外文翻译基于SSH的web技术介绍
基于SSH框架的Web网站设计与实现英文文献

ORIGINAL ARTICLEEffects of artificially intelligent tools on pattern recognitionTanzila Saba • Amjad RehmanInt. J. Mach. Learn. & Cyber. (2013) 4:155–162DOI 10.1007/s13042-012-0082-zReceived: 14 July 2011 / Accepted: 27 January 2012 / Published online: 10 February 2012 Springer-Verlag 2012AbstractPattern recognition is classification process thatattempts to assign each input value to one of a given set of classes. The process of pattern recognition in the state ofart has been achieved either by training of artificiallyintelligent tools or using heuristic rule based approaches.The objective of this paper is to provide a comparativestudy between artificially trained and heuristics rule based techniques employed for pattern recognition in the state ofthe art focused on script pattern recognition. It is observed that mainly there are two categories of script pattern rec-ognition techniques. First category involves assistance ofartificial intelligent learning and next, is based on heuristic-rules for cursive script pattern segmentation/recognition.Accordingly, a detailed critical study is performed thatfocuses on size of training/testing data and implication ofartificial learning on script pattern recognition accuracy.Moreover, the techniques are described in details that are employed to identify character patterns. Finally, perfor-mances of different techniques on benchmark database arecompared regarding pattern recognition accuracy, errorrate, single or multiple classifiers being employed. Prob-lems that still persist are also highlighted and possible directions are set.Keywords Pattern recognition Features extraction Character segmentation Heuristic-rule based approaches Neural validation Genetic algorithm BenchmarkDatabase1 IntroductionPattern recognition is automatic machine recognition, cat-egorized according to the type of learning procedure usedto generate the output valu e. But what is pattern? ‘‘it isopposite of chaos; an entity, vaguely defined, that could begiven a label’’. For instance, a pattern could be a fingerprintimage, a handwritten cursive word, a human face, or aspeech signal.Given a pattern, its recognition/classificationis either based on artificially trained tools that may besupervised/unsupervised classification [ 1 – 6 ] or based onheuristic rule based approaches [ 7 , 8 ].Handwriting pattern recognition is the one of specialcase of pattern recognition that has several real life appli-cations such as postal systems, check reading systems,signature verification and so on [ 9 , 10]. Efficiency of pat-tern recognition is heavily depended on character seg-mentation accuracy and processing time. To enhancepattern recognition accuracy, researchers are working continually in this domain and have published tremendoustechniques. These techniques are supported by artificiallyintelligent or heuristic rule based approaches. However,character segmentation techniques could be divided intosegmentation-free, segmentation-based and perception-oriented methods [ 11– 13]. Generally, there are three mainsegmentation approaches: external, internal and segmen-tation-free/holistic approach [ 14, 15]. Character segmen-tation is an operation to extract a pattern of individualsymbol from sequence of characters/word images [16].However, symbols could be valid or invalid when identi-fied as pattern (character). Error rates in recognition systemare mainly affected by this phenomenon. The challenges inhandwritten cursive character segmentation process are dueto high variability and intrinsic ambiguity of handwritten cursive letters [11, 12]. Hence, it is hard to obtain perfectcharacter segmentation results of cursive handwritten pat-terns. Actually, some systems could tolerate over-seg-mentation up to recognition stage [2 ].Handwritten cursive word images naturally consist ofuseless details. To enhance accuracy, handwriting effectsare normalized by means of preprocessing techniques.There are several strategies to simplify handwrittenimages and to suppress extra detail such as thinning,slant and skew correction, noise removal and lineremoval [ 17–19]. Using graph-based representation[ 20, 21] handwritten images convey basic information onthe word shape without retaining useless details (seeFig. 1 ).Character segmentation as part of cursive handwritingrecognition system has examined in [ 14, 15, 22–26]. Spe-cific surveys about character segmentation techniques forboth printed and cursive characters have been reported in[ 16]. Global character segmentation overviews particularlypresent the segmentation of cursive handwritten wordsinto two sections which are segmented as preprocess toword recognition and segmentation-recognition constrainedto database size. However, recent published research oncharacter segmentation of cursive handwriting has not yetsurveyed presence of artificial learning/non-learning tech-niques and size of train/test data. Hence, this paper com-pares how artificially trained tools affect the performanceof character segmentation process as well as what are theeffects of size of train/test database. Some of segmentationtechniques in this survey are part of handwriting recogni-tion system and the others are as an independent charactersegmentation system.The organization of the rest of the paper is as such: Sect.2 presents learning and non-learning character segmenta-tion systems separately. The systems are described step bystep and their performance is evaluated regarding the use ofbenchmark/non-benchmark database, training and testingdata size. In Sect. 3 , comparisons are performed into twomain categories that include database size and existence/non-existence of artificially learning process. Differentlogics have been used by the learning processes forexample, neural network, fuzzy logic, support vectormachines or genetic algorithm. Finally, conclusions aredrawn in the last section.2 Segmentation approachesSince last few decades many researchers have proposednovel character segmentation techniques. However, thefirst common step is to find prospective segmentation point(PSP) heuristically [27]. Then to reduce over-segmentation,some of them include artificial neural network to identifycorrect/incorrect segmentation points and the othersemploy optimum path algorithm. This section describesseveral comprehensive approaches categorized into twoparts: artificially learned and non-learned system. The mainmotivation is to analyze how learning and non-learningsystem affect the techniques’ performance. Additionally,size of database for training and testing the techniques isalso taken into account.2.1 Learning systemTo solve variability of cursive handwritten words, a systemwith artificial learning ability could benefit the charactersegmentation process. Ordinarily, segmentation algorithmwith learning ability reduces over-segmentation points bytrained neural networks on some data set of correct/incor-rect segmentation points. Moreover, ANN could be used togenerate segmentation points [ 2 , 28, 29– 31]. This sectiondescribes the valuable achievement of character segmen-tation involving learning processes.Few researchers divide segmentation process into twocomponent, simple heuristic segmenter algorithm [ 32, 7 , 8 ,27] and feed-forward neural network using back-propaga-tion algorithm [ 3 , 4 ]. Heuristic segmentation algorithmseeks minima or arcs between letters, then ‘‘hole-seeking’’is used to handle incorrect segmentation on letters like ‘‘a’’,‘‘u’’ and ‘‘o’’. Last step is to check segmentation pointposition that closest or greater than average width of somewords. Incorrect segmentation is reduced by using neuralcomponent. Experiments utilize approximately 50 hand-written words taken from Griffith University andaccuracyrate up to 78 and 66% respectively for one