Feasibility of using EMG driven neuromusculoskeletal model for prediction
《2024年基于多尺度和注意力机制融合的语义分割模型研究》范文
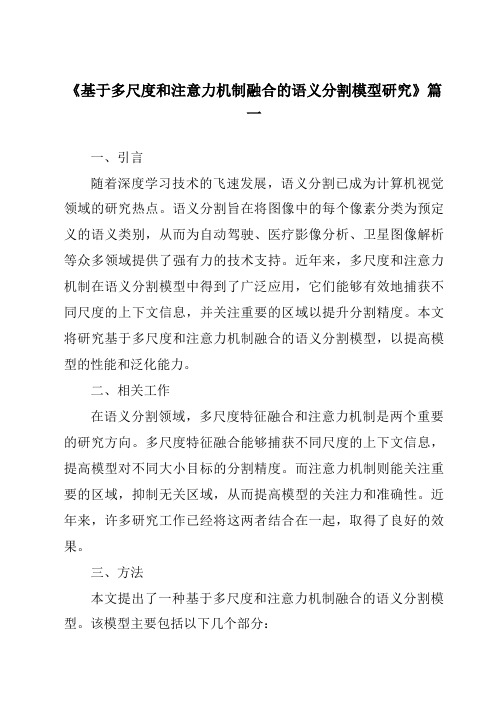
《基于多尺度和注意力机制融合的语义分割模型研究》篇一一、引言随着深度学习技术的飞速发展,语义分割已成为计算机视觉领域的研究热点。
语义分割旨在将图像中的每个像素分类为预定义的语义类别,从而为自动驾驶、医疗影像分析、卫星图像解析等众多领域提供了强有力的技术支持。
近年来,多尺度和注意力机制在语义分割模型中得到了广泛应用,它们能够有效地捕获不同尺度的上下文信息,并关注重要的区域以提升分割精度。
本文将研究基于多尺度和注意力机制融合的语义分割模型,以提高模型的性能和泛化能力。
二、相关工作在语义分割领域,多尺度特征融合和注意力机制是两个重要的研究方向。
多尺度特征融合能够捕获不同尺度的上下文信息,提高模型对不同大小目标的分割精度。
而注意力机制则能关注重要的区域,抑制无关区域,从而提高模型的关注力和准确性。
近年来,许多研究工作已经将这两者结合在一起,取得了良好的效果。
三、方法本文提出了一种基于多尺度和注意力机制融合的语义分割模型。
该模型主要包括以下几个部分:1. 多尺度特征提取:采用不同尺度的卷积核和池化操作,提取多尺度的上下文信息。
这些不同尺度的特征图将作为后续模块的输入。
2. 注意力机制模块:采用自注意力机制和交叉注意力机制,对每个尺度的特征图进行加权,以关注重要的区域并抑制无关区域。
3. 特征融合与上采样:将加权后的多尺度特征图进行融合,并采用上采样操作使特征图恢复到原始图像的大小。
4. 损失函数设计:采用交叉熵损失和Dice损失相结合的损失函数,以平衡正负样本的比例并提高模型的鲁棒性。
四、实验为了验证本文提出的模型的有效性,我们在多个公开数据集上进行了实验。
实验结果表明,本文提出的模型在语义分割任务中取得了良好的效果。
具体来说,我们在Cityscapes、ADE20K 等数据集上进行了实验,并与其他先进的语义分割模型进行了比较。
实验结果显示,本文提出的模型在分割精度、速度和泛化能力等方面均有所提升。
五、结果与分析1. 性能提升:通过多尺度和注意力机制的融合,本文提出的模型在语义分割任务中取得了较好的性能提升。
基于十字注意力机制改进U-Transformer_的新冠肺炎影像分割
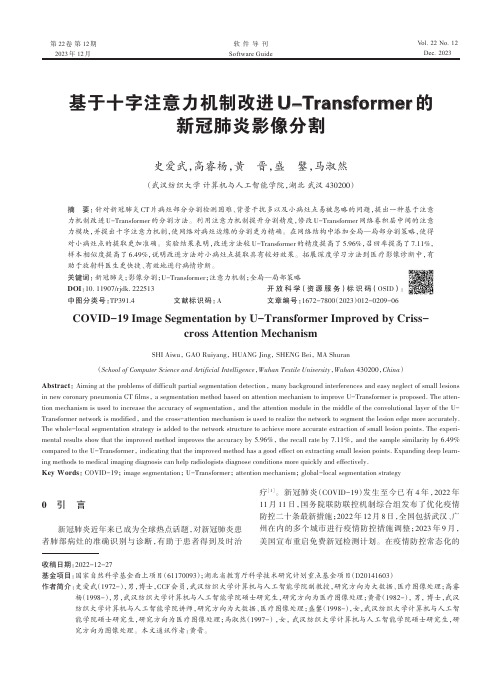
第 22卷第 12期2023年 12月Vol.22 No.12Dec.2023软件导刊Software Guide基于十字注意力机制改进U-Transformer的新冠肺炎影像分割史爱武,高睿杨,黄晋,盛鐾,马淑然(武汉纺织大学计算机与人工智能学院,湖北武汉 430200)摘要:针对新冠肺炎CT片病灶部分分割检测困难、背景干扰多以及小病灶点易被忽略的问题,提出一种基于注意力机制改进U-Transformer的分割方法。
利用注意力机制提升分割精度,修改U-Transformer网络卷积层中间的注意力模块,并提出十字注意力机制,使网络对病灶边缘的分割更为精确。
在网络结构中添加全局—局部分割策略,使得对小病灶点的提取更加准确。
实验结果表明,改进方法较U-Transformer的精度提高了5.96%,召回率提高了7.11%,样本相似度提高了6.49%,说明改进方法对小病灶点提取具有较好效果。
拓展深度学习方法到医疗影像诊断中,有助于放射科医生更快捷、有效地进行病情诊断。
关键词:新冠肺炎;影像分割;U-Transformer;注意力机制;全局—局部策略DOI:10.11907/rjdk.222513开放科学(资源服务)标识码(OSID):中图分类号:TP391.4 文献标识码:A文章编号:1672-7800(2023)012-0209-06COVID-19 Image Segmentation by U-Transformer Improved by Criss-cross Attention MechanismSHI Aiwu, GAO Ruiyang, HUANG Jing, SHENG Bei, MA Shuran(School of Computer Science and Artificial Intelligence,Wuhan Textile University,Wuhan 430200,China)Abstract:Aiming at the problems of difficult partial segmentation detection, many background interferences and easy neglect of small lesions in new coronary pneumonia CT films, a segmentation method based on attention mechanism to improve U-Transformer is proposed. The atten‐tion mechanism is used to increase the accuracy of segmentation, and the attention module in the middle of the convolutional layer of the U-Transformer network is modified, and the cross-attention mechanism is used to realize the network to segment the lesion edge more accurately. The whole-local segmentation strategy is added to the network structure to achieve more accurate extraction of small lesion points. The experi‐mental results show that the improved method improves the accuracy by 5.96%, the recall rate by 7.11%, and the sample similarity by 6.49% compared to the U-Transformer, indicating that the improved method has a good effect on extracting small lesion points. Expanding deep learn‐ing methods to medical imaging diagnosis can help radiologists diagnose conditions more quickly and effectively.Key Words:COVID-19; image segmentation; U-Transformer; attention mechanism; global-local segmentation strategy0 引 言新冠肺炎近年来已成为全球热点话题,对新冠肺炎患者肺部病灶的准确识别与诊断,有助于患者得到及时治疗[1]。
应用分层自适应匹配追踪重构MEMS陀螺信号

应 用 分 层 自适 应 匹 配 追 踪 重 构 M E M S陀 螺 信 号
杨金 显 , 杨 闯
( 河南理工大学 电气工程与 自动化学院导航制导实验室, 河南 焦作 4 5 4 0 0 0 )
摘要 : 对含噪微机械 系统( ME MS ) 陀螺信号进行小 波分解重构 时, 真实 信号对应 的小波 系数很 难选取 , 故 本 文 提 出 一 种 分 层 自适 应 匹 配 追 踪 算 法 ( L AMP ) 来 解 决 上 述 问 题 。建 立 了 含 噪 ME MS陀螺 信 号 中信 号 小 波 系 数 稀 疏 提 取 架 构 , 将 信 号 小 波 系 数 提 取 问题 转 化 为 含 噪 信 号 中 信 号 小 波 系 数 稀 疏 性 的 恢 复 问 题 。 比 较 已 有 稀 疏 重 构 算 法 , 采 用 一 种 新 的
第2 5卷
第1 2 期
工 程 光 学 精 密
Engi ne e r i ng 0 pt i c s a nd Pr e c i s i on
Vo 1 . 2 5 NO . 1 2
Dec .2 01 7
2 0 1 7 年 1 2月
文章编号
1 0 0 4 — 9 2 4 X( 2 0 1 7 ) 1 2 - 3 1 6 O — O 9
效果。
关 键 词 : ME Ms陀螺 ; 信号重构 ; 小 波 变换 ; 分 层 自适 应 匹 配 追踪 ( L AMP ) 算 法
中图分类号 : T N9 6 6 : T P 3 9 1 . 4 文献标识码 : A d o i : 1 0 . 3 7 8 8 / OP E . 2 O 1 7 2 5 1 2 . 3 1 6 O
L AMP算 法 , 在 各 种 可 能 的小 波 系数 组 合 中 挑 选 出分 解 系数 最 为 稀 疏 的 一 组 , 以此 消 除 信 号 中 的 噪 声 小 波 系 数 , 进 而 重
基于YOLOv5s的轻量化行人检测算法
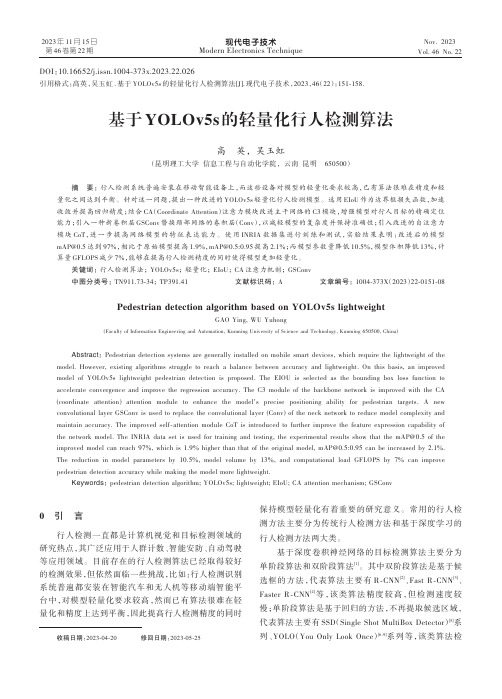
现代电子技术Modern Electronics TechniqueNov. 2023Vol. 46 No. 222023年11月15日第46卷第22期0 引 言行人检测一直都是计算机视觉和目标检测领域的研究热点,其广泛应用于人群计数、智能安防、自动驾驶等应用领域。
目前存在的行人检测算法已经取得较好的检测效果,但依然面临一些挑战,比如:行人检测识别系统普遍都安装在智能汽车和无人机等移动端智能平台中,对模型轻量化要求较高,然而已有算法很难在轻量化和精度上达到平衡,因此提高行人检测精度的同时保持模型轻量化有着重要的研究意义。
常用的行人检测方法主要分为传统行人检测方法和基于深度学习的行人检测方法两大类。
基于深度卷积神经网络的目标检测算法主要分为单阶段算法和双阶段算法[1]。
其中双阶段算法是基于候选框的方法,代表算法主要有R⁃CNN [2]、Fast R⁃CNN [3]、Faster R⁃CNN [4]等,该类算法精度较高,但检测速度较慢;单阶段算法是基于回归的方法,不再提取候选区域,代表算法主要有SSD (Single Shot MultiBox Detector )[5]系列、YOLO (You Only Look Once )[6⁃9]系列等,该类算法检DOI :10.16652/j.issn.1004⁃373x.2023.22.026引用格式:高英,吴玉虹.基于YOLOv5s 的轻量化行人检测算法[J].现代电子技术,2023,46(22):151⁃158.基于YOLOv5s 的轻量化行人检测算法高 英, 吴玉虹(昆明理工大学 信息工程与自动化学院, 云南 昆明 650500)摘 要: 行人检测系统普遍安装在移动智能设备上,而这些设备对模型的轻量化要求较高,已有算法很难在精度和轻量化之间达到平衡。
针对这一问题,提出一种改进的YOLOv5s 轻量化行人检测模型。
选用EIoU 作为边界框损失函数,加速收敛并提高回归精度;结合CA (Coordinate Attention )注意力模块改进主干网络的C3模块,增强模型对行人目标的精确定位能力;引入一种新卷积层GSConv 替换颈部网络的卷积层(Conv ),以减轻模型的复杂度并保持准确性;引入改进的自注意力模块CoT ,进一步提高网络模型的特征表达能力。
《2024年多模态深度学习综述》范文
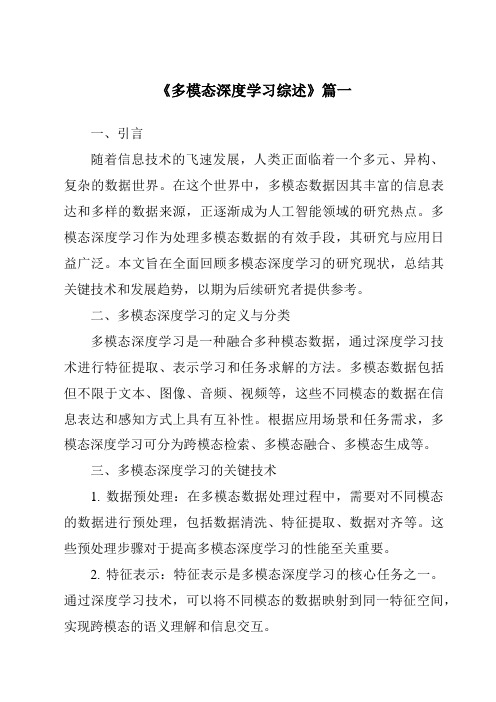
《多模态深度学习综述》篇一一、引言随着信息技术的飞速发展,人类正面临着一个多元、异构、复杂的数据世界。
在这个世界中,多模态数据因其丰富的信息表达和多样的数据来源,正逐渐成为人工智能领域的研究热点。
多模态深度学习作为处理多模态数据的有效手段,其研究与应用日益广泛。
本文旨在全面回顾多模态深度学习的研究现状,总结其关键技术和发展趋势,以期为后续研究者提供参考。
二、多模态深度学习的定义与分类多模态深度学习是一种融合多种模态数据,通过深度学习技术进行特征提取、表示学习和任务求解的方法。
多模态数据包括但不限于文本、图像、音频、视频等,这些不同模态的数据在信息表达和感知方式上具有互补性。
根据应用场景和任务需求,多模态深度学习可分为跨模态检索、多模态融合、多模态生成等。
三、多模态深度学习的关键技术1. 数据预处理:在多模态数据处理过程中,需要对不同模态的数据进行预处理,包括数据清洗、特征提取、数据对齐等。
这些预处理步骤对于提高多模态深度学习的性能至关重要。
2. 特征表示:特征表示是多模态深度学习的核心任务之一。
通过深度学习技术,可以将不同模态的数据映射到同一特征空间,实现跨模态的语义理解和信息交互。
3. 融合策略:多模态融合策略包括早期融合、晚期融合和混合融合等。
早期融合主要在数据预处理阶段进行融合,晚期融合则是在特征或决策层面进行融合。
混合融合则结合了早期和晚期融合的优点,根据任务需求灵活调整融合策略。
4. 模型训练:多模态深度学习需要设计合适的模型结构和训练方法。
常用的模型包括循环神经网络、卷积神经网络、生成对抗网络等。
针对多模态数据的特性,需要设计具有跨模态交互能力的模型结构,并采用合适的优化算法进行训练。
四、多模态深度学习的应用领域多模态深度学习在各个领域都有广泛的应用,包括但不限于以下方面:1. 图像与文本的跨模态检索:通过多模态深度学习技术,实现图像与文本之间的跨模态检索,提高检索的准确性和效率。
2. 人机交互:多模态深度学习可以实现在自然语言处理、语音识别、手势识别等多模态信息的融合和处理,提高人机交互的智能性和便捷性。
自然科学基金 多模态 工况辨识建模
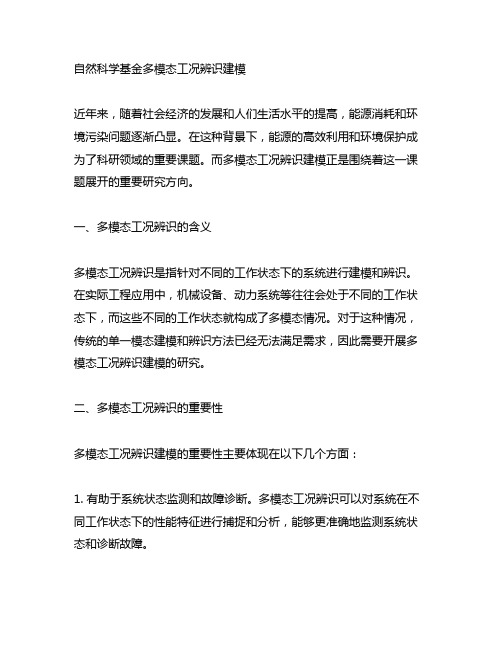
自然科学基金多模态工况辨识建模近年来,随着社会经济的发展和人们生活水平的提高,能源消耗和环境污染问题逐渐凸显。
在这种背景下,能源的高效利用和环境保护成为了科研领域的重要课题。
而多模态工况辨识建模正是围绕着这一课题展开的重要研究方向。
一、多模态工况辨识的含义多模态工况辨识是指针对不同的工作状态下的系统进行建模和辨识。
在实际工程应用中,机械设备、动力系统等往往会处于不同的工作状态下,而这些不同的工作状态就构成了多模态情况。
对于这种情况,传统的单一模态建模和辨识方法已经无法满足需求,因此需要开展多模态工况辨识建模的研究。
二、多模态工况辨识的重要性多模态工况辨识建模的重要性主要体现在以下几个方面:1. 有助于系统状态监测和故障诊断。
多模态工况辨识可以对系统在不同工作状态下的性能特征进行捕捉和分析,能够更准确地监测系统状态和诊断故障。
2. 有助于系统控制和优化。
针对不同的工作状态,多模态工况辨识可以建立相应的控制模型,从而实现对系统的精确控制和优化调节。
3. 有助于能源利用和环境保护。
多模态工况辨识建模可以有效提高能源利用效率,降低污染物排放,从而实现对环境的更好保护。
三、多模态工况辨识建模的研究内容多模态工况辨识建模的研究内容主要包括以下几个方面:1. 多模态特征提取与分析。
针对不同工作状态下的系统,需要提取和分析其在不同工况下的特征,包括振动、声音、温度等多种信号特征。
2. 多模态数据处理与融合。
针对不同工况下获得的数据,需要进行有效的数据处理和融合,以实现对系统多模态特征的综合分析。
3. 多模态辨识建模方法研究。
需要开展多模态辨识建模的方法研究,包括基于统计学习的方法、基于深度学习的方法、基于物理模型的方法等。
4. 多模态辨识建模算法设计与优化。
需要设计并优化适用于多模态辨识建模的算法,以实现对系统多模态特征的准确辨识和建模。
四、多模态工况辨识建模的研究现状目前,多模态工况辨识建模的研究已取得了一些进展,涉及到了多领域的应用。
JWatcher软件用户手册说明书
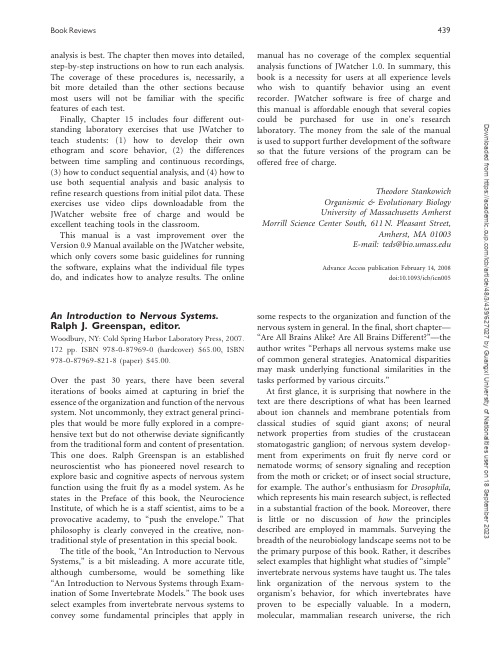
