QTL-seq:用重测序方法进行数量性状基因座(QTL)定位的方法
QTL定位的原理和方法
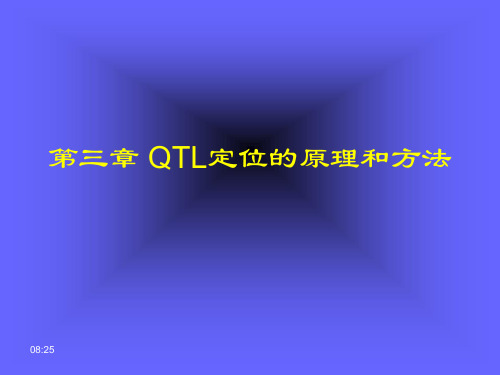
Fine Mapping Strategies
Genomewide-based strategies:
Large scale BC, F2, half sibs, etc. Recombinant inbred lines (RIL) Advanced Intercross Lines (AIL)
标 记 和
概 率
19:27
数据分析
• yij为具有QTL基因型 j的个体 i 的性状记录; • m j为具有QTL基因型 j的个体的期望效应(如 m d
或 m a ); • eij为随机误差,并且 eij ~ N (0, 2 ),因此有:
yij ~ N (mj , 2 )
19:27
– 1 LOD下降对应97%的QTL置信区间;
– 2 LOD下降对应99.8%的QTL置信区间;
19:27
19:27
Bootstrap置信区间
1. 对于一个大小为 n的群体,抽取 n个带有覆盖性
质的记录(有些记录被抽取多次,而有些记录 没被抽取);
2. 分析并估计QTL位置;
3. 重复上面的1和2两个过程,如200次或更多;
4. 在分布的两尾去掉2.5%的极端的QTL位置估计 值;
5. 剩余的95%表示置信区间的估计值。
19:27
QTL位置估计的置信区间
19:27
预测置信区间
• 置信区间的长度受样本大小、QTL效应和标记密 度的影响,对一个高密度标记图谱,Darvasi and Soller (1997)给出了一个预测的近似95%的置信 区间(单位cM):
19:27
19:27
多次检测问题
• 如果有许多独立的零假设被检验,而且事 先知道所有的零假设都为真,则,至少出 现一次假显著(false positive)的概率为
qtl定位的原理和方法

qtl定位的原理和方法QTL定位(定位关联分析)是一种现代分子遗传学研究中最受欢迎的方法之一,用于检测性状与基因位点之间的关联。
它采用大量的DNA样本进行分析,以定位具有显著影响的基因位点,以便将某个性状与其遗传控制位点联系起来。
下面将对QTL定位的原理和方法进行详细介绍:一、QTL定位的原理1、遗传距离QTL定位所使用的原理是双亲亲代遗传距离(PBT),即从一只父母中继承的遗传信息离该代性状之间的距离。
因此,在发现基因位点与性状间存在关联关系时,这些基因位点应该处于控制该性状的PBT之内。
2、显著的统计功能PBT表明,当两个或多个位点之间存在最大的相互影响时,它们应该被认为是具有可能共同控制某一性状的位点。
计算这些位点之间的关联关系时,QTL定位使用了高度灵活的统计功能来确定可能控制性状的位点。
3、方差分析QTL定位采用了ANOVA(方差分析)的方法对样本位点的遗传信息进行更精确的分析。
这将帮助我们更准确地定位具有良好关联关系的基因位点。
二、QTL定位的方法1、映射表首先,根据QTL定位要求,会有一张明确的映射表,用于建立基因位点与性状之间的关联关系。
该映射表由DNA分子标记(如微卫星)、RNA分子标记和细胞表面标记组成,用于确定基因位点的具体位置。
2、细胞表型接下来,需要拿出相关的细胞表型数据,如果是杂交的环境下,样本的性状可以用已知的表型数据及其位点来与受检样本进行比较。
3、统计分析最后,使用统计分析来研究基因位点与性状之间的关联关系,以确定突变可能使性状发生变化的基因位点。
总之,QTL定位是一种利用映射表、细胞表型和统计分析来定位可能与某个性状紧密关联的基因位点的重要方法。
它可用来帮助我们发现形态性状与遗传物质之间的关联,从而更好地了解遗传分析和分子基因组学。
QTL定位的研究方法

QTL定位的研究方法随着科学技术的发展,越来越多的研究者开始关注基因座上的数量性状变异,而QTL(Quantitative Trait Locus,数量性状基因座)定位就成为了一种重要的研究方法。
QTL定位的研究方法可以帮助我们了解数量性状的遗传基础,从而更好地进行遗传改良和育种工作。
本文将介绍几种常用的QTL定位研究方法。
一、关联分析法关联分析法是一种基于群体中遗传变异与表型变异之间的关系进行研究的方法。
通过对大量个体的基因型和表型数据进行统计分析,可以找到基因座与数量性状之间的关联。
常见的关联分析方法包括单点分析和多点分析。
单点分析是一种最简单的关联分析方法,它将一个个基因座与数量性状逐一进行关联分析,从而确定与数量性状相关的基因座。
然而,由于单点分析忽略了多个基因座之间的相互作用,因此其精度和效果有一定的限制。
为了克服单点分析的局限性,人们提出了多点分析方法。
多点分析可以同时考虑多个基因座之间的相互关系,从而更准确地确定与数量性状相关的基因座。
常见的多点分析方法包括QTL mapping、Linkage Disequilibrium Mapping等。
二、连锁图法连锁图法是一种基于基因座间连锁关系的QTL定位方法。
通过构建连锁图,可以确定数量性状基因座与已知基因座之间的连锁关系,进而找到与数量性状相关的基因座。
连锁图的构建可以通过遗传标记的信息来实现,比如通过分子标记技术得到的SNP(Single Nucleotide Polymorphism,单核苷酸多态性)或SSR(Simple Sequence Repeat,简单序列重复)等。
通过对大量个体的遗传标记和数量性状进行测定和分析,可以确定基因座之间的连锁关系。
三、关键位点法关键位点法是一种基于候选基因的QTL定位方法。
该方法通过先验知识或基因功能等因素,选择可能与数量性状相关的候选基因,并通过对这些候选基因进行测定和分析,确定与数量性状相关的基因座。
QTL 定位方法---

QTL 定位方法分子标记技术和数量遗传学的发展,使得分子遗传学与数量遗传学相互渗透和融合,从而形成了一个新的研究领域—分子数量遗传学(Molecular Quantitative Genetics)。
分子数量遗传学研究的内容,就是借助分子标记,采用适当的统计分析方法明确QTL 在染色体上的位置及其效应。
而QTL 定位的原理是:利用适当的分离群体,构建较高密度的、分布较均匀的、覆盖全基因组的分子标记连锁图。
根据遗传连锁的基本遗传学原理,对分离群体中单株的标记基因型和性状的表型值进行一定的统计分析,将决定数量性状的QTL 定位在分子标记连锁图中。
目前,QTL 定位的方法主要有单标记分析法(Edwards et al, 1987),区间作图法(Lander and Botstein, 1989)和复合区间作图法(Zeng, 1994)等。
单标记法(Single marker analysis)是最简单的分析标记与性状关联的方法,包括以标记为基础的分析方法(Marker-based analysis, MBA)和以性状为基础的分析方法(Trait-based analysis, TBA,Lebowitz et al., 1987)。
前者利用每个标记位点不同基因型间的性状均值差异,以传统的单因素方差分析法测验被研究的数量性状在标记基因型间的差异显著性。
对于一个作图群体而言,任意标记位点具有三种基因型(F2群体)或两种基因型(回交群体、重组自交系、双单倍体系),分析每一基因型个体的数量性状均值的差异,并进行F 测验,当F 测验显著时,则表明该标记位点可能与一个或多个QTL 连锁。
利用这种方法进行的数量性状分析,既简单又符合QTL 定位的基本统计原理,且不需要完整的分子标记连锁图,是定位QTL 的最为有效方法。
但不足之处是不能准确估计QTL 的位置,且往往会低估其遗传效应。
以性状为基础的分析方法的原理是假定因选择而使数量性状的高表型个体中的QTL 增效等位基因和低表型个体中的QTL 减效等位基因的频率增加,当QTL 的等位基因与某一标记基因连锁时,会因相互关联而导致高、低表型个体间标记基因频率的差异。
数量性状基因座(QTL)定位的原理及研究进展

利用染色体上1个QTL两侧的各1对标记,建立个体数量性 状观察值对双侧标记基因型指示变量的线性回归关系,以 分离检验统计量中重组率和QTL效应;
其统计原理是对基因组上两邻近分子标记间,按一定遗 传距离的片段逐一分析。
优点
能从支撑区间推断QTL的可能位置;
可利用标记连锁图在全染色体组系统地搜索QTL,如果一条染色体上 只有一个QTL,则QTL的位置和效应估计趋于渐进无偏;
3、QTL定位方法
根据采用的统计分析方法的不同分为:方差与 均值分析法、回归及相关分析法、最大似然法 等; 根据标记区间数分为:零区间作图、单区间作 图和多区间作图。
将不同方法结合起来的综合分析方法:QTL复合区间作图、多 区间作图、多QTL作图、多性状作图等。
(1) 均值比较(mean comparison,MC)
容易出现假阳性; 检测效率不高,所需的个体数较多;
目前MC法仅用于对数据的初步分析。
(2) 联合定位法(joint mapping,JM)
原理
针对MC无法对多个QTL定位的缺点,一种改进方法是将同 一条染色体上各标记的t测验或方差分析联合于一个回归分 析之中,即JM法。
优点
JM法可同时估计多个QTL的位置和效应,适用范围广(与 性状分布无关),计算简单。
优点
将多维检测问题简化为一维检测问题,单个QTL的效应和位置的估计是渐进无偏的;
以连锁标记为检验条件,极大地提高了QTL作图的精度;
利用了所有信息,比其它QTL作图方法更为有效; 可利用QTL似然图谱来表示整个基因组上每一点上QTL的证据强度,从而保留 了区间作图的特征。 因此,它是目前普遍认为同时标定多个QTL更有效、更精确的方法。
异大和亲缘关系较远的材料
利用重测序染色体片段代换系群体定位水稻籽粒长宽比QTL
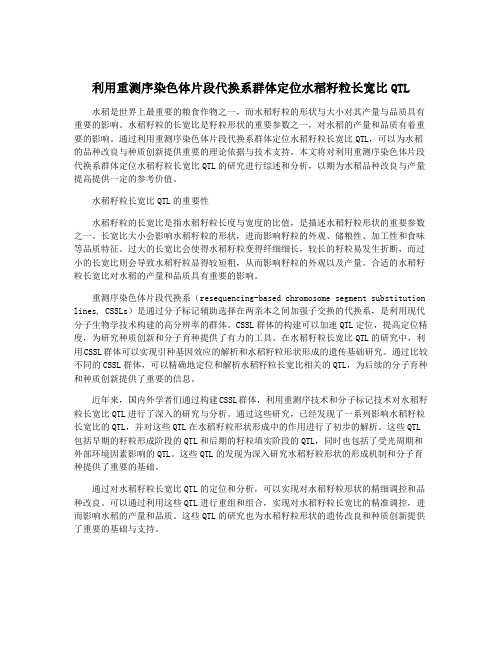
利用重测序染色体片段代换系群体定位水稻籽粒长宽比QTL水稻是世界上最重要的粮食作物之一,而水稻籽粒的形状与大小对其产量与品质具有重要的影响。
水稻籽粒的长宽比是籽粒形状的重要参数之一,对水稻的产量和品质有着重要的影响。