and two writersare claimed.Blumenstein et al. [3 , 4 ] include preprocessing tomodify handwritten images into binary images usingOtsu’s thresholding algorithm. Samples for experiment aretaken from CEDAR database consist of postal address fromreal envelopes. The authors report accuracy rate up to 76%on cursive words from ‘‘BD/Cities’’ directory of CEDARbenchmark database. Neural network input layer composedof 42 inputs (14 9 3 matrix of pixel). Best result rate is81.21% fo r identification of 3,126 segmentation points’pattern after neural network is trained with 32,034 trainingpatterns; training pattern consists of correct and incorrectsegmentation points.Verma and Gader [13] fuse three techniques MUMLP,GUMLP and MURBF with two different conventionalsegmentation conjunctions to back-propagation and radialbased function neural network. Modification of conven-tional borda count, based on rank and confidence (cf)value, used to combine these three techniques depicted inEq. .MUMLP is over segmentation based on multilayerperceptron, trained by using back propagation and dynamicprogramming. GUMLP, similar to MULP system, but twoimportant differences which GUMLP has are: first, withoutneural network based char compatibility; next, usingheuristic algorithm from [ 5 ]. MURBF use neural networkradial based function. Optimum investigation solution isachieved from 1,000 random distribution from CEDARdatabase. Finally, borda count algorithm yields 91%recognition rate.In the same way, Blumenstein and Verma [ 34] generatepossible segmentation points (PSP) using heuristic feature-based segmenter. Incorrect PSP is removed by calculatingconfidence segmentation values using three neural net-works. First, network provides correct and incorrect con-fidence segmentation value. Next two compute leftcharacter confidence (LCC) and center character confi-dence (CCC) values. High confidence value means goodcandidate for segmentation. Confidence values from threeneural networks calculated by Eq. 2 .All experiments are conducted on CEDAR benchmark database directory ‘‘BD/Cities’’. Errors are distributed into three categories over-segmentation 7.47%, missed 2.04%,bad 11.64% and bad ? correct anchorage 6.3%.Verma [33] performs character segmentation starting from baseline detection and generate over-segmentation points, extracted left, right characters and finally evaluate,join confidence values. Baseline is found by significant changes in the horizontal histogram density until reached the bottom image.Possible segmentation points are cal-culated using heuristic algorithm based on upper and lower word contours, holes, upper and lower contour minima,vertical density histogram and confidence assignment [34].Character segmentation problems that include part of another character are solved by tracing connected black pixels boundary of first and second segmentation points as illustrated in Fig. 2 . To evaluate confidence values, three neural networks are employed [ 13]. 300 words of ‘‘BD/Cities’’ d irectory of CEDAR benchmark database are experimented and accuracy rate 84.87% is claimed.Cheng et al. [35] employ cursive handwritten word images segmenter by means of previous work that is fea-ture-based heuristic segmenter algorithm with segmenta-tion point validation (SPV) [ 5 ], validated LC and CC are also implemented [ 34]. Finally, modified direction feature (MDF) is added to enhance entire segmentation process[ 36]. MDF calculate changes from background to fore-ground pixel vertically and horizontally using two values,Left and Destination Transition (LT, DT). Overlapped and closest characters problem is solved using segmentation path direction (SPD)—a character extraction method. SPD used base-fit line to determine a foreground pixel act as starting point on top of base-fit line, see Fig. 3 . Based on SPD, accurate character segmentation rate for 317 words is 95.27%.Cheng and Blumenstein enhance heuristic segmenter (EHS) to improve feature-based heuristic segmentation (FHS) algorithm by adding ligature detection [ 35]. Actu-ally, FHS failed to determine segmentation point on overlapped characters. T o determine ligature, EHS first calculate upper and lower baseline position to find main body. Then representation of modified vertical histogram counted distance between first black and last pixel in main body. Following histogram normalization, ligatures are determined by lowest value. EHS is examined using CEDAR database with 317 words. Error segmentation rates compared between FHS and EHS for over-segmented-missed, bad segmentation are presented in Table1 .Veloso et al. [37] propose hypothesized character seg-mentation based on natural segmentation point and ligature detection. Natural segmentation referred to such character that are not connected and determined using histogram projection taken from five different angles. Ligature can-didate obtained from morphological operations of opening and closing. While to search for the best structuring ele-ments, genetic algorithm is applied to determine ligature inthe set of training words. Finally Viterbi’s algorithm used to determine qualified ligature. Figure 4 shows word seg-mentation example using this technique.Cheng and Blumenstein [38] improve previous work proposed in [ 39] to determine EHS with segmentation based on neural network and PSP validation to improve character segmentation of cursive script. SPC, LCV and CCV is used for the validation of segmentation point [33].To get PSP more precisely, EHS use ligature detection and neural assistance. Ligatures are determined by minimum value of modified vertical histogram in baseline. Neuralassistant is sought to enhance accuracy. CEDAR bench-mark database is used for training and experiment step.Error rate using EHS with neural assistant are such as over-segmented 7.37%, missed 0.1%, and bad errors 6.79%.Recently, Saba et al. [ 28] present a simple and effective character segmentation approach. Following preprocessing,characters geometric features are explored for over-segmentcharacters. However, to identify incorrect segmentation,neural assistance is sought. Experiments are conducted on IAM benchmark database and character segmentation accuracy rate up to 91.21% is reported.2.2 Heuristics rule based systemActually, there are several features in cursive handwritten words that are unaffected by handwriting variability, for example loops/holes, ligatures, ascenders and descenders.Some of researchers used these features to determine PSP using heuristic algorithm [1 , 40, 7 , 8 ]. In this regards,several approaches exhibit dissimilar results. Thissection served briefly general steps of each algorithm as well as its reported result.Han and Sethi [40] introduce heuristic segmenter based on geometrical features to classify letters in two groups,regular and singular. Segmentation scheme divided char-acter into six classes. Letters boundaries identification based on several global coefficient, reference lines and words zone. Segmentation process started with two branch path, first path seek reference line and zone, to calculate ENC and EWC. Second path started by binary image to extract singular and regular features. Input image in second path is preprocessed into binary and skeleton image. Seg-mentation system tested using cursive handwritten of postal address consisted of 1,119 words and reported accurate character segmentation rate is 85.7%.Nicchiotti and Scagliola [ 41 ] propose simple and effective segmentation methods. Initia lly, images are preprocessed to fix noise, slant and skew [ 17 , 18 ]. Segmentation algorithm has three steps: detect PSP, st roke generation and stroke analysis. Possible segmentation points detection based on contour minima and holes features. On first step, ligature determined by finding minimum value of upper, medium