语音识别系统中英文对照外文翻译文献
通信类中英文翻译、外文文献翻译
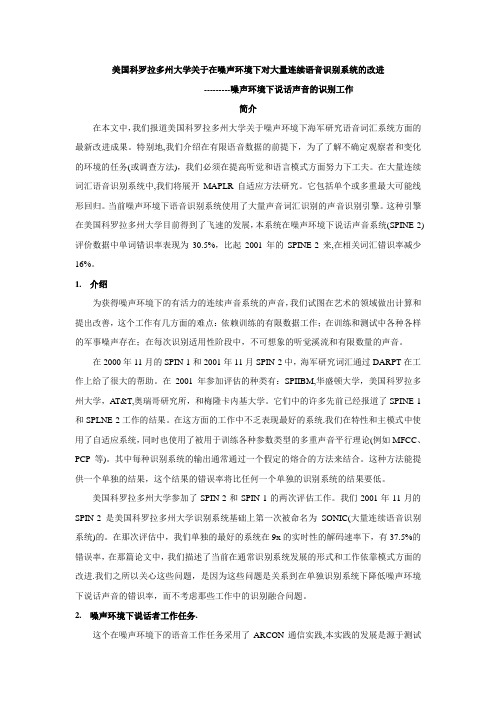
美国科罗拉多州大学关于在噪声环境下对大量连续语音识别系统的改进---------噪声环境下说话声音的识别工作简介在本文中,我们报道美国科罗拉多州大学关于噪声环境下海军研究语音词汇系统方面的最新改进成果。
特别地,我们介绍在有限语音数据的前提下,为了了解不确定观察者和变化的环境的任务(或调查方法),我们必须在提高听觉和语言模式方面努力下工夫。
在大量连续词汇语音识别系统中,我们将展开MAPLR自适应方法研究。
它包括单个或多重最大可能线形回归。
当前噪声环境下语音识别系统使用了大量声音词汇识别的声音识别引擎。
这种引擎在美国科罗拉多州大学目前得到了飞速的发展,本系统在噪声环境下说话声音系统(SPINE-2)评价数据中单词错识率表现为30.5%,比起2001年的SPINE-2来,在相关词汇错识率减少16%。
1.介绍为获得噪声环境下的有活力的连续声音系统的声音,我们试图在艺术的领域做出计算和提出改善,这个工作有几方面的难点:依赖训练的有限数据工作;在训练和测试中各种各样的军事噪声存在;在每次识别适用性阶段中,不可想象的听觉溪流和有限数量的声音。
在2000年11月的SPIN-1和2001年11月SPIN-2中,海军研究词汇通过DARPT在工作上给了很大的帮助。
在2001年参加评估的种类有:SPIIBM,华盛顿大学,美国科罗拉多州大学,AT&T,奥瑞哥研究所,和梅隆卡内基大学。
它们中的许多先前已经报道了SPINE-1和SPLNE-2工作的结果。
在这方面的工作中不乏表现最好的系统.我们在特性和主模式中使用了自适应系统,同时也使用了被用于训练各种参数类型的多重声音平行理论(例如MFCC、PCP等)。
其中每种识别系统的输出通常通过一个假定的熔合的方法来结合。
这种方法能提供一个单独的结果,这个结果的错误率将比任何一个单独的识别系统的结果要低。
美国科罗拉多州大学参加了SPIN-2和SPIN-1的两次评估工作。
我们2001年11月的SPIN-2是美国科罗拉多州大学识别系统基础上第一次被命名为SONIC(大量连续语音识别系统)的。
外文翻译---说话人识别
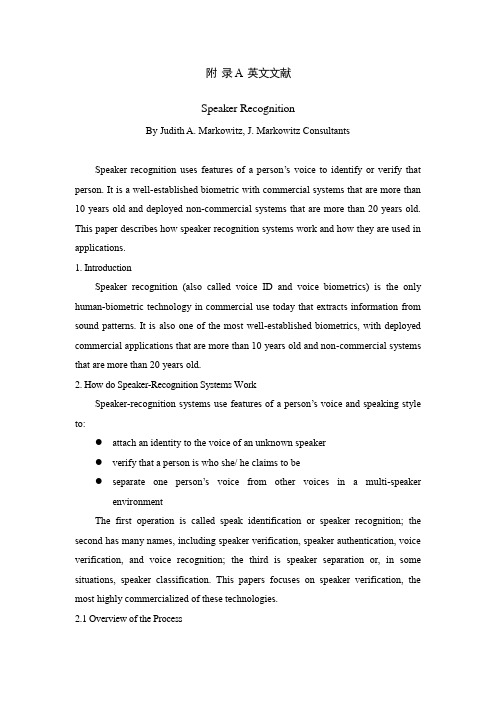
附录A 英文文献Speaker RecognitionBy Judith A. Markowitz, J. Markowitz ConsultantsSpeaker recognition uses features of a person‟s voice to identify or verify that person. It is a well-established biometric with commercial systems that are more than 10 years old and deployed non-commercial systems that are more than 20 years old. This paper describes how speaker recognition systems work and how they are used in applications.1. IntroductionSpeaker recognition (also called voice ID and voice biometrics) is the only human-biometric technology in commercial use today that extracts information from sound patterns. It is also one of the most well-established biometrics, with deployed commercial applications that are more than 10 years old and non-commercial systems that are more than 20 years old.2. How do Speaker-Recognition Systems WorkSpeaker-recognition systems use features of a person‟s voice and speaking style to:●attach an identity to the voice of an unknown speaker●verify that a person is who she/ he claims to be●separate one person‟s voice from other voices in a multi-speakerenvironmentThe first operation is called speak identification or speaker recognition; the second has many names, including speaker verification, speaker authentication, voice verification, and voice recognition; the third is speaker separation or, in some situations, speaker classification. This papers focuses on speaker verification, the most highly commercialized of these technologies.2.1 Overview of the ProcessSpeaker verification is a biometric technology used for determining whether the person is who she or he claims to be. It should not be confused with speech recognition, a non-biometric technology used for identifying what a person is saying. Speech recognition products are not designed to determine who is speaking.Speaker verification begins with a claim of identity (see Figure A1). Usually, the claim entails manual entry of a personal identification number (PIN), but a growing number of products allow spoken entry of the PIN and use speech recognition to identify the numeric code. Some applications replace manual or spoken PIN entry with bank cards, smartcards, or the number of the telephone being used. PINS are also eliminated when a speaker-verification system contacts the user, an approach typical of systems used to monitor home-incarcerated criminals.Figure A1.Once the identity claim has been made, the system retrieves the stored voice sample (called a voiceprint) for the claimed identity and requests spoken input from the person making the claim. Usually, the requested input is a password. The newly input speech is compared with the stored voiceprint and the results of that comparison are measured against an acceptance/rejection threshold. Finally, the system accepts the speaker as the authorized user, rejects the speaker as an impostor, or takes another action determined by the application. Some systems report a confidence level or other score indicating how confident it about its decision.If the verification is successful the system may update the acoustic information in the stored voiceprint. This process is called adaptation. Adaptation is an unobtrusive solution for keeping voiceprints current and is used by many commercial speaker verification systems.2.2 The Speech SampleAs with all biometrics, before verification (or identification) can be performed the person must provide a sample of speech (called enrolment). The sample is used to create the stored voiceprint.Systems differ in the type and amount of speech needed for enrolment and verification. The basic divisions among these systems are●text dependent●text independent●text prompted2.2.1 Text DependentMost commercial systems are text dependent.Text-dependent systems expect the speaker to say a pre-determined phrase, password, or ID. By controlling the words that are spoken the system can look for a close match with the stored voiceprint. Typically, each person selects a private password, although some administrators prefer to assign passwords. Passwords offer extra security, requiring an impostor to know the correct PIN and password and to have a matching voice. Some systems further enhance security by not storing a human-readable representation of the password.A global phrase may also be used. In its 1996 pilot of speaker verification Chase Manhattan Bank used …Verification by Chemical Bank‟. Global phrases avoid the problem of forgotten passwords, but lack the added protection offered by private passwords.2.2.2 Text IndependentText-independent systems ask the person to talk. What the person says is different every time. It is extremely difficult to accurately compare utterances that are totally different from each other - particularly in noisy environments or over poor telephone connections. Consequently, commercial deployment of text-independentverification has been limited.2.2.3 Text PromptedText-prompted systems (also called challenge response) ask speakers to repeat one or more randomly selected numbers or words (e.g. “43516”, “27,46”, or “Friday, c omputer”). Text prompting adds time to enrolment and verification, but it enhances security against tape recordings. Since the items to be repeated cannot be predicted, it is extremely difficult to play a recording. Furthermore, there is no problem of forgetting a password, even though the PIN, if used, may still be forgotten.2.3 Anti-speaker ModellingMost systems compare the new speech sample with the stored voiceprint for the claimed identity. Other systems also compare the newly input speech with the voices of other people. Such techniques are called anti-speaker modelling. The underlying philosophy of anti-speaker modelling is that under any conditions a voice sample from a particular speaker will be more like other samples from that person than voice samples from other speakers. If, for example, the speaker is using a bad telephone connection and the match with the speaker‟s voiceprint is poor, it is likely that the scores for the cohorts (or world model) will be even worse.The most common anti-speaker techniques are●discriminate training●cohort modeling●world modelsDiscriminate training builds the comparisons into the voiceprint of the new speaker using the voices of the other speakers in the system. Cohort modelling selects a small set of speakers whose voices are similar to that of the person being enrolled. Cohorts are, for example, always the same sex as the speaker. When the speaker attempts verification, the incoming speech is compared with his/her stored voiceprint and with the voiceprints of each of the cohort speakers. World models (also called background models or composite models) contain a cross-section of voices. The same