运维自动化平台白皮书
IT数据中心运维服务白皮书
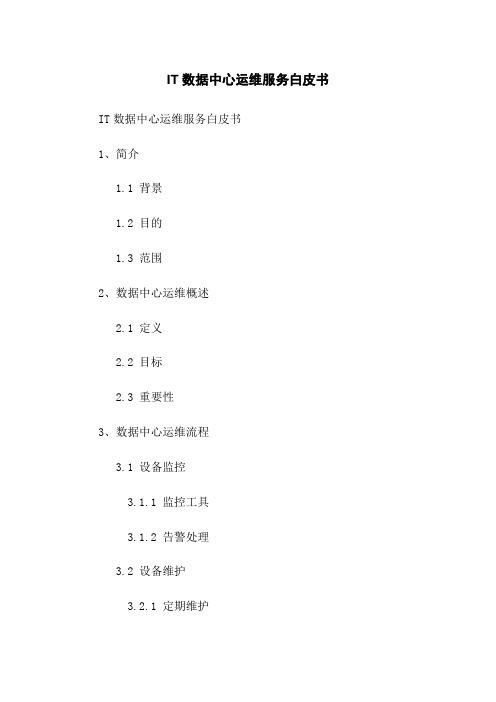
IT数据中心运维服务白皮书IT数据中心运维服务白皮书1、简介1.1 背景1.2 目的1.3 范围2、数据中心运维概述2.1 定义2.2 目标2.3 重要性3、数据中心运维流程3.1 设备监控3.1.1 监控工具3.1.2 告警处理3.2 设备维护3.2.1 定期维护3.2.2 预防性维护3.3 容量规划与增长3.3.1 现有资源评估 3.3.2 容量规划策略 3.4 安全措施3.4.1 物理安全3.4.2 逻辑安全3.5 数据备份与恢复3.5.1 备份策略3.5.2 恢复测试4、运维服务级别协议4.1 定义4.2 协议内容4.2.1 运维响应时间 4.2.2 故障处理时间 4.2.3 售后支持4.3 违约责任和违约处理5、数据中心运维团队5.1 团队组成5.2 人员角色与职责5.3 培训与发展计划6、数据中心运维最佳实践6.1 设备标准化6.2 问题管理6.3 自动化工具和流程6.4 文档管理和知识库6.5 持续改进7、附件附件二、数据中心设备监控工具推荐注释:法律名词及注释:1、运维:维护与运营的合称,是指对设备、系统或网络等进行管理、维护和保养,以确保其正常运行和可靠性。
2、数据中心:指用于集中托管大量计算机服务器、存储系统和网络设备的设施,用于处理、存储和传输大量数据和信息。
3、服务级别协议:是提供给客户和运维服务提供商之间的协议,明确了双方对于服务响应时间、故障处理、售后支持等方面的约定。
4、违约责任和违约处理:指当一方违反了服务级别协议中的约定时,另一方可以要求违约方承担相应责任,并对违约行为进行处理。
5、自动化工具和流程:指使用自动化软件和流程来提高运维效率,减少人为操作错误的发生。
6、持续改进:指持续对运维流程和实践进行审查和改进,以提高运维效率和质量。
银讯IT安全运维管理平台技术白皮书
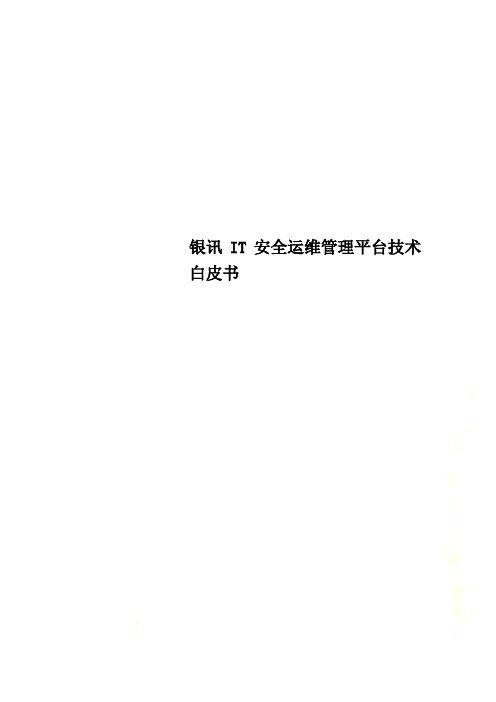
银讯IT安全运维管理平台技术白皮书银讯IT安全运维管理平台技术白皮书银讯IT安全运维管理平台技术白皮书目录一、产品理念 (2)二、市场定位 (3)三、产品架构 (3)四、产品特点 (5)4.1 模块化 (5)4.2 自动发现 (5)4.3 标准化 (5)4.4 无插件 (5)4.5 全IT架构 (5)4.6 跨平台 (5)4.7 扩展性 (6)五、主要功能介绍 (6)5.1 拓扑发现 (6)5.2 设备管理 (7)5.3 网络设备配置文件 (9)5.4 IP地址管理 (10)5.5 SNMP Trap接收与翻译 (12)5.6 Syslog接收与日志审计 (13)5.7 机房管理 (14)5.8 数据库管理 (15)5.9 中间件管理 (16)5.10 丰富的报表 (17)5.11 功能强大的告警 (18)六、系统运行环境 (23)一、产品理念随着我国信息化建设的不断深入,企业的运作越来越依赖于计算机网络。
如何高效地管理好网络和网络资源,以便确保企业的正常运作,是当前所有IT部门面临的主要问题。
政府机构、企业组织对信息技术和系统的依赖性日益加强,IT 系统和业务应用的相互促进和融合,IT 管理走向面向服务的治理,是未来信息部门发展的发展核心。
借助IT 规范管理体系和最佳实践方法的指导,更好的融合业务、管理、技术三者并同步提高,才能让信息部门抓住这次机遇,提升自己的潜在价值,驱动业务的快速发展。
银讯IT安全运维管理平台是通过归纳总结各行业IT运维管理需求,经过三年研发而成的综合IT运维管理系统。
系统以网络管理和运维流程为基点,为IT部门提供全面的企业级解决方案。
通过此系统,在技术上对网络设备集中地进行性能采集和故障预警,大大减少了企业IT人员的日常工作量;在管理上,对日常运维工作进行规范化,合理化,提高决策的科学性。
二、市场定位银讯IT安全运维管理平台面对的客户群为信息化程度比较高、日常IT 运维管理相对比较规范的政府行业、大中型企业等。
中国信通院 企业it运维发展白皮书
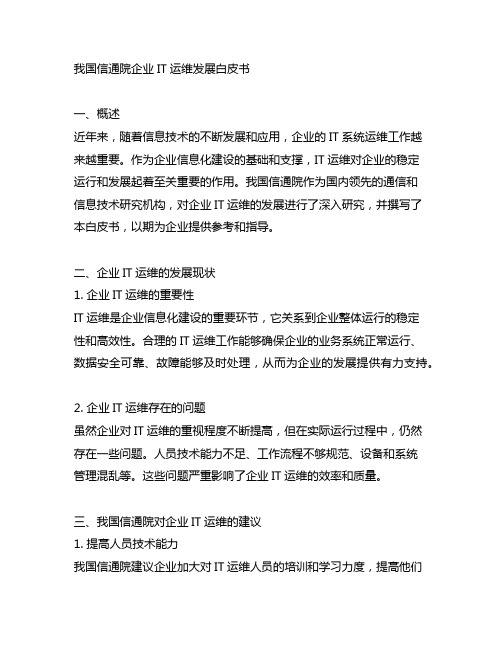
我国信通院企业IT运维发展白皮书一、概述近年来,随着信息技术的不断发展和应用,企业的IT系统运维工作越来越重要。
作为企业信息化建设的基础和支撑,IT运维对企业的稳定运行和发展起着至关重要的作用。
我国信通院作为国内领先的通信和信息技术研究机构,对企业IT运维的发展进行了深入研究,并撰写了本白皮书,以期为企业提供参考和指导。
二、企业IT运维的发展现状1. 企业IT运维的重要性IT运维是企业信息化建设的重要环节,它关系到企业整体运行的稳定性和高效性。
合理的IT运维工作能够确保企业的业务系统正常运行、数据安全可靠、故障能够及时处理,从而为企业的发展提供有力支持。
2. 企业IT运维存在的问题虽然企业对IT运维的重视程度不断提高,但在实际运行过程中,仍然存在一些问题。
人员技术能力不足、工作流程不够规范、设备和系统管理混乱等。
这些问题严重影响了企业IT运维的效率和质量。
三、我国信通院对企业IT运维的建议1. 提高人员技术能力我国信通院建议企业加大对IT运维人员的培训和学习力度,提高他们的技术能力和服务意识。
只有拥有一支高素质的IT运维团队,企业的IT系统才能得到有效保障。
2. 规范IT运维流程规范的运维流程是确保IT系统正常运行的基础。
我国信通院提倡企业建立完善的IT运维管理制度,明确各项工作的责任和流程,保障运维工作的有序进行。
3. 部署先进的运维工具在IT运维过程中,合适的工具和系统对提高工作效率和质量至关重要。
我国信通院建议企业积极引进和使用先进的运维工具,提高系统监控、故障分析和处理的能力。
4. 加强设备和系统管理设备和系统是IT运维的基础,对其进行有效的管理能够提高IT系统的稳定性和可靠性。
我国信通院建议企业加强对设备和系统的管理,定期检查和维护,保证其正常运行。
四、结语企业IT运维的发展是一个系统工程,需要全面的考虑和有效的措施。
我国信通院将继续深入研究和探讨企业IT运维的相关问题,为企业提供更多的指导和支持。
自动化运维管理解决方案--白皮书

自动化运维管理解决方案目录1IT运维管理面临挑战 (3)2应运而生的自动化解决方案 (5)3自动化应用场景 (7)3.1灾备切换自动化 (7)3.2故障现场快照 (8)3.3批量设备操作处理 (8)3.4周期性作业调度 (9)3。
5应急处理流程 (10)3。
6重要配置备份、基线比对 (10)4产品简介 (12)4.1运维脚本集中管理 (12)4.2可视化流程配置引擎 (12)4.3作业流程人工干预 (13)4.4作业执行验证/持续监控 (13)4.5作业操作手册自动生成 (13)4.6作业执行结果展现 (14)4。
7配置备份/基线库管理 (14)5产品优势 (16)6运行环境 (17)1 IT 运维管理面临挑战 24%31%45%IT 运营费用比例新系统开发维护开发运维管理⏹ 分散于各服务器上的运维脚本,存在管理风险,且耗费大量管理成本;⏹ 日常操作消耗大量人力资源,误操作风险较大,操作执行效率低;➢操作过程可控度低,运维风险大:⏹操作与执行方案匹配度无法保证,实际操作过程可控度较低;⏹日常操作对人员水平要求高,人力资源风险大;➢运维操作透明度低:⏹实际操作不便于监督,存在“黑盒”操作风险;⏹日常工作与实际操作无法有效关联,不利于日后审计;2应运而生的自动化解决方案面对IT运维管理中的诸多问题,单靠人工已经无法满足在技术、业务等方面的要求,那么标准化、自动化、架构优化、过程优化等降低IT服务成本的因素越来越被人们所重视.其中,IT运维自动化是指将IT运维中日常的、大量的重复性工作自动化,把过去的手工执行转为自动化操作.自动化是IT运维工作的升华,IT 运维自动化不单纯是一个维护过程,更是一个管理的提升过程,是IT运维的最高层次,也是未来的发展趋势.IT运维自动化从诞生发展至今,其重要属性之一已经不仅仅只是代替人工操作,更重要的是深层探知和全局分析,关注的是在当前条件下如何实现性能与服务最优化,同时保障投资收益最大化。
Viavi Solutions 移动网络自动化优化性能白皮书说明书

