2104数学建模美赛A 交通流 安全性 参考资料 交通量
数学建模报告路口车况分析
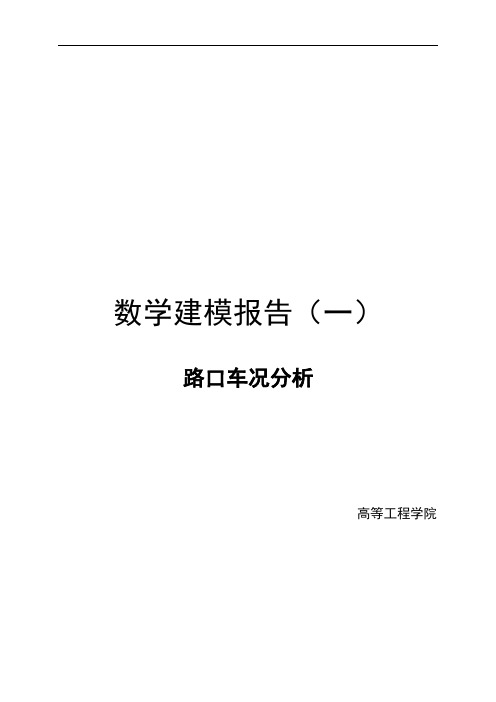
数学建模报告(一)路口车况分析高等工程学院一、路况信息我们在实验前为保证最终结果的客观性与代表性,综合分析了五道口附近各路口的Google Earth卫星地图与Baidu Map提供的实时车流预测信息,并最终选取城府路与学院路交叉十字路口(地理坐标39.99°N,116.35°E卫星照片见Figure 1)完成本次实地测量。
此路口北向车流较为密集,但几乎没有拥堵状况发生,且公交车等大型车数量较少。
南北向路段红灯时(时长60s),北向路段由西至东最内车道等待车数保持在15辆左右。
良好的路况与较大的样本量有利于我们检验教材模型参量取值的正确性,同时也有利于我们根据路口的实际车流情况,对原有模型进行完善。
Figure 1二、原始数据记录与处理我们的实验时间选定在2012年3月10日上午9:00-10:00。
具体测量内容如下:1. 北向路段,最内侧车道,绿灯亮至10s、20s、30s、60s时,通过停车线的汽车数量;2. 北向路段,最内侧车道,红灯区间的车辆间距;3. 北向路段,最内侧车道,停车线内第一辆汽车的启动延时时间,与其跑过位移S 所用时间(见Figure 2)。
关于数据采集的前期设计请参阅本文第五部分。
SFigure 22.1通过车次记录与数据波动分析我们测量了18次绿灯区间,当绿灯亮至10s、20s、30s、60s时汽车通过停车线的数量,具体数据列表如下:1 4812172 4713183 61219224 51014175 389126 4915207 4915198 41117269 410131810 49151611 37101912 410132113 510121514 59141915 48132116 59141517 371319Average 4.229.1713.6718.39数据波动分析如下图:Figure 3在实际观察中,我们发现,在每次红灯区间,停车排队等候的车辆数目稳定在12~15辆左右,且绿灯亮后前30s内通过的车辆,基本为之前停车排队等候的车辆。
数学建模--交通问题
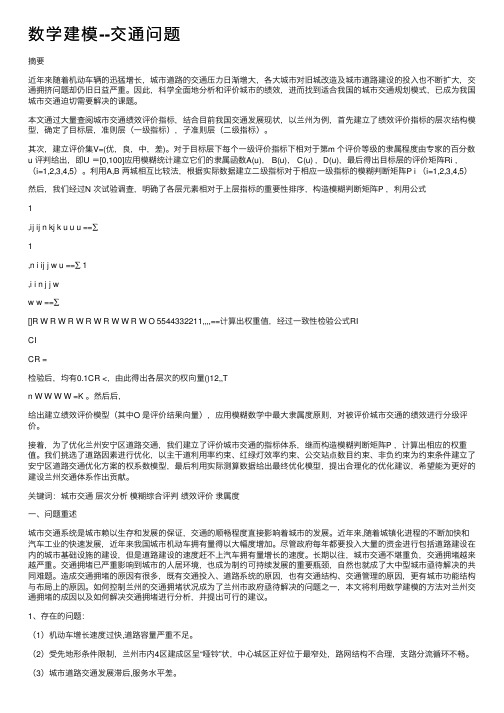
数学建模--交通问题摘要近年来随着机动车辆的迅猛增长,城市道路的交通压⼒⽇渐增⼤,各⼤城市对旧城改造及城市道路建设的投⼊也不断扩⼤,交通拥挤问题却仍旧⽇益严重。
因此,科学全⾯地分析和评价城市的绩效,进⽽找到适合我国的城市交通规划模式,已成为我国城市交通迫切需要解决的课题。
本⽂通过⼤量查阅城市交通绩效评价指标,结合⽬前我国交通发展现状,以兰州为例,⾸先建⽴了绩效评价指标的层次结构模型,确定了⽬标层,准则层(⼀级指标),⼦准则层(⼆级指标)。
其次,建⽴评价集V=(优,良,中,差)。
对于⽬标层下每个⼀级评价指标下相对于第m 个评价等级的⾪属程度由专家的百分数u 评判给出,即U =[0,100]应⽤模糊统计建⽴它们的⾪属函数A(u), B(u), C(u) ,D(u),最后得出⽬标层的评价矩阵Ri ,(i=1,2,3,4,5)。
利⽤A,B 两城相互⽐较法,根据实际数据建⽴⼆级指标对于相应⼀级指标的模糊判断矩阵P i (i=1,2,3,4,5)然后,我们经过N 次试验调查,明确了各层元素相对于上层指标的重要性排序,构造模糊判断矩阵P ,利⽤公式1,ij ij n kj k u u u ==∑1,n i ij j w u ==∑ 1,i i n j j ww w ==∑[]R W R W R W R W R W W R W O 5544332211,,,,==计算出权重值,经过⼀致性检验公式RICICR =检验后,均有0.1CR <,由此得出各层次的权向量()12,,Tn W W W W =K 。
然后后,给出建⽴绩效评价模型(其中O 是评价结果向量),应⽤模糊数学中最⼤⾪属度原则,对被评价城市交通的绩效进⾏分级评价。
接着,为了优化兰州安宁区道路交通,我们建⽴了评价城市交通的指标体系,继⽽构造模糊判断矩阵P ,计算出相应的权重值。
我们挑选了道路因素进⾏优化,以主⼲道利⽤率约束、红绿灯效率约束、公交站点数⽬约束、⾮负约束为约束条件建⽴了安宁区道路交通优化⽅案的权系数模型,最后利⽤实际测算数据给出最终优化模型,提出合理化的优化建议,希望能为更好的建设兰州交通体系作出贡献。
全国数学建模2021a题
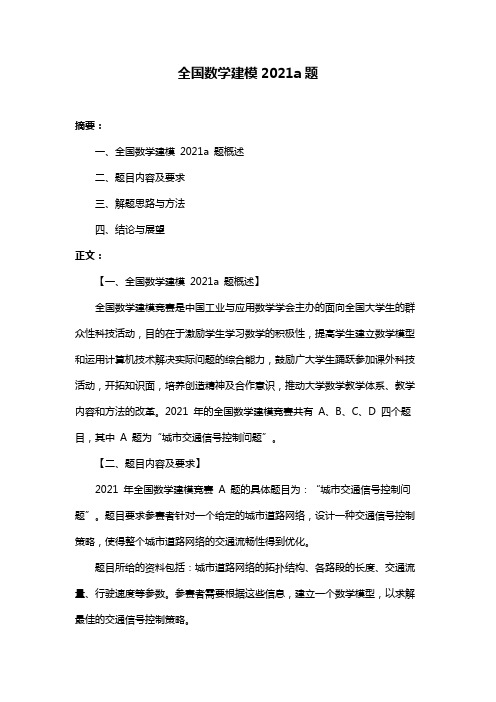
全国数学建模2021a题摘要:一、全国数学建模2021a 题概述二、题目内容及要求三、解题思路与方法四、结论与展望正文:【一、全国数学建模2021a 题概述】全国数学建模竞赛是中国工业与应用数学学会主办的面向全国大学生的群众性科技活动,目的在于激励学生学习数学的积极性,提高学生建立数学模型和运用计算机技术解决实际问题的综合能力,鼓励广大学生踊跃参加课外科技活动,开拓知识面,培养创造精神及合作意识,推动大学数学教学体系、教学内容和方法的改革。
2021 年的全国数学建模竞赛共有A、B、C、D 四个题目,其中A 题为“城市交通信号控制问题”。
【二、题目内容及要求】2021 年全国数学建模竞赛A 题的具体题目为:“城市交通信号控制问题”。
题目要求参赛者针对一个给定的城市道路网络,设计一种交通信号控制策略,使得整个城市道路网络的交通流畅性得到优化。
题目所给的资料包括:城市道路网络的拓扑结构、各路段的长度、交通流量、行驶速度等参数。
参赛者需要根据这些信息,建立一个数学模型,以求解最佳的交通信号控制策略。
【三、解题思路与方法】针对这道题目,我们可以采用以下步骤进行解题:1.首先,根据题目所给的信息,对城市道路网络进行分析,建立道路网络的拓扑结构模型。
2.其次,根据交通流量、行驶速度等参数,建立交通流的动力学模型,描述交通流在各路段上的变化规律。
3.接着,利用图论中的最短路径理论,求解交通流在道路网络中的最短路径,从而确定交通流的传播方向。
4.最后,根据交通流的传播方向和交通流的变化规律,设计交通信号控制策略,使得整个城市道路网络的交通流畅性得到优化。
【四、结论与展望】通过以上步骤,我们可以得出一套针对给定城市道路网络的交通信号控制策略。
