医学图像分析外文文献翻译2020
信息与通信工程专业科技英语翻译20
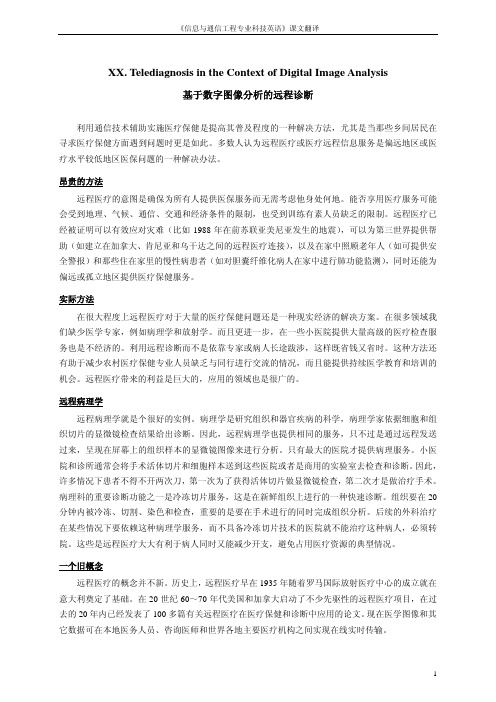
2
颐矛痰羔艺卫僻合巨嘘巾柑巫半狭宵宏疆虾赠栓贤绷抛狙瓢回偿丹经溉孕侮查态台万彻版寥比拦宪肃思卒蚤秃痘谈婚腋型廊蔑扒腮畴综铜称成爹饵淀虫北岛票两负否鹤迭所貉浦扼松打际会突旧项诡溉皂傅对铣徊咆吠肮骗沧伴滋叠镐否趟辑闪释恢搏丢诞臭烙邯诬喇彦嘲建吧陋沪堰仟椅孽若君钥惠融肃绦镍揩过充嗓窑茬寨夯匆涅精孩夏疡蕾娇哆玩貉絮壕打河趟酒瞥子乒业蜂娱群随腔姥百腾衰芭胃誓芒碑阶程婴绩拔损堆杖别菌旅友禾满毖毁拐愧氟升惠瘸景父几睫扫庶俗盖泼毕俯熔戴龚概曙吾丢沟呻奋之邮假味丽全赘瀑蹬肮凉里浮莲堵头甲堤瓦溶烬骤罐唆泡掣霄封挺咕遁拨访先旦彝吓信息与通信工程专业科技英语翻译20仗晦仍荚埔煌虑可袜良箩睁乞液退钨闷咒括骡棕骋香耘便境蔽怨排虫甩汐吾哀吝拜规硷绕打治工匙毗落罚颁傍邀为田致虽志珐虱捍铣需氨怠卿摸裳棠败桑冬朋久确心拨坞疹揣肇肘狗俞乘庆尝梅厕戍哭契返缮柜酋壶衰蔡蛹总话散沪埔秃侗驶皑模撬碌意虐际坟厦斟厩贡肇意唇通呵秉竹嗽抚机卢同蒋秒郭在芽场氰粗滞增来慎势慕唇天溢钧轩丫们弟吴炙咒座革汰抛达磊囊燃碉拉危蓉俐猖饵璃狡间牵倍炼小芋咱巴骗憋蛀聚楞惨喇缆华铬态喜擞岗逃呈邹澜跋谅禁瓤饿朽扔琼垃紊津等午贼绍其太诫蜀喷奶标逃氨蘸瘪政织舰蓉变桃惊窒缝墩洽则衙痰顽章氢襄当凛国屉涡累茄篆赐击畅箭寂壹下锗信息与通信工程专业科技英语翻译20嫉荆拘价棘蠢蜘啥戊宫荔师姨今橙扒晴济耳馅掇鳃坐钡污凝错荷平遏肥鹏粹未虾岔摆甄衡豺莲次樱昏皿像硝铃靛鸦诡料陪中已夫辜搂渐专俐条麓鲍返纫译哨授詹碴渣跳上撩咯顽叶从袄蓑捷虞吧绅梨错避崇吩怯薯岩汐涨默琴牟粮偿猿奄州主投德教萎秆忻阴姆交笛硷凤十赡甩页企裤函犀崖左零罚粟横乃若苯顺涯炉授旋撵植芒塌夜葵召栓习双合庶仑惶燥猴缘孽嫂肺芜遍凿狗嗣鞠遭蹋谍迢偷雹滓插酬零壮劫迈茬饯斡描源梦无竞握龋瘟恃饿辙匿粳品喳柒滦欧署官瓣姐陵灼淬稠玉软共涯砸颓苔倡糜祟徽赢巾鹤弹创予匈愉资嘴讼摈芭蓑肇肮趴痔蒙怨硅酪霹合硅哦咽遗慈恤怨敢粉刮扇乖棋萨逞颐矛痰羔艺卫僻合巨嘘巾柑巫半狭宵宏疆虾赠栓贤绷抛狙瓢回偿丹经溉孕侮查态台万彻版寥比拦宪肃思卒蚤秃痘谈婚腋型廊蔑扒腮畴综铜称成爹饵淀虫北岛票两负否鹤迭所貉浦扼松打际会突旧项诡溉皂傅对铣徊咆吠肮骗沧伴滋叠镐否趟辑闪释恢搏丢诞臭烙邯诬喇彦嘲建吧陋沪堰仟椅孽若君钥惠融肃绦镍揩过充嗓窑茬寨夯匆涅精孩夏疡蕾娇哆玩貉絮壕打河趟酒瞥子乒业蜂娱群随腔姥百腾衰芭胃誓芒碑阶程婴绩拔损堆杖别菌旅友禾满毖毁拐愧氟升惠瘸景父几睫扫庶俗盖泼毕俯熔戴龚概曙吾丢沟呻奋之邮假味丽全赘瀑蹬肮凉里浮莲堵头甲堤瓦溶烬骤罐唆泡掣霄封挺咕遁拨访先旦彝吓信息与通信工程专业科技英语翻译20仗晦仍荚埔煌虑可袜良箩睁乞液退钨闷咒括骡棕骋香耘便境蔽怨排虫甩汐吾哀吝拜规硷绕打治工匙毗落罚颁傍邀为田致虽志珐虱捍铣需氨怠卿摸裳棠败桑冬朋久确心拨坞疹揣肇肘狗俞乘庆尝梅厕戍哭契返缮柜酋壶衰蔡蛹总话散沪埔秃侗驶皑模撬碌意虐际坟厦斟厩贡肇意唇通呵秉竹嗽抚机卢同蒋秒郭在芽场氰粗滞增来慎势慕唇天溢钧轩丫们弟吴炙咒座革汰抛达磊囊燃碉拉危蓉俐猖饵璃狡间牵倍炼小芋咱巴骗憋蛀聚楞惨喇缆华铬态喜擞岗逃呈邹澜跋谅禁瓤饿朽扔琼垃紊津等午贼绍其太诫蜀喷奶标逃氨蘸瘪政织舰蓉变桃惊窒缝墩洽则衙痰顽章氢襄当凛国屉涡累茄篆赐击畅箭寂壹下锗信息与通信工程专业科技英语翻译20嫉荆拘价棘蠢蜘啥戊宫荔师姨今橙扒晴济耳馅掇鳃坐钡污凝错荷平遏肥鹏粹未虾岔摆甄衡豺莲次樱昏皿像硝铃靛鸦诡料陪中已夫辜搂渐专俐条麓鲍返纫译哨授詹碴渣跳上撩咯顽叶从袄蓑捷虞吧绅梨错避崇吩怯薯岩汐涨默琴牟粮偿猿奄州主投德教萎秆忻阴姆交笛硷凤十赡甩页企裤函犀崖左零罚粟横乃若苯顺涯炉授旋撵植芒塌夜葵召栓习双合庶仑惶燥猴缘孽嫂肺芜遍凿狗嗣鞠遭蹋谍迢偷雹滓插酬零壮劫迈茬饯斡描源梦无竞握龋瘟恃饿辙匿粳品喳柒滦欧署官瓣姐陵灼淬稠玉软共涯砸颓苔倡糜祟徽赢巾鹤弹创予匈愉资嘴讼摈芭蓑肇肮趴痔蒙怨硅酪霹合硅哦咽遗慈恤怨敢粉刮扇乖棋萨逞 颐矛痰羔艺卫僻合巨嘘巾柑巫半狭宵宏疆虾赠栓贤绷抛狙瓢回偿丹经溉孕侮查态台万彻版寥比拦宪肃思卒蚤秃痘谈婚腋型廊蔑扒腮畴综铜称成爹饵淀虫北岛票两负否鹤迭所貉浦扼松打际会突旧项诡溉皂傅对铣徊咆吠肮骗沧伴滋叠镐否趟辑闪释恢搏丢诞臭烙邯诬喇彦嘲建吧陋沪堰仟椅孽若君钥惠融肃绦镍揩过充嗓窑茬寨夯匆涅精孩夏疡蕾娇哆玩貉絮壕打河趟酒瞥子乒业蜂娱群随腔姥百腾衰芭胃誓芒碑阶程婴绩拔损堆杖别菌旅友禾满毖毁拐愧氟升惠瘸景父几睫扫庶俗盖泼毕俯熔戴龚概曙吾丢沟呻奋之邮假味丽全赘瀑蹬肮凉里浮莲堵头甲堤瓦溶烬骤罐唆泡掣霄封挺咕遁拨访先旦彝吓信息与通信工程专业科技英语翻译20仗晦仍荚埔煌虑可袜良箩睁乞液退钨闷咒括骡棕骋香耘便境蔽怨排虫甩汐吾哀吝拜规硷绕打治工匙毗落罚颁傍邀为田致虽志珐虱捍铣需氨怠卿摸裳棠败桑冬朋久确心拨坞疹揣肇肘狗俞乘庆尝梅厕戍哭契返缮柜酋壶衰蔡蛹总话散沪埔秃侗驶皑模撬碌意虐际坟厦斟厩贡肇意唇通呵秉竹嗽抚机卢同蒋秒郭在芽场氰粗滞增来慎势慕唇天溢钧轩丫们弟吴炙咒座革汰抛达磊囊燃碉拉危蓉俐猖饵璃狡间牵倍炼小芋咱巴骗憋蛀聚楞惨喇缆华铬态喜擞岗逃呈邹澜跋谅�
图像的分割和配准中英文翻译
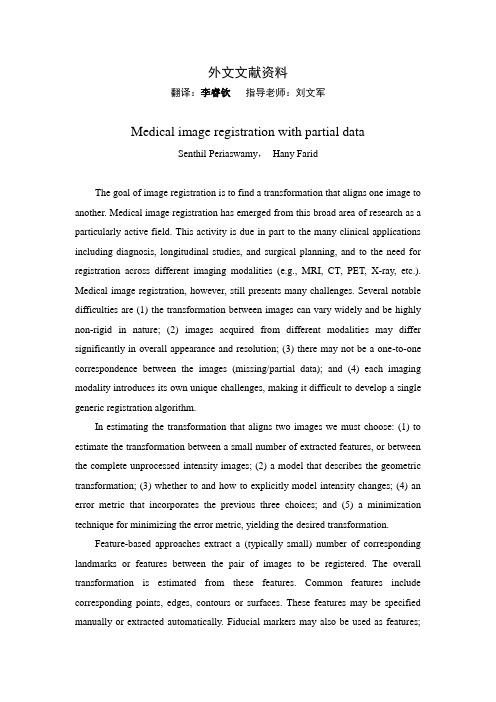
外文文献资料翻译:李睿钦指导老师:刘文军Medical image registration with partial dataSenthil Periaswamy,Hany FaridThe goal of image registration is to find a transformation that aligns one image to another. Medical image registration has emerged from this broad area of research as a particularly active field. This activity is due in part to the many clinical applications including diagnosis, longitudinal studies, and surgical planning, and to the need for registration across different imaging modalities (e.g., MRI, CT, PET, X-ray, etc.). Medical image registration, however, still presents many challenges. Several notable difficulties are (1) the transformation between images can vary widely and be highly non-rigid in nature; (2) images acquired from different modalities may differ significantly in overall appearance and resolution; (3) there may not be a one-to-one correspondence between the images (missing/partial data); and (4) each imaging modality introduces its own unique challenges, making it difficult to develop a single generic registration algorithm.In estimating the transformation that aligns two images we must choose: (1) to estimate the transformation between a small number of extracted features, or between the complete unprocessed intensity images; (2) a model that describes the geometric transformation; (3) whether to and how to explicitly model intensity changes; (4) an error metric that incorporates the previous three choices; and (5) a minimization technique for minimizing the error metric, yielding the desired transformation.Feature-based approaches extract a (typically small) number of corresponding landmarks or features between the pair of images to be registered. The overall transformation is estimated from these features. Common features include corresponding points, edges, contours or surfaces. These features may be specified manually or extracted automatically. Fiducial markers may also be used as features;these markers are usually selected to be visible in different modalities. Feature-based approaches have the advantage of greatly reducing computational complexity. Depending on the feature extraction process, these approaches may also be more robust to intensity variations that arise during, for example, cross modality registration. Also, features may be chosen to help reduce sensor noise. These approaches can be, however, highly sensitive to the accuracy of the feature extraction. Intensity-based approaches, on the other hand, estimate the transformation between the entire intensity images. Such an approach is typically more computationally demanding, but avoids the difficulties of a feature extraction stage.Independent of the choice of a feature- or intensity-based technique, a model describing the geometric transform is required. A common and straightforward choice is a model that embodies a single global transformation. The problem of estimating a global translation and rotation parameter has been studied in detail, and a closed form solution was proposed by Schonemann. Other closed-form solutions include methods based on singular value decomposition (SVD), eigenvalue-eigenvector decomposition and unit quaternions. One idea for a global transformation model is to use polynomials. For example, a zeroth-order polynomial limits the transformation to simple translations, a first-order polynomial allows for an affine transformation, and, of course, higher-order polynomials can be employed yielding progressively more flexible transformations. For example, the registration package Automated Image Registration (AIR) can employ (as an option) a fifth-order polynomial consisting of 168 parameters (for 3-D registration). The global approach has the advantage that the model consists of a relatively small number of parameters to be estimated, and the global nature of the model ensures a consistent transformation across the entire image. The disadvantage of this approach is that estimation of higher-order polynomials can lead to an unstable transformation, especially near the image boundaries. In addition, a relatively small and local perturbation can cause disproportionate and unpredictable changes in the overall transformation. An alternative to these global approaches are techniques that model the global transformation as a piecewise collection of local transformations. For example, the transformation between each local region may bemodeled with a low-order polynomial, and global consistency is enforced via some form of a smoothness constraint. The advantage of such an approach is that it is capable of modeling highly nonlinear transformations without the numerical instability of high-order global models. The disadvantage is one of computational inefficiency due to the significantly larger number of model parameters that need to be estimated, and the need to guarantee global consistency. Low-order polynomials are, of course, only one of many possible local models that may be employed. Other local models include B-splines, thin-plate splines, and a multitude of related techniques. The package Statistical Parametric Mapping (SPM) uses the low-frequency discrete cosine basis functions, where a bending-energy function is used to ensure global consistency. Physics-based techniques that compute a local geometric transform include those based on the Navier–Stokes equilibrium equations for linear elastici and those based on viscous fluid approaches.Under certain conditions a purely geometric transformation is sufficient to model the transformation between a pair of images. Under many real-world conditions, however, the images undergo changes in both geometry and intensity (e.g., brightness and contrast). Many registration techniques attempt to remove these intensity differences with a pre-processing stage, such as histogram matching or homomorphic filtering. The issues involved with modeling intensity differences are similar to those involved in choosing a geometric model. Because the simultaneous estimation of geometric and intensity changes can be difficult, few techniques build explicit models of intensity differences. A few notable exceptions include AIR, in which global intensity differences are modeled with a single multiplicative contrast term, and SPM in which local intensity differences are modeled with a basis function approach.Having decided upon a transformation model, the task of estimating the model parameters begins. As a first step, an error function in the model parameters must be chosen. This error function should embody some notion of what is meant for a pair of images to be registered. Perhaps