基于NIRG的冬小麦叶绿素含量的监测
基于高光谱和PLS-LS-SVM的冬小麦叶绿素含量检测

确、 快速 、 经济的作 物氮素营养水平诊 断方法是农业
生产 的迫 切需 要 。 比较 而 言 , 测量 光 谱 反 射 率 方 法具
有非破坏 、 快速特点 , 以作为植 被生化成分和 生物 可 物理 参数 十分 有效 的检测 方法 J 。图像光 谱技 术 集
成 了传统 的 图像 和光谱 技 术 , 同 时获 得 被 测 物 体 的 可
要 : 定 量测 定 小麦 叶 片 叶绿 素 含量 在 小麦 估 产 、 农情 监 测 等方 面 具有 重 要 意 义 。本 研 究 验 证 高 光 谱 成像 技
术结 合偏 最 小 二乘 一 最小 二 乘 支持 向量机 ( L P S—L S—S M) 模 方 法 预测 大 田冬 小 麦 叶 绿 素含 量 的可 行 性 。 首 V 建 先利 用 所搭 建 高光 谱 成像 系统 以线 扫 描方 式 获 取 大 田冬 小 麦 叶 片 反 射 光 谱 , 而 得 到 其 立 方 体 图像 数 据 , 在 进 并 小 麦 叶 片光 谱 图像 上 选择 感 兴 趣 区域 计算 出 光谱 平 均反 射 率 值 。为 保证 P S一 一S M 模 型 的鲁 棒性 和预测 稳 L V 定 性 , 先通 过 P S方 法解 决 多 重共 线 性 问 题 并 将 输 入 变 量 维 数 减 至 4维 , 后 利 用 L 首 L 然 S—S M 进行 训 练 建 模 。 V
谱分辨率已成为农产 品品质评估 和安全 检测的强有 力工 具 J 国外 众 多 的研 究 都 表 明通 过 定 量 的 将 叶 。 绿素、 水分等与高光谱反射 图像 数据建立关 系, 采用
高 光谱 测量 方 法 可 以 在植 被 叶 片 或 冠 层 水 平 定 量 地 确定 其 生化 成 分 的含量 J 。
低温胁迫下冬小麦叶绿素含量高光谱估测
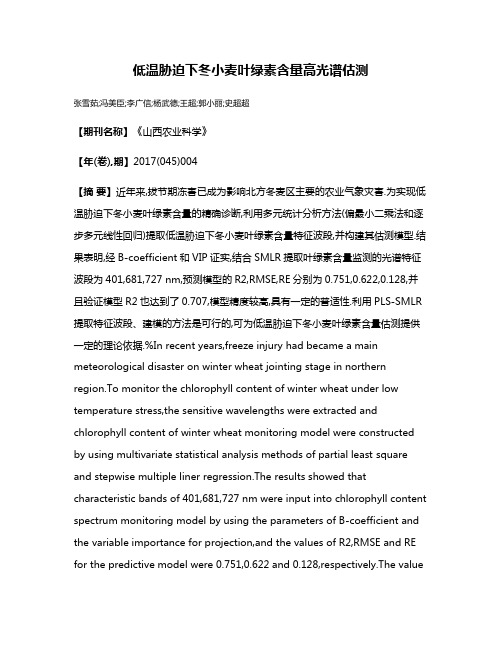
低温胁迫下冬小麦叶绿素含量高光谱估测张雪茹;冯美臣;李广信;杨武德;王超;郭小丽;史超超【期刊名称】《山西农业科学》【年(卷),期】2017(045)004【摘要】近年来,拔节期冻害已成为影响北方冬麦区主要的农业气象灾害.为实现低温胁迫下冬小麦叶绿素含量的精确诊断,利用多元统计分析方法(偏最小二乘法和逐步多元线性回归)提取低温胁迫下冬小麦叶绿素含量特征波段,并构建其估测模型.结果表明,经B-coefficient和VIP证实,结合SMLR提取叶绿素含量监测的光谱特征波段为401,681,727 nm,预测模型的R2,RMSE,RE分别为0.751,0.622,0.128,并且验证模型R2也达到了0.707,模型精度较高,具有一定的普适性.利用PLS-SMLR 提取特征波段、建模的方法是可行的,可为低温胁迫下冬小麦叶绿素含量估测提供一定的理论依据.%In recent years,freeze injury had became a main meteorological disaster on winter wheat jointing stage in northern region.To monitor the chlorophyll content of winter wheat under low temperature stress,the sensitive wavelengths were extracted and chlorophyll content of winter wheat monitoring model were constructed by using multivariate statistical analysis methods of partial least square and stepwise multiple liner regression.The results showed that characteristic bands of 401,681,727 nm were input into chlorophyll content spectrum monitoring model by using the parameters of B-coefficient and the variable importance for projection,and the values of R2,RMSE and RE for the predictive model were 0.751,0.622 and 0.128,respectively.The valueof R2 for the validated model was 0.707,which indicated that the multivariate methods had potential application on extracting the important wavelengths of chlorophyll content and constructing the predictive model.The study would provide some theoretical basis to the evaluation of winter wheat chlorophyll content under low temperature stress.【总页数】4页(P526-529)【作者】张雪茹;冯美臣;李广信;杨武德;王超;郭小丽;史超超【作者单位】山西农业大学农学院,山西太谷030801;山西农业大学农学院,山西太谷030801;山西农业大学农学院,山西太谷030801;山西省农业科学院作物科学研究所,山西太原030031;山西农业大学农学院,山西太谷030801;山西农业大学农学院,山西太谷030801;山西农业大学农学院,山西太谷030801;山西农业大学农学院,山西太谷030801【正文语种】中文【中图分类】S512.1+1【相关文献】1.冬小麦冠层水平叶绿素含量的高光谱估测 [J], 王晓星;常庆瑞;刘梦云;刘秀英;尚艳2.基于光谱变换的低温胁迫下冬小麦叶绿素含量估测研究 [J], 张雪茹;冯美臣;杨武德;王超;郭小丽;史超超3.天然气微泄漏胁迫下大豆冠层叶绿素含量的高光谱估测 [J], 姚胜男;蒋金豹;史晓霞;王文佳;孟豪4.条锈病影响下冬小麦叶绿素含量的高光谱估计 [J], 江道辉;李章成;周清波;李森;刘佳5.低温胁迫下冬小麦叶片叶绿素含量的高光谱估算 [J], 李玮祎;孙明馨;曾风玲;王凤文因版权原因,仅展示原文概要,查看原文内容请购买。
利用无人机高光谱估算冬小麦叶绿素含量

