车牌识别某英文翻译
OCR简介演示
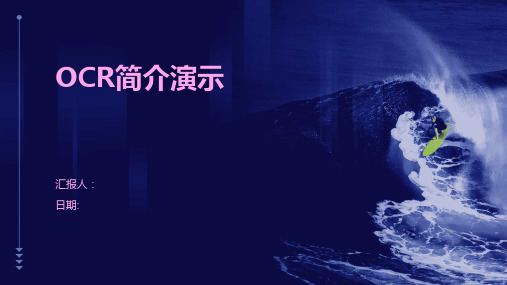
性能分析方法
对比分析
通过与其他OCR系统或算法进行对比,可以直观地评估出本系统 在性能上的优劣。
误差分析
通过对识别结果进行误差分析,找出导致性能下降的关键因素,为 后续的性能优化提供依据。
瓶颈分析
通过对OCR系统各个模块的性能进行瓶颈分析,找出制约整体性能 的短板,从而有针对性地进行优化。
性能优化建议
算机可编辑和检索的格式的技术
。
应用广泛
这种技术主要应用于扫描和识别 印刷或手写文档,使得这些文档 能够被计算机处理和分析。
OCR发展历程
初期阶段
OCR技术最初在20世纪60年代 开始发展,当时的技术基于传统 的图像处理方法和模式识别算法
。
技术演进
随着深度学习技术的快速发展, 现代的OCR系统大多基于深度学 习模型,如卷积神经网络(CNN )和循环神经网络(RNN)。
特征提取
提取字符的特征,如线条 、拐角和闭合区域等,用 于后续的分类和识别。
分类识别
利用机器学习或深度学习 算法,将提取的特征与已 知的字符库进行匹配,实 现字符的识别。
后处理
校验和修正
对识别结果进行校验,对 于识别错误的字符进行修 正,提高整体识别率。
格式转换
将识别的结果转换为所需 的格式,如TXT、DOC或 PDF等,以满足用户的不 同需求。
总结与展望 OCR技术总结
跨语言识别
随着全球化进程的加速,OCR技术将更加注重跨语言识别,支持更多语种和字符 集,促进国际间的信息交流与合作。
与其他技术融合
OCR技术将与自然语言处理、计算机视觉等技术进一步融合,实现更加智能化的 文本识别和理解,推动人工智能技术的整体进步。
THANKS
“车牌号”英文怎么说?别告诉我是carnumber!
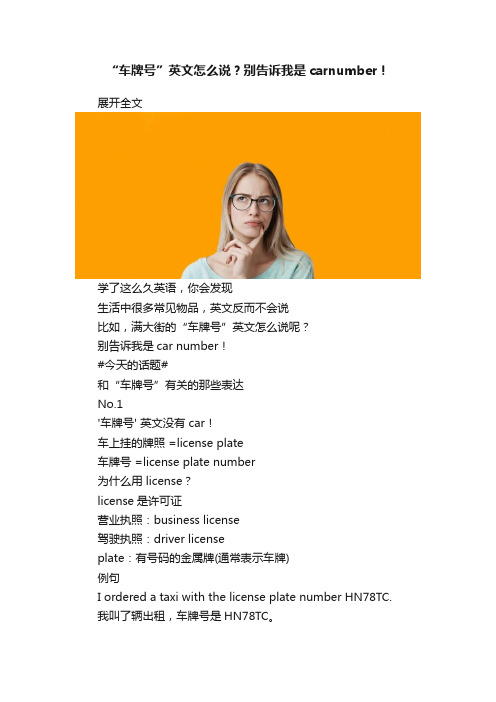
“车牌号”英文怎么说?别告诉我是carnumber!展开全文学了这么久英语,你会发现生活中很多常见物品,英文反而不会说比如,满大街的“车牌号”英文怎么说呢?别告诉我是car number!#今天的话题#和“车牌号”有关的那些表达No.1'车牌号' 英文没有 car!车上挂的牌照 =license plate车牌号 =license plate number为什么用 license?license是许可证营业执照:business license驾驶执照:driver licenseplate:有号码的金属牌(通常表示车牌)例句I ordered a taxi with the license plate number HN78TC. 我叫了辆出租,车牌号是HN78TC。
那 car number 是什么?car number = 车厢编号地铁和火车车厢都有Car No.No.2'摇号' 的 '摇'不是 shake!摇号说成shake number(✘)车牌摇号 = license plate lottery车牌随机抽取lottery = 随机事件例句He failed to get plates through the license plate number lottery.车牌摇号他没摇中。
No.3'限号' 的 '限'也别用 limit!车牌被限号说成My license plate number is limited(✘)我的车牌数字是有限的(✘)“限号”的“限”用这个词restrict:限制行动例句I can't use my car becausemy license plate number is restricted today.我的车今天被限号,不能用。
国际上很多人口密度大的城市都在执行单双号制度但是单号≠single,双号≠double!双数 = 偶数 = even number单数 = 奇数 = odd number单双号限行政策:the even-odd license plate policy尾号 = end-number尾号限行政策:the end-number policy 尾号限行例句Does your city follow the even-odd license plate policy or the end-number policy?你的城市是单双号限行还是尾号限行?On which day is your license plate number restricted?你的车哪天限号?这些措施,统称为Road space rationing道路空间分配俗称交通管制。
车牌识别外文文献翻译中英文

外文文献翻译(含:英文原文及中文译文)文献出处:Gao Q, Wang X, Xie G. License Plate Recognition Based On Prior Knowledge[C]// IEEE International Conference on Automation and Logistics. IEEE, 2007:2964-2968.英文原文License Plate Recognition Based On Prior KnowledgeQian Gao, Xinnian Wang and Gongfu XieAbstract - In this paper, a new algorithm based on improved BP (back propagation) neural network for Chinese vehicle license plate recognition (LPR) is described. The proposed approach provides a solution for the vehicle license plates (VLP) which were degraded severely. What it remarkably differs from the traditional methods is the application of prior knowledge of license plate to the procedure of location, segmentation and recognition. Color collocation is used to locate the license plate in the image. Dimensions of each character are constant, which is used to segment the character of VLPs. The Layout of the Chinese VLP is an important feature, which is used to construct a classifier for recognizing. The experimental results show that the improved algorithm is effective under the condition that the license plates were degraded severely.Index Terms - License plate recognition, prior knowledge, vehiclelicense