卫星通信的信道测量和建模
面向卫星通信的信道建模与误码率分析研究
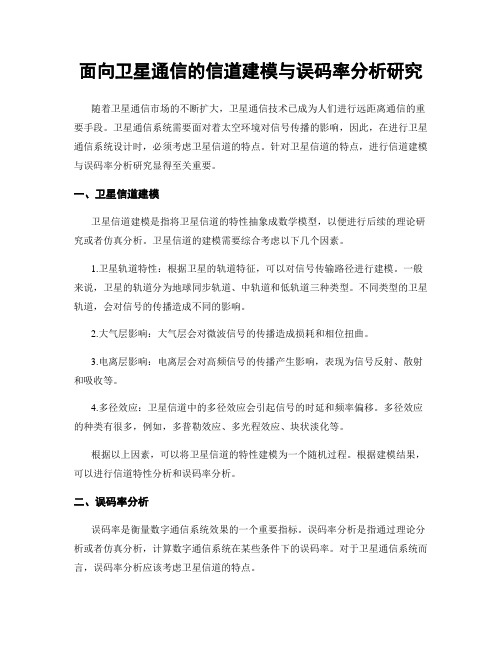
面向卫星通信的信道建模与误码率分析研究随着卫星通信市场的不断扩大,卫星通信技术已成为人们进行远距离通信的重要手段。
卫星通信系统需要面对着太空环境对信号传播的影响,因此,在进行卫星通信系统设计时,必须考虑卫星信道的特点。
针对卫星信道的特点,进行信道建模与误码率分析研究显得至关重要。
一、卫星信道建模卫星信道建模是指将卫星信道的特性抽象成数学模型,以便进行后续的理论研究或者仿真分析。
卫星信道的建模需要综合考虑以下几个因素。
1.卫星轨道特性:根据卫星的轨道特征,可以对信号传输路径进行建模。
一般来说,卫星的轨道分为地球同步轨道、中轨道和低轨道三种类型。
不同类型的卫星轨道,会对信号的传播造成不同的影响。
2.大气层影响:大气层会对微波信号的传播造成损耗和相位扭曲。
3.电离层影响:电离层会对高频信号的传播产生影响,表现为信号反射、散射和吸收等。
4.多径效应:卫星信道中的多径效应会引起信号的时延和频率偏移。
多径效应的种类有很多,例如,多普勒效应、多光程效应、块状淡化等。
根据以上因素,可以将卫星信道的特性建模为一个随机过程。
根据建模结果,可以进行信道特性分析和误码率分析。
二、误码率分析误码率是衡量数字通信系统效果的一个重要指标。
误码率分析是指通过理论分析或者仿真分析,计算数字通信系统在某些条件下的误码率。
对于卫星通信系统而言,误码率分析应该考虑卫星信道的特点。
误码率分析需要进行以下几个步骤。
1.信道建模:通过信道建模,可以得到信道的特点,包括信噪比、多径效应、相位噪声等。
2.传输系统建模:根据传输系统的特点,包括编码方式、调制方式、发射功率等,对数字通信系统进行建模。
3.误码率分析方法:根据误码率分析的目的和需求,选择相应的误码率分析方法。
4.误码率计算:根据所选择的误码率分析方法,进行误码率计算。
误码率可以用公式计算,也可以用仿真方法计算。
在误码率分析中,一般会针对不同的信噪比、多径效应等因素,进行误码率曲线的绘制。
面向卫星通信的信道建模与传输优化方法研究
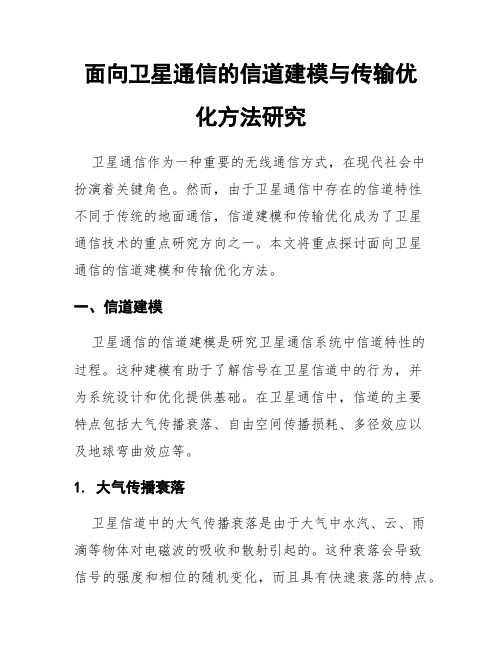
面向卫星通信的信道建模与传输优化方法研究卫星通信作为一种重要的无线通信方式,在现代社会中扮演着关键角色。
然而,由于卫星通信中存在的信道特性不同于传统的地面通信,信道建模和传输优化成为了卫星通信技术的重点研究方向之一。
本文将重点探讨面向卫星通信的信道建模和传输优化方法。
一、信道建模卫星通信的信道建模是研究卫星通信系统中信道特性的过程。
这种建模有助于了解信号在卫星信道中的行为,并为系统设计和优化提供基础。
在卫星通信中,信道的主要特点包括大气传播衰落、自由空间传播损耗、多径效应以及地球弯曲效应等。
1. 大气传播衰落卫星信道中的大气传播衰落是由于大气中水汽、云、雨滴等物体对电磁波的吸收和散射引起的。
这种衰落会导致信号的强度和相位的随机变化,而且具有快速衰落的特点。
研究人员可以使用统计方法对大气传播衰落进行建模,例如使用纯对数正态分布或韦布尔分布来描述衰落的统计特性。
2. 自由空间传播损耗自由空间传播损耗是指信号由卫星发射到地球接收点时经过的自由空间中的损耗。
这种损耗与卫星与接收点之间的距离成正比,其表达式为L=32.44+20log(d)+20log(f),其中L为损耗(dB),d为距离(km),f为频率(GHz)。
在信道建模中,可以使用这个公式来计算信号的损耗。
3. 多径效应多径效应是指信号在传播过程中由于经历了多个路径引起的多次反射、衍射和散射,从而导致信号到达接收点时发生时延和相位畸变等问题。
针对多径效应,可以使用雷克空间CORASAN模型或尺度HATA模型等来进行建模。
4. 地球弯曲效应地球弯曲效应是指信号在经过地球曲率时发生的衰落和有效面积减小现象。
在信道建模中,可以通过考虑地球曲率来模拟这种效应。
二、传输优化方法在对卫星通信信道进行建模之后,研究人员可以基于模型提出一系列传输优化方法来改善卫星通信系统的性能。
1. 编码技术优化在卫星通信中,编码技术是一种常用的传输优化方法。
通过引入纠错码或调制码,可以提高信道的可靠性和抗干扰性。
信道测量与建模技术研究及其应用

信道测量与建模技术研究及其应用英文回答:Channel Measurement and Modeling Techniques for Wireless Communications.Channel measurement and modeling are crucial aspects of wireless communications, providing the foundation for understanding and predicting signal propagation characteristics in various environments. By accurately characterizing the channel, researchers and engineers can design and optimize communication systems to achieve reliable and efficient data transmission.Measurement Techniques:Over-the-Air (OTA) Measurements: Conducted in real-world environments to collect channel impulse responses (CIRs) and other channel parameters. OTA measurements are essential for capturing the effects of path loss, fading,and multipath.Channel Sounders: Specialized equipment that generates and transmits probe signals to measure channel characteristics. Channel sounders provide precise measurements with controlled parameters, allowing