Object retrieval with large vocabularies and fast spatial matching
2011Tell Me a Good Story and I may lend you money,the role of narratives in peer-to-peer decisions
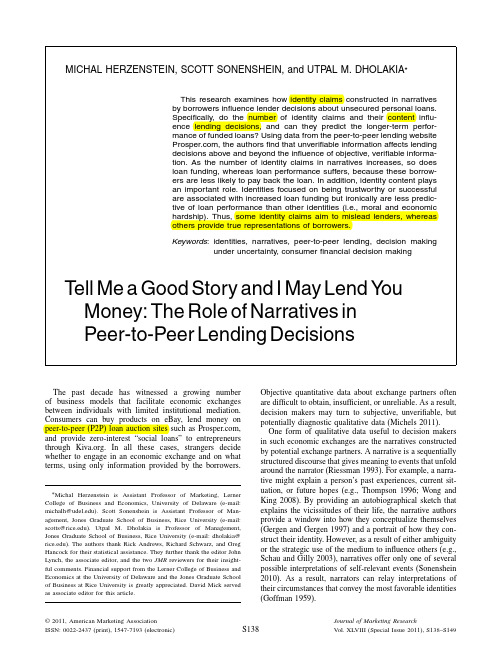
MICHAL HERZENSTEIN,SCOTT SONENSHEIN,and UTPAL M.DHOLAKIAThis research examines how identity claims constructed in narrativesby borrowers influence lender decisions about unsecured personal loans.Specifically,do the number of identity claims and their content influ-ence lending decisions,and can they predict the longer-term perfor-mance of funded loans?Using data from the peer-to-peer lending website,the authorsfind that unverifiable information affects lendingdecisions above and beyond the influence of objective,verifiable informa-tion.As the number of identity claims in narratives increases,so doesloan funding,whereas loan performance suffers,because these borrow-ers are less likely to pay back the loan.In addition,identity content playsan important role.Identities focused on being trustworthy or successfulare associated with increased loan funding but ironically are less predic-tive of loan performance than other identities(i.e.,moral and economichardship).Thus,some identity claims aim to mislead lenders,whereasothers provide true representations of borrowers.Keywords:identities,narratives,peer-to-peer lending,decision makingunder uncertainty,consumerfinancial decision making Tell Me a Good Story and I May Lend Y ou Money:The Role of Narratives inPeer-to-Peer Lending DecisionsThe past decade has witnessed a growing number of business models that facilitate economic exchanges between individuals with limited institutional mediation. Consumers can buy products on eBay,lend money on peer-to-peer(P2P)loan auction sites such as , and provide zero-interest“social loans”to entrepreneurs through .In all these cases,strangers decide whether to engage in an economic exchange and on what terms,using only information provided by the borrowers. *Michal Herzenstein is Assistant Professor of Marketing,Lerner College of Business and Economics,University of Delaware(e-mail: michalh@).Scott Sonenshein is Assistant Professor of Man-agement,Jones Graduate School of Business,Rice University(e-mail: scotts@).Utpal M.Dholakia is Professor of Management, Jones Graduate School of Business,Rice University(e-mail:dholakia@ ).The authors thank Rick Andrews,Richard Schwarz,and Greg Hancock for their statistical assistance.They further thank the editor John Lynch,the associate editor,and the two JMR reviewers for their insight-ful comments.Financial support from the Lerner College of Business and Economics at the University of Delaware and the Jones Graduate School of Business at Rice University is greatly appreciated.David Mick served as associate editor for this article.Objective quantitative data about exchange partners often are difficult to obtain,insufficient,or unreliable.As a result, decision makers may turn to subjective,unverifiable,but potentially diagnostic qualitative data(Michels2011). One form of qualitative data useful to decision makers in such economic exchanges are the narratives constructed by potential exchange partners.A narrative is a sequentially structured discourse that gives meaning to events that unfold around the narrator(Riessman1993).For example,a narra-tive might explain a person’s past experiences,current sit-uation,or future hopes(e.g.,Thompson1996;Wong and King2008).By providing an autobiographical sketch that explains the vicissitudes of their life,the narrative authors provide a window into how they conceptualize themselves (Gergen and Gergen1997)and a portrait of how they con-struct their identity.However,as a result of either ambiguity or the strategic use of the medium to influence others(e.g., Schau and Gilly2003),narratives offer only one of several possible interpretations of self-relevant events(Sonenshein 2010).As a result,narrators can relay interpretations of their circumstances that convey the most favorable identities (Goffman1959).©2011,American Marketing AssociationISSN:0022-2437(print),1547-7193(electronic)S138Journal of Marketing ResearchV ol.XLVIII(Special Issue2011),S138–S149Role of Narratives in Peer-to-Peer Lending Decisions S139RESEARCH MOTIVATIONS AND CONTRIBUTIONS The idea that narratives may involve the construction of a favorable identity poses two key questions for research. First,whereas narratives can provide diagnostic informa-tion to a decision maker who is considering an economic exchange,the veracity of the narrator’s story is difficult to determine.Because a narrative offers the possibility of describing either an authentic,full,true self or a partial, inauthentic,misleading self,potential exchange partners are left to intuit the truth of the presentation.Accordingly a key question to consider is,given their potential for diagnostic and misleading information,to what extent do narratives influence economic exchange transactions?Previous con-sumer research has largely focused on narratives of con-sumption experiences(Thompson1996)or consumption stories(Levy1981),but scholars have not examined the role of narratives in economic exchanges.We believe that narratives may be a particularly powerful lens,in that they allow the consumer to attempt to gain better control over the exchange and thus can provide a means to help con-summate the exchange.Second,to what extent do narratives affect the perfor-mance and outcomes of an economic exchange?Narrative scholars claim that the construction and presentation of a narrative can shape its creator’s future behavior(Bruner 1990)but rarely examine the nature of this influence empir-ically.Such an examination would be critical to understand-ing how a mixture of quantitative and qualitative factors shapes outcome quality(e.g.,Hoffman and Yates2005). We examine these two questions using data from the online P2P loan auctions website,,by studying borrower-constructed narratives(particularly the identities embedded in them),the subsequent decisions of lenders, and transaction performance two years later.We define identity claims as the ways that borrowers describe them-selves to others(Pratt,Rockmann,and Kaufmann2006). Borrowers can construct an identity based on a range of ele-ments,such as religion or success.The elements become an identity claim when they enter public discourse as opposed to private cognition.With this framework,we make several contributions.First,by developing and testing theory around how nar-ratives supplement more objective sources of information that decision makers use when considering afinancial trans-action,we draw attention to how narrators can intentionally exploit uncertainty and favorably shape circumstantial facts to obtain resources,such as access to money in unmediated environments.The narrative,as a supplementary,yet some-times deal-making or deal-breaking,information source for decision makers is predicated on compelling stories versus objective facts.It thus offers a means for people to recon-struct their pasts and describe their futures in positive ways. Second,by linking narratives to objective performance measures,we show how narratives may predict the longer-term performance of lending decisions.Because the deci-sion stakes are high in this unmediated and unsecured financial arena,lenders engage in highly cognitive pro-cessing(Petty and Wegener1998).The strong disincen-tive of potentialfinancial loss leads to cognitive process-ing,which tends to produce accurate attributions about a person and the probabilities of future events(Osborne and Gilbert1992).Because of this motivation for accuracy,we suspect lenders use narratives to help them make invest-ment decisions.Third,from a practice perspective,the recentfinancial crisis has exposedflaws in the criteria used to make lend-ing decisions.Quantitativefinancial metrics,such as credit scores,have proven unreliable for predicting the ability or likelihood of consumers to repay unsecured loans(Feldman 2009).A narrative perspective on the consummation and performance offinancial transactions offers the promise of improving systems for assessing borrowers.RESEARCH SETTINGWe conducted our research on (hereinafter, Prosper),the largest P2P loan auction site in the United States,with more than one million members and$238mil-lion in personal loans originated since its inception in March2006(as of June2011).On Prosper,borrowers and lenders never meet in person,so we can assess the role of narratives in overcoming the uncertainty that arises during financial transactions between unacquainted actors.The process of borrowing and lending money through a loan auction on Prosper is as follows:Before posting their loan request,borrowers give Prosper permission to ver-ify relevant personal information(e.g.,household income, home ownership,bank accounts)and access their credit score from Experian,a major credit-reporting ing this and other information,such as pay stubs and income tax returns,Prosper assigns each borrower a credit grade that reflects the risk to lenders.Credit grades can range from AA, which indicates that the borrower is extremely low risk(i.e., high probability of paying back the loan),through A,B,C, D,and E to HR,which signifies the highest risk of default. Borrowers then post loan requests for auction.When post-ing their loan auctions,borrowers choose the amount(up to $25,000)and the highest interest rate they will pay.They also may use a voluntary open-text area,with unlimited space,to write anything they want—that is,the borrower’s narrative(see Michels2011).After the listing becomes active,lenders decide whether to bid,how much money to offer,and the interest rate. A$1,000loan might befinanced by one lender who lends $1,000or by40lenders,each lending$25for example. Most lenders bid the minimum amount($25)on individual loans to diversify their portfolios(Herzenstein,Dholakia, and Andrews2011).After the auction closes,listings with bids that cover the requested amount are funded.If a list-ing receives bids covering more than its requested amount, the bids with the lowest interest rates win.If the auc-tion does not receive enough bids,the request remains unfunded.Prosper administers the loan,collects payments, and receives fees of.5%to3.0%from borrowers,as well as a1%annual fee from lenders.We employed three dependent variables in our study. First,loan funding is the percentage of the loan request to receive a funding commitment from lenders.For exam-ple,if a loan request for$1,000receives bids worth$500, loan funding is50%.If it receives bids worth$2,000,loan funding equals200%.A higher loan funding value signi-fies greater lender interest.Second,percentage reduction infinal interest rate captures the decrease in the inter-est rate between the borrower’s maximum specified rate and thefinal rate.For example,if a borrower’s maximumS140JOURNAL OF MARKETING RESEARCH,SPECIAL ISSUE 2011T able 1DEFINITIONS OF IDENTITIES AND EXAMPLES FOR DATA CODINGIdentityDefinitionExamplesTrustworthy (Duarte,Siegel,and Young 2009)Lenders can trust the borrower to pay back the money on time.“I am responsible at paying my bills and lending me funds would be a good investment.”(Listing #17118)Successful (Shafir,Simonson,and Tversky 1993)The borrower is someone with a successful business or job/career.“I have [had]a very solid and successful career with an Aviation company for the last 13years.”(Listing #18608)Hardworking (Woolcock 1999)The borrower will work very hard to pay the loan back.“I work two jobs.I work too much really.I work 26days a month with both jobs.”(Listing #18943)Economic hardship (Woolcock 1999)The borrower is someone in need because of hardship,as a result of difficult circumstances,bad luck,or other misfortunes that were,or were not,under the borrower’s control.“Unfortunately,a messy divorce and an irresponsible ex have left me with awful credit.”(Listing #20525)Moral (Aquino et al.2009)The borrower is an honest or moral person.“On paper I appear to be an extremely poor financial risk.In reality,I am an honest,decent person.”(Listing #17237)Religious (Weaver and Agle 2002)The borrower is a religious person.“One night,the Lord awaken me and myspouse our business has been an enormous success with G-d on our side.”(Listing #21308)interest rate is 18%and the final rate is 17%,the per-centage reduction in interest rate is 18−17 /18=5 56%.rate decreases only if the loan request receives full greater lender interest results in greater reduc-of the interest rate.Third,loan performance is the payment status of the loan two years after its origination.We further classify the types of identity claims made by prospective borrowers.Borrowers in our sample employed six identity claims in their narratives:trustworthy,economic hardship,hardworking,successful,moral,and religious.In Table 1,we provide definitions and illustrative examples of each identity claim.Borrowers provided an average of 1.53 SD =1 14 identity claims in their narratives.RESEARCH HYPOTHESESWho Is Likely to Provide More Identity Claims in Their Narratives?Narratives,when viewed as vehicles for identity work,provide opportunities for people to manage the impres-sions that others hold of them.Impression management the-ory posits that people want to create and maintain specific identities (Leary and Kowalski 1990).Narratives provide an avenue for impression management;through discourse,people can shape situations and construct identities that are designed specifically to obtain a desired outcome (Schlenker and Weigold 1992).Some scholars argue that people use narratives strategically to establish,maintain,or protect their desired identities (Rosenfeld,Giacalone,and Riordan 1995).However,the use of impression manage-ment need not automatically signal outright lying;people may select from a repertoire of self-images they genuinely believe to be true (Leary and Kowalski 1990).Nevertheless,strategic use of impression management means that,at a minimum,people select representations of their self-image that are most likely to garner support.In economic exchanges involving repeated transactions,each party receives feedback from exchange partners thateither validates or disputes the credibility of their self-constructions (Leary and Kowalski 1990),so they can determine if an identity claim has been granted.Prior transactions also offer useful information through feedback ratings and other mechanisms that convey and archive rep-utations (Weiss,Lurie,and MacInnis 2008).However,in one-time economic exchanges,such feedback is not avail-able.Instead,narrators have a single opportunity to present a convincing public view of the self,and receivers of the information have only one presentation to deem the presen-ter as credible or not.We hypothesize that in these conditions,borrowers are strategic in their identity claims.Borrowers with satisfac-tory objective characteristics are less likely to construct identity claims to receive funding;they feel their case stands firmly on its objective merits alone.In contrast,borrowers with unsatisfactory objective characteristics may view narratives as an opportunity to influence the attribu-tions that lenders make,because in narratives,they can counter past mistakes and difficult circumstances.In this scenario,borrowers make identity claims that offset the attributions made by lenders about the borrower being fundamentally not a creditworthy person.These disposi-tional attributions are often based on visible characteristics (Gilbert and Malone 1995).The most relevant objective characteristic of borrowers is the credit grade assigned by Prosper,derived from the borrower’s personal credit history (Herzenstein,Dholakia,and Andrews 2011).With more than one identity claim,borrowers can present a more com-plex,positive self to counteract negative objective informa-tion,such as a low credit grade.Thus:H 1:The lower the borrower’s credit grade,the greater isthe number of identities claimed by the borrower in the narrative.Role of Narratives in Peer-to-Peer Lending Decisions S141Impact of the Number of Identity Claims onLenders’DecisionAlthough economists often predict that unverifiable information does not matter(e.g.,Farrell and Rabin1996), we suggest that the number of identity claims in a bor-rower’s narrative play a role in lenders’decision making, for at least two reasons.First,borrower narratives with too few identities may fail to resolve questions about the borrower’s disposition.If a borrower fails to provide suf-ficient diagnostic information for lenders to make attri-butions about the borrower(Cramton2001),lenders may suspect that the borrower lacks sufficient positive or dis-tinctive information or is withholding or hiding germane information.Second,the limited diagnostic information provided by fewer identity claims limits a decision maker’s ability to resolve outcome uncertainties.Research on perceived risk supports this reasoning;decision makers gather informa-tion as a risk-reduction strategy and tend to be risk averse in the absence of sufficient information about the decision (e.g.,Cox and Rich1964).In the P2P lending arena,the loan request and evaluation process unfold online with-out any physical interaction between the parties.Further-more,on Prosper,borrowers are anonymous(real names and addresses are never revealed).This lack of seemingly relevant information is especially salient,because many decision makers view unmediated online environments as ripe for deception(Caspi and Gorsky2006).To the extent that the identity claims presented in a narrative reduce uncertainty about a borrower,lenders should be more likely to view the listing favorably,increase loan funding,and decrease thefinal interest rate.Therefore,the number of identity claims in a borrower’s narrative may serve as a heuristic for assessing the borrower’s loan application and lead to greater interest in the listing.Although we suggest that the number of identities bor-rowers claim result in favorable lending decisions,we also argue that these identity claims may persuade lenders erroneously,such that lenders fund loans with a lower likelihood of repayment.Borrowers can use elaborate multiple-identity narratives to craft“not-quite-true”stories and make promises they mightfind difficult to keep.More generally,a greater number of identity claims suggests that borrowers are being more strategic and positioning them-selves in a manner they believe is likely to resonate with lenders,as opposed to presenting a true self.Therefore,we posit that,consciously or not,borrowers who construct sev-eral identities may have more