Interaction Modeling with Artificial Life Agents
多模态人机交互综述(译文)
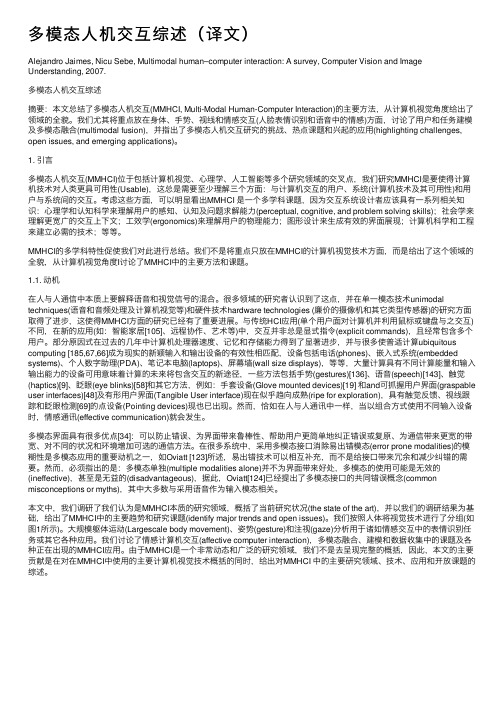
多模态⼈机交互综述(译⽂)Alejandro Jaimes, Nicu Sebe, Multimodal human–computer interaction: A survey, Computer Vision and Image Understanding, 2007.多模态⼈机交互综述摘要:本⽂总结了多模态⼈机交互(MMHCI, Multi-Modal Human-Computer Interaction)的主要⽅法,从计算机视觉⾓度给出了领域的全貌。
我们尤其将重点放在⾝体、⼿势、视线和情感交互(⼈脸表情识别和语⾳中的情感)⽅⾯,讨论了⽤户和任务建模及多模态融合(multimodal fusion),并指出了多模态⼈机交互研究的挑战、热点课题和兴起的应⽤(highlighting challenges, open issues, and emerging applications)。
1. 引⾔多模态⼈机交互(MMHCI)位于包括计算机视觉、⼼理学、⼈⼯智能等多个研究领域的交叉点,我们研究MMHCI是要使得计算机技术对⼈类更具可⽤性(Usable),这总是需要⾄少理解三个⽅⾯:与计算机交互的⽤户、系统(计算机技术及其可⽤性)和⽤户与系统间的交互。
考虑这些⽅⾯,可以明显看出MMHCI 是⼀个多学科课题,因为交互系统设计者应该具有⼀系列相关知识:⼼理学和认知科学来理解⽤户的感知、认知及问题求解能⼒(perceptual, cognitive, and problem solving skills);社会学来理解更宽⼴的交互上下⽂;⼯效学(ergonomics)来理解⽤户的物理能⼒;图形设计来⽣成有效的界⾯展现;计算机科学和⼯程来建⽴必需的技术;等等。
MMHCI的多学科特性促使我们对此进⾏总结。
我们不是将重点只放在MMHCI的计算机视觉技术⽅⾯,⽽是给出了这个领域的全貌,从计算机视觉⾓度I讨论了MMHCI中的主要⽅法和课题。
多模态人机交互综述(译文)
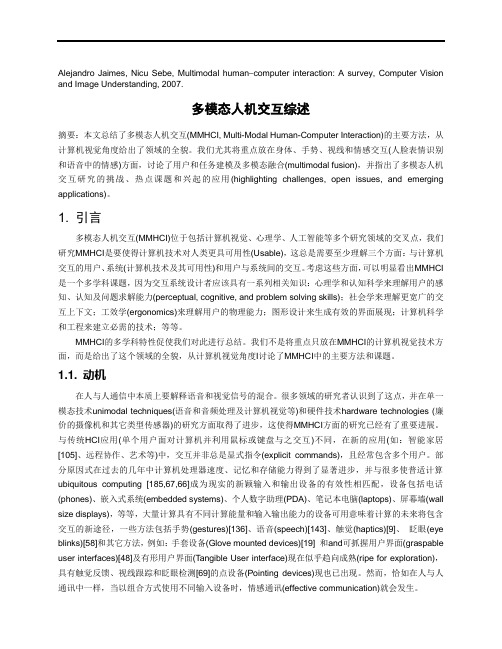
Alejandro Jaimes, Nicu Sebe, Multimodal human–computer interaction: A survey, Computer Vision and Image Understanding, 2007.多模态人机交互综述摘要:本文总结了多模态人机交互(MMHCI, Multi-Modal Human-Computer Interaction)的主要方法,从计算机视觉角度给出了领域的全貌。
我们尤其将重点放在身体、手势、视线和情感交互(人脸表情识别和语音中的情感)方面,讨论了用户和任务建模及多模态融合(multimodal fusion),并指出了多模态人机交互研究的挑战、热点课题和兴起的应用(highlighting challenges, open issues, and emerging applications)。
1. 引言多模态人机交互(MMHCI)位于包括计算机视觉、心理学、人工智能等多个研究领域的交叉点,我们研究MMHCI是要使得计算机技术对人类更具可用性(Usable),这总是需要至少理解三个方面:与计算机交互的用户、系统(计算机技术及其可用性)和用户与系统间的交互。
考虑这些方面,可以明显看出MMHCI 是一个多学科课题,因为交互系统设计者应该具有一系列相关知识:心理学和认知科学来理解用户的感知、认知及问题求解能力(perceptual, cognitive, and problem solving skills);社会学来理解更宽广的交互上下文;工效学(ergonomics)来理解用户的物理能力;图形设计来生成有效的界面展现;计算机科学和工程来建立必需的技术;等等。
MMHCI的多学科特性促使我们对此进行总结。
我们不是将重点只放在MMHCI的计算机视觉技术方面,而是给出了这个领域的全貌,从计算机视觉角度I讨论了MMHCI中的主要方法和课题。
专业英文词汇表
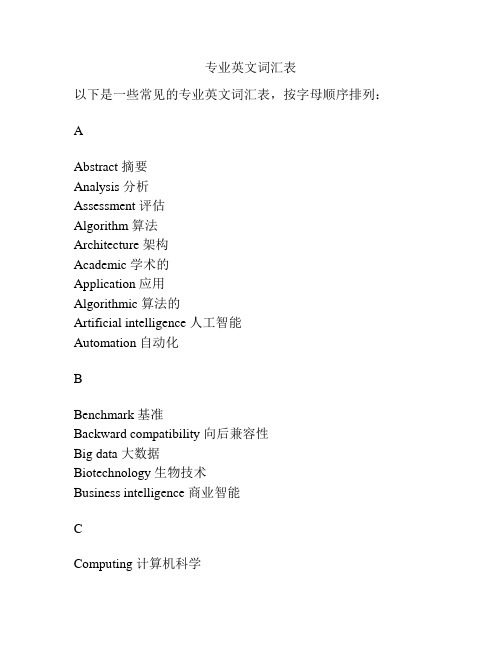
专业英文词汇表以下是一些常见的专业英文词汇表,按字母顺序排列:AAbstract 摘要Analysis 分析Assessment 评估Algorithm 算法Architecture 架构Academic 学术的Application 应用Algorithmic 算法的Artificial intelligence 人工智能Automation 自动化BBenchmark 基准Backward compatibility 向后兼容性Big data 大数据Biotechnology 生物技术Business intelligence 商业智能CComputing 计算机科学Cryptography 密码学Component 组件Computer graphics 计算机图形学Control system 控制系统Cybersecurity 网络安全DData 数据Database 数据库Design 设计Development 开发Digital 数字化Distributed system 分布式系统EEncryption 加密Ethics 伦理学Engineering 工程学Experiment 实验Expert system 专家系统FFramework 框架Functional programming 函数式编程Genetic algorithm 遗传算法Grid computing 网格计算HHardware 硬件Hypothesis 假设Human-computer interaction 人机交互Hierarchical clustering 分层聚类IInformation technology 信息技术Interface 接口Internet of Things 物联网Image processing 图像处理JJava programming language Java编程语言KKnowledge 知识Knowledge management 知识管理LLogic logicLinguistics 语言学Linear programming 线性规划MMachine learning 机器学习Modeling 建模Machine vision 机器视觉Microprocessor 微处理器Multimedia 多媒体NNetwork 网络Neural network 神经网络OObject-oriented programming 面向对象编程Operating system 操作系统Optimization 优化Open-source 开源PProgramming programmingParallel computing 并行计算Quality assurance 质量保证Protocol 协议QQuery 查询RRobotic 嵌入式Robotics 机器人技术Random access memory (RAM) 随机存取存储器SSimulation 模拟Software 软件Systematic system 系统化System 系统Statistical analysis 统计分析Security 安全Storage 存储TTesting 测试Technology 技术Telecommunication 通信Theory 理论Transaction 事务Time series 时间序列Turing machine 图灵机UUser interface 用户界面Undo 撤销VVirtual reality 虚拟现实Validation 验证Visualization 可视化WWeb development 网络开发Wireless 无线XXML (Extensible Markup Language) 可扩展标记语言YYield rate 产率ZZero-day vulnerability 零日漏洞。
Artificial Intelligence and Decision Making
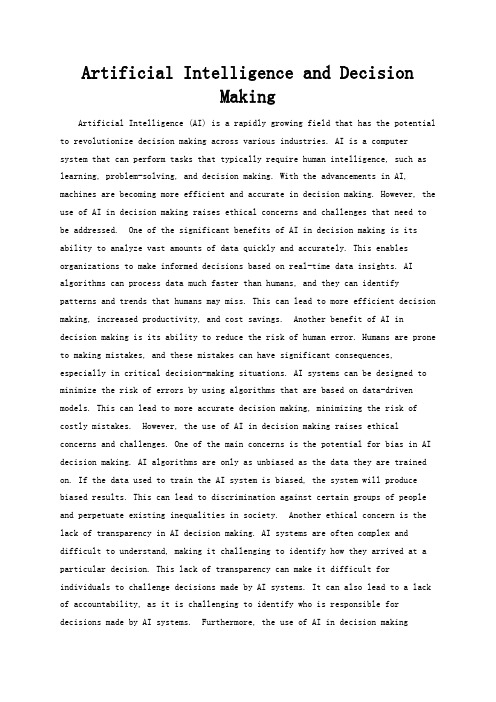
Artificial Intelligence and DecisionMakingArtificial Intelligence (AI) is a rapidly growing field that has the potential to revolutionize decision making across various industries. AI is a computer system that can perform tasks that typically require human intelligence, such as learning, problem-solving, and decision making. With the advancements in AI, machines are becoming more efficient and accurate in decision making. However, the use of AI in decision making raises ethical concerns and challenges that need to be addressed. One of the significant benefits of AI in decision making is its ability to analyze vast amounts of data quickly and accurately. This enables organizations to make informed decisions based on real-time data insights. AI algorithms can process data much faster than humans, and they can identify patterns and trends that humans may miss. This can lead to more efficient decision making, increased productivity, and cost savings. Another benefit of AI in decision making is its ability to reduce the risk of human error. Humans are prone to making mistakes, and these mistakes can have significant consequences, especially in critical decision-making situations. AI systems can be designed to minimize the risk of errors by using algorithms that are based on data-driven models. This can lead to more accurate decision making, minimizing the risk of costly mistakes. However, the use of AI in decision making raises ethical concerns and challenges. One of the main concerns is the potential for bias in AI decision making. AI algorithms are only as unbiased as the data they are trained on. If the data used to train the AI system is biased, the system will produce biased results. This can lead to discrimination against certain groups of people and perpetuate existing inequalities in society. Another ethical concern is the lack of transparency in AI decision making. AI systems are often complex and difficult to understand, making it challenging to identify how they arrived at a particular decision. This lack of transparency can make it difficult for individuals to challenge decisions made by AI systems. It can also lead to a lack of accountability, as it is challenging to identify who is responsible for decisions made by AI systems. Furthermore, the use of AI in decision makingraises concerns about job displacement. As AI systems become more advanced, they can replace human workers in various industries. This can lead to job losses and economic disruption, especially in industries that rely heavily on manual labor. In conclusion, the use of AI in decision making has the potential to revolutionize various industries by improving efficiency, accuracy, and productivity. However, it also raises ethical concerns and challenges that need to be addressed. To ensure that AI is used ethically and responsibly, it is essential to address issues such as bias, transparency, and job displacement. By addressing these challenges, we can harness the benefits of AI while minimizing its potential negative impacts.。
abm建模的基本概念
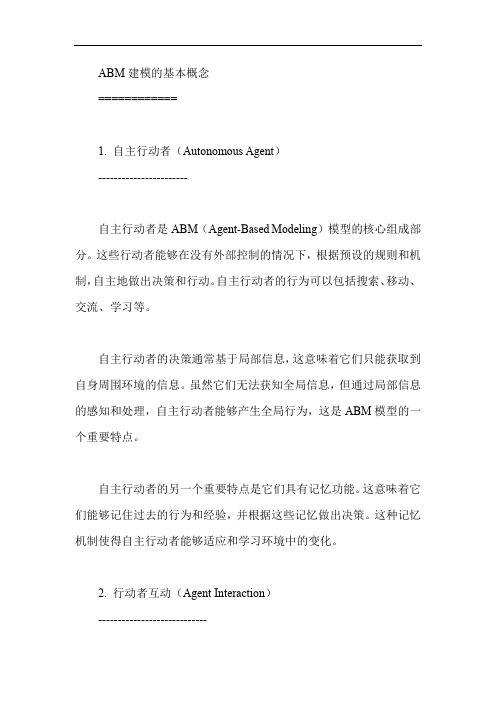
ABM建模的基本概念============1. 自主行动者(Autonomous Agent)-----------------------自主行动者是ABM(Agent-Based Modeling)模型的核心组成部分。
这些行动者能够在没有外部控制的情况下,根据预设的规则和机制,自主地做出决策和行动。
自主行动者的行为可以包括搜索、移动、交流、学习等。
自主行动者的决策通常基于局部信息,这意味着它们只能获取到自身周围环境的信息。
虽然它们无法获知全局信息,但通过局部信息的感知和处理,自主行动者能够产生全局行为,这是ABM模型的一个重要特点。
自主行动者的另一个重要特点是它们具有记忆功能。
这意味着它们能够记住过去的行为和经验,并根据这些记忆做出决策。
这种记忆机制使得自主行动者能够适应和学习环境中的变化。
2. 行动者互动(Agent Interaction)----------------------------在ABM模型中,自主行动者之间会进行互动。
这种互动可以包括物理交互(例如,两个个体在空间中的碰撞)、信息交互(例如,通过语言或信号进行的信息交换)、能量交互(例如,通过食物链进行的能量流动)等。
行动者之间的互动会影响到它们的行为和决策。
例如,如果一个行动者在环境中发现另一个行动者,它可能会选择与对方进行交互,或者避开对方。
这种交互的复杂性可以通过不同的规则和机制来描述和控制。
3. 人工建构世界(Artificial World)---------------------------在ABM模型中,人工建构世界是由自主行动者和其他静态或动态的对象构成的。
这个世界的结构由建模者设计和实现,它可以是真实的(如一个城市、一个生态系统),也可以是虚构的(如一个游戏、一个故事)。
人工建构世界提供了自主行动者的生存环境和交互对象。
这个世界中的物理规律、社会规则、资源分布等因素都会影响到自主行动者的行为和决策。
Philosophical Transactions of the Royal Society London – A (2003) The Role of Social and C
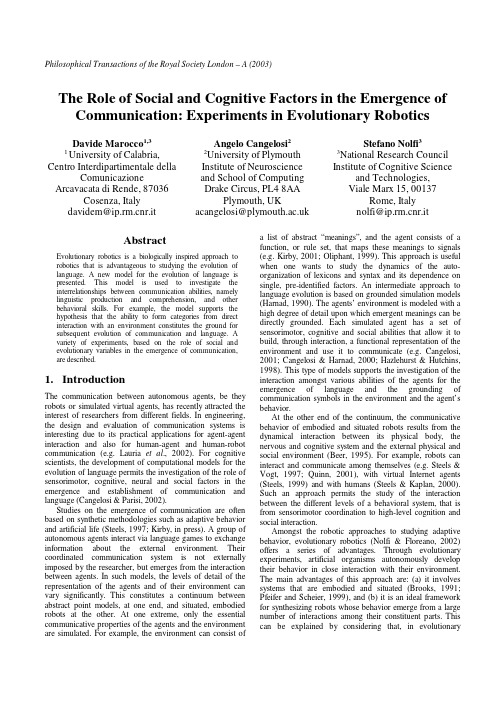