for extensive data analysis.Software-Defined Radios (SDRs): Software-based radios that can be programmed to perform channel measurements. SDRs offer flexibility and versatility, enabling the customization of measurement setups and parameter configurations.Modeling Techniques:Deterministic Models: Use physical principles to calculate channel parameters, such as path loss and shadowing. These models are typically simple and computationally efficient but may not fully capture the complexities of real-world channels.Stochastic Models: Based on statistical distributionsto represent channel variations. Stochastic models can provide a more realistic representation of fading and multipath effects but require more complex computations.Machine Learning (ML)-Based Models: Utilize artificial intelligence algorithms to learn channel characteristics from measured data. ML models offer the ability to adapt to changing environments and provide accurate predictions without the need for explicit mathematical formulations.Applications:Network Planning and Optimization: Channel measurements and models help in determining the optimal placement of base stations, improving coverage and capacity.Signal Processing Algorithms: Channel models guide the development of signal processing algorithms, such as equalization and beamforming, to enhance signal quality and mitigate interference.Wireless Device Design: Channel characterization aids in designing wireless devices with optimal antennas and power control mechanisms to adapt to different propagation environments.Spectrum Regulation: Channel models inform spectrum allocation decisions, ensuring efficient utilization and preventing interference between different services.Conclusion:Channel measurement and modeling techniques play a vital role in understanding and improving the performance of wireless communication systems. By accurately characterizing the channel, researchers and engineers can develop optimization strategies, design algorithms, and innovate device technologies to meet the demands of next-generation wireless networks.中文回答:信道测量与建模技术及其应用。
无线通信中的信道建模与信道估计研究
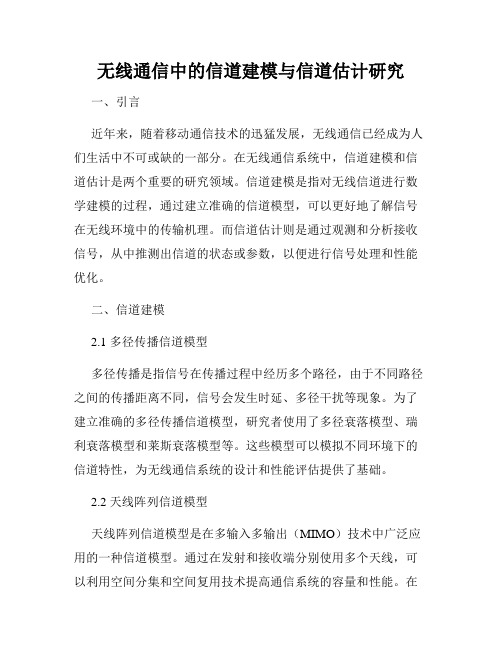
无线通信中的信道建模与信道估计研究一、引言近年来,随着移动通信技术的迅猛发展,无线通信已经成为人们生活中不可或缺的一部分。
在无线通信系统中,信道建模和信道估计是两个重要的研究领域。
信道建模是指对无线信道进行数学建模的过程,通过建立准确的信道模型,可以更好地了解信号在无线环境中的传输机理。
而信道估计则是通过观测和分析接收信号,从中推测出信道的状态或参数,以便进行信号处理和性能优化。
二、信道建模2.1 多径传播信道模型多径传播是指信号在传播过程中经历多个路径,由于不同路径之间的传播距离不同,信号会发生时延、多径干扰等现象。
为了建立准确的多径传播信道模型,研究者使用了多径衰落模型、瑞利衰落模型和莱斯衰落模型等。
这些模型可以模拟不同环境下的信道特性,为无线通信系统的设计和性能评估提供了基础。
2.2 天线阵列信道模型天线阵列信道模型是在多输入多输出(MIMO)技术中广泛应用的一种信道模型。
通过在发射和接收端分别使用多个天线,可以利用空间分集和空间复用技术提高通信系统的容量和性能。
在建立天线阵列信道模型时,需要考虑天线之间的耦合、阻塞和角度扩展等因素,以及天线阵列的位置和布局等参数。
三、信道估计3.1 参数估计方法在无线通信系统中,信道参数估计是一个关键的问题。
通过准确地估计信道参数,可以实现优化的信号处理和自适应调制等技术,提高通信系统的性能。
