Nonmonotonic Probabilistic Logics between Model-Theoretic Probabilistic Logic and Probabili
Expressiveness vs. complexity in nonmonotonic knowledge bases propositional case
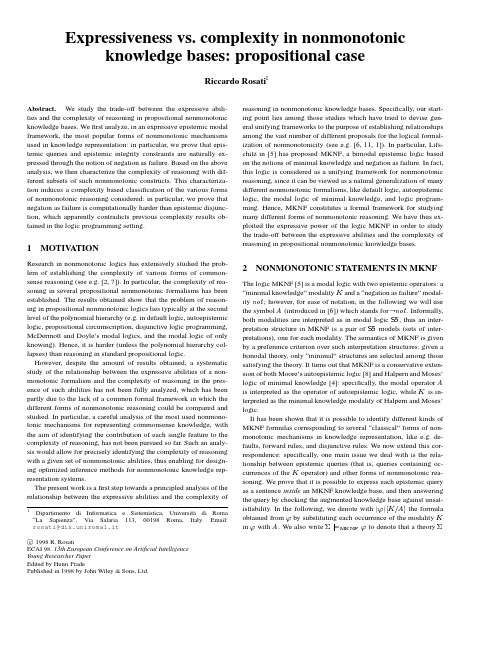
Expressiveness plexity in nonmonotonic knowledge bases:propositional caseRiccardo RosatiAbstract.We study the trade-off between the expressive abili-ties and the complexity of reasoning in propositional nonmonotonicknowledge bases.Wefirst analyze,in an expressive epistemic modalframework,the most popular forms of nonmonotonic mechanismsused in knowledge representation:in particular,we prove that epis-temic queries and epistemic integrity constraints are naturally ex-pressed through the notion of negation as failure.Based on the aboveanalysis,we then characterize the complexity of reasoning with dif-ferent subsets of such nonmonotonic constructs.This characteriza-tion induces a complexity based classification of the various formsof nonmonotonic reasoning considered:in particular,we prove thatnegation as failure is computationally harder than epistemic disjunc-tion,which apparently contradicts previous complexity results ob-tained in the logic programming setting.1MOTIV ATIONResearch in nonmonotonic logics has extensively studied the prob-lem of establishing the complexity of various forms of common-sense reasoning(see e.g.[2,7]).In particular,the complexity of rea-soning in several propositional nonmonotonic formalisms has beenestablished.The results obtained show that the problem of reason-ing in propositional nonmonotonic logics lies typically at the secondlevel of the polynomial hierarchy(e.g.in default logic,autoepistemiclogic,propositional circumscription,disjunctive logic programming,McDermott and Doyle’s modal logics,and the modal logic of onlyknowing).Hence,it is harder(unless the polynomial hierarchy col-lapses)than reasoning in standard propositional logic.However,despite the amount of results obtained,a systematicstudy of the relationship between the expressive abilities of a non-monotonic formalism and the complexity of reasoning in the pres-ence of such abilities has not been fully analyzed,which has beenpartly due to the lack of a common formal framework in which thedifferent forms of nonmonotonic reasoning could be compared andstudied.In particular,a careful analysis of the most used nonmono-tonic mechanisms for representing commonsense knowledge,withthe aim of identifying the contribution of each single feature to thecomplexity of reasoning,has not been pursued so far.Such an analy-sis would allow for precisely identifying the complexity of reasoningwith a given set of nonmonotonic abilities,thus enabling for design-ing optimized inference methods for nonmonotonic knowledge rep-resentation systems.The present work is afirst step towards a principled analysis of therelationship between the expressive abilities and the complexity oflogically implies a formula in MKNF.Theorem1Let be an MKNF knowledge base,and be an epis-temic query.Then,iff the theoryis unsatisfiable in MKNF.The most important consequence of the above theorem is that epis-temic queries are a form of nonmonotonic reasoning which can be reconducted to the notion of negation as failure(since they can be ex-pressed by modal sentences using only the operator).This allows for a better classification of nonmonotonic abilities,since all reason-ing tasks involving epistemic queries actually correspond to using the negation as failure ability.Another consequence of the above theo-rem is that in MKNF it is possible to reduce all the main reasoning problems to satisfiability/unsatisfiability.Moreover,we prove that integrity constraints,under the epistemic interpretation of[9],can actually be expressed as MKNF sentences inside the knowledge base.Roughly speaking,under this interpreta-tion,an integrity constraint is not analogous to a usual piece of information in the knowledge base,which causes inconsistency of iff contradicts other information in:it is a property which must hold in in order to preserve consistency of.Theorem2Any integrity constraint in MKNF is equivalent to a sub-jective-formula.2As in the case of epistemic queries,this result shows that integrity constraints are naturally expressed through the notion of negation as failure.3COMPLEXITY RESULTSThe results above presented,together with previous studies on the expressive abilities of MKNF,allow for expressing all the best known nonmonotonic mechanisms used in knowledge representa-tion(like rules,defaults,negation as failure(NAF),epistemic dis-junction(ED),epistemic queries(EQs),integrity constraints(ICs)) inside MKNF.We are now thus able to characterize the complexity of reasoning with different subsets of such nonmonotonic features. In particular,by exploiting previous results on the computational as-pects of MKNF[10],we establish the complexity of the unsatisfia-bility problem for MKNF knowledge bases under various syntactic restrictions,corresponding to allowing different kinds of nonmono-tonic features inside the knowledge base.LPfacts(objective knowledge)PcoNPfacts and NAF(ICs,EQs)Prules with ED coNPWe call subjective-formula a formula such that each non-modal sub-formula from lies within the scope of an operator.A summary of the complexity results obtained is reported in the second column of the displayed table,3which