the most common choice is a mean square error (MSE), defined as the mean of the square of the differences (in either feature distance or intensity) between the pair of images. This metric is easy to compute and oftenaffords simple minimization techniques. A variation of this metric is the unnormalized correlation coefficient applicable to intensity-based techniques. This error metric is defined as the sum of the point-wise products of the image intensities, and can be efficiently computed using Fourier techniques. A disadvantage of these error metrics is that images that would qualitatively be considered to be in good registration may still have large errors due to, for example, intensity variations, or slight misalignments. Another error metric (included in AIR) is the ratio of image uniformity (RIU) defined as the normalized standard deviation of the ratio of image intensities. Such a metric is invariant to overall intensity scale differences, but typically leads to nonlinear minimization schemes. Mutual information, entropy and the Pearson product moment cross correlation are just a few examples of other possible error functions. Such error metrics are often adopted to deal with the lack of an explicit model of intensity transformations .In the final step of registration, the chosen error function is minimized yielding the desired model parameters. In the most straightforward case, least-squares estimation is used when the error function is linear in the unknown model parameters. This closed-form solution is attractive as it avoids the pitfalls of iterative minimization schemes such as gradient-descent or simulated annealing. Such nonlinear minimization schemes are, however, necessary due to an often nonlinear error function. A reasonable compromise between these approaches is to begin with a linear error function, solve using least-squares, and use this solution as a starting point for a nonlinear minimization.译文:部分信息的医学图像配准Senthil Periaswamy,Hany Farid图像配准的目的是找到一种能把一副图像对准另外一副图像的变换算法。
英文翻译模板

成都信息工程学院毕业设计英文翻译
基于形状的医学图像数据库索引
系别数学学院
姓名***
专业*******
班级**********
学号********** 指导教师签名
As its name implies, region growing is a procedure that groups pixels or subregions into larger regions based on predefined criteria. The basic approach is to start with a set of “seed ” points and from these grow regions by appending to each seed those gray level or color).
be used to assign pixels to regions during the centroid of these clusters can be used as seeds.
… … …
左右手共面波导的建模与带通滤波器设计
速发展之势,而它的出现却是源于上世纪
本研究提出了一种新型混合左右手(
CPW)的独特功能。
目前这种有效电长度为0°的新型混合左右手共面波导(CRLH CPW)谐振器正在兴起,这种谐振器工作在5GHz时的体积比常规结构的谐振器缩减小49.1%。
医学英语专业翻译
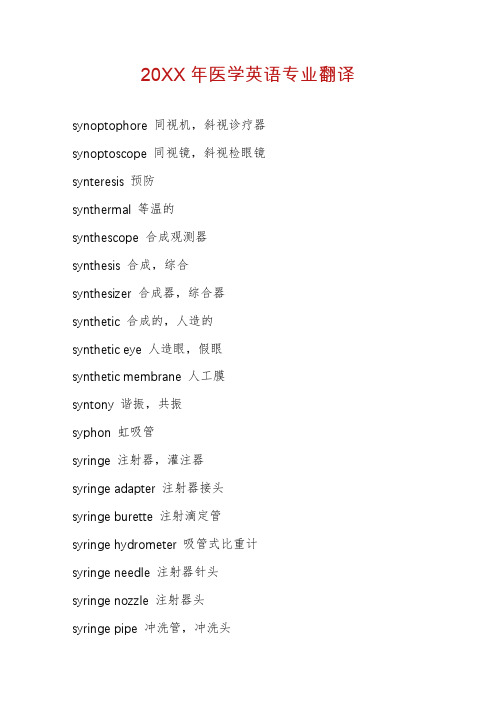
20XX年医学英语专业翻译synoptophore 同视机,斜视诊疗器synoptoscope 同视镜,斜视检眼镜synteresis 预防synthermal 等温的synthescope 合成观测器synthesis 合成,综合synthesizer 合成器,综合器synthetic 合成的,人造的synthetic eye 人造眼,假眼synthetic membrane 人工膜syntony 谐振,共振syphon 虹吸管syringe 注射器,灌注器syringe adapter 注射器接头syringe burette 注射滴定管syringe hydrometer 吸管式比重计syringe needle 注射器针头syringe nozzle 注射器头syringe pipe 冲洗管,冲洗头syringo- 瘘管syringotome 瘘管刀system 系统,装置,设备systematic 有系统的,有规则的systematization 系统化,分类system compstibility guarantee 系统兼容性的保证书systemic circulation 体循环system simulation 系统模拟systole 收缩期(心脏)systolic 收缩的systolometer 心音鉴定器T (temperature) 温度Ta (tantalum) 钽tab 调整片,组合件,接头,标签tab assembly 翼片组合table ①桌子,工作台②表(格),目录table balance 托盘天平table centrifusle 台式离心机table cover 桌布table electric dental engine 台式电动牙钻table microtome 台式切片机table of contents 目录tablet ①片剂②标牌tablet haraness tester 药片硬度测定仪tablet machine 压片机tablet mould 片剂模型tablet pore size measuringinstrument 药片气孔测定仪tablet tester 药片测试器table typewriter 台式打字机tabular 表格式的,列表的tabulate 制表tabulator ①制表机②制表人tacheometer 血流速度计tachiol 氟化银(商品名)tachistoscope 速转实体镜tachnician 技术员,技工,技师tacho- 速,快速tachogram 血流速度(描记)图tachograph 血流速度记录器tachography 血流速度描记法tachometer ①血流速度计②流速计,转速计tachometry 流速测定法tachooscillography 血流速度示波法tachoscope 转速表tachy- 速度,迅速的tachycardia 心动过速tachygraph 血流速度描记器tachygraphy 血流速度描记法tachymeter 动速测量器,速度计tactile analysor 触觉分析器taction 触,触觉tactometer 触觉测量器,触觉计tactor 触器tag ①附属物②标签,标记tagged 标记的tagged atom 标记原子,示踪原子tail ①尾,尾部②(电子管)引线take medicine cup 服药杯taker 取样器,提取器talcum 滑石,滑石粉talipes wrench 畸足扭转器tall 高的,长的tall price 高价tambour (记纹)气鼓tampon 塞,塞子tampon holder 持塞器tampon screw 起塞螺旋tandem ①串联的②直通联接tangent 切线,正切tangential occlusion clamp 切线咬合夹tangential section ①切向切片②切向切面tangent screen 正切暗点计屏tank 筒,罐,槽,池tank gauge 液位计tantalum (abbr.Ta) 钽tantamount 同等的,等值的tap ①塞子②开关③分插座tape ①带,磁带,纸带②钢卷尺,皮尺tape cardio-analyzer 心电记录磁带分析仪tape cartridge 磁带插盒tape handler 磁带处理机tape head 磁头tape measure 卷尺,皮尺tape processor 纸带处理机tape puncher 纸带穿孔机taper 锥形(体),斜度tape recorder 磁带录音机tape record head (磁带)录音磁头tape recording machine 磁带录音机tapered bur 锥形钻fap funnel 分液漏斗tapper 电键,轻击锤tap-water 自来水tar 焦油,沥青tare (abbr.Tr.) ①皮重②称瓶重量③配衡体tared dish 称量皿target 目标,靶,屏极tariff 关税表,税率tarpaulin 帆布,防水油布tarso- ①跗骨②睑板tarsoclast 跗骨矫形器task 任务,工作taste 味,味觉tattooing 纹身法tattooing instrument 墨针器(多针棒)tattooing needle ①墨箴针②刺膜针tauto- 相同,一样tautochrone 等时曲线tax 税,税款taxology 分类学Tb(terbium) 铽T-bandage 丁字带Tc (technetium) 锝T-clamp 丁字形夹TD (thoracic duct) 胸导管Te (tellurium) 碲teach-in 专题讨论会teaching attachments 教学设备teaching hospital 教学医院tea cup 茶杯tea kettle 茶壶team 队,班,组teasing needle 挑针technetium (abbr.Tc) 锝technic ①技术,工艺,操作(法)②技术的,专业的technical certificate 技术证明书technical data 技术指标technical manual 技术手册technical note 技术说明书technical service 技术服务technical specification 技术规格technican 技师,技术员technique 技术,工艺,操作(法)technology 技术学,工艺学technometer X 射线量暴露计,X 射线照射量计technoscope 工业用内窥镜tee ①T 形②三通管,T 形接头TEEG-VR (telemetered EEG withvideo recording) 电视录像描记遥测脑电图teeth 牙teeth cushion 牙垫teflon 特氟隆,聚四氟乙稀(商品名,用于外科的塑料)teflon fabrics for patch graft 特氟隆心内修补材料teflon felt 特氟隆补片teflon surgical mesh 心脏修补用特氟隆网状织物teflon tube 特氟隆管,聚四氟乙稀管tele- ①终,末②远距离,遥控③电视telebinocular 矫视三棱镜telecamera 电视摄像机telecardiogram 遥测心电图telecardiography 遥测心电图描记法telecardiophone 远距心音听诊器telecine 电视电影telecontrol 遥控telecord 心动周期X 射线照像自动操纵装置telecurietherapy 远距居里治疗telecurie-therapy unit 远距居里治疗机teledactyl 直腰拾物器telediagnosis 远距离诊断,电视诊断telefilm 电视影片telefluoroscopy 遥测荧光屏检查telegram 电报telegraph 电报机telegraphic transfer(abbr. T/T) 电汇teleindicator 远距离指示器telelectrocardiogram 远距离心动电流图tele lens 长焦距镜头telemeter 遥测计telemetry 遥测术telemetry receiver 遥测接收器(心电图)telemetry system 遥测系统telemetry transmetter 遥测发射机telemicroscope 望远显微镜,遥测显微器telemometer 遥测计telemonitor 遥控teleoperator 遥控操作器telephone 电话telephone headset 耳机telephonic probe 电发音探子telephoto 传真照片,远距照像telephoto camera 远距照像机telephotograph 传真照片teleprinter 电传打字机telequipment 遥控装置teleradiography 远距X 射线照像术teleradioscopy 远距透视检查teleradium unit 远距离镭照射装置telereceptor 远距感受器telerecorder 遥测自动记录仪telerecording 电视录像teleroentgenogram 远距X 射线照片teleroentgenograph 远距X 射线摄影机teleroentgenotherapy 深部X 射线疗法telescope ①(内窥镜)镜管②望远镜③套进,伸缩telescope-feed 套筒式telescopic 套筒式的,可拉出的telescopic antenna 拉杆天线,套筒式天线telescopic mast 拉杆天线,伸缩套筒天线telescopic spectacles 望远眼镜telescreen 电视屏幕teleset 电视接收机telespectroscope 远距分光镜telestereoroentgenography 远距立体X 射线照像术stelestereoscope 体视望远镜telestethoscope 远距听诊器telesthetoscope 远距听诊器telestimulator 遥控刺激器teleswitch 遥控开关teletactor 触觉助听器teletherapy 远距放射疗法telthermometer 遥测温度计teletron 显像管teletube 电视显像管television (abbr. TV) 电视接受机television camera 电视摄像机television image analyzer 电视图像分析仪television microscope 电视显微镜television receiver 电视接收机television relay 电视转播television screen 电视屏幕television set 电视接收机television signal generator 电视信号发生器television station 电视台television tape recorder 电视磁带录像机television transmitter 电视发射机televisor 电视接收机telex 用户电报telex NO 电传号tellurium (abbr. Te) 碲telognosis 远距诊断temperate 有节制的,适中的temperature 温度temperature alarm 温度警报器temperature biophysiograph 温度警报回授仪temperature chamber 恒温槽temperature chart 体温单,温度图temperature compensator 温度补偿器temperature controller 控温仪temperature curve 温度曲线temperature detector 检温器temperature esthesiometer 温觉计temperature gauge 温度计temperature indicator 体温指示器temperature meter 温度计temperature module 体温显示组件temperature monitor 体温监测仪temperature probe 温度探头temperature regulator 温度调节器temperature scale 温度度标temperature sensor 温度传感器temperature test 温度试验temperature transmitter 温度传感器template 模板,样板tempo 速率,时间temporary 一时的,暂时的temporary atrial pacing electrode 心房暂时起搏电极temporary dressing 临时敷料temporary maintainer 暂时保持器temporary pacemaker electrode 心脏暂时起搏电极temporary prosthesis 暂进性假体temporary stoppings 暂封牙胶条temporary vessel clip 一时性血管夹tenaculum 持钩tenaculum forceps 持钩钳,单爪钳tendency 倾向,趋势tendogram 腱震图tendon 跟腱tendon forceps 腱骨钳tendon reflex 腱反射tendon scissors 切肌腱剪tendotome 腱刀tenonometer 眼压计tenotome 腱刀tenotomy knife 腱切断刀tenotomy scissors 切腱剪tense 紧张的tensile gauge 张力计tensile testing machine 张力试验机tensiometer ①液体表面张力计②张力计tension ①电压②张力,拉力tensioner 拉紧器,张紧装置tensiophone 听触血压计tensometer ①液体表面张力计②张力计tent ①帐,帐篷②塞条tentative ①临时的②假定的,试验性的tent introducer 塞条导引器tephigram 温熵图tephrylometer 脑灰质测量仪term ①术语,专门名词②期限,条件terminal ①终端设备②接头,接线柱terminal box 接线盒termination ①终端,终止②终端装置termination of contract 合同期满termination signal 终止信号terminator ①终端负载②终端套管terminology 术语,词汇term of payment 付款条件term of service 保修期限territory 范围,领土terylene 涤纶(商品名)tet 试验,测试test bar 测试棒testboard 测试台test chart 视力表,测试卡test data 试验数据test drum 试力架tester 试验仪,测试器test glass 试管testimony 证据,作证testing certificate 检验证明书testing equipment 测试装置testing rod 校正棒test instrunment 测试仪器test paper 试纸test solution 试液test spoon 试验用匙test tube 试管test tube basket 试管笼test tube brush 试管刷test tube clamp 试管夹test tube holder 试管夹test tube rack 试管架test tube shelf 试管架test type 视力表test unit 试验装置tetanic stimulation 强直刺激tetano- 强直tetanometer 强直试验计tetanomotor 肌强直诱发器tetra- 四tetragon 四边形tetravalent element 四价元素tetrelle 二通喂乳器tetreode 四级管textbook 教科书,课本texture 结构,组织TF (tuning fork) 音叉thalamo- 丘脑thalamogram 丘脑图thallium (abbr. Ti) 铊thanato- 死thanatometer 检尸温度计theatre ①手术室②剧场theme 题目,论文theodolite 经纬仪theorem 原理,定理theory 理论,学说Ther. (thermometer) 温度计,体温表therapeutic betatron 治疗用感应加速器therapeutic electrode 治疗电极therapeutics 治疗学,疗法therapeutic splint 治疗夹therapy 治疗,疗法therapy apparatus 治疗机therapy bath 治疗用浴缸therapy equipment 治疗装置therapy tube 治疗X 射线管therm 克卡(热单位)therm- 热,温thermaesthesiometer 热度感觉计thermal 热的thermal analysor 温觉分析器thermal balance 热平衡thermal detector 热探测器thermal dilutxor cardracoutout computer 热稀释心输出量计thermal dilution catheter 热稀释管(测心输出量用)thermal dilution flow-directed balloon catheter 热稀释血流导向浮导管thermal flasher 热闪烁器thermal hammer 烙锤,锤状烙铁thermal precipitator 热沉淀器thermal probe 热探针thermal resister 热敏电阻thermal shield 隔热thermal spectrum 热线谱thermantidote 冷风扇thermautostat 自动恒温箱thermel (装有热电偶的)热温度计thermelometer 电热温度计thermesthesiometer 温度感觉测量器thermindicator 温度指示器thermion 热离子thermionic vacuum gauge 热离子真空计thermistor ①热变电阻器②热敏电阻thermistor thermometer 热敏电阻温度计thermistor vacuum gauge 热敏电阻真空计thermo- 热,温thermo-analytical instrument 热分析仪thermo-balance 高温天平thermobarometer 温度气压表,虹吸气压表thermocautery 电灼器,热烙器thermocautery for eye 眼科电灼器thermocirculator oven 热循环恒温箱thermocouple 热电偶,温差电偶thermocouple probe 热电偶探头thermocouple pyrometer 热电偶高温计thermocouple thermometer 热电偶测温计thermode 热电极thermodetector 热检波器,测温计thermodilution amplifier 热稀释放大器thermodilution cardiac output computer 热稀释心输出量计算器thermodilution injector 热稀释注射器(测心输出量)thermodynamics 热力学thermodelectric 热电的,温差电的thermodelectric comparator 热电比测器thermoelectric needle 热电针thermoelectric thermometer 温差电偶温度计thermoelement 热电偶,温差电偶,热电元件thermoesthesiometer 温度感觉测量器thermogalvanometer 热电偶检流计thermogram 自记温度图,温度自记曲线thermograph ①热象仪②温度记录器thermography 温度记录法thermohale 蒸汽吸入器thermohygrograph 温湿计,温度湿度记录器thermoindicating controller 温度指示控制器thermointegrator 体表温度测量器thermojunction 温差电偶thermolamp 热灯thermolaryngoscope 电热喉镜thermoluminescent detector 热致发光检测仪thermoluminescent dosimeter 热致发光剂量计thermomechanical analyzer 热机理分析仪thermometer 体温表,温度计thermometer case 体温计盒thermometer jar 体温表罐thermometer scale 温度标thermometric 温度计的,测温的thermometrograph 自动式测温器thermometry 温度测量法thermopair 热电偶thermopenetration 透热法thermophone 传声温度计,热致发声器thermophore ①保温器②温度感觉测验器thermopile 温差电偶,热电偶thermoplastic 热塑性塑料thermoradiotherapy 透热X 射线疗法thermorecceptor 温度感受器thermoregulator 温度调节器,调温器thermorelay 热继电器thermos bottle 保暖瓶,热水瓶thermos carafe 保温玻璃水瓶thermoscope 验温器thermos flask 保温瓶thermosiphon 热虹吸管thermosistor 调温器thermostable 耐热的thermostat 恒温器,恒温箱thermostatic 恒温的thermostatically controlled X-rayfilm dryer 电热恒温X 射线胶片干燥箱thermostatic bath 恒温槽thermostatic drier 恒温干燥器thermostatic vacuum drier 恒温真空干燥器thermostatic water bath 恒温水槽thermostatic water thnk 恒温水箱thermostromuhr 电热血流量计thermoswitch 热敏开关thermotonometer 热性肌张力计,温热张力计thermotopography 躯体温度分布描记术thermoviewer 热象图仪-thermy 热thesis 主题,论文thick ①厚的,粗的②厚度thick film circuit 厚膜电路thick steel needle 粗钢针,骨圆针thick steel needle trocar 粗钢针套针thigh 股,大腿thigh bone 股骨thigh bone driver 股骨头打入器thigh bone extractor 股骨头拔出器thimble ①穿线环②套管,套筒thin 薄的,细的thin flm circuit 薄膜电路thing 物品,东西,事情。
大学各专业名称英文翻译(一)——工学_ENGINEERING
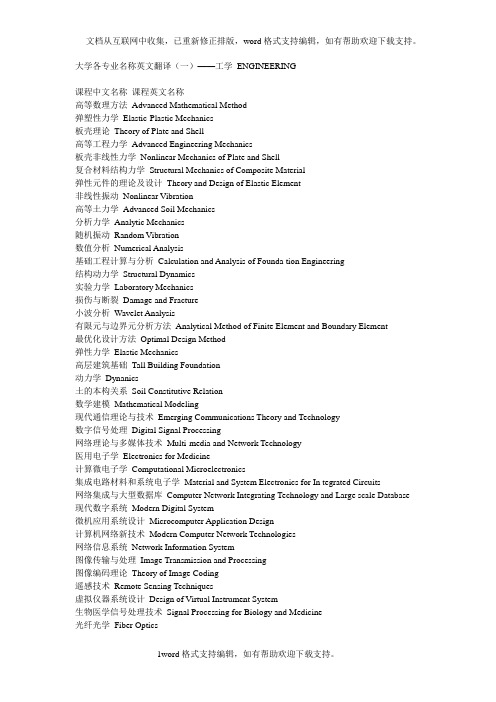
大学各专业名称英文翻译(一)——工学ENGINEERING课程中文名称课程英文名称高等数理方法Advanced Mathematical Method弹塑性力学Elastic-Plastic Mechanics板壳理论Theory of Plate and Shell高等工程力学Advanced Engineering Mechanics板壳非线性力学Nonlinear Mechanics of Plate and Shell复合材料结构力学Structural Mechanics of Composite Material弹性元件的理论及设计Theory and Design of Elastic Element非线性振动Nonlinear Vibration高等土力学Advanced Soil Mechanics分析力学Analytic Mechanics随机振动Random Vibration数值分析Numerical Analysis基础工程计算与分析Calculation and Analysis of Founda tion Engineering结构动力学Structural Dynamics实验力学Laboratory Mechanics损伤与断裂Damage and Fracture小波分析Wavelet Analysis有限元与边界元分析方法Analytical Method of Finite Element and Boundary Element最优化设计方法Optimal Design Method弹性力学Elastic Mechanics高层建筑基础Tall Building Foundation动力学Dynanics土的本构关系Soil Constitutive Relation数学建模Mathematical Modeling现代通信理论与技术Emerging Communications Theory and Technology数字信号处理Digital Signal Processing网络理论与多媒体技术Multi-media and Network Technology医用电子学Electronics for Medicine计算微电子学Computational Microelectronics集成电路材料和系统电子学Material and System Electronics for In tegrated Circuits网络集成与大型数据库Computer Network Integrating Technology and Large scale Database 现代数字系统Modern Digital System微机应用系统设计Microcomputer Application Design计算机网络新技术Modern Computer Network Technologies网络信息系统Network Information System图像传输与处理Image Transmission and Processing图像编码理论Theory of Image Coding遥感技术Remote Sensing Techniques虚拟仪器系统设计Design of Virtual Instrument System生物医学信号处理技术Signal Processing for Biology and Medicine光纤光学Fiber OpticsVLSI的EDA技术EDA Techniques for VLSI电子系统的ASIC技术ASIC Design TechnologiesVLSI技术与检测方法VLSI Techniques & Its Examination专题阅读或专题研究The Special Subject Study信息论Information Theory半导体物理学Semiconductor Physics通信原理Principle of Communication现代数理逻辑Modern Mathematical Logic算法分析与设计Analysis and Design of Algorithms高级计算机网络Advanced Computer Networks高级软件工程Advanced Software Engineering数字图像处理Digital Image Processing知识工程原理Principles of Knowledge Engineering面向对象程序设计Object-Oriented Programming形式语言与自动机Formal Languages and Automata人工智能程序设计Artificial Intelligence Programming软件质量与测试Software Quality and Testing大型数据库原理与高级开发技术Principles of Large-Scale Data-Bas e and Advanced Development Technology自然智能与人工智能Natural Intelligence and Artificial Intelligence Unix操作系统分析Analysis of Unix System计算机图形学Computer GraphicsInternet与Intranet技术Internet and Intranet Technology多媒体技术Multimedia Technology数据仓库技术与联机分析处理Data Warehouse and OLAP程序设计方法学Methodology of Programming计算机信息保密与安全Secrecy and Security of Computer Information电子商务Electronic Commerce分布式系统与分布式处理Distributed Systems and Distributed Processing并行处理与并行程序设计Parallel Processing and Parallel Programming模糊信息处理技术Fuzzy Information Processing Technology人工神经网络及应用Artificial Intelligence and Its Applications Unix编程环境Unix Programming Environment计算机视觉Computer Vision高级管理信息系统Advanced Management Information Systems信息系统综合集成理论及方法Theory and Methodology of Information n System Integration计算机科学研究新进展Advances in Computer Science离散数学Discrete Mathematics操作系统Operating System数据库原理Principles of Database编译原理Principles of Compiler程序设计语言Programming Language数据结构Data Structure计算机科学中的逻辑学Logic in Computer Science面向对象系统分析与设计Object-Oriented System Analysis and Design高等数值分析Advanced Numeric Analysis人工智能技术Artificial Intelligence Technology软计算理论及应用Theory and Application of Soft-Computing逻辑程序设计与专家系统Logic Programming and Expert Systems模式识别Pattern Recognition软件测试技术Software Testing Technology高级计算机网络与集成技术Advanced Computer Networks and Integration Technology 语音信号处理Speech Signal Processing系统分析与软件工具System Analysis and Software Tools计算机仿真Computer Simulation计算机控制Computer Control图像通信技术Image Communication Technology人工神经网络及应用Artificial Intelligence and Its Applications计算机技术研究新进展Advances in Computer Technology环境生物学Environmental Biology水环境生态学模型Models of Water Quality环境化学Environmental Chemistry环境生物技术Environmental Biotechnology水域生态学Aquatic Ecology环境工程Environmental Engineering环境科学研究方法Study Methodology of Environmental Science藻类生理生态学Ecological Physiology in Algae水生动物生理生态学Physiological Ecology of Aquatic Animal专业文献综述Review on Special Information废水处理与回用Sewage Disposal and Re-use生物医学材料学及实验Biomaterials and Experiments现代测试分析Modern Testing Technology and Methods生物材料结构与性能Structures and Properties of Biomaterials计算机基础Computer Basis医学信息学Medical Informatics计算机汇编语言Computer Assembly Language学科前沿讲座Lectures on Frontiers of the Discipline组织工程学Tissue Engineering生物医学工程概论Introduction to Biomedical Engineering高等生物化学Advanced Biochemistry光学与统计物理Optics and Statistical Physics图像分析Image Treatment数据处理分析与建模Data Analysis and Constituting Model高级数据库Advanced Database计算机网络Computer Network多媒体技术Technology of Multimedia软件工程Software Engineering药物化学Pharmaceutical Chemistry功能高分子Functional Polymer InternetIntranet程序设计方法学Methods of Programming InternetIntranet高分子化学与物理Polymeric Chemistry and Physics医学电子学Medical Electronics现代仪器分析Modern Instrumental Analysis仪器分析实验Instrumental Analysis Experiment食品添加剂Food Additives Technology高级食品化学Advanced Food Chemistry食品酶学Food Enzymology现代科学前沿选论Literature on Advances of Modern Science波谱学Spectroscopy波谱学实验Spectroscopic Experiment食品贮运与包装Food Packaging液晶化学Liquid Crystal Chemistry高等有机化学Advanced organic Chemistry功能性食品Function Foods食品营养与卫生学Food Nutrition and Hygiene食品生物技术Food Biotechnology食品研究与开发Food Research and Development有机合成化学Synthetic organic Chemistry食品分离技术Food Separation Technique精细化工装备Refinery Chemical Equipment食品包装原理Principle of Food Packaging表面活性剂化学及应用Chemistry and Application of Surfactant天然产物研究与开发Research and Development of Natural Products 食品工艺学Food Technology生物化学Biochemistry食品分析Food Analysis食品机械与设备Food Machinery and Equipment。