world model is used for all speakers.2.4 Physical and Behavioural BiometricsSpeaker recognition is often characterized as a behavioural biometric. This description is set in contrast with physical biometrics, such as fingerprinting and iris scanning. Unfortunately, its classification as a behavioural biometric promotes the misunderstanding that speaker recognition is entirely (or almost entirely) behavioural. If that were the case, good mimics would have no difficulty defeating speaker-recognition systems. Early studies determined this was not the case and identified mimic-resistant factors. Those factors reflect the size and shape of a speaker‟s speaking mechanism (called the vocal tract).The physical/behavioural classification also implies that performance of physical biometrics is not heavily influenced by behaviour. This misconception has led to the design of biometric systems that are unnecessarily vulnerable to careless and resistant users. This is unfortunate because it has delayed good human-factors design for those biometrics.3. How is Speaker Verification Used?Speaker verification is well-established as a means of providing biometric-based security for:●telephone networks●site access●data and data networksand monitoring of:●criminal offenders in community release programmes●outbound calls by incarcerated felons●time and attendance3.1 Telephone NetworksToll fraud (theft of long-distance telephone services) is a growing problem that costs telecommunications services providers, government, and private industry US$3-5 billion annually in the United States alone. The major types of toll fraud include the following:●Hacking CPE●Calling card fraud●Call forwarding●Prisoner toll fraud●Hacking 800 numbers●Call sell operations●900 number fraud●Switch/network hits●Social engineering●Subscriber fraud●Cloning wireless telephonesAmong the most damaging are theft of services from customer premises equipment (CPE), such as PBXs, and cloning of wireless telephones. Cloning involves stealing the ID of a telephone and programming other phones with it. Subscriber fraud, a growing problem in Europe, involves enrolling for services, usually under an alias, with no intention of paying for them.Speaker verification has two features that make it ideal for telephone and telephone network security: it uses voice input and it is not bound to proprietary hardware. Unlike most other biometrics that need specialized input devices, speaker verification operates with standard wireline and/or wireless telephones over existing telephone networks. Reliance on input devices created by other manufacturers for a purpose other than speaker verification also means that speaker verification cannot expect the consistency and quality offered by a proprietary input device. Speaker verification must overcome differences in input quality and the way in which speech frequencies are processed. This variability is produced by differences in network type (e.g. wireline v wireless), unpredictable noise levels on the line and in the background, transmission inconsistency, and differences in the microphone in telephone handset. Sensitivity to such variability is reduced through techniques such as speech enhancement and noise modelling, but products still need to be tested under expected conditions of use.Applications of speaker verification on wireline networks include secure calling cards, interactive voice response (IVR) systems, and integration with security forproprietary network systems. Such applications have been deployed by organizations as diverse as the University of Maryland, the Department of Foreign Affairs and International Trade Canada, and AMOCO. Wireless applications focus on preventing cloning but are being extended to subscriber fraud. The European Union is also actively applying speaker verification to telephony in various projects, including Caller Verification in Banking and Telecommunications, COST250, and Picasso.3.2 Site accessThe first deployment of speaker verification more than 20 years ago was for site access control. Since then, speaker verification has been used to control access to office buildings, factories, laboratories, bank vaults, homes, pharmacy departments in hospitals, and even access to the US and Canada. Since April 1997, the US Department of Immigration and Naturalization (INS) and other US and Canadian agencies have been using speaker verification to control after-hours border crossings at the Scobey, Montana port-of-entry. The INS is now testing a combination of speaker verification and face recognition in the commuter lane of other ports-of-entry.3.3 Data and Data NetworksGrowing threats of unauthorized penetration of computing networks, concerns about security of the Internet, and increases in off-site employees with data access needs have produced an upsurge in the application of speaker verification to data and network security.The financial services industry has been a leader in using speaker verification to protect proprietary data networks, electronic funds transfer between banks, access to customer accounts for telephone banking, and employee access to sensitive financial information. The Illinois Department of Revenue, for example, uses speaker verification to allow secure access to tax data by its off-site auditors.3.4 CorrectionsIn 1993, there were 4.8 million adults under correctional supervision in the United States and that number continues to increase. Community release programmes, such as parole and home detention, are the fastest growing segments of this industry. It is no longer possible for corrections officers to provide adequate monitoring ofthose people.In the US, corrections agencies have turned to electronic monitoring systems. Since the late 1980s speaker verification has been one of those electronic monitoring tools. Today, several products are used by corrections agencies, including an alcohol breathalyzer with speaker verification for people convicted of driving while intoxicated and a system that calls offenders on home detention at random times during the day.Speaker verification also controls telephone calls made by incarcerated felons. Inmates place a lot of calls. In 1994, US telecommunications services providers made $1.5 billion on outbound calls from inmates. Most inmates have restrictions on whom they can call. Speaker verification ensures that an inmate is not using another inmate‟s PIN to make a forbidden contact.3.5 Time and AttendanceTime and attendance applications are a small but growing segment of the speaker-verification market. SOC Credit Union in Michigan has used speaker verification for time and attendance monitoring of part-time employees for several years. Like many others, SOC Credit Union first deployed speaker verification for security and later extended it to time and attendance monitoring for part-time employees.4. StandardsThis paper concludes with a short discussion of application programming interface (API) standards. An API contains the function calls that enable programmers to use speaker-verification to create a product or application. Until April 1997, when the Speaker Verification API (SV API) standard was introduced, all available APIs for biometric products were proprietary. SV API remains the only API standard covering a specific biometric. It is now being incorporated into proposed generic biometric API standards. SV API was developed by a cross-section of speaker-recognition vendors, consultants, and end-user organizations to address a spectrum of needs and to support a broad range of product features. Because it supports both high level functions (e.g. calls to enrol) and low level functions (e.g. choices of audio input features) itfacilitates development of different types of applications by both novice and experienced developers.Why is it important to support API standards? Developers using a product with a proprietary API face difficult choices if the vendor of that product goes out of business, fails to support its product, or does not keep pace with technological advances. One of those choices is to rebuild the application from scratch using a different product. Given the same events, developers using a SV API-compliant product can select another compliant vendor and need perform far fewer modifications. Consequently, SV API makes development with speaker verification less risky and less costly. The advent of generic biometric API standards further facilitates integration of speaker verification with other biometrics. All of this helps speaker-verification vendors because it fosters growth in the marketplace. In the final analysis active support of API standards by developers and vendors benefits everyone.附录B 中文翻译说话人识别作者:Judith A. Markowitz, J. Markowitz Consultants 说话人识别是用一个人的语音特征来辨认或确认这个人。