must be read as fol-lows:for each row of the table,the complexity of checking unsatis-fiability in MKNF under the syntactic restriction reported in thefirst column(i.e.,considering only formulas whose structure corresponds to the nonmonotonic mechanisms reported)is complete with respect to the complexity class appearing in the second column.The new complexity results appear in the third and thefifth row of the table, while the others were already known.However,the classification of epistemic queries(and integrity constraints)as a form of negation as failure allows for a new reading of the computational properties of several nonmonotonic formalisms.As an example,it is worthwhile to compare the results obtained in MKNF with the results obtained in the case of propositional logic programs,which are reported in the third column of the displayed table.It is known that logic programs (interpreted under the stable model semantics)correspond to MKNF theories of a special form,in which:(i)only atoms(or literals)are allowed within the scope of modalities;(ii)nested occurrences of the modalities are not allowed.Interestingly,under our classification (i.e.separating standard queries from epistemic queries,which are expressed in terms of negation as failure)it turns out that reasoning in logic programs with disjunction in the head of the clauses is eas-ier than reasoning in logic programs with negation as failure in the body of the clauses.This result only apparently contradicts previous complexity analyses of logic programming[3],which establish that reasoning with positive disjunctive logic programs lies at the second level of the polynomial hierarchy,since in such analyses the contri-bution of negation as failure given by queries was not considered. REFERENCES[1] A.Bochman.Biconsequence relations for nonmonotonic rea-soning.In Proc.of KR-96,482–492,1996.[2]M.Cadoli and M.Schaerf.A survey of complexity results fornonmonotonic logics.JLP,17,127–160,1993.[3]T.Eiter and G.Gottlob.On the computational cost of disjunc-tive logic programming.AMAI,15(3,4),1995.[4]J.Y.Halpern and Y.Moses.Towards a theory of knowledge andignorance:Preliminary report.Technical Report CD-TR92/34, IBM,1985.[5]V.Lifschitz.Nonmonotonic databases and epistemic queries.In Proc.of IJCAI-91,381–386,1991.[6] F.Lin and Y.Shoham.A logic of knowledge and justified as-sumptions.AIJ,57:271–289,1992[7]W.Marek and M.Truszczy´n ski.Nonmonotonic Logics–Context-Dependent Reasoning.Springer-Verlag,1993.[8]R.C.Moore.Semantical considerations on nonmonotoniclogic.AIJ,25:75–94,1985.[9]R.Reiter.What should a database know?JLP14,1990.[10]R.Rosati.Reasoning with minimal belief and negation as fail-ure:algorithms and complexity.In AAAI-97,430–435,1997.[11]G.Schwarz and M.Truszczy´n ski.Minimal knowledge prob-lem:a new approach.AIJ,67:113–141,1994.。
关于逻辑与悖论的英语作文
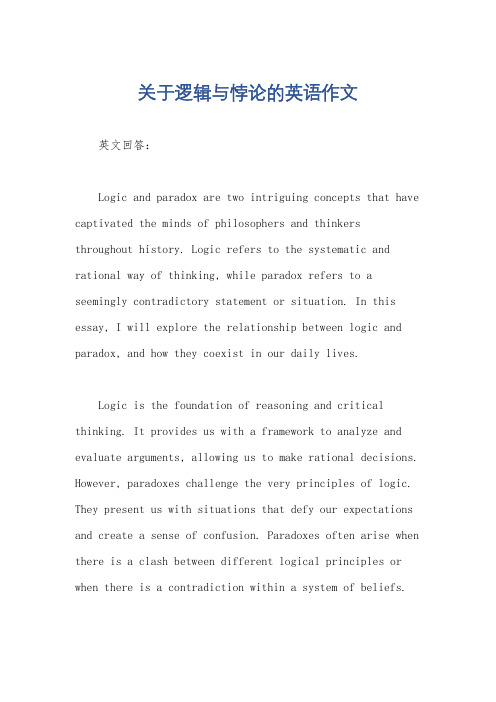
关于逻辑与悖论的英语作文英文回答:Logic and paradox are two intriguing concepts that have captivated the minds of philosophers and thinkers throughout history. Logic refers to the systematic and rational way of thinking, while paradox refers to a seemingly contradictory statement or situation. In this essay, I will explore the relationship between logic and paradox, and how they coexist in our daily lives.Logic is the foundation of reasoning and critical thinking. It provides us with a framework to analyze and evaluate arguments, allowing us to make rational decisions. However, paradoxes challenge the very principles of logic. They present us with situations that defy our expectations and create a sense of confusion. Paradoxes often arise when there is a clash between different logical principles or when there is a contradiction within a system of beliefs.One famous paradox is the "liar paradox," which states: "This statement is false." If the statement is true, thenit must be false, but if it is false, then it must be true. This paradox creates a never-ending loop of contradictions, leaving us puzzled and questioning the very nature of truth and falsehood.Another example is the "grandfather paradox" in time travel. If someone were to go back in time and preventtheir own birth, then how could they have existed in the first place to go back in time? This paradox challenges the logical concept of cause and effect, as it creates a loop of events that cannot be logically explained.Paradoxes can also be found in everyday life. For instance, the "Sorites paradox" raises the question of when a heap of sand becomes a non-heap. If we remove one grain of sand from a heap, it is still a heap. But if we keep removing grains one by one, at what point does it cease to be a heap? This paradox challenges our understanding of boundaries and categories, showing that they are not always clear-cut.In conclusion, logic and paradox are intertwined in a complex relationship. While logic provides us with a framework for rational thinking, paradoxes challenge our logical principles and force us to question our assumptions. Paradoxes exist in various forms, from logical puzzles to everyday situations. They remind us that the world is fullof uncertainties and contradictions, and that our logical reasoning may sometimes fall short in explaining them.中文回答:逻辑与悖论是两个引人入胜的概念,它们在历史上一直吸引着哲学家和思想家的思考。
Probabilistic model checking of an anonymity system
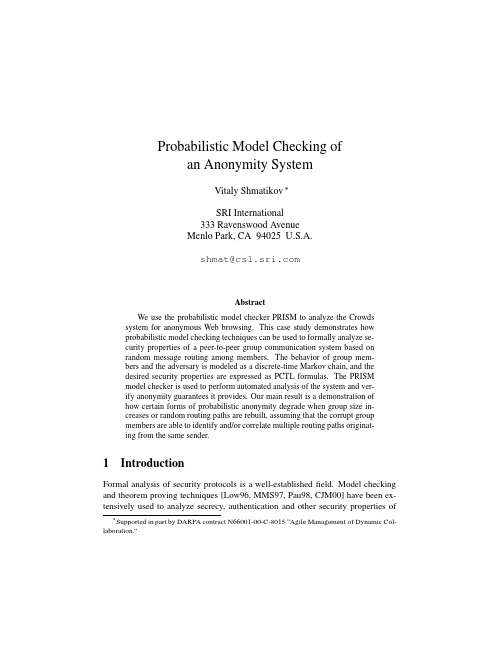