analysis is best.The chapter then moves into detailed, step-by-step instructions on how to run each analysis. The coverage of these procedures is,necessarily,a bit more detailed than the other sections because most users will not be familiar with the specific features of each test.Finally,Chapter15includes four different out-standing laboratory exercises that use JWatcher to teach students:(1)how to develop their own ethogram and score behavior,(2)the differences between time sampling and continuous recordings, (3)how to conduct sequential analysis,and(4)how to use both sequential analysis and basic analysis to refine research questions from initial pilot data.These exercises use video clips downloadable from the JWatcher website free of charge and would be excellent teaching tools in the classroom.This manual is a vast improvement over the Version0.9Manual available on the JWatcher website, which only covers some basic guidelines for running the software,explains what the individual file types do,and indicates how to analyze results.The online manual has no coverage of the complex sequential analysis functions of JWatcher1.0.In summary,this book is a necessity for users at all experience levels who wish to quantify behavior using an event recorder.JWatcher software is free of charge and this manual is affordable enough that several copies could be purchased for use in one’s research laboratory.The money from the sale of the manual is used to support further development of the software so that the future versions of the program can be offered free of charge.Theodore StankowichOrganismic&Evolutionary BiologyUniversity of Massachusetts Amherst Morrill Science Center South,611N.Pleasant Street,Amherst,MA01003E-mail:Advance Access publication February14,2008doi:10.1093/icb/icn005An Introduction to Nervous Systems. Ralph J.Greenspan,editor.Woodbury,NY:Cold Spring Harbor Laboratory Press,2007. 172pp.ISBN978-0-87969-0(hardcover)$65.00,ISBN 978-0-87969-821-8(paper)$45.00.Over the past30years,there have been several iterations of books aimed at capturing in brief the essence of the organization and function of the nervous system.Not uncommonly,they extract general princi-ples that would be more fully explored in a compre-hensive text but do not otherwise deviate significantly from the traditional form and content of presentation. This one does.Ralph Greenspan is an established neuroscientist who has pioneered novel research to explore basic and cognitive aspects of nervous system function using the fruit fly as a model system.As he states in the Preface of this book,the Neurocience Institute,of which he is a staff scientist,aims to be a provocative academy,to“push the envelope.”That philosophy is clearly conveyed in the creative,non-traditional style of presentation in this special book. The title of the book,“An Introduction to Nervous Systems,”is a bit misleading.A more accurate title, although cumbersome,would be something like “An Introduction to Nervous Systems through Exam-ination of Some Invertebrate Models.”The book uses select examples from invertebrate nervous systems to convey some fundamental principles that apply in some respects to the organization and function of thenervous system in general.In the final,short chapter—“Are All Brains Alike?Are All Brains Different?”—theauthor writes“Perhaps all nervous systems make useof common general strategies.Anatomical disparitiesmay mask underlying functional similarities in thetasks performed by various circuits.”At first glance,it is surprising that nowhere in thetext are there descriptions of what has been learnedabout ion channels and membrane potentials from classical studies of squid giant axons;of neuralnetwork properties from studies of the crustacean stomatogastric ganglion;of nervous system develop-ment from experiments on fruit fly nerve cord or nematode worms;of sensory signaling and receptionfrom the moth or cricket;or of insect social structure,for example.The author’s enthusiasm for Drosophila,which represents his main research subject,is reflectedin a substantial fraction of the book.Moreover,thereis little or no discussion of how the principles described are employed in mammals.Surveying thebreadth of the neurobiology landscape seems not to bethe primary purpose of this book.Rather,it describesselect examples that highlight what studies of“simple”invertebrate nervous systems have taught us.The taleslink organization of the nervous system to the organism’s behavior,for which invertebrates haveproven to be especially valuable.In a modern, molecular,mammalian research universe,the rich439 Downloaded from https:///icb/article/48/3/439/627027 by Guangxi University of Nationalities user on 18 September 2023history of fundamental contributions of invertebrates to neuroscience may too often be overlooked.It is especially in this respect that the book is a welcome contribution to the neurobiological literature.In the introduction to Chapter4—“Modulation,The Spice of Neural Life”—the author writes:“The capabilities of invertebrates have traditionally been underesti-mated.Perhaps this is because they are not warm and fuzzy...For whatever reason,it has taken us an inordinately long time to realize that even the simplest animals have the capacity for modifying their behavior by adjusting the activities of their nervous systems. Perhaps this is a fundamental,inseparable property of nervous systems.”Despite the fact that the book deviates from a traditional style,in its own way it follows a rather traditional sequence,e.g.,membrane potentials,then chemical signaling and sensing,then neural circuits,then neuromodulation,then biological clocks,then higher,or cognitive,function.There is a lot to like in this book,not only in its fascinating content but in the style of presentation. Ralph Greenspan weaves a tapestry about the molecular,cellular and network origins of function and behavior,and the implications for speciation, using a variety of invertebrate models.The images he creates are expressed as interesting,often humorous, readable stories about what some nervous systems do, how they do it,and how that has evolved using some basic principles in novel ways.Each chapter begins with a relevant quote or poem from a literary or scientific giant that sets the stage and tone for the often poetic introduction and description that follows. The stories themselves—about swimming in Paramecium and jellyfish,light detection by barnacles, decision making by marine snails,circadian rhythms, flying,and mating—are fascinating because they are set in a context of understanding the generation and modulation of behavior and,in some cases,the impact on ecology and evolution.Although the author states in the Preface that the book is intended for the neurobiology novice posses-sing a basic introductory knowledge of biology,this reviewer believes that it would be more appropriate for an individual with an introductory neurobiological background.For example,in the very first chapter, one quickly discovers that understanding“simple”systems can be quite complex.In particular,students new to neurobiology often struggle with concepts underlying the generation of membrane potentials and the relationship of voltage and current,yet the text and figures require some understanding of these topics.In this respect,the Glossary at the end of the book seems uneven,defining some very basic biological terms yet not defining“receptor potential,”for example,which is named but not explained in the caption of Figure3.10.Not to quibble,but this reviewer and two other neuroscientists who scanned the book question some statements or generalizations proposed,particularly in the Introduction(“What are Brains