外文文献翻译——基于激光测距仪的行人跟踪
激光测距仪外文翻译

Laser rangefinderA long range laser rangefinder is capable of measuring distance up to 20 km; mounted on a tripod with an angular mount. The resulting system also provides azimuth and elevation measurements.A laser rangefinder is a device which uses a laser beam to determine the distance to an object. The most common form of laser rangefinder operates on the time of flight principle by sending a laser pulse in a narrow beam towards the object and measuring the time taken by the pulse to be reflected off the target and returned to the sender. Due to the high speed of light, this technique is not appropriate for high precision sub-millimeter measurements, where triangulation and other techniques are often used.PulseThe pulse may be coded to reduce the chance that the rangefinder can be jammed. It is possible to use Doppler effect techniques to judge whether the object is moving towards or away from the rangefinder, and if so how fast.PrecisionThe precision of the instrument is determined by the rise or fall time of the laser pulse and the speed of the receiver. One that uses very sharp laser pulses and has a very fast detector can range an object to within a few millimeters.RangeDespite the beam being narrow, it will eventually spread over long distances due to the divergence of the laser beam, as well as due to scintillation and beam wander effects, caused by the presence of air bubbles in the air acting as lenses ranging in size from microscopic to roughly half the height of the laser beam's path above the earth. These atmospheric distortions coupled with the divergence of the laser itself and with transverse winds that serve to push the atmospheric heat bubbles laterally may combine to make it difficult to get an accurate reading of the distance of an object, say, beneath some trees or behind bushes, or even over long distances of more than 1 km in open and unobscured desert terrain.Some of the laser light might reflect off leaves or branches which are closer thanthe object, giving an early return and a reading which is too low. Alternatively, over distances longer than 1200 ft (365 m), the target, if in proximity to the earth, may simply vanish into a mirage, caused by temperature gradients in the air in proximity to the heated surface bending the laser light. All these effects have to be taken into account.CalculationThe distance between point A and B is given byD=ct/2where c is the speed of light in the atmosphere and t is the amount of time for the round-trip between A and B.where is the delay which made by the light traveling and is the angular frequency of optical modulation.Then substitute the values in the equation D=ct/2,D=1/2 ct=1/2 c·φ/ω=c/(4πf) (Nπ+Δφ)=c/4f (N+ΔN)=U(N+)in this equation, U stands for the unit length.Δφ stands for the delay part which does not fulfill π.ΔN stands the decimal value.DiscriminationSome instruments are able to determine multiple returns, as above. These instruments use waveform-resolving detectors, which means they detect the amount of light returned over a certain time, usually very short. The waveform from a laser pulse that hits a tree and then the ground would have two peaks. The first peak would be the distance to the tree, and the second would be the distance to the ground.Using wavefront sensing, it is possible to determine both the closest and the farthest object at a given point. This makes it possible for aircraft-mounted instruments to see "through" dense canopies[clarification needed Please explain how lasers see through canopies]and other semi-reflective surface such as the ocean, leading to many applications for airborne instruments such as:1. Creating "bare earth" topographic maps - removing all trees2. Creating vegetation thickness maps3. Bathymetry(measuring topography under the ocean)4. Forest firehazardTechnologiesTime of flight - this measures the time taken for a light pulse to travel to the target and back. With the speed of light known, and an accurate measurement of the time taken, the distance can be calculated. Many pulses are fired sequentially and the average response is most commonly used. This technique requires very accurate sub-nanosecond timing circuitry.Multiple frequency phase-shift- this measures the phase shift of multiple frequencies on reflection then solves some simultaneous equations to give a final measure.Interferometry - the most accurate and most useful technique for measuring changes in distance rather than absolute distances.ApplicationsMilitaryAn American soldier with a GVS-5 laser rangefinder.A Dutch ISAF sniper team displaying their Accuracy International AWSM .338 Lapua Magnum rifle and Leica/Vectronix VECTOR IV laser rangefinder binoculars. Rangefinders provide an exact distance to targets located beyond the distance of point-blank shooting to snipers and artillery. They can also be used for military reconciliation and engineering.Handheld military rangefinders operate at ranges of 2 km up to 25 km and are combined with binoculars or monoculars. When the rangefinder is equipped with a digital magnetic compass (DMC) and inclinometer it is capable of providing magnetic azimuth, inclination, and height (length) of targets. Some rangefinders can also measure a target's speed in relation to the observer. Some rangefinders have cable or wireless interfaces to enable them to transfer their measurement(s) data to other equipment like fire control computers. Some models also offer the possibility to use add-on night vision modules. Most handheld rangefinders use standard or rechargeable batteries.The more powerful models of rangefinders measure distance up to 25 km and arenormally installed either on a tripod or directly on a vehicle or gun platform. In the latter case the rangefinder module is integrated with on-board thermal, night vision and daytime observation equipment. The most advanced military rangefinders can be integrated with computers.To make laser rangefinders and laser-guided weapons less useful against military targets, various military arms may have developed laser-absorbing paint for their vehicles. Regardless, some objects don't reflect laser light very well and using a laser rangefinder on them is difficult.3-D ModellingThis LIDAR scanner may be used to scan buildings, rock formations, etc., to produce a 3D model. The LIDAR can aim its laser beam in a wide range: its head