White PaperMobile Networks:Automation forOptimized PerformanceMobile networks are becoming increasingly important worldwide as people transition to a more transient lifestyle. People now use mobile networks to work remotely, stream video, and access social media applications. Soon, mobile networks will play a major role in areas such as the Internet of Things (IoT), cloud computing, and vehicle communication.This dependency on mobile networks has increased Quality of Experience (QoE) pressures on service providers at a time when bandwidth demands are also at an all-time high. How can service providers keep up with bandwidth needs and keep QoE at high levels?Service providers are doing their best to meet these demands by making macro level adjustments to networks to achieve incremental improvements in performance. But this has come at a cost. Service providers are seeing profits decline as more money and staff are needed to keep networks running in this new, complex environment. Even with the increase in operating expenditures (OpEx), traditional network optimization is not enough to keep up with the dynamic nature of today’s network traffic.What is needed is a way to automate network performance to create major leaps in optimization on a granular level, while also decreasing OpEx and freeing up staff to maintain the infrastructure and plan for expanding the network to deliver greater capacities. Major advancements have been made in recent months to make automated optimization a reality. Let’s take a closer look at the limitations of current network optimization methods, how automated optimization can overcome these limitations, and how this new method of optimizing networks can create a strategic advantage for service providers when the time comes to deploy 5G.Challenges Facing NetworksAs mobile networks continue to evolve, there are three main challenges that service providers face: interdependency, non-uniformity, and complexity. Each is a problem on its own, but together they create a network environment that is nearly impossible to optimize using traditional methods.Many of the metrics used to optimize networks are now interdependent. Changing a parameter, or parameters, to enhance the characteristics in one part of the network can have implications on other characteristics in other parts of the network. For instance, trying to increase data throughput in a certain area could affect voice traffic – either positively or negatively – in the network.This could also have a detrimental effect on design. Current designs that focus on one Key Performance Indicator (KPI) differ from designs that focus on other KPIs. This means that designs that focus on a specific KPI in isolation may or may not be the right choice for the overall performance of the network – especially as networks become increasingly non-uniform.Extreme non-uniformity is the new normal for mobile networks as regular users become power users and the overall subscriber population becomes more mobile. According to the VIAVI Mobile Data Trends report, 50 percent of data is consumed by only one percent of users. In addition, 50 percent of data is consumed in less than one percent of a network area, and this area is constantly changing. This change can be dramatic. In extreme cases,the amount of data that a cell is expected to support can increase by orders of magnitude over a period of a few minutes.This last data point is an important one. Not only has it become increasingly difficult to optimize networks because of non-uniformity, the non-uniformity is now dynamic. As this trend continues to grow, it will make it impossible to manually optimize networks in the future as this method cannot keep pace with the dynamic changes taking place. This leads to the overall problem with optimizing