For”?).For example,on page1it states“When it comes to brains,size unquestionably matters.”While that is no doubt true,it may be the organization of cells,i.e.the way they interact,that is more relevant.If it is size that is so important,then one should note that about three quarters of cells in mammalian brain are glial cells, not neurons,some potentially capable of modulating chemical signaling at up to100,000synapses,yet their contributions are not mentioned(see below). Furthermore,spinal cords also possess much of the organization and cellular interactions,e.g.,integrating sensory input and generating motor output,yet we view their capabilities as somewhat lacking in comparison with brain.What might be the funda-mental differences between invertebrate and verte-brate nervous systems and between brain and spinal cord that yield unique aspects of functional compe-tence?Or,are they as different as we imagine them to be,particularly in comparing function in invertebrates versus vertebrates?These are some interesting questions—not found in a typical comprehensive text—that might be explored a bit further in the Introduction and perhaps elsewhere in the book.In addition,on page2,the author writes“Chemical sensing is almost certainly the original sense...,”yet mechanically gated ion channels that could sense changes in flow or pressure in the ambient environ-ment are universal and also have been identified in prokaryotic organisms.Also,on page4,the author writes“And because none of us wants to submit to being experimented upon...we study animals.”Yet, there is a substantial and rapidly growing literature that provides insights on the organization and function of human brain from studies of living persons—for example from functional MRI or stimulation/recording of brain of awake epileptic patients—or of postmortem tissue samples.There are several other aspects of the book in its current form that would benefit from revision in a second edition.First,the emphasis is on how invertebrate nervous systems inform on nervous systems in general,but it is not clear in many cases to what extent the general organization of the behaviors is similar in invertebrates and vertebrates or whether similar molecules or mechanisms are used for different purposes.Does evolution mix and match bits and pieces of behavioral components that moves behavior in new directions?One also wonders whether440Book ReviewsDownloaded from https:///icb/article/48/3/439/627027 by Guangxi University of Nationalities user on 18 September 2023there are good examples of invertebrate nervous systems and behaviors that do not translate well to a mammalian equivalent.Second,the book has a traditional neurocentric focus—and some inverte-brates indeed have few glial cells—yet in the past couple of decades it has become abundantly clear from studies of mammalian systems that interactions of neurons with glia play vital roles in regulation of neural function,development and blood flow.Third, some of the figures could benefit from greater clarity or correction of the illustration or of the explanation in the caption,including citing the source link that is listed in the Bibliography at the end of the book.In addition,the Preface could note the location of the relevant Bibliography,currently organized by chapters but separate from them.It should be noted that the author also recently co-edited a much more compre-hensive(800pages),related book(“Invertebrate Neurobiology”)with Geoffrey North.In summary,this is an excellent book for gaining an appreciation for the links between form-function and behavior in the nervous system from invertebrate model systems and one that is interesting and enjoyable to read.It should be particularly valuable in inspiring budding or established life scientists to read more on the subject or even to become engaged in the pursuit of elucidating fundamental principles of neurobiology and behavior.It should stimulate broad questions about nervous systems and behavior. From a pedagogical perspective,I could imagine it being assigned as a short text in a general course on neurobiology and behavior or in a specialized neurobiology course that focuses on invertebrates or as a supplement to a more comprehensive text.Robert M.GrossfeldDepartment of Zoology NC State University,Raleigh,NC27695E-mail:*************************Advance Access publication February15,2008doi:10.1093/icb/icn004Rodent Societies–An Ecological and Evolutionary Perspective.Jerry O.Wolff and Paul W.Sherman,editors. Chicago,IL:University of Chicago Press,2007.610pp. ISBN0-226-90536-5(cloth),$125.00and ISBN0-226-90537-3(paper),$49.00.As the editors point out in the first sentence of the first chapter,“The Rodentia is the largest order of mammals consisting of more than2000species and comprising44%of all mammals.”This breadth makes the task of compiling a definitive and comprehensive anthology on rodent societies a nearly impossible task,but the result is undoubtedly the most exhaustive and progressive analysis of rodent social behavior to date.Deftly edited by Jerry Wolff and Paul Sherman,this well-organized book,consisting of41chapters from61contributors is,without doubt,a significant compendium of more than50years of research.That being said,only a true rodent lover is likely to love this book.Its creation was prompted by the success of the two volumes within this series that preceded it:Primate Societies and Cetacean Societies(published by University of Chicago Press).Thus,the scope and format of Rodent Societies is in many ways similar to that of the previous two volumes.The text is organized into nine sections,beginning with a succinct,but satisfying,overview of rodent evolutionary history and proceeding through sexual behavior,life histories and behavior,behavioral development,social behavior, antipredator behavior,comparative socioecology,con-servation and disease,and a final concluding sectionwritten by the editors on potential directions for future research.Each chapter concludes with a summary thatbriefly reviews the material,identifies caveats,and frequently suggests strategies for future research.The chapters are written by some of the most productiveand well-known scholars in the field but,as expected ina multi-authored work,the quality is uneven.Some chapters do a better job than others of achieving thestated goal to“synthesize and integrate the currentstate of knowledge about the social behavior of rodents”and to“provide ecological and evolutionary contexts for understanding rodent societies.”However,it generally succeeds in combining ideasand strategies from a wide range of disciplines to generate new theoretical and experimental paradigmsfor exploring rodent social behavior.Despite this,itfeels outdated in many places.Much of the work citedin the text is not new,with the majority of citationsdating before2000and a substantial number datingbefore1985.Even the photographs,all in black andwhite,are fairly old and some date back to the1950s.Some of the illustrations are even hand-drawn.Thismakes the book feel like historical retrospective rathera breakthrough collaborative of evolutionary and behavioral biology.441 Downloaded from https:///icb/article/48/3/439/627027 by Guangxi University of Nationalities user on 18 September 2023。
基于自适应遗传算法的多无人机协同任务分配
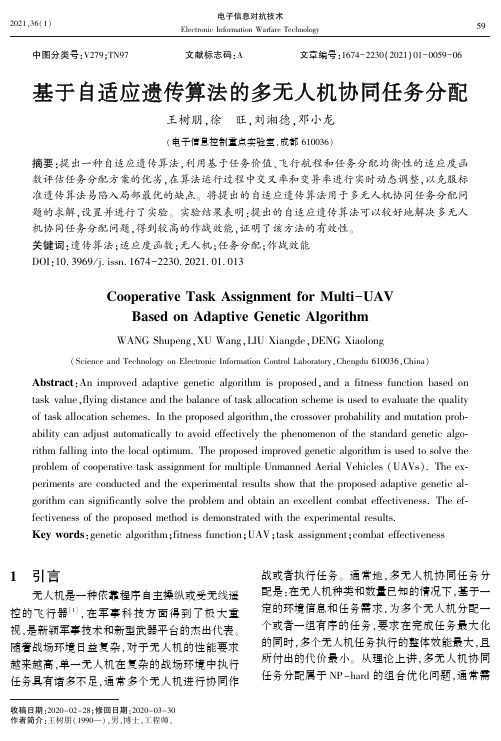
