探索不确定性与遥感数据论文 英译汉
遥感翻译完整版
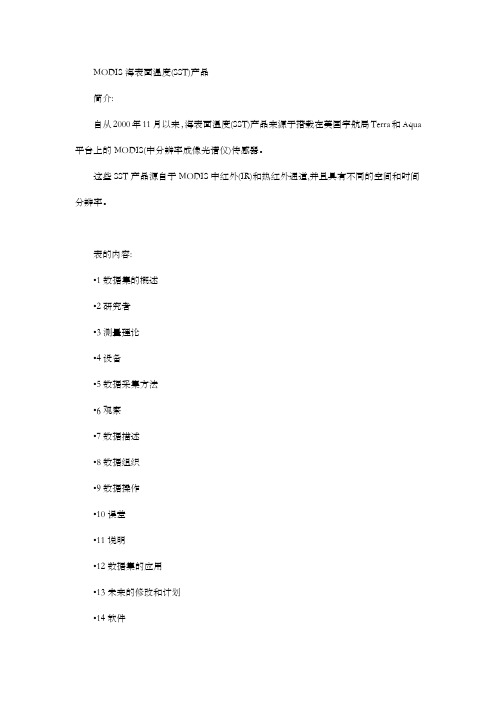
MODIS海表面温度(SST)产品简介:自从2000年11月以来,海表面温度(SST)产品来源于搭载在美国宇航局Terra和Aqua 平台上的MODIS(中分辨率成像光谱仪)传感器。
这些SST产品源自于MODIS中红外(IR)和热红外通道,并且具有不同的空间和时间分辨率。
表的内容:•1数据集的概述•2研究者•3测量理论•4设备•5数据采集方法•6观察•7数据描述•8数据组织•9数据操作•10误差•11说明•12数据集的应用•13未来的修改和计划•14软件•15数据访问•16输出产品和可用性•17 参考文献•18术语表•19缩略语•20文档信息1.数据集的概述:数据集标识:本指南指的是PO.DAAC产品162、163、184和185,它们分别在在Aqua / Terra MODIS全球3级产品绘制的热红外SST图和MODIS全球3级产品绘制的中红外SST图下。
数据集简介:海温资料来源于MODIS红外通道,使用两个通道:热红外通道(11 - 12um)或中红外通道(3.8 - 4.1 um)。
此方法与基于AVHRR传感器用多通道海表面温度(MCSST)产生SST的方法类似。
MODIS数据在各种各样的空间分辨率和时间周期中均可用。
3级产品是全球网格所有点(甚至包含陆地上点)的数据集。
3级产品绘制出的文件来自于3级箱文件。
目的/目标:MODIS SST的目的是提供高质量的参数的全球测量。
MODIS SST优于AVHRR SST是因为MODIS仪器的特性:更高的灵敏度和信噪比低。
与以前的辐射计相比,在高水蒸气,低纬度地区,中红外通道非常有用。
在11-12 um 通道他们也更不容易受气溶胶污染影响。
参数概述:海表面温度讨论:为了了解全球气候变化的过程许多不同的科学测量是必要的。
海洋影响全球范围内的气候的其中一个至关重要的参数是海表面温度(SST)。
气候研究测量中体现其重要性的一个例子是使用SST数据研究世界大洋中西边界流。
第十二章 科技文献的翻译

第十二章科技文献的翻译科技文献是对科技理论、科研过程、科研成果及其推广应用的陈述,其特点是用词准确、语言明了、行文简洁、表达客观、条理清楚、内容确切。
科技作品翻译要注意尽量符合这些持点,力求准确、清楚、通顺。
本章将从词汇、句法和文体三个方面,简要介绍科技文献的特点及其英译处理。
第一节词汇特点及英译科技文献中通常会出现大量的专业术语。
作品越专,专业术语用的也就越多。
因此,翻译此类文献,首先必须弄懂文章的科技内容,然后准确通顺地用英文表达。
一、专业术语翻译专业术语因专业而异。
翻译时,首先要熟悉专业知识,根据所译材料的不同学科和不同专业领域,仔细分析上下文,然后选择正确、符合专业要求的词汇。
拿不准时,要勤查专业英文资料、词典、互联网,或请教他人,以求得确切的译文。
例如:1.高清晰度电视High-definition television (HDTV)2.一艘吃水5米的船a ship of 5m draught3.温室效应the greenhouse effect4.遗传工程生物品种genetically-engineered organisms5. 克隆技术cloning technology6.纳米技术nanometer technology翻译这类词汇切不可望文生义,而应根据其所在学科领域和所处的上下文认真分析,搞清真实含义、选择恰当的英语对应词。
再如:1.如蓄电池的电平降低过甚,电话机随即自动断路。
The telephone turns off by itself if the level of power in the battery falls too low.2.直线被称作是一维的。
A line is said to have one dimension.3. 大气中臭氧层保护地球免受太阳的有害辐射。
Ozone in the atmosphere protects the earth from harmful radiation from the sun.4. 到2050年左右,土壤中细菌的碳释放量有可能大于林木的吸收量,地球会加速变暖。
第十二讲 科技文本的英译
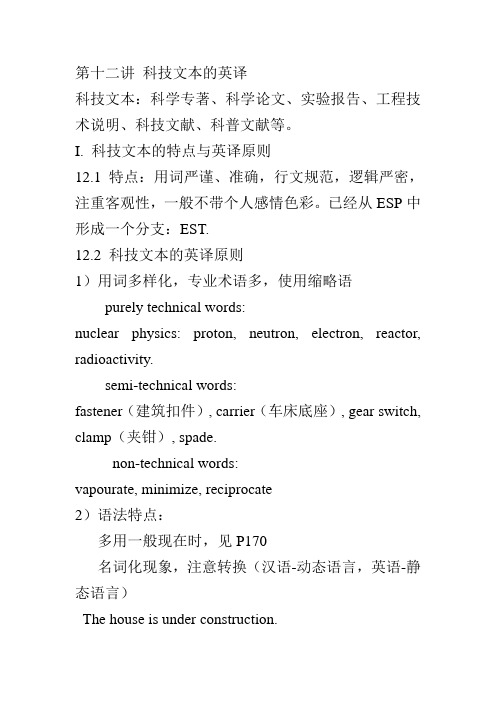
第十二讲科技文本的英译科技文本:科学专著、科学论文、实验报告、工程技术说明、科技文献、科普文献等。
I. 科技文本的特点与英译原则12.1 特点:用词严谨、准确,行文规范,逻辑严密,注重客观性,一般不带个人感情色彩。
已经从ESP中形成一个分支:EST.12.2 科技文本的英译原则1)用词多样化,专业术语多,使用缩略语purely technical words:nuclear physics: proton, neutron, electron, reactor, radioactivity.semi-technical words:fastener(建筑扣件), carrier(车床底座), gear switch, clamp(夹钳), spade.non-technical words:vapourate, minimize, reciprocate2)语法特点:多用一般现在时,见P170名词化现象,注意转换(汉语-动态语言,英语-静态语言)The house is under construction.(The house is being constructed.)例句:中国已经成功地发射了第一课实验通讯卫星。
这颗卫星是由三级火箭推动的,一直运转正常。
它标志着我国在发展运载工具和电子技术方面进入了一个新阶段。
The successful launching of C hina’s first experimental communication satellite, which was propelled by a three-stage rocket and has been in operation ever since, indicates that our nation has entered a new stage in the development of carrier rockets and electronics.科技英语多使用陈述句科技英语被动语态和非人称句较多现决定将垂直测斜仪V15的钻孔作为初次试验,以测试该施工方法的可行性。
Lesson 14 Remote Sensing[27页]
![Lesson 14 Remote Sensing[27页]](https://img.taocdn.com/s3/m/28d6e8ce168884868662d682.png)
2020/10/30
《测控技术与仪器专业英语教程》
19
Launch in use
2. For more than 50 years, photographic images obtained form airborne cameras have used in urban planning, forest management, topographic mapping, soil conservation, military surveillance, and many other applications. 50多年来,用空中摄像机获取的照片一 直用于城市规划、森林管理、地形测量与绘图、土壤保护、军 事监察和其他很多应用中。
3. Thermal infrared sensors can be used to survey the temperatures of water, locate damaged underground pipelines, and map geothermal and geologic structures. 热红外传感器可用来测量水温,找出损 坏的地下管道,绘制地热和地质结构图。
2020/10/30
《测控技术与仪器专业英语教程》
14
Spectrum in dictionary
• 【物】谱, 光[波, 能, 质]谱 • 【无】(射频, 无线电信号)频谱 • 【心理】(眼睛的)余象, 残象 • 范围, 领域, 系列
最新测绘遥感专业英语翻译

1.1 What is Remote Sensing?So, what exactly is remote sensing? For the purposes of this tutorial, we will use thefollowing definition:"Remote sensing is the science (and to some extent, art) of acquiring information about the Earth's surface without actually being in contact with it. This is done by sensing and recording reflected or emitted energy and processing, analyzing, and applying that information."1.1什么是遥感?那么,究竟什么是遥感?这篇教程的目的,我们将使用下面的定义:“遥感科学(在某种程度上,艺术)获取地球表面信息,而不必接触它。
这是通过检测和记录反映或发出能量和处理,进行分析,并应用的信息。
”In much of remote sensing, the process involves an interaction between incident radiation and the targets of interest. This is exemplified by the use of imaging systems where the following seven elements are involved. Note, however that remote sensing also involves the sensing of emitted energy and the use of non-imaging sensors.在许多遥感,过程包括入射辐射和感兴趣的目标之间的相互作用。
地理信息系统英语主要特征及其翻译策略论文
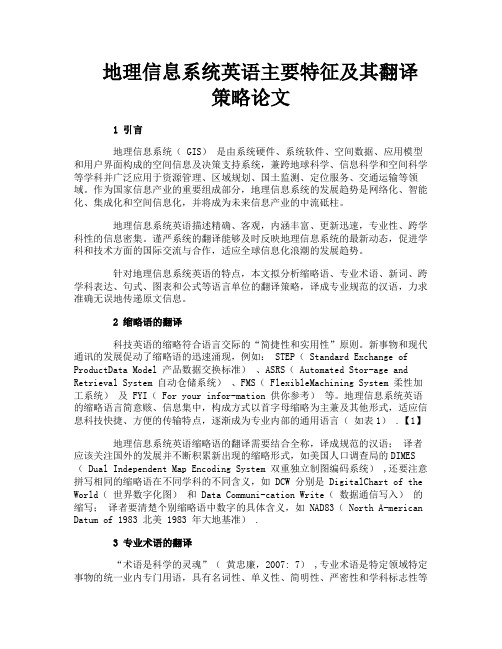
地理信息系统英语主要特征及其翻译策略论文1 引言地理信息系统( GIS)是由系统硬件、系统软件、空间数据、应用模型和用户界面构成的空间信息及决策支持系统,兼跨地球科学、信息科学和空间科学等学科并广泛应用于资源管理、区域规划、国土监测、定位服务、交通运输等领域。
作为国家信息产业的重要组成部分,地理信息系统的发展趋势是网络化、智能化、集成化和空间信息化,并将成为未来信息产业的中流砥柱。
地理信息系统英语描述精确、客观,内涵丰富、更新迅速,专业性、跨学科性的信息密集。
谨严系统的翻译能够及时反映地理信息系统的最新动态,促进学科和技术方面的国际交流与合作,适应全球信息化浪潮的发展趋势。
针对地理信息系统英语的特点,本文拟分析缩略语、专业术语、新词、跨学科表达、句式、图表和公式等语言单位的翻译策略,译成专业规范的汉语,力求准确无误地传递原文信息。
2 缩略语的翻译科技英语的缩略符合语言交际的“简捷性和实用性”原则。
新事物和现代通讯的发展促动了缩略语的迅速涌现,例如: STEP( Standard Exchange of ProductData Model 产品数据交换标准)、ASRS( Automated Stor-age and Retrieval System 自动仓储系统)、FMS( FlexibleMachining System 柔性加工系统)及 FYI( For your infor-mation 供你参考)等。
地理信息系统英语的缩略语言简意赅、信息集中,构成方式以首字母缩略为主兼及其他形式,适应信息科技快捷、方便的传输特点,逐渐成为专业内部的通用语言(如表1) .【1】地理信息系统英语缩略语的翻译需要结合全称,译成规范的汉语;译者应该关注国外的发展并不断积累新出现的缩略形式,如美国人口调查局的DIMES ( Dual Independent Map Encoding System 双重独立制图编码系统) ,还要注意拼写相同的缩略语在不同学科的不同含义,如 DCW 分别是 DigitalChart of the World(世界数字化图)和 Data Communi-cation Write(数据通信写入)的缩写;译者要清楚个别缩略语中数字的具体含义,如 NAD83( North A-merican Datum of 1983 北美 1983 年大地基准) .3 专业术语的翻译“术语是科学的灵魂”(黄忠廉,2007: 7) ,专业术语是特定领域特定事物的统一业内专门用语,具有名词性、单义性、简明性、严密性和学科标志性等特点。
毕业论文英语翻译及原文
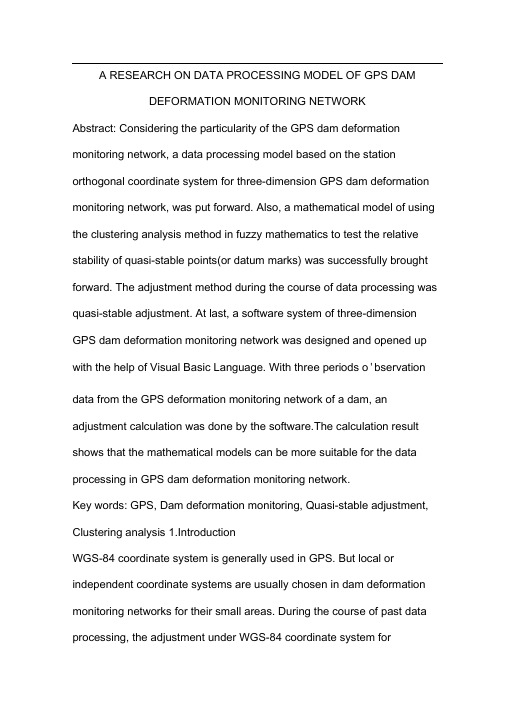