mobile networks: complexity. Not only are subscribers using networks in new and dynamic ways, technologies such as L TE, VoL TE, and heterogeneous networks (HetNets) have added layers of complexity that mean that changes to a network layer will not only change how that layer responds to the traffic it must convey, but it will also change the way that layer interacts with other layers. For example, changing an L TE layer may make it more or less attractive at a given location to traffic on the 3G network, and vice-versa.The number of tunable parameters is now enormous. For example, tuning just two parameters on each of 100 cells – where each parameter has 10 possible values – creates 10200 different ways these cells could be configured. That’s more than the number of atoms in the observable universe!Limitations of Network-Centric OptimizationThe three main challenges put a spotlight on the limitations of current optimization methods. While networks have become increasingly complex and dynamic, most optimization efforts are still primarily network-centric: a problem is located using network statistics and then adjustments are made to the network parameters to solve the problem. This network-centric approach of characterizing a problem using network statistics and then making macro site parameter adjustments no longer works when optimization is needed on a more granular level. This approach is also less effective when the intention is to change the configuration such that the performance is improved, rather than solve a specific problem.T aking this a step further, most macro-based adjustments create and maintain a baseline for overall network performance, but do little to optimize performance for specific locations within the network at any given time. For example, workers based in an office might tend to use voice services during the morning but then leave their office during lunch hour and go outside. While outside, their usage might migrate away from voice to data services. This illustrates the changing nature of the services demanded from the network and where they need to be delivered. An effective optimization would have to configure the network to deliver an acceptable user experience for this cohort of users, not just during the work hours and lunch break, but also during the commute time, evenings,and weekends. At each of these times the usage profile will be different and the locations will generally change. T aking automation to the limit sees the network able to adapt its configuration as the day progresses in response to the changes in the demands placed on it.But current optimization methods can only see macro locations based on overall network metrics. This creates “blind optimization” where multiple types of users at the various locations around the network are blended into one as the network tries to optimize an entire area. Doing so creates an imbalance where some users will have more resources than they need, while others will experience impaired usability.Another limitation is the iterative approach toward optimization – making small, incremental changes over time – due to the inter-dependent nature of today’s networks. This ensures that changes will not have an adverse effect on the network, but it also means that improvements are small with no major step changes in optimization. Most of these changes are use case driven and analyzed in isolation. If there is a problem with VoL TE performance for instance, current methods typically try to solve the problem in isolation without considering how it will affect other parameters such as data performance or energy