2021,36(1)电子信息对抗技术Electronic Information Warfare Technology㊀㊀中图分类号:V279;TN97㊀㊀㊀㊀㊀㊀文献标志码:A㊀㊀㊀㊀㊀㊀文章编号:1674-2230(2021)01-0059-06收稿日期:2020-02-28;修回日期:2020-03-30作者简介:王树朋(1990 ),男,博士,工程师㊂基于自适应遗传算法的多无人机协同任务分配王树朋,徐㊀旺,刘湘德,邓小龙(电子信息控制重点实验室,成都610036)摘要:提出一种自适应遗传算法,利用基于任务价值㊁飞行航程和任务分配均衡性的适应度函数评估任务分配方案的优劣,在算法运行过程中交叉率和变异率进行实时动态调整,以克服标准遗传算法易陷入局部最优的缺点㊂将提出的自适应遗传算法用于多无人机协同任务分配问题的求解,设置并进行了实验㊂实验结果表明:提出的自适应遗传算法可以较好地解决多无人机协同任务分配问题,得到较高的作战效能,证明了该方法的有效性㊂关键词:遗传算法;适应度函数;无人机;任务分配;作战效能DOI :10.3969/j.issn.1674-2230.2021.01.013Cooperative Task Assignment for Multi -UAVBased on Adaptive Genetic AlgorithmWANG Shupeng,XU Wang,LIU Xiangde,DENG Xiaolong(Science and Technology on Electronic Information Control Laboratory,Chengdu 610036,China)Abstract :An improved adaptive genetic algorithm is proposed,and a fitness function based ontask value,flying distance and the balance of task allocation scheme is used to evaluate the qualityof task allocation schemes.In the proposed algorithm,the crossover probability and mutation prob-ability can adjust automatically to avoid effectively the phenomenon of the standard genetic algo-rithm falling into the local optimum.The proposed improved genetic algorithm is used to solve the problem of cooperative task assignment for multiple Unmanned Aerial Vehicles (UAVs).The ex-periments are conducted and the experimental results show that the proposed adaptive genetic al-gorithm can significantly solve the problem and obtain an excellent combat effectiveness.The ef-fectiveness of the proposed method is demonstrated with the experimental results.Key words :genetic algorithm;fitness function;UAV;task assignment;combat effectiveness1㊀引言无人机是一种依靠程序自主操纵或受无线遥控的飞行器[1],在军事科技方面得到了极大重视,是新颖军事技术和新型武器平台的杰出代表㊂随着战场环境日益复杂,对于无人机的性能要求越来越高,单一无人机在复杂的战场环境中执行任务具有诸多不足,通常多个无人机进行协同作战或者执行任务㊂通常地,多无人机协同任务分配是:在无人机种类和数量已知的情况下,基于一定的环境信息和任务需求,为多个无人机分配一个或者一组有序的任务,要求在完成任务最大化的同时,多个无人机任务执行的整体效能最大,且所付出的代价最小㊂从理论上讲,多无人机协同任务分配属于NP -hard 的组合优化问题,通常需95王树朋,徐㊀旺,刘湘德,邓小龙基于自适应遗传算法的多无人机协同任务分配投稿邮箱:dzxxdkjs@要借助于算法进行求解㊂目前,国内外研究人员已经对于多无人机协同任务分配问题进行了大量的研究,并提出很多用于解决该问题的算法,主要有:群算法㊁自由市场机制算法和进化算法等㊂群算法是模拟自然界生物群体的行为的算法,其中蚁群算法[2-3]㊁粒子群算法[4]以及鱼群算法[5]是最为典型的群算法㊂研究人员发现群算法可以用于求解多无人机协同任务分配问题,但是该算法极易得到局部最优而非全局最优㊂自由市场机制算法[6]是利用明确的规则引导买卖双方进行公开竞价,在短时间内将资源合理化,得到问题的最优解和较优解㊂进化算法适合求解大规模问题,其中遗传算法[7-8]是最著名的进化算法㊂遗传算法在运行过程中会出现不容易收敛或陷入局部最优的问题,许多研究人员针对该问题对遗传算法进行了改进㊂本文提出一种改进的自适应遗传算法,在算法运行过程中适应度值㊁交叉率和变异率可以进行实时动态调整,以克服遗传算法易陷入局部最优的缺点,并利用该算法解决多无人机协同任务分配问题,以求在满足一定的约束条件下,无人机执行任务的整体收益最大,同时付出的代价最小,得到较大的效费比㊂2㊀问题描述㊀㊀多无人机协同任务分配模型是通过设置并满足一定约束条件的情况下,包括无人机的自身能力限制和环境以及任务的要求等,估算各个无人机执行任务获得的收益以及付出的代价,并利用评价指标进行评价,以求得到最大的收益损耗比和最优作战效能㊂通常情况下,多无人机协同任务分配需满足以下约束:1)每个任务只能被分配一次;2)无人机可以携带燃料限制造成的最大航程约束;3)无人机载荷限制,无人机要执行某项任务必须装载相应的载荷㊂另外,多无人机协同任务分配需要遵循以下原则:1)收益最高:每项任务都拥有它的价值,任务分配方案应该得到最大整体收益;2)航程最小:应该尽快完成任务,尽可能减小飞行航程,这样易满足无人机的航程限制,同时降低无人机面临的威胁;3)各个无人机的任务负载尽可能均衡,通常以任务个数或者飞行航程作为标准判定; 4)优先执行价值高的任务㊂根据以上原则,提出多无人机协同任务分配的评价指标,包括:1)任务价值指标:用于评估任务分配方案可以得到的整体收益;2)任务分配均衡性指标:用于评估无人机的任务负载是否均衡;3)飞行航程指标:用于评估无人机的飞行航程㊂3㊀遗传算法㊀㊀要将遗传算法用于多无人机协同任务分配问题的求解,可以将任务分配方案当作种群中的个体,确定合适的染色体编码方法,利用按照一定结构组成的染色体表示任务分配方案㊂然后,通过选择㊁交叉和变异等遗传操作进行不断进化,直到满足约束条件㊂通常来说,遗传算法可以表示为GA=(C,E, P0,M,F,G,Y,T),其中C㊁E㊁P0和M分别表示染色体编码方法㊁适应度函数㊁初始种群和种群大小,在本文的应用中,P0和M分别表示初始的任务分配方案集合以及任务分配方案的个数;F㊁G 和Y分别表示选择算子㊁交叉算子和变异算子;T 表示终止的条件和规则㊂因此,利用遗传算法解决多无人机协同任务分配问题的主要工作是确定以上8个参数㊂3.1㊀编码方法利用由一定结构组成的染色体表示任务分配方案,将一个任务分配方案转换为一条染色体的过程可以分为2个步骤:第一步是根据各个无人机需执行的任务确定各个无人机对应的染色体;第二步是将这些小的染色体结合,形成整个任务分配方案对应的完整染色体㊂假设无人机和任务的个数分别为N u和N t,其中第i个无人机U i的06电子信息对抗技术㊃第36卷2021年1月第1期王树朋,徐㊀旺,刘湘德,邓小龙基于自适应遗传算法的多无人机协同任务分配任务共有k个,分别是T i1㊁T i2㊁ ㊁T ik,则该无人机对应的任务染色体为[T i1T i2 T ik]㊂在任务分配时,可能出现N t个任务全部分配给一个无人机的情况,另外为增加随机性和扩展性,提高遗传算法的全局搜索能力,随机将N t-k个0插入到以上的任务染色体中,产生一条全新的长度为N t的染色体㊂最终,一个任务分配方案可以转换为一条长度为N u∗N t的染色体㊂3.2㊀适应度函数在本文的应用中,适应度函数E是用于判断任务分配方案的质量,根据上文提出的多无人机协同任务分配问题的原则和评价指标可知,主要利用任务价值指标㊁任务分配均衡性指标以及飞行航程指标等三个指标判定任务分配方案的质量㊂假设有N u个无人机,F i表示第i个无人机U i的飞行航程,整个任务的总飞行航程F t可以表示为:F t=ðN u i=1F i(1)无人机的平均航程为:F=F t Nu(2)无人机飞行航程的方差D可以表示为:D=ðN u i=1F i-F-()2N u(3)为充分考虑任务价值㊁飞行航程以及各个无人机任务的均衡性,将任务分配方案的适应度函数定义为:E=V ta∗F t+b∗D(4)其中:V t为任务的总价值,F t为总飞行航程,D为各个无人机飞行航程的方差,a和b分别表示飞行航程以及飞行航程均衡性的权重㊂另外,任务分配方案的收益损耗比GL可以表示为:GL=V tF t(5)另外,在遗传算法运行的不同阶段,需要对任务分配方案的适应度进行适当地扩大或者缩小,新的适应度函数E可以表示为:Eᶄ=1-e-αEα=m tE max-E avg+1,m=1+lg T()ìîíïïïï(6)其中:E为利用公式(4)计算得到的原适应度值, E avg为适应度值的平均值,E max为适应度最大值,t 为算法的运行次数,T为遗传算法的终止条件㊂在遗传算法运行初期,E max-E avg较大,而t较小,因此α较小,可以提高低质量任务分配方案的选择概率,同时降低高质量任务分配方案的选择概率;随着算法的运行,E max-E avg将逐渐减小,t 将逐渐增大,因此α会逐渐增大,可以避免算法陷入随机选择和局部最优㊂3.3㊀种群大小㊁初始种群和终止条件按照通常做法,将种群大小M的取值范围设定为20~100㊂首先,随机产生2∗M个符合要求的任务分配方案,利用公式(4)计算各个任务分配方案的适应度值㊂然后,从中选取出适应度值较高的M 个任务分配方案组成初始种群P0,即初始任务分配方案集合㊂终止条件T设定为:在规定的迭代次数内有一个任务分配方案的适应度值满足条件,则停止进化;否则,一直运行到规定的迭代次数㊂3.4㊀选择算子首先,采用精英保留策略将当前适应度值最大的一个任务分配方案直接保留到下一代,提高遗传算法的全局收敛能力㊂随后,利用最知名的轮盘赌选择法选择出剩余的任务分配方案㊂3.5㊀交叉算子和变异算子在算法运行过程中需随时动态调整p c和p m,动态调整的原则如下:1)适当降低适应度值比平均适应度值高的任务分配方案的p c和p m,以保护优秀的高质量任务分配方案,加快算法的收敛速度;2)适当增大适应度值比平均适应度值低的任务分配方案的p c和p m,以免算法陷入局部最优㊂另外,任务分配方案的集中度β也是决定p c 和p m的重要因素,β可以表示为:16王树朋,徐㊀旺,刘湘德,邓小龙基于自适应遗传算法的多无人机协同任务分配投稿邮箱:dzxxdkjs@β=E avgE max(7)其中:E avg 表示平均适应度值;E max 表示最大适应度值㊂显然,β越大,任务分配方案越集中,遗传算法越容易陷入局部最优㊂因此,随着β增大,p c 和p m 应该随之增大㊂基于以上原则,定义p c 和p m 如下:p c =0.8E avg -Eᵡ()+0.6Eᵡ-E min ()E avg -E min +0.2㊃βEᵡ<E avg 0.6E max -Eᵡ()+0.4Eᵡ-E avg ()E max -E avg +0.2㊃βEᵡȡE avgìîíïïïïïp m =0.08E avg -E‴()+0.05E‴-E min ()E avg -E min +0.02㊃βE‴<E avg and β<0.80.05E max -E‴()+0.0001E‴-E avg ()E max -E avg+0.02㊃βE‴ȡE avg and β<0.80.5βȡ0.8ìîíïïïïïï(8)其中:E max 为最大适应度值,E min 为最小适应度值,E avg 为平均适应度值,Eᵡ为进行交叉操作的两个任务分配方案中的较大适应度值,E‴为进行变异操作的任务分配方案的适应度值,β为任务分配方案的集中度,可利用公式(7)计算得到㊂4㊀实验结果4.1㊀实验设置4架无人机从指定的起飞机场起飞,飞至5个任务目标点执行10项任务,最终降落到指定的降落机场㊂其中,如表1所示,无人机的编号分别为UAV 1㊁UAV 2㊁UAV 3和UAV 4㊂另外,起飞机场㊁降落机场㊁目标如图6所示㊂任务的编号分别为任务1至任务10(简称为T 1㊁T 2㊁ ㊁T 10),每项任务均为到某一个目标点执行侦察㊁攻击㊁事后评估中的某一项,任务设置如表2所示㊂表1㊀无人机信息编号最大航程装载载荷UAV 120侦察㊁攻击UAV 120侦察UAV 125攻击㊁评估UAV 130侦察㊁评估图1㊀任务目标位置示意图表2㊀任务设置任务编号目标编号任务类型任务价值T 11侦察1T 21攻击2T 32攻击3T 42评估3T 53侦察4T 63评估6T 74侦察2T 84攻击3T 94评估5T 105评估14.2㊀第一组实验首先,随机地进行任务分配,得到一个满足多无人机协同任务分配的约束条件的任务分配方案如下:㊃UAV 1:T 2ңT 5㊃UAV 2:T 1ңT 7㊃UAV 3:T 3ңT 6ңT 8㊃UAV 4:T 4ңT 9ңT 10计算可知,4个无人机的飞行航程分别是14.0674㊁12.6023㊁20.1854和22.1873,飞行总航程为69.0423,执行任务的总价值为30,最终的收益损耗比约为0.43㊂另外,各个飞行器飞行航程的方差约为16.18,UAV 1和UAV 2的飞行航程相对较短,而UAV 3和UAV 4的飞行航程相对较长,各个无人机之间的均衡性存在明显不足㊂为提高收益损耗比,分别利用标准遗传算法和本文提出的自适应遗传算法进行优化,两个算法的参数设置如表3所示㊂26电子信息对抗技术·第36卷2021年1月第1期王树朋,徐㊀旺,刘湘德,邓小龙基于自适应遗传算法的多无人机协同任务分配表3㊀遗传算法参数设置参数名称标准遗传算法自适应遗传算法E 公式(4)公式(6)M 2020选择方法精英策略轮盘赌选择法精英策略轮盘赌选择法P c 0.8公式(8)交叉方法单点交叉单点交叉P m 0.2公式(8)T500500最终,利用标准遗传算法得到任务分配方案如下:㊃UAV 1:T 3ңT 8㊃UAV 2:T 1ңT 7㊃UAV 3:T 9㊃UAV 4:T 6ңT 5计算可得,4个无人机的飞行航程分别为12.78㊁12.6023㊁12.434和12.9443,总飞行航程为50.7605,总任务价值为24,计算可知收益损耗比约为0.47,相对于随机任务分配提高约9.3%㊂另外,各个飞行器飞行航程的方差约为0.04,无人机飞行航程比较均衡,未出现飞行航程过长或过短的情况㊂在算法运行过程中,最佳适应度曲线如图2所示,在遗传算法约迭代到第160次时陷入局部最优,全局搜索能力不足㊂图2㊀标准遗传算法的最佳适应度曲线图1为进一步提高算法的效率,利用本文提出的改进自适应遗传算法解决多无人机协同任务分配问题㊂最终,利用自适应遗传算法得到的任务分配方案如下:㊃UAV 1:T 2ңT 3ңT 8ңT 7㊃UAV 2:T 5㊃UAV 3:T 6㊃UAV 4:T 1ңT 4ңT 9计算可知,4个无人机的飞行航程分别为12.8191㊁12.9443㊁12.9443和12.8191,总飞行航程为51.5268,总任务价值为29,收益耗比约为0.56,相对于随机任务分配提高约30.2%,相对于基于标准遗传算法的任务分配方案提高约19.1%㊂另外,各个飞行器飞行航程的方差约为0.004,无人机飞行航程的均衡性相对于基于标准遗传算法的任务分配方案有了进一步的提高㊂在算法运行过程中,最佳适应度值曲线如图3所示,可以有效避免遗传算法陷入局部最优或者随机选择㊂图3㊀自适应遗传算法的最佳适应度曲线图14.3㊀第二组实验在第一组实验中,因任务10(简称为T 10)的价值较低,在最终的任务分配方案中极少被分配㊂在第二组实验中,将T 10的价值由1调整为6,其他设置项不变㊂首先,随机进行任务分配,最终的任务分配方案和第一组实验相同㊂随后,利用标准遗传算法进行多无人机协同任务分配,最终的任务分配方案如下:㊃UAV 1:T 2ңT 3ңT 7ңT 8㊃UAV 2:T 5㊃UAV 3:T 6ңT 10㊃UAV 4:T 9基于此任务分配方案,4个无人机的飞行航程分别为12.8191㊁12.9443㊁13.6883和12.434,总飞行航程为51.8857,总任务价值为31,因此计36王树朋,徐㊀旺,刘湘德,邓小龙基于自适应遗传算法的多无人机协同任务分配投稿邮箱:dzxxdkjs@算可得收益损耗比约为0.6,相对于随机任务分配提高约17.6%㊂另外,各个飞行器飞行航程的方差约为0.21,各个无人机的飞行航程的均衡性一般,相对于随机任务分配有一定的提高㊂在算法运行过程中,最佳适应度值曲线如图4所示,在算法迭代运行约90次时陷入较长时间的局部最优,直到迭代次数为340次时,然后再次陷入局部最优㊂图4㊀标准遗传算法的最佳适应度曲线图2最后,将本文提出的自适应遗传算法用于多无人机协同任务分配问题的求解,得到最终的任务分配方案如下:㊃UAV 1:T 2ңT 3ңT 8ңT 7㊃UAV 2:T 5㊃UAV 3:T 6ңT 10㊃UAV 4:T 1ңT 4ңT 9基于此任务分配方案可得,4个无人机的航程分别是12.8191㊁12.9443㊁13.6883以及12.8191,总飞行航程为52.2708,总任务价值为35,计算可得效益损耗比约为0.67,相对于利用标准遗传算法得到的任务分配方案有了进一步提高㊂另外,各个无人机飞行航程的方差约为0.13,飞行航程的均衡性较好㊂在算法运行过程中,最佳适应度值曲线如图5所示,适应度值一直在实时动态变化,可以有效避免遗传算法陷入局部最优或者随机选择㊂由实验结果可得,当任务10的任务价值从1调整为6以后,不再出现该任务没有无人机执行的情况,这说明利用遗传算法进行多无人机协同任务分配可以根据任务的价值以及代价进行实时动态调整,符合 优先执行价值高的任务 的原则㊂图5㊀自适应遗传算法的最佳适应度曲线图25 结束语㊀㊀本文提出了一种基于自适应遗传算法的多无人机协同任务分配方法,整个遗传过程利用自适应的适应度函数评估任务分配结果的优劣,交叉率和变异率在算法运行过程中可以实时动态调整㊂实验结果表明,和随机进行任务分配相比,本文提出的方法在满足一定的原则和约束条件下,可以得到更高的收益损耗比,并且无人机飞行航程的均衡性更好㊂另外,和标准遗传算法相比,本文提出的改进遗传算法可以有效地扩展搜索空间,具有较高的全局搜索能力,不易陷入局部最优㊂参考文献:[1]㊀江更祥.