A RESEARCH ON DATA PROCESSING MODEL OF GPS DAMDEFORMATION MONITORING NETWORKAbstract: Considering the particularity of the GPS dam deformation monitoring network, a data processing model based on the station orthogonal coordinate system for three-dimension GPS dam deformation monitoring network, was put forward. Also, a mathematical model of using the clustering analysis method in fuzzy mathematics to test the relative stability of quasi-stable points(or datum marks) was successfully brought forward. The adjustment method during the course of data processing was quasi-stable adjustment. At last, a software system of three-dimension GPS dam deformation monitoring network was designed and opened up with the help of Visual Basic Language. With three periods o'bservation data from the GPS deformation monitoring network of a dam, an adjustment calculation was done by the software.The calculation result shows that the mathematical models can be more suitable for the data processing in GPS dam deformation monitoring network.Key words: GPS, Dam deformation monitoring, Quasi-stable adjustment, Clustering analysis 1.IntroductionWGS-84 coordinate system is generally used in GPS. But local or independent coordinate systems are usually chosen in dam deformation monitoring networks for their small areas. During the course of past data processing, the adjustment under WGS-84 coordinate system forindependent networks or networks with several fixed points is often firstly made. Then, the transformation from WGS-84 coordinate system tolocal(or independent) coordinate systems is done. For GPS deformation monitoring networks with repetitive observation data, the obvious change of datum marks coordinates under the two different coordinate systems can be brought by the tiny deformation of datum marks among different periods of observation. And the greater error can be made during the coordinate transformation. If a local Gauss coordinate system is chosen, the projection distortions can also be produced by the transformation itself. For the reasons above, the station orthogonal coordinate system is chosen as the reference coordinate system for data processing of GPS dam deformation networks. And the mathematical model is put forward and deduced.2.Data processing model based on the station orthogonal coordinate system for three-dimension GPS deformation monitoring networks2.1 Coordinate systemThe station orthogonal coordinate system is a left-hand coordinate system. Its origin is set at one of the GPS monitoring points. The E(X) axis points at the meridian passing the origin. It is on the tangent plane of the origin. And the right north is taken as forward direction. The H(Z) axis is on thenormal line of WGS-84 ellipsoid at the point and takes outward as forward directi on . The E(Y) axis is also on the tangent pla ne of the origi n and uses east for forward directi on.If the positi on vector of the stati on orthogo nal coord in ate system origi nr TP o in WGS-84 is expressed as o 二X0 Y 0 Z o , according to the geodetic latitude and Iongitude ( B° , L。
微波遥感翻译
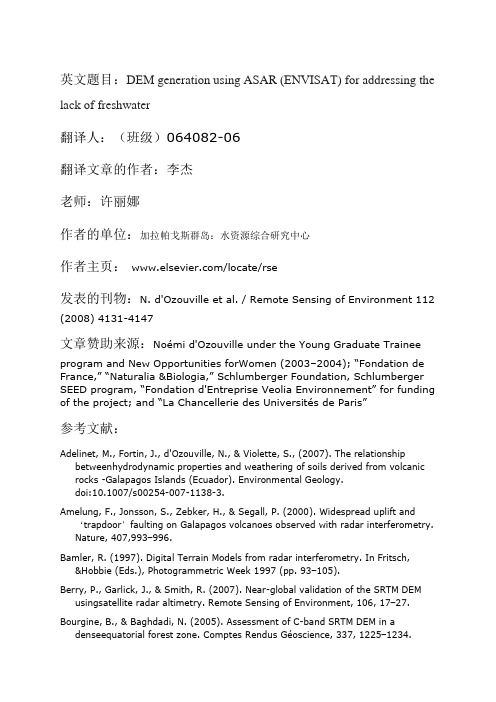
英文题目:DEM generation using ASAR (ENVISAT) for addressing the lack of freshwater翻译人:(班级)064082-06翻译文章的作者:李杰老师:许丽娜作者的单位:加拉帕戈斯群岛:水资源综合研究中心作者主页:/locate/rse发表的刊物:N. d'Ozouville et al. / Remote Sensing of Environment 112 (2008) 4131-4147文章赞助来源:Noémi d'Ozouville under the Young Graduate Trainee program and New Opportunities forWomen (2003–2004); “Fondation de France,” “Naturalia &Biologia,” Schlumberger Foundation, Schlumberger SEED program, “Fondation d'Entreprise Veolia Environnement” for funding of the project; and “La Chancellerie des Universités de Paris”参考文献:Adelinet, M., Fortin, J., d'Ozouville, N., & Violette, S., (2007). The relationship betweenhydrodynamic properties and weathering of soils derived from volcanic rocks -Galapagos Islands (Ecuador). Environmental Geology.doi:10.1007/s00254-007-1138-3.Amelung, F., Jonsson, S., Zebker, H., & Segall, P. (2000). Widespread uplift and ‘trapdoor’faulting on Galapagos volcanoes observed with radar interferometry.Nature, 407,993−996.Bamler, R. (1997). Digital Terrain Models from radar interferometry. In Fritsch, &Hobbie (Eds.), Photogrammetric Week 1997 (pp. 93−105).Berry, P., Garlick, J., & Smith, R. (2007). Near-global validation of the SRTM DEM usingsatellite radar altimetry. Remote Sensing of Environment, 106, 17−27. Bourgine, B., & Baghdadi, N. (2005). Assessment of C-band SRTM DEM in a denseequatorial forest zone. Comptes Rendus Géoscience, 337, 1225−1234.Bow, C. (1979). The geology and petrogeneses of the lavas of Floreana and Santa CruzIslands: Galapagos Archipelago. Ph.D. thesis, University of Oregon, Eugene, USA,308pp.Charleux-Demagne, J. 2001. Qualité des modèles numériques de terrain pourl'hydrologie. Application à la caractérisation du régime de crues desbassinsversants. Ph.D. thesis, Univ. Marne-La-Vallée (France), Sciencesdel'InformationGéographique. 350 p.Crosetto, M. (2002). Calibration and validation of SAR interferometry for DEMgeneration. ISPRS Journal of Photogrammetry & Remote Sensing, 57,213−227.Crosetto, M., & Perez Aragues, F. (1999). Radargrammetry and SAR interferometry forDEM generation: Validation and data fusion. CEOS SAR Workshop,ESA-CNES,Toulouse, 26–29 October 1999 (http://www.estec.esa.nl/ceos99/)Darwin, C., 1859. On the origin of species by means of natural selection, or thepreservation of favoured races in the struggle for life. Published online atThecomplete work of Charles Darwin online (/)d'Ozouville, N. (2007). Etude du fonctionnement hydrologique dans les îles Galápagos:caractérisation d'un milieu volcanique insulaire et préalable a la gestion de laressource. Ph.D. thesis, Univ. Paris 6 Pierre et Marie Curie (France), Sciences de laTerre, Hydrogéologie. 471 pp.d'Ozouville, N., & Merlen, G. (2007). Agua Dulce o la supervivencia en Galápagos. InP.Ospina & C. y Falconí (Eds.), Galápagos: Migraciones, economía, cultura, conflictos yacuerdos. Biblioteca de Ciencias Sociales, 57. Universidad Andina Simón Bolivar, SedeEcuador; Programa de Naciones Unidas para el Desarrollo (pp. 297−313).CorporaciónEditora Nacional, Quito.Eliasson, U. (1984). Chapter 7: Native climax forest. In R. Perry (Ed.), Key environments:Galapagos (pp. 101−115). Bergamon Press.Endreny, T., & Wood, E. (2001). Representing elevation uncertainty in runoff modelingand flowpath mapping. Hydrological Processes, 15, 2223−2236.Endreny, T.,Wood, E., & Lettenmaier, D. (2000). Satellite derived digital elevation modelaccuracy: hydrological modeling requirements. Hydrological Processes, 14(2),177−194.Falorni,G.,Teles,V., Vivoni, E., Bras,R.,&Amartunga,K. (2005).Analysis and characterizationof the vertical accuracy of digital elevation models from Shuttle Radar TopographyMission. Journal of Geophysical Research, 110,doi:10.1029/2003JF000113Fundacion Charles Darwin, Servicio Parque Nacional Galapagos, of New Mexico, U(2004). (/herpetology/research. Data