium can be parameterized as a linear function of volatility(see,for example,Chernov and Ghysels(2000),Benzoni(2002),and Jones(2003)).This may or may not be a good approximation to the true price of risk.Rather than imposing a structural form,we use an unadulterated VIX series.An advan-tage of this approach is that our analysis is simple to replicate.B.2.The Pre-Formation RegressionOur goal is to test whether stocks with different sensitivities to aggregate volatility innovations(proxied by VIX)have different average returns.To measure the sensitivity to aggregate volatility innovations,we reduce the num-ber of factors in the full specification in equation(1)to two,namely,the mar-ket factor and VIX.A two-factor pricing kernel with the market return and stochastic volatility as factors is also the standard setup commonly assumed by many stochastic option pricing studies(see,for example,Heston(1993)).Hence, the empirical model that we examine isr i t=β0+βi MKT MKT t+βi VIX VIX t+εi t,(3) where MKT is the market excess return, VIX is the instrument we use forinnovations in the aggregate volatility factor,andβiMKT andβi VIX are loadingson market risk and aggregate volatility risk,respectively.Previous empirical studies suggest that there are other cross-sectional factors that have explanatory power for the cross-section of returns,such as the size and value factors of the Fama and French(1993)three-factor model(hereafter FF-3).We do not directly model these effects in equation(3),because controlling for other factors in constructing portfolios based on equation(3)may add a lot of noise.Although we keep the number of regressors in our pre-formation portfolio regressions to a minimum,we are careful to ensure that we control for the FF-3factors and other cross-sectional factors in assessing how volatility risk is priced using post-formation regression tests.We construct a set of assets that are sufficiently disperse in exposure to aggregate volatility innovations by sorting firms on VIX loadings over the past month using the regression(3)with daily data.We run the regression for all stocks on AMEX,NASDAQ,and the NYSE,with more than17daily observations.In a setting in which coefficients potentially vary over time,a 1-month window with daily data is a natural compromise between estimatingCross-Section of Volatility and Expected Returns267 coefficients with a reasonable degree of precision and pinning down conditionalcoefficients in an environment with time-varying factor loadings.P´astor andStambaugh(2003),among others,also use daily data with a1-month window insimilar settings.At the end of each month,we sort stocks into quintiles,basedon the value of the realizedβ VIX coefficients over the past month.Firms inquintile1have the lowest coefficients,while firms in quintile5have the highest β VIX loadings.Within each quintile portfolio,we value weight the stocks.We link the returns across time to form one series of post-ranking returns for eachquintile portfolio.Table I reports various summary statistics for quintile portfolios sorted bypastβ VIX over the previous month using equation(3).The first two columnsreport the mean and standard deviation of monthly total,not excess,simplereturns.In the first column under the heading“Factor Loadings,”we report thepre-formationβ VIX coefficients,which are computed at the beginning of eachmonth for each portfolio and are value weighted.The column reports the time-series average of the pre-formationβ VIX loadings across the whole sample.By construction,since the portfolios are formed by ranking on pastβ VIX,thepre-formationβ VIX loadings monotonically increase from−2.09for portfolio1to2.18for portfolio5.The columns labeled“CAPM Alpha”and“FF-3Alpha”report the time-seriesalphas of these portfolios relative to the CAPM and to the FF-3model,respec-tively.Consistent with the negative price of systematic volatility risk found bythe option pricing studies,we see lower average raw returns,CAPM alphas,and FF-3alphas with higher past loadings ofβ VIX.All the differences be-tween quintile portfolios5and1are significant at the1%level,and a jointtest for the alphas equal to zero rejects at the5%level for both the CAPM andthe FF-3model.In particular,the5-1spread in average returns between thequintile portfolios with the highest and lowestβ VIX coefficients is−1.04%permonth.Controlling for the MKT factor exacerbates the5-1spread to−1.15%per month,while controlling for the FF-3model decreases the5-1spread to −0.83%per month.B.3.Requirements for a Factor Risk ExplanationWhile the differences in average returns and alphas corresponding to dif-ferentβ VIX loadings are very impressive,we cannot yet claim that these dif-ferences are due to systematic volatility risk.We examine the premium for aggregate volatility within the framework of an unconditional factor model. There are two requirements that must hold in order to make a case for a fac-tor risk-based explanation.First,a factor model implies that there should be contemporaneous patterns between factor loadings and average returns.For example,in a standard CAPM,stocks that covary strongly with the market factor should,on average,earn high returns over the same period.To test a fac-tor model,Black,Jensen,and Scholes(1972),Fama and French(1992,1993), Jagannathan and Wang(1996),and P´astor and Stambaugh(2003),among others,all form portfolios using various pre-formation criteria,but examine268The Journal of FinanceT a b l e IP o r t f o l i o s S o r t e d b y E x p o s u r e t o A g g r e g a t e V o l a t i l i t y S h o c k sW e f o r m v a l u e -w e i g h t e d q u i n t i l e p o r t f o l i o s e v e r y m o n t h b y r e g r e s s i n g e x c e s s i n d i v i d u a l s t o c k r e t u r n s o n V I X ,c o n t r o l l i n g f o r t h e M K T f a c t o r a s i n e q u a t i o n (3),u s i n g d a i l y d a t a o v e r t h e p r e v i o u s m o n t h .S t o c k s a r e s o r t e d i n t o q u i n t i l e s b a s e d o n t h e c o e f f i c i e n t β V I X f r o m l o w e s t (q u i n t i l e 1)t o h i g h e s t (q u i n t i l e 5).T h e s t a t i s t i c s i n t h e c o l u m n s l a b e l e d M e a n a n d S t d .D e v .a r e m e a s u r e d i n m o n t h l y p e r c e n t a g e t e r m s a n d a p p l y t o t o t a l ,n o t e x c e s s ,s i m p l e r e t u r n s .S i z e r e p o r t s t h e a v e r a g e l o g m a r k e t c a p i t a l i z a t i o n f o r f i r m s w i t h i n t h e p o r t f o l i o a n d B /M r e p o r t s t h e a v e r a g e b o o k -t o -m a r k e t r a t i o .T h e r o w “5-1”r e f e r s t o t h e d i f f e r e n c e i n m o n t h l y r e t u r n s b e t w e e n p o r t f o l i o 5a n d p o r t f o l i o 1.T h e A l p h a c o l u m n s r e p o r t J e n s e n ’s a l p h a w i t h r e s p e c t t o t h e C A P M o r t h e F a m a –F r e n c h (1993)t h r e e -f a c t o r m o d e l .T h e p r e -f o r m a t i o n b e t a s r e f e r t o t h e v a l u e -w e i g h t e d β V I X o r βF V I X w i t h i n e a c h q u i n t i l e p o r t f o l i o a t t h e s t a r t o f t h e m o n t h .W e r e p o r t t h e p r e -f o r m a t i o n β V I X a n d βF V I X a v e r a g e d a c r o s s t h e w h o l e s a m p l e .T h e s e c o n d t o l a s t c o l u m n r e p o r t s t h e β V I X l o a d i n g c o m p u t e d o v e r t h e n e x t m o n t h w i t h d a i l y d a t a .T h e c o l u m n r e p o r t s t h e n e x t m o n t h β V I X l o a d i n g s a v e r a g e d a c r o s s m o n t h s .T h e l a s t c o l u m n r e p o r t s e x p o s t βF V I X f a c t o r l o a d i n g s o v e r t h e w h o l e s a m p l e ,w h e r e F V I X i s t h e f a c t o r m i m i c k i n g a g g r e g a t e v o l a t i l i t y r i s k .T o c o r r e s p o n d w i t h t h e F a m a –F r e n c h a l p h a s ,w e c o m p u t e t h e e x p o s t b e t a s b y r u n n i n g a f o u r -f a c t o r r e g r e s s i o n w i t h t h e t h r e e F a m a –F r e n c h f a c t o r s t o g e t h e r w i t h t h e F V I X f a c t o r t h a t m i m i c s a g g r e g a t e v o l a t i l i t y r i s k ,f o l l o w i n g t h e r e g r e s s i o n i n e q u a t i o n (6).T h e r o w l a b e l e d “J o i n t t e s t p -v a l u e ”r e p o r t s a G i b b o n s ,R o s s a n d S h a n k e n (1989)t e s t f o r t h e a l p h a s e q u a l t o z e r o ,a n d a r o b u s t j o i n t t e s t t h a t t h e f a c t o r l o a d i n g s a r e e q u a l t o z e r o .R o b u s t N e w e y –W e s t (1987)t -s t a t i s t i c s a r e r e p o r t e d i n s q u a r e b r a c k e t s .T h e s a m p l e p e r i o d i s f r o m J a n u a r y 1986t o D e c e m b e r 2000.F a c t o r L o a d i n g sN e x t M o n t h F u l l S a m p l e S t d .