Probabilistic Model Checking ofan Anonymity SystemVitaly ShmatikovSRI International333Ravenswood AvenueMenlo Park,CA94025U.S.A.shmat@AbstractWe use the probabilistic model checker PRISM to analyze the Crowds system for anonymous Web browsing.This case study demonstrates howprobabilistic model checking techniques can be used to formally analyze se-curity properties of a peer-to-peer group communication system based onrandom message routing among members.The behavior of group mem-bers and the adversary is modeled as a discrete-time Markov chain,and thedesired security properties are expressed as PCTL formulas.The PRISMmodel checker is used to perform automated analysis of the system and ver-ify anonymity guarantees it provides.Our main result is a demonstration ofhow certain forms of probabilistic anonymity degrade when group size in-creases or random routing paths are rebuilt,assuming that the corrupt groupmembers are able to identify and/or correlate multiple routing paths originat-ing from the same sender.1IntroductionFormal analysis of security protocols is a well-establishedfield.Model checking and theorem proving techniques[Low96,MMS97,Pau98,CJM00]have been ex-tensively used to analyze secrecy,authentication and other security properties ofprotocols and systems that employ cryptographic primitives such as public-key en-cryption,digital signatures,etc.Typically,the protocol is modeled at a highly ab-stract level and the underlying cryptographic primitives are treated as secure“black boxes”to simplify the model.This approach discovers attacks that would succeed even if all cryptographic functions were perfectly secure.Conventional formal analysis of security is mainly concerned with security against the so called Dolev-Yao attacks,following[DY83].A Dolev-Yao attacker is a non-deterministic process that has complete control over the communication net-work and can perform any combination of a given set of attacker operations,such as intercepting any message,splitting messages into parts,decrypting if it knows the correct decryption key,assembling fragments of messages into new messages and replaying them out of context,etc.Many proposed systems for anonymous communication aim to provide strong, non-probabilistic anonymity guarantees.This includes proxy-based approaches to anonymity such as the Anonymizer[Ano],which hide the sender’s identity for each message by forwarding all communication through a special server,and MIX-based anonymity systems[Cha81]that blend communication between dif-ferent senders and recipients,thus preventing a global eavesdropper from linking sender-recipient pairs.Non-probabilistic anonymity systems are amenable to for-mal analysis in the same non-deterministic Dolev-Yao model as used for verifica-tion of secrecy and authentication protocols.Existing techniques for the formal analysis of anonymity in the non-deterministic model include traditional process formalisms such as CSP[SS96]and a special-purpose logic of knowledge[SS99].In this paper,we use probabilistic model checking to analyze anonymity prop-erties of a gossip-based system.Such systems fundamentally rely on probabilistic message routing to guarantee anonymity.The main representative of this class of anonymity systems is Crowds[RR98].Instead of protecting the user’s identity against a global eavesdropper,Crowds provides protection against collaborating local eavesdroppers.All communication is routed randomly through a group of peers,so that even if some of the group members collaborate and share collected lo-cal information with the adversary,the latter is not likely to distinguish true senders of the observed messages from randomly selected forwarders.Conventional formal analysis techniques that assume a non-deterministic at-tacker in full control of the communication channels are not applicable in this case. Security properties of gossip-based systems depend solely on the probabilistic be-havior of protocol participants,and can be formally expressed only in terms of relative probabilities of certain observations by the adversary.The system must be modeled as a probabilistic process in order to capture its properties faithfully.Using the analysis technique developed in this paper—namely,formalization of the system as a discrete-time Markov chain and probabilistic model checking of2this chain with PRISM—we uncovered two subtle properties of Crowds that causedegradation of the level of anonymity provided by the system to the users.First,if corrupt group members are able to detect that messages along different routingpaths originate from the same(unknown)sender,the probability of identifyingthat sender increases as the number of observed paths grows(the number of pathsmust grow with time since paths are rebuilt when crowd membership changes).Second,the confidence of the corrupt members that they detected the correct senderincreases with the size of the group.Thefirstflaw was reported independently byMalkhi[Mal01]and Wright et al.