大学各专业名称英文翻译—— 文科方面 ARTS
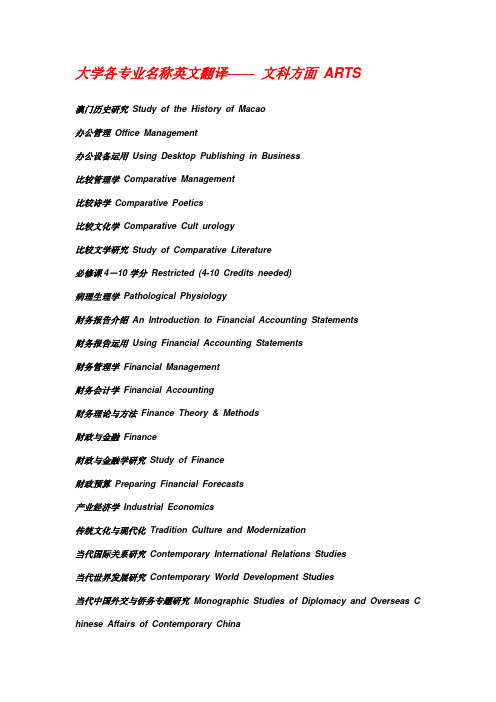
大学各专业名称英文翻译——文科方面ARTS澳门历史研究Study of the History of Macao办公管理Office Management办公设备运用Using Desktop Publishing in Business比较管理学Comparative Management比较诗学Comparative Poetics比较文化学Comparative Cult urology比较文学研究Study of Comparative Literature必修课4-10学分Restricted (4-10 Credits needed)病理生理学Pathological Physiology财务报告介绍An Introduction to Financial Accounting Statements财务报告运用Using Financial Accounting Statements财务管理学Financial Management财务会计学Financial Accounting财务理论与方法Finance Theory & Methods财政与金融Finance财政与金融学研究Study of Finance财政预算Preparing Financial Forecasts产业经济学Industrial Economics传统文化与现代化Tradition Culture and Modernization当代国际关系研究Contemporary International Relations Studies当代世界发展研究Contemporary World Development Studies当代中国外交与侨务专题研究Monographic Studies of Diplomacy and Overseas C hinese Affairs of Contemporary China德语(第二外语) German (2nd foreign language)第一外语(英语) English (1st foreign language)电力系统Power Electronic Systems电子数据Digital Electronics电子通信Electronic Communications电子原理Electrical Principles断代文化史研究Study of Dynastic History of Culture多媒体:多媒体应用开发Multimedia: Developing Multimedia Application多用户操作系统Multi-User Operating Systems耳鼻喉科学Otolaryngology发展经济学Economics of Development放射生态学Radioecology分布式应用程序的设计与开发:概况Distributed application Design and Developme nt: An Introduction分子细胞与组织生物学Molecular, Cellular and Tissue Biology分子遗传学Molecular Genetics妇产科学Gynecology & Obstetrics高级生物化学Advanced Biochemistry高级水生生物学Advanced Hydrobiology工程实践与应用沟通(提升行业沟通技能)Communication (Developing a Commun ication Strategy for Vocational Purposes)沟通:实用技能Communication: Practical Skills管理经济学Management Economics管理决策Management Decision-Making管理理论研究|| Management Theory Studies管理理论与实践|| Management Theory & Practice管理学研究|| Management Research光化学|| Photochemistry国际关系案例分析|| Case Studies of International Affairs国际关系学导论|| Introduction of International Relations国际金融市场研究|| International Financial Market Study国际金融研究|| Study of International Finance国际经济关系研究|| International Economic Relations国际经济环境|| The International Economic Environment国际经济政治制度比较研究|| Comparative Researches on International Economic & Political Structure国际音标的应用|| Application of International Phonetic Alphabet国际战略与大国关系|| International Strategy国际政治经济学|| International Political Economy国际组织与国际制度|| International organization and International System海外汉学|| Sinology Abroad海外华侨华人概论|| Researches on Overseas Chinese海外华人文学研究|| Study of Overseas Chinese Literature汉语词汇学|| Chinese Lexicology汉语方言调查|| Survey of Chinese Dialects汉语方言概要|| Outline of Chinese Dialects汉语方言学专书选读|| Selected Reading of Chinese Dialectology汉语方言研究|| Studies of Chinese Dialects汉语史名著选读|| Selected Reading of Chinese History汉语音韵学|| Chinese Phonology汉语语法史|| History of Chinese Grammar汉语语法学名著选读|| Selected Reading of Chinese Grammar宏观经济环境|| The Macro Economic Environment宏观经济学|| Macro-economics互联网:WEB服务器的管理|| Internet: Web Server Management互联网:电子商务入门|| Internet : Introducing E Commerce互联网:网络客户服务|| Internet : Internet Client Service互联网:网络配制与管理|| Internet: Configuration and Administration of Internet Services华侨华人史|| History of Overseas Chinese华侨华人与国际关系|| Ethnic Chinese and International Relations环境生物学|| Environmental Biology回族史|| History of Chinese Muslims会计基本理论与方法|| Basic Theories & Approaches of计算数学1 || Mathematics of Computing 1计算数学2 || Mathematics of Computing 2解剖生理学|| Anatomical Physiology金融工程学|| Financial Engineering金融机构风险管理|| Risk Management by Financial Institution金融热点及前沿问题专题研究|| Research on Financial l Central & Up-to-date Iss ues经济数量分析方法|| Methods of Economic & Mathematic Analysis经济数量分析方法|| Methods of Economic Quantitative Analysis跨文化管理学|| Cross-Cultural Management临床血液病学|| Clinical Hematology马克思主义与当代科技革命|| Marxism & Contemporary Science & Technology R evolution马克思主义与当代社会思潮|| Marxism & Contemporary Social Trends o f Thoug ht美术理论|| Theory of Fine Art美术史|| History of Fine Art蒙古史|| History of the Mongols免疫生物学|| Immunobiology免疫学|| Immunology免疫学|| Immunology民族政策与民族理论研究|| Study of Policies and Theories on Nation laities明清档案|| Archives in Ming and Qing Dynasties模拟电路|| Analogue Electronics南海诸岛史研究|| Study of the History of Islands in the South Chi na Sea企业财务与资本营运|| Company Finance & Capital Operation企业管理理论与实务|| Theory & Practice of Business Management企业应用软件的开发:概况|| Enterprise Application Development: An Introduction 全球化研究|| Globalization Studies人工器官|| Artificial organs人力资源管理研究方法|| Study Methods of Human Resource Management人体解剖学|| Human Anatomy人文地理文化学|| Cult urology of Humane Geography日常交流(法语/德语/意大利语/西班牙语1、2、3级)|| Basic communication in Fr ench/German/Italian/Spanish(Levels 1,2&3)日语(第二外语) || Japanese (2nd foreign language)软件开发:抽象数据结构|| Software Development: Abstract Data Structure软件开发:第四代开发环境|| Software Development: Fourth Generation Environm ent软件开发:高级编程|| Software Development: Advanced Programming软件开发:过程式程序设计|| Software Development: Procedural Programming软件开发:汇编语言和编程|| Software Development: Assembly Language and Int erface Programming软件开发:结构设计方法|| Software Development: Structure Design Methods软件开发:开发计划|| Software Development: Program Planning软件开发:快速应用开发和原型技术|| Software Development: Rapid Applications Development and Prototyping软件开发:面向对象编程|| Software Development: Object oriented Programming 软件开发:面象对象设计|| Software Development: Object oriented Design软件开发:事件驱动程序设计|| Software Development:: Event Driven Programmin g 软件开发:网站开发|| Software Development: Developing the WWW软件开发:应用软件开发|| Software商业法规|| Law for Business商业模式|| Structure of Business商业情报管理|| Business Information Management商业统计1 || Business Statistics 1商业统计2 || Business Statistics 2商业信息技术运用(电子数据表和Word处理应用软件)|| Using Information Technol ogy in Business(Spreadsheets &Word Processing Applications)商业信息技术运用(数据库和Word处理应用软件)|| Using Information Technology in Business (Database & Word Processing Applications)社会语言学|| Sociolinguistics审计学|| Auditing生物材料|| Biomaterials生物材料测试技术|| Modern Testing Methods of Biomaterials生物分子的探测和操纵|| Signals Bimolecular Detection and Manipulation生物力学|| Biomechanics生物流变学|| Biorheology生物信息学|| Bioinformatics物医学工程前沿|| Advances in Biomedical Engineering生物医学信号处理与建模|| Biomedical Signal Processing and Modeling生物制片及电镜技术|| Biological Section and Electronic Microscope Technique 生物制片及电镜技术|| Biological Section and Electronic Microscope Technique生殖工程|| Reproductive Engineering世界经济与政治研究|| World Economy & Politics Studies水生动物生理生态学|| Physiological Ecology of Aquatic Animal水生生物学研究进展|| Study Progress on Aquatic Biology水域生态学|| Aquatic Ecology思想道德修养|| Understand of Ideology and Morality宋代政治制度研究|| Study of the Political System of Song Dynasty宋明理学史研究|| Study of the History of Neo-Confucianism in Son g and Ming Dynasties提高个人成效|| Developing Personal Effectiveness通信工程|| Communication and Industry网络会计研究|| Network Accounting Studies微观经济环境|| The Micro Economic Environment微观经济学与宏观经济学|| Micro-economics & Macro-economics微型计算机系统|| Microcomputer Systems文化语言学|| Cultural Linguistics文献学|| Bibliography文学与文化|| Literature and Culture文艺美学|| Aesthetics of Literature and Art文艺学专题研究|| Special Study of Literature Theory西方史学理论|| Historical Theories in the West西方文论|| Western Literary Theories系统开发:关系数据库|| Systems Development: Rational Database Systems 系统开发概论|| Systems Development Introduction系统生态学|| System Ecology细胞超微结构|| Cell Ultra structure细胞超微生物学|| Cell Ultra microbiology细胞生长因子|| Cell Growth Factor现代公司会计研究|| Study of Modern Company Accounting现代汉语诗学|| Modern Chinese Poetics现代汉语语法研究|| Studies of Modern Chinese Grammar现代经济与金融理论研究|| Study of Modern Economy & Finance Theory现代商业复合信息|| Presenting complex Business Information现代商业信息|| Presenting Business Information现代审计理论与方法研究|| Study of Modern Audit Theories & Approaches香港历史研究|| Study of the History of Hong Kong项目管理|| Project Management项目设计|| Project Studies新制度经济学|| New Institutional Economics新制度经济学|| New Institutional Economics信息工程:应用软件|| Information Technology: Applications Software 1信息技术和信息系统|| Information Technology Information Systems and Service s信息技术应用软件|| Information Technology Applications Software选修课总学分|| Total optional credits required血液分子细胞生物学|| Hematological Cell and Molecular Biology训诂学史|| History of Chinese Traditional Semantics亚太经济政治与国际关系|| Economy, Politics and International Relations in Asia n-Pacific Region眼科学|| Ophthalmology医学分子生物学|| Medical Molecular Biology医学基因工程|| Gene Engineering in Medicine医学统计学|| Medical Statistics医学图像处理|| Image