%M k t C A P M F F -3P r e -F o r m a t i o n P r e -F o r m a t i o n P o s t -F o r m a t i o n P o s t -F o r m a t i o n R a n kM e a nD e v .S h a r e S i z e B /M A l p h a A l p h aβ V I X βF V I Xβ V I XβF V I X11.645.539.4%3.700.890.270.30−2.09−2.00−0.033−5.06[1.66][1.77][−4.06]21.394.4328.7%4.770.730.180.09−0.46−0.42−0.014−2.72[1.82][1.18][−2.64]31.364.4030.4%4.770.760.130.080.030.080.005−1.55[1.32][1.00][−2.86]41.214.7924.0%4.760.73−0.08−0.060.540.620.0153.62[−0.87][−0.65][4.53]50.606.557.4%3.730.89−0.88−0.532.182.310.0188.07[−3.42][−2.88][5.32]5-1−1.04−1.15−0.83[−3.90][−3.54][−2.93]J o i n t t e s t p -v a l u e0.010.030.00。
海外文献原文-推荐参考文献列表

海外文献推荐-第一期参考文献:[1] I-Cheng Yeh, Che-Hui Lien, Tao-Ming Ting, 2015, Building multi-factor stock selection models using balanced split regression trees with sorting normalisation and hybrid variables, Foresight and Innovation Policy, V ol. 10, No. 1, 48-74[2] Eugene F.Fama, KennethR.French, 2015, A Five-factor Asset Pricing Model, Journal of Financial Economics 116, 1-22[3] Achim BACKHAUS, Aliya ZHAKANOV A ISIKSAL, 2016, The Impact of Momentum Factors on Multi Asset Portfolio, Romanian Journal of Economic Forecasting XIX (4), 146-169[4] Francisco Barillas, Jay Shanken, 2016, Which Alpha? Review of Financial Studies海外文献推荐-第二期参考文献:[1] PRA VEEN KUMAR, DONGMEI LI, 2016, Capital Investment, Innovative Capacity, and Stock Returns, The Journal of Finance, VOL. LXXI, NO. 5, 2059-2094[2] Houda Ben Mabrouk, Abdelfettah Bouri, 2013, New insight on the CAPM: a copula-based approach Tunisian and international evidence, Accounting and Finance, Vol. 4, No. 1, 35-62 [3] FERHAT AKBAS, 2016, The Calm before the Storm, The Journal of Finance, VOL. LXXI, NO. 1,225-266海外文献推荐-第三期参考文献:[1] Yufeng Han, Guofu Zhou, Yingzi Zhu, 2016, A trend factor: Any economic gains from using information over investment horizons? Journal of Financial Economics 122, 352-375[2] Andrea Frazzini, LasseHeje Pedersen, 2014, Betting against beta, Journal of Financial Economics 111, 1-25[3] Doron Avramov, Si Cheng, and Allaudeen Hameed, 2016, Time-Varying Liquidity and Momentum Profits, JOURNAL OF FINANCIAL AND QUANTITATIVE ANAL YSI, Vol. 51, No. 6, 1897-1923[4] Nicholas Barberis, Abhiroop Mukherjee, Baolian Wang, 2014, Prospect Theory and Stock Returns: An Empirical Test, Review of Financial Studies海外文献推荐-第四期参考文献:[1] Brad M. Barber, Xing Huang, Terrance Odean, 2014, Which risk factors matter to investors? Evidence from mutual fund flows, Review of Financial Studies[2] MICHAEL J. COOPER, HUSEYIN GULEN, & MICHAEL J. SCHILL. (2008). Asset growth and the cross‐section of stock returns. Social Science Electronic Publishing, 63(4), 1609–1651.[3]Bollerslev, T., Li, S. Z., & Todorov, V. (2016). Roughing up beta: continuous versus discontinuous betas and the cross section of expected stock returns. Journal of Financial Economics, 120(3), 464-490.[4]Baker, M., Wurgler, J., & Yuan, Y. (2012). Global, local, and contagious investor sentiment ⋆. Journal of Financial Economics, 104(2), 272-287.海外文献推荐-第五期参考文献:[1] Nicole Choi, Mark Fedenia, Tatyana Sokolyk, 2017, Portfolio Concentration and Performance of Institutional Investors Worldwide, Journal of Financial Economics[2]Cronqvist, H., Siegel, S., & Yu, F. (2015). Value versus growth investing: why do differentinvestors have different styles? ☆. Journal of Financial Economics, 117(2), 333-349.[3]Rapach, D. E., Ringgenberg, M. C., & Zhou, G. (2016). Short interest and aggregate stock returns . Journal of Financial Economics, 121(1), 46-65.[4]Novy-Marx, R. (2013). The other side of value: the gross profitability premium ☆. Journal of Financial Economics, 108(1), 1-28.海外文献推荐-第六期参考文献:[1] Suk Joon Byun, Sonya S. Limy, and Sang Hyun Yun, 2012, Continuing Overreaction and Stock Return Predictability, Journal of Financial and Quantitative Analysis[2]Eugene F. Fama, & Kenneth R. French. (2016). International tests of a five-factor asset pricing model. Journal of Financial Economics, 123.[3]Keloharju, M., Linnainmaa, J. T., & Nyberg, P. (2016). Return seasonalities. Journal of Finance, 71(4), n/a-n/a.[4]Seasholes, M. S., & Wu, G. (2007). Predictable behavior, profits, and attention. Journal of Empirical Finance, 14(5), 590-610.[5]PA VEL SAVOR, & MUNGO WILSON. (2016). Earnings announcements and systematic risk. The Journal of Finance, 71(1).海外文献推荐-第七期参考文献:[1] Cary Frydman and Colin Camerer, 2016, Neural Evidence of Regret and its Implications for Investor Behavior, Review of Financial Studies 29, 3108-3139[2] Haghani, V., & Dewey, R. (2016). A case study for using value and momentum at the asset class level. Journal of Portfolio Management, 42(3), 101-113.[3] Tarun, C., Amit, G., & Narasimhan, J. (2011). Buyers versus sellers: who initiates trades, and when?. Journal of Financial & Quantitative Analysis, 51(5), 1467-1490.[4] Hartzmark, M. S. (2015). The worst, the best, ignoring all the rest: the rank effect and trading behavior. Review of Financial Studies, 28(4), 1024.[5] Daniel, K., & Moskowitz, T. J. (2016). Momentum crashes. Journal of Financial Economics, 122(2), 221-247.海外文献推荐-第八期参考文献:[1]Hua, R., Kantsyrev, D., & Qian, E. (2012). Factor-timing model.Journal of Portfolio Management,39(1), 75-87.[2]Leshem, R., Goldberg, L. R., & Cummings, A. (2015). Optimizing value.Journal of Portfolio Management,42(2).[3]Chemmanur, Thomas J., Gang Hu and Jiekun Huang, 2015, Institutional Investors and the Information Production Theory of Stock Splits,Journal of Financial and Quantitative Analysis50(3), 413–445.海外文献推荐-第九期参考文献:[1]Penaranda, F. (2016). Understanding portfolio efficiency with conditioning information. Economics Working Papers, 51(3), 985-1011.[2]Cederburg, S., & O'Doherty, M. S. (2016). Does it pay to bet against beta? on the conditional performance of the beta anomaly. Journal of Finance, 71(2), 737-774.[3]Lindsey, R. R., & Weisman, A. B. (2016). Forced liquidations, fire sales, and the cost of illiquidity. Journal of Portfolio Management, 20(1), 45-57.海外文献推荐-第十期参考文献:[1] Easley, D., Hvidkjaer, S., & O'Hara, M. (2010). Factoring information into returns. Journal of Financial & Quantitative Analysis, 45(2), 293-309.[2]Babenko, I., Boguth, O., & Tserlukevich, Y. (2016). Idiosyncratic cash flows and systematic risk. Journal of Finance, 71(1).[3]Chow, V., & Lai, C. W. (2015). Conditional sharpe ratios. Finance Research Letters, 12, 117-133.海外文献推荐-第十一期参考文献:[1] Mladina, P. (2017). Illuminating hedge fund returns to improve portfolio construction. Social Science Electronic Publishing, 41(3), 127-139.[2] Choi, N., Fedenia, M., Skiba, H., & Sokolyk, T. (2016). Portfolio concentration and performance of institutional investors worldwide. Journal of Financial Economics.[3] Martijn Boons, 2016, State variables, macroeconomic activity, and the cross section of individual stocks, Journal of Financial Economics 119, 489-511海外文献推荐-第十二期参考文献:[1] Blanchett, D., & Ratner, H. (2015). Building efficient income portfolios. Journal of Portfolio Management, 41(3), 117-125.[2] Özde Öztekin. (2015). Capital structure decisions around the world: which factors are reliably important?. Journal of Financial & Quantitative Analysis, 50(3).