rotates horizontally, a mirror flips vertically. The laser beam is used to measure the distance to the first object on its path.Laser rangefinders are used extensively in 3-D object recognition, 3-D object modelling, and a wide variety of computer vision-related fields. This technology constitutes the heart of the so-called time-of-flight3D scanners. In contrast to the military instruments described above, laser rangefinders offer high-precision scanning abilities, with either single-face or 360-degree scanning modes.A number of algorithms have been developed to merge the range data retrieved from multiple angles of a single object to produce complete 3-D models with as little error as possible. One of the advantages that laser rangefinders offer over other methods of computer vision is that the computer does not need to correlate features from two images to determine depth information as in stereoscopic methods.Laser rangefinders used in computer vision applications often have depth resolutions of tenths of millimeters or less. This can be achieved by using triangulation or refraction measurement techniques as opposed to the time of flight techniques used in LIDAR.ForestryLaser rangefinder TruPulse used for forest inventories (in combination with Field-Map technology)Special laser rangefinders are used in forestry. These devices have anti-leaf filtersand work with reflectors. Laser beam reflects only from this reflector and so exact distance measurement is guaranteed. Laser rangefinders with anti-leaf filter are used for example for forest inventories.SportsLaser rangefinders may be effectively used in various sports that require precision distance measurement, such as golf, hunting, and archery. Some of the more popular manufacturers are: Opti-logic Corporation, Bushnell, LaserTechnology, Trimble, Leica, Newcon Optik, Nikon, and Swarovski Optik.Industry production processesAn important application is the use of laser Range finder technology during the automation of stock management systems and production processes in steel industry.SafetyLaser rangefinders for consumers are laser class 1 devices and therefore are considered eyesafe. Some laser rangefinders for military use exceed the laser class 1 energy levels.HistoryDevelopment of the methods used in modern printed circuit boards started early in the 20th century. In 1903, a German inventor, Albert Hanson, described flat foil conductors laminated to an insulating board, in multiple layers. Thomas Edison experimented with chemical methods of plating conductors onto linen paper in 1904. Arthur Berry in 1913 patented a print-and-etch method in Britain, and in the United States Max Schoop obtained a patent[1] to flame-spray metal onto a board through a patterned mask. Charles Durcase in 1927 patented a method of electroplating circuit patterns.The Austrian Jewish engineer Paul Eisler invented the printed circuit while working in England around 1936 as part of a radio set. Around 1943 the USA began to use the technology on a large scale to make proximity fuses for use in World War II . After the war, in 1948, the USA released the invention for commercial use. Printed circuits did not become commonplace in consumer electronics until the mid-1950s, after the Auto-Sembly process was developed by the United States Army.Before printed circuits (and for a while after their invention), point-to-point construction was used. For prototypes, or small production runs, wire wrap or turret board can be more efficient. Predating the printed circuit invention, and similar in spirit, was John Sargrove's 1936–1947 Electronic Circuit Making Equipment (ECME) which sprayed metal onto a Bakelite plastic board. The ECME could produce 3 radios per minute.During World War II, the development of the anti-aircraft proximity fuse required an electronic circuit that could withstand being fired from a gun, and could be produced in quantity. The Centralab Division of Globe Union submitted a proposal which met the requirements: a ceramic plate would be screenprinted with metallic paint for conductors and carbon material for resistors, with ceramic disc capacitors and subminiature vacuum tubes soldered in place.Originally, every electronic component had wire leads, and the PCB had holes drilled for each wire of each component. The components' leads were then passed through the holes and soldered to the PCB trace. This method of assembly is called through-hole construction. In 1949, Moe Abramson and Stanislaus F. Danko of the United States Army Signal Corps developed the Auto-Sembly process in which component leads were inserted into a copper foil interconnection pattern and dip soldered. The patent they obtained in 1956 was assigned to the U.S. Army. [4] With the development of board lamination and etching techniques, this concept evolved into the standard printed circuit board fabrication process in use today. Soldering could be done automatically by passing the board over a ripple, or wave, of molten solder in a wave-soldering machine. However, the wires and holes are wasteful since drilling holes is expensive and the protruding wires are merely cut off.In recent years, the use of surface mount parts has gained popularity as the demand for smaller electronics packaging and greater functionality has grown.激光测距仪激光测距仪是一种设备,它采用了激光束来确定对象的距离。
外文文献翻译——基于激光测距仪的行人跟踪
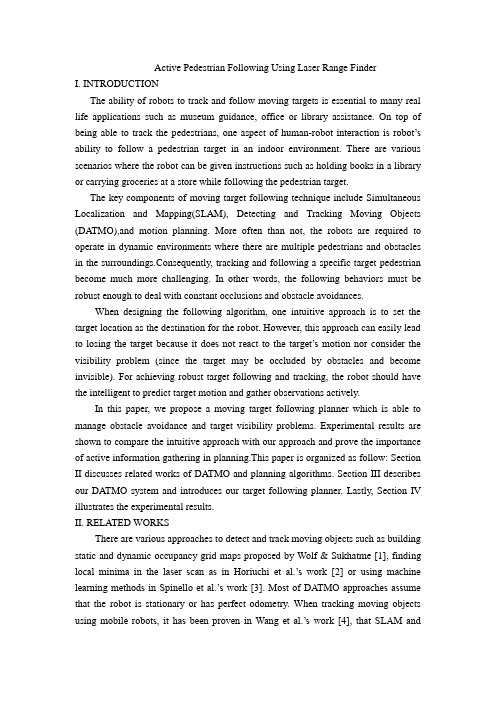
Active Pedestrian Following Using Laser Range FinderI. INTRODUCTIONThe ability of robots to track and follow moving targets is essential to many real life applications such as museum guidance, office or library assistance. On top of being able to track the pedestrians, one aspect of human-robot interaction is robot’s ability to follow a pedestrian target in an indoor environment. There are various scenarios where the robot can be given instructions such as holding books in a library or carrying groceries at a store while following the pedestrian target.The key components of moving target following technique include Simultaneous Localization and Mapping(SLAM), Detecting and Tracking Moving Objects (DATMO),and motion planning. More often than not, the robots are required to operate in dynamic environments where there are multiple pedestrians and obstacles in the surroundings.Consequently, tracking and following a specific target pedestrian become much more challenging. In other words, the