difficulty fulfilling their obli-gations and be more likely to fall behind on or stop loan repayments altogether.Thus,despite the high stakes of the decision,lenders swayed by multiple identities are more likely to fall prey to borrowers that underperform or fail (Goffman1959).H2:Controlling for objective,verifiable information,the more identities borrowers claim in their loan requests,the morelikely lenders are to(a)fund the loan and(b)reduce itsinterest rate,but then(c)the lower is the likelihood of itsrepayment.Role of the Content of Identity Claims onLender Decision Making and Loan PerformanceWe also examine the extent to which select identities affect lenders’decision making and the longer-term per-formance of loans.With a limited theoretical basis for determining the types of identities most likely to influ-ence lenders’decision making,this part of our study is exploratory.Research on trust offers a promising starting point(Mayer,Davis,and Schoorman1995),because it sug-gests that identities may reduce dispositional uncertainty and favorably influence lenders.Trust is a crucial element for the consummation of an economic exchange(Arrow 1974).Scholars theorize that trust involves three compo-nents:integrity(borrowers adhere to principles that lenders accept),ability(borrowers possess the skills necessary to meet obligations),and benevolence(borrowers have some attachment to lenders and are inclined to do good)(Mayer, Davis,and Schoorman1995).We theorize that trustworthy,religious,and moral iden-tities increase perceptions of integrity because they lead lenders to believe that borrowers ascribe to the lender-endorsed principle of fulfilling obligations,either directly (trustworthy)or indirectly by adhering to a philosophy (religious or moral).Specifically,a moral identity tells potential lenders that the person has“a self-conception organized around a set of moral traits”(Aquino et al.2009, p.1424),which should increase perceptions of integrity.A religious identity signals a set of role expectations to which a person is likely to adhere,and though religions vary in the content of these expectations(Weaver and Agle 2002),many of them include principles oriented against lying or stealing and toward honoring contractual agree-ments.A hardworking identity should increase perceptions of integrity,because hardworking people are determined and dependable,which often makes them problem solvers (Witt et al.2002),meaning that they will do their best to meet their obligations,a disposition likely to resonate with lenders.We also reason that the religious and moral identities invoke in lenders a sense of benevolence,which is a foun-dational principle of many religions and moral philoso-phies.Similarly,the economic hardship identity may invoke benevolence,because the borrower exhibits forthrightness about his or her past mistakes and thus suggests to lenders that the borrower is trying to create a meaningful relation-ship based on transparency.We theorize that an identity claim of success can increase perceptions of ability and the belief that the narrator is capable of fulfilling promises(Butler1991).Lenders are more likely to lend money to a borrower if they perceive that the person is capable of on-time repayment(Newall and Swan2000).A successful identity likely describes the past or present,but it also can serve as an indication of a probable future(i.e.,the borrower will continue to be successful),which helps“fill in the blanks”about the bor-rower in a positive way.In contrast,economic hardship likely constructs the borrower as someone who has had a setback,which ultimately undermines perceptions of ability and thus negatively affects lenders’decisions.We have offered some preliminary theory in support of these specific relationships between identity content and loan funding/interest rate reductions,but this examinationS142JOURNAL OF MARKETING RESEARCH,SPECIAL ISSUE2011remains exploratory,so we pose these relationships as exploratory research questions(ERQ):ERQ1:Which types of identity claims influence lending deci-sions,as indicated by(a)an increase in loan fundingand(b)a decrease in thefinal interest rate?We also explore the impact of the content of identity claims on loan performance.We envision two potential sce-narios.In thefirst,identities are diagnostic of the borrower or serve as self-fulfilling prophecies.Examining the ability aspect of trustworthiness,we anticipate a negative relation between an economic hardship identity and loan perfor-mance(borrowers validate their claim of setbacks)but a positive relation between a successful identity and loan per-formance(borrowers prove their claim of past success). Moreover,we expect the four integrity-related identities—trustworthy,hardworking,moral,and religious—to indicate better loan performance.After a self-presentation as having integrity,the borrower probably has a strong psychological desire for consistency between the narrative and his or her actions(Cialdini and Trost1998).That is,in their narra-tives,borrowers may make an active,voluntary,and public commitment that psychologically binds them to a partic-ular set of beliefs and subsequent behaviors(Berger and Heath2007).Because these four identities speak to funda-mental self-beliefs versus predicted outcomes(e.g.,success or hardship),they can strongly motivate borrowers to live up to their claims.Thus these identities,regardless of their accuracy,can become true and predict the performance of the lending decision.In the other scenario,however,identities improve the lender’s impression of the borrower,thereby allowing bor-rowers to exert control over the provided impressions (Goffman1959).Borrowers(or narrators,more generally) construct positive impressions and may misrepresent them-selves and send signals that may not be objectively war-ranted.Despite the belief that self-constructing identities are helpful for a lending decision,they actually may have no impact or even be harmful to lenders.These mixed pos-sibilities lead to another exploratory research question: ERQ2:How are the content of identity claims and loan perfor-mance related?STUDYDataOur data set consists of1,493loan listings posted by borrowers on Prosper in June2006and June2007.We extracted this data set using a stratified random sampling ing a web crawler,we extracted all loan listings posted in June2006and June2007(approximately5,400 and12,500listings,respectively).A significant percentage of borrowers on Prosper have very poor credit histories, and most loan requests do not receive funding.To avoid overweighting high-risk borrowers and unfunded loans,we sampled an equal number of loan requests from each credit grade.To do so,wefirst separated funded loan requests from unfunded ones,then divided each group by the seven credit grades assigned by Prosper.We also eliminated all loan requests without any narrative text,for three reasons. First,including loan requests without narratives could con-found the borrower’s choice to write something other than narratives in the open text box with the choice to write nothing at all.Second,the vast majority of listings lack-ing a narrative do not receive funding.Third,loan requests without text represent only9%of all loans posted in June 2006and4%of those posted in June2007.We nevertheless used the“no text”loans in our robustness check.We randomly sampled posts from the14subgroups (2funding status×7credit grades).In2006,we sampled 40listings from each subgroup(until data were exhausted) to obtain513listings;in2007,we sampled70listings from each subgroup to obtain980listings,for a total of 1,493listings.Each listing includes the borrower’s credit grade,requested loan amount,maximum interest rate,loan funding,final interest rate of funded loans,payback sta-tus of funded loans after two years,and open-ended text data.Before combining the data from2006and2007,we tested for a year effect but found none,which supports their combination.Dependent VariablesThefirst dependent variable,loan funding,ranges from 0%to905%in our data set,but requiring an equal inclu-sion of all credit ratings skews these statistics.The mean percentage funded(including all listings)is105.74%(SD= 129 2)and that for funded listings is205.45%(SD=119 6). Because it was skewed,we log-transformed loan fund-ing as follows:Ln(percent funded+1).The second depen-dent variable,percentage reduction in thefinal interest rate, ranges from0%to56%in our data set.The mean per-centage reduction in interest rate for all listings is6.4% (SD=10 7)and for funded listings is11.88%(SD=12 75). Because the distribution is skewed,we log-transformed it (we provide the distributionfigures in the Web Appendix, /jmrnov11).The third dependent variable is loan performance,mea-sured two years after loan funding.For each funded loan in our data set,we obtained data about whether the loan was paid ahead of schedule and in full(31.1%of funded list-ings),was current and paid as scheduled(40.5%),involved payments between one and four months late(7.1%),or had defaulted(21.3%).This dependent variable may appear ordered,but the likelihood ratio tests reveal that a multino-mial logit model fares better than an ordered logit model for analyzing these data(for both the number and content of identities).Thus,in the following analysis,we employ a multinomial logit model.Independent VariablesWe read approximately one-third of all narratives and developed our inductively derived list of six identity claims (Miles and Huberman1994):trustworthy,economic hard-ship,hardworking,successful,moral,and religious,as we define in Table1.Two research assistants examined the same data and determined these six identities were exhaustive.Next,five additional pairs of research assistants (10total)coded the entire data set.We coded each iden-tity as a dichotomous variable that receives the value of1 if the identity claim was present in a borrower’s narrative and0if otherwise.A pair of research assistants read each listing in the data set,independently atfirst,then discussed them to determine the unified code for each listing.Accord-ing to20randomly sampled listings from our data set,used。
1 Object Acquaintance Selection and Binding
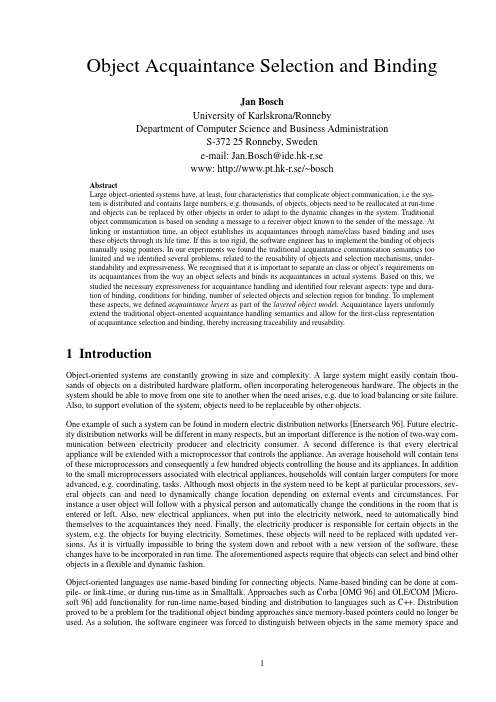
Object Acquaintance Selection and BindingJan BoschUniversity of Karlskrona/RonnebyDepartment of Computer Science and Business AdministrationS-372 25 Ronneby, Swedene-mail: Jan.Bosch@ide.hk-r.sewww:http://www.pt.hk-r.se/~boschAbstractLarge object-oriented systems have, at least, four characteristics that complicate object communication, i.e the sys-tem is distributed and contains large numbers, e.g. thousands, of objects, objects need to be reallocated at run-timeand objects can be replaced by other objects in order to adapt to the dynamic changes in the system. Traditionalobject communication is based on sending a message to a receiver object known to the sender of the message. Atlinking or instantiation time, an object establishes its acquaintances through name/class based binding and usesthese objects through its life time. If this is too rigid, the software engineer has to implement the binding of objectsmanually using pointers. In our experiments we found the traditional acquaintance communication semantics toolimited and we identified several problems, related to the reusability of objects and selection mechanisms, under-standability and expressiveness. We recognised that it is important to separate an class or object’s requirements onits acquaintances from the way an object selects and binds its acquaintances in actual systems. Based on this, westudied the necessary expressiveness for acquaintance handling and identified four relevant aspects: type and dura-tion of binding, conditions for binding, number of selected objects and selection region for binding. To implementthese aspects, we defined acquaintance layers as part of the layered object mode l. Acquaintance layers uniformlyextend the traditional object-oriented acquaintance handling semantics and allow for the first-class representationof acquaintance selection and binding, thereby increasing traceability and reusability.1 IntroductionObject-oriented systems are constantly growing in size and complexity. A large system might easily contain thou-sands of objects on a distributed hardware platform, often incorporating heterogeneous hardware. The objects in the system should be able to move from one site to another when the need arises, e.g. due to load balancing or site failure. Also, to support evolution of the system, objects need to be replaceable by other objects.One example of such a system can be found in modern electric distribution networks [Enersearch 96]. Future electric-ity distribution networks will be different in many respects, but an important difference is the notion of two-way com-munication between electricity producer and electricity consumer. A second difference is that every electrical appliance will be extended with a microprocessor that controls the appliance. An average household will contain tens of these microprocessors and consequently a few hundred objects controlling the house and its appliances. In addition to the small microprocessors associated with electrical appliances, households will contain larger computers for more advanced, e.g. coordinating, tasks. Although most objects in the system need to be kept at particular processors, sev-eral objects can and need to dynamically change location depending on external events and circumstances. For instance a user object will follow with a physical person and automatically change the conditions in the room that is entered or left. Also, new electrical appliances, when put into the electricity network, need to automatically bind themselves to the acquaintances they need. Finally, the electricity producer is responsible for certain objects in the system, e.g. the objects for buying electricity. Sometimes, these objects will need to be replaced with updated ver-sions. As it is virtually impossible to bring the system down and reboot with a new version of the software, these changes have to be incorporated in run time. The aforementioned aspects require that objects can select and bind other objects in a flexible and dynamic fashion.Object-oriented languages use name-based binding for connecting objects. Name-based binding can be done at com-pile- or link-time, or during run-time as in Smalltalk. Approaches such as Corba [OMG 96] and OLE/COM [Micro-soft 96] add functionality for run-time name-based binding and distribution to languages such as C++. Distribution proved to be a problem for the traditional object binding approaches since memory-based pointers could no longer be used. As a solution, the software engineer was forced to distinguish between objects in the same memory space andobjects in other memory spaces. The uniformity of this approach is clearly less than optimal and the software engi-neer, in some way, has to implement the interaction with remote objects manually.Regardless of distribution, name-based binding is too rigid in many situations. For this reason, most object-oriented languages provide some form of object pointers. Object pointers provide a primitive mechanism that allows the soft-ware engineer to hand-code customised object selection and binding mechanisms. The problem of pointers, however, is that the specifications for selecting and binding an acquaintance object becomes an integral part of the class specifi-cation and that the object nor the selection and binding mechanism can be reused separately.The name-based object binding and the manual object selection which is programmed as part of the class specification increase the dependence of the class on the environment in which it is used. If the class should be reused in a context different from the context for which it was originally defined the name of one of its acquaintance objects might be dif-ferent as well as that the binding procedures for some of its object pointers might be different. If the selection and binding is embedded in the class code, this reduces reusability considerably.In this paper, we study the problem of acquaintance selection and binding for objects in the new class of objects sys-tems that is appearing: large, distributed and open object systems in which objects are constantly created, moved around and removed from the system. The traditional notion of an application as a closed, specialised entity with its own data stored in its own database and a tailored user-interface is rapidly being replaced with another perspective in which objects operate in large, distributed systems and make use of offered services and work in an integrated manner with other objects. Traditional applications only have vertical interfaces, i.e. interaction with the operating system and the user-interface system, whereas the new type of applications, in addition to the vertical interface, also has a hori-zontal interface, i.e. an interface to other applications and parts of other applications. The conventional object model provides only limited features to deal with the requirements imposed by these new application types and its use may lead to a number of problems with respect to acquaintance handling. As a solution to these problems, we present an extension in the form of a new layer type Acquaintance, to the layered object model (LayOM), our object-oriented research language, that provide solutions to the identified problems.Our approach to a solution of the acquaintance handling problems is to explicitly distinguish between the specification of the various acquaintances required by an object and the actual selection and binding process in which one or more instances play the role of an acquaintance for an object. By separating these two aspects of object acquaintances, one can specify classes as general as possible and define for individual instances of the classes how the required acquaint-ances should be selected and bound.For the discussion of acquaintance handling in this paper, we have adopted a hierarchical object system view which is different from the traditional view. Since the size of the object system that we described before will be like thousands of objects, the traditional ‘sea of object’ approach is really not suitable. Even when one distinguishes between local and remote objects, the number of remote objects will be too large to handle in an efficient way. It is necessary to impose some structure on the object system, and the hierarchical structure is a very appropriate approach. This hierar-chical grouping of objects