该策略能够有效优化城市道路网络的交通流畅性,提高道路通行效率,降低交通拥堵程度。
然而,需要注意的是,由于城市交通信号控制问题具有较强的实时性和动态性,因此,本题的解决方案需要结合实际交通情况进行不断调整和优化。
数学建模在交通流量分析中的应用
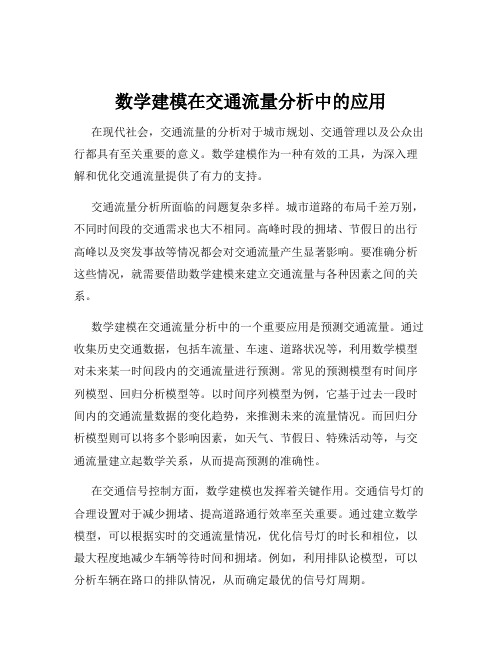
数学建模在交通流量分析中的应用在现代社会,交通流量的分析对于城市规划、交通管理以及公众出行都具有至关重要的意义。
数学建模作为一种有效的工具,为深入理解和优化交通流量提供了有力的支持。
交通流量分析所面临的问题复杂多样。
城市道路的布局千差万别,不同时间段的交通需求也大不相同。
高峰时段的拥堵、节假日的出行高峰以及突发事故等情况都会对交通流量产生显著影响。
要准确分析这些情况,就需要借助数学建模来建立交通流量与各种因素之间的关系。
数学建模在交通流量分析中的一个重要应用是预测交通流量。
通过收集历史交通数据,包括车流量、车速、道路状况等,利用数学模型对未来某一时间段内的交通流量进行预测。
常见的预测模型有时间序列模型、回归分析模型等。
以时间序列模型为例,它基于过去一段时间内的交通流量数据的变化趋势,来推测未来的流量情况。
而回归分析模型则可以将多个影响因素,如天气、节假日、特殊活动等,与交通流量建立起数学关系,从而提高预测的准确性。
在交通信号控制方面,数学建模也发挥着关键作用。
交通信号灯的合理设置对于减少拥堵、提高道路通行效率至关重要。
通过建立数学模型,可以根据实时的交通流量情况,优化信号灯的时长和相位,以最大程度地减少车辆等待时间和拥堵。
例如,利用排队论模型,可以分析车辆在路口的排队情况,从而确定最优的信号灯周期。
数学建模还能够用于评估交通规划方案的效果。
当城市规划新的道路、桥梁或者交通枢纽时,可以通过建立交通流模型来模拟不同方案下的交通流量分布。
这有助于比较不同方案的优劣,选择最能满足交通需求、提高交通效率的规划方案。
比如,在规划一个新的商业区时,可以通过建模预测未来的交通流量增长,从而提前规划相应的交通设施。
在分析交通事故对交通流量的影响时,数学建模同样不可或缺。
事故发生后,道路通行能力下降,车辆排队长度增加。
通过建立事故影响模型,可以估计事故造成的拥堵范围和持续时间,为交通管理部门采取及时有效的疏导措施提供依据。
全美数学建模大赛A论文-环岛城市交通

摘 要一、本文主要有三个数学模型:1. 通过环岛的理想模型,分析推出计算环岛的最大交通能力;对比设置停让交通标志控制以及信号灯控制对环岛通行能力。
得出:当经过环岛的实际流量'Q <环岛的最大通行能力Z Q ,应用指示牌控制法较宜,做法是在交通环岛的各个进口处设置指示牌,并设置环岛内交通车流的方向指示牌。
当'Z Q Q ≥时,宜采用信号灯控制法,并采用指示牌控制法予以辅助。
信号控制的目的在于最大限度地提高交叉口的使用效率。
2. 引入精英蚂蚁寻优策略模型。
针对城市道路交叉口的交通流特性,对单路口交通信号多相位实时控制的模型和算法进行研究。
采用能随交通需求的变化而实时变化的加权系数,将交叉口3 个优化目标函数转化为单目标函数优化的问题。
为提高模型的计算速度以及降低交叉口信号机的单机计算量,采用蚂蚁算法中的精英蚂蚁寻优3. 策略求解模型。
模型的目标方程为:ﻩ 42421411(1(/))min (,)2(1.0)[(1.0/)]/[2(1.0)](2(/))1.1(1.0)0.9(1.0/)/(1.0)2(3600/)(/)i i i i i i i i i i i i i i i Zl c Z x c s y Y c x c y l c s y Y c x c y c Y x c s Q ===-=⋅-⋅-⋅-++⋅⋅-⋅⋅⋅---⋅⋅⋅+∑∑∑4. 基于精英蚂蚁寻优策略模型,对其进行优化得到理想状态下计算信号灯系统中各路口的绿灯时间的目标方程z max (,)[2(3600/)(/)]Q i i Z x c c Y x c s =⋅⋅⋅+∑,引入算例,将算例所提供的数据代入优化得到的模型,使用软件求解。
当通过交通工程师通过观察法得到平稳期、高峰期的Y,S,当预设C 值,即可通过上述计算方法获得最大的通行量的四个信号的绿灯时长配置。
该优化模型可以将其应用到交通环岛各路口红绿灯时长的控制,并用交通标志配合控制交通流量。
2014美国数学建模竞赛赛题翻译
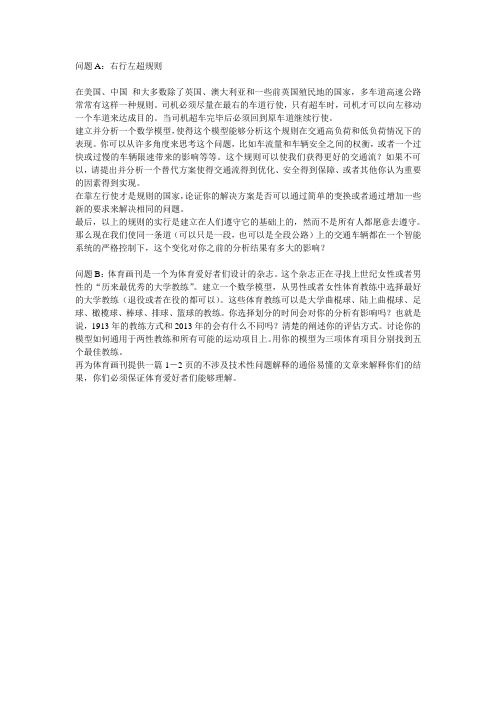
问题A:右行左超规则在美国、中国和大多数除了英国、澳大利亚和一些前英国殖民地的国家,多车道高速公路常常有这样一种规则。
司机必须尽量在最右的车道行使,只有超车时,司机才可以向左移动一个车道来达成目的。
当司机超车完毕后必须回到原车道继续行使。
建立并分析一个数学模型,使得这个模型能够分析这个规则在交通高负荷和低负荷情况下的表现。
你可以从许多角度来思考这个问题,比如车流量和车辆安全之间的权衡,或者一个过快或过慢的车辆限速带来的影响等等。
这个规则可以使我们获得更好的交通流?如果不可以,请提出并分析一个替代方案使得交通流得到优化、安全得到保障、或者其他你认为重要的因素得到实现。
在靠左行使才是规则的国家,论证你的解决方案是否可以通过简单的变换或者通过增加一些新的要求来解决相同的问题。
最后,以上的规则的实行是建立在人们遵守它的基础上的,然而不是所有人都愿意去遵守。
那么现在我们使同一条道(可以只是一段,也可以是全段公路)上的交通车辆都在一个智能系统的严格控制下,这个变化对你之前的分析结果有多大的影响?问题B:体育画刊是一个为体育爱好者们设计的杂志。
这个杂志正在寻找上世纪女性或者男性的“历来最优秀的大学教练”。
建立一个数学模型,从男性或者女性体育教练中选择最好的大学教练(退役或者在役的都可以)。
这些体育教练可以是大学曲棍球、陆上曲棍球、足球、橄榄球、棒球、排球、篮球的教练。
你选择划分的时间会对你的分析有影响吗?也就是说,1913年的教练方式和2013年的会有什么不同吗?清楚的阐述你的评估方式。
讨论你的模型如何通用于两性教练和所有可能的运动项目上。
用你的模型为三项体育项目分别找到五个最佳教练。
再为体育画刊提供一篇1-2页的不涉及技术性问题解释的通俗易懂的文章来解释你们的结果,你们必须保证体育爱好者们能够理解。
2014美赛A题讲解