following behaviors must be robust enough to deal with constant occlusions and obstacle avoidances.When designing the following algorithm, one intuitive approach is to set the target location as the destination for the robot. However, this approach can easily lead to losing the target because it does not react to the target’s motion nor consider the visibility problem (since the target may be occluded by obstacles and become invisible). For achieving robust target following and tracking, the robot should have the intelligent to predict target motion and gather observations actively.In this paper, we propose a moving target following planner which is able to manage obstacle avoidance and target visibility problems. Experimental results are shown to compare the intuitive approach with our approach and prove the importance of active information gathering in planning.This paper is organized as follow: Section II discusses related works of DATMO and planning algorithms. Section III describes our DATMO system and introduces our target following planner. Lastly, Section IV illustrates the experimental results.II. RELATED WORKSThere are various approaches to detect and track moving objects such as building static and dynamic occupancy grid maps proposed by Wolf & Sukhatme [1], finding local minima in the laser scan as in Horiuchi et al.’s work [2] or using machine learning methods in Spinello et al.’s work [3]. Most of DATMO approaches assume that the robot is stationary or has perfect odometry. When tracking moving objects using mob ile robots, it has been proven in Wang et al.’s work [4], that SLAM andDATMO can be done simultaneously if the measurements can be divided into static and dynamic parts. In our work, we implement a DATMO algorithm which is similar to the one in Montesano et al.’s work [5]. A scan matching method is used to correct robot odometry and moving points are detected by maintaining a local occupancy grid map. Moreover, Extended Kalman Filter(EKF) is applied to track the moving objects.This paper aims to solve moving target following problem with the existence of obstacles. For navigating in static environments, there are many successful works such as Fox et al. [6], Ulrich & Borenstein [7] Minguez & Montano [8], Seder & Petrovi’c [9]. However, those methods are de signed to reach a fixed goal and assume that the environment and robot states are fully-observable. Applying traditional obstacle avoidance algorithms on the target following task can fail easily because a moving target can change its speed and moving direction at anytime and the target can be occluded by obstacles. For planning under imperfect perception, Partially-Observable Markov Decision Process (POMDP) provides a general framework. However, using POMDP to compute optimal policies are usually very computationally expensive because it has to compute a plan over belief space (typically N-1 dimensional for an N-state problem.). For applying POMDPs on practical problems, most works aimed on reducing the dimension of belief space. Such like PBVI Pineau et al. [10], AMDP Roy et al. [11] and MOMDP Sylvie et al.[12]. Those methods are much faster than original POMDP, but their computational complexity are still too high to do real-time planning. Our method in this paper is more like a sub-optimal but fast approach: assuming that the robot always receives the most possible observations and plan a path which can reach the goal and minimize the uncertainty on particular states. For example, in Prentice & Roy [13], the robot aims on reaching the goal with minimum uncertainty on its position, so it may choose a path which is long but has enough localization landmarks.In this paper, we propose a motion planner for moving target following. The planner uses an extension of dynamic window approach propsed by Chou & Lian [14] to find collision-free velocities and choose a proper velocity using heuristic search. Cost functions are designed for minimizing the distance between robot and target and maximize the possibility that the robot can keep observing the target in a fixed time horizon. Additionally, we apply the concept in nearness diagram algorithm such as the one in Minguez &Montanos’ work [8] for computing a better estimation of the distance between robot and target and therefore achieve a smooth, non-hesitating performance.III. MOVING TARGET FOLLOWING AND OBSTACLE A VOIDANCEIt is essential that our DATMO system is able to track the moving target, pedestrian in this case, with great accuracy.The more precise pedestrian location acquired, the better the robot performs when following the target.A.D etecting and Tracking Moving ObjectsFor detecting moving points in the laser data, we adopt the concept of occupancy grid map proposed by Wolf &Sukhatme [1]. A local occupancy grid map is aintained and used to differentiate the moving points. For robot localization, a scan matching technique called Iterated Closest Point (ICP) is used to acquire robot pose. The moving points are filtered out of the data prior to scan matching in order to maintain the pose accuracy. The detected moving points are segmented into numerous clusters and determined if they belong to a pedestrian using features such as motion velocity, local minima, and size. Finally, each of the pedestrian is trackedusing Extended Kalman Filter (EKF) which solves the occlusion problem and provides us the computational advantage in real time performance.B. Following MethodOur goal is to accomplish moving target following with the existence of obstacles. A reasonable solution is to see it as a path planning problem and use obstacle avoidance algorithms to find collision-free actions. In this paper, we apply our previous work, DWA*, Chou et al. [10] as the obstacle avoidance algorithm.The procedure of DWA* is shown in Fig. 1 (a), it is a trajectory-rollout algorithm. The right side of Fig. 1 (a) shows the procedure for computing proper motion commands. First,the environment information is realized as interval configuration for faster processing. Each interval value represents the maximum distance can be raveled by the robot on a certain circular trajectory. Second, the intervals are clustered as navigable areas. Third, for each area, a candidate velocity is determined according to an objective function. For each candidate velocity, a new robot position is computed as a new node and saved