is also used in object-oriented analysis and design through the notion of subsystems. A large application can recursively be decomposed into subsystems, leading to a hierarchical structure. However, at the implementation level no corresponding concept is available, leading to two possible approaches. Most software engi-neers do not implement subsystems and the resulting situation is the ‘sea of objects’ in which the software engineer has to specify which objects communicate with each other. The other approach which is taken is that subsystems are implemented as composed objects, encapsulating the objects in the subsystem. The advantage of this approach is that the objects are organised in a hierarchical structure, which improves the overview over the system. An important dis-advantage, however, is that since the objects are fully encapsulated in the subsystem, but still need to communicate with objects in other subsystems, the subsystem objects need to implement considerable amounts of methods that only forward messages to objects contained in them. Thus, subsystems have different requirements on encapsulation than objects and both not implementing subsystems and implementing subsystems as composed objects lead to problems. As a solution, we allow objects to extend their interface, which conventionally only consists of public methods, with part objects. So, part objects that are explicitly specified to be visible from outside the encapsulating object can be called directly by other objects. By providing flexible encapsulation, it becomes much more feasible to represent sub-systems at the implementation level as composed objects that can partially be seen through. However, these aspects are currently under investigation and will be described in a future paper.The remainder of this paper is organised as follows. In the next section, our view on the object-oriented system think-ing is presented that provides a context and justification for the work presented in this paper. In section 3, the prob-lems that we identified with acquaintance selection and binding in the conventional object-oriented model aredescribed. Subsequently, in section 4, the expressiveness that we believe to be necessary for dealing with object acquaintances is described. In section 5 the layered object model is introduced and the extensions that deal with object acquaintances are described. Section 6 is concerned with related work and the paper is concluded in section 7.2 System OverviewWhereas traditional applications of object-oriented systems have been closed and relatively static in their nature, a new class of systems is arising in which necessary properties are openness and dynamicity. Other characteristics of these new systems are distribution and their large size, both in the number of objects and in the geographical coverage of these systems. Parts of applications such as object communication that previously were hard-coded in the class code need to be defined in a more flexible and expressive manner.An important aspect is the notion of communication between cooperating objects. The binding of cooperating objects traditionally was performed statically during the compilation or linking stage or dynamically through passing object references. Since selecting and binding an acquaintance is a more complex and dynamic process in large, distributed systems, additional functionality is required from the object-oriented language in which these systems are imple-mented. In these distributed systems, the number of possibilities to select an object to communicate with is much larger. Consequently, more expressive mechanisms for specifying acquaintance handling are required.Before an object can communicate with an acquaintance, three aspects have to be fulfilled. The first is that the object contains a specification of the requirements it puts on its acquaintance, i.e. the acquaintance has to fulfil certain requirements, e.g. be an instance of a particular class. Secondly, at some point, be it at the time of definition, linking or instantiation or when the object tries to send the first message to the acquaintance, a selection process has to be per-formed to select the acquaintance from the objects in the context of the object. Finally, a binding step has to take place in which the object binds itself to the acquaintance and uses the acquaintance for its communication. These steps can be identified in virtually all module-based systems, but the way these steps are performed is often embedded and hard-coded in the language implementation, leaving no flexibility to the software engineer.To understand the communication mechanisms required in the aforementioned new class of systems, the characteris-tics of large, distributed systems need to be described. The following characteristics of these systems we consider rel-evant for object communication: the notion of system, hierarchical object organisation and object group communication. To justify the relevance of these characteristics, three example system domains where acquaintance handling is an important issue are described in the next section. Subsequently, in section 2.2, the architecture of large object systems, as used in the remainder of the paper, is discussed.2.1 Example SystemsThe issues of acquaintance handling addressed in this paper are perhaps primarily relevant for open, dynamic applica-tions, although also traditional, closed applications can benefit. In these traditional applications, the references between objects often are rather static during the execution of the program. The need for more expressive communica-tion constructs is, consequently, not so acute. However, in current and future application domains, several authors have recognised a need for more expressive communication constructs. In the following sections, three application domains in which we have experienced the need for flexible object communication are described.2.1.1 Integrated manufacturing systemsManufacturing systems have already reached some level of integration. Especially at the level of the production cell, there is a rather high level of integration. The different equipment parts of the production cell communicate frequently and perform tasks together. The equipment in the production cell has hard real-time tasks, such as the control of a robot arm, soft real-time tasks, such as changing a tool in a machine, and non real-time computation, such as the gen-eration of the production figures for the last hour.Already today and even more so in the future, manufacturing systems are becoming more and more integrated in their architecture. This is necessary to achieve the flexibility and openness that is required by the new demands on manu-facturing systems, such as efficient and cost effective production of small series and individual products. In such an integrated manufacturing system, the production demands on the system and its structure will be constantly changing. This flexibility will also affect the communication between the objects in the system.The software part of a manufacturing system can be viewed as a large, distributed system in which the relevant entities in the factory are represented by objects. Several objects, e.g. objects representing the products under manufacturing, are changing context rather frequently and therefore need to select and bind themselves to new objects after every context change. The manufacturing system is supposed to be highly flexible, meaning that production cells can dynamically be added to and removed from the system. The system has to dynamically reconfigure itself and product objects need to start using the functionality provided by the new production cells automatically. Unless one prefers to explicitly program this behaviour, more expressive acquaintance handling constructs are required to deal with these types of behaviour.2.1.2 Electricity distribution automationFuture electricity distribution networks will be different in many respects, but one major difference will be the notion of two-way communication between electricity producer and electricity consumer. A second difference is that every electrical appliance will be extended with a microprocessor that controls the appliance. An average household will contain tens of these microprocessors and consequently at least a few hundred objects controlling the house and its appliances. In addition to the small microprocessors associated with electrical appliances, households will contain larger computers for more advanced, e.g. coordinating tasks. Although some objects in the system need to be kept at particular processors, most objects can and need to dynamically change location depending on external events and cir-cumstances. In addition, new appliances can be added dynamically and the objects in these appliances need to auto-matically select and bind their required acquaintance objects. An additional problem is that the electricity producer is responsible for certain objects in the system, e.g. the objects for buying electricity. Sometimes, these objects will need to be replaced with updated versions. As it is virtually impossible to bring the system down and reboot with a new ver-sion of the software, these changes have to be incorporated in run time. This requires that an object can be replaced by another object without disturbing the clients of the object.2.1.3 Mobile telecommunicationsIn the domain of telecommunications, especially mobile communication is a large and increasing market. The infra-structure required for mobile telephony, however, is more complex than for traditional telephony. This is primarily due to the dynamicity in the location of the phones. For example, a physical mobile phone is represented by a mobile phone object in the distributed information system of a mobile telephony operator. A mobile phone object has several acquaintances, among others a base station object, an operator object and a voice mail object. Since the owner of the physical phone moves around, the phone object also moves through the distributed information system. In some cases, the phone user travels outside the region covered by the operator, in which case another operator that covers the par-ticular region provides telephone services for the phone, under the condition that the two operators have such an agreement. Another example is the situation where the user of the phone is moving during a phone call. This may require that the phone changes base station, requiring the phone object to be moved from one base station object to a remote base station object. The consequence of these types of behaviour is that the mobile phone object needs to real-locate and to reselect and re-bind some of its acquaintances. For instance, the base station acquaintance needs to be rebound after every relocation and needs to be selected from the immediate context of the object. The operator object only needs to be rebound if the new base station object is owned by a different operator, whereas the voice mail object is bound permanently to the object.2.2 System OrganisationBased on the three example domains mentioned in the previous section, but also other examples not mentioned in this paper, one can abstract a number of properties of open, dynamic object-oriented systems. Important issues while dis-cussing such systems are the system concept itself, the organisation of objects and other entities and the communica-tion between objects.2.2.1 System ConceptThe notion of a system with closed boundaries and single instantiation time is no longer valid in the systems addressed in this paper. The ‘system’ is becoming more of a context or a space in which objects are created, changed and removed constantly. Since these systems are so flexible and dynamic, the different entities in the system cannot, up to the same degree, create permanent dependencies on each other. Since these systems generally are distributed, they arealso dynamic at the level of physical entities. For example, nodes in the system network can be added and removed dynamically. An illustrative, though extreme, example of the type of system we are referring to is Internet. Internet cannot be considered as a system in the traditional meaning of the word. No single person has complete overview over the system or has the right to reorganise the system. Similarly, in intranets, i.e. intra-organisational networks, gener-ally no single person has complete control over and understanding of the systemA consequence of the different system view can be found in the way applications are instantiated and configured in the system. In the traditional systems, applications have only a ‘vertical’ interface, in that these applications only com-municate with the underlying operating system and with the user interface on top of the application. The interface of the application to the entities it interact with is very rigid, since both the operating system and the user interface have well-defined, static interfaces on which one can rely without limiting the useability of the application. The few dynamic aspects of the application, e.g. as a consequence of different versions of the operating system or user inter-face, could be hand-coded into the application without too much overhead.The development in the open flexible object systems is that the application has, next to the vertical interface, also a horizontal interface that tends to be more important than its vertical counterpart. The horizontal interface consists of references to and communication with objects external to the application. These objects can be data or service provid-ers, but they can, in principle, represent any type of behaviour.The notion of an application, in these systems, is no longer an independent entity executing separated from everything else in the system. Whereas an application previously formed an impenetrable encapsulation boundary for the entities that it contained, its role in the new systems is limited to two aspects, i.e. to represent a unit of instantiation and, per-haps, removal and, secondly, an encapsulation boundary for security means. The first role is to instantiate a group of objects that combined form a relevant entity as a single action. These objects, however, will in general communicate with each other, but also with other objects outside the application. That leads to the second role of the application, i.e. to provide an encapsulation boundary. The encapsulation boundary can be used to restrict access to certain objects contained in the application, or to restrict access by certain clients. However, communication between external objects and internal objects is necessary requirement, so the encapsulation cannot restrict all access by external objects.2.2.2 Hierarchical object organisationIf one takes the system perspective in the previous section, it becomes clear that the objects in that system need to be organised in a structured manner in order to be able to deal with the large number of objects. The traditional ‘sea of objects’ approach where all objects in the system are visible to each other and exist at the same level is infeasible. Although this seems an obvious requirement from a design perspective, fact is that, although software engineers make use of subsystems, very often the implementation is performed in a sea of objects approach. On the other hand, for inter-application communication, the traditional approach is to take a hand-coded interfacing approach, causing the application to treat objects external to it very different from objects contained inside the application.The lack of uniformity in treating internal and external objects is obviously problematic for several reasons. One rea-son is that an object that previously used an internal object and needs to change to using an external object requires considerable adaptation of the code. A second reason is that the interface generally only provides access to those fea-tures required at application development time. The intra-application object communication, as described, is often based on a ‘sea of objects’ approach. The disadvantage of this approach is, at least, twofold, i.e. complexity and access control. An average application can easily contain hundreds of objects, leading to problems of complexity when searching, replacing and accessing objects. Secondly, since each object is directly accessible by all other objects, the object might be called by clients that should not have access to that object. A solution would, of course, be to program access control checks into each object, but that leads to considerable implementation and execution overhead and reduces the reusability of the resulting object dramatically.To address the aforementioned problems, a unified object organisation is required that deals with the problems of communication with external as well as internal objects. The approach that is used in this paper is to organise objects in an hierarchical manner. Each object is located inside another object, that, in turn, together with other objects is encapsulated by a higher-level object. This process can continue for some levels before a top level is reached at which the complete system is represented as a top-level object. Although this organisation may seem very hierarchical, most systems fit the description. For example, an distributed object-oriented system contains at least two sites at which objects can execute. In virtually all situations, the objects in the system will distinguish between objects located at the same site and objects on a different site. This consequently leads to a two level hierarchical organisation. However, it is important to note that the hierarchical object organisation should be based on the logical structure of the system andnot on its physical organisation. Higher level composed objects in general represent entities like subsystems and not the set of objects located at a particular workstation. Nevertheless, there is, in certain cases, a relation between an object and a physical entity, but this issue is not discussed here.From the aforementioned perspective, the complete system can be viewed as a single, large object. This object con-sists of nested objects, that recursively may be composed of nested objects. This system organisation provides more structure, when compared to the traditional object-oriented model in which nesting is not explicitly offered as a sys-tem organisation property. In these systems, all relevant objects generally are in the same name space, leading to a potentially very large number of