generated byFundacionCharles Darwin, Servicio Parque Nacional Galapagos and University of NewMexico).Garbrecht, J., & Martz, L. (1994). Grid size dependency of parameters extracted fromdigital elevation models. Computers and Geoscience, 20, 85−87.Garbrecht, J., & Martz, L. (1997). The assignment of drainage direction over flat surfacesin raster digital elevation models. Journal of Hydrology, 193, 204−213.Garbrecht, J., & Martz, L. (1999). DEM issues inwater resources modeling. Proceedings ofESRI User Conference, 1999.Gelautz, M., Paillou, P., Chen, C., & Zebker, H. (2003). Radar stereo and interferometryderived digital elevation models: Comparison and combination using Radarsat andERS-2 imagery. International Journal of Remote Sensing, 24,5243−5264.Gorokhovich, Y., & Voustianiouk, A. (2006). Accuracy assessment of the processedSRTM-based elevation data by CGIAR using field data from USA and Thailand and itsrelation to the terrain characteristics. Remote Sensing ofEnvironment, 104, 409−415.Guth, P. (2006). Geomorphometry from SRTM: Comparison to NED.PhotogrammetryEngineering & Remote Sensing. Journal of the American Society for Photogrammetryand Remote Sensing, 72.Grohmann, H. C., Riccomini, C., & Machado Alves, F. (2007). SRTM-based morphotectonicanalysis of the Poços de Caldas Alkaline Massif, southeastern Brazil.Computers& Geoscience, 33, 10−19.Hensley, S., Rosen, P., & Zebker, H. (1994). Generation of high resolution topographicmaps of the Galapagos Islands using TOPSAR data. Geoscience and Remote SensingSymposium, IGARSS 1994. Surface and Atmospheric Remote Sensing Technologies, DataAnalysis and Interpretation. Vol. 2. pp. 704–706.Hirano, A., Welch, R., & Lang, H. (2003). Mapping from ASTER stereo image data: DEMvalidation and accuracy assessment. ISPRS Journal of Photogrammetry & RemoteSensing, 57, 356−370.Hodgson, R., Jensen, J., Schmidt, L., Schill, S., & Davis, B. (2003). An evaluation of LIDARandIFSAR-derived digital elevation models in leaf-on conditions with USGS Level 1and Level 2 DEMs. Remote Sensing of Environment, 84, 295−308. Holzner, J., Eineder, M., & Schattler, B. (2002). First analysis of Envisat/ASAR image modeproducts for interferometry. Proceedings of the Envisat Calibration Review, SpecialPublication SP-520, 9–13 September 2002, ESTEC, Noordwijk, TheNetherlands (http://envisat.esa.int/calval/proceedings)Huttel, C. (1995). Vegetación en coladas de lava, Islas Galápagos, Ecuador. Quito, Ecuador-ORSTOM: Fundacion Charles Darwin 178pp.Ingala, Pronareg, & Orstom (Eds.). (1989). Inventario cartográfico de los recursosnaturales, geomorfología, vegetación, híídricos, ecológicos y biofísicos de las IslasGalápagos, Ecuador. Ingala (pp.159). Quito, Ecuador.Ingala (2002). Plan Regional para la Conservación y el Desarrollo Sustentable de Galápagos.Tech. rep., Instituto Nacional Galápagos.Jensen, S. K., & Domingue, J. O. (1998). Extracting topographic structure from digitalelevation data for geographic information systemanalysis.PhotogrammetricEngineering and Remote Sensing, 54, 1593−1600.Kenward, T., Lettenmaier, D., & Wood, E. E. F. (2000). Effects of digital elevation modelaccuracy on hydrological predictions. Remote Sensing of Environment, 74(2), 432−444.Kervyn, F. (2001). Modelling topography with SAR interferometry: Illustrations of afavourable and less favourable environment. Computer & Geoscience, 27,1039−1050.Kooij van der, M., Armour, B., Ehrismann, J., McConnell, P., Lynch, B., & Nazarho,D.(1999). SAR repeat pass interferometry —Operational accomplishments andfuturepotential. 21st CSRS/4th Airborne RS Conference, Ottawa, Canada, 21–24 June 1999.Ludwig, R., & Schneider, P. (2006). Validation of digital elevation models from SRTM XSARfor applications in hydrologic modeling. ISPRS Journal of Photogrammetry andRemote Sensing, 60, 339−358.Marinelli, L., Toutin, T., & Dowman, I. (1997). Génération de MNT par radargrammétrie:Etat de l'art et perspectives. Bulletin de la Société Française de PhotogrammétrieTélédetection, 148, 89−95.Martz, L.,&Garbrecht, J. (1992).Numerical definitionof drainagenetwork andsubcatchmentareas from Digital Elevation Models. Computers & Geosciences, 18, 747−761.Meisels, A., Raizman, S., & Karnieli, A. (1995). Skeletonizing a DEM into drainagenetworks. Computers & Geosciences, 21, 187−196.Navarro Latorre, J., Soler Liceras, C., & Jimenez Suarez, J. (1991). Bases para un PlanHidrologico del Archipielago de Galápagos —Republica de Ecuador. Tech.rep.,Gobierno Autonomo de Canarias and Consejeria de Obras Publicas Vivienda y Aguas,Direccion General de Aguas.Parcharidis, I., Sakkas, V., Ganas, A., & Katopodii, A. (2002). A production of DigitalElevation Model (D.E.M.) by means of SAR tandem images in a volcanic landscapeand its quality assessment. 6th Pan-Hellenic Geographical Congress, Thessalonik,volume II pp. 201–207.Rabus, B., Eineder, M., Roth, A., & Bamler, R. (2003). The shuttle radar topographymission —A new class of digital elevation models acquired byspaceborne radar.ISPRS Journal of Photogrammetry & Remote Sensing, 57,241−262.Rocca, F., Prati, C.,&Ferretti, A. (1997).Overviewof SAR interferometry. 3rd ERS Symposium,14–21 March 1997 (opening speech —http://earth.esa.int/symposia/program-details/speeches/rocca-et-al/. Opening speech 3rd ERS Symposium, 14–21 March 1997.Rodgriguez, E., Morris, C., & Belz, J. (2006). A global assessment of SRTM performance.Photogrammetric Engineering and Remote Sensing, 72, 249−260.Rosen, P., Hensley, S., Joughin, I., Li, F., Madsen, S., Rodriguez, E., & Goldstein, R.