[W ALS02],while the second,to the best ofour knowledge,was reported for thefirst time in the conference version of thispaper[Shm02].In contrast to the analysis by Wright et al.that relies on manualprobability calculations,we discovered both potential vulnerabilities of Crowds byautomated probabilistic model checking.Previous research on probabilistic formal models for security focused on(i)probabilistic characterization of non-interference[Gra92,SG95,VS98],and(ii)process formalisms that aim to faithfully model probabilistic properties of crypto-graphic primitives[LMMS99,Can00].This paper attempts to directly model andanalyze security properties based on discrete probabilities,as opposed to asymp-totic probabilities in the conventional cryptographic sense.Our analysis methodis applicable to other probabilistic anonymity systems such as Freenet[CSWH01]and onion routing[SGR97].Note that the potential vulnerabilities we discovered inthe formal model of Crowds may not manifest themselves in the implementationsof Crowds or other,similar systems that take measures to prevent corrupt routersfrom correlating multiple paths originating from the same sender.2Markov Chain Model CheckingWe model the probabilistic behavior of a peer-to-peer communication system as adiscrete-time Markov chain(DTMC),which is a standard approach in probabilisticverification[LS82,HS84,Var85,HJ94].Formally,a Markov chain can be definedas consisting in afinite set of states,the initial state,the transition relation such that,and a labeling functionfrom states to afinite set of propositions.In our model,the states of the Markov chain will represent different stages ofrouting path construction.As usual,a state is defined by the values of all systemvariables.For each state,the corresponding row of the transition matrix de-fines the probability distributions which govern the behavior of group members once the system reaches that state.32.1Overview of PCTLWe use the temporal probabilistic logic PCTL[HJ94]to formally specify properties of the system to be checked.PCTL can express properties of the form“under any scheduling of processes,the probability that event occurs is at least.”First,define state formulas inductively as follows:where atomic propositions are predicates over state variables.State formulas of the form are explained below.Define path formulas as follows:Unlike state formulas,which are simplyfirst-order propositions over a single state,path formulas represent properties of a chain of states(here path refers to a sequence of state space transitions rather than a routing path in the Crowds speci-fication).In particular,is true iff is true for every state in the chain;is true iff is true for all states in the chain until becomes true,and is true for all subsequent states;is true iff and there are no more than states before becomes true.For any state and path formula,is a state formula which is true iff state space paths starting from satisfy path formula with probability greater than.For the purposes of this paper,we will be interested in formulas of the form ,evaluated in the initial state.Here specifies a system con-figuration of interest,typically representing a particular observation by the adver-sary that satisfies the definition of a successful attack on the protocol.Property is a liveness property:it holds in iff will eventually hold with greater than probability.For instance,if is a state variable represent-ing the number of times one of the corrupt members received a message from the honest member no.,then holds in iff the prob-ability of corrupt members eventually observing member no.twice or more is greater than.Expressing properties of the system in PCTL allows us to reason formally about the probability of corrupt group members collecting enough evidence to success-fully attack anonymity.We use model checking techniques developed for verifica-tion of discrete-time Markov chains to compute this probability automatically.42.2PRISM model checkerThe automated analyses described in this paper were performed using PRISM,aprobabilistic model checker developed by Kwiatkowska et al.[KNP01].The toolsupports both discrete-and continuous-time Markov chains,and Markov decisionprocesses.As described in section4,we model probabilistic peer-to-peer com-munication systems such as Crowds simply as discrete-time Markov chains,andformalize their properties in PCTL.The behavior of the system processes is specified using a simple module-basedlanguage inspired by Reactive Modules[AH96].State variables are declared in thestandard way.For example,the following declarationdeliver:bool init false;declares a boolean state variable deliver,initialized to false,while the followingdeclarationconst TotalRuns=4;...observe1:[0..TotalRuns]init0;declares a constant TotalRuns equal to,and then an integer array of size,indexed from to TotalRuns,with all elements initialized to.State transition rules are specified using guarded commands of the form[]<guard>-><command>;where<guard>is a predicate over system variables,and<command>is the tran-sition executed by the system if the guard condition evaluates to mandoften has the form<expression>...<expression>, which means that in the next state(i.e.,that obtained after the transition has beenexecuted),state variable is assigned the result of evaluating arithmetic expres-sion<expression>If the transition must be chosen probabilistically,the discrete probability dis-tribution is specified as[]<guard>-><prob1>:<command1>+...