[3] 2015, Does the number of stocks in a portfolio influence performance? Investment Sights海外文献推荐-第十三期参考文献:[1]Glushkov, D., & Statman, M. (2016). Classifying and measuring the performance of socially responsible mutual funds.Social Science Electronic Publishing,42(2), 140-151.[2]KLAUS ADAM, ALBERT MARCET, & JUAN PABLO NICOLINI. (2016). Stock market volatility and learning.The Journal of Finance,71(1), 419–438.[3]Miller, K. L., Li, H., Zhou, T. G., & Giamouridis, D. (2012). A risk-oriented model for factor timing decisions.Journal of Portfolio Management,41(3), 46-58.海外文献推荐-第十四期参考文献:[1]Feldman, T., Jung, A., & Klein, J. (2015). Buy and hold versus timing strategies: the winner is ….Journal of Portfolio Management,42(1), 110-118.[2]Eric H Sorensen, Nicholas F Alonso. The Resale Value of Risk-Parity Equity Portfolios[J]. Journal of Portfolio Management, 2015, 41(2):23-32.海外文献推荐-第十五期参考文献:[1]Barroso, P., & Santa-Clara, P. (2015). Momentum has its moments ☆.Journal of Financial Economics,116(1), 111-120.[2]Bender, J., & Nielsen, F. (2015). Earnings quality revisited.Social Science Electronic Publishing,39(4), 69-79.海外文献推荐-第十六期参考文献:[1]Greenberg, D., Abhilash, B., & Ang, A. (2016). Factors to assets: mapping factor exposures to asset allocations. Journal of Portfolio Management, 42(5), 18-27.[2]Goyal, A., Ilmanen, A., & Kabiller, D. (2015). Bad habits and good practices. Journal of Portfolio Management, 41(4), 97-107.海外文献推荐-第十七期参考文献:[1]Vermorken, M. A., Medda, F. R., & Schröder, T. (2012). The diversification delta: a higher-moment measure for portfolio diversification. Journal of Portfolio Management, 39(1), 67-74.[2]Asl, F. M., & Etula, E. (2012). Advancing strategic asset allocation in a multi-factor world.Journal of Portfolio Management,39(1), 59-66.海外文献推荐-第十八期参考文献:[1]Chakrabarty, B., Moulton, P. C., & Trzcinka, C. (2016). The performance of short-term institutional trades. Social Science Electronic Publishing, 1-26.[2]Stubbs, R. A., & Jeet, V. (2015). Adjusted Factor-Based Performance Attribution. USXX.海外文献推荐-第十九期参考文献:[1]Copeland, M., & Copeland, T. (2016). Vix versus size. Journal of Portfolio Management, 42(3), 76-83.[2]Kritzman, M., & Turkington, D. (2016). Stability-adjusted portfolios. Journal of Portfolio Management, 42(5), 113-122.海外文献推荐-第二十期参考文献:[1]Benos, E., Brugler, J., Hjalmarsson, E., & Zikes, F. (2016). Interactions among high-frequency traders. Journal of Financial & Quantitative Analysis, 52, 1-28.[2]Richardson, S., Sloan, R., & You, H. (2011). What makes stock prices move? fundamentals vs. investor recognition. Financial Analysts Journal, 68(2), 30-50.海外文献推荐-第二十一期参考文献:[1]Bogousslavsky, V. (2016). Infrequent rebalancing, return autocorrelation, and seasonality. Journal of Finance, 71(6), 2967-3006.[2]Marcos, L. D. P. (2015). The future of empirical finance. Journal of Portfolio Management, 41(4), 140-144.海外文献推荐-第二十二期参考文献:[1] Fabian, H., & Marcel, P. (2016). Estimating beta. Journal of Financial & Quantitative Analysis, 51(4), 1437-1466.[2] Christopher Cheung, George Hoguet, & Sunny Ng. (2014). Value, size, momentum, dividend yield, and volatility in china’s a-share market. Journal of Portfolio Management, 41(5), 57-70.海外文献推荐-第二十三期参考文献:[1]Mclean, R. D., & Zhao, M. (2014). The business cycle, investor sentiment, and costly external finance.Journal of Finance, 69(3), 1377–1409.[2]Kaniel, R., & Parham, R. (2017). The impact of media attention on consumer and mutual fund investment decisions. Journal of Financial Economics, 123, págs. 337-356海外文献推荐-第二十四期参考文献:[1]Chang, X., Chen, Y., & Zolotoy, L. (2017). Stock liquidity and stock price crash risk. Journal of Financial & Quantitative Analysis.[2]Bisetti, E., Favero, C. A., Nocera, G., & Tebaldi, C. (2013). A multivariate model of strategic asset allocation with longevity risk. Ssrn Electronic Journal.海外文献推荐-第二十五期参考文献:[1] Lou, X., & Shu, T. (2013). Price impact or trading volume: why is the amihud (2002) measure priced?. Social Science Electronic Publishing.[2]Lins, K. V., Servaes, H., & Tamayo, A. (2017). Social capital, trust, and firm performance: the value of corporate social responsibility during the financial crisis. Journal of Finance, 72.海外文献推荐-第二十六期参考文献:[1] Golez, B., & Koudijs, P. (2014). Four centuries of return predictability. Social Science Electronic Publishing.[2]Ledoit, O., and Wolf, M. (2017). Nonlinear shrinkage of the covariance matrix for portfolio selection: Markowitz meets Goldilocks. The Review of Financial Studies, 30(12), 4349-4388.海外文献推荐-第二十七期参考文献:[1]Ray Dalio, Bob Prince, Greg Jensen (2015), our thoughts about risk parity and all weather, Bridgewater Associates, LP[2]Thierry, R. and Guillaume, W. (2013). Risk Parity Portfolios with Risk Factors. MPRA Paper No. 44017.海外文献推荐-第二十八期参考文献:[1] Golubov, A., & Konstantinidi, T. (2015). Where is the risk in value? evidence from a market-to-book decomposition. Social Science Electronic Publishing.[2] Moreira, A., and Muir, T. (2017). Volatility‐Managed Portfolios. Journal of Finance, 72(4).海外文献推荐-第二十九期参考文献:[1]Wahalab S. Style investing, comovement and return predictability ☆[J]. Journal of Financial Economics, 2013, 107(1).[2]Pástor Ľ, Stambaugh R F, Taylor L A. Do funds make more when they trade more?[J]. The Journal of Finance, 2017, 72(4): 1483-1528.海外文献推荐-第三十期参考文献:[1] K Hou, C Xue, L Zhang, Digesting Anomalies: An Investment Approach, NBER Working Papers, 2015, 28(3)[2]Berk, J. B., & Binsbergen, J. H. V. (2013). Measuring skill in the mutual fund industry. Journal of Financial Economics, 118(1), 1-20.海外文献推荐-第三十一期参考文献:[1]Klein, Rudolf F. and V. K. Chow. "Orthogonalized factors and systematic risk decomposition." Quarterly Review of Economics & Finance 53.2(2013):175-187.[2]Sorensen E H, Hua R, Qian E E. Contextual Fundamentals, Models, and Active Management[J]. Journal of Portfolio Management 32.1(2005):23-36.海外文献推荐-第三十二期参考文献:[1] Hong, H. Torous, W. & Valkanov, R. (2007). Do industries lead stock markets? Journal of Financial Economics,83 (2), 367-396.[2]Dhillon, J. Ilmanen, A. & Liew, J. (2016). Balancing on the life cycle: target-date funds need better diversification. Journal of Portfolio Management, 42(4), 12-27.海外文献推荐-第三十三期参考文献:[1]Kenneth Froot and Melvyn Teo, Style Investing and Institutional Investors, JOURNAL OF FINANCIAL AND QUANTITATIVE ANALYSIS V ol. 43, No. 4, Dec. 2008, pp. 883–906.[2]Israel R, Palhares D, Richardson S A. Common factors in corporate bond returns[J]. Social Science Electronic Publishing, 2015.海外文献推荐-第三十四期参考文献:[1] DM Smith, N Wang, Y Wang, EJ Zychowicz, Sentiment and the Effectiveness of Technical Analysis: Evidence from the Hedge Fund Industry,Journal of Financial & Quantitative Analysis, 2016 , 51 (6) :1991-2013[2]Ronen Israel, Sarah Jiang, and Adrienne Ross (2018). Craftsmanship Alpha: An Application to Style Investing. Journal of Portfolio Management.海外文献推荐-第三十五期参考文献:[1] Huang J. The customer knows best: The investment value of consumer opinions [J]. Journal of Financial Economics, 2018.[2]Alberg J, Lipton Z C. Improving Factor-Based Quantitative Investing by Forecasting Company Fundamentals, Time Series Workshop at the 31st Conference on Neural Information Processing Systems (NIPS 2017). 2017.海外文献推荐-第三十六期参考文献:[1] Davis, J. H., Aliagadiaz, R. A., Ahluwalia, H., & Tolani, R. (2017). Improving U.S. stock return forecasts: a 'fair-value' cape approach.Social Science Electronic Publishing.海外文献推荐-第三十七期参考文献:[1] Fama, E. F., & French, K. R.(2018). Choosing factors. Journal of Financial Economics, 128: 234–252.[2] Bruder, Benjamin, Culerier, Leo, & Roncalli, Thierry. (2013). How to design target-date funds?. Ssrn Electronic Journal.海外文献推荐-第三十八期参考文献:[1] David Aboody, Omri Even-Tov, Reuven Lehavy, Brett Trueman. (2018). Overnight Returns and Firm-Specific Investor Sentiment. Journal of Financial and Quantitative Analysis.[2] Arnott R, Beck N, Kalesnik V, et al. How Can 'Smart Beta' Go Horribly Wrong?[J]. Social Science Electronic Publishing, 2017.