objects that can ‘see’ each other.However, modelling the parts of the system as normal objects leads to problems with accessing objects that are in dif-ferent subsystems. If the subsystem is represented as a traditional object, all objects contained in the subsystem are encapsulated and hidden for objects in other subsystems. Although this property is very useful in object-oriented pro-gramming, it is not as appropriate in large objects for representing subsystems. If the functionality of objects in the subsystem needs to be accessible by objects in different subsystems, the subsystem, in the traditional object model, would need to put methods on its interface that forward requests by external objects to the appropriate object in the subsystem. In case of large or deeply nested subsystems, this behaviour is rather tedious since potentially many meth-ods have to be implemented and, in case of nested subsystems, the same method may have to be defined several times, once for every encapsulating subsystem.A solution to this problem would be some notion of flexible encapsulation, but we are investigating this issue. The concept builds on the ability of objects to make nested objects visible to external clients. Based on this, a subsystem can make a subset of the encapsulated objects visible and encapsulate all other objects. The flexible encapsulation concept solves the aforementioned problems related to encapsulation by subsystems.2.2.3 Object group communicationObject communication in general considers a sender of a message and a known receiver of the message. However, in several situations a message involves operations at several objects. Examples of this type of behaviour are multi-casts and update messages in model-view-controller structures. In both cases, a message, e.g. notifying a state change, will be send to multiple objects, which are selected based on some criterion.Object group communication requires some form of object set that is used for selection of objects for, e.g. multi-casts. The hierarchical object structure, discussed in the previous section, provides object sets, since each object is encapsu-lated in some composition object representing, e.g. a subsystem, and the object encapsulated in the composition object can be used as the selection set. An additional aspect of the composition objects is that the composition object itself is encapsulated in yet another encapsulation object. This leads to nested contexts and an object that intends to perform a multi-cast or another form of object group communication can decide whether only the immediate context of the object is used, the complete system or some level in between, i.e. the n encapsulating contexts. Such selections can be based on any property, including the object type, and are rather similar to queries in object-oriented databases. Although object group communication is not supported in the traditional object-oriented model, it has been studied by several authors. Most authors are concerned with representing complex communication, or interaction, patterns between a group of objects where each object plays some roles in the pattern, e.g. [Helm et al. 90] [Aksit et al. 93] and [Pintado 95]. All these approaches are concerned with representing an interaction pattern between a group of objects as a separate entity that interacts with the involved objects. This is different from what we are concerned with in this paper; it is our aim to represent the acquaintance selection and binding process of an individual object in an expressive and reusable manner, even though this communication may be towards a group of objects.3 Problems in Object CommunicationThe traditional object-oriented model only supports communication between a sender object and a known receiver object, i.e. the sender has to know the identity of the receiver object before sending a message to it. As described in the introduction, additional mechanisms for communication are required in large systems containing many objects. The communication mechanisms required for object communication can be categorised into two categories, i.e. communi-cation with single objects and group based object communication.。
On the notion of concept I
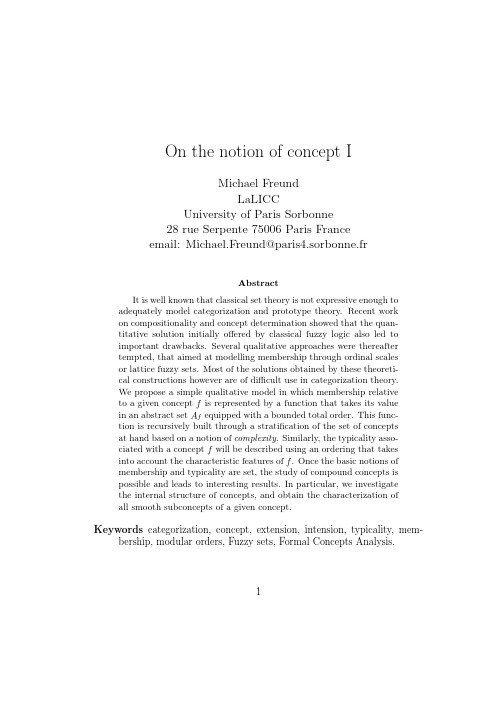
On the notion of concept IMichael FreundLaLICCUniversity of Paris Sorbonne28rue Serpente75006Paris Franceemail:Michael.Freund@paris4.sorbonne.frAbstractIt is well known that classical set theory is not expressive enough to adequately model categorization and prototype theory.Recent workon compositionality and concept determination showed that the quan-titative solution initially offered by classical fuzzy logic also led toimportant drawbacks.Several qualitative approaches were thereaftertempted,that aimed at modelling membership through ordinal scalesor lattice fuzzy sets.Most of the solutions obtained by these theoreti-cal constructions however are of difficult use in categorization theory.We propose a simple qualitative model in which membership relativeto a given concept f is represented by a function that takes its valuein an abstract set A f equipped with a bounded total order.This func-tion is recursively built through a stratification of the set of conceptsat hand based on a notion of complexity.Similarly,the typicality asso-ciated with a concept f will be described using an ordering that takesinto account the characteristic features of f.Once the basic notions ofmembership and typicality are set,the study of compound concepts ispossible and leads to interesting results.In particular,we investigatethe internal structure of concepts,and obtain the characterization ofall smooth subconcepts of a given concept.Keywords categorization,concept,extension,intension,typicality,mem-bership,modular orders,Fuzzy sets,Formal Concepts Analysis.11IntroductionIn this paper we propose a new framework for the study of some basic notions classically used in categorization theory.In particular,we shall be concerned with the problem offinding a suitable theoretical apparatus to model the notions of membership and typicality that underlie prototype theory.It is well recognized since the work of Eleanor Rosch(17)that membership,for instance,is not an all-or-not matter:the classical set-theoretical or the two-value logic model are of therefore of little use to render count of most of the cognition process.This drove Zadeh and his followers(22)and(23)to propose a representation of concepts by fuzzy sets,membership being mod-elled through a real function with values in the unit interval.Such a repre-sentation nevertheless lead to counterintuitive results:see for instance the seminal papers of Kamp and Partnee and of Osherson and Smith(11)(15) and(16)).At a quite elementary level,for instance,it was observed that the membership degree relative to a compound concept could never be greater than the degree induced by any of its components,a result that cannot be accepted for both theoretical and experimental reasons.Even for elemen-tary concepts,the representation of concepts as quantitative fuzzy sets poses problems:vague concepts like to-be-an-adult or to-lie are given continuous values in the unit interval,but what does it mean to qualify somebody as adult‘with degree.4837’?In particular,as observed by several authors (for instance(13))there is no reason why the same set-the unit interval-should serve as a uniform criterion,being invariably referred to as a measure of membership whatever the concept at hand.True,in practice membership is often evaluated through statistical data,and the membership degree iden-tified with a simple frequency.But the fact that,say,87individuals out of 100consider a car seat as a piece of furniture by no means involves that,in an agent mind,the membership degree of a car-seat relative to the concept to-be-a-piece-of-furniture is equal.87.These drawbacks led to various solutions which all aimed at replacing the primitive quantitative model by a qualitative one:thus,attention focussed on ordinal scales and on lattice fuzzy sets-see for instance(10)or(23).Fora brief analysis of the most recent work on this area,the reader may refer to(13)or(3).However,we consider that the solutions that were proposed are not fully adapted to model prototype theory,and that they cannot be easily exploited to address the classical questions raised by categorization theory.In a different area,Peter G¨a rdenfors(8)or(7)proposed a geometrical2model as a framework for concept theory:a concept is defined as a convex region of a multidimensional space,each dimension corresponding to a basic quality.Convexity is related with a notion of betweenness that is supposed to be meaningful for the relevant quality dimensions:if two objects are ex-emplars of a concept,such will be the case for any object that lies‘between’them.The typical instances of a concept are those which are located‘near the center’of the considered region.This Geometry of Thought,as the au-thor calls it,provides interesting tracks in the analysis of concepts.However, it is mostly based on quantitative notions,which wefind not best appropri-ate to model the cognition process.Furthermore,it does not seem that the distinction between vague and sharp concepts is fully taken into account.For these reasons,we propose to revisit the basic notions linked with cate-gorization theory and treat them from a qualitative point of view.Concerning membership,for instance,and rather than dealing with uniform gradation functions that take their values in the unit interval,we represent member-ship relative to a concept by a function whose set of values depends on the chosen concept.This set is endowed with a total bounded order that can be used to evaluate to which degree a object falls under this concept.We think indeed that such a representation is the most adequate to model notions like: object x plainly falls under the concept f,object x falls definitely not under the concept f or object x falls more than object y under the concept f.These notions,which are the basis of categorization theory,are also thefirsts one should deal with in order to understand the problems that arise with vague concepts:for instance,an agent may consider that an elevator is definitely less a vehicle than a chairlift,while being unable at the same time to attribute a precise numerical membership degree to any of these items.We propose in this paper an example of construction such an order,by making use of the set of defining features attached to the concepts at hand.Postulating the existence of such a set is part of most of the theories on categorization: see for instance(21),(20),(1),(4)or more recently(2),where a concept is assimilated with a set of properties which things that fall under the concept typically have or are believed to have.These defining features,from the point of view of the agent,help understanding the chosen concept;they are indi-vidually necessary and collectively sufficient to decide whether or not an item is an exemplar of this concept.Given a vague concept f,we shall use this associated defining feature set to compare the f-membership of two items in the following way:an object x will be considered as falling less under f than an object y if it falls less than y under the f-defining features.The3circularity of this definition will be avoided by attributing to each concept a complexity level:the sharp concepts,those for which membership is an all-or-not matter,will be given complexity level0;at level1,we shall rank all the vague concepts whose defining feature set only consists of sharp con-cepts;at level2,we will have the vague concepts whose defining feature set consists of concepts that have complexity level equal to0or1,and so on. This ranking will eventually render possible a recursive definition of mem-bership,and,consequently,the construction of a membership order among the set of objects at hand.Having represented concepts by means of order-functions poses the prob-lem offinding an adequate representation of the notion of typicality.Since the work of E.Rosch,a considerable amount of study has been carried out on this notion,and it is now widely accepted that,relative to a given con-cept,objects may be classified following their degree of typicality.Although a precise and general definition of this typicality degree is still missing,one generally agrees on the fact that such a degree has to faithfully reflect the number of characteristic features attached to the concept at hand,together with the relative pertinence,or the frequency,of these features((14),Chapter 2).Nevertheless the attempts at a rigorous construction are rare,and none of them seem to have gained general recognition.Besides,researchers in this domain restricted themselves to elementary cases,dealing with sharp con-cepts,for which membership is an all-or-not matter,or with concepts with sharp features.In particular,they did not seem to be concerned with situa-tions in which the typicality relative to a concept depends on the membership relative to another concept:in order to determine the relative typicality of a hen as a bird,for instance,they would not consider that it is necessary to first evaluate its membership degree relative to the concept to-fly.We think on the contrary that typicality must be determined through membership, and that these two notions are correlatedWe therefore propose the construction of a typicality order,clear and easy to evaluate,that faithfully conforms with our intuition.This order is meant to reflect a particular agent’s judgment at a precise time.It is based on the agent’s choice,for each concept,of a an associated characteristic feature set,partially ordered through a salience relation that is meant to evaluate the relative importance of these features.The typicality of two items will be compared by investigating the characteristic features that apply to them,the way they apply to them,and their relative salience.Once we have completed the construction of the typicality order,it will be possible to define the typical4instances of a concept as those that have maximal order,that is those that fall under all the characteristic features of this concept.This definition of typicality will then enable us to define the intension of a concept as the set of features that apply to all typical instances of the concept.Thus,the intension of a concept may be interpreted as the set of characteristic features that agents belonging to a well-defined cultural environment would generally agree to associate with this concept:it enlarges the more subjective notion of characteristic features sets.A coherent theory of typicality must be able to correctly address the problem of compound concepts.We shall show that our formalism pro-vides natural and intuitive answers concerning composed concepts,provided one departs from the idea that the logic of concepts boils down to a simple propositional calculus.Indeed we do not agree with the commonly admit-ted postulate following which the negation of a concept,the conjunction or the disjunction of two concepts should be again a concept:we do not consider that not-to-be-an-apple or(to-be-an-apple)&(to-be-a-pear)are con-cepts.Consequently,we believe that the treatment of such sentences,which clearly goes beyond the limits of the elementary concept theory we are deal-ing with,should be addressed only after a coherent logical framework for categorization has been proposed.In the present work,we shall therefore content ourselves with a language that only admits a single partial operator, the determination connective,which is meant to represent the determination of a principal concept by a secondary one:for instance,the concept to-be-a-green-apple is the determination of the principal concept to-be-an-apple by the secondary one,to-be-green.Concept determination is not compositional, except in some limit cases:this means that neither the membership,nor the typicality relative to a composed concept can be directly evaluated through a computation of the corresponding magnitudes of its components.However, it remains possible to determine the typical order,hence the typical instances of a composed concept,via the typicality orders induced by its components. This result is important as it can be considered as an answer to the compo-sitionality problem.Plan of this paperAfter introducing in section2the framework we are going to work in and recall the distinction between sharp and vague concepts,we shall introduce in section3the membership orders and functions associated with elemen-5tary concepts.In section4,we shall present the determination connective and extend the membership order to compound concepts.We shall then turn to typicality,and build in section5the typicality order associated with elementary and compound concepts.In section6,we show how the notion of smooth subconcepts can be formalized through the determination con-nective,and we propose an interpretation of our results in the language of Formal Concept Analysis.Section7is a conclusion in which we discuss our future work.2Concepts and objectsWe denote by O the universe of discourse,which we may see as the set of all objects,real orfictive,that an agent has at his disposal.Together with this set,we suppose given a set F of concepts.These concepts constitute the elementary items on which the agent builds its reasoning process,and they reflect its knowledge on the world at a given time.A concept applies to an object if it describes a property that this object possesses,or if it is an attribute of this object.For instance,the concept to-be-a-fruit applies to the object an-apple.We will say indifferently that the concept f applies to the object x,that x falls under f,or that x is an instance of f.In the classical theory,where categories were modelled through set theory,membership rel-ative to a concept was an all-or-none matter:an object could not partially fall under a concept.This perspective was also that of Frege(5),for whom concepts were defined as one-place predicates having a bivalent membership truth function.With prototype theory and the evidence that there existed vague concepts(eg.to-be-a-lie,to-be-an-adult,to-be-employed,to-be-a-sand-heap etc),it became clear that this primitive notion of concepts had to be enlarged and that membership was a question of degree,rather than an all-or-none matter.As observed in(11),“We all have strong intuitions that the concepts encoded by many natural-language predicates are vague;whether something is a chair,or is red,does not seem to be an all-or-none matter but a matter of degree;there may