consumption.Drive testing is often used in network optimization. However, drive testing uses synthetic data and is OpEx heavy. It can also take a considerable amount of time and effort to come to a network design optimized for the drive test traffic rather than the commercial users of the network.Most of all, today’s network-centric methods only focus on the network itself and have limited ability to measure or enhance the subscriber experience of using a network.Benefits of Automated Subscriber-Centric OptimizationNew methods of optimization take the focus from the network to the subscriber. Subscriber-centric optimization considers where subscribers are located, how are they using the network, and what their current QoE is at any given time. But what must happen behind the scenes to make this happen?Several advancements have made subscriber-centric optimization possible. Solutions can now collect, locate, store, and analyze data from mobile connection events, creating a repository of location intelligence from all subscribers throughout a network. This location intelligence is then transformed to deliver subscriber-centric performance engineering and Radio Access Network (RAN) planning information.Most recently, subscriber-centric performance has been taken one step further by automating network performance optimization. This new automated subscriber-centric optimization addresses the network challenges created by interdependency, non-uniformity and complexity, and can keep up with increasingly dynamic traffic patterns.Where traditional network optimization is a manual process and can take up to two weeks per site, automated optimization can optimize multiple sites at a time within hours rather than days. Where the focus of manual optimization must be a single site or a small group of sites, automated network optimization can focus on much larger clusters of hundreds of sites. Not only is the focus on larger clusters of sites possible with an automated approach, it is desirable since the exponential growth in possible parameterizations gives the optimization more scope to find configurations that maximize the performance for the mix of subscribers and applications in that region of the network. Once the area for optimization is selected, goals and success criteria are then established. KPI constraints and trade-off levels are then selected.The optimization task is then scheduled – typically processing tens of millions of events based on subscriber data with granular location intelligence. If the results create the intended improvement, the changes can be actuated into the network. The result is a fast turnaround with major step improvements in optimization without adversely affecting other parts of the network.Because this approach is automated, it also greatly reduces the staffing and OpEx needed to optimize a network.Engineers are typically able to turn around optimized designs for large areas in a very short time. In addition, automated subscriber-centric optimization directly maps revenue to QoE to keep service providers profitable and subscribers happy.In addition, the problems of interdependency and non-uniformity are overcome. Automated optimization can analyze KPIs in parallel and predict the impact of planned changes to make sure other parameters of the network will not be negatively affected. Algorithms calculate effects by predicting gains and the net costs of those gains to the network before any changes are made; and predictive decision making can resolve contradictions before they happen.This more proactive approach saves time and prevents subscribers from experiencing negative