+<probN>:<commandN>;Transition represented by command is executed with probability prob,and prob.Security properties to be checked are stated as PCTL formulas (see section2.1).5Given a formal system specification,PRISM constructs the Markov chain and determines the set of reachable states,using MTBDDs and BDDs,respectively. Model checking a PCTL formula reduces to a combination of reachability-based computation and solving a system of linear equations to determine the probability of satisfying the formula in each reachable state.The model checking algorithms employed by PRISM include[BdA95,BK98,Bai98].More details about the im-plementation and operation of PRISM can be found at http://www.cs.bham. /˜dxp/prism/and in[KNP01].Since PRISM only supports model checking offinite DTMC,in our case study of Crowds we only analyze anonymity properties offinite instances of the system. By changing parameters of the model,we demonstrate how anonymity properties evolve with changes in the system configuration.Wright et al.[W ALS02]investi-gated related properties of the Crowds system in the general case,but they do not rely on tool support and their analyses are manual rather than automated.3Crowds Anonymity SystemProviding an anonymous communication service on the Internet is a challenging task.While conventional security mechanisms such as encryption can be used to protect the content of messages and transactions,eavesdroppers can still observe the IP addresses of communicating computers,timing and frequency of communi-cation,etc.A Web server can trace the source of the incoming connection,further compromising anonymity.The Crowds system was developed by Reiter and Ru-bin[RR98]for protecting users’anonymity on the Web.The main idea behind gossip-based approaches to anonymity such as Crowds is to hide each user’s communications by routing them randomly within a crowd of similar users.Even if an eavesdropper observes a message being sent by a particular user,it can never be sure whether the user is the actual sender,or is simply routing another user’s message.3.1Path setup protocolA crowd is a collection of users,each of whom is running a special process called a jondo which acts as the user’s proxy.Some of the jondos may be corrupt and/or controlled by the adversary.Corrupt jondos may collaborate and share their obser-vations in an attempt to compromise the honest users’anonymity.Note,however, that all observations by corrupt group members are local.Each corrupt member may observe messages sent to it,but not messages transmitted on the links be-tween honest jondos.An honest crowd member has no way of determining whether6a particular jondo is honest or corrupt.The parameters of the system are the total number of members,the number of corrupt members,and the forwarding probability which is explained below.To participate in communication,all jondos must register with a special server which maintains membership information.Therefore,every member of the crowd knows identities of all other members.As part of the join procedure,the members establish pairwise encryption keys which are used to encrypt pairwise communi-cation,so the contents of the messages are secret from an external eavesdropper.Anonymity guarantees provided by Crowds are based on the path setup pro-tocol,which is described in the rest of this section.The path setup protocol is executed each time one of the crowd members wants to establish an anonymous connection to a Web server.Once a routing path through the crowd is established, all subsequent communication between the member and the Web server is routed along it.We will call one run of the path setup protocol a session.When crowd membership changes,the existing paths must be scrapped and a new protocol ses-sion must be executed in order to create a new random routing path through the crowd to the destination.Therefore,we’ll use terms path reformulation and proto-col session interchangeably.When a user wants to establish a connection with a Web server,its browser sends a request to the jondo running locally on her computer(we will call this jondo the initiator).Each request contains information about the intended desti-nation.Since the objective of Crowds is to protect the sender’s identity,it is not problematic that a corrupt router can learn the recipient’s identity.The initiator starts the process of creating a random path to the destination as follows: The initiator selects a crowd member at random(possibly itself),and for-wards the request to it,encrypted by the corresponding pairwise key.We’ll call the selected member the forwarder.The forwarderflips a biased coin.With probability,it delivers the request directly to the destination.With probability,it selects a crowd member at random(possibly itself)as the next forwarder in the path,and forwards the request to it,re-encrypted with the appropriate pairwise key.The next forwarder then repeats this step.Each forwarder maintains an identifier for the created path.If the same jondo appears in different positions on the same path,identifiers are different to avoid infinite loops.Each subsequent message from the initiator to the destination is routed along this path,i.e.,the paths are static—once established,they are not altered often.This is necessary to hinder corrupt members from linking multiple7paths originating from the same initiator,and using this information to compromise the initiator’s anonymity as described in section3.2.3.3.2Anonymity properties of CrowdsThe Crowds paper[RR98]describes