通过利用重测序染色体片段代换系群体定位水稻籽粒长宽比QTL,可以为水稻的品种改良与种质创新提供重要的理论依据与技术支持。
本文将对利用重测序染色体片段代换系群体定位水稻籽粒长宽比QTL的研究进行综述和分析,以期为水稻品种改良与产量提高提供一定的参考价值。
水稻籽粒长宽比QTL的重要性水稻籽粒的长宽比是指水稻籽粒长度与宽度的比值,是描述水稻籽粒形状的重要参数之一。
长宽比大小会影响水稻籽粒的形状,进而影响籽粒的外观、储粮性、加工性和食味等品质特征。
过大的长宽比会使得水稻籽粒变得纤细细长,较长的籽粒易发生折断,而过小的长宽比则会导致水稻籽粒显得较短粗,从而影响籽粒的外观以及产量。
合适的水稻籽粒长宽比对水稻的产量和品质具有重要的影响。
重测序染色体片段代换系(resequencing-based chromosome segment substitution lines, CSSLs)是通过分子标记辅助选择在两亲本之间加强子交换的代换系,是利用现代分子生物学技术构建的高分辨率的群体。
CSSL群体的构建可以加速QTL定位,提高定位精度,为研究种质创新和分子育种提供了有力的工具。
在水稻籽粒长宽比QTL的研究中,利用CSSL群体可以实现引种基因效应的解析和水稻籽粒形状形成的遗传基础研究。
通过比较不同的CSSL群体,可以精确地定位和解析水稻籽粒长宽比相关的QTL,为后续的分子育种和种质创新提供了重要的信息。
近年来,国内外学者们通过构建CSSL群体,利用重测序技术和分子标记技术对水稻籽粒长宽比QTL进行了深入的研究与分析。
通过这些研究,已经发现了一系列影响水稻籽粒长宽比的QTL,并对这些QTL在水稻籽粒形状形成中的作用进行了初步的解析。
QTL定位的原理和步骤(共15张PPT)

三、QTL作图一般步骤
(三)检测分离世代群体中每一个体的标记基因型
21 113 22
第十二页,共15页。
三、QTL作图一般步骤
(四)测量数量性状 测定作图群体的每个个体(系)数量性状值。如:
株高
百粒重 蛋白质含量 ……
第十三页,共15页。
三、QTL作图一般步骤
(五)统计分析
haploids,
DH)群体
QTL(quantitative trait loci):数量性状基因座,它指的是控制数量性状的基因在基因组中的位置。
种三子、生 QT产L作与图经一营般专步业骤教重学资组源库近交系(recombinant inbred lines, RIL)群体
21 113 22
其中DH群体和RIL群体可以永久使用。
第八页,共15页。
三、QTL作图一般步骤
(二)确定和筛选遗传标记 理想的作图标记应具备以下4个特征: 数量丰富
多态性好
中性 共显性
第九页,共15页。
常用的分子标记
中文名称 限制性片段长度多态性
英文缩写 RFLP
扩增片段长度多态性
AFLP
随机扩增多态性DNA
RAPD
Байду номын сангаас
微卫星(简单序列重复) SSR
第十页,共15页。
(一)构建作图群体 适于QTL作图的群体是待测数量性状存在广泛变异,
多个标记位点处于分离状态的群体。一般是由亲缘关系较远 的亲本间杂交,再经自交、回交等方法构建。
第七页,共15页。
三、QTL作图一般步骤
(一)构建作图群体 QTL定位:也称QTL作图,借助分子标记,可以在染色体上检测出QTL,并可以确定影响某一数量性状的QTL在染色体上的数目、位置和
数量性状基因定位的原理及方法
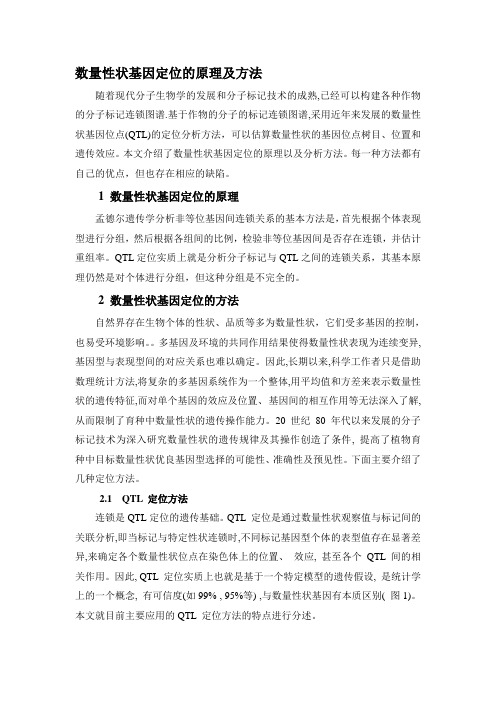
数量性状基因定位的原理及方法随着现代分子生物学的发展和分子标记技术的成熟,已经可以构建各种作物的分子标记连锁图谱.基于作物的分子的标记连锁图谱,采用近年来发展的数量性状基因位点(QTL)的定位分析方法,可以估算数量性状的基因位点树目、位置和遗传效应。
本文介绍了数量性状基因定位的原理以及分析方法。
每一种方法都有自己的优点,但也存在相应的缺陷。
1 数量性状基因定位的原理孟德尔遗传学分析非等位基因间连锁关系的基本方法是,首先根据个体表现型进行分组,然后根据各组间的比例,检验非等位基因间是否存在连锁,并估计重组率。
QTL定位实质上就是分析分子标记与QTL之间的连锁关系,其基本原理仍然是对个体进行分组,但这种分组是不完全的。
2 数量性状基因定位的方法自然界存在生物个体的性状、品质等多为数量性状,它们受多基因的控制,也易受环境影响。
多基因及环境的共同作用结果使得数量性状表现为连续变异,基因型与表现型间的对应关系也难以确定。
因此,长期以来,科学工作者只是借助数理统计方法,将复杂的多基因系统作为一个整体,用平均值和方差来表示数量性状的遗传特征,而对单个基因的效应及位置、基因间的相互作用等无法深入了解, 从而限制了育种中数量性状的遗传操作能力。
20 世纪80 年代以来发展的分子标记技术为深入研究数量性状的遗传规律及其操作创造了条件, 提高了植物育种中目标数量性状优良基因型选择的可能性、准确性及预见性。
下面主要介绍了几种定位方法。
2.1 QTL 定位方法连锁是QTL定位的遗传基础。
QTL 定位是通过数量性状观察值与标记间的关联分析,即当标记与特定性状连锁时,不同标记基因型个体的表型值存在显著差异,来确定各个数量性状位点在染色体上的位置、效应, 甚至各个QTL 间的相关作用。
因此, QTL 定位实质上也就是基于一个特定模型的遗传假设, 是统计学上的一个概念, 有可信度(如99% , 95%等) ,与数量性状基因有本质区别( 图1)。
- 1、下载文档前请自行甄别文档内容的完整性,平台不提供额外的编辑、内容补充、找答案等附加服务。
- 2、"仅部分预览"的文档,不可在线预览部分如存在完整性等问题,可反馈申请退款(可完整预览的文档不适用该条件!)。
- 3、如文档侵犯您的权益,请联系客服反馈,我们会尽快为您处理(人工客服工作时间:9:00-18:30)。
TECHNICAL ADVANCE/RESOURCEQTL-seq:rapid mapping of quantitative trait loci in rice by whole genome resequencing of DNA from two bulked populationsHiroki Takagi 1,2,Akira Abe 2,3,Kentaro Yoshida 1,Shunichi Kosugi 1,Satoshi Natsume 1,Chikako Mitsuoka 1,Aiko Uemura 1,Hiroe Utsushi 1,Muluneh Tamiru 1,Shohei Takuno 4,Hideki Innan 5,Liliana M.Cano 6,Sophien Kamoun 6and Ryohei Terauchi 1,*1Iwate Biotechnology Research Center,Kitakami,Iwate,024-0003,Japan,2United Graduate School of Iwate University,Morioka,Iwate,020-8550,Japan,3Iwate Agricultural Research Center,Kitakami,Iwate,024-0003,Japan,4Department of Plant Sciences,University of California,Davis,CA 95616,USA,5Graduate University for Advanced Studies,Hayama,Japan,and 6The Sainsbury Laboratory,Norwich Research Park,Norwich,UKReceived 7September 2012;revised 13December 2012;accepted 20December 2012;published online 05January 2013.*For correspondence (e-mail terauchi@ibrc.or.jp).SUMMARYThe majority of agronomically important crop traits are quantitative,meaning that theyare controlled by multiple genes each with a small effect (quantitative trait loci,QTLs).Mapping and isolation of QTLs is important forefficient crop breeding by marker-assisted selection (MAS)and fora better understanding of the molecular mechanisms underlying the traits.However,since it requires the development and selection of DNA markers for linkage analysis,QTL analysis has been time-consuming and labor-intensive.Here we report the rapid identification of plant QTLs by whole-genome resequencing of DNAs from two populations each composed of 20–50individuals showing extreme opposite trait values for a given phenotype in a segregating progeny.We propose to name this approach QTL-seq as applied to plant species.We applied QTL-seq to rice recombinant inbred lines and F 2populations and