The Role of Social and Cognitive Factors in the Emergence of Communication: Experiments in Evolutionary RoboticsDavide Marocco1,31 University of Calabria, Centro Interdipartimentale della Comunicazione Arcavacata di Rende, 87036Cosenza, Italydavidem@r.itAngelo Cangelosi22University of PlymouthInstitute of Neuroscienceand School of ComputingDrake Circus, PL4 8AAPlymouth, UKacangelosi@Stefano Nolfi33National Research CouncilInstitute of Cognitive Scienceand Technologies,Viale Marx 15, 00137Rome, Italynolfi@r.itAbstractEvolutionary robotics is a biologically inspired approach to robotics that is advantageous to studying the evolution of language. A new model for the evolution of language is presented. This model is used to investigate the interrelationships between communication abilities, namely linguistic production and comprehension, and other behavioral skills. For example, the model supports the hypothesis that the ability to form categories from direct interaction with an environment constitutes the ground for subsequent evolution of communication and language. A variety of experiments, based on the role of social and evolutionary variables in the emergence of communication, are described.1. IntroductionThe communication between autonomous agents, be they robots or simulated virtual agents, has recently attracted the interest of researchers from different fields. In engineering, the design and evaluation of communication systems is interesting due to its practical applications for agent-agent interaction and also for human-agent and human-robot communication (e.g. Lauria et al., 2002). For cognitive scientists, the development of computational models for the evolution of language permits the investigation of the role of sensorimotor, cognitive, neural and social factors in the emergence and establishment of communication and language (Cangelosi & Parisi, 2002).Studies on the emergence of communication are often based on synthetic methodologies such as adaptive behavior and artificial life (Steels, 1997; Kirby, in press). A group of autonomous agents interact via language games to exchange information about the external environment. Their coordinated communication system is not externally imposed by the researcher, but emerges from the interaction between agents. In such models, the levels of detail of the representation of the agents and of their environment can vary significantly. This constitutes a continuum between abstract point models, at one end, and situated, embodied robots at the other. At one extreme, only the essential communicative properties of the agents and the environment are simulated. For example, the environment can consist of a list of abstract “meanings”, and the agent consists of a function, or rule set, that maps these meanings to signals (e.g. Kirby, 2001; Oliphant, 1999). This approach is useful when one wants to study the dynamics of the auto-organization of lexicons and syntax and its dependence on single, pre-identified factors. An intermediate approach to language evolution is based on grounded simulation models (Harnad, 1990). The agents’ environment is modeled with a high degree of detail upon which emergent meanings can be directly grounded. Each simulated agent has a set of sensorimotor, cognitive and social abilities that allow it to build, through interaction, a functional representation of the environment and use it to communicate (e.g. Cangelosi, 2001; Cangelosi & Harnad, 2000; Hazlehurst & Hutchins, 1998). This type of models supports the investigation of the interaction amongst various abilities of the agents for the emergence of language and the grounding of communication symbols in the environment and the agent’s behavior.At the other end of the continuum, the communicative behavior of embodied and situated robots results from the dynamical interaction between its physical body, the nervous and cognitive system and the external physical and social environment (Beer, 1995). For example, robots can interact and communicate among themselves (e.g. Steels & Vogt, 1997; Quinn, 2001), with virtual Internet agents (Steels, 1999) and with humans (Steels & Kaplan, 2000). Such an approach permits the study of the interaction between the different levels of a behavioral system, that is from sensorimotor coordination to high-level cognition and social interaction.Amongst the robotic approaches to studying adaptive behavior, evolutionary robotics (Nolfi & Floreano, 2002) offers a series of advantages. Through evolutionary experiments, artificial organisms autonomously develop their behavior in close interaction with their environment. The main advantages of this approach are: (a) it involves systems that are embodied and situated (Brooks, 1991; Pfeifer and Scheier, 1999), and (b) it is an ideal framework for synthesizing robots whose behavior emerge from a large number of interactions among their constituent parts. This can be explained by considering that, in evolutionaryexperiments, robots are synthesized through a self-organization process based on random variation and selective reproduction where the selection process is based on the behaviors that emerge from the interactions among the robot's constituent elements and between these elements and the environment. This allows the evolutionary process to freely exploit interactions without the need to understand in advance the relation between interactions and emerging properties as it is necessarily required in other approaches that rely more on explicit design.For these reasons the evolutionary robotics approach has been successfully applied to the synthesis of robots able to exploit sensorimotor coordination (Nolfi, 2002); on-line adaptation (Nolfi and Floreano, 1999); body and brain co-evolution (Lipson and Pollack, 2000); competing and cooperative collective behaviors; (Nolfi and Floreano, 1998, Martinoli, 1999; Baldassarre, Nolfi, and Parisi, 2002).These advantageous aspects of evolutionary robotics are of particular importance for modeling the evolution of language and communication. Sensorimotor coordination, social interaction, evolutionary dynamics and the use of neural systems can all have a potential impact in the emergence of coordinated communication. In this paper, new experiments are presented that study the emergence of communication in evolutionary robotics models. They are based on recent work by Nolfi and Marocco (2002) for the emergence of sensorimotor categorization. Nolfi and Marocco evolved the control system of artificial agents that are asked to categorize objects with different shapes on the basis of tactile information. Each agents uses proprioceptive information to actively explore objects using a three-segment arm. In addition, the agent uses the activation of one output node of its neural network controller as input. Agents are selected only for their performance in discriminating (categorizing) the objects using this unit, not for their ability to explore them. This results in the emergence of an active tactile exploration strategy that differentiate between objects of different shapes. Nolfi and Marocco’s model is an example of explicit self-categorization.In this new model, the robotic agents share the explicit categorization of objects. That is, the activation of the output nodes is the signal (“name”) sent to another agent to instruct it on what to do with the object. Agents will be selected on their ability to manipulate objects correctly, not on their (linguistic) ability to name them correctly. A variety of experiments will test the role of different social and evolutionary variables. These will be used to analyze the role of sensorimotor, social and cognitive factors in the emergence of communication. The direct relations between behavioral and communication abilities, such us language production and comprehension, will also be discussed. 2. MethodThe behavior of each agent consists of exploration within the environment, on the basis of tactile information, and the communication, about the type of objects that are in it. The environment consists of an open three-dimensional space in which one of two different objects is present in each epoch (Figure 1). The two objects used in this simulation are asphere and a cube.Figure 1 – The arm and a spherical object.Figure 2 – A schematic representation of the arm.Agents are provided with a 3-segments arm with 6 degrees of freedom (DOF) and extremely course touch sensors (see Figure 2). Each segment consists in a basic structure of two cylindrical bodies and two joints. This is replicated for three times. The basic structure consists of a shorter body of radius 2.5 and length 3 and a longer body of the same radius and length 10 for the first two segments. The length of the third segment is 5. This shorter segment represents a fingerless hand. The two bodies of each segment are connected by means of a joint (i.e. the Joint E in the Figure) that allows only one DOF on axis Y, while the shorter body is connected at the floor, or at the longer body, by means of a joint (i.e. the Joint R) that provides one DOF on axis X. In practice, the Joint E allows to elevate and lower the connected segments and the Joint R allows to rotate them in both direction. Notice that Joint E is free to moves only in a range between 0 and π/2, just like a human arm that can bend the elbow solely in a direction. The rangeof Joint R is [–π/2, +π/2]. Gravity is {0, –1, 0}. Each actuator is provided with a corresponding motor that can apply a maximum force of 50. Therefore, to reach every position in the environment the control system has to appropriately control several joints