(2000).Synthetic aperture radar interferometry. Proceedings of the IEEE, 88, 333−379.Roth, A., Knopfle, W., Strunz, G., Lehner, M., & Reinartz, P. (2002). Towards a globalelevation product: Combination of multi-source digital elevation models.Symposiumon Geospatial Theory, Processing and Applications, Ottawa, 2002.Smith, D. P., & Atkinson, S. F. (2001). Accuracy of rectification using topographic mapversus GPS ground control points. Photogrammetric Engineering & Remote Sensing,67(5), 585−587.Snell, H., & Rea, S. (1999). The 1997–1998 El Niño in Galapagos: Can 34 years of dataestimate 120 years of pattern? Noticias de Galápagos, 60.Souris,M., 2001. http://rslultra.star.ait.ac.th/souris/ecuador.htm. Data available onwebsite.Stevens, N., Garbeil, H., & Mouginis-Mark, P. (2004). NASA EOS Terra ASTER: Volcanictopographic mapping and capability. Remote Sensing of Environment, 90, 405−414.Thornwaite, C. (1946). An approach towards a rational classification of climate.GeographyReview, 38, 55−89.Toutin, T. (1999). Error tracking of radargrammetric DEM from RADARSAT images.IEEETransactions on Geoscience and Remote Sensing, 37(5), 2227−2238.Toutin, T. (2000). Evaluation of radargrammetric DEM from RADARSAT images in highrelief areas. IEEE Transactions on Geoscience and Remote Sensing, 38(2), 782−789.Toutin, T. (2002). Impact of terrain slope and aspect on radargrammetric DEM accuracy.ISPRS Journal of Photogrammetry and Remote Sensing, 57, 228−240. Toutin, T., & Gray, L. (2000). State-of-the-art of elevation extraction from satellite SARdata. ISPRS Journal of Photogrammetry and Remote Sensing, 55, 13−33.Valeriano, M. M., Kuplich, T. M., Storino, M., Amaral, B. D., Mendes, J. N. J., & Lima, D.J.(2006). Modelling small watersheds in Brazilian Amazonia with shuttleradartopographic mission-90 m data. Computers & Geoscience, 32, 1169−1181.Walker, A.,Muller, J. -P.,Morley, J., Smith, A., & Naden, P. (1999).Multi-pass interferometricSARDEMs for hydrological network derivation. In E. P. Division (Ed.), Proceedings Fringe'99 Workshop: Advancing ERS SAR Interferometry fromApplications towards Operations,10–12 November 1999, Liège, Belgium. Vol. ESA SP-478.Walker, J., & Willgoose, G. (1999). On the effect of digital elevation model accuracy onhydrology and geomorphology. Water Resources Research, 35(7), 2259−2268. Wang, X., & Yin, Z. -Y. (1998). A comparison of drainage networks derived from digitalelevation models at two scales. Journal of Hydrology, 210, 221−241. Wechsler, S., (2000). Effect of DEM Uncertainty on Topographic Parameters, DEM Scaleand Terrain Evaluation. Ph.D. thesis, Sate Univeristy of New York, Syracuse, NewYork. 380pp.Wegmüller, U. (1999). Automated terrain corrected SAR geocoding. Proceedings ofIGARSS'99, Hamburg, 28 June – 2 July 1999.Wegmüller, U., Werner, C., Wiesmann, A., & Strozzi, T. (2003). Radargrammetry andspace triangulation for DEM generation and image ortho-rectification.Proceedingsof IGARSS'03, Toulouse, France, 21–25 July 2003.Werner, C., Wegmüller, U., Strozzi, T., & Wiesmann, A. (2005). Precision estimation oflocal offsets between SAR SLCs and detected SAR images. Proceedings ofIGARSS '05,Seoul, Korea, 25–29 July 2005.Wise, S. (2000). Assessing the quality of hydrological applications of digital elevationmodels derived from contours. Hydrological Processes, 14,1909−1929. Won, J. -H., Lee, J. -Y., & Kim, J. -W. (2006). Groundwater occurrence on Jeju Island, Korea.Hydrogeology Journal, 14, 532−547.Wright, R., Garbeil, H., Baloga, S., & Mouginis-Mark, P. (2006). An assessment of shuttleradar topography mission digital elevation data for studies of volcanomorphology.Remote Sensing of Environment, 105, 41−53.Zebker, H., Werner, C., Rosen, P., & Hensley, S. (1994). Accuracy of topographic mapderived from ERS-1 interferometry radar. IEEE Transactions on Geoscience andRemote Sensing, 32, 823−836.Abstracts(文摘):低浮雕海洋岛屿往往苦于缺乏淡水资源。
- 1、下载文档前请自行甄别文档内容的完整性,平台不提供额外的编辑、内容补充、找答案等附加服务。
- 2、"仅部分预览"的文档,不可在线预览部分如存在完整性等问题,可反馈申请退款(可完整预览的文档不适用该条件!)。
- 3、如文档侵犯您的权益,请联系客服反馈,我们会尽快为您处理(人工客服工作时间:9:00-18:30)。
Exploring uncertainty in remotely sensed data withparallel coordinate plotsYong Ge , Sanping Li , V. Chris Lakhan , Arko LucieerAbstract The existence of uncertainty in classified remotely sensed data necessitates the application of enhanced techniques for identifying and visualizing the various degrees of uncertainty. This paper, therefore, applies the multidimensional graphical data analysis technique of parallel coordinate plots (PCP) to visualize the uncertainty in Landsat Thematic Mapper (TM) data classified by the Maximum Likelihood Classifier (MLC) and Fuzzy C-Means (FCM). The Landsat TM data are from the Yellow River Delta, Shandong Province, China. Image classification with MLC and FCM provides the probability vector and fuzzy membership vector of each pixel. Based on these vectors, the Shannon’s entropy (S.E.) of each pixel is calculated. PCPs are then produced for each classification output. The PCP axes denote the posterior probability vector and fuzzy membership vector and two additional axes represent S.E. and the associated degree of uncertainty. The PCPs highlight the distribution of probability values of different land cover types for each pixel, and also reflect the status of pixels with different degrees of uncertainty. Brushing functionality is then added to PCP visualization in order to highlight selected pixels of interest. This not only reduces the visualization uncertainty, but also provides invaluable information on the positional and spectral characteristics of targeted pixels.1. IntroductionA major problem that needs to be addressed in remote sensing is the analysis,identification and visualization of the uncertainties arising from the classification ofremotely sensed data with classifiers such as the Maximum Likelihood Classifier (MLC)and Fuzzy C-Means (FCM). While the estimation and mapping of uncertainty has beendiscussed by several authors (for example, Shi and Ehlers, 1996; van der Wel et al., 1998;Dungan et al., 2002; Foody and Atkinson, 2002; Lucieer and Kraak, 2004; Ibrahim et al.,2005; Ge and Li, 2008a), very little research has been done on identifying, targeting andvisualizing pixels with different degrees of uncertainty. This paper, therefore, appliesparallel coordinate plots (PCP) (Inselberg, 1985, 2009; Inselberg and Dimsdale, 1990) tovisualize the uncertainty in sample data and classified data with MLC and FuzzyC-Means. A PCP is a multivariate visualization tool that plots multiple attributes on theX-axis against their values on the Y-axis and has been widely applied to data mining andvisualization (Inselberg and Dimsdale, 1990; Guo, 2003; Edsall, 2003; Gahegan et al., 2002; Andrienko and Andrienko, 2004; Guo et al., 2005; Inselberg, 2009). The PCP is useful for providing a representation of high dimensional objects for visualizing uncertainty in remotely sensed data compared with two-dimensional, three-dimensional, animation and other visualization techniques (Ge and