基于智能语音识别技术的语音翻译系统设计
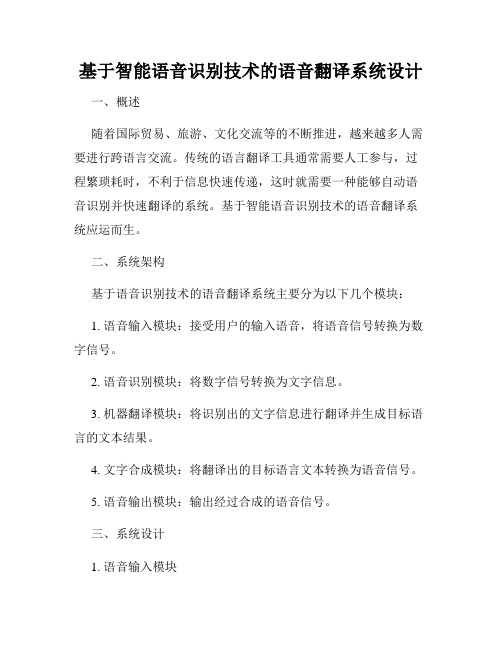
基于智能语音识别技术的语音翻译系统设计一、概述随着国际贸易、旅游、文化交流等的不断推进,越来越多人需要进行跨语言交流。
传统的语言翻译工具通常需要人工参与,过程繁琐耗时,不利于信息快速传递,这时就需要一种能够自动语音识别并快速翻译的系统。
基于智能语音识别技术的语音翻译系统应运而生。
二、系统架构基于语音识别技术的语音翻译系统主要分为以下几个模块:1. 语音输入模块:接受用户的输入语音,将语音信号转换为数字信号。
2. 语音识别模块:将数字信号转换为文字信息。
3. 机器翻译模块:将识别出的文字信息进行翻译并生成目标语言的文本结果。
4. 文字合成模块:将翻译出的目标语言文本转换为语音信号。
5. 语音输出模块:输出经过合成的语音信号。
三、系统设计1. 语音输入模块语音输入模块是语音翻译系统的输入途径,主要用于接收用户的语音指令。
在语音输入模块中,将使用麦克风采集用户的语音信号,并将其转换为数字信号。
数字信号采样频率和量化位数对语音识别的准确度有很大的影响,通常采用16kHz以上的采样频率和16位量化位数。
2. 语音识别模块语音识别模块是语音翻译系统的核心模块,用于将用户输入的语音信号转换为可识别的文本信息。
常用的语音识别技术有隐马尔可夫模型、循环神经网络、卷积神经网络等,其中最常用的是隐马尔可夫模型。
在语音识别模块中,将会对所有能够被识别的语音进行建模,使得系统可以通过比对来判断用户输入的语音信号所属的文本种类。
3. 机器翻译模块机器翻译模块是语音翻译系统的翻译核心模块,用于将用户输入的文本信息翻译成目标语言的文本结果。
通常采用的机器翻译算法有基于规则的机器翻译、统计机器翻译和神经网络机器翻译等,目前最常用的是神经网络机器翻译。
在机器翻译模块中,需要调用前端处理程序对用户输入的文本信息进行预处理,例如分词等,以提高翻译的准确度。
4. 文字合成模块文字合成模块是将翻译出的目标语言文本转换为语音信号的核心模块。
音频信号处理博士论文中英文资料外文翻译文献

音频信号处理博士论文中英文资料外文翻
译文献
音频信号处理是一个广泛研究的领域,涉及到音频信号的获取、分析、传输和处理等方面。
本文翻译了以下两篇外文文献,为音频
信号处理博士论文的写作提供参考。
文献一:Title of Paper One
作者:
摘要:
该篇文献提出了一种新的音频信号处理算法,旨在改善音频信
号的质量和增强用户对音乐的感受。
通过对音频信号进行特征提取
和分析,该算法能够有效地消除噪音和失真,并提供更清晰、更丰
富的音频体验。
文献介绍了算法的原理和实现方式,并通过实验验
证了其在不同音频数据集上的有效性。
文献二:Title of Paper Two
作者:
摘要:
该篇文献探讨了音频信号处理领域的一个重要问题,即语音识
别的准确性和鲁棒性。
通过分析现有的语音识别算法,文献指出了
当前算法存在的一些问题,并提出了一种改进的方法。
该方法基于
深度研究和卷积神经网络,并通过对音频信号进行多层次的特征研
究和表示研究,提高了语音识别的准确性和鲁棒性。
文献还介绍了
该方法的实验结果,并与其他算法进行了比较。
总结
这两篇外文文献介绍了音频信号处理领域的一些重要研究进展
和算法。
它们提供了宝贵的参考和借鉴,可以在音频信号处理博士
论文的写作中起到指导作用。
通过综合运用这些研究成果,我们可
以进一步改进音频信号处理算法,提高音频信号的质量和用户体验。
智能交通系统中英文对照外文翻译文献
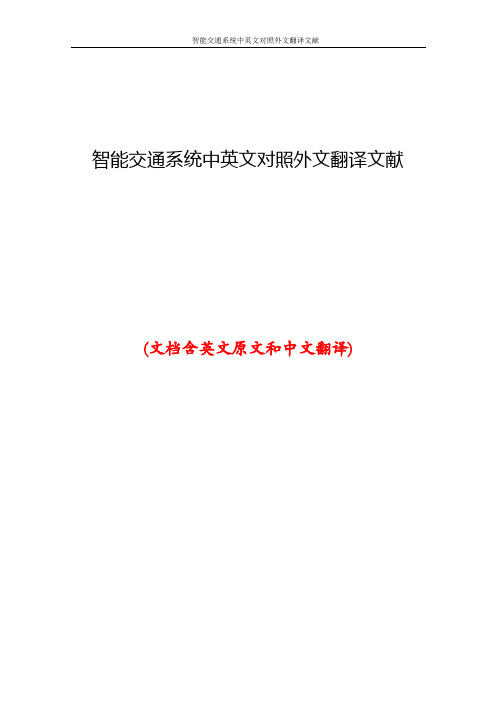
智能交通系统中英文对照外文翻译文献(文档含英文原文和中文翻译)原文:Traffic Assignment Forecast Model Research in ITS IntroductionThe intelligent transportation system (ITS) develops rapidly along with the city sustainable development, the digital city construction and the development of transportation. One of the main functions of the ITS is to improve transportation environment and alleviate the transportation jam, the most effective method to gain the aim is to forecast the traffic volume of the local network and the important nodes exactly with GIS function of path analysis and correlation mathematic methods, and this will lead a better planning of the traffic network. Traffic assignment forecast is an important phase of traffic volume forecast. It will assign the forecasted traffic to every way in the traffic sector. If the traffic volume of certain road is too big, which would bring on traffic jam, planners must consider the adoption of new roads or improving existing roads to alleviate the traffic congestion situation. This study attempts to present an improved traffic assignment forecast model, MPCC, based on analyzing the advantages and disadvantages of classic traffic assignment forecast models, and test the validity of the improved model in practice.1 Analysis of classic models1.1 Shortcut traffic assignmentShortcut traffic assignment is a static traffic assignment method. In this method, the traffic load impact in the vehicles’ travel is not considered, and the traffic impedance (travel time) is a constant. The traffic volume of every origination-destination couple will be assigned to the shortcut between the origination and destination, while the traffic volume of other roads in this sector is null. This assignment method has the advantage of simple calculation; however, uneven distribution of the traffic volume is its obvious shortcoming. Using this assignment method, the assignment traffic volume will be concentrated on the shortcut, which isobviously not realistic. However, shortcut traffic assignment is the basis of all theother traffic assignment methods.1.2 Multi-ways probability assignmentIn reality, travelers always want to choose the shortcut to the destination, whichis called the shortcut factor; however, as the complexity of the traffic network, thepath chosen may not necessarily be the shortcut, which is called the random factor.Although every traveler hopes to follow the shortcut, there are some whose choice isnot the shortcut in fact. The shorter the path is, the greater the probability of beingchosen is; the longer the path is, the smaller the probability of being chosen is.Therefore, the multi-ways probability assignment model is guided by the LOGIT model:∑---=n j ii i F F p 1)exp()exp(θθ (1)Where i p is the probability of the path section i; i F is the travel time of thepath section i; θ is the transport decision parameter, which is calculated by the followprinciple: firstly, calculate the i p with different θ (from 0 to 1), then find the θwhich makes i p the most proximate to the actual i p .The shortcut factor and the random factor is considered in multi-ways probabilityassignment, therefore, the assignment result is more reasonable, but the relationshipbetween traffic impedance and traffic load and road capacity is not considered in thismethod, which leads to the assignment result is imprecise in more crowded trafficnetwork. We attempt to improve the accuracy through integrating the several elements above in one model-MPCC.2 Multi-ways probability and capacity constraint model2.1 Rational path aggregateIn order to make the improved model more reasonable in the application, theconcept of rational path aggregate has been proposed. The rational path aggregate,which is the foundation of MPCC model, constrains the calculation scope. Rationalpath aggregate refers to the aggregate of paths between starts and ends of the trafficsector, defined by inner nodes ascertained by the following rules: the distancebetween the next inner node and the start can not be shorter than the distance betweenthe current one and the start; at the same time, the distance between the next innernode and the end can not be longer than the distance between the current one and theend. The multi-ways probability assignment model will be only used in the rationalpath aggregate to assign the forecast traffic volume, and this will greatly enhance theapplicability of this model.2.2 Model assumption1) Traffic impedance is not a constant. It is decided by the vehicle characteristicand the current traffic situation.2) The traffic impedance which travelers estimate is random and imprecise.3) Every traveler chooses the path from respective rational path aggregate.Based on the assumptions above, we can use the MPCC model to assign thetraffic volume in the sector of origination-destination couples.2.3 Calculation of path traffic impedanceActually, travelers have different understanding to path traffic impedance, butgenerally, the travel cost, which is mainly made up of forecast travel time, travellength and forecast travel outlay, is considered the traffic impedance. Eq. (2) displaysthis