plates, neural network.I. INTRODUCTIONV ehicle License-Plate (VLP) recognition is a very interesting but difficult problem. It is important in a number of applications such as weight-and-speed-limit, red traffic infringement, road surveys and park security [1]. VLP recognition system consists of the plate location, the characters segmentation, and the characters recognition. These tasks become more sophisticated when dealing with plate images taken in various inclined angles or under various lighting, weather condition and cleanliness of the plate. Because this problem is usually used in real-time systems, it requires not only accuracy but also fast processing. Most existing VLP recognition methods [2], [3], [4], [5] reduce the complexity and increase the recognition rate by using some specific features of local VLPs and establishing some constrains on the position, distance from the camera to vehicles, and the inclined angles. In addition, neural network was used to increase the recognition rate [6], [7] but the traditional recognition methods seldom consider the prior knowledge of the local VLPs. In this paper, we proposed a new improved learning method of BP algorithm based on specific features of Chinese VLPs. The proposed algorithm overcomes the low speed convergence of BP neural network [8] and remarkable increases the recognition rate especially under the condition that the license plate images were degrade severely.II. SPECIFIC FEA TURES OF CHINESE VLPSA. DimensionsAccording to the guideline for vehicle inspection [9], all license plates must be rectangular and have the dimensions and have all 7 characters written in a single line. Under practical environments, the distance from the camera to vehicles and the inclined angles are constant, so all characters of the license plate have a fixed width, and the distance between the medium axes of two adjoining characters is fixed and the ratio between width and height is nearly constant. Those features can be used to locate the plate and segment the individual character. B. Color collocation of the plateThere are four kinds of color collocation for the Chinese vehicle license plate .These color collocations are shown in table I.TABLE IMoreover, military vehicle and police wagon plates contain a red character which belongs to a specific character set. This feature can be used to improve the recognition rate.C. Layout of the Chinese VLPSThe criterion of the vehicle license plate defines the characters layout of Chinese license plate. All standard license plates contain Chinese characters, numbers and letters which are shown in Fig.1. The first one is a Chinese character which is an abbreviation of Chineseprovinces. The second one is a letter ranging from A to Z except the letter I. The third and fourth ones are letters or numbers. The fifth to seventh ones are numbers ranging from 0 to 9 only. However the first or the seventh ones may be red characters in special plates (as shown in Fig.1). After segmentation process the individual character is extracted. Taking advantage of the layout and color collocation prior knowledge, the individual character will enter one of the classes: abbreviations of Chinese provinces set, letters set, letters or numbers set, number set, special characters set.