浅谈无人机[J].制造业自动化,2011,33(8):110-112.[2]㊀楚瑞.基于蚁群算法的无人机航路规划[D].西安:西北工业大学,2006.[3]㊀杨剑峰.蚁群算法及其应用研究[D].杭州:浙江大学,2007.[4]㊀刘建华.粒子群算法的基本理论及其改进研究[D].长沙:中南大学,2009.[5]㊀李晓磊.一种新型的智能优化方法-人工鱼群算法[D].杭州:浙江大学,2003.[6]㊀AUSUBEL L M,MILGROM P R.Ascending AuctionsWith Package Bidding[J].Frontiers of Theoretical E-conomics,2002,1(1):1-42.[7]㊀刘昊旸.遗传算法研究及遗传算法工具箱开发[D].天津:天津大学,2005.[8]㊀牟健慧.基于混合遗传算法的车间逆调度方法研究[D].武汉:华中科技大学,2015.46。
- 1、下载文档前请自行甄别文档内容的完整性,平台不提供额外的编辑、内容补充、找答案等附加服务。
- 2、"仅部分预览"的文档,不可在线预览部分如存在完整性等问题,可反馈申请退款(可完整预览的文档不适用该条件!)。
- 3、如文档侵犯您的权益,请联系客服反馈,我们会尽快为您处理(人工客服工作时间:9:00-18:30)。
Feasibility of using EMG driven neuromusculoskeletal modelfor prediction of dynamic movement of the elbowTerry K.K.Koo,Arthur F.T.Mak*Jockey Club Rehabilitation Engineering Centre,The Hong Kong Polytechnic University,Hung Hom,Kowloon,Hong KongAbstractNeuromusculoskeletal (NMS)modeling is a valuable tool in orthopaedic biomechanics and motor control research.To evaluate the feasibility of using electromyographic (EMG)signals with NMS modeling to estimate individual muscle force during dynamic movement,an EMG driven NMS model of the elbow was developed.The model incorporates dynamical equation of motion of the forearm,musculoskeletal geometry and musculotendon modeling of four prime elbow flexors and three prime elbow extensors.It was first calibrated to two normal subjects by determining the subject-specific musculotendon parameters using computational opti-mization to minimize the root mean square difference between the predicted and measured maximum isometric flexion and extension torque at nine elbow positions (0–120°of flexion with an increment of 15°).Once calibrated,the model was used to predict the elbow joint trajectories for three flexion/extension tasks by processing the EMG signals picked up by both surface and fine electrodes using two different EMG-to-activation processing schemes reported in the literature without involving any trajectory fitting procedures.It appeared that both schemes interpreted the EMG somewhat consistently but their prediction accuracy varied among testing pro-tocols.In general,the model succeeded in predicting the elbow flexion trajectory in the moderate loading condition but over-drove the flexion trajectory under unloaded condition.The predicted trajectories of the elbow extension were noted to be continuous but the general shape did not fit very well with the measured one.Estimation of muscle activation based on EMG was believed to be the major source of uncertainty within the EMG driven model.It was especially so apparently when fine wire EMG signal is involved primarily.In spite of such limitation,we demonstrated the potential of using EMG driven neuromusculoskeletal modeling for non-invasive prediction of individual muscle forces during dynamic movement under certain conditions.Ó2004Elsevier Ltd.All rights reserved.Keywords:Electromyography;Neuromusculoskeletal modeling;Muscle mechanics;Elbow1.IntroductionIn vivo estimation of individual muscle forces is important to understanding the mechanisms of injuries,and predicting the outcome of surgical interventions.Unfortunately,direct measurement of muscle force in vivo is beyond the current state-of-the-art.Therefore,indirect methods based on mathematical models are widely used.Four major approaches including static optimization [3],dynamic optimization [50],neural net-work [54],and EMG assisted method [18,38]have been used to estimate individual muscle forces.For the static optimization,an objective function such as minimizing the muscle stress or muscle activation is employed at each instant of a motor task to partition the net joint moments calculated by inverse dynamics into respective muscular components.The drawbacks of this method are that the results are very sensitive to the choice of the objective function and it is very difficult to determine an appropriate objective function,which results in com-parable temporal patterns between the estimated muscle forces and measured EMG.Consequently,the muscle forces predicted by this method are questionable.1050-6411/$-see front matter Ó2004Elsevier Ltd.All rights reserved.doi:10.1016/j.jelekin.2004.06.007*Corresponding author.Tel.:+852********;fax:+852********.E-mail address:rcafmak@.hk (A.F.T.Mak)./locate/jelekinJournal of Electromyography and Kinesiology 15(2005)12–26Dynamic optimization is a process of choosing neural excitations by optimizing a well-defined performance criterion such as maximizing the jumping height in a ver-tical jumping task[50]and combining them with a neu-romusculoskeletal model to predict muscle forces and resulting kinematics.The temporal EMG patterns and the movement kinematics are usually used to validate the model indirectly.Theoretically,this approach pro-vides the preferred avenue for understanding human motor control strategy and muscle excitation signals. However,it is only applicable to normal subjects prima-rily because the so-called‘‘optimal performance’’may not be readily achieved by pathological subjects and is limited to movements in which the performance crite-rion is well defined.Obviously,the lack of versatility over a wide range of movements and its demanding computational cost limited its potential of being a useful tool for muscle force estimation.The use of artificial neural network to predict individual muscle forces is a relatively new approach.Although the method has potential advantages in terms of data collection,adapt-ability,and prediction;and the outputs can be validated indirectly by comparing the predicted net joint moments or kinematics with the measured one,its reliability is yet to be validated as the neural network algorithm acts in essence as a‘‘black box’’and is often not explicitly transparent.Using EMG signals as inputs to a musculoskeletal model to estimate individual muscle forces have been used by several groups for either isometric or dynamic tasks at different anatomical locations such as elbow [7,16,18,31,55],shoulder[35],knee[38–40,49,56],ankle [25–29,49],jaw[52],lower back[12,22,42,43,48],and wrist[9].The theoretical basis behind EMG driven model is that if the EMG signals can be measured precisely and processed adequately to reflect the acti-vation of each muscle crossing the joint and if the activation can be modulated properly by anatomical and musculotendon models,it is possible to accurately estimate individual muscle forces over a wide range of tasks and contraction modes.This makes EMG driven model to be a very attractive