relationship. a a a a F L T C γβα++= (2)Where a C is the traffic impedance of the path section a; a T is the forecast traveltime of the path section a; a L is the travel length of the path section a; a F is theforecast travel outlay of the path section a; α, β, γ are the weight value of that threeelements which impact the traffic impedance. For a certain path section, there aredifferent α, β and γ value for different vehicles. We can get the weighted average of α,β and γ of each path section from the statistic percent of each type of vehicle in thepath section.2.4 Chosen probability in MPCCActually, travelers always want to follow the best path (broad sense shortcut), butbecause of the impact of random factor, travelers just can choose the path which is ofthe smallest traffic impedance they estimate by themselves. It is the key point ofMPCC. According to the random utility theory of economics, if traffic impedance is considered as the negativeutility, the chosen probability rs p of origination-destinationpoints couple (r, s) should follow LOGIT model:∑---=n j jrs rs bC bC p 1)exp()exp( (3) where rs p is the chosen probability of the pathsection (r, s);rs C is the traffic impedance of the path sect-ion (r, s); j C is the trafficimpedance of each path section in the forecast traffic sector; b reflects the travelers’cognition to the traffic impedance of paths in the traffic sector, which has reverseratio to its deviation. If b → ∞ , the deviation of understanding extent of trafficimpedance approaches to 0. In this case, all the travelers will follow the path whichis of the smallest traffic impedance, which equals to the assignment results withShortcut Traffic Assignment. Contrarily, if b → 0, travelers ’ understanding error approaches infinity. In this case, the paths travelers choose are scattered. There is anobjection that b is of dimension in Eq.(3). Because the deviation of b should beknown before, it is difficult to determine the value of b. Therefore, Eq.(3) is improvedas follows:∑---=n j OD j OD rsrs C bC C bC p 1)exp()exp(,∑-=n j j OD C n C 11(4) Where OD C is the average of the traffic impedance of all the as-signed paths; bwhich is of no dimension, just has relationship to the rational path aggregate, ratherthan the traffic impedance. According to actual observation, the range of b which is anexperience value is generally between 3.00 to 4.00. For the more crowded cityinternal roads, b is normally between 3.00 and 3.50.2.5 Flow of MPCCMPCC model combines the idea of multi-ways probability assignment anditerative capacity constraint traffic assignment.Firstly, we can get the geometric information of the road network and OD trafficvolume from related data. Then we determine the rational path aggregate with themethod which is explained in Section 2.1.Secondly, we can calculate the traffic impedance of each path section with Eq.(2),Fig.1 Flowchart of MPCC which is expatiated in Section 2.3.Thirdly, on the foundation of the traffic impedance of each path section, we cancalculate the respective forecast traffic volume of every path section with improvedLOGIT model (Eq.(4)) in Section 2.4, which is the key point of MPCC.Fourthly, through the calculation processabove, we can get the chosen probability andforecast traffic volume of each path section, but itis not the end. We must recalculate the trafficimpedance again in the new traffic volumesituation. As is shown in Fig.1, because of theconsideration of the relationship between trafficimpedance and traffic load, the traffic impedanceand forecast assignment traffic volume of everypath will be continually amended. Using therelationship model between average speed andtraffic volume, we can calculate the travel timeand the traffic impedance of certain path sect-ionunder different traffic volume situation. For theroads with different technical levels, therelationship models between average speeds totraffic volume are as follows: 1) Highway: 1082.049.179AN V = (5) 2) Level 1 Roads: 11433.084.155AN V = (6) 3) Level 2 Roads: 66.091.057.112AN V = (7) 4) Level 3 Roads: 3.132.01.99AN V = (8) 5) Level 4 Roads: 0988.05.70A N V =(9) Where V is the average speed of the path section; A N is the traffic volume of thepath section.At the end, we can repeat assigning traffic volume of path sections with themethod in previous step, which is the idea of iterative capacity constraint assignment,until the traffic volume of every path section is stable.译文智能交通交通量分配预测模型介绍随着城市的可持续化发展、数字化城市的建设以及交通运输业的发展,智能交通系统(ITS)的发展越来越快。
语音识别中英文对照外文翻译文献

中英文资料对照外文翻译(文档含英文原文和中文翻译)Speech Recognition1 Defining the ProblemSpeech recognition is the process of converting an acoustic signal, captured by a microphone or a telephone, to a set of words. The recognized words can be the final results, as for applications such as commands & control, data entry, and document preparation. They can also serve as the input to further linguistic processing in order to achieve speech understanding, a subject covered in section.Speech recognition systems can be characterized by many parameters, some of the more important of which are shown in Figure. An isolated-word speech recognition system requires that the speaker pause briefly between words, whereas a continuous speech recognition system does not. Spontaneous, or extemporaneously generated, speech contains disfluencies, and is much more difficult to recognize than speech read from script. Some systems require speaker enrollment---a user must provide samples of his or her speech before using them, whereas other systems are said to be speaker-independent, in that no enrollment is necessary. Some of the other parameters depend on the specific task. Recognition is generally more difficult when vocabularies are large or have many similar-sounding words. When speech is produced in a sequence of words, language models or artificial grammars are used to restrict the combination of words.The simplest language model can be specified as a finite-state network, where the1permissible words following each word are given explicitly. More general language models approximating natural language are specified in terms of a context-sensitive grammar.One popular measure of the difficulty of the task, combining the vocabulary size and the language model, is perplexity, loosely defined as the geometric mean of the number of words that can follow a word after the language model has been applied (see section for a discussion of language modeling in general and perplexity in particular). Finally, there are some external parameters that can affect speech recognition system performance, including the characteristics of the environmental noise and the type and the placement of the microphone.Table: Typical parameters used to characterize the capability of speech recognition systems Speech recognition is a difficult problem, largely because of the many sources of variability associated with the signal. First, the acoustic realizations of phonemes, the smallest sound units of which words are composed, are highly dependent on the context in which they appear. These phonetic variabilities are exemplified by the acoustic differences of the phoneme,At word boundaries, contextual variations can be quite dramatic---making gas shortage sound like gash shortage in American English, and devo andare sound like devandare in Italian.Second, acoustic variabilities can result from changes in the environment as well as in the position and characteristics of the transducer. Third, within-speaker variabilities can result from changes in the speaker's physical and emotional state, speaking rate, or voice quality. Finally, differences in sociolinguistic background, dialect, and vocal tract size and shape can contribute