in a trajectory tree. Then a node with the minimum estimated cost value will be extracted as the base node for generating new nodes. The procedure is repeated until goal location is expanded or the tree depth reaches a certain value. After the tree expansion stops, the deepest node is determined as a temporal goal, and the present candidate velocity which can lead the robot to the temporal goal is selected.In our work, we used two different methods for integrating DATMO and DWA*: pseudo goal method and trajectory optimization method. Pseudo-goal method is a more intuitive approach which does not consider the target velocity when following.Another approach is trajectory-optimization where a trajectory-rollout controller is used to approximate a predicted target trajectory and maximize target visibility.Their performances will be shown and compared in the next section.1)Pseudo-goal methodWhen implementing people following algorithm, an intuitive approach is setting the present location of the moving target as the goal of navigation algorithm. Consider the tracked target at an angle with respect to the robot, DWA* algorithm will generate an angular and translational velocity which produces an arc-like trajectory. If the goal is within a close proximity of the robot, the angular velocity will be small and the rc-like route often results in an indirect or detour path for the robot to reach the goal (as shown in Fig. 2). On the contrary, if the goal is set further away from the robot at the same angle, any movement of the goal will result in a much larger displacement change compare to when the goal is close to the robot. Therefore, the angular velocity would increase in response to the substantial change of the goal location.Consequently, the trajectory becomes more direct toward the goal.In the pseudo-goal method, the space in front of the robot is divided into 7 trajectories at 35_ , 55_ , 70_ , 90_ ,110_ , 130_ , 145_ as shown in Fig. 3. A pseudo goal is set 3 times the original distance between the goal and robot along each trajectory. After acquiring the pedestrian location (red circle in Fig. 3), the pseudo goal with trajectory closest to the pedestrian location is then selected. By setting the goal further away from the robot, it remedies the issue of small angular velocity and provides a much more direct path to reach the goal.Another problem is the limited sensing ability. In thispaper, the algorithm is implemented on a mobile robot with a 180-degree POV LRF. Here we added an “information gathering mode.” When the moving target enters the“dangerous zone,” (0~35 degree and 145~180 degree in the robot coordinate) the robot will stop and turn to the target direction rapidly. Adding this mode greatly lowers the risk of losing the target.Pseudo-goal method is straight-forward to implement and performs well when the target velocity is nearly constant.However, when the target velocity changes, the pseudo-goal trajectory selected may also alter from one to another and causes the robot to change its moving direction drastically.2)Trajectory optimization methodThough the pseudo-goal method is very simple to implement, it can easily lose track of the target because it does not take the robot’s limited observability into consideration.Another drawback is that the pseudo-goal method can’t react quickly tothe change of target velocity. A better method is to plan according to a reference trajectory but not a fixed location. In this way, target velocity can be considered and the robot observability can be considered by adding terms to the cost function.附录二外文翻译(中文)基于激光测距仪的行人跟踪一、引言在现实生活中机器人跟踪运动目标的能力得到广泛的应用,如博物馆导引,办公室或者图书馆帮助。
激光跟踪仪工作原理 -回复
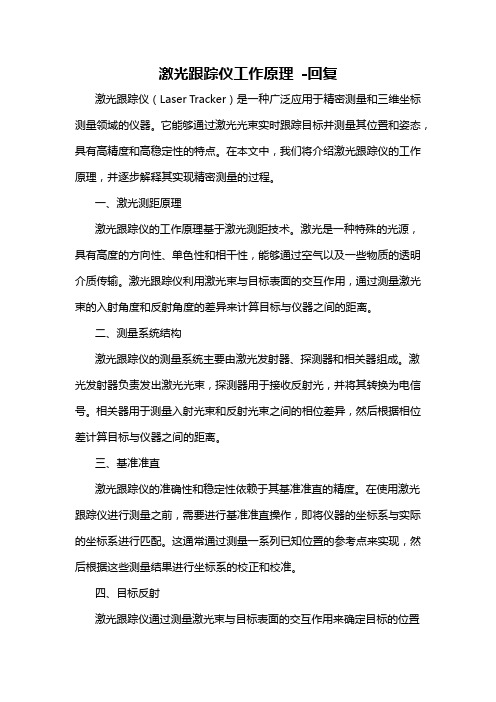
激光跟踪仪工作原理-回复激光跟踪仪(Laser Tracker)是一种广泛应用于精密测量和三维坐标测量领域的仪器。
它能够通过激光光束实时跟踪目标并测量其位置和姿态,具有高精度和高稳定性的特点。
在本文中,我们将介绍激光跟踪仪的工作原理,并逐步解释其实现精密测量的过程。
一、激光测距原理激光跟踪仪的工作原理基于激光测距技术。
激光是一种特殊的光源,具有高度的方向性、单色性和相干性,能够通过空气以及一些物质的透明介质传输。
激光跟踪仪利用激光束与目标表面的交互作用,通过测量激光束的入射角度和反射角度的差异来计算目标与仪器之间的距离。
二、测量系统结构激光跟踪仪的测量系统主要由激光发射器、探测器和相关器组成。
激光发射器负责发出激光光束,探测器用于接收反射光,并将其转换为电信号。
相关器用于测量入射光束和反射光束之间的相位差异,然后根据相位差计算目标与仪器之间的距离。
三、基准准直激光跟踪仪的准确性和稳定性依赖于其基准准直的精度。
在使用激光跟踪仪进行测量之前,需要进行基准准直操作,即将仪器的坐标系与实际的坐标系进行匹配。
这通常通过测量一系列已知位置的参考点来实现,然后根据这些测量结果进行坐标系的校正和校准。
四、目标反射激光跟踪仪通过测量激光束与目标表面的交互作用来确定目标的位置和姿态。
目标通常需要具备一定的反射性能,以便激光光束能够被有效地反射回探测器。
反射性能可以通过目标表面的材料和涂层来控制和改善。
五、跟踪和测量一旦目标反射激光光束被探测器接收到,相关器就会开始测量入射光束和反射光束之间的相位差异。
相位差可以通过不同的技术进行测量,例如在时间上测量或频率上测量。
根据相位差,激光跟踪仪能够计算目标与仪器之间的距离,并通过其他的测量和计算方法来确定目标的位置和姿态。
六、误差校正和数据处理激光跟踪仪的测量过程中会存在一些误差,例如仪器自身的误差、环境影响等。
为了提高测量精度,需要对这些误差进行校正和补偿。
误差校正和数据处理通常采用一些数学模型和算法,根据测量结果进行拟合和计算,以得到最终的测量结果。
机械制造及自动化专业外文翻译--移动机器人基于LFR激光探测器和IR的MFVFA方法

外文原文:Mobile Robot Navigation using Modified Flexible Vector Field Approach with Laser Range Finder and IR sensor Jinpyo Hong ,Youjun Choi and Kyihwan ParkAbstract: In the public space, a mobile robot is adopted as a guider. For guiding a person to the goal position, the mobile robot should make the safe path ,avoid the obstacles and navigate the generated path well. In general, Laser Range Finder is used for the detection of the map around the mobile robot. We propose mobile robot navigation method using our developed a Modified Flexible Vector Field Approach with Laser Range Finder and IR sensor which is used for detecting the emergency status because it has higher response frequency than that of LRF. We will verify that our proposed control scheme and obstacle avoidance algorithm are useful enough to apply to the mobile robot control in the public space by showing experimental results.Keywords: Mobile robot navigation, MFVFA(Modified Flexible Vector Field Approach, LRF(Laser Range Finder), IR sensor)1. INTRODUCTIONWhen a mobile robot is operated as a guider in the public spaces such as a market, a post office, a museumand so on, the most important functionality is the ability to find the path to reach a goal and navigate the goal position. However, In the public space, since there are many obstacles like a shelf, a chair and a person, the mobile robot should avoid the obstacles and reach the goal position simultaneously. For the purpose of navigate the target position, many researchers have addressed a potential field method[1][2], a vector field histogram[3] [5] and a dynamic window approach[6]. Since the market is composed of many narrow passageways and a lot obstacles, there are some difficulty to apply the potential field into our robot as a market application as follows: 1) It is hard to find the passage in the close spaced obstacles. 