海外文献推荐-第三十九期参考文献:[1] CS Asness, A Frazzini, LH PedersenDM, 2013,Quality Minus Junk,Social Science Electronic Publishing[2] Stein, M, & Rachev, S. T. (2011). Style-neutral funds of funds: diversification or deadweight? Journal of Asset Management, 11(6), 417-434.海外文献推荐-第四十期参考文献:[1] Li Y, Sun Q, Tian S. The impact of IPO approval on the price of existing stocks: Evidence from China[J]. Journal of Corporate Finance, 2018.[2] Jennifer Bender,Xiaole Sun,Ric Thomas,V olodymyr Zdorovtsov, The Journal of Portfolio Management , 2018 , 44 (4) :79-92海外文献推荐-第四十一期参考文献:[1] Yi Fang & Haiping Wang (2015) Fund manager characteristics and performance, Investment Analysts Journal, 44:1, 102-116.[2] Roni Israelov, Harsha Tummala. An Alternative Option to Portfolio Rebalancing. The Journal of Derivatives Spring 2018, 25 (3) 7-32海外文献推荐-第四十二期参考文献:[1] Robert Capone, Adam Akant, (2016), Trend Following Strategies in Target-Date Funds, AQR Capital Management.[2] Loh, R. K., & Stulz, R. M. (2018). Is sell‐side research more valuable in bad times?. Journal of Finance, 73(3): 959-1013.海外文献推荐-第四十三期参考文献:[1] Asness, C. S., Frazzini, A., Israel, R., & Moskowitz, T. J. (2015). Fact, fiction, and value investing. Final version published in Journal of Portfolio Management, V ol. 42, No.1[2] Gu, S., Kelly, B. T., & Xiu, D. (2018). Empirical asset pricing via machine learning. Social Science Electronic Publishing.海外文献推荐-第四十四期参考文献:[1] David P. Morton, Elmira Popova, Ivilina Popova, Journal of Banking & Finance 30 (2006) 503–518海外文献推荐-第四十五期参考文献:[1] Lleo, S., & Ziemba, W. T. (2017). A tale of two indexes: predicting equity market downturns in china. Social Science Electronic Publishing海外文献推荐-第四十六期参考文献:[1] Alquist, R., Israel, R., & Moskowitz, T. J. (2018). Fact, fiction, and the size effect. Social Science Electronic Publishing.[2] Kacperczyk M, NIEUWERBURGH S V A N, Veldkamp L. Time-varying fund manager skill[J]. The Journal of Finance, 2014, 69(4): 1455-1484.海外文献推荐-第四十七期参考文献:[1] Tom Idzorek, 2008, Lifetime Asset Allocations: Methodologies for Target Maturity Funds, Ibbotson Associates Research Paper,29-47[2] Da, Z., Huang, D., & Yun, H. (2017). Industrial electricity usage and stock returns. Journal of Financial & Quantitative Analysis, 52(1), 37-69.海外文献推荐-第四十八期参考文献:[1] Clifford Asness and Andrea Frazzini, 2013, The Devil in HML’s Details, The Journal of Portfolio Management, volume 39 number 4.[2] Carvalho, R. L. D., Xiao, L., & Moulin, P. (2011). Demystifying equity risk-based strategies: a simple alpha plus beta description.Journal of Portfolio Management,38(3), 56-70.海外文献推荐-第四十九期参考文献:[1]Jordan Brooks, Diogo Palhares, Scott Richardson, Style investing in fixed income, Journal of Portfolio Management.[2] R Ball,J Gerakos,JT Linnainmaa,V Nikolaev,2015,Deflating profitability,Journal of Financial Economics, 117 (2) :225-248海外文献推荐-第五十期参考文献:[1] Padmakar Kulkarni, Abhishek Gupta, Stuart Doole, 2018, How can Factors be Combined, MSCI.[2] Hsieh, C. C., Hui, K. W., & Zhang, Y. (2016). Analyst report readability and stock returns. Journal of Business Finance & Accounting, 43(1-2), págs. 98-130.海外文献推荐-第五十一期参考文献:[1] Cici G, Rosenfeld C. A study of analyst-run mutual funds: The abilities and roles of buy-side analysts [J]. Journal of Empirical Finance, 2016, 36:8-29.[2] U-Wen Kok, CFA, Jason Ribando, CFA, and Richard Sloan Facts about Formulaic Value Investing Financial Analysts Journal. V olume 73, Issue 2海外文献推荐-第五十二期参考文献:[1] Morningstar Manager Research.(2018)Target-Date Fund Landscape. 7 May 2018[2] Yong Chen, Gregory W. Eaton, Bradley S. Paye, Micro(structure) before Macro? The Predictive Power of Aggregate llliquidity for Stock Returns and Economic Activity, Journal of Financial Economics (2018), doi: 10.1016/j.jfineco.2018.05.011海外文献推荐-第五十三期参考文献:[1]Arnott R D, Chaves D B, Chow T. King of the Mountain:, Shiller P/E and Macroeconomic Conditions[J]. Social Science Electronic Publishing, 2015, 44(1):55-68.[2]Risk Parity Portfolio vs. Other Asset Allocation Heuristic Portfolios [J]. The Journal of Investing. 2010 December海外文献推荐-第五十四期参考文献:[1]Cliff's Perspective, Our Model Goes to Six and Saves Value From Redundancy Along the Way,AQR Capital Management, December 17, 2014[2]D Avramov,S Cheng,A Schreiber,K Shemer,2017,Scaling up Market Anomalies,Social Science Electronic Publishing,26 (3) :89-105海外文献推荐-第五十五期参考文献:[1]Aurélien Philippot,Analysts’ reinitiations of coverage and market underreaction,Journal of Banking and Finance , 94 (2018) 208–220海外文献推荐-第五十六期参考文献:[1]Michael W. Brandt, Earnings Announcements are Full of Surprises,Social Science Electronic Publishing, January 22, 2008[2]Sujin Pyo, Jaewook Lee,Exploiting the low-risk anomaly using machine learning to enhance the Black–Litterman framework: Evidence from South Korea,Pacific-Basin Finance Journal,51 (2018) 1–12[3]Robert F Engle and Andrew J Patton,What good is a volatility model?,Robert F Engle and Andrew J Patton海外文献推荐-第五十七期参考文献:[1]Nic Schaub, The Role of Data Providers as Information Intermediaries,Social Science Electronic Publishing, 2015 :1-34海外文献推荐-第五十八期参考文献:[1]Binu George and Hardik Shah, ESG: Improving Your Risk-Adjusted Returns in Emerging Markets,GMO White Paper, Mar 2018海外文献推荐-第五十九期参考文献:[1]Campbell R. Harvey and Yan Liu. Backtesting. Journal of portfolio management, 2015海外文献推荐-第六十期参考文献:[1]Mclean R D, Pontiff J. Does Academic Research Destroy Stock Return Predictability?[J]. Journal of Finance, 2016, 71(1)海外文献推荐-第六十一期参考文献:[1]Israelov R, Tummala H. Which Index Options Should You Sell?[J]. Social Science Electronic Publishing, 2017海外文献推荐-第六十二期参考文献:[1]Eric H. Sorensen, Keith L. Miller, and Chee K. Ooi,2000,The Decision Tree Approach to Stock Selection,The Journal of Portfolio Management,42-52海外文献推荐-第六十三期参考文献:[1]Donangelo A, Gourio F, Kehrig M, et al. The cross-section of labor leverage and equity returns[J]. Journal of Financial Economics, 2018海外文献推荐-第六十四期参考文献:[1]Qang Bu. Do Persistent Fund Alphas Indicate Manager Skill? [J]. Journal of Wealth Management,2017,20(2)82-93海外文献推荐-第六十五期参考文献:[1]Miguel A. Lejeune A VaR Black–Litterman model for the construction of absolute return fund-offunds [J] Quantitative Finance · January 2009海外文献推荐-第六十六期参考文献:[1]Fan J H, Zhang T. Demystifying Commodity Futures in China [J]. Social Science Electronic Publishing, 2018海外文献推荐-第六十七期参考文献:[1]Jon Hale, Sustainable Funds U.S. Landscape Report. Morningstar Research, 2018.海外文献推荐-第六十八期参考文献:[1]Sun Z, Wang A, Zheng L. Only Winners in Tough Times Repeat: Hedge Fund Performance Persistence over Different Market Conditions[J]. Journal of Financial and Quantitative Analysis, 2018.海外文献推荐-第六十九期参考文献:[1] A´LVARO CARTEA,SEBASTIAN JAIMUNGAL. RISK METRICS AND FINE TUNING OF HIGH-FREQUENCY TRADING STRATEGIES [J]. Mathematical Finance, V ol. 00, No. 0 (xxx 2013), 1-36.海外文献推荐-第七十期参考文献:[1] Dopfel, Frederick E. , and L. Ashley . "Optimal Blending of Smart Beta and Multifactor Portfolios." The Journal of Portfolio Management 44.4(2018):93-105.海外文献推荐-第七十一期参考文献:[1] Avraham Kamara, Robert Korajczyk, Xiaoxia Lou and Ronnie Sadka,2018,Short-Horizon Beta or Long-Horizon Alpha?, The Journal of Portfolio Management,45(1),96-105海外文献推荐-第七十二期参考文献:[1] Masulis, Ronald W., and Emma Jincheng Zhang. "How valuable are independent directors? Evidence from external distractions." Journal of Financial Economics (2018).海外文献推荐-第七十三期参考文献:[1] Hunter D, Kandel E, Kandel S, et al. Mutual fund performance evaluation with active peer benchmarks[J]. Journal of Financial economics, 2014, 112(1): 1-29.海外文献推荐-第七十四期参考文献:[1]Michael Stein and Svetlozar T. Rachev. Style Neutral Funds of Funds: Diversification or Deadweight? [J]. Journal of Asset Management, February 2011, V olume 11, Issue 6, pp 417–434海外文献推荐-第七十五期参考文献:[1] Elisabeth Kashner, 2019.01.31, Bogle led this investing Fee War, ;[2] Cinthia Murphy,2017,03.31, how to launch a successful ETF, ;[3] Drew V oros, 2019.01.23, how a small ETF Issuer Competes, ;[4] 2019.01.04, Invesco focusing on scale,海外文献推荐-第七十六期参考文献:[1] Shpak I , Human B , Nardon A . Idiosyncratic momentum in commodity futures[J]. Social Science Electronic Publishing, 2017.