to across-speaker variabilities.Figure shows the major components of a typical speech recognition system. The digitized speech signal is first transformed into a set of useful measurements or features at a fixed rate, typically once every 10--20 msec (see sectionsand 11.3 for signal representation and digital signal processing, respectively). These measurements are then used to search for the most likely word candidate, making use of constraints imposed by the acoustic, lexical, and language models. Throughout this process, training data are used to determine the values of the model parameters.Figure: Components of a typical speech recognition system.Speech recognition systems attempt to model the sources of variability described above in several ways. At the level of signal representation, researchers have developed representations that emphasize perceptually important speaker-independent features of the signal, and de-emphasize speaker-dependent characteristics. At the acoustic phonetic level, speaker variability is typically modeled using statistical techniques applied to large amounts of data. Speaker adaptation algorithms have also been developed that adapt speaker-independent acoustic models to those of the current speaker during system use, (see section). Effects of linguistic context at the acoustic phonetic level are typically handled by training separate models for phonemes in different contexts; this is called context dependent acoustic modeling.Word level variability can be handled by allowing alternate pronunciations of words in representations known as pronunciation networks. Common alternate pronunciations of words, as well as effects of dialect and accent are handled by allowing search algorithms to find alternate paths of phonemes through these networks. Statistical language models, based on estimates of the frequency of occurrence of word sequences, are often used to guide the searchthrough the most probable sequence of words.The dominant recognition paradigm in the past fifteen years is known as hidden Markov models (HMM). An HMM is a doubly stochastic model, in which the generation of the underlying phoneme string and the frame-by-frame, surface acoustic realizations are both represented probabilistically as Markov processes, as discussed in sections,and 11.2. Neural networks have also been used to estimate the frame based scores; these scores are then integrated into HMM-based system architectures, in what has come to be known as hybrid systems, as described in section 11.5.An interesting feature of frame-based HMM systems is that speech segments are identified during the search process, rather than explicitly. An alternate approach is to first identify speech segments, then classify the segments and use the segment scores to recognize words. This approach has produced competitive recognition performance in several tasks.2 State of the ArtComments about the state-of-the-art need to be made in the context of specific applications which reflect the constraints on the task. Moreover, different technologies are sometimes appropriate for different tasks. For example, when the vocabulary is small, the entire word can be modeled as a single unit. Such an approach is not practical for large vocabularies, where word models must be built up from subword units.Performance of speech recognition systems is typically described in terms of word error rate E, defined as:where N is the total number of words in the test set, and S, I, and D are the total number of substitutions, insertions, and deletions, respectively.The past decade has witnessed significant progress in speech recognition technology. Word error rates continue to drop by a factor of 2 every two years. Substantial progress has been made in the basic technology, leading to the lowering of barriers to speaker independence, continuous speech, and large vocabularies. There are several factors that have contributed to this rapid progress. First, there is the coming of age of the HMM. HMM is powerful in that, with the availability of training data, the parameters of the model can be trained automatically to giveoptimal performance.Second, much effort has gone into the development of large speech corpora for system development, training, and testing. Some of these corpora are designed for acoustic phonetic research, while others are highly task specific. Nowadays, it is not uncommon to have tens of thousands of sentences available for system training and testing. These corpora permit researchers to quantify the acoustic cues important for phonetic contrasts and to determine parameters of the recognizers in a statistically meaningful way. While many of these corpora (e.g., TIMIT, RM, ATIS, and WSJ; see section 12.3) were originally collected under the sponsorship of the U.S. Defense Advanced Research Projects Agency (ARPA) to spur human language technology development among its contractors, they have nevertheless gained world-wide acceptance (e.g., in Canada, France, Germany, Japan, and the U.K.) as standards on which to evaluate speech recognition.Third, progress has been brought about by the establishment of standards for performance evaluation. Only a decade ago, researchers trained and tested their systems using locally collected data, and had not been very careful in delineating training and testing sets. As a result, it was very difficult to compare performance across systems, and a system's performance typically degraded when it was presented with previously unseen data. The recent availability of a large body of data in the public domain, coupled with the specification of evaluation standards, has resulted in uniform documentation of test results, thus contributing to greater reliability in monitoring progress (corpus development activities and evaluation methodologies are summarized in chapters 12 and 13 respectively).Finally, advances in computer technology have also indirectly influenced our progress. The availability of fast computers with inexpensive mass storage capabilities has enabled researchers to run many large scale experiments in a short amount of time. This means that the elapsed time between an idea and its implementation and evaluation is greatly reduced. In fact, speech recognition systems with reasonable performance can now run in real time using high-end workstations without additional hardware---a feat unimaginable only a few years ago.One of the most popular, and potentially most useful tasks with low perplexity (PP=11) is the recognition of digits. For American English, speaker-independent recognition of digit strings spoken continuously and restricted to telephone bandwidth can achieve an error rate of 0.3% when the string length is known.One of the best known moderate-perplexity tasks is the 1,000-word so-called Resource Management (RM) task, in which inquiries can be made concerning various naval vessels in the Pacific ocean. The best speaker-independent performance on the RM task is less than 4%, using a word-pair language model that constrains the possible words following a given word (PP=60). More recently, researchers have begun to address the issue of recognizing spontaneously generated speech. For example, in the Air Travel Information Service (ATIS) domain, word error rates of less than 3% has been reported for a vocabulary of nearly 2,000 words and a bigram language model with a perplexity of around 15.High perplexity tasks with a vocabulary of thousands of words are intended primarily for the dictation application. After working on isolated-word, speaker-dependent systems for many years, the community has since 1992 moved towards very-large-vocabulary (20,000 words and more), high-perplexity (PP≈200), speaker-independent, continuous