(a)Typical layout(b) Special characterFig.1 The layout of the Chinese license plateIII. THE PROPOSED ALGORITHMThis algorithm consists of four modules: VLP location, character segmentation, character classification and character recognition. The main steps of the flowchart of LPR system are shown in Fig. 2.Firstly the license plate is located in an input image and characters are segmented. Then every individual character image enters the classifier to decide which class it belongs to, and finally the BP network decides which character the character image represents.A. Preprocessing the license plate1) VLP LocationThis process sufficiently utilizes the color feature such as color collocation, color centers and distribution in the plate region, which are described in section II. These color features can be used to eliminate the disturbance o f the fake plate ’ s regions. The flowchart of the plate location is shown in Fig. 3.Fig.3 The flowchart of the plate location algorithmThe regions which structure and texture similar to the vehicle plate are extracted. The process is described as followed:Here, the Gaussian variance is set to be less than W/3 (W is the character stroke width), so 1P gets its maximum value M at the center of the stroke. After convolution, binarization is performed according to a threshold which equals T * M (T<0.5). Median filter is used to preserve the edge gradient and eliminate isolated noise of the binary image. An N * N rectangle median filter is set, and N represents the odd integer mostly close to W.Morphology closing operation can be used to extract the candidate region. The confidence degree of candidate region for being a license plate is verified according to the aspect ratio and areas. Here, the aspect ratio is set between 1.5 and 4 for the reason of inclination. The prior knowledge of color collocation is used to locate plate region exactly. The locating process of the license plate is shown in Fig. 4.2) Character segmentationThis part presents an algorithm for character segmentation based on prior knowledge, using character width, fixed number of characters, the ratio of height to width of a character, and so on. The flowchart of the character segmentation is shown in Fig. 5.Firstly, preprocess the license the plate image, such as uneven illumination correction, contrast enhancement, incline correction and edge enhancement operations; secondly, eliminating space mark which appears between the second character and the third character; thirdly, merging the segmented fragments of the characters. In China, all standard license plates contain only 7 characters (see Fig. 1). If the number of segmented characters is larger than seven, the merging process must be performed. Table II shows the merging process. Finally, extracting the individual character’ image based on the number and the width of the character. Fig. 6 shows the segmentation results. (a) The incline and broken plate image, (b) the incline and