events that are common using traditional, reactionary optimization methods. As an added benefit, the ability to use granular data at the subscriber level also allows network optimization to prioritize specific subscriber groups such as VIPs or high-net individuals.In summary , traditional methods focus on network and synthetic data, are OpEx heavy , and take considerable effort and time to come to a conclusion that does not necessarily end up addressing the QoE and capacity issues. However, using subscriber-centric data ensures optimization is aligned with subscriber QoE, is OpEx light, and delivers network designs in a significantly shorter timeframe.Automated Subscriber-Centric Optimization in ActionAutomated optimization sounds good in theory , but does it work with real network traffic? Let’s look at a few real-life examples.A major mobile provider wanted to maximize data coverage and throughput by reducing the number of L TE data users on 3G. The goal was to improve data traffic volumes on an already optimized network while maintaining 3G voice service. The network had 233 cells across two Radio Network Controllers (RNCs).CollectReview areasDrive test prioritized sites Collect PM statsCollectSelect ClustersEstablish Goals & Constraints Schedule T askTIME = 1 HOUR AnalyzeOvershootersDrop Calls, Congestion, Load BalancingAnalyzeProcess Milllions of events Granular Subscriber Data Automatic analysisTIME = 1 HOURActuateOnce manually correlated Then fix all sectors that have the issuesActuateActuate optimization Design into the networkTIME = 1 HOUR ConfirmRe-drive problem areas Make final a djustments Check PM statsConfirmCompare results with predictionsTIME = 1 HOURTraditionalAutomated OptimizationAutomated optimization used subscriber-centric intelligence to analyze the current subscriber usage. Based on this intelligence, power changes were made to 67 cells, and 63 cells received antenna e-tilt changes. The result was a 1.3-point improvement in the L TE quality index and a 24 percent increase in data traffic volume – all without affecting 3G voice services. See diagram on left of page.Another service provider wanted to maximize retainability of VoL TE calls and improve VoL TE throughput while maintaining accessibility. They also wanted to make sure the changes wouldn’t impact data services. Automated subscriber-centric optimization maintained VoL TE accessibility at 99.82 percent while improvingVoL TE retainability from 97.48 percent to 98.03 percent. At the same time, the mean throughput improved by more than 13 percent. This was a major step change improvement without impacting data services. See diagram on right of page.Voice and data are not the only uses of automated optimization. Service providers can also use it to optimize energy consumption to reduce OpEx without affecting subscriber services.One service provider wanted to reduce energy consumption on their 3G network at major sites in a city while ensuring service availability. Automated optimization analyzed subscriber usage at key sites outside of normal hours and analyzed handset carrier capability. The solution also determined the optimal carrier configuration per site to optimize energy consumption while maintaining service levels. The result was a reduction in energy costs by 25 percent, saving the provider an estimated $2.4 million annually.These step changes in optimization were all possible because real subscriber-centric intelligence was being used instead of traditional synthetic data. This allowed the service providers to see what the true results would be once the changes were actuated. Automated optimization