be some clear positive cases and some clear negative cases,but there are many unclear cases in between.”Sharp concepts are defined as those for which membership is an all-or-not matter:an object simply falls or does not fall under such a concept,with-out the possibility of taking intermediate values.To-be-a-human-being,to-be-a-tooth-brush,to-be-an-even-integer may provide examples of sharp con-6cepts.This definition has nevertheless to be understood as tightly related to a given agent’s point of view,and we shall always consider that we work from a particular subjective perspective,and at a particular time:the same concept may appear as sharp to a non-expert agent while being considered as vague for an expert.For vague concepts,membership is indeed not an all-or-not-matter:such are for instance the concepts to-be-a-lie,to-be-poor, to-be-employed,to-be-a-weapon-of-mass-destruction or to-be-a-mammal.In-deed,politeness sometimes drives us to make compliments that,although not sincere,cannot be considered as real lies;to be poor or to be employed is clearly a matter of degree;a gun is more a WMD than a knife;and the platypus is and is not a mammal.Of course,opinions may differ whether a given concept should be considered as a sharp or a vague one,but,and this is the important point,it is well recognized that both kinds of concepts exist. An interesting suggestion of(1)is that,for noun concepts,the opposition be-tween nominal and non-nominal categories reflects the duality between vague and sharp concepts:nominal categories can be defined through their defining features,and may therefore give rise to vague concepts,while non-nominal cannot.Non-nominal categories may be themselves divided between natural kind categories(eg:the category of tigers or of games)and artifact cate-gories(eg:the category of hammers,walls,cars).Note that the distinction between nominal and natural kind concepts is far from being evident:a same concept may be considered as nominal for an expert,and as non-nominal for a non-expert agent.For instance,the concept to-be-a-bird is undoubtedly of a natural kind for a child,but it may turn later to a nominal one once the child has learnt that all and only those animals that have beak and feathers are to be considered as birds.In deciding whether the concept to-be-a-bird is or not a sharp concept,we have therefore tofirst analyze which of these two concepts we are referring to:an agent aware that birdhood may be defined through the sum of a certain number of conditions,will consider to-be-a-bird a vague concept:the octopus,for instance will be more a bird than the bat, since the octopus has a beak.On the other hand,for a child,to-be-a-bird is bond to be a sharp concept,and the penguin will simply not be a member of the category,while the bat will.In the present work,we shall leave the problem how to determine which concepts are vague and which are not.We shall only be concerned with the problem offinding an adequate model that correctly describes how the notion of membership is used in a given agent’s behavior.73Membership for elementary conceptsIn the original fuzzy logic model,a membership degree function is attributedto each concept,measuring how accurately this concept applies to the objectsat hand.This degree however is not explicitly present in an agent’s mind:this is so for example for young children,for whom notions like real numbersor unit interval are totally meaningless.Nevertheless,given a concept,the agent will be generally able to decide whether two objects have the same ordifferent membership degrees,and which one,in the latter case,has higher degree:for instance,the agent may decide that the concept to-be-a-piece-of-furniture applies more to a car-seat than to a blackboard,without being ableat the same time to attribute a numerical membership degree to any of these items.In other words,the agent associates with each concept f an implicit notion of a membership order.It is this order we now want to build.We shallfirst deal with elementary concepts,leaving the case of com-pound concepts in the next section.In order to correctly define a suitable notion of membership for vague concepts,we start from the widely accepted theory following which each such concept f is given together with afinite auxiliary set∆f which,from the point of view of the agent,includes allthe features that explain or illustrate f,helping differentiating it from its neighboring concepts.For instance,for the concept to-be-a-bird,the corre-sponding∆f may consist of the concepts to-be-a-vertebrate,to-have-a-beakand to-have-feathers;for the concept to-be-a-tent,it may list the featuresto-be-a-shelter,to-be-made-of-cloth.We interpret∆f as the set of defining features an agent or a group of agents would associate with f.The sets∆fmay be seen as the outputs a dictionary or an encyclopedia would return when given vague concepts as inputs.The elements of∆f are supposed tobe less complex than the root concept f:in the agent’s mind,they constitutean help for the understanding of f.This notion of complexity will be now given a precise meaning by attributing a complexity level c(f)to the set Fof concepts at hand in the following way:•Sharp concepts are given complexity level0.•If∆f consists of sharp concepts,set c(f)=1.•If c(g)has been defined for all concepts g of∆f,set c(f)=1+Max(c(g))g∈∆f We shall make the assumption that this procedure attributes a well-defined complexity level to every element of F.In other words,our theory8only applies to a set F that consists of concepts that either are sharp,or can be recursively defined through sharp concepts.As a matter of fact,most of the elementary concepts one usually deals with have a small complexity level,and we could have made the assumption that the set of concepts at hand solely consists of concepts f of level less than3.However wefind it more convenient to work in a more general framework,as the results are not more difficult to establish.It may be the case that some elements of∆f are more important than others,when considered as a help for defining or illustrating f:for instance, given the concept to-be-a-bird,an agent may think that the feature to-have-wings is more salient than the feature to-be-an-animal,while both features may be part of the same set∆f.Thus,it is necessary to endow each set∆f with a(possibly empty)salience relation that reflects the relative importance of its elements as defining features of f.In its most general form,such a relation will be represented by a strict partial order>f.This order has to be taken into account when comparing the f-membership of two items: an object x that falls under the most salient defining features of f will be considered a better instance of f than an object y that only falls under some non-salient defining feature of f.We can now proceed to the construction of the membership preorder rela-tion µf ,which will be defined on the set of objects O,and to the constructionof the membership functionϕf,which will take its values in a totally ordered set(A f,<f).We shall omit the subscripts when there is no ambiguity.We begin with the simplest case of sharp concepts:Definition1For every elementary sharp concept f,A f is the set{0,1}, andϕf the function:ϕf(x)=1if x falls under f andϕf(x)=0otherwise. The associated membership preorder is defined by x µfy ifϕf(x)≤ϕf(y).The membership preorder and the membership function relative to an arbitrary elementary concept f will be now defined by induction on c(f). This will be done in two steps.3.1The elementary membership orderDefinition2Let f be an elementary concept,and suppose that the totally ordered sets(A g,<g)and the membership functionsϕg have been defined forall elementary concepts g such that c(g)<c(f).The relation µf is thendefined by:9x µf y if for any concept h of∆f such thatϕh(y)<hϕh(x),there exists a concept k of∆f,k>f h,such thatϕk(x)<kϕk(y).The relation µf thus compares the ways objects inherit the defining fea-tures of f and takes into account the relative salience of these features.We will say that a preorder of this type is induced by the(ordered)set∆f.In the particular case where the salience order on∆f is empty,the relation boilsdown to:x µf y if and only ifϕh(x)≤hϕh(y)for all h in∆f,that is if andonly if no defining feature of f applies more to x than to y.The hypothesis that,for k∈∆f,the membership functionsϕk take theirvalue in a totally ordered set guarantees the transitivity of the relation µf .More precisely we have the following result:Lemma1For any elementary concept f,the relation µf is a partial pre-order on O.Proof:We have to prove that µf is a reflexive and transitive relation.Reflexivity is immediate.For transitivity,suppose that x,y and z are threeobjects such that x µf y and y µfz.We want to show that x µfz.Supposing that there exists a concept h of∆f such thatϕh(z)<ϕh(x),we have to prove the existence of a concept k∈∆f,k more salient than h,such thatϕk(x)<ϕk(z).We make a proof by cases:•Supposefirst thatϕh(x)≤ϕh(y).Then we haveϕh(z)<ϕh(y),and there exists therefore a concept k of∆f,k>f h,such thatϕk(y)<ϕk(z).We can suppose that k is maximal in∆f for this property (∆f is afinite set).Ifϕk(x)≤ϕk(y),we getϕk(x)<ϕk(z)and we are done.Ifϕk(y)<ϕk(x),the hypotheses imply that there exists a concept g in∆f,g>f k such thatϕg(x)<ϕg(y).We cannot have ϕg(z)<ϕg(y),otherwise there would exist a concept l in∆f,l>f g, such thatϕl(y)<ϕl(z),which would contradict the maximality of k.We have thereforeϕg(y)≤ϕg(z)and it follows thatϕg(x)<ϕg(z)as desired.•Suppose now that we haveϕh(y)<ϕh(x).There exists k∈∆f,k>f h, such thatϕk(x)<ϕk(y).Again,we can suppose that k is maximal in∆f for these properties.Ifϕk(y)≤ϕk(z),we getϕk(x)<ϕk(z), as desired.If on the contrary we haveϕk(z)<ϕk(y),there exists a concept g in∆f,g>f k,such thatϕg(y)<ϕg(z).As before,the10maximality of k implies that we necessarily have ϕg (x )≤ϕg (y ).It follows that ϕg (x )<ϕg (z ),and the proof is complete.Let us denote by ≺µf the relation:x ≺µf y iffx µf y and not y µf x .Itfollows from the above lemma that ≺µf is a strict partial order on O .Example 1Let f be the concept to-be-a-bird ,and suppose that,from the point of view of an agent,its defining feature set is given by ∆f ={to-be-an-animal ,to-have-two legs ,to-lay-eggs ,to-have-a-beak ,to-have-wings },all of these concepts being considered as sharp concepts for the agent.Suppose also that the salience order is given by:to-lay-eggs >f to-have-two-legs ,to-have-a-beak >f to-lay-eggs and to-have wings >f to-lay-eggsLet r ,m ,t ,b and d respectively stand for a robin,a mouse,a tortoise,a bat and a dragonfly,and let us compare their relative birdhood.In order to determine the induced membership order,we first build the following array:animaltwo −legs lay −eggs beak wings robinmousetortoisebatdragonflyWe readily check that d ≺µf r ,m ≺µf t ,and m ≺µf b .Note that we haveb µf d ,since the concept to-have-two-legs under which the bat falls,contrary to the dragonfly,is dominated by the concept to-lay-eggs that applies to the dragonfly and not to the bat.On the other hand,we do not have d µf b ,as nothing compensates the fact that the dragonfly lays eggs and the bat does not.This yields b ≺µf d .We also remark that the tortoise and the bat are incomparable,that is,we have neither b µf t ,nor t µf b .The strict f -membership order induced on these five elements is thus given by the following Hasse diagramm:mb d t r &&11We have therefore m≺µf b≺µfd≺µfr and m≺µft≺µfr.We can now precisely translate the notion of membership:an object xwill be considered as falling under f if x is≺µf -maximal in O.We shalldenote by Ext f,the extension of f,the set of all such objects.We close this paragraph with a technical lemma:Lemma2The double inequality x µf y and y µfx holds if and only ifϕh(x)=ϕh(y)for all concepts h of∆f.Proof:Ifϕh(x)=ϕh(y)for all concepts h of∆f,we have clearly x µf y andy µf x.Conversely,suppose that x µfy and y µfx.If we had notϕh(x)=ϕh(y)∀h∈∆f,there would exist a concept h of∆f such thatϕh(x)=ϕh(y), and we could choose h with maximal salience for this property.We wouldhave for instanceϕh(x)<hϕh(y).But since y µf x,there would existk∈∆f,k more salient than h,such thatϕk(y)<kϕk(x),thus contradicting the choice of h.3.2The membership functionIt is clear that the ordering given by the relation µf is not connected:giventwo objects x and y,it may well happen that neither x µf y,nor y µfx.It is nevertheless possible,starting from the strict partial order≺µf ,to build,a membership functionϕf that fairly translates the notion of a degree off-membership.This function will satisfyϕf(x)<ϕf(y)whenever x≺µf y:in a sense,this is the best one can hope(see(12)and her discussion on the impossibility for order relations to correctly represent vagueness).For this purpose,we shall proceed in a way that parallels,though in different context, a construction we proposed in(?).Given an object x,we say that x initializes a membership chain of length n if it is possible tofind n objects x1,x2,...,x n with last term x n∈Ext f,such that x≺µf x1≺µfx2≺f...≺µfx n.For instance,any element x∈Ext finitializes a chain of length0,and any object that does not fall under f initializes an membership-chain of strictly positive length l≤|∆f|.In a sense,the length of such a chain measures how distant x is from the set Ext f.Note that,given an object x,the existence and the length of such a chain is determined by the concepts and the objects the agent has at his disposal.Each link of a chain corresponds for this agent to a real(or afictive) given object,together with some given concepts of the universe at hand.12。
数据挖掘导论第4课数据分类和预测
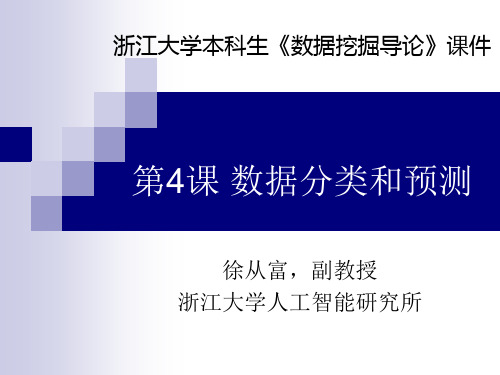
II.
Issues Regarding Classification and Prediction (1): Data Preparation
Data cleaning Preprocess data in order to reduce noise and handle missing values Relevance analysis (feature selection) Remove the irrelevant or redundant attributes Data transformation Generalize and/or normalize data
I.
Classification vs. Prediction
Classification predicts categorical class labels (discrete or nominal) classifies data (constructs a model) based on the training set and the values (class labels) in a classifying attribute and uses it in classifying new data Prediction models continuous-valued functions, i.e., predicts unknown or missing values Typical applications Credit approval Target marketing Medical diagnosis Fraud detection
Issues regarding classification and prediction (2): Evaluating classification methods
高三英语学术研究方法单选题30题

高三英语学术研究方法单选题30题1. In a literature review, which of the following is the most important step?A. Collecting a large number of sourcesB. Selecting relevant and reliable sourcesC. Reading the sources quicklyD. Copying the content of the sources directly答案:B。
本题考查文献综述中最重要的步骤。
选项A 收集大量来源固然重要,但质量更关键;选项C 快速阅读来源可能会忽略重要信息;选项 D 直接复制来源内容是学术不端行为。
选项 B 选择相关可靠的来源是确保文献综述质量的关键步骤。
2. When conducting a literature review, how should you handle contradictory information from different sources?A. Ignore it and focus on the consistent informationB. Choose the information that supports your hypothesisC. Analyze and try to reconcile the differencesD. Just randomly pick one of the pieces of information答案:C。
在进行文献综述时,面对不同来源的矛盾信息,选项A 忽略它只关注一致信息可能会导致研究不全面;选项B 只选择支持假设的信息会使研究有偏差;选项 D 随机挑选信息是不科学的。
选项C 分析并尝试调和差异是正确的处理方式。
3. What is the purpose of citing sources in a literature review?A. To show off your knowledgeB. To increase the word count of your reviewC. To give credit to the original authors and support your argumentsD. To make the review look more complicated答案:C。
ifrs12国际财务报告准则12号
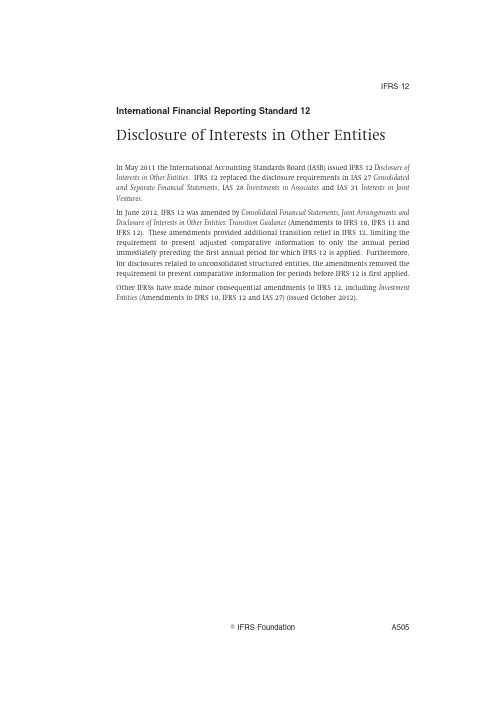
IFRS12 International Financial Reporting Standard12Disclosure of Interests in Other EntitiesIn May2011the International Accounting Standards Board(IASB)issued IFRS12Disclosure of Interests in Other Entities.IFRS12replaced the disclosure requirements in IAS27Consolidated and Separate Financial Statements,IAS28Investments in Associates and IAS31Interests in Joint Ventures.In June2012,IFRS12was amended by Consolidated Financial Statements,Joint Arrangements and Disclosure of Interests in Other Entities:Transition Guidance(Amendments to IFRS10,IFRS11and IFRS12).These amendments provided additional transition relief in IFRS12,limiting the requirement to present adjusted comparative information to only the annual period immediately preceding the first annual period for which IFRS12is applied.Furthermore, for disclosures related to unconsolidated structured entities,the amendments removed the requirement to present comparative information for periods before IFRS12is first applied.Other IFRSs have made minor consequential amendments to IFRS12,including Investment Entities(Amendments to IFRS10,IFRS12and IAS27)(issued October2012).IFRS Foundation A505IFRS12C ONTENTSfrom paragraph INTRODUCTION IN1 INTERNATIONAL FINANCIAL REPORTING STANDARD12 DISCLOSURE OF INTERESTS IN OTHER ENTITIESOBJECTIVE1 Meeting the objective2 SCOPE5 SIGNIFICANT JUDGEMENTS AND ASSUMPTIONS7 Investment entity status9A INTERESTS IN SUBSIDIARIES10 The interest that non-controlling interests have in the group’s activities andcash flows12 The nature and extent of significant restrictions13 Nature of the risks associated with an entity’s interests in consolidatedstructured entities14 Consequences of changes in a parent’s ownership interest in a subsidiarythat do not result in a loss of control18 Consequences of losing control of a subsidiary during the reporting period19 INTERESTS IN UNCONSOLIDATED SUBSIDIARIES(INVESTMENT ENTITIES)19A INTERESTS IN JOINT ARRANGEMENTS AND ASSOCIATES20 Nature,extent and financial effects of an entity’s interests in jointarrangements and associates21 Risks associated with an entity’s interests in joint ventures and associates23 INTERESTS IN UNCONSOLIDATED STRUCTURED ENTITIES24 Nature of interests26 Nature of risks29 APPENDICESA Defined termsB Application guidanceC Effective date and transitionD Amendments to other IFRSsFOR THE ACCOMPANYING DOCUMENTS LISTED BELOW,SEE PART B OF THIS EDITIONAPPROVAL BY THE BOARD OF IFRS12ISSUED IN MAY2011APPROVAL BY THE BOARD OF CONSOLIDATED FINANCIAL STATEMENTS,JOINT ARRANGEMENTS AND DISCLOSURE OF INTERESTS IN OTHERENTITIES:TRANSITION GUIDANCE(AMENDMENTS TO IFRS10,IFRS11ANDIFRS12)ISSUED IN JUNE2012APPROVAL BY THE BOARD OF INVESTMENT ENTITIES(AMENDMENTS TOIFRS10,IFRS12AND IAS27)ISSUED IN OCTOBER2012A506IFRS FoundationIFRS12 BASIS FOR CONCLUSIONSIFRS Foundation A507IFRS12International Financial Reporting Standard12Disclosure of Interests in Other Entities (IFRS12)is set out in paragraphs1–31and Appendices A–D.All the paragraphs have