常用的信道参数估计方法包括最小二乘法、最大似然估计、卡尔曼滤波和粒子滤波等。
3.2 盲估计方法盲估计是在不需要已知训练序列的前提下,从接收信号中估计信道参数的一种方法。
在无线通信系统中,盲估计可以提高系统的灵活性和抗干扰能力。
常见的盲估计方法包括基于统计特性的方法、高阶统计量分析和神经网络等。
四、应用与挑战信道建模和信道估计在无线通信系统中具有重要应用。
在无线通信系统设计和性能评估中,准确的信道建模可以提供仿真和测试的基础。
而通过信道估计,我们可以实现自适应调制、均衡和功率控制等技术,进一步提高通信系统的性能。
卫星通信中的信道建模和性能分析研究
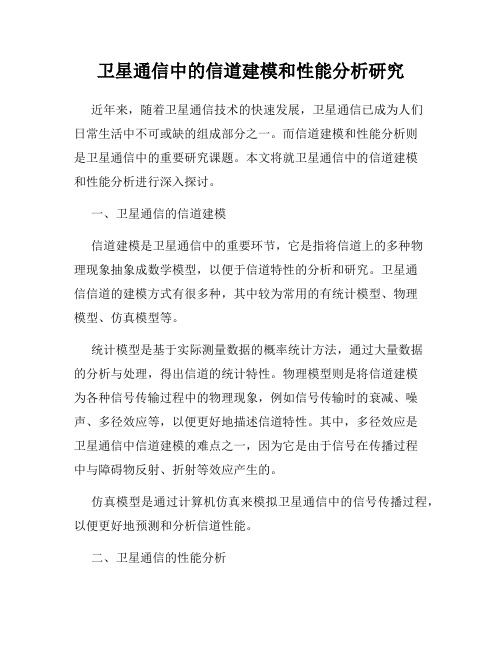
卫星通信中的信道建模和性能分析研究近年来,随着卫星通信技术的快速发展,卫星通信已成为人们日常生活中不可或缺的组成部分之一。
而信道建模和性能分析则是卫星通信中的重要研究课题。
本文将就卫星通信中的信道建模和性能分析进行深入探讨。
一、卫星通信的信道建模信道建模是卫星通信中的重要环节,它是指将信道上的多种物理现象抽象成数学模型,以便于信道特性的分析和研究。
卫星通信信道的建模方式有很多种,其中较为常用的有统计模型、物理模型、仿真模型等。
统计模型是基于实际测量数据的概率统计方法,通过大量数据的分析与处理,得出信道的统计特性。
物理模型则是将信道建模为各种信号传输过程中的物理现象,例如信号传输时的衰减、噪声、多径效应等,以便更好地描述信道特性。
其中,多径效应是卫星通信中信道建模的难点之一,因为它是由于信号在传播过程中与障碍物反射、折射等效应产生的。
仿真模型是通过计算机仿真来模拟卫星通信中的信号传播过程,以便更好地预测和分析信道性能。
二、卫星通信的性能分析性能分析是指对卫星通信中的信号传输过程进行定量和定性评估的过程,一般分为误码率、信噪比、传输速率等多个方面进行分析。
误码率是衡量卫星信道性能的重要指标之一,它指在信道传输中出现错误比例。
误码率越低,证明信道传输品质越好,即信号的正确传输率越高。
信噪比是指信号与噪声的比值,也是衡量信道质量的一个重要指标。
当信号传输时,由于信道中存在着各种噪声和干扰,因此信噪比越高,信号传输质量也就越好。
传输速率则是指在特定的信道条件下,通过信道传输数据的速度。
传输速率越快,则证明信道传输效率越高。
三、卫星通信的应用及未来发展卫星通信是目前最为广泛应用的通信技术之一。
它已经渗透到生活中的方方面面,例如航空、海洋、广播电视、电话、互联网等等。
而随着卫星技术的不断进步和卫星通信网络的不断完善,未来卫星通信将会在更多的领域得到应用。
例如,在互联网迅速发展的当下,卫星通信可以成为解决全球上网难题的重要手段,而在物联网方面,卫星通信将会成为物联网建设中不可或缺的技术手段。
卫星通信系统中的信道建模与性能优化研究

卫星通信系统中的信道建模与性能优化研究引言卫星通信系统作为一种重要的通信技术,广泛应用于航天、航海、气象、农业等领域。
在卫星通信系统中,信道建模与性能优化是一个重要的研究方向。
本文将对卫星通信系统中的信道建模和性能优化进行分析与探讨。
一、卫星通信系统的信道建模1.1 大气信道建模大气在卫星通信中扮演着重要的角色,影响着信号的传输和接收。
大气信道建模是描述大气对信号传输的影响的数学模型。
其中,常见的影响因素包括大气湍流、雨淋、电离层等。
1.2 干扰信道建模卫星通信系统中存在着各种干扰,如天线间干扰、卫星间干扰、地面干扰等。
对这些干扰信道的建模能够为系统的优化提供指导。
1.3 多径信道建模卫星通信信号在传播过程中会遇到多径效应,造成信号的衰落和时延扩展。
多径信道建模可以描述不同路径导致的信号衰落和时延扩展情况,从而优化系统性能。
二、卫星通信系统性能优化2.1 调制与编码技术优化调制与编码技术是卫星通信系统的核心技术,直接影响着系统的传输速率和误码率。
通过优化调制与编码技术,可以提高系统的抗干扰性能和误码纠正能力。
2.2 天线设计与指向优化天线是卫星通信系统的重要组成部分,天线设计直接影响着系统的覆盖范围和信号质量。
通过优化天线的设计与指向,可以提高系统的覆盖能力和信号接收强度。
2.3 功率控制与功率分配优化在卫星通信系统中,功率控制和功率分配是保证信号传输质量的重要措施。
通过优化功率控制与功率分配策略,可以提高系统的传输效率和频谱利用率。
2.4 路径选择与切换优化在卫星通信系统中,路径选择和切换对整个系统的性能有着重要影响。
通过优化路径选择与切换策略,可以提高系统的容错性和数据传输速率。
三、卫星通信系统的性能评估与验证为了验证卫星通信系统的性能优化效果,需要进行性能评估和验证实验。
常用的评估指标包括误码率、传输速率、频谱效率等。
通过实验数据的收集和分析,可以验证系统的性能优化效果,并对系统进行进一步优化。
Ka频段卫星通信信道建模及系统性能仿真

Ka频段卫星通信信道建模及系统性能仿真Ka频段卫星通信信道建模及系统性能仿真一、引言随着信息时代的到来,卫星通信作为一种重要的通信方式,为广大用户提供了广播电视、互联网接入、遥感监测等多种应用服务。
Ka频段卫星通信作为一种新颖的通信技术,具有更高的信道容量和更大的数据传输率,被广泛应用于高速互联网接入、高清视频传输等领域。
本文将探讨Ka频段卫星通信的信道建模及系统性能仿真。
二、Ka频段卫星通信信道建模1. Ka频段信道特点Ka频段信道通常采用高频段的Ka波段进行通信,工作频率在26.5-40GHz之间。
该频段信号传播损耗较大,受大气层影响较严重,同时也容易受到地面环境和建筑物的干扰。
因此,建立准确的信道模型对于系统设计和性能评估至关重要。
2. 大气传输损耗模型大气传输损耗是Ka频段卫星通信的主要性能制约因素之一。
根据大气传输损耗的特点,可以采用Beer-Lambert定律来建立信道模型。
该定律描述了光束在大气中传播时受到的自然衰减,其损耗与传输距离、大气透明度和气溶胶密度等因素有关。
3. 地面环境和建筑物干扰模型除了大气传输损耗,地面环境和建筑物对于Ka频段卫星通信也会产生干扰。
地面环境如建筑、树木等会阻碍信号传输,同时也会产生多径效应。
为了建立准确的信道模型,需要对不同地形和建筑物进行数值模拟,研究其对信号传输的影响。
三、Ka频段卫星通信系统性能仿真1. 信号传输质量评价在卫星通信系统中,信号传输质量是一个重要的指标,可以通过误码率(BER)来评估。
对于Ka频段卫星通信,由于其频率较高,信号传输过程中容易受到大气传输损耗和多径效应的影响,因此需要通过系统性能仿真来评估其信号传输质量。