海外文献推荐-第七十六期参考文献:[1] Ehsani S , Linnainmaa J T . Factor Momentum and the Momentum Factor[J]. Social Science Electronic Publishing, 2017.海外文献推荐-第七十七期参考文献:[1] Iuliia Shpak*, Ben Human and Andrea Nardon. 2017.09.11, Idiosyncratic momentum in commodity futures. ResearchGate海外文献推荐-第七十八期参考文献:[1] Joel Hasbrouck. High-Frequency Quoting: Short-Term V olatility in Bids and Offers. JOURNAL OF FINANCIAL AND QUANTITATIVE ANALYSIS海外文献推荐-第七十九期参考文献:[1] Tarun Gupta and Bryan Kelly. Factor Momentum Everywhere. Institutional Investor Journals海外文献推荐-第八十期参考文献:[1] MICHAEL A. BABYAK , P H D. What You See May Not Be What You Get: A Brief, Nontechnical Introduction to Overfitting in Regression-Type Models. S T A T I S T I C A L C O R N E R海外文献推荐-第八十一期参考文献:[1] Eric Jondeau , Qunzi Zhang , Xiaoneng Zhu. Average Skewness Matters.海外文献推荐-第八十二期参考文献:[1] JOHN A. HASLEM. Morningstar Mutual Fund Measures and Selection Model. THE JOURNAL OF WEALTH MANAGEMENT海外文献推荐-第八十三期参考文献:[1] EUGENE F. FAMA and KENNETH R. FRENCH. Luck versus Skill in the Cross-Section of Mutual Fund Returns. THE JOURNAL OF FINANCE海外文献推荐-第八十四期参考文献:[1] How Transparent Are ETFs?[2] Lara Crigger. Nontransparent Active: Next ETF Revolution?.海外文献推荐-第八十五期参考文献:[1] Olivier Rousse and Benoît Sévi. Informed Trading in Oil-Futures Market. Fondazione Eni Enrico Mattei (FEEM)海外文献推荐-第八十六期参考文献:[1] Ari Levine and Lasse Heje Pedersen. Which Trend is Your Friend?。
- 1、下载文档前请自行甄别文档内容的完整性,平台不提供额外的编辑、内容补充、找答案等附加服务。
- 2、"仅部分预览"的文档,不可在线预览部分如存在完整性等问题,可反馈申请退款(可完整预览的文档不适用该条件!)。
- 3、如文档侵犯您的权益,请联系客服反馈,我们会尽快为您处理(人工客服工作时间:9:00-18:30)。
Multi-factor volatility and stockreturnsZhongzhi(Lawrence)He a,Jie Zhu b,⇑,Xiaoneng Zhu ba Brock University,Ontario,Canadab Shanghai University of Finance and Economics,and Shanghai Key Laboratory of Financial Information Technology,Chinaa r t i c l e i n f oArticle history:Received13January2015 Accepted15September2015 Available online9October2015JEL Classification:C1C58G12Keywords:Multi-factor volatilityCross-sectional returnsOut-of-sample predictability Asset allocation a b s t r a c tIn light of inconclusive evidence on the relation between market volatility and stock returns,this paper proposes amodeland examines its impact on cross-sectional pricing.We alsoevaluate and economic significance of multi-factor volatility.Wefindthat the size and value dynamic factor earn significant and positive variancerisk multi-factor volatility can significantly improve the out-of-sample returnpredictability with a positive economic gain in asset allocation.Ó2015Elsevier B.V.All rights reserved.1.IntroductionThe question of whether volatility affects stock returns is anenduring one infinancial economics.Merton’s(1973)ICAPM inter-prets the change in market volatility as the time-varying invest-ment opportunity that characterizes a shift in the trade-offbetween risk and return.In equilibrium,investors taking on addi-tional risk should be compensated through higher expected return,which implies a positive correlation in the volatility-returnrelationship.However,empirical evidence on the volatility-returnrelationship is still inconclusive within the single-factor volatilityframework.1The assumption of a single market volatility may leadto model misspecification if there exist additional sources of volatil-ity risk that characterize a multi-dimensional change in the invest-ment opportunity set.In this paper,we examine the effect ofmulti-factor volatility on cross-sectional returns,and evaluate theout-of-sample performance and economic significance of multi-factor volatility as compared to existing benchmarks.Althoughfinancial theories do not specify the number and theidentity of volatility risk factors,the prominent Fama–French(FF)three factors provide a natural guidance for our study on multi-factor volatility.This is motivated by some empirical evidence thatthe FF size and value factor are proxies for state variables that arelinked to fundamental risk in the economy(Liew and Vassalou,2000;Vassalou,2003).We therefore investigate whether or notthe volatility of the size and value factor captures the macroeco-nomic uncertainty risk by examining its impact on cross-sectional returns,its out-of-sample performance,and economicsignificance.To accomplish this,we develop a two-stage multi-factor volatil-ity model using a dynamic Fama–French three-factor approach.Thefirst stage allows us to simultaneously estimate conditionalmeans(as an AR process)and conditional variances(as a GARCHprocess)of the market,size,and value factor.The second stageestimates variance risk premia of the three factors and tests thecross-sectional pricing restriction.Non-arbitrage requires the exis-tence of significant variance risk premia that drive pricing errorsinsignificant in the cross-section.In addition,we conduct out-of-sample predictive regressions and asset allocation tests to ensurethat multi-factor volatility is non-elusive and carries a significanteconomic value./10.1016/j.jbankfin.2015.09.0130378-4266/Ó2015Elsevier B.V.All rights reserved.⇑Corresponding author at:School of Finance,Shanghai University of Finance andEconomics,Guoding Road777,Shanghai200433,China.Tel.:+862165908403.E-mail address:zhu.jie@(J.Zhu).1For time-series studies,Ghysels et al.(2005),Guo and Whitelaw(2006)andLudvigson and Ng(2007)find that aggregate volatility is positively related to marketexpected returns,whereas Glosten et al.(1993),Brandt and Kang(2004)andChristensen et al.(2010)document a negative relationship.In the cross-section ofstocks,Ang et al.(2006)and Adrian and Rosenberg(2008)find a negative price ofvolatility risk,which is opposite to the prediction of the variance risk premiumliterature(Bollerslev et al.,2009;Drechsler and Yaron,2011).Our results on the multi-factor volatility model are ing the Fama–French10Â10size and book-to-market sorted portfolios as the primary test portfolios,2wefind that sensi-tivities to the conditional variances of the three factors exhibit wide dispersions across the100portfolios.Furthermore,the conditional variances of the size and value factor carry highly significant and positive risk premia,rendering the new model with a stronger asset-pricing performance than the competing models in terms of higher adjusted R-square,lower sum of squared errors,and lower pricing error statistics.To alleviate the potential model misspecifica-tion problem that might cause a spurious relationship,we test the pricing ability of multi-factor volatility using various asset-pricing models(i.e.,the CAPM,ICAPM,and CCAPM),and stillfind significant results regardless of the models used.As for the out-of-sample fore-casting performance,the multi-factor volatility model beats the existing benchmarks with a wide margin.For example,the model produces lower forecasting errors for at least89out of the100port-folios in the forecast of one-period-ahead expected returns.This sug-gests that its superior pricing power is not caused by adding some random factors;instead,multi-factor volatility is an incremental source of pervasive risk factors that should be priced both in sample and out of sample.To gauge its economic significance,our asset allo-cation results show that the multi-factor volatility model outper-forms other models by about1–3%annualized certainty equivalent returns(CER)in most cases,and the CER gap becomes even larger as investors exhibit more risk aversion.Our model also yields signif-icantly higher Sharpe ratios than most benchmark models.As for practical implications,the asset-allocation results suggest that by accounting for multi-factor volatility into portfolio decisions,asset managers can significantly improve the risk-return trade-off of their portfolios.The contribution of this paper is twofold.First,the paper sheds new light on the relationship between volatility risk and cross-sectional returns.In this literature,Ang et al.