several degrees of anonymity that may be provided by a communication system.Without using anonymizing techniques, none of the following properties are guaranteed on the Web since browser requests contain information about their source and destination in the clear.Beyond suspicion Even if the adversary can see evidence of a sent message,the real sender appears to be no more likely to have originated it than any other potential sender in the system.Probable innocence The real sender appears no more likely to be the originator of the message than to not be the originator,i.e.,the probability that the adversary observes the real sender as the source of the message is less thanupper bound on the probability of detection.If the sender is observed by the adversary,she can then plausibly argue that she has been routing someone else’s messages.The Crowds paper focuses on providing anonymity against local,possibly co-operating eavesdroppers,who can share their observations of communication in which they are involved as forwarders,but cannot observe communication involv-ing only honest members.We also limit our analysis to this case.3.2.1Anonymity for a single routeIt is proved in[RR98]that,for any given routing path,the path initiator in a crowd of members with forwarding probability has probable innocence against collaborating crowd members if the following inequality holds:(1)More formally,let be the event that at least one of the corrupt crowd members is selected for the path,and be the event that the path initiator appears in8the path immediately before a corrupt crowd member(i.e.,the adversary observes the real sender as the source of the messages routed along the path).Condition 1guarantees thatproving that,given multiple linked paths,the initiator appears more often as a sus-pect than a random crowd member.The automated analysis described in section6.1 confirms and quantifies this result.(The technical results of[Shm02]on which this paper is based had been developed independently of[Mal01]and[W ALS02],be-fore the latter was published).In general,[Mal01]and[W ALS02]conjecture that there can be no reliable anonymity method for peer-to-peer communication if in order to start a new communication session,the initiator must originate thefirst connection before any processing of the session commences.This implies that anonymity is impossible in a gossip-based system with corrupt routers in the ab-sence of decoy traffic.In section6.3,we show that,for any given number of observed paths,the adversary’s confidence in its observations increases with the size of the crowd.This result contradicts the intuitive notion that bigger crowds provide better anonymity guarantees.It was discovered by automated analysis.4Formal Model of CrowdsIn this section,we describe our probabilistic formal model of the Crowds system. Since there is no non-determinism in the protocol specification(see section3.1), the model is a simple discrete-time Markov chain as opposed to a Markov deci-sion process.In addition to modeling the behavior of the honest crowd members, we also formalize the adversary.The protocol does not aim to provide anonymity against global eavesdroppers.Therefore,it is sufficient to model the adversary as a coalition of corrupt crowd members who only have access to local communication channels,i.e.,they can only make observations about a path if one of them is se-lected as a forwarder.By the same token,it is not necessary to model cryptographic functions,since corrupt members know the keys used to encrypt peer-to-peer links in which they are one of the endpoints,and have no access to links that involve only honest members.The modeling technique presented in this section is applicable with minor mod-ifications to any probabilistic routing system.In each state of routing path construc-tion,the discrete probability distribution given by the protocol specification is used directly to define the probabilistic transition rule for choosing the next forwarder on the path,if any.If the protocol prescribes an upper bound on the length of the path(e.g.,Freenet[CSWH01]),the bound can be introduced as a system parameter as described in section4.2.3,with the corresponding increase in the size of the state space but no conceptual problems.Probabilistic model checking can then be used to check the validity of PCTL formulas representing properties of the system.In the general case,forwarder selection may be governed by non-deterministic10runCount goodbad lastSeen observelaunchnewstartrundeliver recordLast badObserve4.2Model of honest members4.2.1InitiationPath construction is initiated as follows(syntax of PRISM is described in section 2.2):[]launch->runCount’=TotalRuns&new’=true&launch’=false;[]new&(runCount>0)->(runCount’=runCount-1)&new’=false&start’=true;[]start->lastSeen’=0&deliver’=false&run’=true&start’=false;4.2.2Forwarder selectionThe initiator(i.e.,thefirst crowd member on the path,the one whose identity must be protected)randomly chooses thefirst forwarder from among all group mem-bers.We assume that all group members have an equal probability of being chosen, but the technique can support any discrete probability distribution for choosing for-warders.Forwarder selection is a single step of the protocol,but we model it as two probabilistic state transitions.Thefirst determines whether