successfully identified QTLs for important agronomic traits,such as partial resistance to the fungal rice blast disease and seedling vigor.Simulation study showed that QTL-seq is able to detect QTLs over wide ranges of experimental variables,and the method can be generally applied in population genomics studies to rapidly identify genomic regions that underwent artificial or natural selective sweeps.Keywords:quantitative trait loci,breeding,whole genome sequencing,next generation sequencer,selective sweep,technical advance.INTRODUCTIONThe world’s population has already exceeded 7billion and is still growing,while the amount of land suitable for agri-culture is decreasing due to a variety of factors such as rapid climate change.Therefore there is a great demand for efficient crop improvement to increase yield without further expanding farmland and damaging the environ-ment (Godfray,2010;David et al.,2011).In crop plants,multiple genes each with a relatively minor effect control the majority of agronomically impor-tant traits.These genes are called quantitative trait loci (QTLs)(Falconer and Mackay,1996).Identification of QTLsis an important task in plant breeding.Once a QTL control-ling a favorable trait is mapped with closely linked DNA markers,it is introduced into an elite cultivar by crossing of the recurrent elite parent to the donor plant.Following QTL a process marker-assisted selection and Matsuoka,2006).Marker-assisted selection reduces the effort and time needed for phenotype evaluation of the progeny during successive improves introgression ©2013The AuthorsThe Plant Journal ©2013Blackwell Publishing Ltd174The Plant Journal (2013)74,174–183doi:10.1111/tpj.12105Traditionally QTLs have been identified by linkage analy-sis of progeny derivedfrom a cross between parents show-ing contrasting phenotypes for a trait of interest.Toperform linkage analysis,DNA markers capable of discrimi-nating parental genomes are required.Due to this require-ment,parentsfor crosses are selected from genetically distantly related cultivars.This entails that parents may be different in many QTLs controlling a given phenotype,complicating the isolation of individual loci.On the other hand,whenever closely related parents are used,identifi-analysis Bulked-segregant analysis (BSA)method to identify DNA markers tightly linked to the causal gene for a given phenotype (Giovannoni et al.,1991;Michelmore et al.,1991).Following a cross between parental lines showing contrasting phenotypes,the resulting F 2progeny are scored for segregation of the phenotype.Two bulked DNA samples are generated from the progeny showing contrasting phenotypes,and DNA markers exhibiting dif-ferences between the two bulks are screened.In the origi-nal reports,DNAs bulked from F 2progeny were screened with restriction fragment polymorphisms (RFLPs)and ran-dom amplified polymorphic DNA (RAPD)markers to iden-tify the markers linked to the traits of ter,BSA was applied to identify QTLs (Mansur et al.,1993;Darvasi and Soller,1994),which is sometimes called ‘selective DNA pooling’.However,in these analyses,the availability of DNA markers was the main factor limiting effectiveness of the methods.Furthermore,genotyping of each marker for the time-consuming and costly.sequencing has accelerated the analysis of QTLs in yeast,a model organ-ism with a relatively small genome size (12.5Mb).Ehrenr-eich et al.(2010)made a cross between two diploid yeast strains and obtained a large number of haploid progeny.They then applied BSA to select two populations with extreme phenotypes,and genotyped the bulked DNA with a single nucleotide polymorphism (SNP)microarray and whole genome sequencing,which successfully identified the location of QTLs involved in resistance to various chemical compounds.The proposed method is called X-QTL since an extremely large number of progeny were used in each bulk.Similar applications of whole-genome sequencing to BSA are reported in yeast with successful identification of QTLs for xylose utilization (Wenger et al.,2010),heat tolerance (Parts et al.,2011),and ethanol toler-ance (Swinnen et al.,2012).However,the application of whole genome sequencing to BSA for identifying QTLs in whole-genome resequencing of two DNA bulks of progeny(each with 20–50individuals)showing