distort plate image, (c)the serious fade plate image, (d) the smut license plate image.where Nf is the number of character segments, MaxF is the number of the license plate, and i is the index of each character segment.The medium point of each segmented character is determined by:(3)where 1i Sis the initial coordinates for the character segment, and 2i S is thefinal coordinate for the character segment. The d istance between two consecutive medium points is calculated by:(4)Fig.6 The segmentation resultsB. Using specific prior knowledge for recognitionThe layout of the Chinese VLP is an important feature (as described in the section II), which can be used to construct a classifier for recognizing. The recognizing procedure adopted conjugate gradient descent fast learning method, which is an improved learning method of BP neural network[10]. Conjugate gradient descent, which employs a series of line searches in weight or parameter space. One picks the first descent direction and moves along that direction until the minimum in error is reached. The second descent direction is then computed: this direction the “ conjugate direction” is the one along which the gr adient does not change its direction will not “ spoil ” the contribution from the previous descent iterations. This algorithm adopted topology 625-35-N as shown in Fig. 7. The size of input value is 625 (25*25 ) and initial weights are with random values, desired output values have the same feature with the input values.As Fig. 7 shows, there is a three-layer network which contains working signal feed forward operation and reverse propagation of error processes. The target parameter is t and the length of network outputvectors is n. Sigmoid is the nonlinear transfer function, weights are initialized with random values, and changed in a direction that will reduce the errors.The algorithm was trained with 1000 images of different background and illumination most of which were degrade severely. After preprocessing process, the individual characters are stored. All characters used for training and testing have the same size (25*25 ).The integrated process for license plate recognition consists of the following steps:1) Feature extractingThe feature vectors from separated character images have direct effects on the recognition rate. Many methods can be used to extract feature of the image samples, e.g. statistics of data at vertical direction, edge and shape, framework and all pixels values. Based on extensive experiments, all pixels values method is used to construct feature vectors. Each character was reshaped into a column of 625 rows’ feature vector. These feature vectors are divided into two categories which can be used for training process and testing process.2) Training modelThe layout of the Chinese VLP is an important feature, which can be used to construct a classifier for training, so five categories are divided. The training process of numbers is shown in Fig. 8.As Fig. 8 shows, firstly the classifier decides the class of the inputfeature vector, and then the feature vector enters the neural network correspondingly. After the training process the optimum parameters of the net are stored for recognition. The training and testing process is summarized in Fig. 9.