and lower contours. Holes detection prevented segmentation on hole and could add segmentation points on its both sides.Closest PSP merged into a single PSP. Second step, seek best path to cut images into strokes, if cutting horizontally hit black pixel over a certain length, cut direction changed in the range ±45 around vertical one. Finally strokes from step two checked for consistency, incons istence strokes are discarded or merged with adjacent consistence one. To test algorithm, 850 words are used in the TRAIN directory of CEDAR database. Accurate character segmentation up to 86.9% is observed and ligature detection accuracy is 97.9%.Yanikoglu and Sandon [32] propose segmentation determined by evaluating cost function at each point along baseline using linear programming. First, single character extracted based on connected pixel using a region growing algorithm. Then, each point along baseline evaluated with cost function, linear programming used to determine weight. First lowest cost point is chosen as segmentation point. Using this point, separator line with certain angle cut the images. The angle is taken from 30, - 20, - 10, 0, 10,20, and 30 based on dominant slant. Experiment performed on 750 words is written by 10 writers. Segmentation per-formance up to 92% is claimed.Verma [42] propose novel contour code feature in conjunction with rule-based segmentation based on contour characteristic like loop, hat shape, etc. Baselinecomputa-tion is performed to determine upper, lower, middle,ascender and descenders line. Possible segmentation points are detected by heuristic algorithm to observe changes on vertical histogram projection. Finally, correct segmentation is finalized by validating PSP with rules that detected loop,hat shape (‘^’ or ‘v’), remove two closest PSP and add segmentation point between two PSP that cross threshold value. Rule-based segmentation approach is tested on CEDAR database with test sample size of 1,200 words.Errors rate is reported according to standard criteria that include over-segmentation 10.02%, missed segmentation 0.2% and finally, bad-errors 8.7% observed.Blumenstein et al. [ 3 , 4 ] employ heuristic approach to segment hand-printed and cursive handwritten words. Authors proposed a heuristic algorithm to locate hole location, minima detection and projection pixel density vertically. Characters’ segmentation accuracy up to 76.52% is reported.Recently Rehman et al. [8 ] propose heuristic approach for character segmentation of cursive handwritten words.Accordingly, several heuristics criteria are prescribed to locate character boundaries. To apply proposed heuristic mechanism of character segmentation, characters are divided into three broad categories. The first category includes characters with up/down cusp of characters’ shape such as u, v, w, m, n and second category include char-acters composed of holes/semi holes such as a, b, c, d, x,etc. Finally, third category of characters is without holes/cusp such as t, l, r, etc. Researchers performed experiments on IAM benchmark database and report high accuracy,speed as no training required.3 Performance comparisonThis section performs comparison among various character segmentation techniques reported in the state of art based on database used; sample training/testing size, prepro-cessing involvement, existence/non-existence of artificially learning and segmentation accuracy.3.1 Database sizePerformance of systems heavily depends on the size of database. Some segmentation algorithms have good results on small or medium database, but not encouraging results for large database. Table 2 exhibits performance compar-ison based on various factors. Related information consists of domain/database (DB), number of words (Sample),preprocessing (Pre.), and techniques employed. Number of trained/tested words is also an important criteria to evaluate the techniques and therefore split into small, medium and large numbers respectively with tens of words, with hun-dred of words and with thousands of words as criteria mentioned in [16].Preprocessing could be thresholding (t), slant (sl), ske (sk) correction or thinning/skeleton (th) for the methods briefly described as feature-based (FB), enhanced feature-based(EHB), rule-based (RB), geometric-based (GB),artificial neural network (ANN), neural assistant (NA),genetic algorithm (GA), Viterbi’s algorithm (VA), char-acter extraction (CE), Linear programming (LP), Contour-following algorithm (CFA), Bayesian belief networks (BBN), Naı ¨ve bayes (NB). Noted for (RR) means this value is recognition rates.Some researchers do not report number of experimented words. Therefore, such work is reported in uncategorizedpart. Information on preprocessing step is also missing, so it is hard to know whether the sample is slanted or skewed.This information is important because for difficult words segmentation may lead many problems that may decrease segmentation accuracy. Some researcher use slant separa-tor to cut the slanted word images [ 43]. To draw the graph comparison (Fig. 5 ), average of segmentation rates in each category is calculated.In Fig. 5 , segmentation rate is still around 86%. In medium database, it could reach almost 96% but this case generally does not represent real life problems. Correlation between segmentation and recognition result could be closest, if researcher could improve segmentation rates, it could reach up to 90–95%; it is good enough to solve word recognition in real problem domain.3.2 Existence of learning systemDue to invariability of cursive script, few researchers implement segmentation validation using neural network or employ neural assistant to add segmentation points. On the other hand, feature based approaches are investigated over segmentation of cursive handwritten images. The character segmentation accuracy rates of these two tech-niques are quite encouraging. Table 3 presents perfor-mance comparisons of this survey.Figure 6 shows that segmentation rates of non-learning system are greater than learning system but this is not evidence that non-learning system is better than learning system. This graph just show average segmentation rates taken from several researches. Comprehensive experiment is needed to know how learning system affect segmentation rates. Good segmentation rates are claimed in [ 44], tested on Arabic and Latin text with no slanted words, using joined three by three such as ‘‘valley-summit-valley’’ of upper contour.4 Conclusion and future directionsThis work has presented a survey of cursive script seg-mentation and comparison of character segmentation rates categorized by number of words and existence/non-exis-tence of artificial learning system. Description of several segmentation techniques are provided along with train/test data and segmentation rates in the state of the art are compared. However, it is observed that, in most of the cases training/testing data is extracted from CEDAR benchmark database. Character segmentation using artifi-cially intelligence basically validates characters candidates obtained fromover-segmentation step by calculating confidence values. Artificially learned techniques on cor-rect and incorrect segmentation points validate theseprospective segmentation points. Although, few research-ers also employ more than one learning algorithms to achieve satisfactory results, however, still there is need to investigate these results in large databases. On one hand, artificially trained system in large amount of data couldlead the problem and are also time consuming. On the other hand, although heuristic segmenters are used in segmen-tation process to over-segment the words and to locate the correct character boundaries, but it is observed that neural assistant may reduce incorrect segmentation.prospective segmentation