利用无人机高光谱估算冬小麦叶绿素含量冯海宽;陶惠林;赵钰;杨福芹;樊意广;杨贵军【期刊名称】《光谱学与光谱分析》【年(卷),期】2022(42)11【摘要】叶绿素含量(SPAD)是作物长势评价的重要指标,可以监测农作物的生长状况,对农业管理至关重要,因此快速、准确地估算SPAD具有重要意义。
以冬小麦为研究对象,利用无人机高光谱获取了拔节期、挑旗期和开花期的影像数据,获取植被指数和红边参数,研究植被指数与红边参数估算SPAD的能力。
先将植被指数与红边参数分别与不同生育期的SPAD进行相关性分析,再基于植被指数、植被指数结合红边参数,通过偏最小二乘回归(PLSR)方法估算SPAD,最后制作SPAD分布图验证模型的有效性。
结果表明,(1)大部分植被指数与红边参数在3个主要生育期与SPAD相关性均达到极显著水平(0.01显著);(2)单个植被指数构建的SPAD估算模型中,LCI表现最好(R^(2)=0.56,RMSE=2.96,NRMSE=8.14%),红边参数中Dr/Dr_(min)表现最好(R^(2)=0.49,RMSE=3.18,NRMSE=8.76%);(3)基于植被指数结合红边参数构建的SPAD估算模型效果最佳,优于仅基于植被指数构建的SPAD估算模型,同时,随着生育期推移,两种模型均在开花期达到最高精度,R^(2)分别为0.73和0.78,RMSE分别为2.49和2.22,NRMSE分别为5.57%和4.95%。
因此,基于植被指数结合红边参数,并使用PLSR方法可以更好地估算SPAD,可以为基于无人机遥感的SPAD监测提供一种新的方法,也可为农业管理提供参考。
【总页数】6页(P3575-3580)【作者】冯海宽;陶惠林;赵钰;杨福芹;樊意广;杨贵军【作者单位】农业农村部农业遥感机理与定量遥感重点实验室;南京农业大学国家信息农业工程技术中心;河南工程学院土木工程学院【正文语种】中文【中图分类】S25【相关文献】1.基于无人机高光谱影像玉米叶绿素含量估算2.基于Dualex植物多酚-叶绿素仪的冬小麦叶绿素含量高光谱估算3.低温胁迫下冬小麦叶片叶绿素含量的高光谱估算4.冬小麦不同叶位叶片的叶绿素含量高光谱估算模型5.基于无人机高光谱的冬小麦植株氮含量估算因版权原因,仅展示原文概要,查看原文内容请购买。
冬小麦不同叶位叶片的叶绿素含量高光谱估算模型

冬小麦不同叶位叶片的叶绿素含量高光谱估算模型马春艳;王艺琳;翟丽婷;郭辅臣;李长春;牛海鹏【期刊名称】《农业机械学报》【年(卷),期】2022(53)6【摘要】科学、高效地获取作物不同叶位叶绿素含量的垂直分布信息,可监测农作物长势状况并进行田间管理。
基于冬小麦抽穗期获取的不同叶位叶片的高光谱反射率和叶绿素含量实测数据,将原始光谱、一阶微分光谱、二阶微分光谱、植被指数和连续小波系数与叶绿素含量进行相关性分析,筛选相关性较强的光谱特征参数,然后分别采用偏最小二乘回归、支持向量机、随机森林和反向传播神经网络4种机器学习算法构建冬小麦上1叶、上2叶、上3叶和上4叶的叶绿素含量估算模型,并根据精度评估结果筛选不同叶位叶绿素含量估算的最佳模型。
结果表明,上1叶、上2叶和上3叶采用小波系数结合偏最小二乘回归构建的叶绿素含量估算模型精度最高,建模和验证R^(2)分别为0.82和0.75、0.80和0.77、0.71和0.62;上4叶采用植被指数结合支持向量机构建的叶绿素含量估算模型效果最佳,建模和验证R^(2)为0.74和0.79。
研究结果可为基于遥感技术精准监测作物营养成分的垂直变化特征提供理论和技术支撑。
【总页数】10页(P217-225)【作者】马春艳;王艺琳;翟丽婷;郭辅臣;李长春;牛海鹏【作者单位】河南理工大学测绘与国土信息工程学院【正文语种】中文【中图分类】S512.11【相关文献】1.截形叶螨危害下枣叶片叶绿素含量高光谱估算模型2.不同地类春小麦叶片叶绿素含量高光谱植被指数估算模型研究3.低温胁迫下冬小麦叶片叶绿素含量的高光谱估算4.基于高光谱的石楠叶片叶绿素含量估算模型5.基于高光谱参数建立苗期高温条件下草莓叶片叶绿素含量估算模型因版权原因,仅展示原文概要,查看原文内容请购买。
基于NIRG的冬小麦叶绿素含量的监测

1 引言小麦是我国主要的粮食作物之一,其产量是各级政府进行决策、生产部门指导生产、流通领域安排粮食收购和销售、交通部门安排运输计划的重要经济信息。
因而小麦产量的准确预测对我国农业和经济的发展具有重要意义。
遥感技术和地理信息系统的引入为解决这一问题提供了有效手段[1]。
遥感估产小麦种植是通过卫星可见光和近红外波段进行组合,形成各种植被指数,根据植被的光谱特性,来研究植被演化信息[2]。
近20年来利用遥感进行作物实时监测和营养快速诊断一直是遥感在农业中应用的研究热点。
高光谱遥感技术能通过监测作物生育时期的光谱变化研究作物反射光谱和叶绿素含量、叶面积指数等农学参数之间的关系,为作物遥感长势监测和遥感估产提供依据[3]。
利用卫星遥感信息进行农作物的长势监测和产量估计,主要依据农田作物光谱的反射特征,即同一作物在同一时间的不同波段以及在不同时间内具有不同的反射辐射状况。
然而,农田作物光谱实际上是多种光谱混合的结果,但微分光谱、植被指数等技术在水稻农学参数测定研究应用中有着很好的表现[4],除作物本身状况外,农田作物光谱受土壤背景状况的影响由于栽培措施不同,作物本身又出现各种生长状况,因而可表现出各种各样的光谱状况。
卫星遥感信息反映的是野外条件下地物的反射辐射状况,因而可表现出各种各样的光谱状况。
卫星遥感信息反映的是野外条件下地物的反射辐射状况,田间野外测定的地面光谱与其具有很好的可比性。
从高光谱数据中提取植被指数进行长势监测和氮营养诊断也是其中重要的内容,研究表明红光和近红外波段的不同组合进行植被研究非常好,这些波段包含90%以上的植被信息。
研究发现不同氮水平下的水稻冠层、叶片和稻株的反射光谱具有差异,其叶面积指数和叶绿素含量与高光谱数据有相关性[5]。
高光谱技术在作物监测上的潜力也很大,它是有光谱范围广(400~2500nm)、波段多(512~1024个)、光谱分辨率高(3nm)、数据量大等优点,可以用来监测作物叶片和冠层的生化组分的状况和变化。
冬小麦苗期叶绿素含量检测光谱学参数寻优
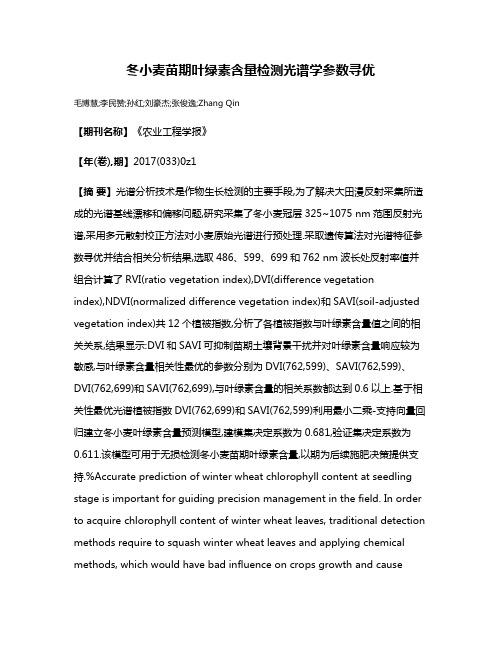