2. 系统容量评估Ka频段卫星通信具有较高的信道容量,能够提供更多的数据传输率。
因此,通过仿真系统性能,可以评估系统的容量,确定系统能够支持的用户数量和数据传输速率。
3. 频谱利用率评估频谱是有限资源,如何充分利用频谱资源是卫星通信系统设计的关键问题。
卫星通信系统中的信道估计和增强技术研究
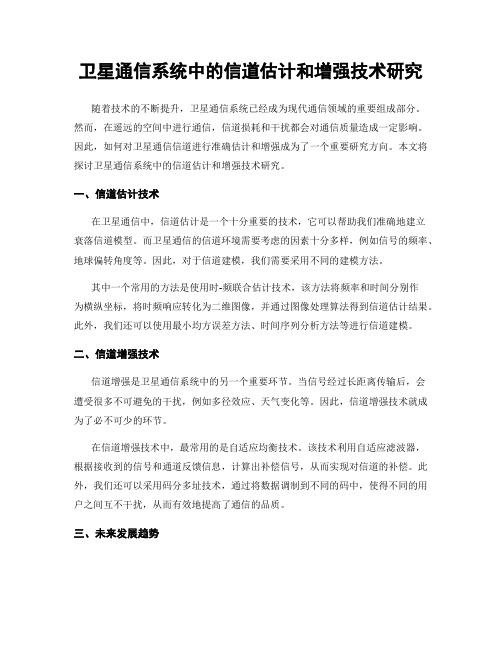
卫星通信系统中的信道估计和增强技术研究随着技术的不断提升,卫星通信系统已经成为现代通信领域的重要组成部分。
然而,在遥远的空间中进行通信,信道损耗和干扰都会对通信质量造成一定影响。
因此,如何对卫星通信信道进行准确估计和增强成为了一个重要研究方向。
本文将探讨卫星通信系统中的信道估计和增强技术研究。
一、信道估计技术在卫星通信中,信道估计是一个十分重要的技术,它可以帮助我们准确地建立衰落信道模型。
而卫星通信的信道环境需要考虑的因素十分多样,例如信号的频率、地球偏转角度等。
因此,对于信道建模,我们需要采用不同的建模方法。
其中一个常用的方法是使用时-频联合估计技术,该方法将频率和时间分别作为横纵坐标,将时频响应转化为二维图像,并通过图像处理算法得到信道估计结果。
此外,我们还可以使用最小均方误差方法、时间序列分析方法等进行信道建模。
二、信道增强技术信道增强是卫星通信系统中的另一个重要环节。
当信号经过长距离传输后,会遭受很多不可避免的干扰,例如多径效应、天气变化等。
因此,信道增强技术就成为了必不可少的环节。
在信道增强技术中,最常用的是自适应均衡技术。
该技术利用自适应滤波器,根据接收到的信号和通道反馈信息,计算出补偿信号,从而实现对信道的补偿。
此外,我们还可以采用码分多址技术,通过将数据调制到不同的码中,使得不同的用户之间互不干扰,从而有效地提高了通信的品质。
三、未来发展趋势作为一个技术领域,卫星通信的发展迅速。
近年来,随着5G技术的兴起和卫星通信技术的不断提升,卫星通信系统也越来越成为人们的关注重点。
未来,卫星通信领域将会进一步发展和创新,这也催生了许多新的发展趋势。
例如,随着人工智能技术的快速发展,卫星通信系统将会进一步优化和智能化,通过精准的预测和应对策略,提高系统的可靠性和灵活性。
此外,我们还可以采用更加高效的信道估计和增强技术,使用不同的信道模型进行分析和优化,从而提高卫星通信系统的工作效率和性能。
总之,卫星通信系统中的信道估计和增强技术研究是一个十分重要的领域。
- 1、下载文档前请自行甄别文档内容的完整性,平台不提供额外的编辑、内容补充、找答案等附加服务。
- 2、"仅部分预览"的文档,不可在线预览部分如存在完整性等问题,可反馈申请退款(可完整预览的文档不适用该条件!)。
- 3、如文档侵犯您的权益,请联系客服反馈,我们会尽快为您处理(人工客服工作时间:9:00-18:30)。
卫星通信信道的建模和测量一、通信卫星分类卫星可以分类的方式有很多种,这里只列出常见的分类。
1.1 轨位卫星可以根据轨道的高度分为以下几种。
其中,近地轨道卫星(Land mobile satellite-LMS)为当前研究的热点。
因为在高轨位上,卫星信道更加趋近于高斯信道。
而在低轨位工作的卫星,由于其运动性,会存在遮挡、时变、多径效应和多普勒效应。
1.LEO (low earth orbit): 160~2000km2.MEO (medium earth orbit): 2000~36000km3.HEO (high earth orbit):>36000km4.GEO (geostationary orbit):36000km1.2 频段按照卫星工作的频段,一般可以分为以下几类。
其中,在卫星信道测量上,要特别考虑高频段所带来的阴影衰落,以及天气状况。
工作在ka波段的卫星,雨衰严重。
1.L-band: 0.3~3G2.S-band: 2-4G3.C-band: 4~8G4.X-band: 8~12G5.Ku-band: 12~18G6.Ka-band: 27~40G1.3 服务区域根据卫星服务的区域不同,又可以把卫星分为以下几类。
如果卫星服务的区域在城区,则遮挡会更加严重。
而在空旷的郊区,则遮挡会相应变少。
另外,最近有些工作是测量热带区域的卫星信道,主要是因为热带区域天气多变,因此,有必要单独考虑。
1.Rural2.Suburban3.Urban4.Tropical area1.4 极化方式根据卫星的极化方式不同,又可以把卫星分为多极化和双极化卫星。
1.Single-polarized2.Dual-polarized目前,大部分信道建模或者测量都是选择其中的一个子集,作为研究对象。
比如,研究近地轨道卫星在Ka波段下城区的信道的测量和建模。
就调研的结果来看,现在大部分文献都集中在低轨卫星条件下,研究卫星信道的测量和建模。
主要是由于低轨卫星高度低,卫星运动速度快,当最小通信仰角为10°,卫星轨道高度为1300km时,用户看到卫星升起到卫星落下的持续时间在10分钟左右。
因此在用户的一次呼叫过程中,用户与卫星之间的仰角变化很快且变化范围大。
因而,在研究信道模型时,必须研究通信全仰角下的信道模型。
多数研究都是假设在大部分时间内卫星和移动台之间存在直射分量;由于建筑物和树木等物体的遮蔽,存在阴影效应;由于信号的反射、散射和绕射造成的多径效应;由于卫星和移动台间的相对运动形成的多普勒效应。
所以,移动卫星通信信道的特点可以归纳为:时变、多径效应、阴影效应和多普勒效应。
在卫星信道建模和测量中,比较有代表性的研究是C.Loo[1]和Lutz[2]等人的工作。
Loo模型假设信道由直射分量和散射分量组成。
其中直射分量(LOS component)服从log-normal 分布,而多径分量服从瑞利分布。
Lutz通过对信道处在不同状态的概率的研究,提出了基于markov链的信道模型。
大部分论文都是针对不同的卫星、不同的地理环境,通过测量或者改进他人的信道模型,得到不同的有针对性的卫星信道模型。
比如,很多论文都是应用C.Loo 的基本模型,但是采用其他概率分布来满足不同场景下的信道特性。
或者,通过状态细分,扩展Lutz模型,建立更加准确的反映实际卫星信道的模型。