speech recognition. The best system in 1994 achieved an error rate of 7.2% on read sentences drawn from North America business news.With the steady improvements in speech recognition performance, systems are now being deployed within telephone and cellular networks in many countries. Within the next few years, speech recognition will be pervasive in telephone networks around the world. There are tremendous forces driving the development of the technology; in many countries, touch tone penetration is low, and voice is the only option for controlling automated services. In voice dialing, for example, users can dial 10--20 telephone numbers by voice (e.g., call home) after having enrolled their voices by saying the words associated with telephone numbers. AT&T, on the other hand, has installed a call routing system using speaker-independent word-spotting technology that can detect a few key phrases (e.g., person to person, calling card) in sentences such as: I want to charge it to my calling card.At present, several very large vocabulary dictation systems are available for document generation. These systems generally require speakers to pause between words. Their performance can be further enhanced if one can apply constraints of the specific domain such as dictating medical reports.Even though much progress is being made, machines are a long way from recognizing conversational speech. Word recognition rates on telephone conversations in the Switchboard corpus are around 50%. It will be many years before unlimited vocabulary, speaker-independentcontinuous dictation capability is realized.3 Future DirectionsIn 1992, the U.S. National Science Foundation sponsored a workshop to identify the key research challenges in the area of human language technology, and the infrastructure needed to support the work. The key research challenges are summarized in. Research in the following areas for speech recognition were identified:Robustness:In a robust system, performance degrades gracefully (rather than catastrophically) as conditions become more different from those under which it was trained. Differences in channel characteristics and acoustic environment should receive particular attention.Portability:Portability refers to the goal of rapidly designing, developing and deploying systems for new applications. At present, systems tend to suffer significant degradation when moved to a new task. In order to return to peak performance, they must be trained on examples specific to the new task, which is time consuming and expensive.Adaptation:How can systems continuously adapt to changing conditions (new speakers, microphone, task, etc) and improve through use? Such adaptation can occur at many levels in systems, subword models, word pronunciations, language models, etc.Language Modeling:Current systems use statistical language models to help reduce the search space and resolve acoustic ambiguity. As vocabulary size grows and other constraints are relaxed to create more habitable systems, it will be increasingly important to get as much constraint as possible from language models; perhaps incorporating syntactic and semantic constraints that cannot be captured by purely statistical models.Confidence Measures:Most speech recognition systems assign scores to hypotheses for the purpose of rank ordering them. These scores do not provide a good indication of whether a hypothesis is correct or not, just that it is better than the other hypotheses. As we move to tasks that require actions,we need better methods to evaluate the absolute correctness of hypotheses.Out-of-Vocabulary Words:Systems are designed for use with a particular set of words, but system users may not know exactly which words are in the system vocabulary. This leads to a certain percentage of out-of-vocabulary words in natural conditions. Systems must have some method of detecting such out-of-vocabulary words, or they will end up mapping a word from the vocabulary onto the unknown word, causing an error.Spontaneous Speech:Systems that are deployed for real use must deal with a variety of spontaneous speech phenomena, such as filled pauses, false starts, hesitations, ungrammatical constructions and other common behaviors not found in read speech. Development on the ATIS task has resulted in progress in this area, but much work remains to be done.Prosody:Prosody refers to acoustic structure that extends over several segments or words. Stress, intonation, and rhythm convey important information for word recognition and the user's intentions (e.g., sarcasm, anger). Current systems do not capture prosodic structure. How to integrate prosodic information into the recognition architecture is a critical question that has not yet been answered.Modeling Dynamics:Systems assume a sequence of input frames which are treated as if they were independent. But it is known that perceptual cues for words and phonemes require the integration of features that reflect the movements of the articulators, which are dynamic in nature. How to model dynamics and incorporate this information into recognition systems is an unsolved problem.语音识别一定义问题语音识别是指音频信号的转换过程,被电话或麦克风的所捕获的一系列的消息。
外文翻译---统一消息系统

附录A 译文统一消息系统所有用户生来都不是一样的。
您的销售人员花一整天时间在手机上和保持以生产力。
如果他们疲惫于语音邮件,从而错过任何一个电话,这可能意味着其收入的损失。
在另一方面,知识型工人由于他们花太多时间在手机上而遭受折磨。
对于他们来说,生产力与在电话上花的小时数成反比。
为了满足双方和那些在此之间的用户的需要,我们期待一个统一的信息系统。
一度,UM简单意味着将电子邮件,传真邮件和语音邮件放到一个邮箱进行管理和操纵。
今天,我们通过电话用户界面,根据一个用户的操作,把更多的实时通信系统,包括即时消息( IM ),现场管理和呼叫路由规则的使用集成在一起。
这听起来可能很繁琐,但供应商将这些功能分开出售,使您的组织可以选择需要的部分。
根据Radicati组织的调查,UC(统一通信)促成了企业的健康增长。
支持融合技术,如VoIP和SIP (会话发起协议),将促进收益从2005年的4.69亿美元增长到2009年的9.39亿美元,其中包括PBX和电话供应商,通讯软件,语音系统,甚至业务流程系统供应商。
为了赶上这股浪潮,我们要求供应商向我们提供他们的SIP兼容产品。
我们希望每个产品与开放原始码的IP PBX在SIP的信令模式下工作并进行测试。
我们的理论依据是PBX上购买已接近生命周期结束的Y2K进行升级,企业期待考虑能结合现有资源的IP PBX从而提升他们的电话系统。
这使得支持SIP的IP PBX 成为一张热门车票。
SIP在终端使用更多的智能化处理,如IP电话和个人电脑,能迅速整合业务应用,电子邮件服务器,目录,甚至实时的UC申请。
此外,基于IP和SIP开放式标准的产品将缩短开发时间。
我们还规定,产品必须支持一个通用电子邮件信箱,语音邮件和传真;TTS (文本语音)被用来管理一个通用邮箱,并使用其它各种各样的电子邮件协议,如IMAP, MAPI, POP3和SMTP ;和Active Directory或LDAP 。
外文文献翻译译稿和原文
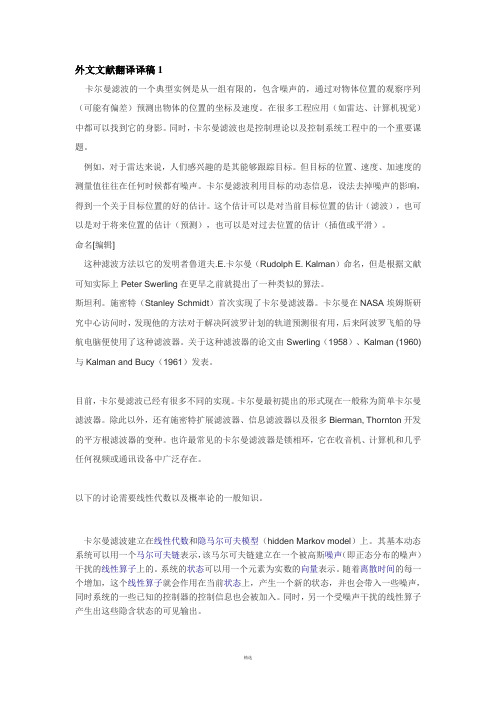
外文文献翻译译稿1卡尔曼滤波的一个典型实例是从一组有限的,包含噪声的,通过对物体位置的观察序列(可能有偏差)预测出物体的位置的坐标及速度。
在很多工程应用(如雷达、计算机视觉)中都可以找到它的身影。
同时,卡尔曼滤波也是控制理论以及控制系统工程中的一个重要课题。
例如,对于雷达来说,人们感兴趣的是其能够跟踪目标。
但目标的位置、速度、加速度的测量值往往在任何时候都有噪声。
卡尔曼滤波利用目标的动态信息,设法去掉噪声的影响,得到一个关于目标位置的好的估计。
这个估计可以是对当前目标位置的估计(滤波),也可以是对于将来位置的估计(预测),也可以是对过去位置的估计(插值或平滑)。
命名[编辑]这种滤波方法以它的发明者鲁道夫.E.卡尔曼(Rudolph E. Kalman)命名,但是根据文献可知实际上Peter Swerling在更早之前就提出了一种类似的算法。
斯坦利。
施密特(Stanley Schmidt)首次实现了卡尔曼滤波器。
卡尔曼在NASA埃姆斯研究中心访问时,发现他的方法对于解决阿波罗计划的轨道预测很有用,后来阿波罗飞船的导航电脑便使用了这种滤波器。
关于这种滤波器的论文由Swerling(1958)、Kalman (1960)与Kalman and Bucy(1961)发表。
目前,卡尔曼滤波已经有很多不同的实现。
卡尔曼最初提出的形式现在一般称为简单卡尔曼滤波器。
除此以外,还有施密特扩展滤波器、信息滤波器以及很多Bierman, Thornton开发的平方根滤波器的变种。
也许最常见的卡尔曼滤波器是锁相环,它在收音机、计算机和几乎任何视频或通讯设备中广泛存在。
以下的讨论需要线性代数以及概率论的一般知识。
卡尔曼滤波建立在线性代数和隐马尔可夫模型(hidden Markov model)上。
其基本动态系统可以用一个马尔可夫链表示,该马尔可夫链建立在一个被高斯噪声(即正态分布的噪声)干扰的线性算子上的。
系统的状态可以用一个元素为实数的向量表示。
- 1、下载文档前请自行甄别文档内容的完整性,平台不提供额外的编辑、内容补充、找答案等附加服务。
- 2、"仅部分预览"的文档,不可在线预览部分如存在完整性等问题,可反馈申请退款(可完整预览的文档不适用该条件!)。
- 3、如文档侵犯您的权益,请联系客服反馈,我们会尽快为您处理(人工客服工作时间:9:00-18:30)。