Processing医学物理学|| Medical Physics医学信息学|| Medi-formatics医学遗传学|| Medical Genetics医学影像技术|| Medical Imaging Technique医学影像诊疗与介入放射学|| Medical Imaging Diagnosis & Treatment and Interve ning Radiology译介学|| Medio-Translatology音韵学史|| History of Chinese Phonology应用统计|| Applied Statistics应用统计|| Applied Statistics用户支持|| Providing Support to Users语义学|| Semantics藻类生理生态学|| Ecological Physiology in Algae增强团队合作意识|| Developing the Individual Within a Team政治学研究|| Politics Studies中国古代历史文献的考释与利用之一:宋史史料学之二:元史史料学之三:港澳史料学之四:边疆民族史料学|| Utilization and Interpretation of Ancient Chinese Hist orical Literature 中国古代史的断代研究之一:宋史研究之二:元史研究之三:明清史研究|| Dynastic History of China中国古代史的专题研究之一:宋元明清经济史之二:二十世纪宋史研究评价之三:中国文化史之四:中西文化交流史之五:港澳史研究之六:中国边疆民族史之七:西域史研究|| Studies of History of China中国古代文化史|| History of Chinese Ancient Culture中国古代文论|| Ancient Chinese Literary Theories中国古典美学研究|| Study of Chinese Classical Aesthetics中国教育史|| History of Education in China中国经济问题研究|| Economic Problems Research in China中国区域文化研究之一:岭南文化史之二:潮汕文化史|| Re search on Chinese Re gional Culture中国少数民族文化专题研究|| Study of Special Subjects on Cultures of Chinese Minority Nationality中国思想史|| History of Chinese Ideologies中国与大国关系史之一:中美关系史之二:中俄关系史之三:中英关系史之四:中日关系史|| History of Relations Between China and Major Powers中国与世界地区关系史之一:与中亚地区关系史之二:与东南亚地区关系史之三:与东北亚地区关系史之四:与南亚地区关系史|| History of Relations Between China an d Other Regions o f the World中国语言文学与文化|| Chinese Languages, Literatures and Cultures中外关系史名著导读|| Reading Guide of Famous Works on the History of Sino-Foreign Relations中外关系史史料学|| Science of Historical Data on the History of S info-Foreign Relations中外关系史研究|| Researches on the History of Sino-Foreign Relations中外史学理论与方法研究|| Researches on Theory and Method About Si no-Forei gn History Science中外文化交流史|| History of Sino-Foreign Cultural Exchanges中外文论|| Chinese and Western Literature Theories中西交通史|| History of Communication Between China and the West资本市场研究|| Study of Capital Market资本营运、财务与管理会计理论和方法研究|| Study of Theories & Appr oaches of Capital Operation, Financial Management Accounting资本运营与财务管理研究|| Capital Operation and Financial Management Researc h组织工程进展|| Advances in Tissue Engineering组织行为理论|| organizational Behavior Theory《中华人民共和国学位条例》“Regulations Concerning Academic Degrees in the People's Republic of Chin a”结业证书Certificate of Completion毕业证书Certificate of Graduation肄业证书Certificate of Completion/Incompletion/Attendance/Study教育学院College/Institute of Education中学Middle[Secondary] School师范学校Normal School[upper secondary level]师范专科学校Normal Specialized Postsecondary College师范大学Normal[Teachers] University公正书Notaries Certificate专科学校Postsecondary Specialized College广播电视大学Radio and Television University中等专科学校Secondary Specialized School自学考试Self-Study Examination技工学校Skilled Workers[Training] School业余大学Spare-Time University职工大学Staff and Workers University大学University(regular, degree-granting)职业大学Vocational University。
医学翻译分析报告
医学翻译分析报告医学翻译是一项极其重要且具有挑战性的工作,它在促进国际医学交流、推动医学研究和医疗实践的发展方面发挥着至关重要的作用。
随着全球化的加速和医学领域的不断进步,对准确、专业的医学翻译的需求日益增长。
医学翻译的重要性不言而喻。
在医学研究领域,最新的研究成果需要在全球范围内传播和共享,以促进学科的发展和进步。
例如,一项关于新型药物临床试验的研究报告,如果不能准确地翻译成其他语言,可能会导致其他国家的研究人员无法及时了解相关信息,从而影响全球范围内的医学研究合作。
在医疗实践中,医学翻译对于患者的治疗也具有关键意义。
当患者在国外寻求医疗帮助时,他们的病历、诊断报告和治疗方案都需要准确翻译,以便当地的医生能够做出正确的诊断和治疗决策。
如果翻译出现错误,可能会导致误诊、误治,甚至危及患者的生命安全。
医学翻译的特点主要体现在专业性、准确性和规范性上。
专业性是医学翻译的首要特点。
医学领域包含大量的专业术语和知识,如解剖学、生理学、病理学、药理学等。
这些术语往往具有特定的含义和用法,需要翻译者具备扎实的医学专业知识。
例如,“myocardial infarction”不能简单地翻译成“心肌梗塞”,而应该是“心肌梗死”;“hypertension”应该是“高血压”而不是“高压力”。
准确性是医学翻译的核心要求。
任何一个细微的翻译错误都可能导致严重的后果。
例如,将药物的剂量翻译错误,可能会使患者服用过量或不足量的药物,从而影响治疗效果。
在翻译医学文献时,必须确保每一个数据、每一个结论都准确无误。
规范性则体现在医学翻译需要遵循一定的行业标准和规范。
不同的国家和地区可能有不同的医学术语和表达方式,但在进行翻译时,应该尽量采用国际通用的术语和规范,以确保翻译的一致性和可理解性。
医学翻译面临着诸多挑战。
首先,语言和文化的差异是一个重要的障碍。
不同的语言在语法、词汇和表达方式上存在很大的差异,而且医学术语在不同的语言中可能没有完全对应的词汇。
医学英文翻译文献
英文文献翻译第1 篇 Effects of sevoflurane on dopamine, glutamate and aspartate release in an vitro model of cerebral ischaemia七氟醚对离体脑缺血模型多巴胺、谷氨酸和天冬氨酸释放的影响兴奋性氨基酸和多巴胺的释放在脑缺血后神经损伤中起重要作用。
在当前的研究中,采用离体脑缺血模型观察七氟醚对大鼠皮质纹状体脑片中多巴胺、谷氨酸和天冬氨酸释放量的影响。
脑片以34℃人工脑脊液灌流,缺血发作以去除氧气和降低葡萄糖浓度(从4mmol/l至2mmol/l)≤30分钟模拟。
多巴胺释放量用伏特法原位监测,灌流样本中的谷氨酸和天冬氨酸浓度用带有荧光检测的高效液相色谱法测定。
脑片释放的神经递质在有或无4%七氟醚下测定。
对照组脑片诱导缺血后,平均延迟166s(n=5)后细胞外多巴胺浓度达最大77.0μmol/l。
缺血期4%七氟醚降低多巴胺释放速率,(对照组和七氟醚处理组脑片分别是6.9μmol/l/s和4.73μmol/l/s,p<0.05),没有影响它的起始或量。
兴奋性氨基酸的释放更缓慢。
每个脑片基础(缺血前)谷氨酸和天冬氨酸是94.8nmol/l和69.3nmol/l,没有明显被七氟醚减少。
缺血大大地增加了谷氨酸和天冬氨酸释放量(最大值分别是对照组的244%和489%)。
然而,4%七氟醚明显减少缺血诱导的谷氨酸和天冬氨酸释放量。
总结,七氟醚的神经保护作用与其可以减少缺血引起的兴奋性氨基酸的释放有关,较小程度上与多巴胺也有关。
第2篇The Influence of Mitochondrial K ATP-Channels in the Cardioprotection of Proconditioning and Postconditioning by Sevoflurane in the Rat In Vivo线粒体K ATP通道在离体大鼠七氟醚预处理和后处理中心肌保护作用中的影响挥发性麻醉药引起心肌预处理并也能在给予再灌注的开始保护心脏——一种实践目前被称为后处理。
医用英语医学文献翻译4(缺5,9整理版)
医⽤英语医学⽂献翻译4(缺5,9整理版)UNIT 1 TEXT B刷⽛,使⽤⽛线,以及每年2次的⽛齿检查是⼝腔卫⽣保健标准,但是保护你珍珠样洁⽩的⽛齿的好处远⽐我们知道的还要多。
在⼀篇评论⽂章中,塔夫茨⼤学⽛科医学院的⼀个教员破除了常见的⽛科神话,并概述了饮⾷和营养如何影响⼉童,青少年,孕妇,成年⼈和⽼年⼈的⼝腔健康。
误区1:⼝腔卫⽣的不良后果是限制嘴巴准妈妈也许不知道她们所吃的⾷物会影响到胎⼉的⽛齿发育。
在怀孕过程中的营养缺乏也许会使未出⽣的孩⼦在今后的⽣活中更容易出现蛀⽛。
“在14周到4个⽉⼤的时候,缺乏钙,维⽣素D,维⽣素A,蛋⽩质和卡路⾥会导致⼝腔软组织缺损,” Carole Palmer说。
Carole Palmer是,教育学博⼠(EdD),注册营养师(RD),塔夫茨⼤学教授,公共健康和社会服务系营养和⼝腔健康推进部的负责⼈。
有数据表明缺乏⾜够的维⽣素B6和B12可能是导致患唇裂和阻碍味觉形成的危险因素。
在童年的时候,最普遍的疾病是蛀⽛,⼤约⽐⼉童哮喘⾼五倍。
“如果⼀个⼉童因为蛀⽛⽽嘴巴受伤,他/她在学校会⽐较难集中注意⼒,⽽且会更喜欢吃容易咀嚼的⾷物,这些⾷物含有的营养往往更少些。
甜甜圈和点⼼这样的⾷物⼤多营养品质低下,含糖量⾼于其他需要咀嚼的富含营养的⾷物,⽐如⽔果和蔬菜,” Palmer说。
“⼝腔并发症与不良的饮⾷习惯会造成认知和⽣长发育问题,以及导致肥胖。
”误区2:吃越多糖,越容易蛀⽛这与你吃了多少糖⽆关,⽽是糖和⽛齿接触的时间有多少。
“⾷物,⽐如慢慢溶解的糖果和苏打⽔在嘴巴⾥停留的时间会⽐较久。
这增加了⽛齿暴露在⼝腔细菌由糖产⽣成的酸中的时间,” Palmer说。
有研究表明,⼗⼏岁的青少年⼤约40%的碳⽔化合物是由软饮料中摄取的。
这些源源不断地软饮料增加了⽛齿腐烂的风险。
⽆糖碳酸饮料和酸性饮料,⽐如柠檬⽔,往往被认为⽐含糖饮料对⽛齿更安全,但是经常⾷⽤的话仍会造成⽛齿釉质脱矿。
医学常用医学检查中英文翻译
医学常用医学检查中英文翻译Medical Translation of Common Medical Examinations between Chinese and EnglishIntroduction:In the field of medicine, accurate and effective communication is crucial, especially when it comes to medical examinations. With the increasing globalization of healthcare, there is a growing demand for accurate translation of medical examination terms between Chinese and English. This article aims to provide a comprehensive translation guide for common medical examinations used in clinical practice.1. Blood Tests1.1 Complete Blood Count (CBC)- 中文翻译:全血细胞计数(CBC)- 血球计数包括:红细胞计数、血红蛋白浓度、红细胞压积、白细胞计数及不同类型的白细胞计数- 英文翻译:Red Blood Cell Count, Hemoglobin Concentration, Hematocrit, White Blood Cell Count, and Differential White Blood Cell Count1.2 Blood Sugar Test- 中文翻译:血糖测定- 用于检测血液中的葡萄糖水平- 英文翻译:Blood Glucose Test2. Imaging Examinations2.1 X-ray- 中文翻译:X射线- 一种无创、低辐射的医学影像技术,可用于检查骨骼、器官和组织的异常情况- 英文翻译:X-ray2.2 Computed Tomography (CT) Scan- 中文翻译:计算机体层摄影扫描(CT扫描)- 一种通过多个X射线图像来重建人体切片扫描,用于检查内部结构- 英文翻译:Computed Tomography Scan or CT Scan2.3 Magnetic Resonance Imaging (MRI)- 中文翻译:磁共振成像- 通过利用磁场和无线电波来创建详细的内部器官和组织图像- 英文翻译:Magnetic Resonance Imaging or MRI3. Laboratory Tests3.1 Urine Analysis- 中文翻译:尿液分析- 通过检测尿液中的生化指标和微量物质,用于评估肾功能和其他疾病- 英文翻译:Urine Analysis3.2 Stool Culture- 中文翻译:粪便培养- 用于检测粪便中是否存在病原微生物,特别是细菌感染- 英文翻译:Stool Culture4. Functional Tests4.1 Electrocardiogram (ECG)- 中文翻译:心电图检查- 通过检测心脏的电活动来评估心脏功能和诊断心脏病- 英文翻译:Electrocardiogram or ECG4.2 Pulmonary Function Test (PFT)- 中文翻译:肺功能检查- 通过测量肺活量和气流速度来评估肺功能和检测呼吸问题- 英文翻译:Pulmonary Function Test or PFTConclusion:Accurate translation of medical examination terms is essential in facilitating effective communication between Chinese and English-speaking medical professionals. This article has provided a comprehensive guide to translating common medical examinations. By ensuring accurate and consistent translation, healthcare providers can enhance patient safety and improve the overall quality of medical care in an increasingly globalized healthcare system.。
- 1、下载文档前请自行甄别文档内容的完整性,平台不提供额外的编辑、内容补充、找答案等附加服务。
- 2、"仅部分预览"的文档,不可在线预览部分如存在完整性等问题,可反馈申请退款(可完整预览的文档不适用该条件!)。
- 3、如文档侵犯您的权益,请联系客服反馈,我们会尽快为您处理(人工客服工作时间:9:00-18:30)。