extreme phenotypic values by next-generation sequencing (NGS)technology.Since this approach has a wide applicability in QTL identification in plant species,including crops,we propose to name the method QTL-seq as specifically applied to plant species.Because it does not require DNA marker development and genotyping,the most time-consuming and costly procedure needed for the conventional QTL analysis,QTL-seq allows the rapid identification of QTLs.RESULTSPrinciple of QTL-seqThe principle of QTL-seq is shown in Figure 1and is explained by taking rice as an example.QTL-seq combines bulked-segregant analysis (Giovannoni et al.,1991;Michel-more et al.,1991;Mansur et al.,1993;Darvasi and Soller,1994)and whole-genome resequencing for rapid identifica-tion of the genomic regions that differ between the two parents used in a genetic cross and also contribute to the higher and lower values of the traits of interest among the resulting progeny.For QTL mapping using QTL-seq,we first generate a mapping population by crossing two culti-can be used for QTL-seq depending on the traits to be studied.Recombinant inbred lines (RILs)and doubled hap-loids (DH)show a high degree of homozygosity,and indi-viduals in each line can be regarded as proxy clones that allow replicated measurements of the phenotype,and thus are suitable for detecting QTLs of minor effects.The advantage of using an F 2population is the a short time required for its generation.However,no replicated mea-surements are possible for each genotype.As a result,the approach is not suitable for detecting minor effect QTLs.After the progeny of a mapping population are measured for the focused trait,we score segregation of the pheno-type.If the number of QTLs involved in the trait variation is multiple,frequency distribution of measured values will be close to the normal (Gaussian)distribution (Figure 1a).Here,we focused on the multiple progeny showing extreme phenotypes,i.e.those exhibiting the highest and the lowest extreme values.We sampled DNA from 10to 20individuals from each extremity and bulked them to gener-ate ‘Highest’bulk and ‘Lowest’bulk.Each of the bulked DNAs was applied to whole genome resequencing with a >6x genome coverage.We expect the bulked DNA to contain genomes from both parents in a 1:1ratio for the majority of genomic regions.However,we should detect unequal representation of the genomes from the two par-ents in the genomic regions harboring QTL for the pheno-typic difference between ‘Highest’and ‘Lowest’bulks.©2013The AuthorsThe Plant Journal ©2013Blackwell Publishing Ltd,The Plant Journal ,(2013),74,174–183Whole genome sequencing reveals QTL 175To examine the relative amount of the genomes derived from the two parents,we evaluated the proportion of short reads corresponding to eachof the two parental genomes that can be discriminated by single nucleotide polymor-phisms (SNPs)available between the two.After aligning the sequence data to the reference sequence of either of the two parents,we counted the number (k )of short reads harboring SNPs that are different from the reference sequence.We defined the proportion of k in the total short reads (n )covering a particular genomic position (=k n À1)means an equal contribution of both parents’genomes to the bulked progeny.Accordingly,the SNP-index is calcu-lated for all the SNPs detected between the two parents,and the relationships between SNP-index and SNP posi-tion in the genome is graphically represented (Figure 1c).We carried out this procedure separately for the ‘Highest’and ‘Lowest’bulk sequences.In practice,SNPs with SNP-index <0.3in both bulked sequences are filtered out during SNP calling because they cannot be discriminated from spurious SNPs caused by sequencing or alignment errors.However,if SNPs with a SNP-index of 0.3or greater are present in only one of the two bulks,we consider them as real SNPs and assume their presence in the other bulk as well.In this case,we make use of the SNP-index value of the other bulked DNA even if it is <0.3(see Experimental Procedures).By taking an average of SNP-indices of SNPs located in a given(a)(b)(c)©2013The AuthorsThe Plant Journal ©2013Blackwell Publishing Ltd,The Plant Journal ,(2013),74,174–183176Hiroki Takagi et al.genomic interval,sliding window analysis can be