冬小麦苗期叶绿素含量检测光谱学参数寻优毛博慧;李民赞;孙红;刘豪杰;张俊逸;Zhang Qin【期刊名称】《农业工程学报》【年(卷),期】2017(033)0z1【摘要】光谱分析技术是作物生长检测的主要手段,为了解决大田漫反射采集所造成的光谱基线漂移和偏移问题,研究采集了冬小麦冠层325~1075 nm范围反射光谱,采用多元散射校正方法对小麦原始光谱进行预处理.采取遗传算法对光谱特征参数寻优并结合相关分析结果,选取486、599、699和762 nm波长处反射率值并组合计算了RVI(ratio vegetation index),DVI(difference vegetation index),NDVI(normalized difference vegetation index)和SAVI(soil-adjusted vegetation index)共12个植被指数,分析了各植被指数与叶绿素含量值之间的相关关系,结果显示:DVI和SAVI可抑制苗期土壤背景干扰并对叶绿素含量响应较为敏感,与叶绿素含量相关性最优的参数分别为DVI(762,599)、SAVI(762,599)、DVI(762,699)和SAVI(762,699),与叶绿素含量的相关系数都达到0.6以上.基于相关性最优光谱植被指数DVI(762,699)和SAVI(762,599)利用最小二乘-支持向量回归建立冬小麦叶绿素含量预测模型,建模集决定系数为0.681,验证集决定系数为0.611.该模型可用于无损检测冬小麦苗期叶绿素含量,以期为后续施肥决策提供支持.%Accurate prediction of winter wheat chlorophyll content at seedling stage is important for guiding precision management in the field. In order to acquire chlorophyll content of winter wheat leaves, traditional detection methods require to squash winter wheat leaves and applying chemical methods, which would have bad influence on crops growth and causeunnecessary waste of time on some level. It is proved that the spectroscopy analysis is an effective method to predict chlorophyll content of winter wheat. However, the drift and offset of spectral baseline has a great influence on the predicting accuracy. So, this study was carried out to eliminate the influence of the drift and offset. The experimental farm was randomly divided into 70 different sampling areas in Xiaotangshan, Beijing, and the winter wheat leaves were collected on April 20th in the period of seedling stage. The visible and near infrared canopy spectral reflectance of winter wheat was measured by an ASD FieldSpec handheld spectroradiometer at seedling stage. The chlorophyll contents of sampling leaves were detected by the spectrophotometer in the laboratory on the same day. The obtained data of the canopy spectral reflectance and chlorophyll content were assembled for each region individually. The multiple scattering correction (MSC) was used on the bands of 325-1025 nm wavelength, because many scattering errors were introduced into the measured spectral data due to the physical factors. The MSC method first requires establishing an ideal spectrum of all samples, and modifying all the other samples of near infrared spectra on the basis of ideal spectrum to, and spectral reflectance changes with the content of chlorophyll components in the sample meet the direct linear relationship. The absolute intensity difference of spectral reflectance of winter wheat canopy was weakened after the MSC pretreatment, and then scattering effect was effectively reduced. Baseline shift and offset problems were resolved, and the correlation coefficients of spectral reflectance and chlorophyll contentwere increasing through the MSC pretreatment. Furthermore, genetic algorithm (GA) was proposed for sensitive band selection. GA is a high efficient and globally random search optimization method which simulates Darwin's evolution by natural selection and genetic mechanism of biological evolution. According to the principles of choosing high frequency bands as characteristic wavelength, 486, 599, 699 and 762 nm crop canopy reflectances were selected to calculate vegetation indices, including ratio vegetation index (RVI), difference