2021/3/7
CHENLI
10
用CA模型描述交通流,主要是通过对驾驶员驾车行为的 分析,建立驾驶员或车辆的行为输出规则,从而建立元胞 自动机的运行规则,然后仿真实际交通背景,模拟整体元 胞群的动态过程,从中得到解决交通问题的方法或信息。 这类模型考虑的主要问题包括:如何用微观规则的建模来 描述元胞的运动过程;如何用宏观规则的建模来描述高速 公路运行车辆的随机性,这是交通现象的重要特征。
2021/3/7
CHENLI
13
2)平均车速( v ):
在固定路段内不同车道上所有车辆的平均时速的平均值,
过程中提取;其次,建立高速公路上车辆的运行状态随时间
变化的微观规则模型,特别是“右行左超车”的规则模型;
第三是运行车辆的不同运行状态改变的宏观概率分布,这是
由交通问题的随机性决定的, 而随机性是交通问题的一个特
别重要的特性;最后是设计元胞自动机的仿真对比试验,特
2别021/是3/7元胞群空间、初始元胞群CH密ENL度I 、分布与状态等。
2014美赛A题建模与求解
讲解人:王永丽
2014年8月11日
2021/3/7
CHENLI
1
提纲
问题描述 求解方案
1、问题分析与模型建立 2、模型求解与计算机仿真
模型优缺点分析与改进
2021/3/7
CHENLI
2
问题描述
题目:除非超车否则靠右行驶的交通规则
问题:在一些汽车靠右行驶的国家(比如美国,中国等),
2021/3/7
CHENLI
数学建模在交通流量中的应用
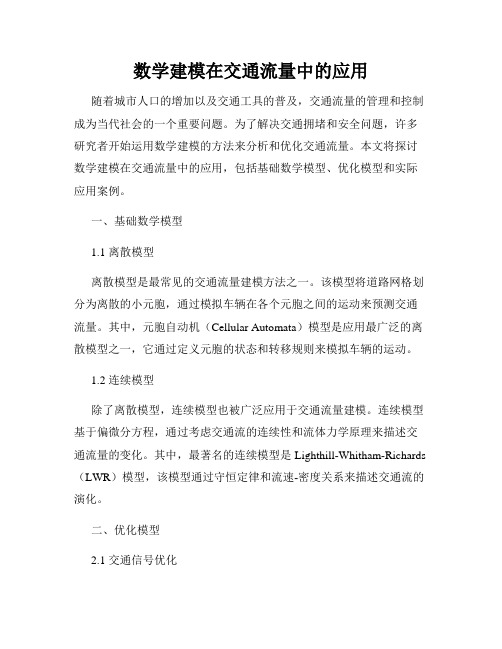
数学建模在交通流量中的应用随着城市人口的增加以及交通工具的普及,交通流量的管理和控制成为当代社会的一个重要问题。
为了解决交通拥堵和安全问题,许多研究者开始运用数学建模的方法来分析和优化交通流量。
本文将探讨数学建模在交通流量中的应用,包括基础数学模型、优化模型和实际应用案例。
一、基础数学模型1.1 离散模型离散模型是最常见的交通流量建模方法之一。
该模型将道路网格划分为离散的小元胞,通过模拟车辆在各个元胞之间的运动来预测交通流量。
其中,元胞自动机(Cellular Automata)模型是应用最广泛的离散模型之一,它通过定义元胞的状态和转移规则来模拟车辆的运动。
1.2 连续模型除了离散模型,连续模型也被广泛应用于交通流量建模。
连续模型基于偏微分方程,通过考虑交通流的连续性和流体力学原理来描述交通流量的变化。
其中,最著名的连续模型是Lighthill-Whitham-Richards (LWR)模型,该模型通过守恒定律和流速-密度关系来描述交通流的演化。
二、优化模型2.1 交通信号优化交通信号优化是交通流量管理的重要方面之一。
数学建模可以帮助确定最佳的信号配时方案,以最大程度地减少车辆的停顿和排队时间。
优化模型通常考虑交通状况、道路容量和信号周期等因素,并利用数学算法来寻找最佳解。
2.2 路网设计优化路网设计是指在给定的地理环境和交通需求下,确定最佳的道路布局和连接方式。
数学建模可以通过考虑交通流量分配、路段容量和成本等因素,来优化路网设计。
常用的优化方法包括线性规划、整数规划和遗传算法等。
三、实际应用案例3.1 城市交通拥堵预测数学建模可以根据历史交通流量数据、天气信息和事件影响等因素,来预测城市交通的拥堵情况。
通过建立拥堵预测模型,交通管理部门可以提前采取措施,减轻拥堵状况并优化交通流量。
3.2 交通事故预测与分析数学建模也可以帮助预测和分析交通事故的发生概率和影响因素。
通过建立统计模型和机器学习算法,研究者可以识别出导致事故的关键因素,并提出相应的安全措施。
- 1、下载文档前请自行甄别文档内容的完整性,平台不提供额外的编辑、内容补充、找答案等附加服务。
- 2、"仅部分预览"的文档,不可在线预览部分如存在完整性等问题,可反馈申请退款(可完整预览的文档不适用该条件!)。
- 3、如文档侵犯您的权益,请联系客服反馈,我们会尽快为您处理(人工客服工作时间:9:00-18:30)。
RELATIONSHIP BETWEEN CONGESTION AND TRAFFIC ACCIDENTS ON EXPRESSWAYS AN INVESTIGATION WITH BAYESIAN BELIEF NETWORKSBy Charitha Dias**, Marc Miska***, Masao Kuwahara****, and Hiroshi Warita*****1. IntroductionAccidents and congestion are two frustrating events, which can be observed very frequently on roads. Accidents, especially on expressways, can trigger heavy traffic congestions imposing huge external costs and reducing the level of service. Therefore it is obvious that accidents clearly have an impact on congestion. But the opposite, i.e. the effect of congestion on occurrence of accidents, is less studied and still questionable 11). One can argue that congestion can reduce the high speeds on expressways and as a result of that the accident rate is reduced. But in a congested road section vehicles are closely packed and as a result of that rear-end collisions, back-up collisions as well as side collisions can occur. Therefore it is important to analyze the impact on the accidents