中英文资料对照外文翻译Speech Recognition1 Defining the ProblemSpeech recognition is the process of converting an acoustic signal, captured by a microphone or a telephone, to a set of words. The recognized words can be the final results, as for applications such as commands & control, data entry, and document preparation. They can also serve as the input to further linguistic processing in order to achieve speech understanding, a subject covered in section.Speech recognition systems can be characterized by many parameters, some of the more important of which are shown in Figure. An isolated-word speech recognition system requires that the speaker pause briefly between words, whereas a continuous speech recognition system does not. Spontaneous, or extemporaneously generated, speech contains disfluencies, and is much more difficult to recognize than speech read from script. Some systems require speaker enrollment---a user must provide samples of his or her speech before using them, whereas other systems are said to be speaker-independent, in that no enrollment is necessary. Some of the other parameters depend on the specific task. Recognition is generally more difficult when vocabularies are large or have many similar-sounding words. When speech is produced in a sequence of words, language models or artificial grammars are used to restrict the combination of words.1The simplest language model can be specified as a finite-state network, where the permissible words following each word are given explicitly. More general language models approximating natural language are specified in terms of a context-sensitive grammar.One popular measure of the difficulty of the task, combining the vocabulary size and the language model, is perplexity, loosely defined as the geometric mean of the number of words that can follow a word after the language model has been applied (see section for a discussion of language modeling in general and perplexity in particular). Finally, there are some external parameters that can affect speech recognition system performance, including the characteristics of the environmental noise and the type and the placement of the microphone.Table: Typical parameters used to characterize the capability of speech recognition systems Speech recognition is a difficult problem, largely because of the many sources of variability associated with the signal. First, the acoustic realizations of phonemes, the smallest sound units of which words are composed, are highly dependent on the context in which they appear. These phonetic variabilities are exemplified by the acoustic differences of the phoneme,At word boundaries, contextual variations can be quite dramatic---making gas shortage sound like gash shortage in American English, and devo andare sound like devandare in Italian.Second, acoustic variabilities can result from changes in the environment as well as in the position and characteristics of the transducer. Third, within-speaker variabilities can result from changes in the speaker's physical and emotional state, speaking rate, or voice quality. Finally, differences in sociolinguistic background, dialect, and vocal tract size and shape can contributeto across-speaker variabilities.Figure shows the major components of a typical speech recognition system. The digitized speech signal is first transformed into a set of useful measurements or features at a fixed rate, typically once every 10--20 msec (see sectionsand 11.3 for signal representation and digital signal processing, respectively). These measurements are then used to search for the most likely word candidate, making use of constraints imposed by the acoustic, lexical, and language models. Throughout this process, training data are used to determine the values of the model parameters.Figure: Components of a typical speech recognition system.Speech recognition systems attempt to model the sources of variability described above in several ways. At the level of signal representation, researchers have developed representations that emphasize perceptually important speaker-independent features of the signal, and de-emphasize speaker-dependent characteristics. At the acoustic phonetic level, speaker variability is typically modeled using statistical techniques applied to large amounts of data. Speaker adaptation algorithms have also been developed that adapt speaker-independent acoustic models to those of the current speaker during system use, (see section). Effects of linguistic context at the acoustic phonetic level are typically handled by training separate models for phonemes in different contexts; this is called context dependent acoustic modeling.Word level variability can be handled by allowing alternate pronunciations of words in representations known as pronunciation networks. Common alternate pronunciations of words, as well as effects of dialect and accent are handled by allowing search algorithms to find alternate paths of phonemes through these networks. Statistical language models, based onestimates of the frequency of occurrence of word sequences, are often used to guide the search through the most probable sequence of words.The dominant recognition paradigm in the past fifteen years is known as hidden Markov models (HMM). An HMM is a doubly stochastic model, in which the generation of the underlying phoneme string and the frame-by-frame, surface acoustic realizations are both represented probabilistically as Markov processes, as discussed in sections,and 11.2. Neural networks have also been used to estimate the frame based scores; these scores are then integrated into HMM-based system architectures, in what has come to be known as hybrid systems, as described in section 11.5.An interesting feature of frame-based HMM systems is that speech segments are identified during the search process, rather than explicitly. An alternate approach is to first identify speech segments, then classify the segments and use the segment scores to recognize words. This approach has produced competitive recognition performance in several tasks.2 State of the ArtComments about the state-of-the-art need to be made in the context of specific applications which reflect the constraints on the task. Moreover, different technologies are sometimes appropriate for different tasks. For example, when the vocabulary is small, the entire word can be modeled as a single unit. Such an approach is not practical for large vocabularies, where word models must be built up from subword units.Performance of speech recognition systems is typically described in terms of word error rate E, defined as:where N is the total number of words in the test set, and S, I, and D are the total number of substitutions, insertions, and deletions, respectively.The past decade has witnessed significant progress in speech recognition technology. Word error rates continue to drop by a factor of 2 every two years. Substantial progress has been made in the basic technology, leading to the lowering of barriers to speaker independence, continuous speech, and large vocabularies. There are several factors that have contributed to this rapid progress. First, there is the coming of age of the HMM. HMM is powerful in that, with theavailability of training data, the parameters of the model can be trained automatically to give optimal performance.Second, much effort has gone into the development of large speech corpora for system development, training, and testing. Some of these corpora are designed for acoustic phonetic research, while others are highly task specific. Nowadays, it is not uncommon to have tens of thousands of sentences available for system training and testing. These corpora permit researchers to quantify the acoustic cues important for phonetic contrasts and to determine parameters of the recognizers in a statistically meaningful way. While many of these corpora (e.g., TIMIT, RM, ATIS, and WSJ; see section 12.3) were originally collected under the sponsorship of the U.S. Defense Advanced Research Projects Agency (ARPA) to spur human language technology development among its contractors, they have nevertheless gained world-wide acceptance (e.g., in Canada, France, Germany, Japan, and the U.K.) as standards on which to evaluate speech recognition.Third, progress has been brought about by the establishment of standards for performance evaluation. Only