vegetation index (DVI), normalized difference vegetation index (NDVI) and soil-adjusted vegetation index (SAVI). The correlation between each vegetation index and chlorophyll content of winter wheat was analyzed. It was found that the correlation between each vegetation index and chlorophyll content of winter wheat significantly increased after the MSC. The results showed that DVI and SAVI could refrain interference of soil background during seedling period, and the optimal parameters were DVI(762, 599), SAVI(762, 599), DVI(762, 699) and SAVI(762, 699), and the correlation coefficients were all above 0.6. The DVI(762, 699) and SAVI(762, 599) were selected to establish the multiple linear regression (MLR) prediction model and the least squares-support vector regression (LS-SVR) prediction model. The 70 winter wheat samples were divided into 2 groups, 50 samples for model calibration and the other 20 samples for model verification. The results of MLR showed the determination coefficient of the calibration model was 0.528 and that of the validation model was 0.487. In order to improve the precision of the forecast model, the LS-SVR prediction model was applied,and the determination coefficient of the calibration model was 0.681 and that of the validation model was 0.611. It showed that the fitting result was ideal. With the application of spectral technology, it provides a feasible method to detect the winter wheat growth status at seedling stage.【总页数】6页(P164-169)【作者】毛博慧;李民赞;孙红;刘豪杰;张俊逸;Zhang Qin【作者单位】中国农业大学现代精细农业系统集成研究教育部重点实验室,北京100083;中国农业大学现代精细农业系统集成研究教育部重点实验室,北京100083;中国农业大学现代精细农业系统集成研究教育部重点实验室,北京 100083;中国农业大学现代精细农业系统集成研究教育部重点实验室,北京 100083;中国农业大学现代精细农业系统集成研究教育部重点实验室,北京 100083;美国华盛顿州立大学精细农业及农业自动化研究中心,Prosser,WA 99350【正文语种】中文【中图分类】S127【相关文献】1.基于近红外光谱苗期玉米叶片叶绿素含量的无损检测方法 [J], 翟哲;李伟凯2.基于高光谱和PLS-LS-SVM的冬小麦叶绿素含量检测 [J], 王伟;彭彦昆;王秀;马伟3.冬小麦叶绿素含量高光谱检测技术 [J], 王伟;彭彦昆;马伟;黄慧;王秀4.增水和施肥对苜蓿-冬小麦轮作系统冬小麦叶片光合速率和叶绿素含量的影响 [J], Zhang Xiaolin;Chen Mengtian;Zhai Penghui;Zhao Xiang;Dong Kuanhu5.苗期低温胁迫对“红颜”草莓叶绿素含量及冠层高光谱的影响 [J], 徐若涵;杨再强;申梦吟;王明田因版权原因,仅展示原文概要,查看原文内容请购买。
基于无人机多光谱遥感的冬小麦参数反演及综合长势监测
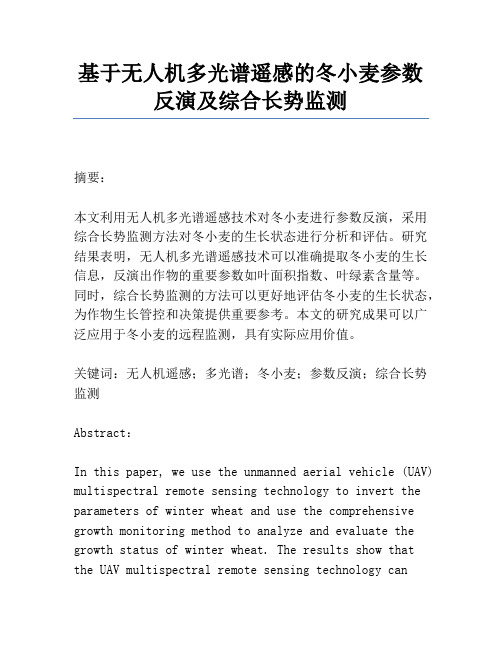
基于无人机多光谱遥感的冬小麦参数反演及综合长势监测摘要:本文利用无人机多光谱遥感技术对冬小麦进行参数反演,采用综合长势监测方法对冬小麦的生长状态进行分析和评估。
研究结果表明,无人机多光谱遥感技术可以准确提取冬小麦的生长信息,反演出作物的重要参数如叶面积指数、叶绿素含量等。
同时,综合长势监测的方法可以更好地评估冬小麦的生长状态,为作物生长管控和决策提供重要参考。
本文的研究成果可以广泛应用于冬小麦的远程监测,具有实际应用价值。
关键词:无人机遥感;多光谱;冬小麦;参数反演;综合长势监测Abstract:In this paper, we use the unmanned aerial vehicle (UAV) multispectral remote sensing technology to invert the parameters of winter wheat and use the comprehensive growth monitoring method to analyze and evaluate the growth status of winter wheat. The results show that the UAV multispectral remote sensing technology canaccurately extract the growth information of winter wheat and invert important parameters of crops such as leaf area index and chlorophyll content. At the same time, the method of comprehensive growth monitoring can better evaluate