2) There is an oscillation motion when the robot moves in the narrow passageways. Also the vector field histogram is sensitive to thechange of the environmental map but it can not find the shortest path to reach the goal because we only get the angle information for the mobile robot to go through. The dynamic window uses the heading to goal, distance to obstacles and the velocity of the mobile robot as parameters. Since the dynamic window has an optimisation process for finding the best velocity, this solution is not unique to the shortest path for avoiding the obstacle. For the safe and stable operation of the mobile robot in the public space, a new navigation method is essentially needed which is sensitive to the change of the environment and capable of moving in the shortest path as a guider. Therefore, we proposed a new mobile robot navigation method using modified flexible vector field approach with Laser Range Finder(LRF) and Infra Red(IR) sensor. In our proposed method, since the path informationis obtained from the geometric relation of the obstacle as a circle and the mobile robot as a point, the processing load can be decreased. Since our developed mobile robot has a differential drive structure, the stable velocity of the mobile robot should be firstly considered in this kinematic condition when the mobile robot is controlled toward the generated path. Usually, if the path planner and the path tracker are divided, the various tracking control method are existed like as sliding mode, linearization, backstepping, neural networks, neuro-fuzzy systems. However, the generated root velocity command using those conventional control approaches start with a very large value, and suffers from velocity jumps when sudden tracking errors occur. Therefore, we propose a new velocity profiling approach guaranteeing the stable movement of the mobile robot in this paper. If we assumed that the initial position of the mobile robot and the goal position are given at the first time, we can generate the reference velocity profile using euclidean distances among the start position , the goal position and the current mobile robot position.2. MOBILE ROBOT PLATFORM AND PATH PLANNING2.1 Mobile robot platformFigure 1 is our developed mobile robot platform, GIMaR. The mobile robot has a differential drive structure that has non-holonomic constraint. It has several sensors for detecting the status around the mobile robot. In this research, we only used two sensorsthat one is LRF for detecting the scan map data and another is IR sensor for emergency stop and obstacle avoidance. These two sensors have advantages and drawbacks respectively. LRF generates the scan map data in detail but it is operated slowly compared with IR sensor. On the other hand, IR sensor operates rapidly compared with LRF but it generates one point data. Therefore, we intend to propose the method combining two sensors data for stable driving control and obstacle avoidance.2.2 Path planningIn order lo reach the goal position, we should know he path before starting lo move. If the map data is given in advance, we can generate the safe path to arrive at the goal position but otherwise, we can not do the entire path. In this paper, we assume as follows.1 .Map data is not given in advanced.2. Only the initial position of the mobile robot and the goal position are given.3. Mobile robot has no slip motion and we can know the position and posture of the mobile robot from the odometry information.Under three assumptions, our proposed path planning strategy is shown in Fig. 2. In Fig. 2. the dangerous region indicates an area that the mobile robot has no collision with obstacles. We can know intuitively (hat red line path is the shortest path in safely. Since we don't have map data in advance, we suggest that we find out the next desired point from the geometric relation of dangerous region circle and the current mobile robot position. Although the obstacle exists anywhere, we can determine the next desired point from the our proposed approach, modified flexible vector field approach(MFVFA) because the path planning process is repealed during scan period continuously.3. MOBILE ROBOT CONTROLA nonholonomic mobile robot can be represented by two coordinate systems which are the world coordinate system XG,YG ,SG and the local coordinate system X L,Y L,6L,. In figure 3, C is the robot center point, d is the distance between the left wheel and the right wheel and b is the distance between the center of the wheel and the caster. V and w mean the linear velocity and the angular velocity of the mobile robot.A freely movable mobile robot that is referred as holo-nomic mobile robot has three degrees of freedom(d.o.f.) X, Y,and 0, However, because of the kinematical constraint, the degrees of the freedom for a nonholonomic mobile robot reduces to two. On the conditions of non-slipping, the kinematic constraint of a nonhomolonomic mobile robot is given asFrom the motion control perspective, our developed mobile robot has 2 d.o.f., v C, w c , where v c is the linear velocity and w c is the angular velocity of the mobile robot.The velocities of the two wheels are represented as the diameter of the wheel, r, and the angular velocity wL, wR. .The linear velocity and angular velocity can be described as the relation of e both wheels' angular velocities as follows.In order to control the mobile robot, the velocities of the two wheels can be divided us two parts: one is the part determining the linear velocity of the mobile robot and another is the part tracking the posture of the mobile robot.VL = V C -W CV R=V C +W CWhere, V C is the linear velocity control part and w c is the angular velocity control part. The objective of the linear velocity control is the velocity control of the mobile robot according to the distance between the robot position and the goal position. In the next subsection, we propose the linear velocity generation method using euclidean distance.The mobile robot can avoid the obstacle by controlling the w c. We will explain the posture error feedback method using MFVFA in the next section.4. SIMULATION AND EXPERIMENTAL RESULTSSince we have suggested the obstacle avoidance algorithm for the purpose of using in the narrow space at the market, shelf-like objects are set in the passageway for a test environment. We made up two sections of the market model as a test environment. The size of the test environment is 6 meter by 6 meter. We assume that there are no people in the test environment. In the experimental results, dotted line indicates the desired position, and the red line indicates laser scanning data.The result of multi-obstacle case is also shown in Fig. 11. As we see this experimental result in Fig. 11, if there are multi-obstacles in the test environments, the proposed algorithm provides the safe passageway that the robot can go through without collision. After that, the algorithm generates the shortest trajectory to approach the desired position.Fig. 10Fig. 10 Simulation Result of Stable Curvature Tracking Algorithm - a) the Right Turning Simulation Result, b) the Left Turning Simulation ResultFig. 