by congestion so that the policy makers can implement relevant measures to reduce the external costs of both accidents and congestion.This paper investigates the effects of traffic congestion on the occurrence of accidents on 8 radial routes (inbound direction) of Metropolitan Expressway (MEX). Data were obtained from the International Traffic Database (ITDb) 6). Two softwares, namely WinMine Toolkit 2) and MSBNx 5), which use the concept of Bayesian Belief Networks (BBN), were used to model the interrelationships among occurrence of accidents and other variables such as congestion index (CI), traffic density and volume.2. Relationship between congestion and accidentsVery limited attempts have been made, in the past by several authors, to describe the relationship between accidents and congestion. Among those, Wang et al.11) claimed that traffic congestion, controlling other factors such as flow, curvature, gradient, section length, no. of lanes etc., has little or no impact on frequency of accidents (fatal or non-fatal), using data for M25 highway. But the CI values in their data were relatively low, i.e. less than 0.5, for most of the cases. Therefore, it is questionable that those data really represented congested situations.Noland and Quddus8) used a series of negative binomial models to analyze the effect of congestion on road safety. Their results were not conclusive, suggesting that there is little effect of congestion on road safety. They suspected that this might be due to the weakness of proxies they used to represent congestion, plus might be due to the method they implemented to model relationships. While above mentioned studies claimed that there is no any significant relationship between accidents and congestion, Golob and Recker 4), using nonlinear multivariate statistical analysis, concluded that rear-end collisions are more likely to occur under heavily congested stop-and-go traffic. Though this is an indication that congestion has an effect on accidents, the* Keywords: traffic accidents, congestion, Bayesian belief networks** Non-member of JSCE, M. Sc., Chodai Co., Ltd.(2-1-3 Higashi-Tabata, Kita-Ku, Tokyo, Japan 114-0013, Tel: +81-3-3894-3236, Fax: +81-3-3894-3265) *** Member of JSCE, Lecturer, Institute of Industrial Science, University of Tokyo(4-6-1 Komaba, Meguro-ku, Tokyo, Japan 153-8505, Tel: +81-3-5452-6419, Fax: +81-3-5452-6420)**** Member of JSCE, Professor, Institute of Industrial Science, University of Tokyo(4-6-1 Komaba, Meguro-ku, Tokyo, Japan 153-8505, Tel: +81-3-5452-6419, Fax: +81-3-5452-6420)***** Member of JSCE, Planning and Environment Department, Metropolitan Expressway Co., Ltd.