the growth status of winter wheat, which provides important reference for crop growth management and decision-making. The research results of this paper can be widely used in the remote monitoring of winter wheat and have practical application value.Keywords: UAV remote sensing; multispectral; winter wheat; parameter inversion; comprehensive growth monitorinWinter wheat is an important crop in many regions of the world, and its growth status plays an important role in its yield and quality. In recent years, with the development of remote sensing technology, UAV remote sensing has become an effective tool for monitoring crop growth. In this study, a multispectral remote sensing method was used to monitor the growth status of winter wheat.The study found that leaf area index (L) and chlorophyll content are important indicators of winter wheat growth status. The multispectral remote sensingmethod was able to accurately estimate these parameters. The results showed that L and chlorophyll content were positively correlated with winter wheat yield and biomass.In addition, a method of comprehensive growth monitoring was proposed in this study. This method combined the estimation of L and chlorophyll content with other growth parameters, such as height, biomass, and vegetation index, to evaluate the overall growth status of winter wheat. The results of the comprehensive growth monitoring provided important reference for crop growth management and decision-making.Overall, the research results of this paper demonstrate the potential of UAV remote sensing for monitoring the growth status of winter wheat. The use of multispectral remote sensing and comprehensive growth monitoring can provide accurate and reliable information for crop management and decision-making. These methods can be widely used in the remote monitoring of winter wheat and have practical application valueIn conclusion, the use of UAV remote sensing for monitoring winter wheat growth can greatly enhancecrop management and decision-making. By employing multispectral remote sensing and comprehensive growth monitoring, accurate and reliable information can be obtained for effective crop management. The results of the study also demonstrate that these methods have practical application value and can be widely used in the remote monitoring of winter wheat.However, there are some limitations that need to be considered when using UAV remote sensing for crop monitoring. For instance, the use of UAVs can be limited by weather conditions such as strong wind and rain, which can affect the accuracy of the data obtained. Additionally, UAVs have limited flight duration and payload capacity, which can limit the scope and accuracy of the data collected.Despite these limitations, the use of UAV remote sensing for crop monitoring is a promising technology that can greatly improve crop management and decision-making. Future research could focus on developing