10 show simulation results of the stable curvature tracking algorithm. These results show how the robot escapes dangerous region when the robot moves into the dangerous region. Wherever the robot is positioned between the dangerous region and collision region, the robot can be controlled toward the circumference of the dangerous region circle. As shown in Fig. 10, the robot makes flexible vector fields with the direction to out-of-dangerous region according to the location of the robot. Therefore, themobile robot moves to the safe region and follows the stable and shortest path for arriving at the goal position.Fig. 11Fig 11 : Result of the Collision Avoidance between Robot and Multi-Obstacles - (a) before applying the collision avoidance algorithm between robot and multi-obstacles (b) after applying the collision avoidance algorithm between robot and multi-obstacles The Trajectories of the robot are shown in Fig. 12 obtained from the experimental results which are shown in Fig. 11. Fig. 12 shows the result that the robot avoids Obstacles safely. We set F{200,10Q), F(150,120), P(200,100), P(16G,180) as starting positions and P(240,450), P(I30,500), (230,500), (170,600) as desired positions. We move the robot with speed or 70cm/s which is same as the walking speed of the human. We made narrow passageway by adding arbitrary obstacles between starting position and desired position. Since there is an obstacle, the robot avoids obstacles, as shown in Fig.12.Fig. 12Fig. 12: Trajectories of robot after applying obstacle avoidance algorithm(a) trajectory of the robot from (200, 100) to (240, 450[Cm]),(b) trajectory of the robot from (180, 160) to (160, 520) [Cm],(c) trajectory of the robot from (200.100) to (230, 500) [Cm],(d) trajectory of the robot from (160,180) to (170, 600) [Cm].5. CONCLUSIONIn this paper, we discussed about the navigation method using the modified flexible vector field with LRF and IR sensors. The controller was divided to the linear velocity control part and the angular control part. The linear velocity stable profile was created considering the stable mobile robot motion using the euclidean distance. For the angular control part, we found out the NOP using the virtual circle originated on the corner point and the tangential straight line. However, since the mobile robot was existed in the collision region, we could control the mobile robot by using the proposed stable curvature algorithm.REFERENCES[1] O. Khalib, Real-rime obstacle avoidance for manipulators and mobile robots,International Journal of Robotics Research, vol. 5. no. 1, pp. 90-98,1995.[2] Peter Vcclaert and Wim Bogacrts . Ultrasonic Potential Field Sensor for ObstacleAvoidance. IEEE Transaction on Robotics and Automation, vol. 15,No. 4, August iyyy.[3]J. Borenstem and Y. Koren, The vector field histogram -fast obstacle avoidance formobile robots, IEEE Transaction on Robotics and Automation, Vol. 7, No. 3, pp.278-288, 1991.[4] Meng Joo Er and Chang Deng, Obstacle Avoidance of a Mobile Robot Using HybridLearning Ap-proachMLMM Transaction on Industrial Electronics. Vol. 52, No, 3, June 2005.[5] J. Borenstein and Y. Korea, Histogramic In Motion Mapping for Mobile RobotObstacle Avoidance,IEEE IRAN. Robotics and Auto, Vol 7, No. 4, 1991.[6] D. Fox, W. Burgard and S. Thrun, The dynamic window approach to collisionavoidance, IEEE Robotics and Automation Magazine, vol 4, pp.23-33, Mar. 1997 [7] R. Balakrishna and A. Ghosal, Modeling of Slip for Wheeled Mobile Robots, IEEETrans. On Robotics and Automation, pp. 126-132, Feb.. 1995中文译文:移动机器人基于LFR激光探测器和IR的MFVFA方法摘要:在公共空间,移动的机器人可以用作导游者。
《2024年基于视觉的行人检测和跟踪技术的研究》范文

《基于视觉的行人检测和跟踪技术的研究》篇一基于视觉的行人检测与跟踪技术的研究一、引言在智能交通系统、安防监控、机器人导航等多个领域,行人检测与跟踪技术具有举足轻重的地位。
这些技术的有效应用能极大提高系统对环境变化的适应能力及决策准确性。
基于视觉的行人检测与跟踪技术,通过图像处理和计算机视觉技术,实现对行人目标的实时检测与跟踪,为上述领域提供了重要的技术支持。
本文将深入探讨基于视觉的行人检测与跟踪技术的研究。
二、行人检测技术行人检测是计算机视觉领域的一个重要研究方向,其目的是在复杂的场景中准确地检测出行人。
目前,基于视觉的行人检测技术主要分为两大类:基于特征的方法和基于深度学习的方法。
1. 基于特征的方法:传统的行人检测方法主要依赖于图像处理技术,如特征提取和分类器设计。
通过提取行人的特定特征,如形状、颜色、纹理等,结合分类器进行行人目标的识别与检测。
然而,这种方法在复杂场景下易受光照、阴影、遮挡等因素的影响,导致检测准确率下降。
2. 基于深度学习的方法:随着深度学习技术的发展,基于深度学习的行人检测方法逐渐成为主流。
通过训练深度神经网络,可以从大量数据中自动学习行人的特征表示,从而更准确地检测出行人。
目前,基于深度学习的行人检测方法已经在许多公开数据集上取得了优秀的性能。
三、行人跟踪技术行人跟踪是通过对行人的位置信息进行预测和更新,实现对行人的持续跟踪。
目前,基于视觉的行人跟踪技术主要采用基于特征匹配和基于深度学习的方法。
1. 基于特征匹配的方法:通过提取行人的特征,如颜色、形状等,在连续的图像帧中进行特征匹配,实现行人的跟踪。
然而,这种方法在复杂场景下易受光照变化、遮挡等因素的影响,导致跟踪准确率下降。
2. 基于深度学习的方法:利用深度神经网络进行行人跟踪,可以有效地解决上述问题。
通过训练神经网络学习行人的动态特征和运动模式,实现对行人的准确跟踪。
目前,基于深度学习的行人跟踪方法在实时性和准确性方面均取得了较好的效果。
《2024年基于视觉的行人检测和跟踪技术的研究》范文
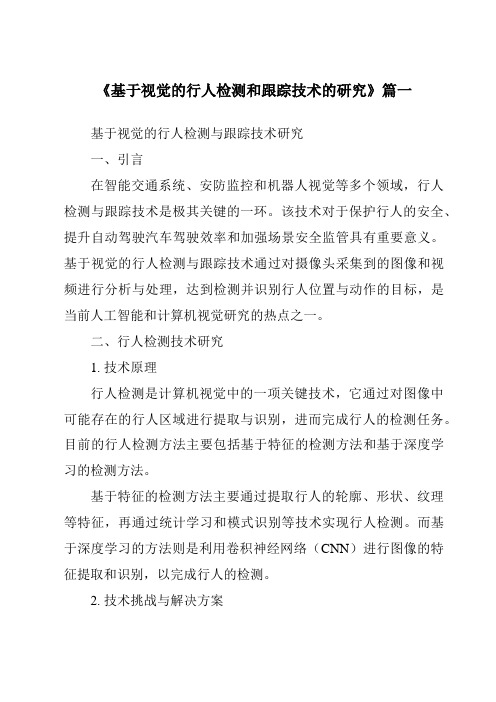
《基于视觉的行人检测和跟踪技术的研究》篇一基于视觉的行人检测与跟踪技术研究一、引言在智能交通系统、安防监控和机器人视觉等多个领域,行人检测与跟踪技术是极其关键的一环。
该技术对于保护行人的安全、提升自动驾驶汽车驾驶效率和加强场景安全监管具有重要意义。
基于视觉的行人检测与跟踪技术通过对摄像头采集到的图像和视频进行分析与处理,达到检测并识别行人位置与动作的目标,是当前人工智能和计算机视觉研究的热点之一。
二、行人检测技术研究1. 技术原理行人检测是计算机视觉中的一项关键技术,它通过对图像中可能存在的行人区域进行提取与识别,进而完成行人的检测任务。
目前的行人检测方法主要包括基于特征的检测方法和基于深度学习的检测方法。
基于特征的检测方法主要通过提取行人的轮廓、形状、纹理等特征,再通过统计学习和模式识别等技术实现行人检测。
而基于深度学习的方法则是利用卷积神经网络(CNN)进行图像的特征提取和识别,以完成行人的检测。
2. 技术挑战与解决方案尽管行人检测技术已经取得了显著的进步,但仍然存在许多挑战。
例如,在复杂的环境中,如光照变化、阴影遮挡、不同视角和姿态变化等情况下,如何准确有效地进行行人检测仍是一个难题。
针对这些问题,研究者们提出了多种解决方案,如使用多尺度特征融合、深度学习模型优化等手段来提高行人检测的准确性和鲁棒性。
三、行人跟踪技术研究1. 技术原理行人跟踪技术主要是通过利用图像序列中的时空信息,对目标行人进行连续的定位和追踪。
该技术通常采用基于滤波器的方法、基于模板匹配的方法或基于深度学习的方法等。
其中,基于深度学习的方法由于其强大的特征提取和学习能力,近年来得到了广泛的应用。
2. 技术应用与挑战行人跟踪技术在智能交通、安防监控等领域有着广泛的应用。
然而,在实际应用中仍存在许多挑战,如遮挡问题、目标行人的快速移动以及光照变化等。
为了解决这些问题,研究者们正在尝试使用更先进的算法和模型结构,如使用多模态信息融合、多目标跟踪算法等来提高行人跟踪的准确性和稳定性。
GPS跟踪系统外文翻译参考文献

GPS跟踪系统外文翻译参考文献(文档含英文原文和中文翻译)译文:基于GPS的动物跟踪系统摘要:野外感知系统是一种用于监测沼泽鹿行为和迁徙模式的无线传感网。
该系统将收集微气候以及动物的位置信息,并将这些信息以数据流的方式使用点对点网络传达到基站。