2.0综述类文献1.Karaliopoulos, M. S. and F.-N. Pavlidou (1999). "Modelling the land mobile satellite channel: areview." Electronics & Communication Engineering Journal 11: 235-248.2.Loo, C. and J. S. Butterworth (1998). "Land mobile satellite channel measurements andmodeling." Proceedings of the IEEE 86: 1442-1463.3.Lutz, E. (2012). "Modelling of the Satellite Communications Channel – With Emphasis on theLand Mobile Satellite Channel." Invited Lecture.2.1卫星信道测量和建模的有关文献2.1.1 Statistical Model1.Abdi, A., et al. (2003). "A new simple model for land mobile satellite channels: first- andsecond-order statistics." IEEE Transactions on Wireless Communications 2: 519-528.2.Cheffena, M., et al. (2012). "Land Mobile Satellite Dual Polarized MIMO Channel AlongRoadside Trees: Modeling and Performance Evaluation." IEEE Transactions on Antennas andPropagation 60: 597-605.3.Corazza, G. E., et al. A Rice-lognormal terrestrial and satellite channel model. Proceedings of1994 3rd IEEE International Conference on Universal Personal Communications, IEEE: 155-159.4.Corazza, G. E. and F. Vatalaro (1994). "A statistical model for land mobile satellite channelsand its application to nongeostationary orbit systems." IEEE Transactions on VehicularTechnology 43: 738-742.5.Channel characteristics analysis of the dual circular polarized land mobile satellite MIMO radiochannel. 2011 IEEE-APS Topical Conference on Antennas and Propagation in WirelessCommunications, IEEE: 781-784.6.Fontan, F. P. (2010). "Channel modeling for land mobile satellite services." Antennas andPropagation (EuCAP), 2010 Proceedings of the Fourth European Conference on: 1-5.7.Hazra, S. and A. Mitra (2012). "Additive statistical modelling of land mobile satellite channelsin three-dimensional scattering environment." IET Communications 6: 2361-2370.8.Kanellopoulos, S. A., et al. (2014). "Channel Model for Satellite Communication Links Above10GHz Based on Weibull Distribution." IEEE Communications Letters 18: 568-571.9.Li, W., et al. (2001). "Ka-band land mobile satellite channel model incorporating weathereffects." IEEE Communications Letters 5: 194-196.10.Li, W., et al. Ka-band land mobile satellite channel model: with rain attenuation and otherweather impairments in equatorial zone. VTC2000-Spring. 2000 IEEE 51st VehicularTechnology Conference Proceedings (Cat. No.00CH37026), IEEE. 3: 2468-2472.11.Li, X. and S. Wu A generalized model for mobile satellite channels and performance analysis.International Conference on Communication Technology Proceedings, 2003. ICCT 2003., Beijing Univ. Posts & Telecommun. Press. 2: 1070-1073.12.Li, Y., et al. (2007). On the second-order statistics of a new fading model for land mobilesatellite channels. IET Conference on Wireless, Mobile and Sensor Networks 2007(CCWMSN07), IEE. 2007: 12-15.13.Liolis, K. P., et al. (2007). On the Relation between Mobility and Rainfall Effects in Ku/Ka-bandLine-of-Sight Land Mobile Satellite Channels: An Analytical Statistical Approach. 