and to deal with the constraints due to gravity.The sensory system consists of a simple contact sensor placed on each longer body that detects when this body collides with another, and two proprioceptive sensors that provide the current position of each joint.The controller of each individual consists of an artificial neural networks with 11 sensory neurons connected to 3 hidden neurons. These connect with 8 output neurons. The first 9 sensory neurons encode the angular position (normalized between 0.0 and 1.0) of the 6 DOF of the joints and the state of the three contact sensors located in the three corresponding segments of the arm. The other 2 sensory neurons receive their input from the other agents. The first 6 motor neurons control the actuators of the corresponding joints. The output of the neurons is normalized between [0, +π/2] and [–π/2, +π/2] in the case of elevation or rotational joints respectively and is used to encode the desired position of the corresponding joint. The motor is activated so to apply a force (up to 50) proportional to the difference between the current and the desired position of the joint. The last 2 output neurons encode the signal to be communicated to the other agents. This works as a small winner-takes-all cluster, where the neuron with the highest activation is set to 1 and the other to 0.The activation state of internal neurons was updated accordingly to the following equations (output neurons were updated according to the logistic function):+=iij j jO w t()()1)1(11−−−+−+=j A j t jj j eO O ττ(1) 10≤≤j τWith Aj being the activity of the j th neuron (or the state of the corresponding sensor in the case of sensory neurons), tj the bias of the j th neuron, Wij the weight from the i th to the j th neuron, Oi the output of the i th neuron. Oj is the output of the j th neuron, τj the time constant of the j th neuron.Each individual was tested for 36 epochs, each epoch consisting of 150 sensorimotor cycles. At the beginning of each epoch the arm is fully extended. A spherical or a cubic object is placed in a random selected position in front of the arm. The position of the object is randomly selected between the following intervals: 15.0 <= X <= 25.0; Y = 7.5; –5.0 <= Z <= 5.0). The object is a sphere (15 units in diameter) during even epochs and a cube (15 units in side) during odd epochs so that each individual has to discriminate the same number of spherical and cubic objects during its lifetime.In addition to the proprioceptive information, agents also receive in input a 2-bit signal produced by some other agent in the population, such as the parent or any agent from the population (linguistic comprehension task). The protocol of interaction and communication between agents was systematically varied and is analyzed in section 3.Before they act as speaker, agents undergo a linguistic production task. That is, each agent is put in the environment and asked to interact with the object. The value of the two output neurons in the last cycle of the epoch is saved and used as the signal produced to “name” the object. A genetic algorithm is used to evolve the behavior of agents. The genotype of each agent consists of 81 parameters that include 67 weights, 11 biases, and 3 time constants. Each parameter is encoded with 8 bits. Weights and biases are normalized between –5.0 and 5.0, time constants are normalized between 0.0 and 1.0.The fitness rewards the behavior of the agent with the current object in the environment. Good communication behavior does not produce any fitness gain for the speaker. Following the behaviors evolved in Nolfi & Marocco’s (2002) simulation, the agent has to touch and stay in contact with one object (the sphere) and has to avoid as much as possible to touch the other object (cube). The fitness of individuals is computed by summing the number of cycles in which the agent touches the sphere or does not touch the cube. Fitness scores decrease for each cycle the agent touches the cube or when it does not touch the sphere.A population of 80 agents is used in each simulation. During selection, the 20 agents with the highest fitness (i.e. behavioral performance) reproduce and each make 4 offspring. The genotype of each offspring is then subject to mutation with an overall probability of 2%. That is, each bit has a 2% probability of being mutated, by generating a random binary value. There is generational overlap between the population of parents and that of new offspring. The first will only act as speakers and cannot reproduce anymore. The population of new offspring will be subject to the fitness test and will reproduce at the end of their lifetime. Evolutionary simulation of embodied robotic agents can be time consuming and computationally expensive. To reduce the time necessary to test individual behaviors and to model the real physical dynamics as accurately as possible, the rigid body dynamics simulation SDK of Vortex TM was used 1. This was linked to the EvoRobot simulator (Nolfi, 2000).3. ResultsThe simulation model was used to run a series of experiments on the role of various social and evolutionary variables in the emergence of shared communication. The first independent variable refers to the selection of speakers (SPEAKER) with two levels: Parent or All. In the first case, each agent receives communication signals only from its own parent. In the second level of the variable, each agent1/products/vortex/can receive signals from any individual of the previous population. This factor is aimed at investigating the role of different social groups of speakers in facilitating shared communication.The second independent variable manipulated during experiments consists in the time in which communication is allowed (COMMUNICATION) with two levels: From_0 and From_50. In the first case, agents were allowed to communicate from the initial random generation. In the second level of the variable, agents start to communicate between themselves only at generation 50, i.e. after they have evolved a good ability to touch/avoid the two objects. Through this variable it will be possible to investigate the initial behavioral and cognitive abilities necessary to evolve communication.For each of the 4 conditions (2 SPEAKER × 2 COMMUNICATION), 10 replications were executed, by changing the initial random population. Fifty generations were necessary to pre-evolve an optimal behavior of object manipulation to be used in the From_50 conditions. Table 1 reports the communication success in each condition in terms of good populations and percentage of good speaker in the population. The criterion for deciding whether a population has successfully evolved communication depends on the fact that, at the last generation, at least 50% of agents produce two signals that differentiate the two objects.Table 1 – Data on the emergence of communication in each experimental condition. The first line contains the number of populations (out of 10) where communication emerged. The second line contains the average percentage of good speakers for the 10 replications and the average for the best performing population (value between brackets).SPEAKER COMMUNICATIONFrom 0COMMUNICATIONFrom 50Parent# good pops % speakers (best pop)527% (75%)763% (100%)All# good pops % speakers (best pop)7% (20%)5% (27%)The results of the number of populations that evolve shared communication clearly show that it is only when the parents act as the speakers there is a selective pressure for the emergence and preservation of a shared communication system. In particular, 7 populations out of 10 reach a stable communication system when language is introduced after agents have learned to use both objects. Figure 3 shows the fitness curves and the proportion of good speaker in the best seed of the condition From_50 - Parent speaker.When communication is introduced directly from the initial random population, the probability of evolving a good language, together with a good behavior, is lower (5 populations out of 10). This advantage for evolving languages after the basic behavioral skills have evolved is similar to that observed by Cangelosi & Parisi (2001) in a grounded simulation model on the emergence of verbs and nouns.When agents listen to all individuals of the previous generation, no stable communication exists in the last generations. In fact, during evolution good lexicons sometimes emerge for a short time, but they are not maintained or further developed by the whole population. A temporary good lexicon is defined as the case in which at least 20% of agents use two different signals to name the two objects. In 8 of