the selected forwarder is honest or corrupt,the second determines the forwarder’s identity.The randomly selected forwarder is corrupt with probability badCbe next on the path.Any of the honest crowd members can be selected as the forwarder with equal probability.To illustrate,for a crowd with10honest members,the following transition models the second step of forwarder selection: []recordLast&CrowdSize=10->0.1:lastSeen’=0&run’=true&recordLast’=false+0.1:lastSeen’=1&run’=true&recordLast’=false+...0.1:lastSeen’=9&run’=true&recordLast’=false;According to the protocol,each honest crowd member must decide whether to continue building the path byflipping a biased coin.With probability,the forwarder selection transition is enabled again and path construction continues, and with probability the path is terminated at the current forwarder,and all requests arriving from the initiator along the path will be delivered directly to the recipient.[](good&!deliver&run)->//Continue path constructionPF:good’=false+//Terminate path constructionnotPF:deliver’=true;The specification of the Crowds system imposes no upper bound on the length of the path.Moreover,the forwarders are not permitted to know their relative position on the path.Note,however,that the amount of information about the initiator that can be extracted by the adversary from any path,or anyfinite number of paths,isfinite(see sections4.3and4.5).In systems such as Freenet[CSWH01],requests have a hops-to-live counter to prevent infinite paths,except with very small probability.To model this counter,we may introduce an additional state variable pIndex that keeps track of the length of the path constructed so far.The path construction transition is then coded as follows://Example with Hops-To-Live//(NOT CROWDS)////Forward with prob.PF,else deliver13[](good&!deliver&run&pIndex<MaxPath)->PF:good’=false&pIndex’=pIndex+1+notPF:deliver’=true;//Terminate if reached MaxPath,//but sometimes not//(to confuse adversary)[](good&!deliver&run&pIndex=MaxPath)->smallP:good’=false+largeP:deliver’=true;Introduction of pIndex obviously results in exponential state space explosion, decreasing the maximum system size for which model checking is feasible.4.2.4Transition matrix for honest membersTo summarize the state space of the discrete-time Markov chain representing cor-rect behavior of protocol participants(i.e.,the state space induced by the abovetransitions),let be the state in which links of the th routing path from the initiator have already been constructed,and assume that are the honestforwarders selected for the path.Let be the state in which path constructionhas terminated with as thefinal path,and let be an auxiliary state. Then,given the set of honest crowd members s.t.,the transi-tion matrix is such that,,(see section4.2.2),i.e.,the probability of selecting the adversary is equal to the cumulative probability of selecting some corrupt member.14This abstraction does not limit the class of attacks that can be discovered using the approach proposed in this paper.Any attack found in the model where indi-vidual corrupt members are kept separate will be found in the model where their capabilities are combined in a single worst-case adversary.The reason for this is that every observation made by one of the corrupt members in the model with separate corrupt members will be made by the adversary in the model where their capabilities are combined.The amount of information available to the worst-case adversary and,consequently,the inferences that can be made from it are at least as large as those available to any individual corrupt member or a subset thereof.In the adversary model of[RR98],each corrupt member can only observe its local network.Therefore,it only learns the identity of the crowd member imme-diately preceding it on the path.We model this by having the corrupt member read the value of the lastSeen variable,and record its observations.This cor-responds to reading the source IP address of the messages arriving along the path. For example,for a crowd of size10,the transition is as follows:[]lastSeen=0&badObserve->observe0’=observe0+1&deliver’=true&run’=true&badObserve’=false;...[]lastSeen=9&badObserve->observe9’=observe9+1&deliver’=true&run’=true&badObserve’=false;The counters observe are persistent,i.e.,they are not reset for each session of the path setup protocol.This allows the adversary to accumulate observations over several path reformulations.We assume that the adversary can detect when two paths originate from the same member whose identity is unknown(see sec-tion3.2.2).The adversary is only interested in learning the identity of thefirst crowd mem-ber in the path.Continuing path construction after one of the corrupt members has been selected as a forwarder does not provide the adversary with any new infor-mation.This is a very important property since it helps keep the model of the adversaryfinite.Even though there is no bound on the length of the path,at most one observation per path is useful to the adversary.To simplify the model,we as-sume that the path terminates as soon as it reaches a corrupt member(modeled by deliver’=true in the transition above).This is done to shorten the average path length without decreasing the power of the adversary.15Each forwarder is supposed toflip a biased coin to decide whether to