2007International Workshop on Satellite and Space Communications, IEEE: 26-30.14.Liolis, K. P., et al. (2010). "Statistical Modeling of Dual-Polarized MIMO Land Mobile SatelliteChannels." IEEE Transactions on Communications 58: 3077-3083.15.Loo, C. (1985). "A statistical model for a land mobile satellite link." IEEE Transactions onVehicular Technology 34: 122-127.16.Lutz, E. (2012). "Modelling of the Satellite Communications Channel – With Emphasis on theLand Mobile Satellite Channel."17.Lutz, E. (2013). Modelling of the land mobile satellite communications channel. 2013 IEEE-APS Topical Conference on Antennas and Propagation in Wireless Communications (APWC), IEEE: 199-202.18.Lutz, E., et al. (1991). "The land mobile satellite communication channel-recording, statistics,and channel model." IEEE Transactions on Vehicular Technology 40: 375-386.19.Patzold, M., et al. (1998). "A study of a land mobile satellite channel model with asymmetricalDoppler power spectrum and lognormally distributed line-of-sight component." IEEETransactions on Vehicular Technology 47: 297-310.20.Scalise, S., et al. A Comparison of the Statistical Properties of the Land Mobile SatelliteChannel at Ku, Ka and EHF Bands. 2005 IEEE 61st Vehicular Technology Conference, IEEE.4: 2687-2691.21.Seyedi, Y., et al. (2013). "Use of Shadowing Moments to Statistically Model Mobile SatelliteChannels in Urban Environments." IEEE Transactions on Wireless Communications 12: 3760-3769.22.Zhang, X. and Z. Wang (2014). "Characteristics of narrow band dual-polarized MIMO oversatellite channel model." 5th International Conference on Computing Communication andNetworking Technologies, ICCCNT 2014: 3-7.2.1.2 Empirical Model1.Moraitis, N., et al. (2007). On the Empirical Model Comparison for the Land Mobile SatelliteChannel. 2007 IEEE 65th Vehicular Technology Conference - VTC2007-Spring, IEEE: 1405-1409.2.1.3Geometric Model1.Dottling, M., et al. (2001). "Two- and three-dimensional ray tracing applied to the land mobilesatellite (LMS) propagation channel." IEEE Antennas and Propagation Magazine 43: 27-37.2.Ni Mhearain, F., et al. (2015). A Comparison of Statistical and Geometric Models for the DualPolarised MIMO Land Mobile Satellite Channel. 2015 IEEE 81st Vehicular TechnologyConference (VTC Spring), IEEE: 1-5.3.Oestges, C., et al. (2014). A geometry-based physical-statistical model of land mobile satellitechannels in urban environments. The 8th European Conference on Antennas and Propagation (EuCAP 2014), IEEE: 2261-2263.4.Oestges, C., et al. (1999). "Physical statistical modelling of the land mobile satellite channelbased on ray tracing." IEE Proceedings - Microwaves, Antennas and Propagation 146: 45.5.Sofos, T., et al. A deterministic ray-tracing based model for land mobile satellite channel inurban environment. VTC '98. 