equal authority.Paragraphs in bold type state the main principles.Terms defined in Appendix A are in italics the first time they appear in the IFRS.Definitions of other terms are given in the Glossary for International Financial Reporting Standards.IFRS12 should be read in the context of its objective and the Basis for Conclusions,the Preface to International Financial Reporting Standards and the Conceptual Framework for Financial Reporting.IAS8Accounting Policies,Changes in Accounting Estimates and Errors provides a basis for selecting and applying accounting policies in the absence of explicit guidance.A508IFRS FoundationIFRS12 IntroductionIN1IFRS12Disclosure of Interests in Other Entities applies to entities that have an interest in a subsidiary,a joint arrangement,an associate or an unconsolidatedstructured entity.IN2The IFRS is effective for annual periods beginning on or after1January2013.Earlier application is permitted.Reasons for issuing the IFRSIN3Users of financial statements have consistently requested improvements to the disclosure of a reporting entity’s interests in other entities to help identify theprofit or loss and cash flows available to the reporting entity and determine thevalue of a current or future investment in the reporting entity.IN4They highlighted the need for better information about the subsidiaries that are consolidated,as well as an entity’s interests in joint arrangements and associatesthat are not consolidated but with which the entity has a special relationship.IN5The global financial crisis that started in2007also highlighted a lack of transparency about the risks to which a reporting entity was exposed from itsinvolvement with structured entities,including those that it had sponsored.IN6In response to input received from users and others,including the G20leaders and the Financial Stability Board,the Board decided to address in IFRS12theneed for improved disclosure of a reporting entity’s interests in other entitieswhen the reporting entity has a special relationship with those other entities.IN7The Board identified an opportunity to integrate and make consistent the disclosure requirements for subsidiaries,joint arrangements,associates andunconsolidated structured entities and present those requirements in a singleIFRS.The Board observed that the disclosure requirements of IAS27Consolidatedand Separate Financial Statements,IAS28Investments in Associates and IAS31Interestsin Joint Ventures overlapped in many areas.In addition,many commented thatthe disclosure requirements for interests in unconsolidated structured entitiesshould not be located in a consolidation standard.Therefore,the Boardconcluded that a combined disclosure standard for interests in other entitieswould make it easier to understand and apply the disclosure requirements forsubsidiaries,joint ventures,associates and unconsolidated structured entities. Main features of the IFRSIN8The IFRS requires an entity to disclose information that enables users of financial statements to evaluate:(a)the nature of,and risks associated with,its interests in other entities;and(b)the effects of those interests on its financial position,financialperformance and cash flows.IFRS Foundation A509IFRS12General requirementsIN9The IFRS establishes disclosure objectives according to which an entity discloses information that enables users of its financial statements(a)to understand:(i)the significant judgements and assumptions(and changes tothose judgements and assumptions)made in determining thenature of its interest in another entity or arrangement(iecontrol,joint control or significant influence),and indetermining the type of joint arrangement in which it has aninterest;and(ii)the interest that non-controlling interests have in the group’sactivities and cash flows;and(b)to evaluate:(i)the nature and extent of significant restrictions on its ability toaccess or use assets,and settle liabilities,of the group;(ii)the nature of,and changes in,the risks associated with itsinterests in consolidated structured entities;(iii)the nature and extent of its interests in unconsolidatedstructured entities,and the nature of,and changes in,the risksassociated with those interests;(iv)the nature,extent and financial effects of its interests in jointarrangements and associates,and the nature of the risksassociated with those interests;(v)the consequences of changes in a parent’s ownership interest in asubsidiary that do not result in a loss of control;and(vi)the consequences of losing control of a subsidiary during thereporting period.IN10The IFRS specifies minimum disclosures that an entity must provide.If the minimum disclosures required by the IFRS are not sufficient to meet thedisclosure objective,an entity discloses whatever additional information isnecessary to meet that objective.IN11The IFRS requires an entity to consider the level of detail necessary to satisfy the disclosure objective and how much emphasis to place on each of therequirements in the IFRS.An entity shall aggregate or disaggregate disclosuresso that useful information is not obscured by either the inclusion of a largeamount of insignificant detail or the aggregation of items that have differentcharacteristics.IN12Investment Entities(Amendments to IFRS10,IFRS12and IAS27),issued in October 2012,introduced an exception to the principle in IFRS10Consolidated FinancialStatements that all subsidiaries shall be consolidated.The amendments define aninvestment entity and require a parent that is an investment entity to measureits investment in particular subsidiaries at fair value through profit or loss inaccordance with IFRS9Financial Instruments(or IAS39Financial Instruments: A510IFRS FoundationIFRS12Recognition and Measurement,if IFRS9has not yet been adopted)instead of consolidating those subsidiaries in its consolidated and separate financial statements.Consequently,the amendments also introduced new disclosure requirements for investment entities in this IFRS and IAS27Separate Financial Statements.IFRS Foundation A511IFRS12International Financial Reporting Standard12Disclosure of Interests in Other EntitiesObjective1The objective of this IFRS is to require an entity to disclose information that enables users of its financial statements to evaluate:(a)the nature of,and risks associated with,its interests in otherentities;and(b)the effects of those interests on its financial position,financialperformance and cash flows.Meeting the objective2To meet the objective in paragraph1,an entity shall disclose:(a)the significant judgements and assumptions it has made in determining:(i)the nature of its interest in another entity or arrangement;(ii)the type of joint arrangement in which it has an interest(paragraphs7–9);(iii)that it meets the definition of an investment entity,if applicable(paragraph9A);and(b)information about its interests in:(i)subsidiaries(paragraphs10–19);(ii)joint arrangements and associates(paragraphs20–23);and(iii)structured entities that are not controlled by the entity(unconsolidated structured entities)(paragraphs24–31).3If the disclosures required by this IFRS,together with disclosures required by other IFRSs,do not meet the objective in paragraph1,an entity shall disclosewhatever additional information is necessary to meet that objective.4An entity shall consider the level of detail necessary to satisfy the disclosure objective and how much emphasis to place on each of the requirements in thisIFRS.It shall aggregate or disaggregate disclosures so that useful information isnot obscured by either the inclusion of a large amount of insignificant detail orthe aggregation of items that have different characteristics(see paragraphsB2–B6).Scope5This IFRS shall be applied by an entity that has an interest in any of the following:(a)subsidiaries(b)joint arrangements(ie joint operations or joint ventures)A512IFRS FoundationIFRS12(c)associates(d)unconsolidated structured entities.6This IFRS does not apply to:(a)post-employment benefit plans or other long-term employee benefitplans to which IAS19Employee Benefits applies.(b)an entity’s separate financial statements to which IAS27SeparateFinancial Statements applies.However,if an entity has interests inunconsolidated structured entities and prepares separate financialstatements as its only financial statements,it shall apply therequirements in paragraphs24–31when preparing those separatefinancial statements.(c)an interest held by an entity that participates in,but does not have jointcontrol of,a joint arrangement unless that interest results in significantinfluence over the arrangement or is an interest in a structured entity.(d)an interest in another entity that is accounted for in accordance withIFRS9Financial Instruments.However,an entity shall apply this IFRS:(i)when that interest is an interest in an associate or a joint venturethat,in accordance with IAS28Investments in Associates and JointVentures,is measured at fair value through profit or loss;or(ii)when that interest is an interest in an unconsolidated structuredentity.Significant judgements and assumptions7An entity shall disclose information about significant judgements and assumptions it has made(and changes to those judgements andassumptions)in determining:(a)that it has control of another entity,ie an investee as described inparagraphs5and6of IFRS10Consolidated Financial Statements;(b)that it has joint control of an arrangement or significant influenceover another entity;and(c)the type of joint arrangement(ie joint operation or joint venture)when the arrangement has been structured through a separate vehicle.8The significant judgements and assumptions disclosed in accordance with paragraph7include those made by the entity when changes in facts andcircumstances are such that the conclusion about whether it has control,jointcontrol or significant influence changes during the reporting period.9To comply with paragraph7,an entity shall disclose,for example,significant judgements and assumptions made in determining that:(a)it does not control another entity even though it holds more than half ofthe voting rights of the other entity.(b)it controls another entity even though it holds less than half of thevoting rights of the other entity.IFRS Foundation A513IFRS12(c)it is an agent or a principal(see paragraphs B58–B72of IFRS10).(d)it does not have significant influence even though it holds20per cent ormore of the voting rights of another entity.(e)it has significant influence even though it holds less than20per cent ofthe voting rights of another entity.Investment entity status9A When a parent determines that it is an investment entity in accordance with paragraph27of IFRS10,the investment entity shall discloseinformation about significant judgements and assumptions it has made indetermining that it is an investment entity.If the investment entity does nothave one or more of the typical characteristics of an investment entity(seeparagraph28of IFRS10),it shall disclose its reasons for concluding that it isnevertheless an investment entity.9B When an entity becomes,or ceases to be,an investment entity,it shall disclose the change of investment entity status and the reasons for the change.Inaddition,an entity that becomes an investment entity shall disclose the effect ofthe change of status on the financial statements for the period presented,including:(a)the total fair value,as of the date of change of status,of the subsidiariesthat cease to be consolidated;(b)the total gain or loss,if any,calculated in accordance withparagraph B101of IFRS10;and(c)the line item(s)in profit or loss in which the gain or loss is recognised(ifnot presented separately).Interests in subsidiaries10An entity shall disclose information that enables users of its consolidated financial statements(a)to understand:(i)the composition of the group;and(ii)the interest that non-controlling interests have in thegroup’s activities and cash flows(paragraph12);and(b)to evaluate:(i)the nature and extent of significant restrictions on itsability to access or use assets,and settle liabilities,of thegroup(paragraph13);(ii)the nature of,and changes in,the risks associated with itsinterests in consolidated structured entities(paragraphs14–17);(iii)the consequences of changes in its ownership interest in asubsidiary that do not result in a loss of control(paragraph18);andA514IFRS FoundationIFRS12(iv)the consequences of losing control of a subsidiary duringthe reporting period(paragraph19).11When the financial statements of a subsidiary used in the preparation of consolidated financial statements are as of a date or for a period that is differentfrom that of the consolidated financial statements(see paragraphs B92and B93of IFRS10),an entity shall disclose:(a)the date of the end of the reporting period of the financial statements ofthat subsidiary;and(b)the reason for using a different date or period.The interest that non-controlling interests have in thegroup’s activities and cash flows12An entity shall disclose for each of its subsidiaries that have non-controlling interests that are material to the reporting entity:(a)the name of the subsidiary.(b)the principal place of business(and country of incorporation if differentfrom the principal place of business)of the subsidiary.(c)the proportion of ownership interests held by non-controlling interests.(d)the proportion of voting rights held by non-controlling interests,ifdifferent from the proportion of ownership interests held.(e)the profit or loss allocated to non-controlling interests of the subsidiaryduring the reporting period.(f)accumulated non-controlling interests of the subsidiary at the end of thereporting period.(g)summarised financial information about the subsidiary(seeparagraph B10).The nature and extent of significant restrictions13An entity shall disclose:(a)significant restrictions(eg statutory,contractual and regulatoryrestrictions)on its ability to access or use the assets and settle theliabilities of the group,such as:(i)those that restrict the ability of a parent or its subsidiaries totransfer cash or other assets to(or from)other entities within thegroup.(ii)guarantees or other requirements that may restrict dividendsand other capital distributions being paid,or loans and advancesbeing made or repaid,to(or from)other entities within thegroup.(b)the nature and extent to which protective rights of non-controllinginterests can significantly restrict the entity’s ability to access or use theassets and settle the liabilities of the group(such as when a parent isobliged to settle liabilities of a subsidiary before settling its ownIFRS Foundation A515IFRS12liabilities,or approval of non-controlling interests is required either toaccess the assets or to settle the liabilities of a subsidiary).(c)the carrying amounts in the consolidated financial statements of theassets and liabilities to which those restrictions apply.Nature of the risks associated with an entity’s interestsin consolidated structured entities14An entity shall disclose the terms of any contractual arrangements that could require the parent or its subsidiaries to provide financial support to aconsolidated structured entity,including events or circumstances that couldexpose the reporting entity to a loss(eg liquidity arrangements or credit ratingtriggers associated with obligations to purchase assets of the structured entity orprovide financial support).15If during the reporting period a parent or any of its subsidiaries has,without having a contractual obligation to do so,provided financial or other support to aconsolidated structured entity(eg purchasing assets of or instruments issued bythe structured entity),the entity shall disclose:(a)the type and amount of support provided,including situations in whichthe parent or its subsidiaries assisted the structured entity in obtainingfinancial support;and(b)the reasons for providing the support.16If during the reporting period a parent or any of its subsidiaries has,without having a contractual obligation to do so,provided financial or other support to apreviously unconsolidated structured entity and that provision of supportresulted in the entity controlling the structured entity,the entity shall disclosean explanation of the relevant factors in reaching that decision.17An entity shall disclose any current intentions to provide financial or other support to a consolidated structured entity,including intentions to assist thestructured entity in obtaining financial support.Consequences of changes in a parent’s ownershipinterest in a subsidiary that do not result in a loss ofcontrol18An entity shall present a schedule that shows the effects on the equity attributable to owners of the parent of any changes in its ownership interest in asubsidiary that do not result in a loss of control.Consequences of losing control of a subsidiary duringthe reporting period19An entity shall disclose the gain or loss,if any,calculated in accordance with paragraph25of IFRS10,and:(a)the portion of that gain or loss attributable to measuring any investmentretained in the former subsidiary at its fair value at the date whencontrol is lost;andA516IFRS FoundationIFRS12(b)the line item(s)in profit or loss in which the gain or loss is recognised(ifnot presented separately).Interests in unconsolidated subsidiaries(investment entities)19A An investment entity that,in accordance with IFRS10,is required to apply the exception to consolidation and instead account for its investment in a subsidiaryat fair value through profit or loss shall disclose that fact.19B For each unconsolidated subsidiary,an investment entity shall disclose:(a)the subsidiary’s name;(b)the principal place of business(and country of incorporation if differentfrom the principal place of business)of the subsidiary;and(c)the proportion of ownership interest held by the investment entity and,if different,the proportion of voting rights held.19C If an investment entity is the parent of another investment entity,the parent shall also provide the disclosures in19B(a)–(c)for investments that arecontrolled by its investment entity subsidiary.The disclosure may be providedby including,in the financial statements of the parent,the financial statementsof the subsidiary(or subsidiaries)that contain the above information.19D An investment entity shall disclose:(a)the nature and extent of any significant restrictions(eg resulting fromborrowing arrangements,regulatory requirements or contractualarrangements)on the ability of an unconsolidated subsidiary to transferfunds to the investment entity in the form of cash dividends or to repayloans or advances made to the unconsolidated subsidiary by theinvestment entity;and(b)any current commitments or intentions to provide financial or othersupport to an unconsolidated subsidiary,including commitments orintentions to assist the subsidiary in obtaining financial support.19E If,during the reporting period,an investment entity or any of its subsidiaries has,without having a contractual obligation to do so,provided financial orother support to an unconsolidated subsidiary(eg purchasing assets of,orinstruments issued by,the subsidiary or assisting the subsidiary in obtainingfinancial support),the entity shall disclose:(a)the type and amount of support provided to each unconsolidatedsubsidiary;and(b)the reasons for providing the support.19F An investment entity shall disclose the terms of any contractual arrangements that could require the entity or its unconsolidated subsidiaries to providefinancial support to an unconsolidated,controlled,structured entity,includingevents or circumstances that could expose the reporting entity to a loss(egliquidity arrangements or credit rating triggers associated with obligations topurchase assets of the structured entity or to provide financial support).IFRS Foundation A517IFRS1219G If during the reporting period an investment entity or any of its unconsolidated subsidiaries has,without having a contractual obligation to do so,providedfinancial or other support to an unconsolidated,structured entity that theinvestment entity did not control,and if that provision of support resulted inthe investment entity controlling the structured entity,the investment entityshall disclose an explanation of the relevant factors in reaching the decision toprovide that support.Interests in joint arrangements and associates20An entity shall disclose information that enables users of its financial statements to evaluate:(a)the nature,extent and financial effects of its interests in jointarrangements and associates,including the nature and effects of itscontractual relationship with the other investors with joint control of,or significant influence over,joint arrangements and associates(paragraphs21and22);and(b)the nature of,and changes in,the risks associated with its interestsin joint ventures and associates(paragraph23).Nature,extent and financial effects of an entity’sinterests in joint arrangements and associates21An entity shall disclose:(a)for each joint arrangement and associate that is material to thereporting entity:(i)the name of the joint arrangement or associate.