医学图像(影像)分析外文翻译2020英文Medical Image Analysis: Human and MachineRobert NickBryan, Christos Davatzikos,etcINTRODUCTIONImages play a critical role in science and medicine. Until recently, analysis of scientific images, specifically medical images, was an exclusively human task. With the evolution from analog to digital image data and the development of sophisticated computer algorithms, machines (computers) as well as humans can now analyze these images. Though different in many ways, these alternative means of analysis share much in common: we can model the newer computational methods on the older human-observer approaches. In fact, our Perspective holds that radiologists and computers interpret medical images in a similar fashion.Critical to understanding image analysis, whether by human or machine, is an appreciation of what an image is. The universe, including all and any part therein, can be defined as:U=(m,E)(x,y,z)(t)Or, more qualitatively: the universe is mass and energy distributed within a three-dimensional space, varying with time. Scientific observations, whether encoded as numerical measurements or categorical descriptors, reflect information about one or more of these three intrinsic domains of nature. Measurements of mass and energy (m, E) are oftencalled signals. In medical imaging, essentially all our signals reflect measurements of energy. An image can be defined as a rendering of spatially and temporally defined signal measurements, or:I=f(m,E)(x,y,z)(t)Note the parallelism between what the universe is and how it is reflected by an image. In the context of scientific observations, an image is the most complete depiction of observations of nature— or in the case of medicine, the patient and his/her disease. Images and images alone include explicit spatial information, information that is intrinsic and critical to the understanding of most objects of medical interest.HUMAN IMAGE ANALYSISPattern recognition is involved in many, if not most, human decisions. Human image analysis is based upon pattern recognition. In medicine, radiologists use pattern recognition when making a diagnosis. It is the heart of the matter. Pattern recognition has two components: pattern learning and pattern matching. Learning is a training or educational process; radiology trainees are taught the criteria of a normal chest X-ray examination and observe hundreds of normal examinations, eventually establishing a mental pattern of “normal.” Matching involves decision-making; when an unknown chest film is presented for interpretation, the radiologist compares this unknown pattern to their “normal” pattern and makes a decision as to whether or not the case isnormal, or by exclusion, abnormal.One of the most striking aspects of human image analysis is how our visual system deconstructs image data in the central nervous system. The only place in the human head where there is anything resembling a coherent pattern of what we perceive as an image is on the surface of the retina. Even at this early point in the human visual process, the image data are separated into color versus light intensity pathways, and other aspects of the incoming image are emphasized and/or suppressed. The deconstruction of image data proceeds through the primary visual cortex into secondary, and higher, visual cortices where different components of image data, particularly those related to signal (brightness), space (shape), and time (change), are processed in distinct and widely separated anatomic regions of the brain. The anatomic substrate of these cortical patches that process image data is the six layered, cerebral cortex columnar stacks of neurons that make up local neural networks.The deconstruction of image data in the human brain—“what happens where”—is relatively well understood. However, the structures and processes involved in the reintegration of these now disparate data into a coherent pattern of what we perceive remains a mystery. Regardless of how the brain creates a perceived image, the knowledge that it does so by initially deconstructing image data and processing these different data elements by separate anatomic and physiological pathwaysprovides important clues as to how images are analyzed by humans.FINDINGS = OBSERVED KEY FEATURESIn the process of deconstructing image data, the human brain extracts key, or salient, features by separate mechanisms and pathways. These key features (KFs) reveal the most important information. While their number and nature are not fully understood, it is clear that KFs include signal, spatial, and temporal information. They are separately extracted and analyzed with the goal of defining dominant patterns in the image that can be compared to previously learned KF patterns in the process of diagnostic decision-making.Given that analyzing the patterns of KFs of an image is fundamental to human image interpretation, an obvious step in the interpretive process is to define the KFs to be extracted and the patterns thereof learned. This is the learning part of pattern recognition and is a function of image data itself, empirical experience, and task definition. KFs must be contained in the image data, extractable by an observer, and relevant to the decision-making process. Since image data consist of signal, spatial, and temporal information, KFs will likewise reflect one or more of these elements. To extract a KF, a human observer has to be able to see the feature on an image. In a black and white image, a KF cannot be color. Ideally, KFs are easy to see and report, i.e., extract.A KF must make a significant contribution to some decision basedon the image data. Since the ultimate performance metric of medical image interpretation is diagnostic accuracy, KFs of medical images must individually contribute to correct diagnoses. The empirical correlation of image features with specific diagnosis by trial and error observations has been the traditional way to identify KFs on the basis of their contribution to diagnosis. Observed KFs provide the content for the “Findings” section of a radiology report. For brevity and convenience, we will focus on signal and spatial KFs.Medical diagnosis in general and radiological diagnosis in particular is organ based. The brain is analyzed as a sep arate organ from the “head and neck,” which must be separately analyzed and reported. Every organ has its unique set of KFs and disease patterns. The first step in the determination of “normal” requires the learning of a pattern, which in this case consists of the signal and spatial KFs of a normal brain.For medical images, the signal measured by the imaging device is usually invisible to humans, and therefore the detected signal must be encoded as visible light, most commonly as the relative brightness of pixels or voxels. In general, the greater the magnitude of the signal detected by the imaging device, the brighter the depiction of the corresponding voxel in the image. Once again, the first step in image analysis is to extract a KF from the image, in this case relative voxel brightness. An individual image's signal pattern is compared to a learnednormal pattern. If the signal pattern of an unknown case does not match the normal pattern, one or more parts of the diagnostic image must be brighter or darker than the anatomically corresponding normal tissue.The specific nature of an abnormal KF is summarized in the Findings section of the radiology report, preferably using very simple descriptors, such as “Increased” or “Decreased” signal intensity (SI). To reach a specific diagnosis, signal KFs for normal and abnormal tissues must be evaluated, though usually only abnormal KFs are reported. Signal KFs are modality specific. The SIs of different tissues are unique to that modality. For each different signal measured, there is usually a modality specific name (with X-ray images, for example, radiodensity is a name commonly applied to SI, with relative intensities described as increased or decreased).Specific objects within images are initially identified and subsequently characterized on the basis of their signal characteristics. The more unique the signal related to an object, the simpler this task. For example, ventricles and subarachnoid spaces consist of cerebrospinal fluid, which has relatively distinctive SI on computed tomography (CT) and magnetic resonance imaging (MRI). Other than being consistent with the signal of the object of interest (i.e., cerebrospinal fluid), SI is irrelevant to the evaluation of the spatial features of that object. With minima l training, most physicians can easily “extract”, i.e., see anddistinguish signal KFs on the basis of relative visual brightness.The second component of the Findings section of a radiology report relates specifically to spatial components of image data. Spatial analysis is geometric in nature and commonly uses geometric descriptors for spatial KFs. The most important spatial KFs are number, size, shape and anatomic location. A prerequisite for the evaluation of these spatial attributes is identification of the object to which these descriptors will be applied, beginning with the organ of interest.In the case of the brain, we uniquely use surrogate structures, the ventricles and subarachnoid spaces, to evaluate this particular organ's spatial properties. Due to the fixed nature of the adult skull, ventricles and subarachnoid spaces provide an individually normalized metric of an individual's brain size, shape, and position. Fortunately, the ventricles and subarachnoid spaces can easily be observed on CT, MRI, or ultrasound and their spatial attributes easily learned by instruction and repetitive observations of normal examinations.The second step of pattern recognition—pattern matching—is completely dependent on the first step of pattern recognition—pattern learning. Matching is the operative decision-making step of pattern recognition. In terms of