(1-4-1 Kasumigaseki, Chiyoda-ku, Tokyo, Japan 100-8930, Tel: +81-3-3539-9389, Fax: +81-3-3502-2412)link between accidents and congestion has not elaborated clearly.Though some other authors have attempted to explore the relationship between accidents and traffic volume or flow 1)3), these studies may not describe the relationship between accidents and congestion, because according to speed-flow characteristics not only flow but also speeds are also needed to determine whether the prevailing traffic condition is congested or un-congested.These limited previous studies suggest us the need for more studies to better understand the impact of congestion on accidents. In this study BBNs, which can model and graphically interpret the relationships in any system, were used to model the interrelationships among CI, volume, density and occurrence of accidents.3. Methodology(1) Data descriptionFive-minutes aggregated volume, average speed, occupancy and incident data on inbound direction of 8 radial routes (Route numbers 1, 2, 3, 4, 5, 6, 7 and 9) of the MEX, collected over one week were used. One-week data (from 5th June 2006 to 11th June 2006) of ITDb on MEX is free for public and that free data was used for this analysis. Format of raw data is shown in Figure 2.(2) Data arrangementa) Pre-accident period.First the other incidents such as car breakdowns, road works etc. were removed and the 15 minutes time period before an accident was defined as pre-accident period (Figure 2). Here, 15 minutes was selected just to represent the average traffic conditions on the road section before an accident. b) Congestion Index (CI)Congestion index expresses the congestion level of a given road section relative to the free-flow conditions. It is non-negative and dimensionless value. Average speed data was converted in to congestion data, i.e. congestion indexes (CI), with slightly modified version of Taylor’s 9) method, as follows.{tionroad the for speed flow Free tionroad the for speed Average Where ifand if CI VV VV V VV V V V FFFFFF FFFFFF sec sec ;;0;/)(−−>>≤−=Figure 1: Metropolitan expressway (MEX)networkc) V olume classes, CI classes and density classes V olumes, CI’s and occupancies were categorized in to classes as shown in Table 1. This was done because categorized data were needed to learn BBNs.Table 1: Categorized volumes, CIs and densitiesFigure 2: Available data(3) Bayesian belief Networks (BBN)BBNs are probabilistic graphical models that represent a set of variables and their conditional independencies via a directed acyclic graph 12). BBN is an effective technique to understand the relationships among variables because BBNs can model the interrelationships among variables with