more advanced UAVs that can overcome the limitations posed by weather conditions, flight duration, and payload capacity. Further research could also focus on improving the accuracy of the data collected and developing models that can accurately predict crop growth and yield based on this data.In conclusion, the use of UAV remote sensing has the potential to revolutionize crop monitoring and management. The technology can provide accurate and reliable data for effective decision-making and can help farmers optimize their crop yields while minimizing their environmental impact. It is a promising tool that could be widely used in the future to enhance food security and sustainabilityAnother potential benefit of UAV remote sensing is its ability to detect crop stress and disease at an early stage. By collecting images of crops with high resolution cameras, it is possible to identify areas of plants that are experiencing stress or displaying signs of disease. This information can then be used to take preventative measures such as applyingfertilizers in certain areas or implementing disease management strategies before the issue becomes widespread.Furthermore, UAV remote sensing can also help reduce the cost of crop management. Traditional crop management practices such as manual scouting and data collection can be time-consuming and expensive. With UAVs, large areas of crops can be surveyed in a short amount of time, and data can be automaticallycollected and analyzed, reducing the need for manual labor and increasing efficiency.Despite the benefits of UAV remote sensing, there are also some challenges that need to be addressed. One of the biggest challenges is the regulatory framework around UAV use. In some countries, regulations can be restrictive in terms of operating UAVs in agricultural areas. Additionally, the cost of the technology may be prohibitive for some farmers, particularly smallholders.Another challenge is the need for accurate andreliable data processing and analysis. UAVs can produce vast amounts of data, which must be processed and analyzed in a timely manner to be useful. This can require specialized knowledge and equipment, which may not be available to all farmers.In conclusion, UAV remote sensing has the potential to greatly benefit the agriculture industry by providing accurate and reliable data for crop monitoring and management. With the ability to detect crop stress and disease at an early stage, reduce costs and increase efficiency, UAVs are a promising tool that could be widely used in the future to enhance food security and sustainability. However, it is important to addressthe challenges around regulation and data processing to ensure that the technology can be effectively utilized by all farmersIn conclusion, unmanned aerial vehicles (UAVs) have the potential to revolutionize the agriculture industry. They can provide accurate and reliable data for crop monitoring and management, enable early detection of crop stress and disease, reduce costs, and increase efficiency. However, the challenges of regulation and data processing must be addressed to ensure that farmers of all sizes can benefit from this promising technology. UAVs have the potential to enhance food security and sustainability, and their adoption could be a game-changer for the agriculture industry。