48th IEEE Vehicular Technology Conference. Pathway to Global Wireless Revolution (Cat. No.98CH36151), IEEE. 1: 658-660.2.1.4 Markov Chain Based Model1.Alasseur, C., et al. Application of Monte Carlo Markov chain to determination of hiddenMarkov model for mobile satellite channels. 2004 IEEE 59th Vehicular TechnologyConference. VTC 2004-Spring (IEEE Cat. No.04CH37514), IEEE. 1: 186-190.2.Alasseur, C., et al. (2008). "A novel approach to model the land mobile satellite channelthrough reversible jump markov chain monte carlo technique." IEEE Transactions on Wireless Communications 7: 532-542.3.Dottling, M., et al. (2001). "Two- and three-dimensional ray tracing applied to the land mobilesatellite (LMS) propagation channel." IEEE Antennas and Propagation Magazine 43: 27-37.4.Drougas, A. E., et al. (2008). "Stochastic Verification of the First-Order Markovian Assumptionof Rain Attenuation for Satellite Channel Dynamic Modeling." IEEE Communications Letters12: 663-665.5.Hofmann, C. A., et al. (2015). Measurement and modeling of the UHF satellite channel foranimal tracking systems. 2015 IEEE International Conference on Communications (ICC),IEEE: 903-909.6.Meng, D. and W. Wu A model of mobile satellite channel. International Conference onCommunication Technology Proceedings, 2003. ICCT 2003., Beijing Univ. Posts &Telecommun. Press. 2: 1067-1069.7.Lin, H.-P., et al. (2001). "Satellite propagation channel modelling using photogrammetry andhidden Markov model approach." IEE Proceedings - Microwaves, Antennas and Propagation148: 375.8.Lin, H.-P., et al. A non-stationary hidden Markov model for satellite propagation channelmodeling. Proceedings IEEE 56th Vehicular Technology Conference, IEEE. 4: 2485-2488.9.Ming, H., et al. (2008). A new Five-State Markov model for land mobile satellite channels.2008 8th International Symposium on Antennas, Propagation and EM Theory, IEEE: 1512-1515.10.Matolak, D. W. On the multi-state modeling of mobile satellite channels. MILCOM 2000Proceedings. 21st Century Military Communications. Architectures and Technologies forInformation Superiority (Cat. No.00CH37155), IEEE. 1: 261-265.11.Tropea, M., et al. (2013). A new DVB-RCS satellite channel model based on Discrete TimeMarkov Chain and Quality Degree. 2013 IEEE Wireless Communications and NetworkingConference (WCNC), IEEE: 2615-2619.12.Yang, M., et al. (2011). Markov Chain Based Two-State Satellite Mobile Channel Model. 