applied to facilitate visualization of the graphs.We expect the SNP-index graphs of ‘Highest’and ‘Lowest’bulks to be identical for the genomic regions that are not relevant to the pheno-typic difference between the two.However,the genomic regions harboring QTLs that contribute to the difference in the phenotype between the two bulks should exhibit unequal contributions from the two parental genomes.Furthermore,SNP-indices of these regions for‘Highest’and ‘Lowest’bulks would appear asmirror images with respect to the line of SNP-index =0.5.Such regions are expected to have a high probability of containing QTLs responsible for the trait difference between the ‘Highest’and ‘Lowest’parison of the two graphs is important to discern the QTLs from the genomic regions showing segregation distortion caused by reasons other than the imposed artificial selection (e.g.meiotic drive),and result in departure of the SNP-index from 0.5in both bulks in the same direction.It is therefore convenient to combine the two graphs for ‘Highest’and ‘Lowest’bulks by subtracting the SNP-index value of the latter from the parent A genome only and D (SNP-index)=0if both par-ents have the same SNP-indices at the genomic regions.QTL-seq applied to RILs:detection of QTLs controlling partial resistance to rice blast in NortaiWe applied QTL-seq for the detection of QTLs involved in partial resistance of the rice cultivar Nortai against the fun-gal pathogen Magnaporthe oryzae ,the causal agent of rice blast disease.Resistance of Nortai to M.oryzae race 037.1does not seem to be mediated by typical R-genes;the hypersensitive response cannot be clearly distinguished and the trait is quantitative and difficult to measure.We crossed Nortai to the cultivar Hitomebore that is highly susceptible to the race 037.1and obtained F 2(Figure 2a).Each F 2progeny was established as a line and brought to the F generation by a single-seed descent method to gen-Using the 241RILs,we carried out M.oryzae inoculation assay to assess the resistance of the progeny.Susceptibil-ity of the progeny was measured and categorized to seven classes from class 4(resistant)to class 10(highly suscepti-ble;Figure 2b).The inoculation assay was conducted four times over 4years to ensure the correct scoring of each RIL (Figure S1in Supporting Information).(a)(d)(b)(c)©2013The AuthorsThe Plant Journal ©2013Blackwell Publishing Ltd,The Plant Journal ,(2013),74,174–183Whole genome sequencing reveals QTL 177ing intodifferent classes isclose to normal distribution,suggesting that multiple genes control the partial resis-tance of RILs(Figure2c).We defined20RILs consistentlyshowing high resistance(class4progeny,and an additional setshowing high susceptibility Sus-ceptible(S-)progeny.Genomic DNA of R-progeny wasbulked in an equal ratio to generate R-bulk DNA,and thatof S-progeny was bulked to generate S-bulk DNA.reference sequence of the Hitomebore cultivar using BWAsoftware(Li and Durbin,2009).The average read depthwas>6.88x in both bulked DNA(Table S1).A total of161563SNPs were identified between Nortai and Hitome-bore genomes(Table S2),and the SNP-index was calcu-lated for each SNP(Figure1b;Abe et al.,2012a).Graphsshowing relationships between SNP-index and genomicpositions are given in Figures2(d)and S2.We foundhighly contrasting patterns of SNP-index graphs for R-bulkand S-bulk in the region between2.39and4.39Mb onchromosome6as shown in Figure2(d).The resistant RILsmainly had Nortai-type genomic segments in the2.39to4.39Mb region of chromosome6,whereas susceptiblesome genomic regions exhibit positive or negative valuesof D(SNP-index).These may correspond to QTLs governingthe difference between the R-and S-progeny.We calcu-lated statistical confidence intervals of D(SNP-index)for allthe SNP positions with given read depths under the nullhypothesis of no QTLs,and plotted them along with D(SNP-index)(Experimental Procedures;Figures2d and S2).The chance that D(SNP-index)becomes higher than0.79asobserved for the chromosomal region of2.39–4.39Mb isP<0.01under the null hypothesis.odds)scores of linkage between SNP markers and the traitwere obtained(Figure2d).The highest LOD score(LOD=7.38)was observed in the interval of SNP makerslocated at0and4.9Mb.This interval corresponded to thegenomic region identified by the