基于光谱指数的冬小麦冠层叶绿素含量估算模型研究

基于光谱指数的冬小麦冠层叶绿素含量估算模型研究罗丹;常庆瑞;齐雁冰;李媛媛;李松【期刊名称】《麦类作物学报》【年(卷),期】2016(36)9【摘要】为探索对冬小麦冠层叶绿素含量反应敏感的高光谱波段组合,同时比较不同光谱指数对小麦冠层叶绿素含量的估测效果,通过分析350-2500nm波段范围内原始光谱反射率及其一阶导数光谱的任意两两波段交叉组合而成的主要高光谱指数与冬小麦冠层叶片叶绿素含量的定量关系,建立冬小麦冠层叶绿素含量估算模型。
结果表明,选用归一化光谱指数(NDSI)、比值光谱指数(RSI)、差值光谱指数(DSI)和土壤调节光谱指数(SASI)建立的冬小麦冠层叶绿素含量监测模型决定系数均大于0.71,标准误差均小于1.842。
利用独立试验资料进行检验,表现最好的是RSI(FD689,FD609)和SASI(R491,R666)L=0.01,预测精度高达98.2%,模型精确度和可靠性较高。
【总页数】9页(P1225-1233)【关键词】冬小麦;叶绿素;光谱指数;估算模型【作者】罗丹;常庆瑞;齐雁冰;李媛媛;李松【作者单位】西北农林科技大学资源环境学院【正文语种】中文【中图分类】S512.1;S313【相关文献】1.基于光谱变换的冬小麦冠层SPAD值估算模型研究 [J], 林少喆;彭致功;张宝忠;魏征;张倩;韩娜娜;刘露;王春堂;冯哲2.基于无人机多光谱遥感的冬小麦冠层叶绿素含量估测研究 [J], 魏青;张宝忠;魏征;韩信;段晨斐3.植被指数方法估算冬小麦冠层叶绿素含量的角度效应研究 [J], 何宇航;周贤锋;张竞成;张垚;陈冬梅;吴开华;黄文江;孔维平;徐俊锋4.基于优化光谱指数的新疆春小麦冠层叶绿素含量估算 [J], 亚森江.喀哈尔;尼加提.卡斯木;尼格拉.塔什甫拉提;张飞;茹克亚.萨吾提;阿不都艾尼.阿不里;师庆东;苏比努尔.居来提5.基于PROSAIL模型的玉米冠层叶面积指数及叶片叶绿素含量反演方法研究 [J], 张明政;苏伟;朱德海因版权原因,仅展示原文概要,查看原文内容请购买。
- 1、下载文档前请自行甄别文档内容的完整性,平台不提供额外的编辑、内容补充、找答案等附加服务。
- 2、"仅部分预览"的文档,不可在线预览部分如存在完整性等问题,可反馈申请退款(可完整预览的文档不适用该条件!)。
- 3、如文档侵犯您的权益,请联系客服反馈,我们会尽快为您处理(人工客服工作时间:9:00-18:30)。
1 引言小麦是我国主要的粮食作物之一,其产量是各级政府进行决策、生产部门指导生产、流通领域安排粮食收购和销售、交通部门安排运输计划的重要经济信息。
因而小麦产量的准确预测对我国农业和经济的发展具有重要意义。
遥感技术和地理信息系统的引入为解决这一问题提供了有效手段[1]。
遥感估产小麦种植是通过卫星可见光和近红外波段进行组合,形成各种植被指数,根据植被的光谱特性,来研究植被演化信息[2]。
近20年来利用遥感进行作物实时监测和营养快速诊断一直是遥感在农业中应用的研究热点。
高光谱遥感技术能通过监测作物生育时期的光谱变化研究作物反射光谱和叶绿素含量、叶面积指数等农学参数之间的关系,为作物遥感长势监测和遥感估产提供依据[3]。
利用卫星遥感信息进行农作物的长势监测和产量估计,主要依据农田作物光谱的反射特征,即同一作物在同一时间的不同波段以及在不同时间内具有不同的反射辐射状况。
然而,农田作物光谱实际上是多种光谱混合的结果,但微分光谱、植被指数等技术在水稻农学参数测定研究应用中有着很好的表现[4],除作物本身状况外,农田作物光谱受土壤背景状况的影响由于栽培措施不同,作物本身又出现各种生长状况,因而可表现出各种各样的光谱状况。
卫星遥感信息反映的是野外条件下地物的反射辐射状况,因而可表现出各种各样的光谱状况。
卫星遥感信息反映的是野外条件下地物的反射辐射状况,田间野外测定的地面光谱与其具有很好的可比性。
从高光谱数据中提取植被指数进行长势监测和氮营养诊断也是其中重要的内容,研究表明红光和近红外波段的不同组合进行植被研究非常好,这些波段包含90%以上的植被信息。
研究发现不同氮水平下的水稻冠层、叶片和稻株的反射光谱具有差异,其叶面积指数和叶绿素含量与高光谱数据有相关性[5]。
高光谱技术在作物监测上的潜力也很大,它是有光谱范围广(400~2500nm)、波段多(512~1024个)、光谱分辨率高(3nm)、数据量大等优点,可以用来监测作物叶片和冠层的生化组分的状况和变化。
但它作为成熟的技术应用到作物遥感监测上之前,首先要进行地面模拟和测试,确定传感器测量光谱范围、波段设置(波段数、宽度、位置)和评价遥感数据的应用潜力[6]。
由于地面遥感技术的发展,应用冠层反射光谱估测植物冠层化学组成的前景正引起越来越多的关注。
国外学者早在20世纪80年代开始了大量研究[7,8],我国起步相对较晚。
近年来,已有一些有关作物冠层反射光谱的变化规律及氮素敏感波段选择的研究[9,10],并对地面高光谱数据的红边特性与作物生化参数的关系作了有意义的探讨[11]。
随着高分辨率遥感技术研究的深入,利用弱光谱差异对地物特征进行定量分析的研究得以广泛开。
许多学者利用宽波段光谱和高光谱技术对玉米的生物物理和化学参数作了大量研究。
在玉米上研究表明,近红外光谱反射率(760~900nm)与红光光谱反射率(630~690nm)的比值比单一波段的光谱反射率能更好地区分氮的不同处理[12]。
吴长山等提出利用玉米群体的反射光谱数据能很好地反映叶片叶绿素密度的信息[13]。
周学秋和朱雨杰依据不同生育阶段和不同灌溉条件下小麦冠层反射光谱特征,找出最能有效区分生育阶段和灌溉条件的波段,并利用模糊聚类的方法加以区分[14,15]。
薛利红等通过光谱分析可以识别不同栽培措施和生长状况下的水稻群体[16]。
谭昌伟等[17]探讨了夏玉米叶片全氮、叶绿素及叶面积指数的光谱响应,并建立了相关估测模型。
但就利用某单一叶片光谱反射率对玉米整个生育期进行监测,找出诊断氮素营养状况的敏感时期并没有做一揭示;而且对叶片全氮和叶绿素含量与整个生育期某单一叶片光谱反射率的相关性未做系统的研究。