their conditional probabilities in any kind of a system and represent them graphically.Two applications namely WinMine Toolkit2) and MSBNx5), which use the BBN concept, were used to identify conditional independencies among variables and to perform inference, respectively. WinMine toolkit is set of tools, designed for Windows environment, that allow constructing statistical models from categorized data. WinMine has many advantages compared with other traditional methods such as bar graphs, contingency tables and odd ratios to mine for information in data because it can provide a better picture of the factor interrelationships among variables 7). MSBNx is a component-based application, which can be used to create, assess and evaluate Bayesian networks 5). It is an excellent tool for inference or updating probabilities. Categorized volume, CI, density and event for each route were fed in to WinMine toolkit separately in order to obtain the dependency network, which is very similar to Bayesian network. For inference purposes the conditional probabilities, determined with WinMine, were fed in to MSBNx. Figure 3 depicts dependency network along with the conditional probability table (CPT) for the node “Event”, constructed with WinMine, and Figure 4 depicts the BBN with un-conditional probability tables for all nodes, constructed with MSBNx, for the inbound direction of Route 4 of MEX.At a glance some important information can be drawn from these figures. Figure 3 describes the dependencies among the variables in data fed in to WinMine. Figure 4 shows how the inbound direction of Route 8 functions. 15% of the time, within the considered one week time period, it is congested or heavily congested, 76% of the time medium volumes and 81% of the times low densities could be observed. And there is around 1% of chance of an accident.Figure 3: Dependency network constructed with WinMineFigure 4: BBN constructed with MSBNxUn-conditional probabilities, which are shown in Figure 4, are probabilities before any evidence is observed and those are called prior probabilities. When evidence is observed, for example when it is known that the CI reflects heavily congested situation, probabilities for all other nodes can be updated and those updated probabilities are called posterior probabilities. And this process of updating probabilities is called inference. Inference was performed for BBNs for each considered routes with MSBNx and updated probabilities were noted. Results are described in Section 4.4. ResultsProbabilities of occurring a pre-accident period were plotted against congestion levels, as in Figure 5, and probability of occurring a pre-accident period