QTL-seq method(Fig-the location of the partial resistance in Nortai.Using RILs derived from two other cross-combinationsof rice cultivars,we applied QTL-seq and identified thepeaks of the D(SNP-index)plot presumably correspondingto a major QTL:a D(SNP-index)peak for lower grain amy-lose content in the cultivar Iwate96as compared withHitomebore(Figure S3),although this peak was not statis-tically significant(0.05<P<0.1).We identified fourD(SNP-index)peaks for enhanced seedling vigor underlow-temperature conditions of a cultivar Arroz da Terra aspeaks were statistically significant(the peak for qLTG3-1,P<0.01;and the peaks for qLTG3-2and qLTG11,P<0.05).Application of QTL-seq to F2progenyWe further examined the possibility of applying QTL-seq toan F2population,which is much easier to generate thanRILs of advanced generations.A japonica type cultivar Dun-ghan Shali is known to have a strong seedling vigor com-pared with Hitomebore(Figure3a).We have recentlyfine-mapped a major QTL,qPHS3-2,on chromosome3thatconfers the seedling vigor in Dunghan Shali using conven-tional QTL analysis of RILs of the F7generation derivedfrom a cross between Dunghan Shali and a japonica culti-var Kakehashi(Abe et al.,2012b).The QTL most likely cor-responds to a gene OsGA20ox1,a gene involved ingibberelllin(GA)byosynthesis(Abe et al.,2012b;Yanoet al.,2012).Using Dunghan Shali,we addressed whetherQTL-seq can detect qPHS3-2in the F2progeny derived froma cross between Dunghan Shali and Hitomebore.Aftercrossing Dunghan Shali to Hitomebore,we obtained F2progeny.scoredin water at25°C.The variation in seedling height in seed-ling followed a normal distribution,indicative of theinvolvement of multiple genes in determining this charac-ter(Figure3b).Two DNA bulks were prepared;the50tall-est individuals as‘H-bulk’and the50shortest individualsas‘L-bulk’,and were used for QTL-seq analysis(Figures3cand S2).By examining the D(SNP-index)plot,we identifiedtwo genomic positions exhibiting the highest D(SNP-index)values:the region on chromosome3from36.21to©2013The AuthorsThe Plant Journal©2013Blackwell Publishing Ltd,The Plant Journal,(2013),74,174–183 178Hiroki Takagi et al.37.31Mb with D (SNP-index)=0.61(statistical significance under the null hypothesis:P <0.01)and the region on chro-mosome 1from 39.08to 41.08Mb with D (SNP-index)=0.67(P <0.05).This former position corresponded exactly to the reportedqPHS3-2,most probably the locus of OsGA20ox1.Likewise,the latter position was also previ-Simulation of QTL-seqAs shown above,QTL-seq successfully identified genomic regions controlling quantitative traits in the examples of rice RILs and F 2families.size recombination rate 4cM Mb À1.We also postulated that 150000SNPs between the two parents are distributed with equal inter-vals (i.e.a SNP every 2.4kb).For the QTL-seq process,it is assumed that from among all progeny individuals of F 2or F 7generations,we select p %each of progeny with oppo-site extreme trait values to make ‘Highest’and ‘Lowest’bulks,and we sample n random alleles from each bulk to of m become narrower as the coverage and the percentage of individuals in each bulk (p )increases (for our application to rice,the 99%cutoff of |m |would be 0.29given n =10and p =0.15).We also applied the same simulation to RILs of the F 7generation (Figure 4a,right).The null distribution of |m |is wider than that for an F 2population.We next explored the power to detect a QTL.In practice,we place a QTL in the simulated genome assuming that the relative contribution of the QTL to the total phenotype vari-ation is given by Qp .Then,we evaluated the power as the proportion of the simulation replications with |m |around the QTL larger than the 99%cutoff value obtained earlier.In this power simulation,bi-allelic states were allowed at the focal QTL,and for the dominance effect we considered two cases,codominance and complete dominance.For Qp ,(a)(c)(b)©2013The AuthorsThe Plant Journal ©2013Blackwell Publishing Ltd,The Plant Journal ,(2013),74,174–183Whole genome sequencing reveals QTL 179two values(0.05and0.1)were used.Note that Qp is the rel-ative contribution of this QTL to the total phenotype varia-tion,which includes everything other than the geneticeffect of the focal QTL(that is,the environmental factorsand the genetic contributions