Li, 2008b). Several advantages of the PCP technique for visualizing multidimensional data have been outlined by Siirtoia and Ra¨iha¨ (2006).Data for the PCPs are from a 1999 Landsat Thematic Mapper (TM) image acquired over the Yellow River Delta, Shandong Province, China. After classifying the data the paper emphasizes the uncertainties arising from the classification process. The probability vector and fuzzy membership vector of each pixel, obtained with classifiers MLC and FCM, are then used in the calculation of Shannon’s entropy (S.E.), a measure of the degree of uncertainty. Axes on the PCP illustrate S.E. and the degrees of uncertainty. Brushing is then added to PCP visualization in order to highlight selected pixels. As demonstrated by Siirtoia and Ra¨iha¨(2006) the brushing operation enhances PCP visualization by allowing interaction with PCPs whereby polylines can be selected and highlighted.2. Remarks on parallel coordinate plots and brushingParallel coordinate plots, a data analysis tool, can be applied to a diverse set of multidimensional problems (Inselberg and Dimsdale, 1990). The basis of PCP is to place coordinate axes in parallel, and to present a data point as a connection line between coordinate values. A given row of data could be represented by drawing a line that connects the value of that row to each corresponding axis (Kreuseler, 2000; Shneiderman, 2004). A single set of connected line segments representing one multidimensional data item is called a polyline (Siirtoia and Ra¨iha¨, 2006). An entire dimensional dataset can be viewed whereby all observations are plotted on the same graph. All attributes in two-dimensional feature space could, therefore, be distinguished thereby allowing for the recognition of uncertainties and outliers in the dataset.The PCP can be used to visualize not only multidimensional data but alsonon-numerical multidimensional data. Jang and Yang (1996) discussed applications of PCP especially its usefulness as a dynamic graphics tool for multivariate data analysis. In this paper the PCP is applied to Landsat TM multidimensional data. This paper follows the procedures of Lucieer (2004) and Lucieer and Kraak (2004) who adopted the PCP from Hauser et al. (2002) and Ledermann (2003) to represent the uncertainties in the spectral characteristics of pixels and their corresponding fuzzy membership. To enhance the visualization of the PCP tinteractive brushing functionality is employed. In the brushing operation, pixels of interest are selected and then highlighted. Brushing permits not only highlighting but also masking and deletion of specific pixels and spatial areas. 3. Use of PCP to explore uncertainty in sample dataIn the supervised classification process of remotely sensed imagery, the quantity of samples is a major factor affecting the accuracy of the image classification. In this section, the uncertainty in the sample data is, therefore, first explored with PCP.3.1. Data acquiredIn this paper, the Landsat TM image representing the study area was acquired on August 28, 1999, and covers an area of the Yellow River delta. The image area is at the intersection of the terrain between Dongying and Binzhou, Shandong Province. The upper left latitude and longitude coordinates of the image are 11880034.0700E and 37822024.0000N, respectively. The lower right latitude and longitude coordinates are 118810052.8300E and 37813058.1300N, respectively. The image size is 515 by 515 pixels, with each pixel having a resolution of 30 m. Fig. 1 is a pseudo-color composite image of bands 5, 4 and 3.3.2. Exploring the uncertainty in sample dataThe image includes six land cover types, namely Water, Agriculture_1, Agriculture_2 (Agriculture_1 and Agriculture_2 are different crops), Urban, Bottomland (the channel of the Yellow River), and Bareground. Sample data are selected from the region of interest (see Fig. 2), and represents a total of 26,639 pixels.The Parbat software developed by Lucieer (2004) and Lucieer and Kraak (2004) is used to produce the PCP. The PCP depicts the multidimensional characteristics of pixels in the remote sensing image througha set of parallel axes and polylines in a twodimensional plane (Fig. 3). It is noticeable that there is a clear representation of sample data from different land cover types, as shown by clustering of spectral signatures, and the dispersion and overlapping of spectral signatures from different land cover types. The digital numbers (DNs) of all pixels in the land cover type,Fig. 1. Pseudo-color composition image of the study area.Fig. 2. Sample data in the region of interestBottomland, are very concentrated within a narrow band. The range of DNs of pixels in the Water class is also narrow, except for band 3. The land cover types of Water and Bottomland in Fig. 3 can be easily distinguished from the other land covers in bands 5 and 7. Further differentiation is provided in band 3. The radiation responses for Agriculture_1 and Agriculture_2 have close similarity in bands 1, 2, 3, 6 and 7, with a degree of overlap in bands 4 and 5. There is an almost perfect positive correlation between bands 1 and 2 for all categories. This occurrence presents difficulties in clearly differentiating pixels for Agriculture_1 and Agriculture_2. Hence, it is evident that there is uncertainty in differentiating pixels for the land cover types Agriculture_1 and Agriculture_2.4. Classification and measurement of uncertainty in classified remote sensing images 4.1. Uncertainties arising from the classification processThe supervised classifiers, MLC and FCM, are applied to the image, with the condition that no pixel is assigned to a null class. The classified images are shown in Fig. 4a and b.Comparison of Fig. 4a and b reveals that the classified results from MLC and FCM are not identical for the data pertaining to the same region of interest (ROI).Fig. 3. PCP of sample data.The difference images between these two classified results are presented in Fig. 5a and b, with Fig. 5a showing the classified results for MLC. The classified results of FCMfor the difference pixels are illustrated in Fig. 5b. The number of difference pixels total 16,416. These difference pixels are distributed mainly on the banks of the river, and in mixed areas of Bareground, Agriculture_1, and Agriculture_2. For the MLC classified result, 57.1% of the difference pixels are classified as Agriculture_1, and 36.7% are classified as Agriculture_2. The FCM classified results, however, demonstrate that 90.5% of difference pixels are Bareground while 9.3% are Agriculture_2.The number of pixels in the ROI and in each of the classification categories from MLC and FCM are illustrated in Fig. 6. Evidently, there is a significant difference in the number of pixels for Agriculture_1, Agriculture_2, and Bareground, while the number of pixels for Water and Bottomland are very similar. This is also demonstrated in Fig.Fig. 4. (a) Classification result from MLC; (b) classification result from fuzzy CmeansFig. 