叶绿素含量和植被的发育阶段以及氮素状况有较好的相关性,它通常是氮素胁迫和植被发育阶段的指示器。
由于叶片含氮量和叶绿素之间的变化趋势相似,所以可以通过测定叶绿素来监测植株氮素营养[18]。
近年来,遥感无损监测技术的发展为作物生理生化参数的监测提供了新的方法和手段,对于作物长势监测、营养诊断和产量估测等均呈现出良好的应用前景。
叶片氮素营养对于作物长势、产量和品质的形成都具有重要意义。
在利用冠层反射光谱监测叶片氮素积累量的基础上,结合小麦氮素营养诊断的理论知识与实践经验,构建小麦氮素营养光谱诊断模型,可以推动精确农业的发展及应用,这也是今后进一步研究的内容[19]。
不同氮素营养水平冬小麦的冠层光谱存在差异,表明冬小麦光谱反射率曲线变化的规律性和提取的植被指数变化规律性与其生长发育的群体变化规律是对应的。
对冬小麦冠层的光谱测定,从中提取有用的植被信息可以估算冬小麦的农学参数,包括物理指数和叶片生化成分,进行不同氮素营养水平下的冬小麦长势监测和氮素营养亏盈诊断。
本文研究了不同氮素营养水平冬小麦生育期间叶片叶绿素含量的变化,并利用多光谱技术对其叶片冠层的光谱反射率进行了监测,而且将这两个光谱参数和农学参数作了对应分析。
通过分析叶绿素含量CHLC与近红外与绿光比值NIR/G在生育期内各个阶段的相关性,得出灌浆期间CHLC与近红外与绿光比值存在极显著的正相关,表明运用光谱指数NIR/G来反演叶绿素含量,进而用来预测预报籽粒品质是切实可行的,为采用光谱方法监测作物长势和诊断作物氮素营养状况提供新的技术措施。
2 材料与方法2.1实验材料供试土壤:山西农业大学校内试验田,土壤质地为黄土母质上发育的石灰性褐土,土壤肥力水平中等,土壤有机质含量22.01g/kg;碱解氮53.8mg/kg;有效磷18.43mg/kg;速效钾236.9mg/kg。
供试品种:京9428(直立叶多穗型)P1组,超优66(披散叶大穗型)P2组。
供试肥料:N肥(尿素含氮46.4%),P肥(过磷酸钙含P2O516%)。
2.2实验设计肥料单因素试验,采用随机区组设计,氮肥为尿素,用做基肥一次性施入;磷肥不设处理作为基肥在播种前一次施入,施入量为9Kg/亩。
此试验为常规灌溉。
小区面积2×3m2,行距为20cm,重复3次,田间管理各处理相同。
进行5个不同N素水平处理,分别为:N0(不施氮)、N1(缺氮10kg/hm2)、N2(氮适中20kg/hm2)、N3(氮过量25kg/hm2)、N4(氮严重过量30kg/hm2)。
播种时间2009年10月11日,播量为225kg/hm2。
2.3测定项目与方法2.3.1叶绿素含量(CHLC)测定分别于试管中加入5mL提取液,取当时已全展的最顶部及其邻位下叶,取中部去叶脉剪碎后混匀供试。
把叶片剪成宽度小于2mm的细丝或小块,混匀,分别准确称取100mg放入盛有提取液的容量瓶中,封口,置于黑暗条件下直接浸提。
丙酮与乙醇(2∶1)效果最佳,且最优的浸提效果出现在16h左右。
试剂:80%丙酮叶绿素含量(mg·g-1)=(C×V)/(W×1000)式中,C:叶绿素浓度(mg·L-1);V:提取液总体积(ml);W:叶片鲜重(g)2.3.2光谱测定和取样时期田间光谱测试日期分别为:3月25日(返青期),4月15日(拔节期),5月6日(孕穗期),5月16日(抽穗期),5月22日(开花期),6月9日(灌浆期),6月19日(成熟期)。
对小麦进行光谱采集,同时进行破坏性采样。
2.3.3小麦冠层光谱的测定实验区小麦各小区的群体光谱数据测量采用美国Cropscan公司生产的MSR-16R型多光谱野外便携式辐射计,仪器视场角为31度,波段特征见表1。
表1.Cropscan波段的中心波长和带宽波段Band460510560610660680710760中心波长(nm)Center wavelength带宽(nm)Band width 461.38.3510.68.1561.68.5609.910.0659.110.8680.911.3711.89.2759.59.8波段Band81087095011001220148015001650中心波长(nm)Center wavelength带宽(nm)Band width 810.110.7871.612.5951.011.01101.916.31222.811.51481.113.51500.913.21669.0195.0该仪器的光谱分辨率已经达到高光谱的光谱分辨率范围,且覆盖了小麦的敏感波段区(500nm-900nm)。
其中可见光为460nm、500nm、550nm、600nm、650nm、670nm、730nm;近红外为780nm、800nm、850nm、950nm、1050nm、1100nm、1200nm、1260nm、1460nm。
小麦冠层反射光谱测量从拔节期、孕穗期、抽穗期、开花期、灌浆期、成熟期各测量一次,每个小区各重复测量三次后取平均值。
测量选择在晴朗无云的日子进行,时段在10:00-14:00之间。
测量时探头垂直向下,距冠层垂直高度保持在1.5m左右。
仪器每季度用白色标准版校准一次。
2.4数据分析通过实验测定计算并记录叶绿素含量数据以及R800与R550光谱反射率,并通过近红外与绿光比值(NIR/G)NIR/G=R800/R550公式得出数值(R为反射率;NIR为近红外光反射率;G为绿光反射率)。
分析冬小麦在不同氮素水平下CHLC与NIR/G随不同生育时期的变化,分析冬小麦叶绿素含量和高光谱植被指数近红外与绿光比值的相关性,然后利用线性回归分析建立相应的模型。
3 结果与分析3.1不同氮素水平下叶绿素含量(CHLC)随生育期的变化图1 不同氮素水平京9428(a)和超优66(b)叶绿素含量CHLC随生育期的变化由图1可知,冬小麦京9428叶绿素含量从孕穗期开始增加,到开花期达到最大值,开花期后,其CHLC随生育期推进迅速降低。
这主要是由于孕穗期到开花期,冬小麦叶面积持续增加,叶绿素含量不断增加;而开花期后冬小麦慢慢成熟,叶片衰老,叶绿体解体,叶绿素含量明显下降。
超优66N3和N4处理叶绿素含量在灌浆期达到最大值,灌浆期之后迅速降低,这是由于施肥量的过量造成植株的徒长。
冬小麦京9428在开花期前叶绿素含量(CHLC)增加较为突出,而超优66在开花期之前叶绿素含量(CHLC)上升则较为缓慢。
3.2不同氮素水平下冬小麦(NIR/G)随生育期的变化图2 京9428(a)和超优66(b)不同氮素水平下冬小麦NIR/G随生育期的变化上图为京9428和超优66在不同氮素水平下NIR/G值随生育时期的变化图。
从中可以看出,京9428从返青期开始NIR/G值不断增加,到抽穗期达到最大值;抽穗期以后到灌浆期有减少,回升过程。
其后随生育时期的推进,NIR/G值值逐渐下降。
在不同施氮水平下冬小麦NIR/G存在差异,总体上随施氮量的增加呈上升趋势。