from other QTLs that are notspecified here).Figure4(b)and(c)shows the results forthe cases of codominance and complete dominance,respectively.We found that the larger read depth increasesthe power in all cases.The power is higher when Qp=0.1than the cases of Qp=0.05.It appears that higher power isexpected when the QTL allele is codominant(additive)ascompared with complete dominance,and there is an opti-mum value for the percentage of individuals in each bulk(p).When the value is small,the power is low,probablybecause there would be too much sampling variance.As pincreases,the power increases,but it starts to decreasewhen the sample size is so large that many individuals withintermediate phenotype are included in the bulking.Wefound higher power in F7RILs than in F2populations,andwith a relative contribution of roughly10%(Qp=0.1).DISCUSSIONTwo types of genetic variations,the ones derived from arti-ficial mutagenesis and those naturally occurring in landrac-es and wild crop relatives,have been used in plantbreeding.Mutant lines generated by artificial mutagenesisare valuable for isolating agronomically important genes.To this end,we have recentlycient method to identify the causal mutation of a givenphenotype by whole genome resequencing of the bulkedDNA of progeny showing mutant phenotype(Abe et al.,2012a).Although MutMap is a powerful technique,cropbreeding has mostly depended on genetic variations avail-able among different cultivars and species in what is calledThis is in part because naturally occurringvariants harbor a potentially larger repertoire of usefulalleles than the artificially generated mutants due to thelarger number of mutations accumulated over long time innature.Therefore,analysis of the QTL variations amongnatural variants is important for enhancing breeding byisolating useful alleles of the genes controlling agronomi-cally important traits(Yano,2001).However,conventionalQTL analysis is a laborious process requiring the develop-ment of DNA markers and the generation of a largenumber of advanced generation progeny.Here we demon-strated the successful application of whole-genome rese-quencing for detecting rice QTLs for agronomicallyimportant traits,including partial resistance and seedlingvigor,using RILs and F2populations,respectively.Themajor advantage of QTL-seq is that it does not necessitateDNA marker development and marker genotyping for map-ping purposes.The SNPs available between the parentallines serve as such markers,thus reducing the cost and(a)(b)(c)©2013The AuthorsThe Plant Journal©2013Blackwell Publishing Ltd,The Plant Journal,(2013),74,174–183 180Hiroki Takagi et al.time required for marker development and genotyping.Furthermore,the use of SNP-index allows accurate evalua-tion of the frequencies of parental alleles in a subset of progeny of a given genomic position.These two key attri-butes make QTL-seq an attractive method for quick and cost-effective identification of QTLs.Bulked-segregant analysis was first applied to facilitate the linkage analysis of discrete characters in F 2populations (Giovannoni et al.,1991;Michelmore et al.,1991).In these studies,F 2progeny showing two discrete characters were isolated,and DNA from the F 2individuals were pooled to make two DNA bulks corresponding to the two character types.After a battery of DNA markers including RAPD markers (Williams et al.,1990)were tested for these two bulked DNAs,markers showing differences between the two DNA bulks were selected to represent the DNA mark-ers linked to the gene(s)responsible for the difference in the characters.This original bulked-segregant method was later extended to QTL analysis.After RILs or F 2were scored for the phenotypes,progeny showing extreme opposite phenotypes were selected,and these DNAs were separately bulked to find DNA markers showing linkage with the phenotypic differences (‘selective DNA pooling’;Mansur et al.,1993;Darvasi and Soller,1994).This latter method is in principle similar to QTL-seq,but requires DNA marker development and testing of bulked DNA with each marker,both time-consuming and labor-intensive processes that are circumvented by QTL-seq.Conse-quently,QTL-seq is much more rapidly performed.QTL-seq also allows an accurate quantitative evaluation of the genomic contribution from the