5. Spatial distribution of difference pixels between MLC and FCM: (a) difference pixels in the classified result from MLC; (b) difference pixels in the classified result from FCM.Fig. 6. Comparison of numbers of pixels in the ROI; each category from MLC and FCM.5a and b. Based on the classified results from MLC and FCM it is possible to claim that there are relatively high uncertainties in identifying Agriculture_1, Agriculture_2 and Bareground. There are, however, lower uncertainties in the identification of Water and Bareground.4.2. Measurement4.2.1. Probability/fuzzy membershipFCM produces a fuzzy membership vector for each pixel. This fuzzy membership can be taken as the area proportion within a pixel (Bastin et al., 2002). It is possible for pixels to have the same class type, but their posterior probabilities or fuzzy memberships could be different. Hence, the probability vector or fuzzy membership is normally used as a measure for uncertainty on a pixel scale. The posterior probability and fuzzy membership of Water and Agriculture_2 are illustrated in Figs. 7 and 8, respectively. The uncertainty in spatial distributions can be clearly observed in Figs. 7 and 8. In Fig. 7, pixels belonging to the Water class have larger posterior probability or fuzzy membership, thereby indicating that these pixels have smaller uncertainties. While there are insignificant variations between Fig. 7a and b there are, however, noticeable differences between Fig.8a and b, especially for the class,Agriculture_2.Fig. 7. Water class: (a) posterior probability from MLC; (b) fuzzy membership fromFCM. 4.2.2. Shannon entropy [S.E.]Entropy is a measure of uncertainty and information formulated in terms of probability theory, which expresses the relative support associated with mutually exclusive alternative classes (Foody, 1996; Brown et al., 2009). Shannon ’s entropy (Shannon, 1948), applied to the measurement of the quality of a remote sensing image, is defined as the required amount of information that determines a pixel completely belonging to a category and expresses the overall uncertainty information of the probability or membership vector, and all elements in the probability vector are used in the calculation (Maselli et al., 1994). Therefore, it is an appropriate method to measure the uncertainty for each pixel in classified remotely sensed images (van der Wel et al., 1998). The use of S.E. in this paper is represented by considering the following. Given U as the universe of discourse in the remotely sensed imagery, U contains all pixels in this image and is partitioned by {X1, X2, . . ., Xn} where n is the number of classes. The probability of each partition is denoted as pi = P(Xi) giving the S.E. as()1log 2n i ii H X p p ==∑ (1) where H(X) is the information entropy of the information source. When pi = 0, theequation becomes 0 log 0 = 0 (Zhang et al., 2001; Liang and Li, 2005). It is accepted that: 0 _ H(X) _ log n.On the basis of Bayesian decision rules, MLC determines the class type of every pixel according to its maximum posterior probability in a probability vector. The classification process could, therefore, be associated with uncertainty. S.E. derived from a probability vector or fuzzy membership vector can represent the variation in the posterior probability vector and can be taken as one of the measures on a pixel scale (van der Wel et al., 1998). Similarly, applying FCM to remotely sensed data can produce fuzzy membership values in all land cover classes. Hence, fuzzy membership can also be considered as a measure of uncertainty on a pixel scale. On the basis of the fuzzy membership of each pixel the corresponding S.E. can be calculated. To compare the MLC and FCM methods it is necessary to normalize the computed S.E. values.Fig. 8. Agriculture_2 class: (a) posterior Fig. 9.probability from MLC; (b) fuzzymembership from FCM.From Eq. (1) S.E. can be calculated through posterior probability and fuzzy memberships. Fig. 9a and b displays normalized S.E. values of classified pixels from MLC and FCM, respectively. When the grey value of a pixel is zero then the uncertainty is zero, and when the grey value is 255 then the uncertainty will be at the maximum value of 1. From Fig. 9a and b it can be observed that the classes of Water and Bottomland have lower uncertainties while the classes of Bareground, Agriculture_1 and Agriculture_2 have higher uncertainties. These results emphasize that S.E. values calculated from MLC are comparatively higher than those obtained from FCM. Obviously, FCM produces more information about the end members within a pixel than MLC.4.2.3. Degree of uncertaintyWhile S.E. provides information on the uncertainties of pixels it is, however, known that when there is a large range of greyscale values representing brightness values [0,255] the subtle differences in greyscales are not easily discernible to humans. Hence, there are difficulties in differentiating the degree of uncertainty. To overcome this problem the S.E. is, therefore, discretized equidistantly. For instance, the S.E. is discretized into the following intervals: 0.00, (0.00, 0.20], (0.20, 0.40], (0.40, 0.60], (0.60, 0.80], (0.80, 1.00]. Measurements falling into the same interval have the same degree of uncertainty. By assigning a color to each degree of uncertainty, a pixel-based uncertainty visualization is produced (see Fig. 10a and b). This discretization clearly highlights the degrees of uncertainty in the classified remotely sensing image. In Fig. 10a, representing