(a) Training process(b)Testing processFig.9 The recognition process3) Recognizing modelAfter training process there are five nets which were completely trained and the optimum parameters were stored. The untrained feature vectors are used to test the net, the performance of the recognition system is shown in Table III. The license plate recognition system is characterized by the recognition rate which is defined by equation (5).Recognition rate =(number of correctly read characters)/ (number of found characters) (5)IV. COMPARISON OF THE RECOGNITION RA TE WITH OTHER METHODSIn order to evaluate the proposed algorithm, two groups of experiments were conducted. One group is to compare the proposed method with the BP based recognition method [11]. The result is shown in table IV. The other group is to compare the proposed method with themethod based on SVM [12].The result is shown in table V. The same training and test data set are used. The comparison results show that the proposed method performs better than the BP neural network and SVM counterpart.V. CONCLUSIONIn this paper, we adopt a new improved learning method of BP algorithm based on specific features of Chinese VLPs. Color collocation and dimension are used in the preprocessing procedure, which makes location and segmentation more accurate. The Layout of the Chinese VLP is an important feature, which is used to construct a classifier for recognizing and makes the system performs well on scratch and inclined plate images. Experimental results show that the proposed method reduces the error rate and consumes less time. However, it still has a few errors when dealing with specially bad quality plates and characters similar to others. This often takes place among these characters (especially letter and number): 3—8 4—A 8—B D—0.In order to improve the incorrect recognizing problem we try to add template-based model [13] at the end of the neural network.中文译文基于先验知识的车牌识别Qian Gao, Xinnian Wang and Gongfu Xie摘要- 本文介绍了一种基于改进的BP(反向传播)神经网络的中国车牌识别(LPR)算法。
6.2 人工智能的应用 同步习题(含解析)
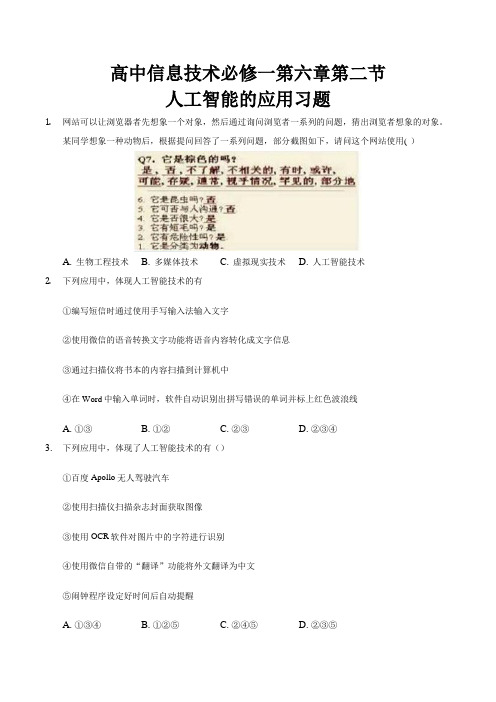
高中信息技术必修一第六章第二节人工智能的应用习题1.网站可以让浏览器者先想象一个对象,然后通过询问浏览者一系列的问题,猜出浏览者想象的对象。
某同学想象一种动物后,根据提问回答了一系列问题,部分截图如下,请问这个网站使用( )A. 生物工程技术B. 多媒体技术C. 虚拟现实技术D. 人工智能技术2.下列应用中,体现人工智能技术的有①编写短信时通过使用手写输入法输入文字②使用微信的语音转换文字功能将语音内容转化成文字信息③通过扫描仪将书本的内容扫描到计算机中④在Word中输入单词时,软件自动识别出拼写错误的单词并标上红色波浪线A. ①③B. ①②C. ②③D. ②③④3.下列应用中,体现了人工智能技术的有()①百度Apollo无人驾驶汽车②使用扫描仪扫描杂志封面获取图像③使用OCR软件对图片中的字符进行识别④使用微信自带的“翻译”功能将外文翻译为中文⑤闹钟程序设定好时间后自动提醒A. ①③④B. ①②⑤C. ②④⑤D. ②③⑤4.下列应用中,体现人工智能技术的是①声卡将模拟信号转换成数字信号②机器人导游回答游客的问题,并提供帮助③扫描仪扫描书籍内容并保存为bmp文件④使用有道词典将文章中的中文翻译成英文⑤某商店通过人脸识别技术支付费用A. ②④⑤B. ①③⑤C. ①④⑤D. ②③④5.下面描述中,属于人工智能应用案例的是①通过语音应答交互系统和移动应用程序对人类语言进行转录的系统②利用统计和机器学习方法理解句子的结构、含义、情绪和意图的系统③帮助我们发送短信,拨打电话,记录备忘,甚至还可以陪用户聊天的个人助理④模拟人类大脑,处理数据并创建决策模式的平台A. ①②③④B. ①②④C. ①②③④D. ②③④6.以下不属于人工智能的应用的有A. 虚拟主播通过语音合成、唇形合成以及深度学习等技术实现像真人主播一样的新闻播报;B. 5G网络+8K屏幕实时回传高清直播,画面清晰可见C. 无人车也即将真正实现,无人驾驶采用纯电动动力系统,行驶系统和技术也大有特色D. 宾客“刷脸”入住,观众“刷脸”观展7.下列描述中不属于人工智能应用范畴的是()A. 使用人脸识别系统登记考勤B. 某现代化农场,能自动的定时为作物浇水C. 