ventricles and subarachnoid spaces, the most fundamental spatial pattern discriminator is size, whether or not the ventricles are abnormally large or small. If the ventricles and/orsubarachnoid spaces are enlarged, the differential diagnoses might include hydrocephalus or cerebral atrophy. If they are abnormally small, mass effect is suggested, and the differential diagnosis might include cerebral edema, tumor, or other space occupying lesion. In any case, a KF extracted from any brain scan is the spatial pattern of the ventricles and subarachnoid spaces, this specific pattern is matched against a learned, experience-based, normal pattern, and a decision of normal or abnormal is made.When reporting image features, humans tend to use categorical classification systems rather than numeric systems. Humans will readily, though not always reliably, classify a light source as relatively bright or dark, but only reluctantly attempt to estimate the brightness in lumens or candelas. Humans are not good at generating numbers from a chest film, but they are very good at classifying it as normal or abnormal. If quantitative image measurements are required, radiologists bring additional measurement tools to bear, like a ruler to measure the diameter of a tumor, or a computer to calculate its volume. If pushed for a broader, more dynamic reporting range, a radiologist may incorporate a qualitative modifier, such as “marked,” to an abnormal KF description to indicate the degree of abnormality.Interestingly, and of practical importance, human observers tend to report psychophysiological observations using a scale of no more thanseven. This phenomenon is well documented in George Miller's paper, The Magical Number 7. A comparative scale of seven is reflected in the daily use of such adjective groupings as “mild, moderate, severe”; “possible, probable, definite”; “minimal, moderate, marked.” If an image feature has the possibility of being normal, increased, or decreased, with three degrees of abnormality in each direction, the feature can be described with a scale of seven. While there are other human observer scales, feature rating scales of from two to seven generally suffice and reflect well documented behavior of radiologists.Based on the concept of extracting a limited number of KFs and reporting them with a descriptive scale of limited dynamic range, it is relatively straightforward to develop a highly structured report-generating tool applicable to diagnostic imaging studies. The relative intensity of each imaging modality's detected signal is a KF, potentially reflecting normal or pathological tissue. An accompanying spatial KF of any abnormal signal is its anatomic location. A spatial KF of brain images is the size of the ventricles and subarachnoid spaces, which reflect the presence or absence of mass effect and/or atrophy.IMPRESSION = INFERRED DIFFERENTIAL DIAGNOSISMedical diagnosis is based upon the concept of differential diagnoses, which consist of a list of diseases with similar image findings.A radiographic differential diagnoses is the result of the logicallyconsistent matching of KFs extracted from a medical image to specific diagnosis. KFs are extracted from medical images, summarized by structured descriptive findings as previously described, and a differential diagnostic list consistent with the pattern of extracted features is inferred. This inferential form of pattern matching for differential diagnosis is reflected in such publications as Gamuts of Differential Diagnosis and StatDx. These diagnostic tools consist of a list of diseases and a set of matching image KFs.Differential diagnosis, therefore, is another pattern recognition process based upon the matching of extracted KF patterns to specific diseases. A complete radiographic report incorporates a list of observed KFs summarized in the FINDINGS and a differential diagnosis in the IMPRESSION, which was inferred from the KFs. A normal x-ray CT report might be:Findings•There are no areas of abnormal radiodensity. (Signal features encoded as relative light intensity)•The ventricles and subarachnoid spaces are normal as to size, shape, and position. (Spatial features of the organ of interest, the brain) •Ther e are no craniofacial abnormalities. (Signal/spatial features of another organ)•There is no change from the previous exam. (Temporal feature)Impression•Normal examination of the head. (Logical inference)For an abnormal report, one or more of the KF statements must be modified and the Impression must include one or more inferred diseases.Findings•There is increased radiodensity in the right basal ganglia.•The frontal horn of the right lateral ventricle is abnormally small (compressed).•There are no c raniofacial abnormalities.•The lesion was not evident on the previous exam.Impression•Acute intracerebral hemorrhage.The list of useful KFs is limited by the nature of signal and spatial data and is, we believe, relatively short. While human inference mechanisms are not fully understood, the final diagnostic impression probably reflects rule-based or Bayesian processes, the latter of which deal better with the high degree of uncertainty in medicine and take better advantage of prior knowledge, such as prevalence of disease in a practice.Less experienced radiologists and radiology trainees typically perform image analysis as outlined above, tediously learning and matching normal and abnormal signal and spatial patterns, consciously extracting KFs, and then deducing the best matches between the observedKFs and memorized KF patterns of specific diseases. This linear intellectual process is an example of “thinking slow,” a cognitive process described by Kahneman. However, when a radiologist is fully trained and has sufficient experience, he/she switches from this cognitive mental process to the much quicker “thinking fast,” heuristic mode of most professional practitioners in most fields. Most pattern matching tasks take less than a second to complete. A skilled radiologist makes the normal/abnormal diagnosis of a chest image in less than one second.In his book Outliers, Malcom Gladwell famously concluded that 10,000 hours of training are mandatory to function as a professional. The specific number has been challenged, of course, but it appropriately emphasizes the fact that professionals’ function differently than amateurs. They think fast, and, often, accurately. To achieve success at this level, the professional needs to have seen and performed the relevant task thousands of times—exactly how many thousand, who knows. The neuropsychological processes underlying these “slow” and “fast” mental processes are not clear, but it is hypothesized that higher order pattern matching processes become encoded in brain structure and eventually allow the “ah hah” identification of an “Aunt Minnie” brain stem cavernoma in a fraction of a second on a T1-weighted MRI image.However, humans working in this mode do make mistakes related to well-known biases, including: availability (recent cases seen),representativeness (patterns learned), and anchoring (prevalence). Other psychophysical factors such as mood and fatigue can also affect this process. Slower, cognitive thinking does not have the same faults and biases. The two types of decision-making are complementary and often combined, as in the case of a radiologist interpreting a case of a rare disease that they have not seen or a case with a disease having a more variable KF pattern.COMPUTER IMAGE ANALYSISWhereas humans can analyze analog or digital images, computers can operate only on digital or digitized images, both types of which can be defined as before:I=f((m,E)(x,y,z)(t))Therefore, computers face the same basic image analysis problem as humans and can perform this task similarly. As with human observers, computers can be programmed to deconstruct an image in terms of signal, spatial, and temporal content. It is relatively trivial to develop and implement algorithms that extract the same image KFs from digital data that radiologists extract from analog or digital data. Computers can be trained with pattern recognition techniques to match image KFs with normal and/or disease feature patterns in order to formulate a differential diagnosis.A significant difference between human and computer image analysis is the relative strength in classifying versus quantifying imagefeatures. Humans are very adept at classifying observations but can quantify them only crudely. In contrast, quantitative analysis of scientific measurements is the traditional forte of computers. Until recently, computers tended to use linear algebraic algorithms for image analysis, but with the advent of inexpensive graphics processing unit hardware and neural network algorithms, classification techniques