points. Although, few research-ers also employ more than one learning algorithms to achieve satisfactory results, however, still there is need to investigate these results in large databases. On one hand,artificially trained system in large amount of data could lead the problem and are also time consuming. On the other hand, although heuristic segmenters are used in segmen-tation process to over-segment the words and to locate the correct character boundaries, but it is observed that neural assistant may reduce incorrect segmentation.Segmentation-recognition methods are most successful approaches in large database. It is interesting because recently many researchers investigate segmentation prob-lems using artificial intelligent approaches. Over-segmen-tation using geometric features is capable of accurately locating the letter boundaries in large amount of data.Obviously, there are number of error segmentation, due to ambiguity of segmenting a sequence of specific letters.Although, good segmentation rates are claimed in [ 44], yet it needs to be tested on difficult cursive handwriting (with slanted, skewed, merged, etc.) or use of common bench-mark database like CEDAR, IAM. Investigation upondifficult cursive handwriting to solve ambiguity is needed to improve segmentation rates. As future works, combined best achievement of non-learning and learning approaches is interesting. Authors have investigated effects of exis-tence/non-existence of learning system on segmentation,recognition accuracy of cursive script. Additionally, size of training/test set is investigated. However, as future work,comprehensive experiments using same samples and number of words is desired to confirm real achievement in this domain.Acknowledgments This research work is partially supported by TWAS fellowship cycle (2010) and Higher Education Commission of Pakistan. The authors are sincerely thankful to Prof. Dr. Ghazali Sulong and Prof. Dr. Dzulkifli Mohammad Faculty of Computer Science and Information Systems, Universiti T eknologi Malaysia (UTM) Skudai Johor Malaysia for support, guidance and layout of this research.References1. Rehman A, Kurniawan F, Dzulkifli M (2009) Neuro-heuristic approach for segmenting cursive handwritten words. Int J Inf Process 3(2):37–462. Rehman A, Dzulkifli M (2008) A simple segmentation approach for unconstrained cursive handwritten words in conjunction of neural network. Int J Image Process 2(3):29–353. Blumenstein M, Verma B (1999) Neural-based solutions for the segmentation and recognition of difficult handwritten words from a benchmark database. Bangalore, India, pp 281–2844. Blumenstein M, Verma B (1999) A new segmentation algorithm for handwritten word recognition. Int Jt Conf Neural Netw(IJCNN 0 99) 4:2893–28985. Bozinovic RM, Srihari SN (1989) Off-line cursive script word recognition. IEEE Trans Pattern Anal Mach Intell 11:68–836. Wang X, Chena A, Feng H (2011) Upper integral network with extreme learning mechanism. Neurocomputing 74(16):2520–25257. Saba T, Sulong G, Rahim S, Rehman A (2010) On the segmen-tation of multiple touched characters: a heuristics approach.Lecturer Notes in Computer Science (LNCS). Springer, Berlin,p 5408. Rehman A, Kurniawan F, Dzulkifli M (2008) Off-line cursive handwriting segmentation, a heuristic rule-based approach. J Inst Math Comput Sci (Computer Science Series) Kolkata, India, vol. 19(2), pp 135–1399. Shafry R, Rehman A, Faizal-Ab-Jabal M, Saba T (2011) Close spanning tree approach for error detection and correction for 2D CAD drawing. Int J Acad Res 3(4):525–53510. Wang XZ, Zhai JH, Lu SX (2008) Induction of multiple fuzzy decision trees based on rough set technique. Inf Sci 178(16):3188–320211. Kurniawan F, Rehman A, Dzulkifli M (2009) Contour vs. non-contour based word segmentation from handwritten text lines. An experimental analysis. Int J Digit Content Technol Appl 3(2):127–13112. Rehman A, Saba T (2011) Performance analysis of segmentation approach for cursive handwritten word recognition on benchmark database. Digit Signal Process 21:486–49013. Verma B, Gader P (2000) Fusion of multiple handwritten word recognition techniques. Neural networks for signal processing. In: Proceedings of IEEE signal processing society workshop, vol 2,pp 926–934。
基于SSH框架的电子商务网站主要用到的技术
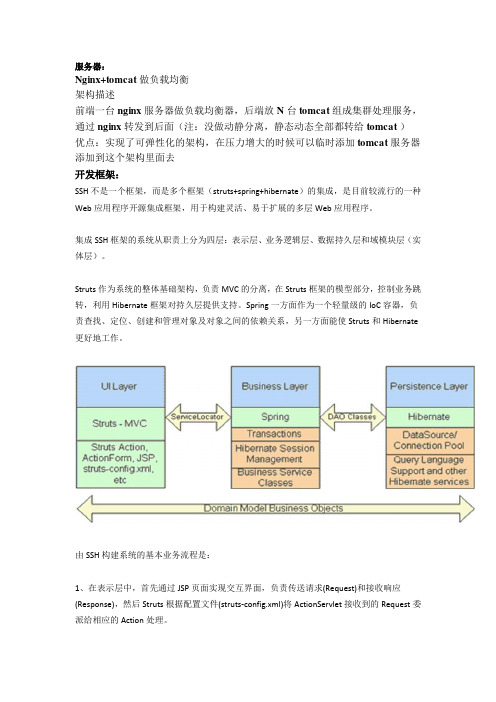
服务器:Nginx+tomcat 做负载均衡架构描述前端一台nginx 服务器做负载均衡器,后端放N 台tomcat 组成集群处理服务,通过nginx 转发到后面(注:没做动静分离,静态动态全部都转给tomcat )优点:实现了可弹性化的架构,在压力增大的时候可以临时添加tomcat 服务器添加到这个架构里面去开发框架:SSH不是一个框架,而是多个框架(struts+spring+hibernate)的集成,是目前较流行的一种Web应用程序开源集成框架,用于构建灵活、易于扩展的多层Web应用程序。
集成SSH框架的系统从职责上分为四层:表示层、业务逻辑层、数据持久层和域模块层(实体层)。
Struts作为系统的整体基础架构,负责MVC的分离,在Struts框架的模型部分,控制业务跳转,利用Hibernate框架对持久层提供支持。
Spring一方面作为一个轻量级的IoC容器,负责查找、定位、创建和管理对象及对象之间的依赖关系,另一方面能使Struts和Hibernate 更好地工作。
由SSH构建系统的基本业务流程是:1、在表示层中,首先通过JSP页面实现交互界面,负责传送请求(Request)和接收响应(Response),然后Struts根据配置文件(struts-config.xml)将ActionServlet接收到的Request委派给相应的Action处理。
2、在业务层中,管理服务组件的Spring IoC容器负责向Action提供业务模型(Model)组件和该组件的协作对象数据处理(DAO)组件完成业务逻辑,并提供事务处理、缓冲池等容器组件以提升系统性能和保证数据的完整性。
3、在持久层中,则依赖于Hibernate的对象化映射和数据库交互,处理DAO组件请求的数据,并返回处理结果。
采用上述开发模型,不仅实现了视图、控制器与模型的彻底分离,而且还实现了业务逻辑层与持久层的分离。
这样无论前端如何变化,模型层只需很少的改动,并且数据库的变化也不会对前端有所影响,大大提高了系统的可复用性。
JSP技术与SSH技术中英文对照外文翻译文献

中英文对照外文翻译(文档含英文原文和中文翻译)JSP technology and mainstream open-source framework for JA V AEE1.JSP ProfileJSP (Java Server Pages) is initiated by Sun Microsystems, Inc., with many companies to participate in the establishment of a dynamic web page technical standards. JSP technology somewhat similar to ASP technology, it is in the traditional HTML web page document (*.htm, *. html) to insert the Java programming paragraph (Scriptlet) and JSP tag (tag), thus JSP documents (*.jsp). Using JSP development of the Web application is cross-platform that can run on Linux, is also available for other operating systems.JSP technology to use the Java programming language prepared by the category of XML tags and scriptlets, to produce dynamic pages package processing logic. Page also visit by tags and scriptlets exist in the services side of the resources of logic. JSP page logic and web page design and display separation, support reusablecomponent-based design, Web-based application development is rapid and easy.Web server in the face of visits JSP page request, the first implementation of the procedures of, and then together with the results of the implementation of JSP documents in HTML code with the return to the customer. Insert the Java programming operation of the database can be re-oriented websites, in order to achieve the establishment of dynamic pages needed to function.JSP and Java Servlet, is in the implementation of the server, usually returned to the client is an HTML text, as long as the client browser will be able to visit.JSP 1.0 specification of the final version is launched in September 1999, December has introduced 1.1 specifications. At present relatively new is JSP1.2 norms, JSP2.0 norms of the draft has also been introduced.JSP pages from HTML code and Java code embedded in one of the components. The server was in the pages of client requests after the Java code and then will generate the HTML pages to return to the client browser. Java Servlet JSP is the technical foundation and large-scale Web application development needs of Java Servlet and JSP support to complete. JSP with the Java technology easy to use, fully object-oriented, and a platform-independent and secure mainly for all the characteristics of the Internet. JSP technology strength: (1) time to prepare, run everywhere. At this point Java better than PHP, in addition to systems, the code not to make any changes.