choice if individual mus-cle force estimation,rather than others like under-standing the motor control strategy,is the primary concern of the researchers.Implicit to the EMG dri-ven model is the EMG-to-activation processing,the musculotendon model,and the geometrical model of the joint,which entail a set of parameters that could affect the behavior of the model.Among many param-eters,some of them are regarded as calibration param-eters,which required to be tuned by some kinds of calibration procedure and the other parameters are re-garded asfixed parameters,whose values are either extracted from the literature[42]or assigned based on some subjective criteria[55].Depending on the model structure and assumptions made,the calibra-tion parameters can range from parameters with no physiological meaning such as gain or error factor [29],and EMG coefficients[9]to parameters with physiological meaning such as muscle rest length [11,33],tendon slack length[33,38],maximum isomet-ric stress[22,33],shape factor of the EMG–force rela-tionship[38,48],and electromechanical delay[18,48]. Different methods have also been used to determine the calibration parameters.For instance,some researchers use many calibration trials that manipulate certain conditions while attempting to hold others constants so that contribution of individual muscle can be isolated[27,56].Others attempt to determine multiple calibration parameters simultaneously using a set of calibration trials by systematically vary the parameters using non-liner least square optimization until the difference between the measured and pre-dicted net joint moment is minimized[7,11,33,40].An-other method is to use electrical stimulation to selectively activate a particular muscle and determine the parameters specific to that muscle[33,34].One of the limitations of most of the EMG driven models reported in the literature is that they tend to as-sume that once the model was calibrated by a limited set of calibration trials,the same set of model parameters could still yield reasonable prediction in other tasks and testing configurations.That is especially so for the previous elbow joint models[7,16,18,55].Before an EMG driven model can be used as a reliable tool for the estimation of muscle forces,additional validation across other tasks and testing configurations must be done.This study sought to develop an EMG driven neuro-musculoskeletal(NMS)model of the elbow that predicts individual muscle forces using surface andfine wire EMG recorded during voluntary elbowflexion–exten-sion movements.A set of subject-specific musculoten-don parameters within the model wasfirst determined based on a series of maximum isometricflexion and extension trials at nine elbow positions(0–120°offlex-ion with an increment of15°).Once calibrated,we sought to use the same set of parameters and the EMG signals as inputs to test the validity and versatility of the model at different dynamic movement activities by comparing the accuracy and consistency of the joint tra-jectories predicted by the model.2.Methods2.1.SubjectsTwo normal subjects(subject A:male,25years,174 cm,64.5kg;subject B:female,30years,156cm,44.5 kg)were recruited.Subjects gave their informed consent to the investigation,which was approved by the HongT.K.K.Koo,A.F.T.Mak/Journal of Electromyography and Kinesiology15(2005)12–2613Kong Polytechnic University human subject ethics committee.2.2.Experimental protocolThe subject sat on an assessment chair with the upper arm vertical and supported by an adjustable wooden plate.The shoulder was orientated at0°offlexion,10°of abduction,and neutral rotation.The forearm was in a supinated position and the wrist was in a neutral position with the hand completely relaxed.Aflexible electrogoniometer(Penny&Giles,UK),which was at-tached on the lateral side of the arm when the forearm was in a fully supinated position,was used to measure the angular displacement of the elbow joint.The elbow angle was defined as0°when the elbow was fully ex-tended.An eight channel telemetric surface EMG sur-face system with a bandwidth of10–500Hz and a gain of2000(Noraxon U.S.A.Inc.,Scottsdale,AZ)was used to capture the surface EMG signals of the long and short head of biceps(LHB and SHB),the brachioradi-alis(BRD),and the medial,lateral,and long heads of triceps(MHT,LatHT,and LngHT)picked up by pre-gelled Ag/AgCl6mm diameter bipolar surface elec-trodes(Blue Sensor,Medicotest,Denmark).Only a pair of electrodes was attached over the muscle belly of the biceps because the LHB was assumed to have the same activation as the SHB.The electrodes were placed paral-lel to the presumed direction of the musclefibers and at the locations suggested by Cram et al.[13]with inter-electrode distance set to2cm.A pre-sterilized Teflon coated nickel alloy pairedfine wire electrode(Nicolet Biomedical,Madison,WI),which was ready-loaded in a27Ga.30mm long hypodermic needle was used to measure the EMG signals of the brachialis(BRA). Diameter of each wire was50l m with2mm of metal exposed at the tips of the hooked ends.The hook ends were positioned so that2mm of one wire and5mm of the second wire exited at the end of the hypodermic needle,which ensured that there was no overlap of the bared tips.With the forearm positioned to the extended and supinated position,thefine wire electrode was in-serted about twofingerbreadths proximal to elbow crease along and just lateral to the biceps tendon to a depth about20mm.Correct placement of the electrode was confirmed by asking the subjects toflex the forearm in pronation against minimal resistance and see whether the EMG signals augmented significantly[51].After insertion,thefine wire electrode was connected to a dif-ferential amplifier(B&L Engineering,Tustin,CA)with a CMRR of95dB,an input impedance>100M X,a bandwidth of10–1000Hz,and a gain of330via a pair of screw-springs adaptors.A2cm loop was formed be-tween the insertion site and the pre-amplifier to avoid pulling on thefine wire electrode during movement.In addition,a pre-gelled Ag/AgCl10mm diameter surface ground electrode(Blue Sensor,Medicotest,Denmark) was placed over the olceranon.The skin around the elec-trode attachment or insertion sites were shaved and rubbed lightly by a cotton wool thatfilled with abrasive skin prepping gel(Nuprep e,USA)so that skin imped-ance could be greatly reduced.A cotton wool pad that soaked with alcohol was then used to clean the skin.Prior to the test,each subject was instructed to be completely relaxed with his/her forearm in a fully ex-tended position(i.e.0°)for theflexion test or in a fully flexed position($130°)for the extension test.The sub-jects were then instructed toflex/extend the elbow at his/her preferred speed(mean speed:151.6°±31.1°). Three experimental tasks were conducted and each pro-tocol was repeated three times.Thefirst task involved voluntary elbowflexion from a fully extended position to a fullyflexed position with a1.82-kg dead weight strapped around the arm at the location just proximal to the wrist.The second and the third tasks involved vol-untary elbowflexion and extension,respectively,with-out any added weight.The subjects had several practice trials prior to data collection.During the tests, both the EMG signals and elbow joint angles were re-corded simultaneously at2000Hz and stored in a PC computer via