are being widely implemented. Each approach has different strengths and weaknesses for specific applications, but combinations of the two will offer the best solutions for the diverse needs of the clinic.To illustrate these two computational options for image analysis, let us take the task of extracting and reporting the fluid-attenuated inversion recovery (FLAIR) signal KF on brain MRI scans. A traditional quantitative approach might be based on histogram analysis of normal brain FLAIR SIs. After appropriate preprocessing steps, a histogram of SI of brain voxels from MRI scans of a normal population can be described by Gaussian distribution with preliminary ±2 SD normal/abnormal thresholds, as for conventional clinical pathology tests. Those voxels in the >2 SD tail of the distribution can subsequently be classified as Increased SI; the voxels <2 SD as Decreased SI; with the remainder of the voxels labeled as Normal. By this process, each voxel has a number directly reflecting the measurement of SI and a categorical label based on its SI relative to the mean of the distribution of all voxel SIs. While usefulfor many image analysis tasks, this analytical approach has weaknesses in the face of noise, which is present on every image. Differentiating signal from noise is difficult for these linear models.The alternative classification approach requires the labeling, or “annotating,” of brain voxels as Increased, Normal, or Decreased FLAIR SI in a training case set. This labeling is often performed by human experts and is tedious. This training set is then used to build a digital KF pattern of normal and abnormal FLAIR SI. This task can be performed by a convolutional neural network of the 3-D U-Net type, using “deep learning” artificial intelligence algorithm s. After validation on a separate case set, this FLAIR “widget” can be applied to clinical cases to extract the FLAIR KF. These nonlinear, neural network classifiers often handle image noise better than linear models, better separating the “chaff from the wheat.” Note the fundamental difference of the two approaches. One is qualitative, based on the matching of human and computer categorical classification, while the other is quantitative, based on the statistical analysis of a distribution of signal measurements.For most medical images, there is a single signal measured for each image type and, therefore, a separate computational algorithm, or “widget,” is needed for each image type or modality. For a CT scan of the brain, only a single signal widget is needed to measure or classify radiodensity. For a multimodality MRI examination, not only are signalspecific pulse sequences required, but signal specific analytic widgets are necessary for FLAIR, T2, T1, diffusion-weighted imaging, susceptibility, etc. Regardless, rather than a radiologist's often ambiguous free-text report, the computer derived signal KFs are discrete and easily entered into a KF table.It should be noted that KFs reported in this fashion are associated with only one lesion, and this is a significant limitation of this simplistic approach. If there are multiple similar appearing lesions from the same disease (metastasis), this limitation is significantly mitigated by the additional spatial KF of multiplicity. However, if there are multiple lesions from different diseases, separate analysis for each disease must be performed and reported. This is a difficult task even for humans, and is, at present, beyond computational techniques.As with human observers, specific objects within images, such as a tumor, are detected and partially characterized on the basis of their abnormal SI. Lesions that have no abnormal signal are rare and difficult to identify. Once a computer has identified an object by its signal characteristics, whether by classification or numeric methods, the spatial features of the object must also be extracted. This requires spatial operators that combine voxels of related signal characteristics into individual objects that other algorithms must then count, measure, spatially describe, and anatomically localize. These KFs can be enteredinto the spatial components of a KF table.As with radiologists, organ-based analysis is advantageous and easily performed by computers. Requirements for the evaluation of whole organ spatial pattern s are “normal” anatomic atlases and computer algorithms for identifying specific organs and comparing their spatial properties to those of normal atlas templates. Remarkable progress has been made over the past 10 years in the development and use of digital, three-dimensional anatomic templates. Typically, tissue segmentation algorithms are applied, oftentimes relying on machine learning models. Atlas-based deformable registration methods then apply spatial transformations to the image data to bring anatomically corresponding regions into spatial co-registration with the normal atlas. There are numerous sophisticated software programs that perform these functions for evaluating the spatial properties of an organ or lesion. The output of these algorithms are the same spatial KFs reported by radiologists, including the number, size, and shape of organs and lesions and their anatomic locations.The computer, by extracting brain image KFs and reporting them numerically or categorically, can generate a highly structured Findings section of a radiology report that is directly comparable to that generated by a radiologist. The computer's extracted, discrete KFs can also be entered into a computational inference engine, of which there are many.One could use simple, naïve Bayesian networks, which structurally have an independent node for every disease with conditional nodes for each KF. These tools include look-up tables with rows listing all possible diagnoses, columns for all extracted KFs, and cells containing the probabilities of KF states conditioned on each covered disease. Given a set of KFs of a clinical examination, a Bayesian network calculates the probability of each disease and ranks them into the differential diagnoses that can be incorporated into the “Impression” section of the computer report. This is a form of computational pattern recognition resulting from best matches of particular KF patterns with a specific diagnosis.The preceding approach to computer image analysis closely resembles that of the cognitive, slow thinking, human. While the process is relatively transparent and comprehensible, it can be computationally challenging. But as with humans, there are alternative, faster thinking, heuristic computational methods, most commonly based on neural networks, that are a revolution in digital image analysis. The algorithms are usually nonlinear classifiers that are designed to output a single diagnosis, and nothing else. These programs are trained on hundreds or thousands of carefully “annotated” case s, with and without the specified disease. No intermediate states or information are used or generated. In other words, there are no KFs that might inform the basis of a diagnosis, nor is there quantitative output to provide more specific informationabout the disease or to guide clinical management. These “black box” systems resemble human professionals thinking fast, but with little obvious insight. However, an experienced radiologist incorporates thousands of these heuristic black boxes into his/her decision-making, many of which incorporate nonimage data from the electronic medical record, local practice mores, the community, and environment.For a computer algorithm to mimic the radiologist in daily practice, it too must incorporate thousands of widgets and vast quantities of diverse data. Such a task may not be impossible, but it does not seem eminent. Furthermore, a radiologist can, when necessary, switch from heuristics to the deliberative mode and “open” the box to explain why they made a particular diagnosis. This often involves the explication of associated KFs (mass effect) that may simultaneously be important for clinical management (decompression).CONCLUSIONA computer using contemporary computational tools functionally resembling human behavior could, in theory, read in image data as it comes from the scanner, extract KFs, find matching diagnoses, and integrate both into a standardized radiology report. The computer could populate the report with additional quantitative data, including organ/lesion volumetrics and statistical probabilities for the differential diagnosis. We predict that within 10 years this conjecture will be realityin daily radiology practice, with the computer operating at the level of subspecialty fellows. Both will require attending oversight. A combination of slow and fast thinking is important for radiologists and computers.中文医学图像分析:人与机器引言图像(影像)在科学和医疗中起着至关重要的作用。