was plotted against density levels, as in Figure 6. Figure 5clearly shows that the chance of an accident is increasedwith the congestion level.High density reflects high congestion levels. When occurrence of accidents is increased with the increase of density levels, as shown in Figure 6, it reflects that probability of accident occurrence is increased with congestion levels. Therefore both Figure 5 and Figure 6 describe that the accident occurrence is increased with congestion levels.Table 2 compares the congestion levels and density levels when a pre-accident condition is evidenced and non-pre-accident condition is evidenced.When a pre-accident situation is evidenced, the probability that the road section is in congested conditions is in the range of [60%, 87%]. And compared to that, when none (a non-pre-accident condition or any other incident) is observed the probability that the road section is congested is pretty low, i.e. in the range of [8%, 20%]. This reflects that when an accident occurred most of the time road section was congested.These results probably describe the drivers’ behavior at congested situations. On congested roads, where stop-and-go conditions are prevailed, frustrated drivers try to accelerate resulting rear-end collisions. And some drivers may try to change their lanes resulting side collisions plus back-up collisions. However, it should be kept in mind that fatalities are less in congested road sections compare to un-congested sections where operating speeds are high 10).Figure 5: Probability of observing a pre-accidentcondition given the congestion level5. Summary(1) ConclusionsFigure 6: Probability of observing a pre-accidentcondition given the density levelFlow, speed, occupancy and event data on inbound direction of 8 radial routes of MEX, collected over one week period, were used to explore the impact of congestion on occurrence of accidents. BBNs were used to model the relationships among pre-accident conditions and other variables. It was found that when a road section is getting congested, i.e. when the CI is increased and density is increased, the chance for an accident could be increased. Analysis of accident types will more clearly provide the answer for why probability of accident occurrence increases with congestion level.