allows engineers to establish specific goals to optimize aspects such as capacity, throughput, service drops or energy savings. Service providers can also focus on a select set of parameters for the most cost effective improvements such as only changing power or e-tilt parameters.Automated Optimization and 5GSubscriber-centric automation will become even more important as mobile networks become more complex.An analysis of a number of third-party industry resources shows that networks will see several major changes by 2025:y 720 percent increase in video trafficy 700 Billion things will be connected to the Internet y 66 times increase in wireless traffic y 2000 times increase in cloud objectsy 620 times increase in data analyzed in the cloudFor mobile networks, service providers are looking to 5G to keep up with this changing demand. Although a lot of progress has been made, the standards for 5G have not been finalized. But the capabilities 5G must have to keep up with demand are staggering. According to the GSMA, 5G must accomplish: y 1G to 10G connections to end points in the field y Have 99.999 percent availability y Reduce energy usage by 90 percentA key characteristic of 5G is the expectation that it will be able to deliver connectivity to an even wider range of devices than are seen today. This will include public safety , and a plethora of Io T devices such as connected cars, smart meters and asset trackers. These devices will have a vast range of different requirements in terms of bandwidth, latency , jitter, reliability , and dynamics that will require a network to tailor the service to each set of subscribers and devices. The specific requirements for each group further compounds the problem of network-centric optimization as it’s unable to discern the impact on each device and how it needs to change to meet QoE targets.There will also be a trend towards RAN centralization and virtualization with the functionality of a traditional base station being split between centralized units and distributed units. In many cases these will need to be configured, managed and optimized in the context of their topology and transport constraints, and the subscribers they are serving. Advanced, coordinated radio transmission and reception schemes will be available which will provide better resilience to adverse radio conditions such as poor coverage and interference, but will come at a cost by placing more demands on the transport network.10 10 10101010WIRELESS FIBER© 2017 VIAVI Solutions Inc.Product specifications and descriptions in this document are subject to change without notice.mobilenetworks-wp-maa-nse-ae 30186254 900 1017Contact Us +1 844 GO VIAVI (+1 844 468 4284)To reach the VIAVI office nearest you, visit /contacts.The advent of 5G will also bring more use of Network Function Virtualization (NFV) and Software Defined Networks (SDN) to deliver network infrastructure. This will also require configuration, management andoptimization. Other inflections such as Mobile Edge Computing will mean that functionality can be distributed and configured to meet constraints such as service latency and usage of transmission bandwidth.5G will need to coexist and interwork with older technologies such as 2, 3 and 4G. Networks will gain another layer that must work optimally with the older technologies so that devices are still able to achieve their QoE targets. Any system that automates network optimization must perform effectively by taking advantage of all the layers, managing the selection of each layer, and transitions between them such that it sweats the assets and drives performance.T aken