the 10 From_50 - All speaker populations, such temporary appearances of good signal production is observed. Figure 4 shows the best population in the From_50 - All speaker conditions. Here the longest period of good production only lasts for 17 generations,with a maximum peak of best language at 41%.Figure 3 – Data for the best population of the condition Parentspeaker - From_50.Figure 4 – Data for the best population in condition All speakers - From_50.The lexicon produced by agents in successful replications has been tested to investigate whether individuals actually use this language in a meaningful way, i.e. avoid the cube when the signal produced in response to the cube is used, and touch the sphere when the other signal is used. Figure 5 shows the behavior of an agent that interacts with the cube with or without language. This tests the linguistic comprehension ability of agents. The pictures on the left column (Figure 5 - left) show the behavior of the agent when no input signal is used. The agent needs to touch the cube, at least once (in cycle 95), to identify it as a cubeand then retract from it. The pictures on the right (Figure 5 - right) show the behavior of the agent when the signal “10” is used as additional input. This signal is produced by the parent organism at the end of the interaction with a cube. During this scene, the agent does not need to touch the cube at all because the signal “10” identifies it as a cube. The meaning of “10” can be interpreted as “cube”2, because the listener treats the object as a cube, and the speaker produces it after its interaction with a cube. When the signal “01” is used, the agent touches the object regardless of its shape. Inthis case, “01” has the meaning of “sphere”.Figure 5 – Agent’s interaction with the cube and test of linguistic understanding ability. Left column: Only the proprioceptive input is given to the agent. Right column: An additional communication signal is given as input. This is produced by another agent at the end of its interaction with a cube. Figures from the best individual of a From_50 - Parent speaker population.Fitness data shows that final scores in the 4 experimental conditions reflect the pattern of results on the emergence of successful communication. The two conditions with Parent speakers reach the highest fitness scores, with a significant2This signal can also be interpreted as the verb avoid , instead of as the noun cube . In fact, in this model it is not possible to distinguish between syntactic word classes (cf. Cangelosi & Parisi 2001 and Cangelosi 2001 for a discussion)advantage for the From_50 populations (e.g. average fitness of best individuals = 0.55; fitness peak in best population = 0.72) versus the From_0 population (average = 0.45, peak = 0.66). The baseline for the behavior without communication is the fitness at generation 50 of the From_50 simulation, before agents start to communicate (average = 0.44, peak = 0.52). Consider that the maximum hypothetical fitness score is 1. This can never be reached because, for example, at the beginning of each epoch some negative fitness cycles are always necessary for agents to reach the spherical object and start gaining fitness.4. DiscussionThere are several issues that can be discussed regarding these results, and what we can learn from the model. A series of questions will be used to analyze the results.Question 1: Is there any benefit to be in a population wheregood communication has emerged?Question 2: Is there any direct advantage to evolving agood linguistic comprehension ability?To answer the first question, it is possible to compare the fitness results in the simulations where no shared communication emerged, and those where good communication systems evolved. The condition in which communication emerged more frequently (From_50, Parent speaker) will be used as example. In this condition, 7 populations evolved good languages, whilst 3 did not. Figure 6 shows the average fitness of the good communication populations (thick lines) and that of the no communication populations (thin lines). The chart clearly shows that agents who use communication reach fitness values that are higher that those not communicating. This is true both for the fitness of the best individual and for that of the whole population. For example, at the final generation the average fitness of the 7 successful communication replications is 0.35, while it is 0.21 for the 3 unsuccessful populations. Moreover, the fitness in these 3 populations remains relatively constant during the simulation. In the first 50 generations after communication is permitted (i.e. from 50 to 100), there is no increase and the average fitness at generation 100 is very similar to that at generation 50. In the remaining generations, the agents gain some extra fitness points, which are due to the continuation of the evolutionary algorithm search.The extra fitness gain in populations that evolve communication is easily explained by the direct benefits for the behavior (i.e. fitness) of using two different signals: one for the cube, and one for the sphere. As already shown in Figure 5, during the interaction with a cube the input of its “name” produces significant improvements to behavioral performance. Agents do not need to touch the object to recognize it, and therefore do not lose fitness due to such exploratory behavior. In addition, they gain fitness in every cycle. There is also some benefit for the use of the signal for the sphere. If an agent initially is told that there is a spherical object in the environment, it can go directlytowards the object and touch it, without having to use some interaction cycles for recognizing the object as a non cube.The previous explanations also answer the second question, since they identify a direct adaptive advantage for evolving a good comprehension ability.Figure 6 – Average fitnesses of the conditions From_50 - Parent speaker. Thick lines refer to the average fitness of the 7 replications where good communication emerged (continuous line for the best agent and dotted line for the average of all agents). Thin lines refer to the average fitness of the 3 replications where no shared communication emerged.Question 3: Is there any “direct” advantage to evolving good linguistic production abilities?This question is more difficult to answer. In fact, there seems to be no direct fitness advantage to the agents to speaking well. Individuals only update their fitness when they hear others speaking. When agents act as speakers, some have already reproduced, whilst the others have not been selected at all. In the condition Parent speaker, agents only speak to their own children. Therefore, the kinship relationship can partially explain this apparent altruistic behavior and the indirect fitness gain for the common genes shared by the parent and its offspring (e.g. Ackley & Littman, 1994). The benefits of kin selection can also explain the successful evolution of communication in the Parent speaker versus the All speaker conditions. However, there is another important phenomenon to be considered. In the Parent speaker conditions, the linguistic input to each listener is constant, since its parent will always use the same signal for the same object. In addition, when the parent is a good speaker (i.e. it uses two different signals to refer to the two objects), its signals are more reliable. The child can then try to use them to improve its fitness performance. In the All speaker conditions, the high variability of the linguistic input coming from all agents of previous generation can be too unreliable, and agents will tend to ignore it.In the All speaker conditions, some communication abilities also emerge, although the number of good speakers never reaches the critical amount needed to allow it to remain stable until the end of the simulation (cf. Figure 4).In addition, in the Parent speaker conditions, there are threecases when shared communication does not evolve. According to the altruistic, kin selection explanation, allParent speaker populations should evolve communicationbecause of it indirect adaptive advantage. The fact that this does not always happen raises the issues of understanding the relation between linguistic comprehension/productionabilities and other behavioral/cognitive abilities (question4), and the identification of factors that cause and favor the emergence of shared communication (question 5). First, thedata in Table 1 indicates that it is easier to evolve goodcommunication when language is introduced after the pre-evolution of good behavioral capacities (7 out of 10 