基站使用网关,将收集到的所有数据上传到互联网上的一个数据库和用基于可视化软件的浏览器将这些信息描绘出来。
每一个点将显示五个信息,即:位置(用GPS),温度,湿度,前进方向和环境亮度。
此外,该节点将有一个实时时钟同步网络和保持时间信息。
一个外部数据闪存空间将会用于记录从传感器和网络节点上获取的数据。
无线电收发机通过点对点的通信协议将数据传送到基站。
一个太阳能接受系统为电池提供节点能量,用于延长节点的寿命。
这个系统将会被做成项圈的形式,这样能比较容易的套在动物的脖子上。
关键词:GPS跟踪;野外感知系统;无线传感网,野生动物跟踪,微型气候感知器I.前言无线传感器网络(WSN)总是使用从分布式自治节点空间获得的感知。
把微型传感器、微控制器、无线电收发机和一种带有电源,低功耗和廉价的传感器节点(我们将简单地称之为节点)结合可以用于监视物理或环境的条件,如不同位置上的温度、声音、振动、压力、运动等。
对于正在移动的节点这个任务将变得更具挑战性。
工程所要进一步研究的问题是如何使节点的电力供应足够维持到最后一年。
这篇文章的重点就是如何通过对数据的处理,改善无限传感网的设计来突破无限传感网在能量方面的束缚。
过去已经见过各种各样的无线传感器网络应用于栖息地监控、地震检测,环境监测、健康系统监统等,这些领域很少会遇到移动节点,动态网络拓扑,通信失败,电力供应有限,恶劣的环境条件变化等情况。
为了从对野生动物监测中寻到解决问题的方法和了解动物与其周围环境的复杂的关系,科学家必须亲自到现场去收集所需要的数据。
在某些情况下将无线电发射机安置动物身上能让研究变得更加容易,但是图像的大部分仍然不能被标记出来,因为缺少有效的数据收集。
行人检测与跟踪国内外研究现状
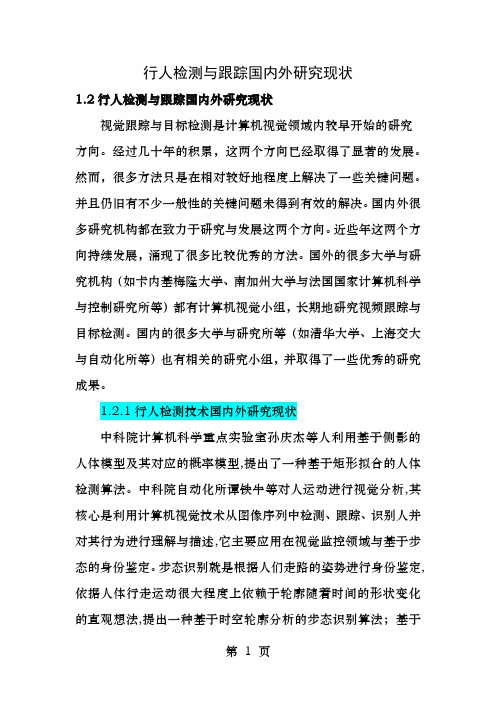
行人检测与跟踪国内外研究现状1.2行人检测与跟踪国内外研究现状视觉跟踪与目标检测是计算机视觉领域内较早开始的研究方向。
经过几十年的积累,这两个方向已经取得了显著的发展。
然而,很多方法只是在相对较好地程度上解决了一些关键问题。
并且仍旧有不少一般性的关键问题未得到有效的解决。
国内外很多研究机构都在致力于研究与发展这两个方向。
近些年这两个方向持续发展,涌现了很多比较优秀的方法。
国外的很多大学与研究机构(如卡内基梅隆大学、南加州大学与法国国家计算机科学与控制研究所等)都有计算机视觉小组,长期地研究视频跟踪与目标检测。
国内的很多大学与研究所等(如清华大学、上海交大与自动化所等)也有相关的研究小组,并取得了一些优秀的研究成果。
1.2.1行人检测技术国内外研究现状中科院计算机科学重点实验室孙庆杰等人利用基于侧影的人体模型及其对应的概率模型,提出了一种基于矩形拟合的人体检测算法。
中科院自动化所谭铁牛等对人运动进行视觉分析,其核心是利用计算机视觉技术从图像序列中检测、跟踪、识别人并对其行为进行理解与描述,它主要应用在视觉监控领域与基于步态的身份鉴定。
步态识别就是根据人们走路的姿势进行身份鉴定,依据人体行走运动很大程度上依赖于轮廓随着时间的形状变化的直观想法,提出一种基于时空轮廓分析的步态识别算法;基于行走运动的关节角度变化包含着丰富的个体识别信息的思想,提出一种基于模型的步态识别算法。
实验结果表明该算法不仅获得了令人鼓舞的识别性能,而且拥有相对较低的计算代价。
但是该方法只能检测出运动的行人。
西安交通大学郑南宁等研究了利用支持向量机识别行人的方法,通过稀疏Gabor滤波器提取行人样本图像中行人的特征,然后利用支持向量机来训练所提取的样本特征,并用训练得到的分类器通过遍历图像的方式将图像中可能属于行人的窗口提取出来。
尽管用Gabor滤波器提取特征效果相对较好,但耗时很长,不适合于实时图像的处理。
上海交通大学田广等提出了一种coarse-to-fine的行人检测方法,将一个人建模成人体自然部位的组装,人体的所有部位包括头肩、躯干与腿、采用绝对值类Haar特征集与Edgelet特征集,在这些特征集上,采用softcascade训练各个部位的检测器与全身检测器。
- 1、下载文档前请自行甄别文档内容的完整性,平台不提供额外的编辑、内容补充、找答案等附加服务。
- 2、"仅部分预览"的文档,不可在线预览部分如存在完整性等问题,可反馈申请退款(可完整预览的文档不适用该条件!)。
- 3、如文档侵犯您的权益,请联系客服反馈,我们会尽快为您处理(人工客服工作时间:9:00-18:30)。
Active PedestrianFollowing Using Laser RangeFinderI. INTRODUCTIONThe ability of robots to track and follow moving targetsis essentialto many real life applications such as museum guidance, office or library assistance.On top of being able to track the pedestrians,one aspectof human-robot interaction is robot 's ability to follow a pedestrian target in an indoor environment. There are various scenarioswhere the robot can be given instructions such as holding books in a library or carrying groceriesat a storewhile following the pedestriantarget.The key componentsof moving target following technique include Simultaneous Localization and Mapping(SLAM), Detecting and Tracking Moving Objects (DATMO),and motion planning. More often than not, the robots are required to operate in dynamic environments where there are multiple pedestriansand obstacles in the surroundings.Consequently,tracking and following a specific target pedestrian become much more challenging. In other words, the following behaviors must be robust enoughto deal with constantocclusionsandobstacleavoidances.When designing the following algorithm, one intuitive approach is to set the target location as the destination for the robot. However, this approachcan easily lead to losing the target becauseit doesnot react to the target 'mo s tion nor consider the visibility problem (since the target may be occluded by obstacles and become invisible). For achieving robust target following and tracking, the robot should have the intelligent to predict targetmotion and gather observationsactively.In this paper, we propose a moving target following planner which is able to manage obstacle avoidance and target visibility problems. Experimental results are shown to compare the intuitive approachwith our approachand prove the importance of active information gathering in planning.This paper is organized as follow: Section II discussesrelated works of DATMO and planning algorithms. Section III describes our DATMO system and introduces our target following planner. Lastly, Section IV illustrates the experimental results.II. RELATED WORKSThere are various approachesto detectand track moving objects suchas building static and dynamic occupancy grid maps proposedby Wolf & Sukhatme[1], finding local minima in the laser scan as in Horiuchi et al. 'w s ork [2] or using machine learning methods in Spinello et al. 'w s ork [3]. Most of DATMO approachesassume that the robot is stationary or has perfect odometry. When tracking moving objects using mobile robots, it has been proven in Wang et al. 'w s ork [4], that SLAM andDATMO can be done simultaneouslyif the measurementscan be divided into static anddynamic parts. In our work, we implement a DATMO algorithm which is similar to the one in Montesanoet al. 'w s ork [5]. A scan matching method is used to correct robot odometry andmoving points aredetectedby maintaining a local occupancygridmap.Moreover, ExtendedKalman Filter(EKF) is applied to track themoving objects.This paperaims to solve moving targetfollowing problem with the existenceof obstacles. For navigating in static environments, there are many successful works such as Fox et al. [6], Ulrich & Borenstein [7] Minguez & Montano [8], Seder & Petrovi '[9c]. However,thosemethodsaredesignedto reach a fixed goal and assume that the environment and robot states are fully-observable. Applying traditional obstacle avoidance algorithms on the target following task can fail easily becausea moving targetcan changeits speedandmoving direction at anytime and the target can be occluded by obstacles. For planning under imperfect perception, Partially- Observable Markov Decision Process (POMDP) provides a general framework. However, using POMDP to compute optimal policies are usually very computationally expensive because it has to compute a plan over belief space (typically N-1 dimensional for an N-state problem.). For applying POMDPs on practical problems, most works aimed on reducing the dimension of belief space. Such like PBVI Pineauet al. [10], AMDP Roy et al. [11] and MOMDP Sylvie et al. [12]. Those methods are much faster than original POMDP, but their computational complexity are still too high to do real-time planning. Our method in this paper is more like asub-optimal but fast approach:assumingthat the robot always receivesthe most possible observationsand plan a path which can reachthe goal and minimize the uncertainty on particular states.For example, in Prentice & Roy [13], the robot aims on reaching the goal with