MLC, the degrees of uncertainty for most of the pixels are 0, 1 and 2 while in Fig. 10b, associated with FCM, the degrees of uncertainty for most of the pixels are 1, 2 and 3 and occasionally 4. It is worthwhile to note that the interval map of S.E. permits a comparison of the degrees of uncertainty in classified results from different classifiers.5. PCP and brushingThe PCP is useful for visually exploring the degree of dispersion or aggregation of the DN values of pixels in each band, and can be conveniently used to investigate the reasons contributing to uncertainty. With the brushing operation, the pixels of interest could be selected and highlighted.5.1. PCPFrom the MLC results two sets of pixels have been, respectively, randomly selected from the classes of Water and Agriculture_2 tobe represented on a PCP. The Parbat software (Lucieer, 2004;Fig. 10. Degree of uncertainty: (a) derived from MLC; (b) derived from FCM.Lucieer and Kraak, 2004) is used to produce a PCP of Water (see Fig. 11) and Agriculture_2 (see Fig. 12) classified by MLC. The first six axes are posterior probabilities of the six land cover types and the last two axes are the S.E. and degree of uncertainty. For instance, Fig. 12 illustrates the uncertainties of the pixels selected from the class type of Agriculture_2 and their distributions. The posterior probability of Agriculture_2 to each pixel is relatively higher than other categories. Of significance, there is negative correlation between Agriculture_1 and Agriculture_2. In this example, the S.E. is equally divided into five intervals to obtain the degree of uncertainty. The uncertainties of the pixels selected from Water and Agriculture_2, respectively, and their distributions are illustrated by Figs. 11 and 12.Figs. 13 and 14 are obtained by placing all the DNs of these pixels, fuzzy memberships, S.E., and associated degree of uncertainty as attribute dimensions on the horizontal axis of the PCP. Similarly, pixels are randomly selected to be represented on the PCP, and the S.E. is divided into five equal intervals to obtain the degree of uncertainty. Fig. 13 highlights the uncertainty characteristics associated with the spectralsignatures of pixels from the Water class while Fig. 14 shows the spectral signatures of pixels from the Agriculture_2 class and their related uncertainty characteristics. B.1–B.7 and C.1–C.6 denote the seven bands of the TM image and their fuzzy memberships in the six land cover types, which are Water, Agriculture_1, Agriculture_2, Urban, Bottomland, and Bareground, respectively. The S.E. from the FCM classifier and the degree of uncertainty within the range 0–5 are denoted by ShE and UnL. The red line within bands 1–7 emphasize pixels with a degree of uncertainty of zero. Different colors denote different degrees of uncertainty in the PCP. From the PCP it is possible to discern the distribution of fuzzy memberships and Shannon entropies of pixels with different degrees of uncertainty.A comparison of Figs. 11 and 13 reveals that the MLC posterior probabilities results on classified pixels in the Water class have a non-zero value which are concentrated in the Bareground class. For the FCM (see Fig. 13) most of the classified pixels in the Water class have a fuzzy membership closer to 1. The fuzzy memberships of some pixels in the Bareground and Agriculture_1 and 2 classes are away from zero. It is, therefore, apparent that pixels with a highdegree of uncertainty are mixed pixels in the Water and Bareground classes, namely the boundary pixels between Water and Bareground. As expected, the spectral response for these pixels contains characteristics of both land cover types.Fig. 13 provides additional information on the spectral characteristics of Water and its uncertainty distribution. For instance, the degrees of dispersion in bands 2, 3, 4 and 5 are high, and the distance to the red line in these four bands is also relatively high. As such, their uncertainties are high. Pixels with a degree of uncertainty of 3, represented by the blue line, are relatively far The fuzzy memberships of pixels for Bareground are in the range 0.2–0.4 thereby giving rise to a high degree of uncertainty.from MLC.Fig. 13. Spectral features of the Water class and their uncertainty characteristics.Pixels with a degree of uncertainty of 4, represented by the pink line, are relatively far from those pixels with a degree of uncertainty of zero, as shown by the red line in band 4. There are two independent distribution ranges in band 3. In the case of the Water class pixels with a high degree of uncertainty, assuming their fuzzy membership on Bottomland is high, then the DNs in band 3 will be greater than the DNs of pixels with a degree ofuncertainty of zero.Fig. 14. Spectral features of pixels from the Agriculture_2 class and their uncertainty characteristicsFig. 15. (a) Class of Water: pixels with a low degree of uncertainty in the PCP; (b) class of Water: pixels with a high degree of uncertainty degree in the PCP.A comparison of Figs. 12 and 14 reveals that the classes of Agriculture_1 and Bareground have the highest uncertainty in Agriculture_2. The difference between the PCPs from MLC and FCMis because the influence of Agriculture_1 on Agriculture_2 in MLC is greater than the influence of Bareground on Agriculture_ 2. For FCM the two influences on Agriculture_2 are almost similar. When the fuzzy memberships of pixels for Agriculture_1 are greater, their DNs in bands 4 and 5 are less than that of pixels with a degree of uncertainty of zero. The DNs in all bands are dispersed for pixels with large fuzzy membership for Bareground.5.2. BrushingFrom Figs. 11 and 14 it is demonstrated that when different colors are used to represent different degrees of uncertainty, a certain amount of overlap develops between color lines, especially on the axes where the distribution of polylines is concentrated. The superposition of polylines definitely increases the difficulty in visual uncertainty analysis.A new ‘‘visual’’uncertainty is, thereby, introduced. To improve on this visualization the brushing operation is introduced to the PCP (Hauser et al., 2002; Ledermann, 2003). The difference from that of a conventional approach is that the user selects pixels of