(2) the multi-platform support. Basically on all platforms of any development environment, in any environment for deployment in any environment in the expansion. Compared ASP / PHP limitations are obvious. (3) a strong scalability. From only a small Jar documents can run Servlet / JSP, to the multiple servers clustering and load balancing, to multiple Application for transaction processing, information processing, a server to numerous servers, Java shows a tremendous Vitality. (4) diver sification and powerful development tools support. This is similar to the ASP, Java already have many very good development tools, and many can be free, and many of them have been able to run on a variety of platforms under. JSP technology vulnerable:(1) and the same ASP, Java is the advantage of some of its fatal problem. It is precisely because in order to cross-platform functionality, in order to extreme stretching capacity, greatly increasing the complexity of the product. (2) Java's speed is class to complete the permanent memory, so in some cases by the use of memory compared to the number of users is indeed a "minimum cost performance." On the other hand, it also needs disk space to store a series of. Javadocuments and. Class, as well as the corresponding versions of documents.2. J2EE Development FrameworkJava2 Enterprise Edition middleware unified ideology played a significant role. For example, J2EE for distributed transaction management, directory services and messaging services provide a standard programming interface. J2EE-based -Java2Standard Edition (J2SE), successfully access for Java provides a standard relational database.But, as this article "J2EE programming of the lack of support", as mentioned, J2EEplatform does not provide a satisfactory application programming model. Sun and some of the major application server vendors wanted to use the development tools to reduce the complexity of J2EE development, but these tools are no other outstanding JA V A development tools, which have advanced refactoring tools, and. NET platform compared, J2EE tool support appeared to be very inferior.Many J2EE development tools automatically generate the code for the same complex as the tools themselves. In many small-scale J2EE open source community developers chose another way of development - some can be difficult to reduce the development of J2EE development framework, the more popular such as: Struts, Hibernate, and Spring Framework, J2EE project types in many of today they play an important the role.2.1 Spring FrameworkThe Spring Framework is an open source application framework for the Java platform.The first version was written by Rod Johnson who released the framework with the publication of his book Expert One-on-One J2EE Design and Development in October 2002. The framework was first released under the Apache 2.0 license in June 2003. The first milestone release, 1.0, was released in March 2004, with further milestone releases in September 2004 and March 2005. The Spring 1.2.6 framework won a Jolt productivity award and a JAX Innovation Award in 2006. Spring 2.0 was released in October 2006, and Spring 2.5 in November 2007. In December 2009 version 3.0 GA was released. The current version is 3.0.5.The core features of the Spring Framework can be used by any Java application, but there are extensions for building web applications on top of the Java EE platform.Although the Spring Framework does not impose any specific programming model, it has become popular in the Java community as an alternative to, replacement for, or even addition to the Enterprise JavaBean (EJB) model.Modules The Spring Framework comprises several modules that provide a range of services:Inversion of Control container: configuration of application components and lifecycle management of Java objectsAspect-oriented programming: enables implementation of cross-cutting routines Data access: working with relational database management systems on the Java platform using JDBC and object-relational mapping toolsTransaction management: unifies several transaction management APIs and coordinates transactions for Java objectsModel-view-controller: an HTTP and Servlet-based framework providing hooks for extension and customizationRemote Access framework: configurative RPC-style export and import of Java objects over networks supporting RMI, CORBA and HTTP-based protocols including web services (SOAP)Convention-over-configuration: a rapid application development solution for Spring-based enterprise applications is offered in the Spring model.Batch processing: a framework for high-volume processing featuring reusable functions including logging/tracing, transaction management, job processing statistics, job restart, skip, and resource managementAuthentication and authorization: configurable security processes that support a range of standards, protocols, tools and practices via the Spring Security sub-project (formerly Acegi Security System for Spring).Remote Management: configurative exposure and management of Java objects for local or remote configuration via JMXMessaging: configurative registration of message listener objects for transparent message consumption from message queues via JMS, improvement of message sending over standard JMS APIsTesting: support classes for writing unit tests and integration testsInversion of Control container Central to the Spring Framework is its Inversion of Control container, which provides a consistent means of configuring and managing Java objects using callbacks. The container is responsible for managing objectlifecycles: creating objects, calling initialization methods, and configuring objects by wiring them together.Objects created by the container are also called Managed Objects or Beans. Typically, the container is configured by loading XML files containing Bean definitions which provide the information required to create the beans.Objects can be obtained by means of Dependency lookup or Dependency injection. Dependency lookup is a pattern where a caller asks the container object for an object with a specific name or of a specific type. Dependency injection is a pattern where the container passes objects by name to other objects, via either constructors, properties, or factory methods.In many cases it's not necessary to use the container when using other parts of the Spring Framework, although using it will likely make an application easier to configure and customize. The Spring container provides a consistent mechanism to configure applications and integrates with almost all Java environments, from small-scale applications to large enterprise applications.The container can be turned into a partially-compliant EJB3 container by means of the Pitchfork project. The Spring Framework is criticized by some as not being standards compliant. However, Spring Source doesn't see EJB3 compliance as a major goal, and claims that the Spring Framework and the container allow for more powerful programming models.Aspect-oriented programming framework The Spring Framework has its own AOP framework which modularizes cross-cutting concerns in aspects. The motivation for creating a separate AOP framework comes from the belief that it would be possible to provide basic AOP features without too much complexity in either design, implementation, or configuration. The SAOP framework also takes full advantage of the Spring Container.The Spring AOP framework is interception based, and is configured at runtime. This removes the need for a compilation step or load-time weaving. On the other hand, interception only allows for public or protected method execution on existing objects at a join point.Compared to the AspectJ framework, Spring AOP is less powerful but also less complicated. Spring 1.2 includes support to configure AspectJ aspects in the container. Spring 2.0 added more integration with AspectJ; for example, the pointcut language is reused and can be mixed with SpAOP-based aspects. Further, Spring 2.0 added aSpring Aspects library which uses AspectJ to offer common Spring features such as declarative transaction management and dependency injection via AspectJ compile-time or load-time weaving. Spring Source also uses AspectJ for AOP in other Spring projects such as Spring Roo and Spring Insight, with Spring Security also offering an AspectJ-based aspect library.Spring AOP has been designed to make it able to work with cross-cutting concerns inside the Spring Framework. Any object which is created and configured by the container can be enriched using Spring AOP.The Spring Framework uses Spring AOP internally for transaction management, security, remote access, and JMX.Since version 2.0 of the framework, Spring provides two approaches to the AOP configuration:schema-based approach.