together, these various developments make tomorrow’s network more powerful by allowing devices more ways to achieve their various QoE needs. But this also creates a problem for management and optimization since there will be many more parameters to tune, the number of possible configurations explodes exponentially , and finding the optimal configurations becomes much harder.The other impact of this increased configurability is the interdependency between different parts of the network. If changes are made in the RAN to address an interference problem, this may change the backhaul demands on a network. This issue is further compounded as some subscribers may derive service from different cells. The relationship between a 5G network and the 2/3/4G layers may change as subscribers derive a service from these other layers in addition to – or instead of – the 5G layer. In addition, more devices may be attracted to the 5G layer. The network load could change as a result and place more demands on virtualized core elements. Any optimization solution must be able to consider the holistic impact of configuration changes that are under consideration, as well as their ability to deliver the variety of QoE required by the different devices. Doing this effectively in the complex and configurable network will require advanced modelling of radio, RAN, transport and core elements along with mature configuration optimization capability to optimize the infrastructure and spectrum assets while delivering the required service.The only way for this to happen is to automate optimization using subscriber-centric methods as a starting point and then add more automated features as they become available. Eventually , networks will need to have the capabilities of self-configuration, self-optimization and self-healing to keep up with subscriber demand and maintain a high level of QoE.This may sound like science fiction, but it must happen and time is not on the industry’s side. Currently , most service providers are planning mass deployments of 5G by 2020. Some service providers are already planning to make smaller deployments in 2018 and 2019. This means that automated subscriber-centric optimization is not a “nice to have” feature, but a vital step toward future networks. It’s the only way service providers will be able to keep up with the complexity of networks and the dynamic traffic patterns of the future.。
企业AIOps智能运维方案白皮书
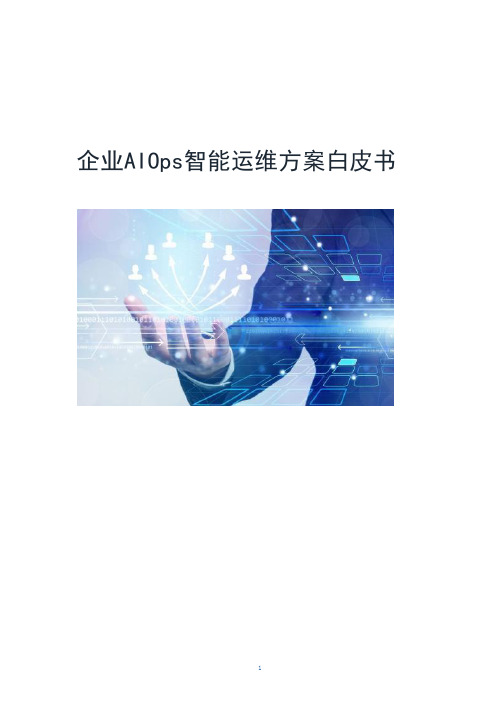
企业AIOps智能运维方案白皮书目录背景介绍4组织单位4编写成员5发起人5顾问5编审成员5本版本核心编写成员61、整体介绍82、AIOps 目标103、AIOps 能力框架114、AIOps 平台能力体系145、 AIOps 团队角色17 5.1 运维工程师17 5.2 运维开发工程师175.3 运维 AI 工程师176、AIOps 常见应用场景19 6.1 效率提升方向216.1.1 智能变更226.1.2 智能问答226.1.3 智能决策236.1.4 容量预测23 6.2 质量保障方向246.2.1 异常检测246.2.2 故障诊断256.2.3 故障预测256.2.4 故障自愈26 6.3 成本管理方向266.3.1 成本优化266.3.2资源优化276.3.3容量规划286.3.4性能优化287、AIOps 实施及关键技术29 7.1数据采集29 7.2数据处理30 7.3数据存储30 7.4离线和在线计算30 7.5面向 AIOps 的算法技术30说明:31附录:案例33案例1:海量时间序列异常检测的技术方案331、案例陈述332、海量时间序列异常检测的常见问题与解决方案333、总结34案例2:金融场景下的根源告警分析351、案例概述352、根源告警分析处理流程353、根源告警分析处理方法374、总结39案例3:单机房故障自愈压缩401、案例概述402、单机房故障止损流程403、单机房故障自愈的常见问题和解决方案414、单机房故障自愈的架构435、总结44背景介绍AIOps 即智能运维,其目标是,基于已有的运维数据(日志、监控信息、应用信息等),通过机器学习的方式来进一步解决自动化运维所未能解决的问题,提高系统的预判能力、稳定性、降低 IT 成本,并提高企业的产品竞争力。
Gartner 在 2016 年时便提出了 AIOps 的概念,并预测到 2020 年,AIOps 的采用率将会达到 50%。
IT运维管理平台产品技术白皮书
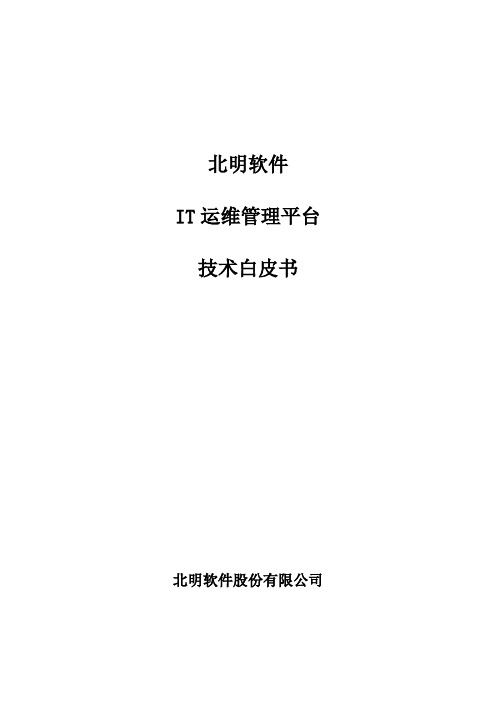
运维 白皮书
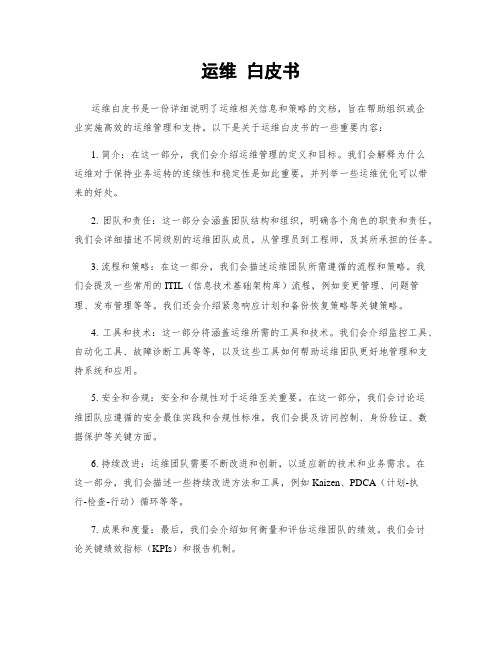
运维白皮书运维白皮书是一份详细说明了运维相关信息和策略的文档,旨在帮助组织或企业实施高效的运维管理和支持。
以下是关于运维白皮书的一些重要内容:1. 简介:在这一部分,我们会介绍运维管理的定义和目标。
我们会解释为什么运维对于保持业务运转的连续性和稳定性是如此重要,并列举一些运维优化可以带来的好处。
2. 团队和责任:这一部分会涵盖团队结构和组织,明确各个角色的职责和责任。
我们会详细描述不同级别的运维团队成员,从管理员到工程师,及其所承担的任务。
3. 流程和策略:在这一部分,我们会描述运维团队所需遵循的流程和策略。