(2006)estimate a neg-ative price of volatility risk of approximatelyÀ1%per annum.3The idea is that since innovations in volatility are higher during reces-sions,stocks that co-vary with volatility are stocks that pay off in bad states,and these should require a smaller risk premium.In a sim-ilar vein,Adrian and Rosenberg(2008)decompose market volatility into the short-run and long-run component andfind that both com-ponents constitute negative volatility risk premia.On the other hand, theoretical work of Bollerslev et al.(2009)and Drechsler and Yaron (2011)predict a positive variance risk premium that compensates for macroeconomic uncertainty risk.Han and Zhou(2011)take a cross-sectional pricing approach andfind that stocks with higher var-iance risk premium earn higher expected returns.These studies esti-mate a positive price of volatility risk using options on the aggregate market and individual stocks.It is infeasible,however,to estimate multi-factor volatility using options ing the cross-section of stock returns,rather than options data,allows us(1)to create portfo-lios of stocks that have different sensitivities to variations in multiple sources of volatility risk;(2)to control for a battery of other well-known risk-return effects.Ourfinding of positive prices of multi-factor volatility risk supports the prediction of the variance risk premium literature.We show that each factor volatility is positively correlated with variance risk premium.This implies that multi-factor volatility can serve as empirical proxy for variance risk premium,but with a stronger pricing ability.Indeed,in the presence of highly sig-nificant and positive premia for the volatility of the size and value factor,the risk premium for the market volatility becomes insignifi-cant.Thisfinding is robust to in-sample pricing,out-of-sample fore-casting,alternative model and volatility specifications,and model test concerns raised by Lewellen et al.(2010).Second,this paper contributes to the growing body of literature on out-of-sample return predictability by adding multi-factor vol-atility as a reliable set of predictors.In this strand of literature, Welch and Goyal(2008)and Simin(2008)argue that historical average returns forecast future returns better than predictive vari-ables for both the market and individual stocks.Campbell and Thompson(2008)show that imposing meaningful restrictions on coefficients can largely improve the out-of-sample explanatory power of regressions.Rapach et al.(2010)recommend combining information from numerous economic variables to improve out-of-sample equity premium prediction.More recent studies propose technical indicators(Neely et al.,2014),time-varying coefficients (Dangl and Halling,2012),sum-of-the-parts method(Ferreira and Santa-Clara,2011),and conditioning on market state(Henkel et al.,2011;Zhu and Zhu,2013;Zhu,forthcoming),among others, to predict stock market returns.In light of the literature,this paper argues that multi-factor volatility can significantly improve the out-of-sample return predictability.The economic gains of multi-factor volatility are evaluated by a dynamic asset-allocation approach of Tu(2010).The remainder of our paper is organized as follows.Section2 introduces thefirst stage of the multi-factor volatility model. Section3discusses the model estimation and estimated parame-ters.Section4conducts in-sample asset-pricing tests of the multi-factor volatility model as compared to the competing models.Section5performs out-of-sample forecasting tests of the multi-volatility model as compared to various benchmark models. Section6provides the asset allocation test to evaluate the economic value of the multi-factor volatility model.Section7 concludes the paper with future research directions.The appendix includes details of the estimation procedure and results from Monte Carlo simulations.2.The multi-factor volatility modelThe Fama–French three-factor model(1993,1996;FF3 hereafter)has been an empirical benchmark in the modern asset pricing literature.An open issue that has yet to be addressed in the literature is the relationship between the volatility of the Fama–French factors and expected stock returns.Fama and French(2012)explore the volatility of the market,size,and value premia of the three factors.Our paper formalizes this idea into con-ditional means and conditional variances of the three factors within a dynamic factor framework.In the FF3model,three factors originate from the6portfolios formed on size and book-to-market ratio(BTM).He et al.(2010) (HHL hereafter)use the Kalmanfilter to extract3latent dynamic factors from the6size and BTM portfolios by properly restricting factor loadings(betas).We adopt the HHL method and estimate the conditional means and conditional variances of the three fac-tors simultaneously.Denote the6size and BTM portfolios as SL,SM,SH,BL,BM, and BH.Let R t¼½R SL;t R SM;t R SH;t R BL;t R BM;t R BH;t 0be a vector of demeaned excess returns on the6portfolios in month t.The latent MKT,SIZE,and BTM dynamic factors are given by D t¼ðD MKT;t D SIZE;t D BTM;tÞ0.With these notations,the dynamic factor model is represented in the state-space form,with the6observa-tion or measurement equations given by:2Given the extreme returns in some corner portfolios,the100portfolios pose amuch greater challenge than the25portfolios(commonly used in the literature)interms of in-sample pricing and out-of-sample forecasting.In addition,using100portfolios partially alleviates the model test problem raised by Lewellen et al.(2010).3Another majorfinding of Ang et al.(2006)is that the idiosyncratic volatilityrelative to the Fama–French factors earns a significantly negative risk premium,which cannot be explained by the systematic market volatility.There is a contentiousdebate between Ang et al.(2009),Bali and Cakici(2008)and Fu(2009)with regard tothe sign of idiosyncratic volatility risk premium.The asset-pricing role of idiosyn-cratic volatility is beyond the scope of this paper.Zhongzhi(Lawrence)He et al./Journal of Banking&Finance61(2015)S132–S149S133R SL ;tR SM ;t R SH ;t R BL ;t R BM ;t R BH ;t266666666666664377777777777775¼b SL b S b LbSM b S b M b SHb S b H b BLb B b L b BMb B b M b BHb Bb H266666666666664377777777777775D MKT ;t D SIZE ;t D BTM ;t 2666437775þw SL ;tw SM ;t w SH ;t w BL ;t w BM ;t w BH ;t266666666666664377777777777775;ð1Þwhere b s are the factor loadings on the unobserved factors and w t ¼½w SL ;t w SM ;t w SH ;t w BL ;t w BM ;t w BH ;t 0is the (6Â1)vector of error terms.It is important to note that some equality restrictions must be imposed on the factor loading matrix so that the extracted factors have a predetermined interpretation of the market factor (D MKT ;t ),the size factor (D SIZE ;t ),and the BTM factor (D BTM ;t ).The market factor is identified from the 1st column of the factor loading matrix,with no restrictions on the factor loadings.Without imposing restrictions,the market factor captures common price dynamics across the 6portfolios consisting of market-wide assets.In this sense,the market factor can be interpreted as a composite index.The size factor is used to capture the size-related variation in returns that is not captured by the market factor.The intuition for identifying the size factor is straightforward:assets within the same size group should have the same sensitivity to the size factor,whereas assets across size groups should have different sensitivities to the size factor.Hence,the equality restrictions imposed on the 2nd column of the factor loading matrix are:(1)the first 3coefficients in the 2nd column are all equal to b S so that it captures the small-size effect;(2)the last 3coefficients in the 2nd column are all equal to b B so that it captures the large-size effect.Similarly,the BTM factor can be identified by imposing equal-ity restrictions on the 3rd column of the factor loading matrix.These restrictions reflect the fact that assets within the same BTM group have the same sensitivity to the BTM factor and assets across BTM groups have different sensitivities to the BTM factor.Specifically,these equality restrictions are:(1)the first and fourth coefficients in the 3rd column are equal to b L so that it captures the low BTM effect;(2)the second and fifth coefficients in the 3rd column are equal to b M so that it captures the medium BTM effect;and (3)the third and sixth coefficients in the 3rd column are equal to b H so that it captures the high BTM effect.The three state equations are expressed asD MKT ;tD SIZE ;t D BTM ;t 264375¼/MKT000/SIZE 00/BTM264375D MKT ;t À1D SIZE ;t À1D BTM ;t À1264375þv MKT ;tv SIZE ;t v BTM ;t 264375;ð2Þwhere /s are the autoregressive coefficients.With the conventional assumption of white noise errors,Eqs.