minimum uncertainty on its position, so it may choose a path which is long but hasenoughlocalization landmarks.In this paper, we propose a motion planner for moving target following. The planner usesan extensionof dynamic window approachpropsedby Chou & Lian [14] to find collision-free velocities and choose a proper velocity using heuristic search. Cost functions are designedfor minimizing the distancebetween robot and target and maximize the possibility that the robot can keepobserving the target in a fixed time horizon. Additionally, we apply the conceptin nearnessdiagram algorithm such asthe one in Minguez &Montanos'work [8] for computing a better estimation of the distance between robot and target and therefore achieve a smooth, non-hesitating performance. III. MOVING TARGET FOLLOWING AND OBSTACLE AVOIDANCE It is essentialthat our DATMO systemis able to track the moving target, pedestrian in this case, with great accuracy.Themore precise pedestrianlocation acquired, the better the robot performs when following the target.A.Detecting and Tracking Moving ObjectsFor detecting moving points in the laser data, we adopt the conceptof occupancy grid map proposed by Wolf &Sukhatme [1]. A local occupancy grid map is aintained and used to differentiate the moving points. For robot localization, ascan matching techniquecalled Iterated Closest Point (ICP) is used to acquire robot pose. The moving points are filtered out of the data prior to scan matching in order to maintain the poseaccuracy.The detectedmoving points are segmentedinto numerous clusters and determinedif they belong to a pedestrianusing featuressuch as motion velocity, local minima, and size. Finally, each of the pedestrian is trackedusing Extended KalmanFilter (EKF) which solves the occlusion problem and provides us the computational advantagein real time performance.B. Following MethodOur goal is to accomplish moving target following with the existence of obstacles. A reasonable solution is to seeit as a path planning problem and use obstacle avoidance algorithms to find collision-free actions. In this paper, we apply our previous work, DWA*, Chou et al. [10] as the obstacle avoidancealgorithm.The procedure of DWA* is shown in Fig. 1 (a), it is a trajectory-rollout algorithm. The right side of Fig. 1 (a) shows the procedure for computing proper motion commands. First,the environment information is realized as interval configuration for faster processing. Each interval value represents the maximum distance can be raveled by the robot on a certain circular trajectory. Second, the intervals are clusteredas navigable areas.Third, for each area,a candidatevelocity is determined according to an objective function. For each candidate velocity, a new robot position is computed as a new node and savedin a trajectory tree. Then a node with the minimum estimated cost value will be extracted as the base node for generating new nodes. The procedure is repeated until goal location is expandedor the treedepth reachesa certain value. After the tree expansionstops,the deepestnode is determinedas a temporal goal, and the presentcandidate velocity which can lead the robot to thetemporal goal is selected.In our work, we used two different methods for integrating DATMO and DWA*: pseudogoal methodand trajectory optimization method. Pseudo-goalmethod is a more intuitive approach which does not consider the target velocity when following. Another approach is trajectory-optimization where a trajectory-rollout controller is used to approximate a predicted target trajectory and maximize target visibility.Their performanceswill be shownand comparedin the next section.1) Pseudo-goalmethodWhen implementing people following algorithm, an intuitive approachis setting thepresentlocation of the moving target asthe goal of navigation algorithm. Consider the tracked target at an angle with respectto the robot, DWA* algorithm will generate an angular and translational velocity which producesan arc-like trajectory. If the goal is within a closeproximity of the robot, the angularvelocity will besmall andthe rc- like route often resultsin anindirect or detour path for the robot to reachthe goal (as shown in Fig.2). On the contrary,if the goal is set further away from the robot at the same angle, any movement of the goal will result in a much larger displacement changecompareto when the goal is close to the robot. Therefore,the angularvelocity would increase in response to the substantial change of the goal location.Consequently,the trajectory becomesmore direct toward the goal.In the pseudo-goal method, the space in front of the robot is divided into 7 trajectoriesat 35_ , 55_, 70_ , 90_ ,110_, 130_ , 145_ asshown in Fig. 3. A pseudo goal is set 3 times the original distance between the goal and robot along each trajectory. After acquiring the pedestrian location (red circle in Fig. 3), the pseudo goal with trajectory closest to the pedestrianlocation is then selected.By setting the goal further away from the robot, it remediesthe issue of small angular velocity and provides a much more direct path to reachthe goal.Another problem is the limited sensing ability. In thispaper, the algorithm is implemented on a mobile robot with a 180-degree POV LRF. Here we added an “informationgathering mode.”When the moving target entersthe “dangerouzsone, ” (0~35 degreeand 145~180 degreein the robot coordinate) the robot will stop and turn to the target direction rapidly. Adding this mode greatly lowers the risk of losing the target.Pseudo-goalmethod is straight-forward to implement andperforms well when the target velocity is nearly constant.However, when the target velocity changes, the pseudo-goaltrajectory selected may also alter from oneto another and causesthe robot to changeits moving direction drastically.2) Trajectory optimization methodThough the pseudo-goalmethod is very simple to implement, it can easily lose track of the target because it does not take the robot 'li s mited observability into consideration.Anotherdrawback is that the pseudo-goalmethod can'r t eact quickly to the change of target velocity. A better method is to plan according to a reference trajectory but not a fixed location. In this way, target velocity can be consideredand the robot observability can be consideredby adding terms to the cost function.附录二外文翻译(中文)基于激光测距仪的行人跟踪一、引言在现实生活中机器人跟踪运动目标的能力得到广泛的应用,如博物馆导引,办公室或者图书馆帮助。