two parents to the bulked DNAs by using SNP-index,whereas the conventional method has to rely on analog assessment of marker states,e.g.the relative strength of intensity of DNA amplicons after PCR amplification of the markers.Therefore,we believe that QTL-seq is quicker and has a much higher power than the previous methods used for QTL identifica-tion.Applications of whole genome sequencing to two DNA bulks of progeny with extreme phenotypes have been reported in yeast (X-QTL;Ehrenreich et al.,2010;Wenger et al.,2010;Parts et al.,2011;Swinnen et al.,2012),and its statistical property applied to yeast was also addressed (Magwene et al.,2011).QTL-seq has the same principle as these methods.However,QTL-seq is the first application of a similar method in plant species with a much larger gen-ome size (rice,380Mb),and we demonstrated that it can be carried out with a significantly smaller number of prog-eny (20–50)in each bulk than the methods previously reported in yeast.In crop species,bulking of an extremely large number of progeny is not practical.QTL-seq applied to seedling vigor in rice demonstrated that this method successfully identifies QTL in an F 2genera-tion,which is a much earlier generation than the F 7one that we used for conventional QTL analysis based on RILs.Oursimulation analysis showed that if the phenotypic effect of the focal QTL accounts for more than 10%of the entire varia-tion and if the read depth is more than or equal to 20its genomic position may be readily detected by QTL-seq even in the F 2generation.We also demonstrated that QTL-seq is applicable to progeny obtained from crosses made between genetically closely related cultivars.The rice cultivars Nortai and Hitomebore and Dunghan Shali used in the current experiments all belong to japonica species,and DNA poly-morphisms among them are low,making DNA marker devel-opment difficult in the conventional scheme of QTL analysis.We envisage that QTL-seq can be applied to any popula-tion for detecting genomic regions that underwent artificial or natural selection.For instance,a population of a species is distributed over a certain environmental gradient (high temperature versus low temperature).We could then make two DNA bulks:one from multiple individuals from high temperature zones and the other from low temperature zones.Sequence reads from these two DNA bulks are com-pared to a reference sequence,and D (SNP-index)is calcu-lated for all the genomic regions.The regions showing higher D (SNP-index)than the background genome should point to the regions responsible for the adaptation of pop-ulation to high/low temperature.In this regard,QTL-seq could be perceived as a general method for detecting genomic regions showing signatures of recent selective sweep by whole-genome resequencing of DNAs from two groups of individuals that underwent recent artificial or natural selection in the opposite directions.In view of the recent rapid development in sequencing technology,we foresee that methods that make use of whole-genome sequencing-based techniques,including et al.,2012a),NGM (Austin et others (Mokry et al.,2011;Trick et al.,2012),will dramatically accelerate crop improvement in a cost-effective manner.These and other related technologies that take full advanta-ges of the rapidly declining cost of genome sequencing are expected to significantly contribute to the on-going efforts aimed at addressing the world food security prob-lem by reducing breeding time.EXPERIMENTAL PROCEDURES Evaluation of partial resistance of RILsTo evaluate the partial resistance of RILs to leaf blast disease we conducted upland nursery trials in 2006and 2011at Iwate Agri-cultural Research Center in Kitakami,Iwate,Japan.Overall,a total of four independent inoculation assays were carried out.For each RIL,about 200seeds were sown in single 40-cm long rows that were spaced at 10cm apart.For use as inoculum,seedlings of a highly susceptible cultivar Moukoto were grown on both sides of each block of RIL rows.Nitrogen was applied at the rate of 20kg per 1000m 2as a basal fertilizer.Disease severity was visually scored according to the procedure of Asaga (1981).©2013The AuthorsThe Plant Journal ©2013Blackwell Publishing Ltd,The Plant Journal ,(2013),74,174–183Whole genome sequencing reveals QTL 181。