(1)and (2)constitute the HHL dynamic factor model.To capture the conditional variance of the risk factors,we generalize the model and allow for conditional variance in risk factors.Let v t ¼½v MKT ;t v SIZE ;t v BTM ;t 0denote the (3Â1)vector of state equation innovations,andv t ¼H 1=2t g t ;ð3Þwhere H t is the conditional covariance matrix of v t .In addition,g t is the normalized residual,which is assumed to follow a standard nor-mal distribution.We follow the convention and assume a diagonal H t ,where each of the diagonal elements follows a GARCH (1,1)process:h MKT ;th SIZE ;t h BTM ;t264375¼x MKT x SIZE x BTM 264375þa MKT 000a SIZE 000a BTM 264375v 2MKT ;t À1v 2SIZE ;t À1v 2BTM ;t À126643775þc MKT 000c SIZE 000c BTM 264375h MKT ;t À1h SIZE ;t À1h BTM ;t À1264375:ð4ÞThe state-space model as in Eqs.(1)and (2)can be expressed ina more succinct form:R t ¼BD t þw t ;ð5ÞD t ¼U D t À1þv t ;ð6Þwhere v t follows a GARCH process;B is a prespecified constant factor-loading matrix whose columns have identifying restrictions on MKT,SIZE,and BTM;w t is a vector of idiosyncratic returns on the 6portfolios;and U is a diagonal autoregressive coefficient matrix.Following the convention,we also assume that w t follows a normal distribution and w t is uncorrelated with v t .These restric-tions are given by:E ½w t w 0s ¼D ;for t ¼s ;0;for t –s ;ð7ÞE ½w t v 0s ¼0;for all t and s ;ð8Þwhere D ¼diag ½r 2SL r 2SM r 2SH r 2BL r 2BM r 2BH is a (6Â6)diagonal covari-ance matrix of idiosyncratic risk for the observation equations.The conditional variance process can also be expressed in a more suc-cinct form:h t j t À1¼x þA v 2t À1þC h t À1;ð9Þwhere h t j t À1is the time-varying variance conditional on information set I t À1;x ¼½x MKT x SIZE x BTM 0is the (3Â1)vector of unconditional variance;and A and C are diagonal coefficient matrices.With these assumptions,Eqs.(5),(6),and (9)constitute the multi-factor vola-tility model.Another identification issue is associated with the GARCH pro-cess.Indeed,v t is unobservable,so the estimation of the model via the Kalman filter is not operable.Following Kim and Nelson (1999,Chapter 6)and Harvey et al.(1992),we circumvent the unobservable error issue by normalizing the parameters and impose the following restriction:x j ¼1Àa j Àc j ;ð10Þwhere j ¼ðMKT ;SIZE ;BTM Þ.3.Model estimationUnder the assumptions in Eqs.(3),(7),and (8),the Kalman filteris a statistically optimal procedure to extract the unobserved fac-tors from a finite set of observed returns.While using the Kalman filter,we simultaneously run the GARCH process according to Eq.(4)to estimate the conditional variance,h t ,with the normalization condition Eq.(10).The parameters in Eqs.(1),(2),and (4)are esti-mated via the Kalman filter and the GARCH procedure,with the state vector and variance being initialized by their unconditional means and variances of D t .The Kalman filter and the GARCH pro-cedure is then iterated for t ¼1;...;T to recursively extract the conditional expectations of the dynamic factors,D t j t À1and D t j t ,and their conditional variance,h t j t À1.The procedure for extracting the factors and factor variances via the Kalman filter and the GARCH procedure is briefly described in Appendix A .S134Zhongzhi (Lawrence)He et al./Journal of Banking &Finance 61(2015)S132–S1493.1.Data and estimation methodologyWe obtain monthly returns on the6portfolios formed by sort-ing on size and BTM from Kenneth French’s data library.4To com-pute excess returns on the6portfolios,we subtract the1-month T-bill rate.The sample used in this study ranges from January1961toDecember2010,covering600months(50years).After computing the excess returns on the6portfolios,wefirstestimate26model parameters in the observation equations,thestate equations,and the GARCH process using the maximumlikelihood method.To this end,we run the Kalmanfilter combinedwith the GARCH model,as described in Kim and Nelson(1999),with iterations until all parameters converge according to aprespecified precision.After obtaining these point estimates forparameters,we run the Kalmanfilter and the GARCH modelagain to recursively extract the ex ante(forecast at month tÀ1) factors D t j tÀ1¼ðD MKT;t j tÀ1;D SIZE;t j tÀ1;D BTM;t j tÀ1Þ0,the ex post (updated at month t)factors D t j t¼ðD MKT;t j t;D SIZE;t j t;D BTM;t j tÞ0,as well as the conditional variance H t j tÀ1¼ðh MKT;t j tÀ1;h SIZE;t j tÀ1;h BTM;t j tÀ1Þ0, for t¼1961=01—2010=12.3.2.Empirical results3.2.1.Descriptive StatisticsPanel A of Table1presents the mean and variance of the excessreturns(over the1-month T-bill rate,in percentage)for the6size-and BTM-sorted portfolios.Note that the mean returns increasemonotonically with the BTM within each of the2size groups,and the results are consistent with the well-known value effect.For the portfolio of small and growth stocks(SL),wefind that ithas the highest variance with only the second smallest meanreturns.This inverted risk-return feature for the SL group remainsa challenge for asset pricing.The point estimates of the model parameters from the maxi-mum likelihood method are presented in Panel B of Table1.Mostof the parameter estimates are statistically significant at the1%level,indicating that excess returns on the6portfolios conformto the three-dimensional factor structure.Specifically,the loadings on the market factor,D MKT,in thesmall-stock group(b SL;b SM,and b SH)are significantly larger than those in the big-stock group(b BL;b BM,and b BH).Since small stocks are relatively riskier than big stocks,this result implies that thedynamic factor,D MKT,captures the market risk well.As for the load-ings on the size factor,D SIZE,the magnitude of loadings in the big-stock group(b B)is roughly twice as large as that of the small-stockgroup(b S),which indicates that returns are more sensitive to thesize factor in the big-stock group.We alsofind that low(L)BTMstocks have a significantly negative loading(À1.291)on the BTMfactor,D BTM,while the high(H)BTM stocks have a significantlypositive loading(0.856),providing evidence of the growth andvalue effects.In contrast,the loading on the factor in the mediumBTM group(b M)is much smaller and insignificant.This is a desir-able feature since it implies that in a portfolio with a medium levelof BTM,stock returns are not sensitive to the BTM factor.AllGARCH parameters(a j s and c j s)are significant at any conventional level.Furthermore,the stationary condition is satisfied:a jþc j<1 for any j,where j¼ðMKT;SIZE;BTMÞ.The results confirm thatthere exists the GARCH effect in the dynamic process of factorvariances.Regarding the AR(1)coefficients on the3factors,wefind that both/MKTand/BTMare significant at the1%level,while/SIZEis mar-ginally significant around the level of10%.The result indicates that a change in the factor realizations by1standard deviation in the current month will affect the unobserved factor realizations by 7–30%in magnitude in the following month.To visualize the level of time variation in investors’optimal forecasts about the reward/ risk ratio,we draw a graph of the maximum ex ante squared Sharpe Ratio(SSR t j tÀ1)based on Eq.