populations) than when agents are allowed to communicatefrom the initial generation (5 out of 10 seeds). In addition,the onset of effective communication (i.e. when at least 20% of agents speak well) is much earlier in the From_50 populations (on average after 16 generations) that in theFrom_0 simulations (on average after 41 generations). Thisdata is consistent with Cangelosi and Parisi’s (2001) model on the evolution of syntactic languages. This researchshowed that agents learn languages more efficiently whencommunication is introduced after the pre-evolution of goodbehavioral skills. Effectively, the pre-evolution of good behavior “prepares” a cognitive ground upon which goodlinguistic abilities can start to develop. Analyses of thecategorical perception effects in language learning models have shown that language uses and modifies the space of similarities between members of different perceptual andlinguistic categories (Cangelosi & Harnad, 2000). Question 4: What is the relation between comprehension,production and behavioral abilities? Question 5: What are the underlying factors that cause andfavor the emergence of communication?To understand better the relations betweencommunication abilities and behavioral skills, thecorrelations between fitness scores and a measure of the quality of produced language have been computed. Figure 7and 8 present the averages of the fitness curves, theproportions of good speakers (i.e. language index), thefitness/language correlation r all for the whole population, and the fitness/language correlation r best for the best 20agents. Figure 7 refers to the 7 successful populations of the From_50 - Parent speaker condition. Figure 8 refers to data from the remaining 3 populations without communication.For the computation of the language index based on theproportion of good speakers, an agent is classified as goodspeaker when it produces two opposite signals respectively for the two objects in at least half of the 36 epochs. ThePearson r correlations index was used.Overall, the two figures show that the correlationbetween the fitness of all agents and their language production index is positive and quite high (r all ≈ 0.5) after good communication emerges. This can explain themaintenance of good communication, since it reflects a link。
人机结合的空间认知
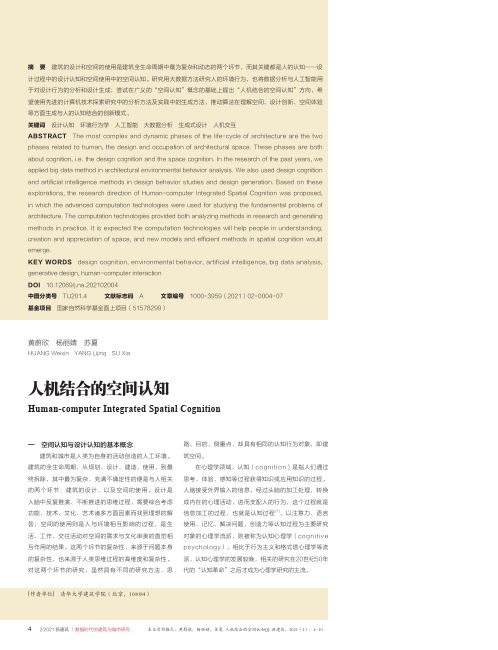
4 2/2021 新建筑 | 数据时代的建筑与城市研究[作者单位] 清华大学建筑学院(北京,100084)人机结合的空间认知Human-computer Integrated Spatial Cognition摘 要 建筑的设计和空间的使用是建筑全生命周期中最为复杂和动态的两个环节,而其关键都是人的认知——设计过程中的设计认知和空间使用中的空间认知。
研究用大数据方法研究人的环境行为,也将数据分析与人工智能用于对设计行为的分析和设计生成;尝试在广义的“空间认知”概念的基础上提出“人机结合的空间认知”方向,希望使用先进的计算机技术探索研究中的分析方法及实践中的生成方法,推动算法在理解空间、设计创新、空间体验等方面生成与人的认知结合的创新模式。
关键词 设计认知 环境行为学 人工智能 大数据分析 生成式设计 人机交互ABSTRACT The most complex and dynamic phases of the life-cycle of architecture are the two phases related to human, the design and occupation of architectural space. These phases are both about cognition, i.e. the design cognition and the space cognition. In the research of the past years, we applied big data method in architectural environmental behavior analysis. We also used design cognition and artificial intelligence methods in design behavior studies and design generation. Based on these explorations, the research direction of Human-computer Integrated Spatial Cognition was proposed, in which the advanced computation technologies were used for studying the fundamental problems of architecture. The computation technologies provided both analyzing methods in research and generating methods in practice. It is expected the computation technologies will help people in understanding, creation and appreciation of space, and new models and efficient methods in spatial cognition would emerge.KEY WORDS design cognition, environmental behavior, artificial intelligence, big data analysis, generative design, human-computer interaction DOI 10.12069/j.na.202102004中图分类号 TU201.4 文献标志码 A 文章编号 1000-3959(2021)02-0004-07基金项目 国家自然科学基金面上项目(51578299)黄蔚欣 杨丽婧 苏夏HUANG Weixin YANG Lijing SU Xia一 空间认知与设计认知的基本概念建筑和城市是人类为自身的活动创造的人工环境。
(完整版)用户界面交互设计中英术语
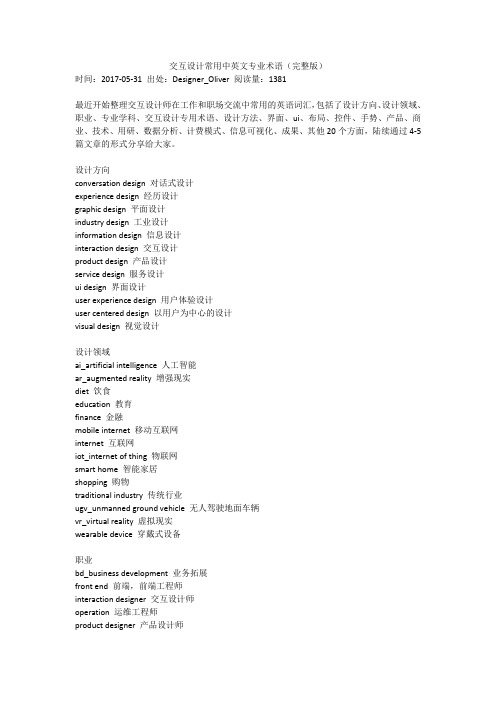
交互设计常用中英文专业术语(完整版)时间:2017-05-31 出处:Designer_Oliver 阅读量:1381最近开始整理交互设计师在工作和职场交流中常用的英语词汇,包括了设计方向、设计领域、职业、专业学科、交互设计专用术语、设计方法、界面、ui、布局、控件、手势、产品、商业、技术、用研、数据分析、计费模式、信息可视化、成果、其他20个方面,陆续通过4-5篇文章的形式分享给大家。
设计方向conversation design 对话式设计experience design 经历设计graphic design 平面设计industry design 工业设计information design 信息设计interaction design 交互设计product design 产品设计service design 服务设计ui design 界面设计user experience design 用户体验设计user centered design 以用户为中心的设计visual design 视觉设计设计领域ai_artificial intelligence 人工智能ar_augmented reality 增强现实diet 饮食education 教育finance 金融mobile internet 移动互联网internet 互联网iot_internet of thing 物联网smart home 智能家居shopping 购物traditional industry 传统行业ugv_unmanned ground vehicle 无人驾驶地面车辆vr_virtual reality 虚拟现实wearable device 穿戴式设备职业bd_business development 业务拓展front end 前端,前端工程师interaction designer 交互设计师operation 运维工程师product designer 产品设计师product manager 产品经理project manager 项目经理qa_quality assurance 测试,测试工程师r&d_research&develop 研发,研发工程师ui designer 界面设计师user experience designer 用户体验设计师visual designer 视觉设计师专业与学科computer science and technology 计算机科学与技术ergonomics 人体工程学,人因学ethnology 人种学hci_human computer interaction 人机交互industrial design 工业设计interaction design 交互设计multimedia design and production 多媒体设计与制作psychics 心理学software engineering 软件工程statistics 统计学service design 服务设计visual communication design 视觉传达设计设计专用术语business 业务/商业business requirement 业务需求competitive analysis 竞品分析deepness 深度dimension 维度emotional design 情感化设计flow 流程goal 目标ia_information architecture 信息架构information 信息motivation 动机path 路径range 广度usage scenario 使用场景usability 可用性user behavior 用户行为user requirement 用户需求user study/user research用户调研设计方法与工具brainstorming 头脑风暴card sorting 卡片分类法emotional design 情感化设计fitts' law费茨定律gestalt psychology 格式塔心理学storyboard 故事版storyline 故事大纲user analysis 用户分析ucd user centered design 以用户为中心的设计界面cli_command line interface 命令行界面gui_graphical user interface 图形用户界面nui_natural user interface 自然用户界面vui_voice user interface 语音用户界面布局absolutelayout 绝对布局autolayout 自动布局banner 横幅border 边界线card based design 卡片式设计column 列content 内容dashboard 仪表盘framelayout 单帧布局float 悬浮grid 网格horizontal 水平layout 布局linearlayout 线性布局margin 外间距navigation bar 导航栏padding 内间距pinterest style layout 瀑布流relativelayout 相对布局row 行tablelayout 表格布局tool bar 工具栏widget 小部件vertical 垂直控件alert 警告anchors 锚点bottom sheet 底部动作条button 按钮canvas 画布card 卡片checkbox 复选框chip 纸片(android material design专有名词)data picker 日期选择器dialog 提示框,对话框divider 分隔线float 悬浮image 图像item 条,项目label 只读文本link 链接list 列表listview 列表视图loading 加载menu 菜单pagecontrol 多页控件(即小圆点)panel 面板password 密码picker 选择器progress bar 进度条radio 单选框table 表格tile 瓦片(android material design专有名词)time picker 时间选择器title 标题toast toast(无准确翻译,一种会自动消失的轻量提示框)scroll 滚动scroll bar 滚动条scrollview 滚动视图selector 选择器selection control 选择控制器slider 滑块snackbar snackbar(无准确翻译,一种会自动消失,带有操作的轻量提示框)sub header 副标题submit 提交switch 开关tab tab(无准确翻译,更多指导航上的选项)tag 标签textview 文本框toggle 开关tooltips 工具提示webview 网页视图手势click 点击drag 拖曳finger 手指hotspot 热区pinch 捏press 压,按stretch 伸展swipe 滑动tap 轻触zoom in 放大zoom out 缩小成果draft 草稿demo 演示interaction design document 交互文档hi fi prototype_high fidelity prototype 高保真原型lo fi prototype_low fidelity prototype 低保真原型prototype 原型wireframe 线框图ux workflow 交互流程图用户研究a/b test a/b测试expert evaluation 专家评估eye tracking 眼动跟踪focus group 焦点小组heuristic evaluation 启发式评估persona 用户画像questionnaire问卷调研usability testing 可用性测试user interview 用户访谈user experience map 用户体验地图user study/user research 用户调研data analyse 数据分析产品与商业account 账号advertisement 广告as 客户服务aso_app store optimization 应用商店优化business 商业copy 文案cms 内容管理系统customer 客户customer service 客服feed 信息流fsd_functional specifications document 功能详细说明function 功能individualization 个性化market 市场mrd_market requirements document 市场需求文档mvp_minimum viable product 最小化可行产品pgc_professionally generated content 专业生产内容prd_product requirements document 产品需求文档product design 产品设计process 项目,进度product 产品requirement 需求share 份额stickiness 黏性slogan 口号/标语/广告语strategy 策略user 用户ugc_user generated content 用户原创内容uml_unified modeling language 统一建模语言viral marketing 病毒式营销/病毒性营销uialignment 对齐art 艺术art base 美术/设计出身brand 品牌color 颜色icon 图标flat design 扁平化设计font 字体grid 栅格系统hierarchy 层次kv_key visual 主视觉, 主画面layer 层legibility 可读性logo 商标,徽标material 素材opacity 透明度responsive design 响应式设计resolution 分辨率sans serif typeface 非衬线体scale 比例缩放serif typeface 衬线字体skeuomorphic design 拟物化设计style 样式texture 纹理theme 主题typography 排版visual design 视觉设计技术api 应用程序编程接口/应用程序界面background 后台client 客户端container 容器data 数据database 数据库deep learning 深度学习developer 开发者format 格式化framework 框架machine learning 机器学习library 库optimize 优化performance 性能plug in 插件program 