@AspectJ-based annotation style.The Spring team decided not to introduce new AOP-related terminology; therefore, in the Spring reference documentation and API, terms such as aspect, join point, advice, pointcut, introduction, target object (advised object), AOP proxy, and weaving all have the same meanings as in most other AOP frameworks (particularly AspectJ).Data access framework Spring's data access framework addresses common difficulties developers face when working with databases in applications. Support is provided for all popular data access frameworks in Java: JDBC, iBatis, Hibernate, JDO, JPA, Oracle Top Link, Apache OJB, and Apache Cayenne, among others.For all of these supported frameworks, Spring provides these features:Resource management - automatically acquiring and releasing database resourcesException handling - translating data access related exception to a Spring data access hierarchyTransaction participation - transparent participation in ongoing transactionsResource unwrapping - retrieving database objects from connection pool wrappersAbstraction for BLOB and CLOB handlingAll these features become available when using Template classes provided by Spring for each supported framework. Critics say these Template classes are intrusive and offer no advantage over using (for example) the Hibernate API.. directly. In response, the Spring developers have made it possible to use the Hibernate and JPAAPIs directly. This however requires transparent transaction management, as application code no longer assumes the responsibility to obtain and close database resources, and does not support exception translation.Together with Spring's transaction management, its data access framework offers a flexible abstraction for working with data access frameworks. The Spring Framework doesn't offer a common data access API; instead, the full power of the supported APIs is kept intact. The Spring Framework is the only framework available in Java which offers managed data access environments outside of an application server or container. While using Spring for transaction management with Hibernate, the following beans may have to be configured.Transaction management framework Spring's transaction management framework brings an abstraction mechanism to the Java platform. Its abstraction is capable of working with local and global transactions (local transaction does not require an application server).working with nested transactions working with transaction safe points working in almost all environments of the Java platform In comparison, JTA only supports nested transactions and global transactions, and requires an application server (and in some cases also deployment of applications in an application server).The Spring Framework ships a Platform Transaction Manager for a number of transaction management strategies:Transactions managed on a JDBC ConnectionTransactions managed on Object-relational mapping Units of WorkTransactions managed via the JTA Transaction Manager and User Transaction Transactions managed on other resources, like object databasesNext to this abstraction mechanism the framework also provides two ways of adding transaction management to applications:Programmatically, by using Spring's Transaction TemplateConfiguratively, by using metadata like XML or Java 5 annotationsTogether with Spring's data access framework — which integrates the transaction management framework —it is possible to set up a transactional system through configuration without having to rely on JTA or EJB. The transactional framework also integrates with messaging and caching engines.The BoneCP Spring/Hibernate page contains a full example project of Spring used in conjunction with Hibernate.Model-view-controller framework The Spring Framework features its own MVC framework, which wasn't originally planned. The Spring developers decided to write their own web framework as a reaction to what they perceived as the poor design of the popular Jakarta Struts web framework, as well as deficiencies in other available frameworks. In particular, they felt there was insufficient separation between the presentation and request handling layers, and between the request handling layer and the model.Like Struts, Spring MVC is a request-based framework. The framework defines strategy interfaces for all of the responsibilities which must be handled by a modern request-based framework. The goal of each interface is to be simple and clear so that it's easy for Spring MVC users to write their own implementations if they so choose. MVC paves the way for cleaner front end code. All interfaces are tightly coupled to the Servlet API. This tight coupling to the Servlet API is seen by some as a failure on the part of the Spring developers to offer a high-level abstraction for web-based applications [citation needed]. However, this coupling makes sure that the features of the Servlet API remain available to developers while offering a high abstraction framework to ease working with said API.The Dispatcher Servlet class is the front controller of the framework and is responsible for delegating control to the various interfaces during the execution phases of a HTTP request.The most important interfaces defined by Spring MVC, and their responsibilities, are listed below:Handler Mapping: selecting objects which handle incoming requests (handlers) based on any attribute or condition internal or external to those requests Handler Adapter: execution of objects which handle incoming requestsController: comes between Model and View to manage incoming requests and redirect to proper response. It essentially is like a gate that directs the incoming information. It switches between going into model or view.View: responsible for returning a response to the client. It is possible to go straight to view without going to the model part. It is also possible to go through all three.View Resolver: selecting a View based on a logical name for the view (use is not strictly required)Handler Interceptor: interception of incoming requests comparable but not equalto Servlet filters (use is optional and not controlled by Dispatcher Servlet).Locale Resolver: resolving and optionally saving of the locale of an individual userMultipart Resolver: facilitate working with file uploads by wrapping incoming requestsEach strategy interface above has an important responsibility in the overall framework. The abstractions offered by these interfaces are powerful, so to allow for a set of variations in their implementations, Spring MVC ships with implementations of all these interfaces and together offers a feature set on top of the Servlet API. However, developers and vendors are free to write other implementations. Spring MVC uses the Java java.util.Map interface as a data-oriented abstraction for the Model where keys are expected to be string values.The ease of testing the implementations of these interfaces seems one important advantage of the high level of abstraction offered by Spring MVC. Dispatcher Servlet is