高速公路ETC(电子不停车收费系统)通道自动识别车牌收费D. 使用指纹解锁功能打开手机8.下列应用中,体现人工智能技术的是( )①用VR技术进行远程医疗②某手机利用刷脸进行支付认证③用手机进行语音聊天④用智能手机编辑微信内容,并分享到朋友圈⑤利用“百度翻译”将一段中文翻译成英文A. ②⑤B. ①④C. ②③D. ①③9.下列不属于...人工智能学科中模式识别的应用的是A. OCR字符识别B. 声纹识别C. 指纹锁D. 与在线机器人对话10.下列描述中不属于人工智能应用范畴的是()①通过手机操控“无人机”高空拍摄视频②医生在B超可视环境下给病人进行腹腔手术③通过在线翻译网站将中文翻译为英文④Word软件中的“自动更正”功能⑤通过指纹解锁功能打开手机⑥在手机上通过手写的方式输入文字A. ①②④B. ③⑤⑥C. ①②⑥D. ③④⑤11.在使用手机“微信”过程中,下列操作体现了人工智能技术的是()①使用微信扫一扫功能中的“翻译”,对准英文版产品说明书,翻译成中文;②使用手机拍照功能获取图象;③选中语音信息,进行播放;④使用语音输入法纠正错误的识别结果;⑤使用微信扫一扫功能中的“街景”,显示所在位置的景物;A. ①③B. ②④C. ③⑤D. ①④12.下列说法中都体现人工智能技术应用的是()①使用人体测温筛检仪,实现人脸识别无感通关,迅速发现体温异常者②楼道LED声控感应灯,在光线不足且有人走动时,自动感应亮灯③使用控制软件在家里对公司里的电脑进行远程控制,完成文件下载等操作④车辆进出停车场时,通过车牌识别技术自动完成车辆停车计费工作A. ①②B. ②③C. ①④D. ②④13.下列应用中采用了人工智能技术的是()A. 手机采用人脸识别技术解锁B. 网络课堂直播时老师与学生进行实时语音交流C. 采用5G+8K实时直播2019年乌镇互联网大会过程D. 用Word软件“拒绝修订”功能,自动恢复修订内容14.下列应用中,体现人工智能技术的有( )手机根据环境光自动调节屏幕亮度高速公路ETC收费系统自动识别车牌号用语音控制音响播放音乐以手写方式输入短信内容在Word中输入一段英文,软件能自动更正拼写错误A. B. C. D.15.下列()不属于人工智能技术应用的产物。
5.2人工智能的应用 第2课时(分层作业)

5.2人工智能的应用第2课时(分层作业)【夯实基础】1.下列应用中不能体现人工智能技术的是()A.电子支付系统通过扫描二维码收付款B.机器翻译实现不同语言之间的翻译C.手写输入系统将手写内容识别为文本D.语音输入系统辨识不同用户的发音2.“文心一言”是一款能够与人对话互动、回答问题、协助创作,高效便捷地帮助人们获取信息、知识和灵感的智能文本生成产品。
其背后的核心技术是人工智能引擎,这款引擎采用了深度学习算法,能够自动分析和理解用户输入的信息,并根据语境生成符合逻辑、通顺流畅的文本,并在此基础上采用监督精调、人类反馈强化学习、提示等技术,具备知识增强、检索增强和对话增强的技术优势。
结合材料,下列关于人工智能说法正确的是()A.该智能搜索引擎采用的深度学习算法,需要通过对大量的数据进行训练B.随着人工智能的不断发展完善,人类不再需要学习C.人工智能可以完成一切人类能完成的任务D.“文心一言”在使用过程中根据用户的反馈不断强化学习,属于联结主义3.亚运会期间,杭州市人民政府对交通施行管理限制,受限的车辆可在“浙里办”、“支付宝”等APP的“非浙A急事通”应用中申领通行证。
小林通过支付宝平台链接实名人脸认证后进入应用,根据界面提示,依次填写车牌号、号牌种类、申请人等内容办理“非浙A急事通”业务。
下列应用中,体现人工智能技术的是()A.提交数据到“非浙A急事通”服务器B.通过人脸识别认证后方可使用“非浙A急事通”C.系统自动生成用户的申请反馈数据D.通过历史记录快速填写申请表单信息4.智能语音技术在当代已经全面普及人类的生活,下面活动中应用了智能语音技术的是()A.通过“小爱同学”唤醒小米电视机,并通过“开机”控制它打开电视B.当天黑了家里的窗帘自动拉合C.智慧家庭应用中,下雨天窗户自动关闭D.清晨到起床时间,小度智能音箱开始播放音乐提醒主人起床5.下列选项中,不属于人工智能技术应用的是()A.使用微信视频聊天 B.使用语音方式输入文本C.使用“谷歌翻译”将一段中文翻译为英语D.某单位员工上下班使用指纹签到6.为了使城市道路更为通畅,一些城市开始采用AI来控制红绿灯。
车牌用英语怎么说
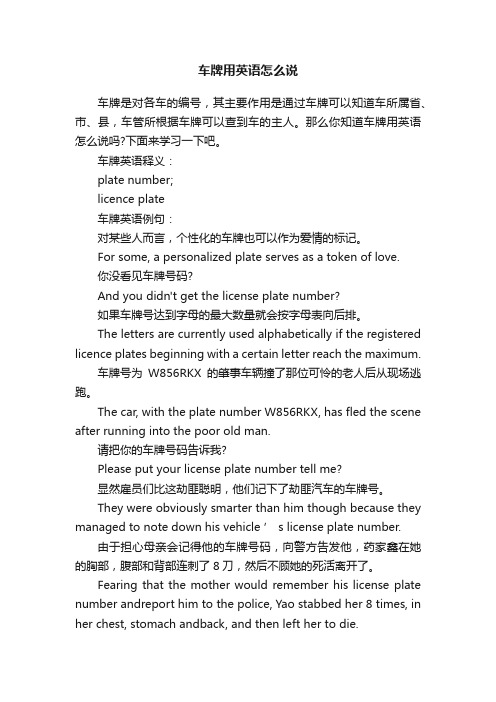
车牌用英语怎么说车牌是对各车的编号,其主要作用是通过车牌可以知道车所属省、市、县,车管所根据车牌可以查到车的主人。
那么你知道车牌用英语怎么说吗?下面来学习一下吧。
车牌英语释义:plate number;licence plate车牌英语例句:对某些人而言,个性化的车牌也可以作为爱情的标记。
For some, a personalized plate serves as a token of love.你没看见车牌号码?And you didn't get the license plate number?如果车牌号达到字母的最大数量就会按字母表向后排。
The letters are currently used alphabetically if the registered licence plates beginning with a certain letter reach the maximum.车牌号为W856RKX的肇事车辆撞了那位可怜的老人后从现场逃跑。
The car, with the plate number W856RKX, has fled the scene after running into the poor old man.请把你的车牌号码告诉我?Please put your license plate number tell me?显然雇员们比这劫匪聪明,他们记下了劫匪汽车的车牌号。
They were obviously smarter than him though because they managed to note down his vehicle ’ s license plate number.由于担心母亲会记得他的车牌号码,向警方告发他,药家鑫在她的胸部,腹部和背部连刺了8刀,然后不顾她的死活离开了。
Fearing that the mother would remember his license plate number andreport him to the police, Yao stabbed her 8 times, in her chest, stomach andback, and then left her to die.在学习并掌握了数字图像处理和模式识别的一些基本原理后,使用VC++6.0软件利用以上原理针对车牌识别任务进行编程。
(完整版)MATLAB车牌识别
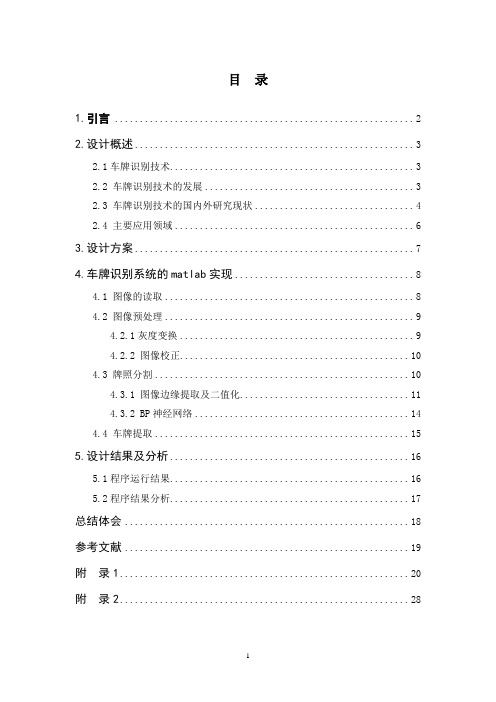