程序script 脚本sdk_software development kit 软件开发工具包seo 搜索引擎优化server 服务器technology 技术type 类型timer 定时器,计时器url 统一资源定位、网址visuality 可视性信息可视化bar chart 柱状图bubble chart 气泡图chart 图表dashboard 仪表盘information visualization 信息可视化line chart 折线图pie chart 饼图radar chart 雷达图scatter chart 散点图tree view树状图广告计费模式cpa_cost per action 每次行动(下载、注册等)成本cpc_cost per click 每次点击成本cpm_cost per mille 每千次展现成本数据acu_average concurrent users 平均同时在线用户数cac_ customer acquisition cost 用户获取成本click_click through 点击量/点击次数cpc 点击成本ctr_click rate_click through rate 点击率dau_daily active user 日活跃用户量dnu_day new user 日新增用户量gmv_gross merchandise volume 商品交易总量kpi_key performance indicator 关键绩效指标mau_monthly active user 月活跃用户量pcu_peak concurrent users 最高在线用户数pv_page view 页面浏览量roi_return on investment 投资回报率uv_unique visitor 独立访客数wau_weekly active users 周活跃用户量其他fyi/fyr 供参考kpi 关键绩效指标manual 手册schedule 工作进度计划表, 日程安排产品与商业account 账号advertisement 广告as 客户服务aso_app store optimization 应用商店优化business 商业copy 文案cms 内容管理系统customer 客户customer service 客服feed 信息流fsd_functional specifications document 功能详细说明function 功能individualization 个性化market 市场mrd_market requirements document 市场需求文档mvp_minimum viable product 最小化可行产品pgc_professionally generated content 专业生产内容prd_product requirements document 产品需求文档product design 产品设计process 项目,进度product 产品requirement 需求share 份额stickiness 黏性slogan 口号/标语/广告语strategy 策略user 用户ugc_user generated content 用户原创内容uml_unified modeling language 统一建模语言viral marketing 病毒式营销/病毒性营销uialignment 对齐art 艺术art base 美术/设计出身brand 品牌color 颜色icon 图标flat design 扁平化设计font 字体grid 栅格系统hierarchy 层次kv_key visual 主视觉, 主画面layer 层legibility 可读性logo 商标,徽标material 素材opacity 透明度responsive design 响应式设计resolution 分辨率sans serif typeface 非衬线体scale 比例缩放serif typeface 衬线字体skeuomorphic design 拟物化设计style 样式texture 纹理theme 主题typography 排版visual design 视觉设计布局absolutelayout 绝对布局autolayout 自动布局banner 横幅border 边界线card based design 卡片式设计column 列content 内容dashboard 仪表盘framelayout 单帧布局float 悬浮grid 网格horizontal 水平layout 布局linearlayout 线性布局margin 外间距navigation bar 导航栏padding 内间距pinterest style layout 瀑布流relativelayout 相对布局row 行tablelayout 表格布局tool bar 工具栏widget 小部件vertical 垂直技术api 应用程序编程接口/应用程序界面background 后台client 客户端container 容器data 数据database 数据库deep learning 深度学习developer 开发者format 格式化framework 框架machine learning 机器学习library 库optimize 优化performance 性能plug in 插件program 程序script 脚本sdk_software development kit 软件开发工具包seo 搜索引擎优化server 服务器technology 技术type 类型timer 定时器,计时器url 统一资源定位、网址visuality 可视性UI :用户界面,是英文User和interface的缩写。
- 1、下载文档前请自行甄别文档内容的完整性,平台不提供额外的编辑、内容补充、找答案等附加服务。
- 2、"仅部分预览"的文档,不可在线预览部分如存在完整性等问题,可反馈申请退款(可完整预览的文档不适用该条件!)。
- 3、如文档侵犯您的权益,请联系客服反馈,我们会尽快为您处理(人工客服工作时间:9:00-18:30)。
Interaction Modeling with Artificial Life AgentsErnesto Germán-Soto, Leonid B. Sheremetov, Christian Sánchez-SánchezMexican Petroleum Institute, Mexico{egerman, cssanche, sher}@imp.mxAbstract. In this work, an interaction model between artificial life agents(creatures) is proposed, which allows studying emergent social behavior ofagents. This model describes the environment of artificial life and autonomouscreatures in terms of goal-states, rules of behavior based on agents’ goals andactions, initial knowledge and the use of communication instructions. For thecase of study, the technology and environment of Creatures Labs are used toimplement artificial life agents based on the proposed model.1 IntroductionThe goal to construct autonomous agents has been explored within the artificial life (AL) community focusing mostly on such aspects of reactive, non-predictable and spontaneous behavior as action generation, adaptation and learning, than on knowledge and reasoning. An extensive discussion on autonomous agents and their typical AL architectures can be found in [9]. Within the AL paradigm, the agents are entities inhabiting the digital world of the computers and networks (i) with the purpose of simulating tasks like survival and exploration in the environments inaccessible or dangerous for the humans or (ii) developed for training purposes [3, 8]. Recently, more attention has been paid to interaction, considering such aspects as emotions modeling, intentions, social behavior and communication [1, 12].This work has its origin in the interest to apply some of the fundamental aspects of the agent theory in combination with the AL technology called Cyberlife [5, 6], with which intelligent organisms (called creatures or agents) can be created. The idea is that creatures interact with others through the implementation of diverse interaction mechanisms for the cooperative problem solving, actions coordination or synchronization, conflicts resolution and, this way to handle the social, learning and adaptation issues. In order to obtain the emergent social behavior based on the individual behavior of the agents, their design is an integration of (i) the reactive architecture [2] based on situation-action rules for the basic behavior and (ii) a deliberative architecture based on individual and group goals [11] adding intelligent social functionality.The infrastructure used for the accomplishment of this work is the Cyberlife Creature Labs platform [10], based on the biological metaphor of the artificial life. This work explores new capabilities to simulate interaction and social coexistence usually limited in traditional behaviorist models. In order to obtain this, the AL environment and autonomous creatures are modeled in terms of goal-states, rules ofbehavior based on agents’ goals and actions, initial knowledge and use of more sophisticated communication instructions than those that the creatures have at the moment. The artificial life agent model is defined including these elements. As the case study, several scenarios of a planet exploration are implemented and discussed.2 Model of Artificial Life AgentThe creatures developed using the Creature Labs technology have a set of mandatory elements like organs, cells, sanguineous flow, chemical reactions and can handle emotions, diseases and abilities to survive in an environment where they are able to simulate an emergent reactive behavior. The creatures use artificial neural networks as controls for the coordination of perceptions and actions and also the ideas of biological evolution in the form of genetic algorithms and genetic evolution. In both cases, the aspects of the agent design, like the values of certain parameters that govern his structure or operation, are codified like genetic material or genome of the agent.Creatures simulate the senses of vision, smell, and tact. All are modeled using semi-symbolic techniques. The vision simulation, for example, does not involve a simulation of the retinal image processing, but if a certain object is within the vision scope, a neuron is activated representing its presence in the field of view.The features of the creatures are specified in their genome. This is the genetic structure composed of two main parts. On the one hand, the brain is responsible for the sensorial - motor coordination and the selection of behavior, on the other, biochemical system models a simple power metabolism interacting with the brain. Taking the stimuli from the environment, the neurons of an input lobe are excited where each one represents a different object type and by means of an output lobe the signals are transformed to determine the attention focus. Three layers participate in the decision making: the perception layer, the concepts space and the decision layer itself establishing the relations between memories and decision making where each neuron represents an action like to activate, to deactivate, to walk, etc. Finally, reinforcement learning layer is implemented by changes in the decision layer in function of the existence of a chemical award or punishment. In the following sections, the elements composing the structures that we have added to agents in order to produce social behavior during their interaction are explained in more details.2.1 ActionsThe actions are the activities allowing interaction between the elements of the environment, and as well denoting a behavior class. Creatures make decision on an action to execute through their neural networks. From the actions, three levels of interaction can be defined. First, called agent-internal state, occurs when an agent executes some action and registers internal changes in his internal state and components that later can influence the other actions. Another type of interaction we called agent-environment, which takes place when an agent interacts with its elements, producing effects on the environment and enabling the interactions between its objects that further can be perceived and manipulated by