tightly coupled to the Spring Inversion of Control container for configuring the web layers of applications. However, applications can use other parts of the Spring Framework—including the container—and choose not to use Spring MVC.Because Spring MVC uses the Spring container for configuration and assembly, web-based applications can take full advantage of the Inversion of Control features offered by the container. This framework allows for multi layering. It allows for the code to be broken apart and used more effectively in segments, while allowing the mvc to do the work. It allows for back and forth transmission of data. Some designs are more linear without allowing a forward and backward flow of information. MVC is designed very nicely to allow this interaction. It is used more than just in web design, but also in computer programming. It's very effective for web design. Basically allows a checks and balance system to occur where before being viewed it can be properly examined.Remote access framework Spring's Remote Access framework is an abstraction for working with various RPC-based technologies available on the Java platform both for client connectivity and exporting objects on servers. The most important feature offered by this framework is to ease configuration and usage of these technologies as much as possible by combining Inversion of Control and AOP.The framework also provides fault-recovery (automatic reconnection after connection failure) and some optimizations for client-side use of EJB remote statelesssession beans.Convention-Over-Configuration Rapid Application Development Spring Roo is Spring's Convention-over-configuration solution for rapidly building applications in Java. It currently supports Spring Framework, Spring Security and Spring Web Flow, with remaining Spring projects scheduled to be added in due course. Roo differs from other rapid application development frameworks by focusing on:The following diagram represents the Spring Framework Architecture2.2 Struts IntroductionApache Struts From Wikipedia, the free encyclopedia Jump to: navigation, search"Struts" redirects here. For the structural component, see strut. For other meanings, see strut (disambiguation).This article includes a list of references, but its sources remain unclear because it has insufficient inline citations.Please help to improve this article by introducing more precise citations where appropriate.Apache Struts is an open-source web application framework for developing Java EE web applications. It uses and extends the Java Servlet API to encourage developers to adopt a model-view-controller (MVC) architecture. It was originally created by Craig McClanahan and donated to the Apache Foundation in May, 2000. Formerly located under the Apache Jakarta Project and known as Jakarta Struts, it became a top level Apache project in 2005.Design goals and over view in a standard Java EE web application, the client will typically submit information to the server via a web form. The information is then either handed over to a Java Servlet that processes it, interacts with a database and produces an HTML-formatted response, or it is given to a Java Server Pages (JSP) document that inter mingles HTML and Java code to achieve the same result. Both approaches are often considered inadequate for large projects because they mix application logic with presentation and make maintenance difficult.The goal of Struts is to separate the model (application logic that interacts with a database) from the view (HTML pages presented to the client) and the controller (instance that passes information between view and model). Struts provides the controller (a servlet known as ActionServlet) and facilitates the writing of templatesfor the view or presentation layer (typically in JSP, but XML/XSLT and Velocity are also supported). The web application programmer is responsible for writing the model code, and for creating a central configuration file struts-config.xml that binds together model, view and controller.Requests from the client are sent to the controller in the form of "Actions" defined in the configuration file; if the controller receives such a request it calls the corresponding Action class that interacts with the application-specific model code. The model code returns an "ActionForward", a string telling the controller what output page to send to the client. Information is passed between model and view in the form of special JavaBeans. A powerful custom tag library allows it to read and write the content of these beans from the presentation layer without the need for any embedded Java code.Struts is categorized as a request-based web application framework Struts also supports internationalization by web forms, and includes a template mechanism called "Tiles" that (for instance) allows the presentation layer to be composed from independent header, footer, and content components History The Apache Struts Project was launched in May 2000 by Craig R. McClanahan to provide a standard MVC framework to the Java community. In July 2001, version 1.0 was released.Struts2 was originally known as WebWork 2. After having been developed separately for several years, WebWork and Struts were combined in 2008 to create Struts 2.Competing MVC frameworks Although Struts is a well-documented, mature, and popular framework for building front ends to Java applications, there are other frameworks categorized as "lightweight" MVC frameworks such as Spring MVC, Stripes, Wicket, Play!, and Tapestry. The new XForms standards and frameworks may also be another option to building complex web Form validations with Struts in the future.The WebWork framework spun off from Apache Struts aiming to offer enhancements and refinements while retaining the same general architecture of the original Struts framework. However, it was announced in December 2005 that Struts would re-merge with WebWork. WebWork 2.2 has been adopted as Apache Struts2, which reached its first full release in February 2007.In 2004 Sun launched an addition to the Java platform, called Java Server Faces (JSF). Aside from the original Struts framework, the Apache project previouslyoffered a JSF-based framework called Shale, which was retired in May 2009.In this section we will discuss about Architecture. Struts is famous for its robust Architecture and it is being used for developing small and big software projects.Struts is an open source framework used for developing J2EE web applications using Model View Controller (MVC) design pattern. It uses and extends the Java Servlet API to encourage developers to adopt an MVC architecture. Struts framework provides three key components:A request handler provided by the application developer that is used to mapped to a particular URI. A response handler which is used to transfer the control to another resource which will be responsible for completing the response.A tag library which helps developers to create the interactive form based applications with server pages Learn Struts 2.2.1 framework Struts provides you the basic infrastructure infra structure for implementing MVC allowing the developers to concentrate on the business logic The main aim of the MVC architecture is to separate the business logic and application data from the presentation data to the user.Here are the reasons why we should use the MVC design pattern They are resuable: When the problems recurs, there is no need to invent a new solution, we just have to follow the pattern and adapt it as necessary. They are expressive: By using the MVC design pattern our application becomes more expressive.1). Model: The model object knows about all the data that need to be displayed. It is model who is aware about all the operations that can be applied to transform that object. It only