(2) Further researchThese results may not totally describe the mechanism of occurrence of accidents and congestion on MEX. Broad analysis is needed, which considers all factors that may cause accidents and congestion such as weather, road geometry, driver characteristics etc., to get a betterTable 2: Conditional probabilities of CI and density ifpre-accident condition is observedpicture of pre-accident conditions.Although the probability of occurring a pre-accident situation is increased with the increase of congestion, accidents occur under congested situations are not fatal compared to accidents occur under high-speed situations. Here in this analysis, severity of accidents and types of accidents were not considered due to lack of data. Therefore, it is interesting to extend the analysis to consider the severity levels as well as types of accidents, too.6. AcknowledgementAll traffic measurement data for this study was provided by the International Traffic Database (ITDb). Data available to the public can be accessed through .References1) Ceder, A.: Relationships between road accidents and hourly traffic flow. II. Probabilistic approach, Accident Analysis and Prevention, 14 (1), pp.35–44, 1982.2) Chickering, D.: The WinMine Toolkit. Redmond, WA., Microsoft technical report no. MSR-TR- 2002-103, 2002.3) Dickerson, A., Peirson, J., Vickerman, R.: Road accidents and traffic flows: An econometric investigation, Economica, London School of Economics and Political Science, V ol. 67(265), pp. 101-21, 2000.4) Golob, T. F. and Recker, W. W.: Relationships Among Urban Freeway Accidents, Traffic Flow, Weather and Lighting Conditions, Institute of Transportation Studies, University of California, Berkeley, 2001.5) Kadie, C. M., Hovel, D., and Horvitz, H.: MSBNx: A Component-Centric Toolkit for Modeling and Inference with Bayesian Networks, Microsoft Research Technical Report MSR-TR-2001-67, 2001.6) Miska, M., et al.: The International Traffic Database Project, 14th World congress on intelligent transport systems, CD-ROM, 2007.7) Milburn, N. J. et al: Mining for information in accident data, Final report, Federal aviation administration, Civil Aerospace medical institute, Oklahoma city, 2006.8) Noland, R.B., Quddus, M.A.: Congestion and safety: a spatial analysis of London, Transportation Research Part A: Policy and Practice 39 (7-9), pp. 737-754, 2005.9) Taylor, M.A.P., Woolley, J.E., Zito, R.: Integration of the global positioning system and geographical information systems for traffic congestion studies. Transportation Research Part C: Emerging Technologies 8 (1-6), pp.257-285. 2000.10) Taylor, M. C.: The speeds of vehicles which are involved in fatal accidents, Traffic Engineering and Control 42(2), 2001.11) Wang, C., Quddus, M. A., Ison, S. G.: Impact of traffic congestion on road accidents: A spatial analysis of theM25motorway in England, Accident Analysis and Prevention, 2009.12) Wikipedia contributors: Bayesian network, Wikipedia, The Free Encyclopedia, /w/index.php?title=Bayesian_network&oldid=293220919 (accessed May 30, 2009).。