the creatures. Finally, theInteraction Modeling with Artificial Life Agents 3 third type allows creatures to interact with each other. This leads to the development of the awareness capacity both on his own actions and those of the others, since by means of this interaction, agreements, distribution of tasks, etc. can be achieved.2.2 Goal-StatesA goal is defined in the sense to represent a state that the agent can obtain if some preconditions in the environment, in the realized actions and in his internal state are fulfilled. Its purpose is to guide agent’s behavior within the community. The goals found to be useful for this type of AL environments have been classified in two ways: first, called permanent goals, stimulate the agent guiding his existence during the life cycle; second, called temporal goals, stimulate him until they are fulfilled.The permanent goals from the beginning of the creatures’ life take care of the survival conditions, although an agent also can acquire permanent goals on run time. The temporal goals are adopted to obtain the permanent goals. These two types of goals can be used for individual or group effects. A group goal is such an objective that involves a group of agents because if being reached it brings common benefits. Normally, this type of goals is composed of a set of activities or responsibilities (roles) that the agents can acquire as their own goals. When creatures obtain a role to fulfill a group goal, they activate their neurons to fire the rules allowing them, by means of the temporal goals, to reach their group goal. Whenever a group goal is reached, the system sends a stimulus that the creatures interpret to finish their participation in an associated role.2.3 Rules of social behaviorA rule of social behavior is defined in terms of the internal states that an agent has, indicating by means of this, the execution of the actions as a result of being in the situation defined by such states. With this class of rules, the execution of actions that guide the behavior of the creatures during their interaction with the environment and its objects is characterized. Each one of these rules is represented in the brain of the creatures and its activation is defined by the priority of their internal goals and the group goals at the moment. One of the main objectives of the rules of social behavior is to try to avoid the interference in the actions executed by different agents, and this way they can fulfill their goals.2.4 Initial knowledgeKnowledge is the information considered useful to reach the goal that resides internally in the creatures’ brain. An agent begins to operate with primary knowledge. Much like the humans do in their rationality, the creatures use instincts, activated by this initial knowledge. This way, creatures can identify objects (through creature’s neurons that represent them), be aware of the actions (neurons that represent the actions, see section 3.1) and the rules of social behavior to execute.2.5 Instructions of interactionInteraction results in a series of actions by means of which agents communicate with each other. Perceived information is interpreted according to the receptor’s goal-state and finally generates a change in his internal state, keeping or modifying some knowledge or changing the goal-state. Perception is implemented by different specialized senses as follows. By means of the vision sense, the agents can recognize objects and their states. With the ear sense, the messages like an order are received. Orders are represented like action-object (get-food) or action-object-direction (walk-room-right). Different composed forms can be obtained enabling to implement more complex behaviors. For example, when an agent requires to take an object and to walk to the right followed by another order like to stop and to eat, a composed instruction like ((get, object, right), (stop, eat)) can be used. The things that an agent can touch (like a chemical substance) are perceived by the tactile sense.On the other hand, the communication scheme being used for the agents is based on two types of information: the first is called “help”, when the agents emit information that indicates request or dangerous situations. The second type is called “call for agreement”, representing the choice to participate in collaborative interactions in a multi-agent system. It needs the answer to enable the posterior course of actions. A predefined protocol of interaction does not exist to control the communication between agents. Rather, the interchanged objects of communication are codified like scripts that represent goals or actions coded within the agents’ neurons. The receiving agent perceives the message, its type and intensity. The latter is determined by the level of authority of the emitting creature. If it is high and the goal or action has greater priority than the current one, then the agent commits to it. 2.6 LearningThe creatures are equipped with a source that emits information arriving to the receiver by means of its sensors. In the case of unknown source, this information is related to its source by means of neurons. The receiver has the capability to remember it. Also he can find some features that make the received information unique. This information represents an object allowing its recognition. For example, in the case of a place, if the creature does not have initial knowledge of the location of the other places, he begins moving from a starting point being the first that he relates, and then according to the movements (left, right, up, down, etc.) he makes the distinctions between the places along the trajectory. This way, a mental map is generated, that makes easier the future displacements in the environment.The actions are based both on the initial knowledge and that acquired by means of the reinforcement learning that occurs by changes in the brain at the layer that perceives creature’s action effects in response to the existence of a chemical award or punishment. These stimuli serve as exciters and inhibitors of the neurons representing actions. Learning enables a creature to show a certain level of autonomy, i.e. his behavior is not only based on some basic rules, usually called instincts, but as well he acquires knowledge from the environment’s state and make decisions based priority rules to fulfill his goals. This way an adaptation to the environment occurs.Interaction Modeling with Artificial Life Agents 5 3 Case Study: Exploring a Distant PlanetIn this section, an AL application is described that has served to implement the defined concepts. The environment represents an unknown planet being explored by the creatures. This environment has been designed considering the static objects [4] (plants, water, food), dynamic objects (objects that can move from one place to another), and classes of creatures. Creatures are designed and implemented with different types of behavior and capabilities of learning and coexistence, modeled over the basic genetic and reactive attributes [9]. All these elements can be configured through the application’s GUI and be manipulated by the user during the execution.It is a primitive society that is self-controlled by the agreements generated from the interaction between the agents. The design of the creatures involved the definition of a set of actions, goals and rules, focused to set the behavior to be acquired under the scheme of social interaction being studied.3.1 CreaturesTwo types of creatures exist in the environment: native and explorer. They try to adapt, survive and coexist taking into consideration the group goals being modified during the system execution. Both types of agents are governed by the behavior of a goal-based agent; in addition to being autonomous, they can realize individual and collective actions (Table 1). Initial knowledge elements that the creatures have are defined and represented for the case of the explorer agents in Table 2.Table 1. Creatures actions, goal-states and behavior rulesActions Goal-States BehaviorrulesPay attention Hungry If there are a lot of creatures ->escape Communicate Tired If find elements ->informExpress necessities Collecting If has pain->runLearn With many creatures If it is hot ->exploreTurn Angered If communicate and find elements->collect food Fight Exploring If locked up or lost->exploreExplore Found food If on the ship ->exploreEscape Informing If has elements and on the ship->leaveTable 2. Examples of explorer creatures’ initial knowledge elementsEnvironment Creature itself Creature’s goalsElevators can contain creatures and what they are carrying Can