interest, and then highlights them with a brush, instead of using colored polylines. This is a suitable and convenient method for conducting targeted analysis on the spectral characteristics of pixels and their associated uncertainty.Brushing is applied to a set of pixels with a low degree of uncertainty (see Fig. 15a) and a set of pixels with a high degree of uncertainty (see Fig. 15b). The pixels targeted for investigation belong to the class type,Water.Agreen polyline denotes the pixels being brushed, while a grey polyline represents the distribution characteristics of all pixels belonging to the class type, Water. The red line for bands 1–7 represents pixels with a zero degree of uncertainty. In Fig. 15a, there is a strong negative correlation between C.5 (Bottomland) and C.6 (Bareground). This means thatthe larger the memberships of pixels in the class of Bottomland, the smaller the memberships of pixels in the class of Bareground. To further investigate the class type, Agriculture_2, PCP and brushing are used to visualize pixels with low uncertainty (seeFig. 16a) and pixels with high uncertainty (see Fig. 16b). From the Figures it becomes noticeable that when PCP is combined with brushing the user could focus on the spectral characteristics and the uncertainty distribution of pixels of interest. This effectively reduces the uncertainty introduced by the visualization of the remotely sensed data.Fig. 16. (a) Class of Agriculture_2: pixels with a low degree of uncertainty in the PCP; (b) class of Agriculture_2: pixels with a high degree of uncertainty in the PCP.6. Discussion and conclusionA major unresolved problem in image processing is how to identify and visualize the uncertainty arising out of the classification of remotely sensed data. Without doubt, targeting uncertainties will not only permit better visualization of features in geographical space but also enhance the capabilities of policymakers who have to make reliabledecisions on a broad range of geospatial issues. This paper has demonstrated the effectiveness of the combined PCP and brushing operation to explore and visualize the uncertainties in remotely sensed data classified with MLC and FCM.The MLC and FCM results demonstrate that water class pixels, with a high degree of uncertainty, have high posterior probability or fuzzy membership for the type Bottomland. Furthermore, some Agriculture_2 class pixels, with a high degree of uncertainty, have high fuzzy membership for the Bareground class type. Therefore, it is possible to compare the pixel distribution for Water and Bottomland or Agriculture_2 and Bareground, and further analyze the possible reasons causing the uncertainty by investigating the spectral characteristics for all bands. Essentially, it is necessary to use the probability vector and fuzzy membership vector of each pixel to compute the S.E. The degree of uncertainty of each pixel can then be represented on a PCP. As illustrated in this paper two axes on the PCP represent Shannon’s entropy and the degree of uncertainty.The PCP technique is also advantageous for highlighting the distribution of probability values of different land covers of each pixel, and also reflects the status of pixels with different degrees of uncertainty. Moreover, a PCP can be produced for the spectral characteristics of sample data and uncertainty attributes of classified data. The class type of the sample data can be included in the PCP to evaluate the quality of the data. Moreover, the sample data can then be compared to the classified data to evaluate whether the sample data are a reasonable reflection of the spectral characteristics of all bands. The identification of any dissimilarities or uncertainties is a definite indication of improvement in the visualization process. This paper demonstrates that there could be enhancements in PCP visualization with the addition of the brushing operation. Instead of using color polylines, as done with previous approaches, brushing permits the user to select pixels of interest. These pixels could then be highlighted with brushing instead of with color polylines. Evidently, brushing facilitates targeted analysis of the spectral characteristics of pixels and any associated uncertainty. It could, therefore, be concluded that the integration of PCP with the brushing operation is beneficial for not only visualizing uncertainty but also gaining insights on the spectral characteristics andattribute information of pixels of interest. By interacting with the PCP through the brushing operation it is possible to conduct an exploration of uncertainty, even at the sub-pixel level.AcknowledgementsThis research received partial support from the National Natural Science Foundation of China (Grant No. 40671136) and the National High Technology Research and Development Program of China (Grant No. 2006AA120106).ReferencesAndrienko, G., Andrienko, N., 2004. Parallel coordinates for exploring properties of subsets CMV. In: Roberts, J. (Ed.), Proceedings International Conference on Coordinated & Multiple Views in Exploratory Visualization, London, England, July 13, 2004, pp. 93–104.Bastin, L., Fisher, P.F., Wood, J., 2002. Visualizing uncertainty in multi-spectral remotely sensed imagery. Computers & Geosciences 28, 337–350.Brown, K.M., Foody, G.M., Atkinson, P.M., 2009. Estimating per-pixel thematicuncertainty in remote sensing classifications. International Journal of RemoteSensing 30, 209–229.Dungan, J.L., Kao, D., Pang, A., 2002. The uncertainty visualization problem in remote sensing analysis. In: Proceedings of IEEE International Geoscience and Remote Sensing Symposium, Toronto, Canada, June 2, 2002, pp. 729–731.Edsall, R.M., 2003. The parallel coordinate plot in action: design and use for geographic visualization. Computational Statistics and Data Analysis 43, 605–619.Foody, G.M., Atkinson, P.M., 2002. Uncertainty in Remote Sensing and GIS. Wiley Blackwell, London.Foody, G.M., 1996. Approaches for the production and evaluation of fuzzy land cover classifications from remotely-sensed data. International Journal of Remote Sensing 17, 1317–1340.Gahegan, M., Takatsuka, M., Wheeler, M., amd Hardisty, F., 2002. Introducing geo VISTA studio: an integrated suite of visualization and computational methods for。