目录1.引言 (2)2.设计概述 (3)2.1车牌识别技术 (3)2.2 车牌识别技术的发展 (3)2.3 车牌识别技术的国内外研究现状 (4)2.4 主要应用领域 (6)3.设计方案 (7)4.车牌识别系统的matlab实现 (8)4.1 图像的读取 (8)4.2 图像预处理 (9)4.2.1灰度变换 (9)4.2.2 图像校正 (10)4.3 牌照分割 (10)4.3.1 图像边缘提取及二值化 (11)4.3.2 BP神经网络 (14)4.4 车牌提取 (15)5.设计结果及分析 (16)5.1程序运行结果 (16)5.2程序结果分析 (17)总结体会 (18)参考文献 (19)附录1 (20)附录2 (28)1.引言伴随着世界各国车辆数量的增加,城市交通状况日益受到人们的重视。
如何有效地进行交通管理,越来越成为各国政府的相关部门所关注的焦点。
针对这一问题,人们运行先进的信息处理技术、导航定位技术、无线通信技术、自动控制技术、图像处理和识别技术及计算机网络技术等科学技术,相继研发了各种交通道路监视管理系统、车辆控制系统及公共交通系统。
这些系统将车辆和道路综合起来进行考虑,运行各种先进的技术解决道路交通的问题,统称为智能交通系统( Intelligent Transportation System,简称ITS)。
ITS 是20世纪90年代兴起的新一代交通运输系统。
它可以加强道路、车辆、驾驶员和管理人员的联系,实现道路交通管理自动化和车辆行驶的智能化,增强交通安全,减少交通堵塞,提高运输效率,减少环境污染,节约能源,提高经济活力。
智能交通系统以车辆的自动检测作为信息的来源,因而对车牌照等相关信息的自动采集和处理的一门新的交通信息获取技术——车牌识别(License Plate Recognition ,LPR) 技术逐渐发展起来,成为信息处理技术的一项重要研究课题。
车牌自动识别是智能交通管理系统中的关键技术之一。
【精】数字的表达(学习资料)

与数字有关一些表达的读法英语中有很多内容涉及到数字的内容,如:时间、年龄、价格、距离、号码、尺寸等。
许多学生抱怨听不懂数字、说不出数字,这是因为对英文数字的组成、排列及读法不太熟悉而造成的。
当碰到一个数字时,不能作出迅速准确的反映,而往往要先在心里进行翻译、默读,这就大大影响了听说英文的能力。
本文将介绍一些数字在不同场合的表达方式、发音特点及相应对策。
一、熟读牢记关键数字迅速无误识别数字的前提是必须能够流利地读出数字。
要从读两位数起,然后练习读三位和四位数乃至五位或六位以上的数字。
其中两位和三位数的读法是读所有数字的基础。
英文数字中的每一个逗点的读法也要牢记:有一个逗点读“thousand”,两个逗点读“million”,三个逗点读“billion”。
还要清楚,每个逗点间由三位数组成。
英文数字中的第四位数、第七位数、第十位数是很关键的数位。
Examples:1,234 读作:one thousand,two hundred and thirty-four4,567,809 读作:four million,five hundred and sixty-seventhousand,eight hundred and nine 5,678,120,000 读作:five billion,six hundred and seventy-eight million,one hundred and twe nty thousand二、“-teen和“-ty”的区别“-teen”和“-ty”是比较容易混淆的一对读音。
含有“- teen ”的词有两个重音,即“-teen”要重读,且“-teen”中的元音为长元音[ti:n],发音长而清晰;而含有“-ty”的词只有一个重音,即“-ty”不重读,且“-ty ”中的元音为短元音[ti],发音短而急促。
Examples:fifteen['fif'ti:n] fifty['fifti]nineteen['nai'ti:n] ninety['naiti]三、英美数字读法的差异有时,同样一个数字,英国人和美国人的读法也不尽相同,这无疑会使本来就棘手的数字雪上加霜。
- 1、下载文档前请自行甄别文档内容的完整性,平台不提供额外的编辑、内容补充、找答案等附加服务。
- 2、"仅部分预览"的文档,不可在线预览部分如存在完整性等问题,可反馈申请退款(可完整预览的文档不适用该条件!)。
- 3、如文档侵犯您的权益,请联系客服反馈,我们会尽快为您处理(人工客服工作时间:9:00-18:30)。
车牌自动识别摘要——车牌自动识别(LPR)在众多的应用程序和一些已经被提出的重要技术中扮演了重要的角色。
然而,他们中的大多数工作在特定的约束条件下,如固定照明,有限的车辆速度,设计好的路线,和固定的背景。
在这项研究中,考虑尽可能减少约束工作环境。
LPR技术包括两个主要模块:车牌定位模块和号码识别模块。
前者是试图从的输入图像中提取车牌上模糊的字符,后者就神经学科概念化而言的目的是识别车牌的号码。
各个模块已进行了实验。
在定位车牌实验研究中,1088个图像从各种场景和不同的条件下拍摄得出。
其中,23个图像未能在图像上找到车牌;车牌定位的成功率是97.9%。
在识别车牌号码实验中,1065个被成功定位的车牌图像进行实验。
其中,47个图像未能识别位于图像中车牌号码,识别成功率是95.6%的。
结合上述两个比率,对于我们的车牌识别算法的总体成功率是93.7%。
索引术语——色彩边沿探测器,模糊化,识别号码许可,车牌定位,车牌识别(LPR),自行组织(SO),字符识别,弹性模型、拓扑分类、两级模糊聚集。
一、引言自动车牌识别(LPR)在许多应用中占有重要的位置,,如无人值守的停车地段[ 31 ],[ 35 ]安全控制限制区[ 8 ]交通执法[ 7 ],[ 33 ],和堵车调查[ 5 ],,自动收费[ 20 ]。
由于不同的工作环境,车牌识别技术的程序多种多样。
大多数以前的技术从某些方面限制了他们的工作环境[ 9 ],如限制他们只能在室内工作,固定的背景[ 30 ],固定的照明[ 7 ],规定的车道[ 22 ],[ 26 ]限定车辆速度[ 1 ],或指定相机和车辆之间的距离范围[ 23 ]。
的目标,这次研究的目的是减少这些限制。
在不同的工作条件下,室外场景和非平稳的背景这两个因素可能是最影响获得图像的质量并且在技术上的需要更加复杂的技术支持。
在一个室外环境中,白天的照明条件变化虽然缓慢,但是由于天气条件和传递的对象(例如,汽车,飞机,云,和立交桥)的变化可能导致的迅速改变。
此外,当他们移动或变焦时,定向相机会创建动态的场景。
这个动态场景的图像可能包含多个牌照或无牌照。
此外,当他们出现在一个图像中时,车牌可能以任意大小,方向和位置出现。
而且,如果涉及复杂的背景,检测的车牌将会变成一个相当有挑战性的事情。
通常,一个车牌识别的过程包括两个主要阶段:1)车牌定位和2)识别车牌号码。
在第一阶段,在候选车牌的基础上确定车牌特征。
常用的特点来有自车牌格式数字和字符构成的车牌编号。
该特征对于车牌格式包括形状,对称性[ 15 ],高度—宽比[ 23 ],[ 25 ],颜色[ 17 ],25 ],灰度纹理[[ 2 ],[ 25 ],空间频率[ 26 ],[ 8 ]和强度值的方差,[ 10 ]。
字符特征包括线[ 34 ],点[ 13 ],梯度值过度标志,字符的纵横比[ 12 ],[ 28 ]的字符之间的间隔分布,和字符对齐【32】。
在现实中,一小部分强大,可靠并且易于检测的对象特点是足能够胜任的。
候选车牌的定位是在车牌号码识别阶段。
有参与识别阶段的两大过程,字符分割和字符识别。
在过去已经完善的车牌分割技术有投影[ 11 ],[ 30 ],形态[ 2 ],[ 10 ],[ 28 ]放宽标记,连接部件[ 25 ],和斑点着色。