(11)over the sample period (1961/01–2010/12).SSR t j tÀ1¼D0t j tÀ1PÀ1t j tÀ1D t j tÀ1;ð11Þwhere D t j tÀ1¼ðD MKT;t j tÀ1;D SIZE;t j tÀ1;D BTM;t j tÀ1Þ0is the(3Â1)vector of the ex ante dynamic factors,and P t j tÀ1is a(3Â3)matrix including the mean squared error(MSE)of D t j tÀ1.Fig.1illustrates that substantial variation exists in investors’forecast about their risk-return trade-off.The average value of the ex ante SSR is0.10,which is comparable to that of the FF3fac-tors(0.07).The ratio is the highest(0.632)in March2000,when the S&P500index reached its peak in our sample period.We report in Table2descriptive statistics and correlation coef-ficients for the ex post factors D t j t¼ðD MKT;t j t;D SIZE;t j t;D BTM;t j tÞ0,the ex ante factors D t j tÀ1¼ðD MKT;t j tÀ1;D SIZE;t j tÀ1;D BTM;t j tÀ1Þ0,and the conditional variance H t j tÀ1¼ðr2MTK;t j tÀ1;r2SIZE;t j tÀ1;r2BTM;t j tÀ1Þ0.Fornotational simplicity,we denoteðD1MKT;;D1SIZE;D1BTMÞ0andðD0MKT;;D0SIZE;D0BTMÞ0as the time series collection of D t j t and D t j tÀ1, respectively;andðr2MTK;r2SIZE;r2BTMÞ0as the conditional variance estimated at time tÀ1.For comparison purposes,we also report the statistics for the FF3factors and the excess return on the CRSP value-weighted market(VWM)index.Thefirst column of Table2illustrates that the means of the ex post and ex ante estimates of the dynamic factors are all zero by construction.The means of the conditional variance are close to one for r2MTK and r2SIZE,but the mean of r2BTM is larger than one. The magnitude of these mean values is consistent with the vari-ance(STD squared)of the ex post dynamic factorsðD1MKT;;D1SIZE;D1BTMÞ0.The middle part of the table presents the corre-lation coefficients between the dynamic factors,conditional vari-ance,and the FF3factors.We can see from the table that the FF 3factors are correlated with each other:the absolute values of the correlation coefficients range from24%to31%.However,the ex post and the ex ante factors are weakly correlated with each other within each of the two groups:the absolute values of the cor-relation coefficients are1–10%.This implies that each of D MKT;D SIZE, and D BTM independently captures specific aspects in return varia-tions,whereas MKT,SMB,and HML tend to jointly explain return variations.The correlation of conditional variance is positive and relatively high with each other within the group:the values of the correlation coefficients are from58%to62%,which implies that the variance of the dynamic factors tends to move together.As for the cross-correlation coefficients between conditional variances,dynamic factors,and FF3factors,we note that condi-tional variances of the dynamic factors are weakly correlated with other variables,including the ex ante and ex post dynamic factors, and FF3factors.The absolute values of the correlation coefficients range from1%to19%,and are less than10%in most cases.The weak correlation between conditional variances and other factors implies that the dynamics of conditional variances contain addi-tional information that is not captured by either dynamic factors or FF3factors.We aim to examine whether or not and to what extent this additional information about return dynamics is impor-tant in asset pricing and out-of-sample forecasting.4The6portfolios are the original assets used by Fama and French(1993)toconstruct the FF3factors.For robustness,we also use5Â5portfolios sorted on sizeand book-to-market ratio to extract dynamic factors.Wefind that dynamic factorsextracted from25portfolios are highly correlated with those from6portfolios.Thepricing performance is also very similar.Zhongzhi(Lawrence)He et al./Journal of Banking&Finance61(2015)S132–S149S135We alsoplot the conditional volatility processðr MKT ;t j t À1;r SIZE ;t j t À1;r BTM ;t j t À1Þfor the three factors,as given by Fig.2.The figure illustrates that all three series of factor volatility exhibit a large time variation.Specifically,the market volatility peaked during the years of 1974,1987,2000,and 2007,which cor-responds to the Oil Crisis,the Black Monday stock market crash,the Dotcom Bubble burst,and the Subprime Crisis,respectively,indicating that market uncertainty or risk increased significantly during these crisis periods.In contrast,the size factor volatility fluctuates less than the market factor volatility in most time peri-ods.However,it increased sharply when the Dotcom Bubble stly,the dynamic of the BTM factor volatility exhibits a large time-variation along with the market volatility.3.2.2.Relation with variance risk premiumIn the variance risk premium literature,Bollerslev et al.(2009)and Drechsler and Yaron (2011)predict a positive variance risk premium.Bollerslev et al.(2009)relate this result to the volatility of the variance of consumption which should be priced in an equilibrium model with recursive preference.In this subsection,we demonstrate the empirical relationship between variance risk premium and our dynamic volatility.Following Bollerslev et al.(2009),we define variance risk premium as the implied volatility (ImV)minus the realized volatility (RV)on S&P 500index:VRP t ¼Im V t ÀRV t ;ð12Þin which RV t is calculated as followsTable 1Summary statistics and parameter estimates.ItemSLSM SH BL BM BH Panel A:descriptive statistics about the 6portfolios returns (in percent)formed on size and BTM Mean 0:4490:8651:0450:4330:5070:685Variance 48:0629:8931:8822:3119:1022:14Panel B:maximum likelihood estimates of the parameters of the model Parameter Estimated value Parameter Estimated value Parameter Estimated value b SL 6:297ÃÃÃb M 0:192/SIZE À0:0684(0.439)(0.226)(0.0549)b SM 5:152ÃÃÃb H0:856ÃÃÃ/BTM0:296ÃÃÃ(0.357)(0.280)(0.0578)b SH 5:218ÃÃÃr 2SL 1:134ÃÃÃa MKT 0:0932ÃÃÃ(0.363)(0.219)(0.0314)b BL 3:052ÃÃÃr 2SM 0:353ÃÃÃc MKT 0:827ÃÃÃ(0.332)(0.0653)(0.0548)b BM 2:856ÃÃÃr 2SH 0:906ÃÃÃa SIZE 0:196ÃÃÃ(0.309)(0.119)(0.0506)b BH 3:208ÃÃÃr 2BL 1:689ÃÃÃc SIZE 0:735ÃÃÃ(0.317)(0.229)(0.0681)b S 1:680ÃÃÃr 2BM 1:387ÃÃÃa BTM 0:228ÃÃÃ(0.477)(0.134)(0.0511)b B 3:144ÃÃÃr 2BH1:757ÃÃÃc BTM0:738ÃÃÃ(0.464)(0.172)(0.0544)b LÀ1:291ÃÃÃ/MKT0:222ÃÃÃ(0.346)(0.0470)Panel A of Table 1reports the means and variance of excess returns (over the 1-month T-bill rate,in percent)on the 6size and book-to-market (BTM)sorted portfolios over600months (50years,1961/01–2010/12).Panel B reports the maximum likelihood estimates of the parameters for the model obtained using the Kalman filter and GARCH procedure.Standard errors are reported in the parentheses.The 6size-and BTM-sorted portfolios are notated as SL,SM,SH,BL,BM,and BH,where S and B denote ‘‘small”and ‘‘big”in firm size,respectively,and L,M,and H denote ‘‘low”,‘‘medium”,and ‘‘high”in the BTM ratio,respectively.R t ¼½R SL ;t R SM ;t R SH ;t R BL ;t R BM ;t R BH ;t 0is a ð6Â1Þvector of demeaned excess returns on the 6portfolios at month t ;and D t ¼½D MKT ;t D SIZE ;t D BTM ;t 0is a vector of zero-mean unobserved state vector at month t .The model is specified as(i)observation equationsR SL ;t R SM ;t R SH ;t R BL ;t R BM ;t R BH ;t 26666666643777777775¼b SL b S b Lb SM b S b M b SH b S b H b BL b B b L b BM b B b M b BHb Bb H26666666643777777775DMKT ;tD SIZE ;t D BTM ;t 264375þw SL ;t w SM ;t w SH ;t w BL ;t w BM ;t w BH ;t 26666666643777777775;(ii)state equationsD MKT ;t D SIZE ;t D BTM ;t264375¼/MKT000/SIZE 00/BTM264375D MKT ;t À1D SIZE ;t À1D BTM ;t À1264375þv MKT ;t v SIZE ;t v BTM ;t264375;and (iii)conditional variance equationsv t¼H 1=2t g t ;h MKT ;th SIZE ;t h BTM ;t264375¼x MKT x SIZE x BTM 264375þa MKT 000a SIZE00a BTM 264375v 2MKT ;t À1v 2SIZE ;t À1v 2BTM ;t À126643775þcMKT 00c SIZE 000c BTM 264375h MKT ;t À1h SIZE ;t À1h BTM ;t À1264375:ÃÃÃindicates coefficients significantly different from zero at the 1%level.S136Zhongzhi (Lawrence)He et al./Journal of Banking &Finance 61(2015)S132–S149。