a decade ago, researchers trained and tested their systems using locally collected data, and had not been very careful in delineating training and testing sets. As a result, it was very difficult to compare performance across systems, and a system's performance typically degraded when it was presented with previously unseen data. The recent availability of a large body of data in the public domain, coupled with the specification of evaluation standards, has resulted in uniform documentation of test results, thus contributing to greater reliability in monitoring progress (corpus development activities and evaluation methodologies are summarized in chapters 12 and 13 respectively).Finally, advances in computer technology have also indirectly influenced our progress. The availability of fast computers with inexpensive mass storage capabilities has enabled researchers to run many large scale experiments in a short amount of time. This means that the elapsed time between an idea and its implementation and evaluation is greatly reduced. In fact, speech recognition systems with reasonable performance can now run in real time using high-end workstations without additional hardware---a feat unimaginable only a few years ago.One of the most popular, and potentially most useful tasks with low perplexity (PP=11) is the recognition of digits. For American English, speaker-independent recognition of digit strings spoken continuously and restricted to telephone bandwidth can achieve an error rate of 0.3%when the string length is known.One of the best known moderate-perplexity tasks is the 1,000-word so-called Resource Management (RM) task, in which inquiries can be made concerning various naval vessels in the Pacific ocean. The best speaker-independent performance on the RM task is less than 4%, using a word-pair language model that constrains the possible words following a given word (PP=60). More recently, researchers have begun to address the issue of recognizing spontaneously generated speech. For example, in the Air Travel Information Service (ATIS) domain, word error rates of less than 3% has been reported for a vocabulary of nearly 2,000 words and a bigram language model with a perplexity of around 15.High perplexity tasks with a vocabulary of thousands of words are intended primarily for the dictation application. After working on isolated-word, speaker-dependent systems for many years, the community has since 1992 moved towards very-large-vocabulary (20,000 words and more), high-perplexity (PP≈200), speaker-independent, continuous speech recognition. The best system in 1994 achieved an error rate of 7.2% on read sentences drawn from North America business news.With the steady improvements in speech recognition performance, systems are now being deployed within telephone and cellular networks in many countries. Within the next few years, speech recognition will be pervasive in telephone networks around the world. There are tremendous forces driving the development of the technology; in many countries, touch tone penetration is low, and voice is the only option for controlling automated services. In voice dialing, for example, users can dial 10--20 telephone numbers by voice (e.g., call home) after having enrolled their voices by saying the words associated with telephone numbers. AT&T, on the other hand, has installed a call routing system using speaker-independent word-spotting technology that can detect a few key phrases (e.g., person to person, calling card) in sentences such as: I want to charge it to my calling card.At present, several very large vocabulary dictation systems are available for document generation. These systems generally require speakers to pause between words. Their performance can be further enhanced if one can apply constraints of the specific domain such as dictating medical reports.Even though much progress is being made, machines are a long way from recognizing conversational speech. Word recognition rates on telephone conversations in the Switchboardcorpus are around 50%. It will be many years before unlimited vocabulary, speaker-independent continuous dictation capability is realized.3 Future DirectionsIn 1992, the U.S. National Science Foundation sponsored a workshop to identify the key research challenges in the area of human language technology, and the infrastructure needed to support the work. The key research challenges are summarized in. Research in the following areas for speech recognition were identified:Robustness:In a robust system, performance degrades gracefully (rather than catastrophically) as conditions become more different from those under which it was trained. Differences in channel characteristics and acoustic environment should receive particular attention.Portability:Portability refers to the goal of rapidly designing, developing and deploying systems for new applications. At present, systems tend to suffer significant degradation when moved to a new task. In order to return to peak performance, they must be trained on examples specific to the new task, which is time consuming and expensive.Adaptation:How can systems continuously adapt to changing conditions (new speakers, microphone, task, etc) and improve through use? Such adaptation can occur at many levels in systems, subword models, word pronunciations, language models, etc.Language Modeling:Current systems use statistical language models to help reduce the search space and resolve acoustic ambiguity. As vocabulary size grows and other constraints are relaxed to create more habitable systems, it will be increasingly important to get as much constraint as possible from language models; perhaps incorporating syntactic and semantic constraints that cannot be captured by purely statistical models.Confidence Measures:Most speech recognition systems assign scores to hypotheses for the purpose of rank ordering them. These scores do not provide a good indication of whether a hypothesis is corrector not, just that it is better than the other hypotheses. As we move to tasks that require actions, we need better methods to evaluate the absolute correctness of hypotheses.Out-of-Vocabulary Words:Systems are designed for use with a particular set of words, but system users may not know exactly which words are in the system vocabulary. This leads to a certain percentage of out-of-vocabulary words in natural conditions. Systems must have some method of detecting such out-of-vocabulary words, or they will end up mapping a word from the vocabulary onto the unknown word, causing an error.Spontaneous Speech:Systems that are deployed for real use must deal with a variety of spontaneous speech phenomena, such as filled pauses, false starts, hesitations, ungrammatical constructions and other common behaviors not found in read speech. Development on the ATIS task has resulted in progress in this area, but much work remains to be done.Prosody:Prosody refers to acoustic structure that extends over several segments or words. Stress, intonation, and rhythm convey important information for word recognition and the user's intentions (e.g., sarcasm, anger). Current systems do not capture prosodic structure. How to integrate prosodic information into the recognition architecture is a critical question that has not yet been answered.Modeling Dynamics:Systems assume a sequence of input frames which are treated as if they were independent. But it is known that perceptual cues for words and phonemes require the integration of features that reflect the movements of the articulators, which are dynamic in nature. How to model dynamics and incorporate this information into recognition systems is an unsolved problem.语音识别一定义问题语音识别是指音频信号的转换过程,被电话或麦克风的所捕获的一系列的消息。