每一个技术有自己的优点和缺点。
由于投影方法是假设车牌定位,而另一种形态学方法需要知道字符的尺寸,由于其所需的假设,所以这两种应用方法不适合我们的应用程序。
放宽标记本质上会导致反复和耗时。
在这项研究中,一种混合的连接部件和斑点着色技术被认为最适合字符分割。
已经有大量的字符识别技术报告。
他们包括遗传算法[17],人工神经网络[2], [16], [26], 模糊c-均值聚类[25], 向量计算机支持[16], 马尔科夫过程[6], 和有限时序机[1]。
这些方法大致可分为迭代和非迭代方法。
有一个权衡这两组的方法;迭代方法实现更好的精度,但在增加时间复杂度的成本。
在这项研究中,当需要我们在两者中做出选择时,精度比时间复杂度需要我们投入更多的注意。
为此,我们开发了我们自己的字符识别技术,它是基于人工神经网络和力学学科。
本文的其余部分安排如下,部分II将基于车牌识别技术的思想来阐述车牌类型,所提出的技术的两个主要阶段,车牌定位和车牌号码识别,将分别在部分III 和IV中详细讨论,而部分V将展示新的实验结果,未来的工作想法则在部分VI 给出。
II. 车牌识别技术在这一部分中,车牌的种类在这项研究中进行了讨论,其次简要描述了车牌识别过程。
表一显示了在台湾车辆上车牌的不同种类。
每一个种类都和一类特殊的车辆相关。
这些种类包括私人汽车,出租车,旅游巴士,卡车,及政府车辆。
其他类别的车辆,如汽车和军事外交车辆,都没有解决,因为他们很少看到。
车牌样式可以很容易地基于属性区分:1)所使用的颜色组合,2)车牌号的成分语义。
如表所示I,每个种类都有不同的前景和背景颜色。
然而,在所有四个不同的颜色(白色,黑色,红色,绿色)被使用在这些车牌。
当在输入图像中搜索车牌时,我们要注意这四种颜色。
车牌号码成分中的语义提供了额外的信息来区分车牌样式。
可以在表I中看到,每一个车牌号码是由连字符分隔的两部分(例如,e1-2345)。
第一部分是由两个字组成的,其中之一必须是一个字母字符(例如,E1,2F,和EF)。
第二部分可能含有四(例如,2345)或三(例如,234)个数字,前者只用于私人汽车,后者被用在其他车辆类别。
图1显示了车牌识别过程。
我们假设这个过程发生在一个事件检测系统中,例如,车辆检测器或交通执法系统。
一旦系统检测到一个事件,那么相机会随着系统而激活,然后由相机获得的图像进入车牌识别过程中,从图像中我们提取潜在的车牌。
如果没有牌照被发现,过程返回到等待另一个输入图像。
然而,在通常情况下我们会检测到多个候选车牌,它们在车牌号码识别阶段被仔细检查。
涉及这一阶段的有两个基本任务,字符分割和识别。
这两个任务选择调用以实现分割和识别的最佳结果。
字符从候选车牌在这个阶段,在确认验证阶段之后。
字符组将被视为一个有效的车牌号码如果它符合在前文提到的车牌号码的语义成分。
无论是有效的车牌号码还是相关的车辆类别将由车牌识别过程返回。
然后所有的候选车牌重复进行识别和确认阶段。
之后过程返回到等待图像输入阶段。
在部分III和IV,看看我们的车牌定位模块和车牌识别模块的细节。
III 车牌定位模块A、基本概念一个车牌定位模块的流程图如图2所示。
该模块的输入是RGB彩色图像。
记得,我们认为只有四种颜色(白,黑,红,绿)在牌照中使用。
还注意到,有许多的边缘,这是密切的相互接近和分散在一个重复的方式,包含在一个车牌。
上述的观察促使我们开发的彩色边缘检测。
边缘检测是只有三种敏感的边缘,黑色和白色,红色和白色,绿白色(见最后一列表1)。
由于其他的车牌边缘检测对象很少,即使当图像的背景很混乱,也可以通过忽略图像中的其他类型的边缘来从输入图像的彩色边缘检测,并设E 计算表示地图的边缘。
其次,在HSI空间中对输入的彩色图像的RGB空间转换。
让(R,G,B)和(H,S,I)来表示(红,绿,蓝)和(色调,饱和度,亮度)的图像值像素,分别从(R,G,B)到(H,S,I)[ 3 ]是在那里,有一些有趣的特性与恒生指数相关的颜色模型是有用的应用程序,包括色调和光照阴影的不变性,以及饱和的不变性来观察方向和表面方向。
让H.S和I分别保持映射的色相,饱和度,和变换后的图像的亮度分量。
图像E,H,S 和I对于噪音,误差和不完善处理方面的缺陷是不可避免的。
为了弥补这个缺点,我们呼吁软计算技术植根于模糊(车牌定位)和神经(牌照号码识别)学科。
让H,S,I和E是模糊版本的H,S,I和E,在模糊映射条目表示程度的属于一个车牌。
一个两阶段的模糊集合引入到集成地图。
在第一阶段,模糊地图H,S和I成为一体,由此产生的地图与E在导致一个单一的地图第二阶段下结合,表示M。
使用两阶段聚合的原因是因为固有的特性(颜色相关)的H,S,并且I和E是不同的(边缘的大小有关)。
然后基于地图M,其中在输入图像中感兴趣的区域是区域的最大值m。
然后确定候选车牌中那些感兴趣的区域的大小是足够大的。
B、彩色边缘检测彩色边缘检测器只针对三种边缘(即,黑的白的,红、白、绿白边)。
考虑一个黑色白边,并假设输入的RGB彩色图像被归一化到一个图像。
理想情况下,该(r,g,b)的一个白色像素和黑色像素值分别应该是(1,1,1)和(0,0,0)。
他们的区别是(1,1,1)和(0.0.0)所以所有的组成部分差矢量白色和黑色像素之间会有相同的符号。
这个属性是相当稳定的环境下的变化。
黑白边缘像素是基于此属性如下定义。
图像的像素被视为一个黑白边缘点,如果所有的像素和它的一个邻居是相同的之间的差异向量分量的迹象,例如在N是邻居像素的图像集。
我们也将它的边缘的大小定义为边缘的大小被使用后产生边缘模糊的地图。
在类似的方式,一个图像的像素是一个边缘的是红白色的点,如果它的差分向量对于一些满足以下条件:1);2)和,对边缘像素的大小被定义为,最后,如果对于一些一个图像像素的边缘像素是绿色和白色,那么1),2)和它的边缘的大小是由决定,图像的像素,不是边缘点的那个给出零边值。
C.模糊图生成一个模糊图的基本思想是从一个如下给定的地图(例如H,S,I或E),由于每个地图编码对场景的一些特征,在地图上的任何细胞进入细胞具有这种特性的表现程度。
为了突出对应的感兴趣的对象的细胞(例如,车牌),我们将对这些细胞大条目是与对象的已知特征的兼容。
这样大的作品显示了高度的一个有趣的对象存在。
我们把得到的地图的原始地图的特征地图。
由于输入数据(包括给定的地图和对象的字符—特性)是不完美的,不确定性应考虑—计数的特征映射计算中。
模糊集被称为建模提供了一个优雅的工具不确定性[ 14 ],[ 18 ],[ 27 ]。
在这项研究中,我们引入了模糊—感的特征映射条目和指结果作为模糊的地图。
有几种方法来实现模糊—性。
我们定义了一个广义模糊集,称为“像一个车牌号码,在色调,饱和度,强度相应的设置,和边缘强度。
每四组作为一种通用的设置模糊集合的。
考虑色调值的通用设置。
假设对象感兴趣的颜色是C,而C的色彩值对应的是,在地图H的入口,说h的隶属度是,本条目属于模糊集的“对象”可以写作那里是一个正的常数。
如果给定的输入等于感兴趣的对象,然后隶属度为1。
由于色彩之间的差异增加,会员减少至0的渐近值的程度。
记得,有四种颜色(黑,白,红,绿)用于感兴趣的车牌。
让和的色调值分别变为红色和绿色,请注意,消色差的颜色的色调(即,各级灰色,包括黑色和白色)没有定义由于色调方程的分母为零(1)。