a12bit A–D converter(Data Translation, USA)for further off-line processing using Matlab(The Mathworks,Inc.,Natick,MA).A series of maximum voluntary contraction(MVC) trials was done right after completion of the experimen-tal tasks.The goals were to determine the subject specific musculotendon parameters and collect the MVC EMG amplitude of each muscle to normalize the EMG signals recorded during the experimental tasks.The MVC test-ing procedure has been reported elsewhere[33,34]. Briefly speaking,a Cybex dynamometer(Cybex Interna-tional Inc.,Medway,MA)was used to measure the tor-que during elbowflexion and extension while the subjects exerted maximum isometric voluntaryflexion (MIVF)and maximum isometric voluntary extension (MIVE)torques,respectively.Nine elbow positions (i.e.0–120°offlexion in increments of15°)were tested. At each position,the subject wasfirst instructed to relax and then gradually to increase theflexion or extension torque to maximum and to maintain at that level for approximately2s.Visual feedback of the torque was gi-ven to the subjects.The MIVF and MIVE were con-ducted alternatively,and a2-min recovery period was allowed between contractions to avoid muscle fatigue. Before the test,warm-up trials were allowed until the subjects became familiar with the testing protocol.2.3.Model developmentAn EMG driven NMS model of the elbow was devel-oped using Matlab(The Mathworks,Inc.,Natick,MA), which consisted of the linkage dynamics of the forearm14T.K.K.Koo,A.F.T.Mak/Journal of Electromyography and Kinesiology15(2005)12–26(including the hand)(Section2.5),the musculoskeletal geometry(Section2.7),the EMG-activation processing (Section2.4)and the musculotendon modeling(Section 2.6)of four prime elbowflexors(i.e.,LHB,SHB,BRA, BRD)and three prime elbow extensors(i.e.,MHT, LatHT,LngHT).Selection of these muscles was based on computing and comparing the potential moment contribution among the24muscles wrapping across the elbow joint[2].Inputs to the model included the EMG signals of each targeted muscle as well as the ini-tial elbow joint position and ing the subject-specific musculotendon parameters estimated from a series of maximum isometricflexion and extension trials (Section2.9),the model computed the elbow joint trajec-tories without trajectoryfitting procedure involved.The overall structure of the EMG driven model is illustrated in Fig.1.2.4.EMG-activation processingThefiltered EMG signals were converted to muscle activations using two different EMG-activation process-ing schemes reported in the literature–EMG to active state converter[26]and linear envelope processing[49].A brief description of each of them is as follows.2.4.1.EMG to active state converterBriefly speaking,the EMG to active state converter can be described mathematically asaðtÞ¼MaxMax½EðtÀtÃÞ 8tÃ:3:06tÃ<s2;Max½EðtÀtÃÞeÀðtÃÀs2Þs3 8tÃ:3:tÃP s2;(ð1Þwhere.’.stands for‘‘such that’’;a(t)is the active state, E(t)is processed EMG(i.e.bandpassfiltered,full-wave rectified,moving averaged with a50ms window and normalized by the corresponding MVC EMG).The ‘‘Max’’operator denotes that the maximal value of the respective functions is to be taken for the interval indi-cated.The working is such that a(t)follows E(t)when E(t)is rising.When a relative maximum of E(t)occurs, this maximum is held for a time period of s2and fol-lowed by an exponential decay with a time constant s3.This plateau or decay of a(t)goes on until E(t)or the plateau resulting from a subsequent relative maxi-mum E(t)becomes larger than a(t).The plateau dura-tion s2and decay constant s3were set to be30and60 ms,respectively,according to[28].This EMG-active state converter captures some well known properties of the muscle activation.For example, the response to a short EMG burst has a form of muscle twitch.Moreover,in quasi-static state,the smoothed rectified EMG is linearly proportional to the active state.Furthermore,the model shows summation effects in response to a series of impulses,to a step function, and to some combinations of steps and impulses.2.4.2.Linear envelope processingLinear envelope processing can be regarded as an EMG processing scheme that converts the raw EMG signals to a linear envelope(LE)profile that mimics the muscle tension waveform during dynamic changes of isometric tension.The LE profile is obtained by full-wave rectification of the bandpass EMG signals fol-lowed by forward and backward lowpassfiltering using a second-order Butterworthfilter with cut-offfrequency of3Hz andfinally normalized by the corresponding MVC EMG.The theoretical basis of LE processing is that the frequency characteristics of muscle twitches could be regarded as a second order,critically damped, low-passed system[44].Since each twitch can be consid-ered to be the impulse response of an active motor unit associated with a motor unit action potential(MUAP) impulse,the full-wave-rectified EMG can be considered as a summation of MUAPs of various amplitudes, whereas,muscle tension can be considered as a graded summation of the twitches resulting from all active mo-tor units.It has been reported that the contracting timeT.K.K.Koo,A.F.T.Mak/Journal of Electromyography and Kinesiology15(2005)12–2615of a twitch for different muscles ranged between20and 90ms[10,44].Since the cut-offfrequency of3Hz of a second-order Butterworthfilter corresponds approxi-mately to a contracting time of50ms,which can be re-garded as the averaged value reported in the literature,it represents a reasonable selection for the elbow muscles.2.5.Linkage dynamicsThe linkage dynamics of the EMG driven model de-scribed theflexion/extension of the elbow in the sagittal plane.The upper extremity was treated as consisting of two rigid bodies–the upper arm and the forearm,which included the hand.Theflexion/extension movement of the elbow joint was modeled as a frictionless hinge joint rotation of the ulna with respect to the humerus,with the axis of rotation passing through the centers of capi-tellum and trochlear sulcus[41].Carrying angle of the elbow joint was assumed to be unchanged as the fore-armflexed and extended about the elbow joint.For each subject,the segment mass,the moment of inertia,and the location of center of gravity of the fore-arm with respect to theflexion/extension axis were esti-mated using the formulas suggested by Winter[57]and Dempster[15].For thefirst protocol,the dead weight was treated as a hollow cylinder so that its moment of inertia about theflexion/extension axis could be approx-imated.Therefore,the second-order differential equa-tions that govern the motion of the forearm with and without the dead weight attached can be described, respectively,as follows:€q¼1I CX7j¼1F tjðtÞÃMA jðqðtÞÞÀm B gLÃBþm A gL AI CsinðqðtÞÞ;ð2aÞ€q¼1I BX7j¼1F tjðtÞÃMA jðqðtÞÞÀm B gLÃBI BsinðqðtÞÞ;ð2bÞwhere q;€q are the elbow angle and angular acceleration,respectively,F tj are tendon force transients for the j thmuscle,MA j are theflexion–extension moment arms ofF tj relative to the elbow joint center,g is the gravita-tional acceleration,m A and m B are the masses of the dead weight and the forearm,respectively.I B and I C are the moment of inertia of the forearm and the com-bined moment of inertia of the forearm and the dead weight about theflexion–extension axis,respectively.The parameter LÃB indicates the distance between thecenter of elbow joint and the mass center of the forearm and L A indicates the distance between the dead weight and the elbow joint center.2.6.Musculotendon modelingThe musculotendon model used in the current study was based on Giat et al.