(ii)the nature of the entity’s relationship with the joint arrangementor associate(by,for example,describing the nature of theactivities of the joint arrangement or associate and whether theyare strategic to the entity’s activities).(iii)the principal place of business(and country of incorporation,ifapplicable and different from the principal place of business)ofthe joint arrangement or associate.(iv)the proportion of ownership interest or participating share heldby the entity and,if different,the proportion of voting rightsheld(if applicable).(b)for each joint venture and associate that is material to the reportingentity:(i)whether the investment in the joint venture or associate ismeasured using the equity method or at fair value.(ii)summarised financial information about the joint venture orassociate as specified in paragraphs B12and B13.A518IFRS FoundationIFRS12(iii)if the joint venture or associate is accounted for using the equitymethod,the fair value of its investment in the joint venture orassociate,if there is a quoted market price for the investment.(c)financial information as specified in paragraph B16about the entity’sinvestments in joint ventures and associates that are not individuallymaterial:(i)in aggregate for all individually immaterial joint ventures and,separately,(ii)in aggregate for all individually immaterial associates.21A An investment entity need not provide the disclosures required by paragraphs21(b)–21(c).22An entity shall also disclose:(a)the nature and extent of any significant restrictions(eg resulting fromborrowing arrangements,regulatory requirements or contractualarrangements between investors with joint control of or significantinfluence over a joint venture or an associate)on the ability of jointventures or associates to transfer funds to the entity in the form of cashdividends,or to repay loans or advances made by the entity.(b)when the financial statements of a joint venture or associate used inapplying the equity method are as of a date or for a period that isdifferent from that of the entity:(i)the date of the end of the reporting period of the financialstatements of that joint venture or associate;and(ii)the reason for using a different date or period.(c)the unrecognised share of losses of a joint venture or associate,both forthe reporting period and cumulatively,if the entity has stoppedrecognising its share of losses of the joint venture or associate whenapplying the equity method.Risks associated with an entity’s interests in jointventures and associates23An entity shall disclose:(a)commitments that it has relating to its joint ventures separately fromthe amount of other commitments as specified in paragraphs B18–B20.(b)in accordance with IAS37Provisions,Contingent Liabilities and ContingentAssets,unless the probability of loss is remote,contingent liabilitiesincurred relating to its interests in joint ventures or associates(includingits share of contingent liabilities incurred jointly with other investorswith joint control of,or significant influence over,the joint ventures orassociates),separately from the amount of other contingent liabilities.IFRS Foundation A519。
地表水环境质量标准-欧盟

SECTION 1:
PHYSICO-CHEMICAL PARAMETERS
WATER TEMPERATURE (TWATER)
PART A: EXISTING QUALITY STANDARDS Abstraction of surface water for drinking water supply
Super and Second class first class cold waters: 20 oC summer, 5 oC winter warm waters: 28 oC summer, 8 oC winter
MAC -
Bathing Water /Recreation
EU: 76/160/EEC [oC] MD: Hygienic Regulation Nr. 06.6.3.23 (1997) [oC] G (Annex I) I MAC (Annex II) -
Ambient Standards
RO: GD 161 [oC] ICPDR [oC] ECE [oC] I II I II (TV) I II Quality class III Class III Quality class III IV V -
IV -
V -
IV -
V -
Footnotes (1) The directive 78/659/EEC contains two sets of standards. The first set (not mentioned in the table above) reads (Annex I): “1. Temperature measured downstream of a point of thermal discharge (at the edge of the mixing zone) must not exceed the unaffected temperature by more than: 1.5 oC, I value salmonid waters; 3 oC, I value for cyprinid waters. Derogations limited in geographical scope may be decided by Member States in particular conditions if the competent authority can prove that there are no harmful consequences for the balanced development of the fish population.” (2) Annex I of 78/659/EEC mentions: “Thermal discharges must not cause the temperature downstream of the point of thermal discharge (at the edge of the mixing zone) to exceed the following values [see table above] … The 10 oC temperature limit applies only to breeding periods of species which need cold water for reproduction and only to waters which may contain such species.” (3) Derogations are possible in accordance with Article 11: “The Member States may derogate from this Directive: (a) in the case of certain parameters marked (0) in Annex I, because of exceptional weather or special geographical conditions; (b) when designated waters undergo natural enrichment in certain substances, so that the values set out in Annex I are not respected. Natural enrichment means the process whereby, without human intervention, a given body of water receives from the soil certain substances contained therein.” (0) Exceptional climatic or geographical conditions
IEC61400-1-2005风电机组设计要求标准英汉对照
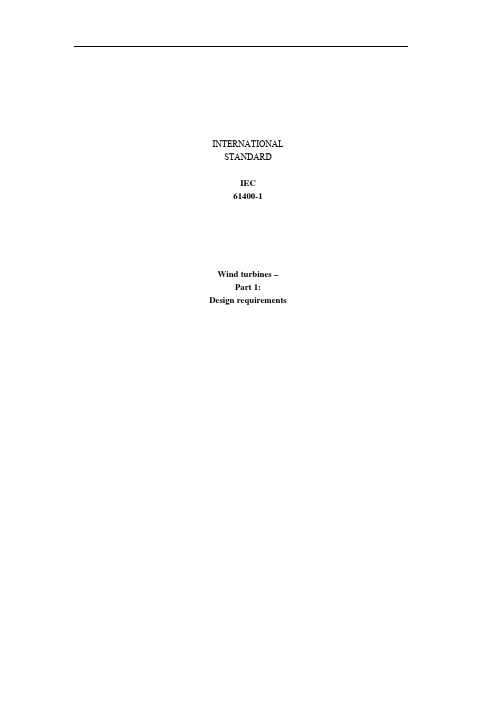
需要什么文档直接在我的文档里搜索比直接在网站大海捞针要容易的多也准确省时的多
INTERNATIONAL STANrbines – Part 1:
Design requirements
Publication numbering As from 1 January 1997 all IEC publications are issued with a designation in the 60000 series. For example, IEC 34-1 is now referred to as IEC 60034-1.
Further information on IEC publications The technical content of IEC publications is kept under constant review by the IEC, thus ensuring that the content reflects current technology. Information relating to this publication, including its validity, is available in the IEC Catalogue of publications (see below) in addition to new editions, amendments and corrigenda. Information on the subjects under consideration and work in progress undertaken by the technical committee which has prepared this publication, as well as the list of publications issued,is also available from the following: IEC Web Site (www.iec.ch) Catalogue of IEC publications The on-line catalogue on the IEC web site (www.iec.ch/searchpub) enables you to search by a variety of criteria including text searches,technical committees and date of publication. Online information is also available on recently issued publications, withdrawn and replaced publications, as well as corrigenda. IEC Just Published This summary of recently issued publications (www.iec.ch/online_news/justpub) is also available by email. Please contact the Customer Service Centre (see below) for further information. Customer Service Centre If you have any questions regarding this publication or need further assistance, please contact the Customer Service Centre: Email: custserv@iec.ch Tel: +41 22 919 02 11 Fax: +41 22 919 03 00 .
- 1、下载文档前请自行甄别文档内容的完整性,平台不提供额外的编辑、内容补充、找答案等附加服务。
- 2、"仅部分预览"的文档,不可在线预览部分如存在完整性等问题,可反馈申请退款(可完整预览的文档不适用该条件!)。
- 3、如文档侵犯您的权益,请联系客服反馈,我们会尽快为您处理(人工客服工作时间:9:00-18:30)。
Object retrieval with large vocabularies and fast spatial matching James Philbin1,Ondrej Chum1,Michael Isard2,Josef Sivic1and Andrew Zisserman1 1Department of Engineering Science,University of Oxford2Microsoft Research,Silicon Valley{james,ondra,josef,az}@ misard@AbstractIn this paper,we present a large-scale object retrieval system.The user supplies a query object by selecting a region of a query image,and the system returns a ranked list of images that contain the same object,retrieved from a large corpus.We demonstrate the scalability and perfor-mance of our system on a dataset of over1million images crawled from the photo-sharing site,Flickr[3],using Ox-ford landmarks as queries.Building an image-feature vocabulary is a major time and performance bottleneck,due to the size of our dataset. To address this problem we compare different scalable methods for building a vocabulary and introduce a novel quantization method based on randomized trees which we show outperforms the current state-of-the-art on an exten-sive ground-truth.Our experiments show that the quanti-zation has a major effect on retrieval quality.To further improve query performance,we add an efficient spatial ver-ification stage to re-rank the results returned from our bag-of-words model and show that this consistently improves search quality,though by less of a margin when the visual vocabulary is large.We view this work as a promising step towards much larger,“web-scale”image corpora.1.Object retrieval from a large corpusWe are motivated by the problem of retrieving,from a large corpus of images,the subset of images that contain a query object.1In practice,no algorithm will be able to make a perfect binary determination of whether or not an image lies in the query subset,and in fact even human judges may disagree on this due to occlusion,distortion,etc.We there-fore address the slightly different problem of ranking each image in the corpus to determine the likelihood that it con-tains the query object,and aim to return to the user some 1The query object is specified by a user selecting part of a query image, so it is really a“query region”however we will refer to it as an object to avoid overloading the term region.prefix of this ranked list,in descending rank order.A naive and inefficient solution to this task would be to formulate a ranking function and apply it to every image in the dataset before returning a ranked list.This is very com-putationally expensive for large corpora and the standard method in text retrieval[4,8]is to use a bag of words model, efficiently implemented as an invertedfile data-structure. This acts as an initial“filtering”step,greatly reducing the number of documents that need to be considered.Recent work in object based image retrieval[20,24]has mimicked simple text-retrieval systems using the analogy of“visual words.”Images are scanned for“salient”regions and a high-dimensional descriptor is computed for each re-gion.These descriptors are then quantized or clustered into a vocabulary of visual words,and each salient region is mapped to the visual word closest to it under this cluster-ing.An image is then represented as a bag of visual words, and these are entered into an index for later querying and retrieval.Typically,no spatial information about the image-location of the visual words is used in thefiltering stage.Despite the analogy between“visual words”and words in text documents,the trade-offs in ranking images and web pages are somewhat different.An image query is generated from an example image region and typically contains many more words than a text query.The words are“noisier”how-ever:in the web search case the user deliberately attempts to choose words that are relevant to the query,whereas the choice of words is abstracted away by the system in the image-retrieval case,and cannot be understood or guided by the user.Consequently,while web-search engines usu-ally treat every query as a conjunction,object-retrieval sys-tems typically include images that contain only,for exam-ple,90%of the query words,in thefiltered set.The biggest difference,however,is that the visual words in an image-retrieval query encode vastly more spatial structure than a text query.A user who types a three-word text query may in general be searching for documents con-taining those three words in any order,at any positions in the document.A visual query however,since it is selected from a sample image,automatically and inescapably in-cludes visual words in a spatial configuration corresponding to some view of the object;it is therefore reasonable to try to make use of this spatial information when searching the corpus for different views of the same object.In this paper we investigate two directions for improving visual object-retrieval performance.In both cases we are guided by the constraint that the methods should be scalable to extremely large image corpora.1.Improving the visual vocabulary.As noted above, image-based retrieval systems extract high-dimensional re-gion descriptors from images,then cluster these to form a vocabulary of visual words.Early systems[24]used aflat k-means clustering that was effective but difficult to scale to large vocabularies.More recent work has used cluster hi-erarchies[17]and greatly increased the visual-word vocab-ulary size[20]using them.We show in section3thatflat k-means can be scaled to similarly large vocabulary sizes by the use of approximate nearest neighbor methods.As will be demonstrated,this method has similar complexity to the vocabulary tree,but far superior performance.For the approximate nearest neighbors we employ a random for-est algorithm[5,15,23].This algorithm has recently been extensively used for supervised classification[19,25]and unsupervised matching[21].2.Incorporating spatial information into the ranking. Ideally we would like to verify that the target and query im-age regions were generated by the same object/scene region. Correspondence issues have been well studied both in the transformations required and in their estimation[14].For example,two views of a rigid object are related by epipo-lar geometry,two views of a planar patch are related by a homography,etc.Such mappings can be computed from correspondences of salient regions between the target and query images.Indeed,correspondences(to verify a match) can even be extended to deformations[11].In practice for collections such as consumer photographs,it may not be necessary to consider such general geometric transforma-tions.This is a question that can be assessed empirically by re-ranking on transformations of varying generality,and to this end we investigate a set of ranking methods,all of which can be implemented efficiently,in section4.Wefind that we are able to deal with image variations such as light-ing,shadows and partial occlusions without explicitly mod-eling them.For a concrete application,we choose searching on building facades and architectural features.This can be very challenging because of the near ambiguities that arise from repetitions of architectural building blocks:windows,doors etc.We use photos from the photo-sharing site Flickr[3], as this contains many examples of the typical building shots that touristscapture.Figure1.42randomly sampled images from the5K dataset.Note that the dataset contains difficult distractors which may easily be confused with those used in the query set.Dataset#images#features Size of descriptors5K5,06216,334,970 1.9GB 100K99,782277,770,83333.1GB1M1,040,8011,186,469,709141.4GBTotal1,145,6451,480,575,512176.4GB Table1.The number of images,features and descriptor sizes for each dataset.2.The Datasets,Evaluation&Implementation Oxford5K dataset.To evaluate performance when com-paring different visual vocabularies and spatial rankings, we have collected a set of images comprising11different Oxford“landmarks”–by landmark here we mean a par-ticular part of a building–together with distractors.Im-ages for each landmark were retrieved from Flickr[3],using queries such as“Oxford Christ Church”and“Oxford Rad-cliffe Camera.”We also retrieved further distractor images by seaching on“Oxford”alone.The entire dataset consists of5,062high resolution(1024×768)images.Sample im-ages from the dataset are shown infigure1.For each landmark we chose5different query regions,as shown infigure2.Thefive queries are used so that retrieval performance can be averaged over any individual query pe-culiarities.We obtain ground truth manually by searching over the entire dataset for the11landmarks.Images are assigned one of four possible labels:(1)Good–a nice,clear picture of the object/building.(2)OK–more than25%of the ob-ject is clearly visible.(3)Junk–less than25%of the object is visible,or there is a very high level of occlusion or dis-tortion.