2011IEEE 73rd Vehicular Technology Conference (VTC Spring), IEEE: 1-5.2.1.5 Measurements1.Al-Saegh, A. M., et al. (2017). "Channel Measurements, Characterization, and Modeling forLand Mobile Satellite Terminals in Tropical Regions at Ku-band." IEEE Transactions onVehicular Technology 66: 897-911.2.Butt, G., et al. (1992). "Narrowband channel statistics from multiband propagationmeasurements applicable to high elevation angle land-mobile satellite systems." IEEE Journal on Selected Areas in Communications 10: 1219-1226.3. Cid, E. L., et al. (2016). "Wideband Analysis of the Satellite Communication Channel at Ku-and X-Bands." IEEE Transactions on Vehicular Technology 65: 2787-2790.4.King, P. and S. Stavrou (2007). "Low Elevation Wideband Land Mobile Satellite MIMOChannel Characteristics." IEEE Transactions on Wireless Communications 6: 2712-2720.5. Lemos Cid, E., et al. (2014). "Measurement, Characterization, and Modeling of the HelicopterSatellite Communication Radio Channel." IEEE Transactions on Antennas and Propagation62: 3776-3785.6.Loo, C. and J. S. Butterworth (1998). "Land mobile satellite channel measurements andmodeling." Proceedings of the IEEE 86: 1442-1463.7.Neul, A., et al. (1987). Propagation measurements for the aeronautical satellite channel. 37thIEEE Vehicular Technology Conference, IEEE: 90-97.8.Nikolaidis, V., et al. (2017). "Dual-Polarized Narrowband MIMO LMS Channel Measurementsin Urban Environments." IEEE Transactions on Antennas and Propagation 65: 763-774.9.Petropoulou, P., et al. (2014). "Radio Propagation Channel Measurements for Multi-AntennaSatellite Communication Systems: A Survey." IEEE Antennas and Propagation Magazine 56: 102-122.10.Rougerie, S., et al. (2016). Mobile satellite propagation channels for Ku and Ka band. 201610th European Conference on Antennas and Propagation (EuCAP), IEEE: 1-5.11.Scalise, S., et al. (2008). "Measurement and Modeling of the Land Mobile Satellite Channel atKu-Band." IEEE Transactions on Vehicular Technology 57: 693-703.12.Smith, W. M. Channel Characterization and Modeling for Satellite Communications on theMove. MILCOM 2005 - 2005 IEEE Military Communications Conference, IEEE: 1-7.13.Storek, K.-U., et al. (2015). Interferometer for Measurements of the MIMO Satellite Channel atKu-Band. 2015 IEEE 82nd Vehicular Technology Conference (VTC2015-Fall), IEEE: 1-5. 14.Parks, M. A. N. (1997). Wideband characterization and modelling of the mobile satellitepropagation channel at L- and S-bands. Tenth International Conference on Antennas and Propagation (ICAP), IEE. 1997: v2-39-v32-39.参考文献1.Loo, C., A statistical model for a land mobile satellite link. IEEE Transactions onVehicular Technology, 1985. 34: p. 122-127.2.Lutz, E., et al., The land mobile satellite communication channel-recording,statistics, and channel model. IEEE Transactions on Vehicular Technology, 1991.40: p. 375-386.。