[20].Briefly speaking,each mus-cle was biomechanically modeled as a unit of three par-allel components(i.e.,CE,PE,and VE)where CE represents the active contractile element,and PE and VE represent the non-linear elastic component and the viscous component of thefluid-filled connective tissues of the muscles,respectively(Fig.2).It was assumed that all the musclefibers are parallel,equal in length and ori-ented at the same pennation angle(a).T represents the tendon,which was modeled as a stiffand non-linear elastic spring.M is the muscle mass and l m,l t,and l mt represent the musclefiber length,tendon length,and musculotendon length,respectively.The tendon length value represents the total of proximal and distal tendon lengths within a muscle.The empirical relationship be-tween muscle,tendon,and musculotendon lengths can be stated as follows:l mt¼l m cos aþl tð3Þand the equation of motion of each muscle along the tendon axis of pull can be given asMÁ€l m cos a¼F tÀF m cos a;ð4Þwhere F m=F p+F d+F a and F a¼r mÁPCSAÁf lðl mÞÁf vð_l mÞÁaðtÞ.Herein,F t is the tendon force,which was modeled to have an exponential force–strain relation-ship for strain<2%and a linear relationship afterwards[59].The muscle force(F m)equals the sum of the passive(F p),viscous(F v),and active(F a)force components of a muscle.F a is a function of the maximum muscle stress (r m),the physiological cross-section area(PCSA),the generic force-length function(f l),the generic force-ve-locity function(f v),and the level of muscle activation (a).F p was modeled to increase exponentially with re-spect to the musclefiber length[20]and F v was assumed to be proportional to the rate of change of musclefiber length[20].f l and f v were those employed by Giat et al.[20].Values of thefixed parameters such as PCSA and pennation angle within the model were either extracted or scaled from the literature[1,2,46,59],leaving three subject-specific musculotendon parameters per muscle16T.K.K.Koo,A.F.T.Mak/Journal of Electromyography and Kinesiology15(2005)12–26(i.e.,maximum muscle stress(r m),muscle optimal length(l mo),and tendon slack length(l to)),which were used to specify the force generating characteristics of each musculotendon unit.r m was assumed to be the same for muscles that belonged to the same func-tional group.Hence,the maximum muscle stress of the flexors muscles(i.e.,LHB,SHB,BRA,BRD)was de-noted as r f and that of the extensor muscles(i.e., MHT,LatHT,LngHT)was denoted as r e.All together, the EMG driven model featured16musculotendon parameters.2.7.Musculoskeletal geometryMoment arms(MA j)and musculotendon lengthsðl mtj Þof each targeted muscle over the elbow range ofmotion were estimated using a subject-specific interac-tive graphic-based geometrical model of the elbow joint, which was scaled from a generic geometrical model of the elbow joint[33,34]developed in SIMM(Musculo-Graphics,Chicago,USA)environment[14].The generic model defined the bone surfaces of the humerus,ulna, radius,hand,rib cage,scapula and clavicle,the joint kinematics of the elbow,and the musculotendon paths of those selected muscles.The bone surfaces were pro-vided by the SIMM,which were obtained by digitizing a male skeleton with known anthropometric dimen-sions.The musculotendon paths of those selected mus-cles were modeled as a series of points connected by line segments,which included the muscle origin and insertion points as well as the additional intermediate points that were defined when the muscle wrapped over the joint surface.Muscle origin and insertion points of each selected muscle were defined as the centroids of muscle attachment areas marked on a commercially available A11muscle skeleton(Kappa Medical,Pres-cott,USA).For each bone segment,three anatomical landmarks were identified to define a local coordinate system and the coordinates of those muscle origin and insertion points were digitized with respect to the afore-said local coordinate systems using a mechanical3-D digitizer(MicroScribe-3D,USA).These coordinates were then transformed and scaled to match the local coordinate systems of the generic model.The generic model was then scaled to specific subject model based on the ratio of segment lengths.2.8.Solution procedurePutting together,the dynamics of the NMS system can be represented as a system of second-order ordinary differential equations,which consists of the linkage dynamics(Eq.(2))and the musculotendon dynamics (Eq.(4))of each muscle.The system can also be ex-pressed as afirst-order vector–matrix differential equa-tion(Eq.(5))by defining a set of state variables:x1¼q;x2¼_q;x2jþ1¼l mj;x2jþ2¼_l mjfor j¼1;2; (7)_xðtÞ¼fðxðtÞ;aðtÀEMD emÞÞ;ð5Þwhere x=[x1,x2,...,x16]T is the state vector,a=[a1, a2,...,a7]T is the input vector,whose elements represent the muscle activation of each targeted muscle,and EM-D em is the electromechanical delay,which was set to50 ms for all muscles.The initial elbow position,i.e.x1(0), was set to0°forflexion tasks and120°for extension tasks.The initial elbow angular velocity,i.e.,x2(0), and the initial muscle velocity,i.e.,x2j+2(0)(for j=1,2,...,7)were all set to zero,and the initial muscle lengths,i.e.,x2j+1(0)(for j=1,2,...,7)were estimated from Eq.(3)by setting muscle velocity and acceleration as zero.A variable-order solver that based on the numerical differentiation formulas was used to solve the initial value problem owing to its high efficiency in solving stiffproblem[23].2.9.Determination of musculotendon parametersThe musculotendon parameters of theflexor(exten-sor)muscles were estimated by minimizing the root mean square difference between the measured and pre-dicted maximum isometricflexion(extension)torques using Nelder–Mead simplex method[47].A number of constraints were imposed on the optimization process. First,the total moment produced by the summation of passive elastic component of each muscle at10°offlex-ion was set to be less than2N m.This constraint is con-sistent with in vivo measurement of passive moment [21].Second,the tendon slack lengths must be greater than zero.Third,the muscle optimal lengths(l mo)fol-lowed the sequence:l mo(BRA)<l mo(LHB)<l mo (SHB)<l mo(BRD)for theflexors and l mo(MHT)<l mo (LatHT)<l mo(LngHT)for the extensors.This con-straint is consistent with cadaver data[1,2,46].Muscle optimal angles(/o)were either estimated using electrical stimulation[33]or adapted from the cadaver data[46]to act as constraints(i.e.,l mt(/o)=l to+l mo cos a)to guide the parameter searching process.Initial estimates of the length-related parameters of each muscle were based on anthropometric scaling of the cadaver data[46]and an initial estimate of maximum muscle stress was set to100N/cm2,which is within the range reported in the literature.2.10.Data analysisThe accuracy of the prediction was quantified by cal-culating the root mean square(RMS)difference between the predicted and measured joint trajectories.One-way ANOVA was used to test whether the prediction accu-racy was task dependent and paired t test was used toT.K.K.Koo,A.F.T.Mak/Journal of Electromyography and Kinesiology15(2005)12–2617。