represents the data of an application. The model represents enterprise data and the business rules that govern access to and updates of this data. Model is not aware about the presentation data and how that data will be displayed to the browser.2). View: The view represents the presentation of the application. The view object refers to the model. It uses the query methods of the model to obtain the contents and renders it. The view is not dependent on the application logic. It remains same if there is any modification in the business logic. In other words, we can say that it is the responsibility of the view's to maintain the consistency in its presentation when the model changes.3). Controller: Whenever the user sends a request for something then it always go through the controller. The controller is responsible for intercepting the requests from view and passes it to the model for the appropriate action. After the action has been taken on the data, the controller is responsible for directing the appropriate viewto the user. In GUIs, the views and the controllers often work very closely together.The Struts framework is composed of approximately 300 classes and interfaces which are organized in about 12 top level packages. Along with the utility and helper classes framework also provides the classes and interfaces for working with controller and presentation by the help of the custom tag libraries. It is entirely on to us which model we want to choose. The view of the Struts architecture is given below: The Struts Controller Components Whenever a user request for something, then the request is handled by the Struts Action Servlet. When the ActionServlet receives the request, it intercepts the URL and based on the Struts Configuration files, it gives the handling of the request to the Action class. Action class is a part of the controller and is responsible for communicating with the model layer.The Struts View Components: The view components are responsible for presenting information to the users and accepting the input from them. They are responsible for displaying the information provided by the model components. Mostly we use the Java Server Pages (JSP) for the view presentation. To extend the capability of the view we can use the Custom tags, java script etc.The Struts model component The model components provides a model of the business logic behind a Struts program. It provides interfaces to databases or back- ends systems. Model components are generally a java class. There is not any such defined format for a Model component, so it is possible for us to reuse Java code which are written for other projects. We should choose the model according to our client requirement.2.3 Hibernate FrameWorkHibernate is an object-relational mapping (ORM) library for the Java language, providing a framework for mapping an object-oriented domain model to a traditional relational database. Hibernate solves object-relational impedance mismatch problems by replacing direct persistence-related database accesses with high-level object handling functions.Hibernate is free software that is distributed under the GNU Lesser General Public License Hibernate's primary feature is mapping from Java classes to database tables (and from Java data types to SQL data types). Hibernate also provides data query and retrieval facilities. Hibernate generates the SQL calls and attempts to relieve the developer from manual result set handling and object conversion and keep。
基于SSH2 框架的Web 系统的设计与实现
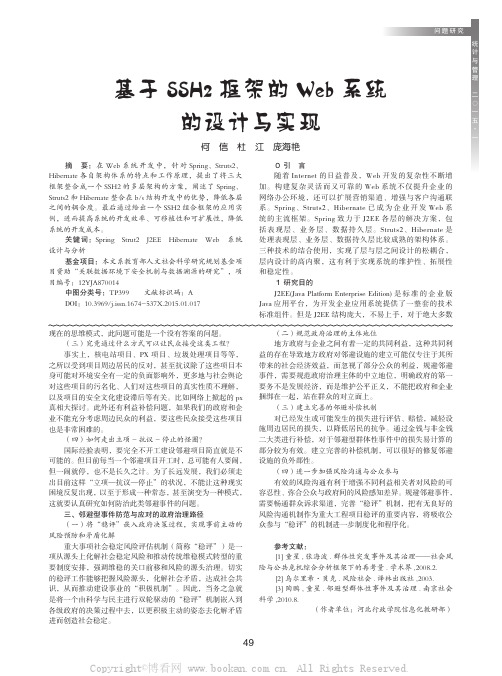
49
Copyright©博看网 . All Rights Reserved.
○
问题研究
统计与管源自理开发人员都是望而却步。在技术的不断演进的过程中,Web
的应用形成了鲜明的层次,在各层次上基于不同的技术产生
二
了成熟的架构体系,如:业务层的 Spring 框架体系、控制
一
层的 Struts 框架体系以及数据持久层的 Hibernate 框架体系。
五
如何整合利用这些成熟的框架,既能发挥各个架构的优势,
·
又能实现系统的“低耦合、高内聚”,成为本文讨论的重点
一
问题。
2 SS2H 技术架构
2.1 JSP 技术
JavaServer Page(JSP) 是一种动态的网页技术标准,它允
(二)规范政府治理的主体地位 地方政府与企业之间有着一定的共同利益,这种共同利 益的存在导致地方政府对邻避设施的建立可能仅专注于其所 带来的社会经济效益,而忽视了部分公众的利益,规避邻避 事件,需要规范政府治理主体的中立地位,明确政府的第一 要务不是发展经济,而是维护公平正义,不能把政府和企业 捆绑在一起,站在群众的对立面上。 (三)建立完善的邻避补偿机制 对已经发生或可能发生的损失进行评估、赔偿,减轻设 施周边居民的损失,以降低居民的抗争。通过金钱与非金钱 二大类进行补偿,对于邻避型群体性事件中的损失易计算的 部分较为有效。建立完善的补偿机制,可以很好的修复邻避 设施的负外部性。 (四)进一步加强风险沟通与公众参与 有效的风险沟通有利于增强不同利益相关者对风险的可 容忍性、弥合公众与政府间的风险感知差异。规避邻避事件, 需要畅通群众诉求渠道,完善“稳评”机制,把有无良好的 风险沟通机制作为重大工程项目稳评的重要内容,将吸收公 众参与“稳评”的机制进一步制度化和程序化。
基于SSH框架的Web网站设计与实现

基于SSH框架的Web网站设计与实现毕业论文(设计)基于SSH框架的Web网站设计与实现学生姓名:赵辰宇指导教师:张妍(讲师)专业名称:通信工程所在学院:信息工程学院6 月目录摘要....................................................................................................... Abstract ................................................................................................. 第一章前言 . 01.1 研究目的和意义 01.2 国内外研究现状 (1)1.3 研究内容与方法 (1)第二章可行性与需求分析 (3)2.1可行性分析 (3)2.2需求分析 (4)第三章设计技术与系统运行环境 (9)3.1设计技术 (9)3.2系统运行环境 (24)第四章系统设计 (26)4.1 官网系统 (26)4.2 图书出售系统 (27)4.3 办公系统 (32)4.4 数据库设计 (36)第五章系统实现 (39)5.1 系统架构 (39)5.2 持久层Hibernate实现 (44)5.3 控制层Struts实现 (45)5.4 业务层Spring实现 (50)第六章结论与建议 (54)致谢 (56)附录 (60)摘要本次毕业设计主要以电子商务为主题进行Web网站的设计与开发,在框架方面应用了现今比较主流的SSH框架。
设计背景以大连海洋大学出社为主题,并针对其进行设计与开发电子商务系统。
本次设计包括三个大模块分别为,后台管理OA系统、官网系统、图书出售系统,每个模块又包括了诸多小模块。
项目结构方面采用MVC架构大致上分为视图层、控制层、持久层,持久层细分为DAO层与业务层。
这种结构使得开发开发过程中逻辑清项目结构简明便于后期的功能扩展与二次开发。
SSH框架的Web网站设计与实现研究
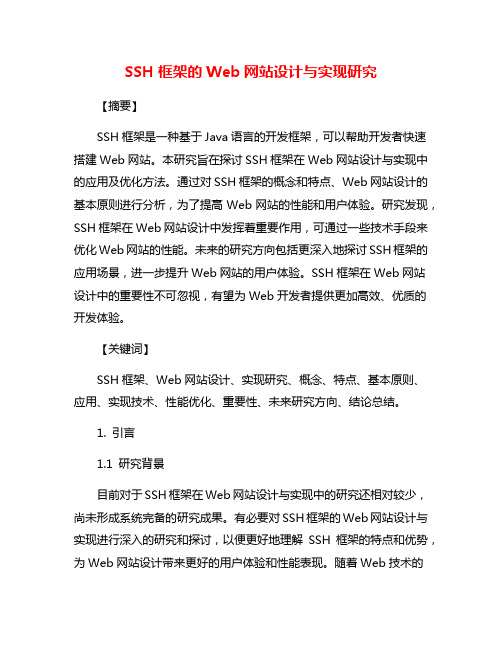
SSH框架的Web网站设计与实现研究【摘要】SSH框架是一种基于Java语言的开发框架,可以帮助开发者快速搭建Web网站。
本研究旨在探讨SSH框架在Web网站设计与实现中的应用及优化方法。
通过对SSH框架的概念和特点、Web网站设计的基本原则进行分析,为了提高Web网站的性能和用户体验。
研究发现,SSH框架在Web网站设计中发挥着重要作用,可通过一些技术手段来优化Web网站的性能。
未来的研究方向包括更深入地探讨SSH框架的应用场景,进一步提升Web网站的用户体验。
SSH框架在Web网站设计中的重要性不可忽视,有望为Web开发者提供更加高效、优质的开发体验。
【关键词】SSH框架、Web网站设计、实现研究、概念、特点、基本原则、应用、实现技术、性能优化、重要性、未来研究方向、结论总结。
1. 引言1.1 研究背景目前对于SSH框架在Web网站设计与实现中的研究还相对较少,尚未形成系统完备的研究成果。
有必要对SSH框架的Web网站设计与实现进行深入的研究和探讨,以便更好地理解SSH框架的特点和优势,为Web网站设计带来更好的用户体验和性能表现。
随着Web技术的不断升级和发展,SSH框架在Web网站设计中的应用前景将会更加广阔。
本研究旨在探究SSH框架在Web网站设计与实现中的应用,为Web技术的进步和发展提供一定的参考和借鉴。
1.2 研究目的研究目的是为了深入探讨SSH框架在Web网站设计中的应用,并分析其在实际项目中的优势和局限性。
通过对SSH框架的特点和Web 网站设计基本原则的理解,我们可以更好地把握如何利用SSH框架来构建高效、稳定、安全的Web网站。
本研究旨在探讨SSH框架在Web网站性能优化方面的方法,以及未来在这一领域的研究方向。
通过对SSH框架在Web网站设计与实现中的研究,可以为开发人员提供参考和指导,帮助他们更好地应用SSH框架,提升Web网站的用户体验和性能表现。
通过本研究的实践分析,可以为SSH框架在Web网站设计中的重要性提供具体案例支撑,并为未来相关研究方向提供有益的思路和启示。
外文翻译 Java技术及SSH框架和Jsp技术的介绍
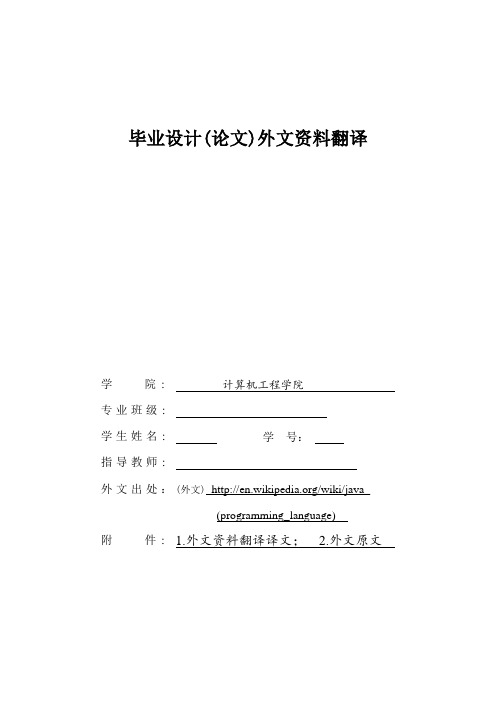
毕业设计(论文)外文资料翻译学院:计算机工程学院专业班级:学生姓名:学号:指导教师:外文出处:(外文)/wiki/java_(programming_language)附件:1.外文资料翻译译文; 2.外文原文Java技术及SSH框架和Jsp技术的介绍Java,是一种可以撰写跨平台应用软件的面向对象的程序设计语言,由当时任职太阳微系统的詹姆斯·高斯林(James Gosling)等人于1990年代初开发。
它最初被命名为Oak,目标设置在家用电器等小型系统的编程语言,来解决诸如电视机、电话、闹钟、烤面包机等家用电器的控制和通讯问题。
由于这些智能化家电的市场需求没有预期的高,Sun放弃了该项计划。
就在Oak 几近失败之时,随着互联网的发展,Sun看到了Oak在计算机网络上的广阔应用前景,于是改造了Oak,在1995年5月以“Java”的名称正式发布了。
Java 伴随着互联网的迅猛发展而发展,逐渐成为重要的网络编程语言。
Java编程语言的风格十分接近C++语言。
继承了C++ 语言面向对象技术的核心,Java 舍弃了C++语言中容易引起错误的指针(以引用取代)、运算符重载(operator overloading)、多重继承(以接口取代)等特性,增加了垃圾回收器功能用于回收不再被引用的对象所占据的内存空间。
在Java SE 1.5版本中Java又引入了泛型编程(Generic Programming)、类型安全的枚举、不定长参数和自动装/拆箱等语言特性。
Java不同于一般的编译运行计算机语言和解释执行计算机语言。
它首先将源代码编译成字节码(bytecode),然后依赖各种不同平台上的虚拟机来解释执行字节码,从而实现了“一次编译、到处执行”的跨平台特性。
不过,这同时也在一定程度上降低了Java程序的运行效率。
但在J2SE1.4.2发布后,Java的运行速度有了大幅提升。
与传统程序不同Sun公司在推出Java之际就将其作为一种开放的技术。
基于SSH架构的Web系统的开发方法
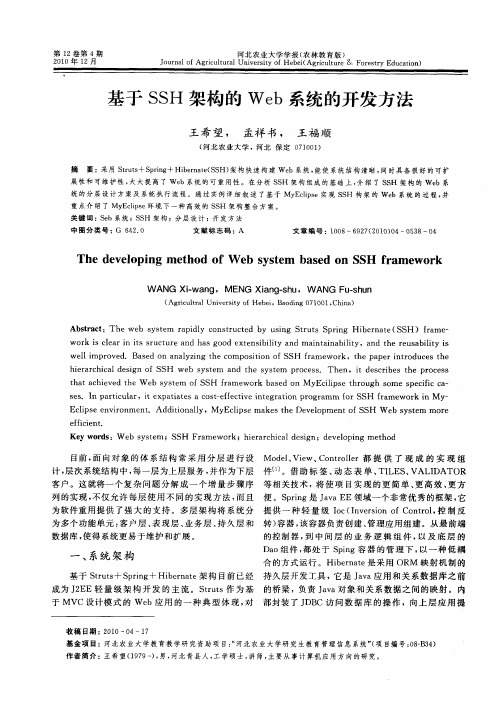
t a c i v d t e W e y t m fS H r me r a e n M y i p e t r u h s m e s e i c c — h ta h e e h b s s e o S fa wo k b s d o Ecl s h o g o p cf a i i S S I a tc l r te p ta e o te f c i e i t g a i n p o r mm o S fa wo k i y e . n p riu a ,i x a i t s a c s - fe tv n e r t r g a o f rS H r me r n M —
The d v l p n e h d o e y t m a e n S H r m e r e e o i g m t o f W b s s e b s d o S f a wo k
W ANG i a g, M ENG n - h X w n - Xi g s u, W ANG — h n a Fu s u
目前 , 向对象 的体 系结 构 常 采 用 分 层 进 行 设 Mo e、 e C nr l r都 提 供 了 现 成 的 实 现 组 面 d lVi w、 o t l oe 计, 层次 系统结构 中 , 每一 层为上 层服务 , 并作 为下 层 件 L 。借 助 标 签 、 态 表 单 、 I E 、 1 ] 动 T L S VAL D I ATO R 客 户 。这 就将 一个 复 杂 问题 分解 成 一个 增 量 步骤 序 等 相关技 术 , 使 项 目实 现 的更 简 单 、 高效 、 方 将 更 更
第 1 卷 第 4期 2
21 0 0年 1 2月
河 北 农 业 大 学学 报 ( 农林 教育 版 )