我们会提及一些常用的ITIL(信息技术基础架构库)流程,例如变更管理、问题管理、发布管理等等。
我们还会介绍紧急响应计划和备份恢复策略等关键策略。
4. 工具和技术:这一部分将涵盖运维所需的工具和技术。
我们会介绍监控工具、自动化工具、故障诊断工具等等,以及这些工具如何帮助运维团队更好地管理和支持系统和应用。
5. 安全和合规:安全和合规性对于运维至关重要。
在这一部分,我们会讨论运维团队应遵循的安全最佳实践和合规性标准。
我们会提及访问控制、身份验证、数据保护等关键方面。
6. 持续改进:运维团队需要不断改进和创新,以适应新的技术和业务需求。
在这一部分,我们会描述一些持续改进方法和工具,例如Kaizen、PDCA(计划-执行-检查-行动)循环等等。
7. 成果和度量:最后,我们会介绍如何衡量和评估运维团队的绩效。
我们会讨论关键绩效指标(KPIs)和报告机制。
通过运维白皮书的指导,组织和企业可以建立健全的运维管理框架,并提高效率、降低风险、提供更稳定的服务。
这样的白皮书不仅可以帮助运维团队更好地组织和管理工作,也可以为其他团队和利益相关者提供清晰的指导和了解。
- 1、下载文档前请自行甄别文档内容的完整性,平台不提供额外的编辑、内容补充、找答案等附加服务。
- 2、"仅部分预览"的文档,不可在线预览部分如存在完整性等问题,可反馈申请退款(可完整预览的文档不适用该条件!)。
- 3、如文档侵犯您的权益,请联系客服反馈,我们会尽快为您处理(人工客服工作时间:9:00-18:30)。
运维自动化平台白皮书
目录
一、概述 (3)
二、功能介绍 (3)
1.平台整体功能 (3)
2.安装部署 (4)
3.配置更新 (4)
4.任务执行 (4)
5.监控报警 (5)
6.巡检管理 (5)
三、技术特点 (6)
1.Python语言开发 (6)
2.融合云计算平台 (6)
3.规则知识库 (6)
4.标准RESTful API (6)
5.运维控制台 (6)
一、概述
本产品为运维自动化平台,集安装部署、配置更新、任务执行、监控报警、巡检管理等功能为一体,将运维管理员的经验和运维工具有效的结合,引入丰富的运维规则库,辅助管理员完成日常运维工作。
运维自动化平台立足于传统的数据中心架构,也能更好的支持Openstack 等框架下的私有云平台和公有云平台,做到传统运维和云运维的结合。
其设计原则是“平台化、模块化、松耦合、全开放”,以平台化、模块化实现工具集成、功能聚合,改变原有运检工具分散独立运行的现状,将运维工作全部整合在统一的平台中,并且各模块均提供标准化接口,满足模块化、松耦合的原则,可以与其他系统的功能模块方便地集成;其核心是从配置管理着手,配合监控工具,对各类应用系统进行从基础资源的部署到应用发布,再到运行维护的全生命周期的管理,最终实现运维的自动化、可视化、智能化。
二、功能介绍
1.平台整体功能
(1) 权限管理
目前的权限管理主要指对平台的普通用户可使用的运维功能模块进行管理,由管理员统一进行权限的管理。
如用户A只拥有安装部署的权限,则其他的权限对用户A来说是隐藏的。
(2) 用户管理
管理员对平台的普通用户进行增加、修改和删除的操作,也可以由使用者自己注册平台用户,并申请权限。
注册功能可以启用或者禁用。
(3) 通知管理
用户可以接收到平台运行中发生较严重的事件,在平台使用界面的菜单栏中可以查看。
(4) 规则库管理
平台中的每个模块都需要建立规则库,以支撑运维操作的执行。
目前规则库分散到各个模块中独立管理。
2.安装部署
本功能主要分为两部分,一是实现对物理机的操作系统的推送和自动化安装,二是实现在目标操作系统上实现对中间件、数据库及其他软件的自动化安装、更新及卸载。
平台可以自动发现需要安装操作系统的物理服务器,然后再根据预先在模板库中定义的系统镜像来安装操作系统,支持主流的操作系统,如Ubuntu、Redhat、CentOS等。
安装软件需要从“软件商店”中选择,将软件和主机进行关联,实现对应操作系统的软件的安装及卸载。
安装方式支持从软件源中安装,也支持自定义软件包安装。
对传统的物理环境、Openstack私有云以及公有云提供全面的支撑,有效减少企业内部基础设施环境的差异化。
3.配置更新
平台提供丰富的配置更新管理功能,实现自动化修改目标主机的配置文件并使之生效。
支持操作系统的配置文件,例如:hosts;也支持应用程序的配置文件,例如:sshd_config。
配置更新包括两个角度的实现:配置参数的修改以及配置文件的替换。
配置参数的修改可以对多数的配置文件进行修改,完成参数的新增、更新以及删除;而复杂格式的配置文件目前只能通过配置文件的替换完成,该功能将替换掉原有的配置文件。
4.任务执行
统一运维自动化平台能通过简单的操作在平台上完成对指定节点的命令行或脚本任务的创建与执行。
任务执行模块主要分为三个子模块完成相应的功能,分别是:规则库、运行记录与统计分析。
用户在规则库中自定义名称、规则描述和需要执行的脚本命令,定义好后在平台上创建一个任务,选择指定的节点和指定的脚本模板,提交后后端程序立即执行或周期性执行任务,执行结束后平台会将任务执行的结果和自动化处理的结果反馈给用户。
用户在查询任务执行结果时,能鲜明的查看到结果与对应的脚本详情,包
括名称、状态、命令、节点和创建时间等,并且,如果一个脚本对多个节点执行,平台可以让用户选择查看全部结果或者是单条结果,单条结果即为某一个节点上的任务执行情况,可以根据用户的需求进行相应的选择。
在任务执行模块,每一个任务在执行后,后端都会对其进行统计分析,平台会实时将所有的任务执行的数量情况,以天为单位,反映到折线图上,用户可以在网页上随时进行查看与分析。
5.监控报警
运维自动化平台采集服务器节点的性能数据和状态数据,以及数据库、中间件等的运行数据,生成监控图表,并根据预设策略,以短信、邮件方式发出报警信息。
对数据的监控,首先需要定义监控规则、监控项及监控模板。
监控项定义了需要采集的监控数据,在定义的过程中需要设置一些属性。
监控模板定义了监控项的集合。
对监控功能的管理,包括管理服务器节点的监控项,监控模板及触发器,可以关闭对某个节点的监控功能。
当应用监控项后,就可以采集节点上这个监控项的数据。
监控模板采集节点上多个监控项的数据。
触发器可以根据监控项的数据在某种情况下触发事件。
平台还需要维护报警策略和报警对象。
报警策略的定义,主要通过与触发器的关联来实现。
告警方式包括短信告警和邮件告警,需要有邮件网关和短信网关,才能发出告警信息。
报警对象包括联系人及联系人组。
6.巡检管理
对主机的巡检管理,获取巡检对象的状态和性能数据,对系统的运行状态进行检查,并生成巡检报告。
包括以下内容:
巡检规则。
用户在执行巡检任务前,需要自定义巡检规则,即如何对软件或硬件进行巡检。
比如定义巡检的项目和阈值、巡检的策略、需要配置部署的软件等。
规则是由脚本组成,支持shell脚本和python脚本。
巡检任务制定。
巡检任务分为手动执行和自动执行两种。
手动巡检在创建完巡检任务之后立即执行,而自动巡检在巡检执行周期自动执行,用户可以根据需要灵活设定。
巡检报告。
手动巡检和自动巡检在结束后将巡检结果存储在数据库中,用户可以在界面中查看到结果,并输出巡检报告。
三、技术特点
1.Python语言开发
平台的前端和后端统一采用Python语言进行开发。
严格来说,Python是一门脚本语言,但由于其拥有非常多的模块以及优秀的Web框架,使其成为设计开发运维平台的首选。
2.融合云计算平台
本产品在开发技术选择上和架构的设计上,考虑了与Openstack、Cloudstack等目前流行的开源云计算平台的融合,可以很好的支持云平台上的虚拟主机的自动化运维工作,可以将资源申请和运维工作更加的流程化、自动化。
3.规则知识库
运维平台需要维护规则知识库,将运维人员的实际运维经验总结成规则知识库,并通过自动化的方式,完成运维操作。
规则知识库以Shell脚本或Python脚本的方式规定了实际动作,如通过哪种方式完成软件的安装,怎么收集巡检数据等。
随着平台使用的深入以及知识库中规则的积累,运维自动化平台的运行会更加的稳定,功能会得到更多的实现。
4.标准RESTful API
平台提供的运维功能均提供标准的RESTful API,方便与其他系统进行集成,以及做二次开发工作。
5.运维控制台
运维自动化平台的控制台采用与开源云计算平台Openstack相同的技术,即Python的Django框架、Bootstrap样式和AngularJS库实现,充分考虑运维人员的操作习惯和方式,。