(4)Absent–the object is not present.The number of occurrences of the different landmarks range between7 and220good and ok images.The dataset of images and theR a d c l i f f e C a m P i t t R i v e r sM a g d a l e n K e b l e H e r t f o r d C o r n m a r k e t C h r i s t C h u r c hB o d l e i a n B a l l i o l A s h m o l e a nA l l S o u lsFigure 2.All 55query images used in the ground truth evaluation.Each row shows different queries for the same scene landmark.Note the large variation in scale of the query regions and the vari-ation in position,lighting,etc .of the images themselves.ground truth labelling are available at [1].In addition to this labelled set,we use two other datasets to stress-test retrieval performance when scaling up.These consist of images crawled from Flickr’s list of most popu-lar tags.The images in our datasets will not in general bedisjoint when crawled from Flickr,so we remove exact du-plicates from the sets.We then assume that these datasets contain no occurrences of the objects being searched for,so they act as distractors ,testing both the performance and scalability of our system.100K dataset.This data was crawled from Flickr’s 145most popular tags and consists of 99,782high resolution (1024×768)images.1M dataset.This data was crawled from Flickr’s 450most popular tags and consists of 1,040,801medium resolution (500×333)images.Table 1summarizes the relative sizes of the datasets.2.1.Performance evaluationTo evaluate the performance we use the average pre-cision (AP)measure computed as the area under the precision-recall curve for a query.Precision is defined as the ratio of retrieved positive images to the total number retrieved.Recall is defined as the ratio of the number of retrieved positive images to the total number of positive im-ages in the corpus.An ideal precision-recall curve has pre-cision 1over all recall levels and this corresponds to an av-erage precision of 1.We compute an average precision score for each of the 5queries for a landmark,averaging these to obtain a mean Average Precision (mAP)score.The average of these mAP scores is used as a single number to evaluate the overall performance.In computing the average precision,we use the Good and Ok images as positive examples of the landmark in ques-tion,Absent images as negative examples and Junk images as null examples.These null examples are treated as though they are not present in the database –our score is unaffected whether they are returned or not.2.2.ImplementationThis section overviews our bag-of-visual-words real-time object retrieval engine.Image description.For each image in the dataset,we find affine-invariant Hessian regions [18].Typically there are 3,300regions detected on an image of size 1024×768.For each of these affine regions,we compute a 128-D SIFT de-scriptor [16].The number of descriptors generated for each of our datasets is shown in table 1.A sample of the visual descriptors are quantized and then used to index the images for the search engine.In sec-tion 3.3we describe the number of descriptors and words used for quantization,but in all cases the visual vocabulary is computed on the 5K dataset.Search Engine.Our search engine uses the vector-space model [7]of information-retrieval.The query and each doc-ument in the corpus is represented as a sparse vector of term (visual word)occurrences and search proceeds by calculat-ing the similarity between the query vector and each doc-ument vector,using an L2distance.We use the standard tf-idf weighting scheme[7],which down-weights the con-tribution that commonly occurring,and therefore less dis-criminative,words make to the relevance score.For computational speed,the engine stores word occur-rences in an index,which maps individual words to the doc-uments in which they occur.In the worst case,the compu-tational complexity of querying the index is linear in the corpus size,but in practice it is close to linear in the number of documents that match a given query,generally a major saving.For sparse queries,this can result in a substantial speedup,as only documents which contain words present in the query need to be examined.The scores for each docu-ment are accumulated so that they are identical to explicitly computing the similarity.With large corpora of images,memory usage becomes a major concern.To help ameliorate this problem,the in-verted index is stored in a space-efficient binary-packed structure.Additionally,when main memory is exhausted, the engine can be switched to use an inverted indexflat-tened to disk,which caches the data for the most frequently requested words.For example,for a vocabulary size of1M words,our search engine implementation can query the combined 5K+100K datasets in approximately0.1s for a typical query and the inverted index consumes1GB of main memory.The size of the index for the combination of the5K+100K+1M datasets is4.3GB,larger than our available main memory, so we use an offline version of the indexflattened to disk. Querying this corpus from disk takes around15s–35s fora typical query.3.Visual vocabularies from scalable clusteringGenerating clusters for such a large quantity of data presents challenges to traditionally used algorithms.Even sub-sampling5%of the100K dataset would still require clustering roughly28million128dimensional descriptors. The size of the data essentially rules out methods such as mean-shift,spectral and agglomerative clustering.Even “simpler”clustering algorithms such as exact k-means fail to scale to this kind of size.Some work has been done on accelerating exact k-means[10],but this requires O(K2) extra storage,where K is the number of cluster centers,ren-dering it impractical for our purposes.In this work,we compare the performance of two differ-ent clustering methods:(a)approximate k-means,and(b) hierarchical k-means[20].The two methods are described in detail below.3.1.Approximate k-means(AKM)Thefirst method is an alteration to the original k-means algorithm.In typical k-means,the vast majority of compu-tation time is spent on calculating nearest neighbours be-tween the points and cluster centers.We replace this exact computation by an approximate nearest neighbor method, and use a forest of8randomized k-d trees[5,15,23] built over the cluster centers at the beginning of each it-eration to increase speed.We use randomized k-d tree code,optimized for matching SIFT descriptors,supplied by Lowe[16].Usually in a k-d tree,each node splits the dataset using the dimension with the highest variance for all the data points falling into that node and the value to split on is found by taking the median value along that dimension (although the mean can also be used).In the randomized version,the splitting dimension is chosen at random from among a set of the dimensions with highest variance and the split value is randomly chosen using a point close to the me-dian.The conjunction of these trees creates an overlapping partition of the feature space and helps to mitigate quanti-zation effects,where features which fall close to a partition boundary are assigned to an incorrect nearest neighbour. This robustness is especially important in high-dimensions, where due to the“curse of dimensionality”[22],points will be more likely to lie close to a boundary.A new data point is assigned to the(approximately)clos-est cluster center as follows.Initially,each tree is descended to a leaf and the distances to the discriminating boundaries are recorded in a single priority queue for all trees[6].Then, we iteratively choose the most promising branch from all trees and keep adding unseen nodes into the priority queue. We stop once afixed number of tree paths have been ex-plored.This way,we can use more trees without signifi-cantly increasing the search time.The algorithmic complexity of a single k-means iteration is now reduced from O(NK)to O(N log(K)),where N is the number of features being clustered from.Our tests have shown that at least for moderate values of K,the percentage of points assigned to different cluster centers differs from the exact version by less than1%,motivating the approach. Note that this method has the same time and memory com-plexity as the hierarchical vocabulary tree clustering of[20] described below.If a scaling-up beyond reasonable mem-ory requirements is needed,the top level branches of each tree can be distributed to different machines.3.2.Hierarchical k-means(HKM)Nist´e r and Stew´e nius[20]propose generating a“vocab-ulary tree”using a hierarchical k-means clustering scheme (also called tree structured vector quantization[13]).On the first level of the tree,all data points are clustered to a small number(K=10)of cluster centers.On the next level,k-means(with K=10again)is applied within each of the partitions independently.The result is K n clusters at the n-th level.For example,using a branching factor of10with6 levels results in1M leaf nodes.A new data point is assigned by descending the tree.Instead of assigning each data point to the single leaf node at the bottom of the tree,the pointsClustering parameters mAP#of descr.V oc.size k-means AKM800K10K0.3550.3581M20K0.3840.3855M50K0.4640.45316.7M1M0.618parison of the performance of exact k-means to our AKM method on the5K dataset,using different numbers of train-ing descriptors and clusters.can additionally be assigned to some internal nodes which their path from root to leaf passes through.This can help mitigate the effects of quantization error,for cases when the data point lies close to the V oronoi region boundary for each cluster center.It is important to note that traditionalflat k-means mini-mizes the total distortion between the data points and their assigned,closest cluster centers,whereas the hierarchical tree minimizes this distortion only locally at each node and this does not in general result in a minimization of the total distortion.3.3.Results on comparing vocabulariesOur goal is to evaluate the retrieval performance of vi-sual vocabularies built using the two clustering methods de-scribed above.Here,we test only thefiltering stage of the retrieval system,i.e.retrieval is performed using only the invertedfile(including the tf-idf weighting),and no rank-ing using the spatial configuration of regions is used.We perform three main experiments.Firstly,we compare per-formance using AKM toflat k-means.This is to establish how much,if any,performance is lost by the approximation. Secondly,we compare AKM to HKM.Thirdly,we investi-gate how the performance using AKM degrades as we scale up the number of images in the corpus.k-means vs AKM.For the small5K dataset,we compare AKM to exact k-means,using varying amounts of sub-sampled data and cluster centers with identical cluster ini-tialization.These results are given in table2,and show that our approximate method gives very similar performance to exact k-means,differing in mAP by less than1%and out-performing k-means in two cases.This justifies the use of AKM as an effective proxy for exact k-means,but with a fraction of the computational cost.HKM vs AKM.We compare our method to HKM in two ways.First,we compare performance on the Recognition Benchmark introduced by[20].This consists of10,200im-ages split into four image groups of the same scene taken from different viewpoints.A perfect result is to return, given a query,the other three images from that query’s group before images from other groups.This is expressed as an average over the number of the top four correctly re-turned,taken over all possible query images.We also dis-play a graph,showing how the query performance changesMethod Scoring AverageLevels TopHKM1 3.16HKM2 3.07HKM3 3.29HKM4 3.29AKM 3.45Subset SizeAverageTopTable3.A comparison of the AKM and HKM on the Recog-nition Benchmark of[20]using the descriptors for training and testing provided by the authors of[20].“HKM”is the hierarchi-cal k-means quantization,where the numbers are taken from[2].“AKM”is the result of our approximate k-means clustering.Both methods use a vocabulary of1M visual words and an L1distance.Method Dataset mAPBag-of-words Spatial(a)HKM-15K0.4390.469(b)HKM-25K0.418(c)HKM-35K0.372(d)HKM-45K0.353(e)AKM5K0.6180.647(f)AKM5K+100K0.4900.541(g)AKM5K+100K+1M0.3930.465 Table4.V ocabulary comparison over the three datasets.For the HKM method,the number of levels used for scoring is listed in the method name.All methods use1M cluster centers,generated from all16.7M descriptors in the5K dataset.The“spatial”method is described in section4.as increasingly large subsets of the data are searched over. To train our clusters,we use identical training and testing descriptors to[20]provided at[2],and an L1distance to compute the ranking.From table3,we see that for the same number of visual words,our method significantly out-performs the hierarchical method.Second,we have also compared the performances of the two methods on our own5K dataset,shown in table4,rows (a)–(e),using our descriptors.Here,we have used our own implementation of HKM which we have found gives almost identicalfigures on the dataset from[2].The AKM method clearly outperforms the best HKM method,by0.618to 0.439mAP.This might be attributed to quantization effects of the vocabulary tree–data points may be suffering from bad initial splits close to the root of the vocabulary tree.As a result,descriptors arising from the same object/scene re-gion in different images can be assigned(due to e.g.noise) to different clusters.Hierarchical scoring might partially overcome this problem,but wefind that the hierarchical scoring actually hurts the performance of the HKM method. However,if we switch the vector scoring to use the L1dis-tance(instead of L2),wefind that the hierarchical scoring improves performance,but doesn’t produce as good a result as in the L2case(0.427best L1vs.0.439best L2).Clearly, more work is needed to understand the HKM performance here.V ocab Bag ofSize words Spatial 50K0.4730.599 100K0.5350.597 250K0.5980.633 500K0.6060.642 750K0.6090.630 1M0.6180.645 1.25M0.6020.625x 105Vocabulary SizemAPTable5.Examining the effect of vocabulary size on performance for the5K dataset.Each vocabulary is trained using AKM on all16.7M descriptors.There is a performance peak at1mil-lion words.The spatial verification consistently improves perfor-mance.Scaling up with AKM.We explore a number of different vocabulary sizes for the5K dataset in table5.This shows a peak in performance at1M visual words,although for large numbers of clusters,the performance curve appears quite flat and we predict the performance would not significantly degrade for moderately larger vocabularies.We evaluate the scalability of our method on the5K, 5K+100K and5K+100K+1M datasets in table4,rows(e)–(g),using the1M words visual vocabulary.In going from the smallest dataset to the largest,a226-fold increase in the number of images,the performance falls by just over20%. We attribute this drop in performance to a lack of sufficient discrimination in the quantization for the larger dataset.As will be seen,this performance loss is ameliorated to some extent once spatial ranking is included.4.Spatial re-rankingThe output from performing a query on the invertedfile described previously is a ranked list of images for a sig-nificant section of the corpus.We have until now consid-ered the features in each image as a visual bag-of-words and have ignored the spatial configurations of features.We now investigate re-ranking the top-ranked results using spa-tial constraints.The spatial verification procedure estimates a transformation between the query region and each target image,based on how well its feature locations are predicted by the estimated transformation.We then re-rank target im-ages based on the discriminability of the spatially verified visual words.4.1.Transformations and their estimationAs is now standard in estimation algorithms on visual data,two types of measurement error must be considered: errors in a detected feature’s position and shape;and errors due to outliers from mismatched or missing features,be-cause of detector failure,occlusion,etc.The standard solu-tion is to use the RANSAC algorithm[12];this involves gen-erating transformation hypotheses using a minimal num-ber of correspondences and then evaluating each hypothesis based on the number of“inliers”among all featuresunder that hypothesis.Table6.(a)The three affine sub-groups compared in the spatial re-ranking.(b)Computing H as H−12H1,preserving“upness”for the5dof case.Typically,photos are taken from a restricted range of canonical views and we can use this prior information to speed up transformation estimation.We choose to use LO-RANSAC[9],a variant of RANSAC.It involves generating hypotheses of an approximate model and then iteratively re-evaluating promising hypotheses using the full transforma-tion.By selecting a restricted class of transformations for the hypothesis generation stage and exploiting shape infor-mation in the affine-invariant image regions,we are able to generate hypotheses with only a single pair of correspond-ing features.This greatly reduces the number of possible hypotheses which need to be considered and significantly speeds up the matching procedure.We therefore choose to enumerate all such hypotheses,which removes the random-ness from our algorithm,resulting in a deterministic proce-dure.We compare three affine sub-groups for hypothesis gen-eration,with degrees of freedom ranging between3and5, that are listed in table6(a).This is to evaluate whether or not there is any significant performance difference between transformation types.In each case we use a general(6dof) affine transformation for the iterative re-estimation step of LO-RANSAC.The3dof transformation approximately cov-ers situations such as a change in zoom or camera distance to the scene,but not foreshortening.The4dof transforma-tion approximately covers foreshortening by either a hori-zontal or vertical scaling between views.The5dof trans-formation preserves the vertical direction and allows for anisotropic scaling and vertical shear.These three models take advantage of the fact that images are usually displayed on the web with the correct(upright)orientation.For this reason,we have not allowed for in-plane image rotations. Implementation details.The3dof transformation(method (i)in the following results)is computed from a single region correspondence using the regions’centroids to estimate the translation,and each region’s scale to estimate the isotropic scale change between the query region and the target image.For the4dof transformation(method(ii))from a single region correspondence,the scaling in the x direction is com-puted from the ratio of the regions’x extents(and similarly for the y scaling).The5dof transformation(method iii)is estimated from。