carry and transportelementsI must not dieButtons are in machines, doors and elevators Needs water My goal is to collectfood (Group goal)Food can be found on the trees, it is ingestible, it is an element Can die if the food, water and health levels are lowThere are food and fruits I am a creatureThe explorer type of creatures begins exploration by interacting with different objects that he finds, like elevators, button, doors, food, etc. moving through the scenes in which his operation has been designed. The second type is the natives representing the wild creatures. The latter ones have aggressive behavior and increase their anger during coexistence and interaction with those who are not of their species.3.2 The environmentThere are a number of objects on the planet, with which the creatures can interact in several forms. Some objects are automated, like for example the elevators that can move up and down when the corresponding buttons (within the cabin and an outer one) are pressed by the creatures. The user can interact both with the objects by moving, gathering and dropping them in the environment, and with the creatures granting awards or assigning punishments to them.3.3 Scenarios of interactionIn the first scene, an explorer agent finds “rucksack” objects that indicate him to play a collector role. This is done by activating the neuron that indicates that an agent has a rucksack, and a new goal is settled, which can be fulfilled by executing the actions “collect elements”. Since then, he begins to explore the other scenes with a new goal added to the initial one “survive” (always of highest priority). If another creature is found playing the same role, he communicates with him trying to collaborate in order to fulfill a group goal, dividing the work (collect-food) as shown in Fig 1.Fig. 1. Explorer creatures playing a collecting role and trying to get the elements.In the second scene, the creatures learn and communicate the places and locations of the food to the others. The location layer of their brain allows them to relate a scene to an object, creature or scene maintaining therefore a mental map with theInteraction Modeling with Artificial Life Agents 7 visited places, activating the lobes of their memory to learn and to remember. When the explorer begins to collect elements, a stimulus is sent to the brain that allows finding the food. If he travels to another scene and finds another creature of his type and playing the same collector role, he communicates towards what direction he has to move to find more food (walk-towards-direction) (Fig 2).Fig. 2. An explorer group collecting and storing elementsThe third scene is the home of the native creatures where elements like food, water and toys are stored. Here the interaction between groups of two different classes of creatures can be explored. When creatures of different classes meet, they try to communicate with the creatures of their species. The natives show their aggressiveness, attacking if they are more (Fig.3), or flee away in the case of numerical inferiority. Being attacked, the explorer creatures can ask for help or inform the others not to approach this place.Fig. 3. Explorer attacked by native creatures3.4 Implementation detailsFor the environment generation the following tools were used: Creatures Map Editor 1.08 for the world edition, gimp to develop .bmp images, and sprite builder to convert them into the frame format. Creatures or agents living in the virtual world are composed of images, scripts and genome. Since our goal was not to develop a new world for entertainment purposes but to study social interactions between agents, we have used already developed creature images obtained from Creatures 3. To develop creatures’ behaviour, scripts were modified by adding new actions and behaviour rules described above. For implementation, CAOS (Creatures Agent Object Script) 1.002 and Creatures Engine 2.286, which enable the execution of our world in Creature 3 and Docking-Station were used. The creatures’ brains (neural network) and their biological structure defined by the genome (.gen) were generated using Genetics kit for Creatures 3 1.16.4 enabling adding initial knowledge, states, goals and actions.4 ConclusionsIn this paper, a set of elements that we considered important for applications of artificial life through the Cyberlife technology integrated with autonomous agents are developed through a hybrid architecture consisting of the reactive and deliberative layers, each based on behavior rules and goals respectively.The elements that appear in this work are focused on the three main aspects of interaction: (i) between agents of the environment, (ii) between agents and objects of the environment and (iii) of agents with their internal structure. Agents must have initial knowledge to represent objects of the environment. Goal-states serve to direct the individual and social behavior of agents within the environment. Behavior rules allow the agents to behave on the basis of their individual and goal states. Actions represent the means by which agents reach their group goals and interact with others by means of communication actions. Actions permit to obtain their group goals considering the priorities for each goal within the genome of the agent, which is possible to manipulate during the design and execution. The necessity to establish communication and learning that influence the interactive processes of agents and that represents the key to obtain more advanced forms of interaction in this class of artificial environments is argued.The results obtained during the tests indicate that the Cyberlife technology is an excellent base to develop AL systems that in combination with the construction of autonomous agents based on the proposed elements allows obtaining interesting cooperative behaviors. With this type of environments, it is possible to develop collaborative agents based on their goals, agents that can adapt and learn on run time, agents that can compete for resources and agents who develop their capabilities to negotiate on the basis of their internal states and the group goals.Different experimentation schemes to study interaction models in more details are under development. We are also analyzing now the possibility to use different aspects of this technology in the system of information security.Interaction Modeling with Artificial Life Agents 9 AcknowledgementsThe authors want to thank Rogelio Mendoza-Avellaneda for his contributions in the implementation of the AL environment. Special thanks to the School of Computing of the Mexican National Technical Institute for the support of this work. Partial support for this research work has also been provided by the IMP, project D.00006 “Distributed Intelligent Computing”.References[1] Bates, J. The Role of Emotion in Believable Characters, Communications of the ACM,37(7), 1994.[2] Brooks, R. A. Challenges for Complete Creature Architectures, Proc. of the FirstInternational Conference on Simulation of Adaptive Behavior, Paris, France, 1990, pp. 434–443.[3] Ferber, J. Multi-Agent Systems, Editorial Addison-Wesley, E.U.A, pag. 8-15. 1999[4] Fuhrer, P. and Pasquier-Rocha, J. Massively Distributed Virtual Worlds: a FormalApproach, Department of Informatics, Internal Working Paper 03-14, University of Fribourg, Suitzerland, 2003.[5] Grand, S., Cliff, D. and A. Malhotra. Creatures: Artificial Life Autonomous SoftwareAgents for Home Entertainment, Proc. of the First International Conference on Autonomous Agents, Marina del Rey, California, USA, 1997, pp. 22-29.[6] Grand, S. and Cliff, D. Creatures: Entertainment Software Agents with Artificial Life,Journal of Artificial Life, Autonomous Agents and Multi-Agent Systems, 1(1): 39 - 57, 1998.[7] Maes, P. Agents that Reduce Work and Information Overload, Communications of theACM, 37(7), 1994.[8] Maes, P. Modeling Adaptive Autonomous Agents, Journal of Artificial Life, MIT Press, 1(1-2): 135-162, 1994.[9] Odell, J., Parunak, H. Fleischer, M and Brueckner, S. Modeling Agents and theirEnvironment. In Proceedings of the AOSE 2002, Bologna, Italy, 2002.[10] Official site Creature Labs. /[11] Rao, A. and Georgeff, M. P. Autonomous Agents. Department of Computer Science,North Carolina State University, Raleigh, NC 27695-7534, USA. Modeling rational agents within a BDI-architecture Tech. Rep. 14, Australian Artificial Intelligence Institute, Melbourne, Australia, 1991.[12] Singh, M. P. Sinthesizing Coordination Requirements for Heterogeneous AutonomousAgents. Department of Computer Science, North Carolina State University, Raleigh, NC 27695-7534, USA.。