terminate the path,but the coinflips are local to the forwarder and cannot be observed by other members.Therefore,honest members cannot detect without cooperation that corrupt members always terminate paths.In any case,corrupt members can make their observable behavior indistinguishable from that of the honest members by continuing the path with probability as described in section4.2.3,even though this yields no additional information to the adversary.4.4Multiple pathsThe discrete-time Markov chain defined in sections4.2and4.3models construc-tion of a single path through the crowd.As explained in section3.2.2,paths have to be reformulated periodically.The decision to rebuild the path is typically made according to a pre-determined schedule,e.g.,hourly,daily,or once enough new members have asked to join the crowd.For the purposes of our analysis,we sim-ply assume that paths are reformulated somefinite number of times(determined by the system parameter=TotalRuns).We analyze anonymity properties provided by Crowds after successive path reformulations by considering the state space produced by successive execu-tions of the path construction protocol described in section4.2.As explained in section4.3,the adversary is permitted to combine its observations of some or all of the paths that have been constructed(the adversary only observes the paths for which some corrupt member was selected as one of the forwarders).The adversary may then use this information to infer the path initiator’s identity.Because for-warder selection is probabilistic,the adversary’s ability to collect enough informa-tion to successfully identify the initiator can only be characterized probabilistically, as explained in section5.4.5Finiteness of the adversary’s state spaceThe state space of the honest members defined by the transition matrix of sec-tion4.2.4is infinite since there is no a priori upper bound on the length of each path.Corrupt members,however,even if they collaborate,can make at most one observation per path,as explained in section4.3.As long as the number of path reformulations is bounded(see section4.4),only afinite number of paths will be constructed and the adversary will be able to make only afinite number of observa-tions.Therefore,the adversary only needsfinite memory and the adversary’s state space isfinite.In general,anonymity is violated if the adversary has a high probability of making a certain observation(see section5).Tofind out whether Crowds satisfies16。
关于逻辑与悖论的英语作文
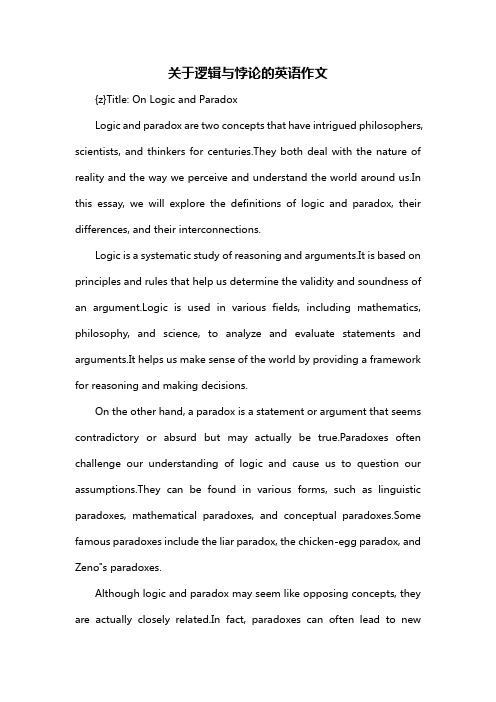
关于逻辑与悖论的英语作文{z}Title: On Logic and ParadoxLogic and paradox are two concepts that have intrigued philosophers, scientists, and thinkers for centuries.They both deal with the nature of reality and the way we perceive and understand the world around us.In this essay, we will explore the definitions of logic and paradox, their differences, and their interconnections.Logic is a systematic study of reasoning and arguments.It is based on principles and rules that help us determine the validity and soundness of an argument.Logic is used in various fields, including mathematics, philosophy, and science, to analyze and evaluate statements and arguments.It helps us make sense of the world by providing a framework for reasoning and making decisions.On the other hand, a paradox is a statement or argument that seems contradictory or absurd but may actually be true.Paradoxes often challenge our understanding of logic and cause us to question our assumptions.They can be found in various forms, such as linguistic paradoxes, mathematical paradoxes, and conceptual paradoxes.Some famous paradoxes include the liar paradox, the chicken-egg paradox, and Zeno"s paradoxes.Although logic and paradox may seem like opposing concepts, they are actually closely related.In fact, paradoxes can often lead to newinsights and advancements in logic.For example, the liar paradox led to the development of truth tables and the study of formal logic.Paradoxes challenge us to question our assumptions and think critically, which can lead to the development of new logical theories and principles.One way to understand the relationship between logic and paradox is through the concept of dialectics.Dialectics is a form of reasoning that involves arguing for and against a statement or position.It is based on the idea that truth emerges from a process of debate and argument.In dialectics, paradoxes are used as tools to challenge existing ideas and promote critical thinking.By examining paradoxes, we can uncover hidden assumptions, contradictions, and limitations in our logical reasoning.In conclusion, logic and paradox are two essential concepts that help us understand the nature of reality and the limits of our knowledge.Logic provides us with a framework for reasoning and making sense of the world, while paradoxes challenge us to question our assumptions and think critically.By examining the interconnections between logic and paradox, we can deepen our understanding of these complex and fascinating phenomena.。
Dept.ofComputerScience
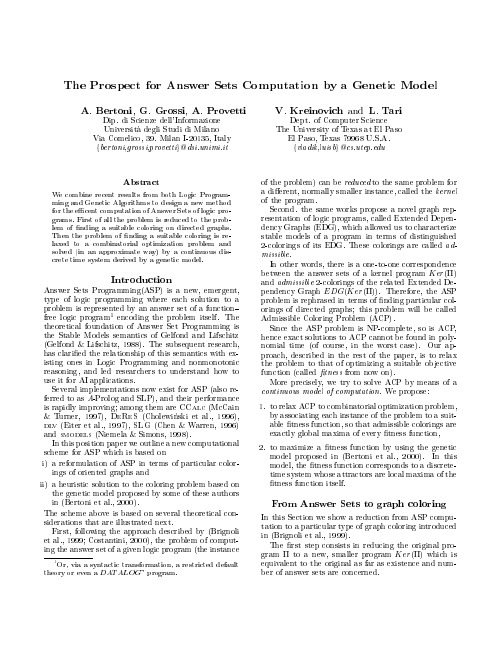
Introduction
of the problem) can be reduced to the same problem for a di erent, normally smaller instance, called the kernel of the program. Second. the same works propose a novel graph representation of logic programs, called Extended Dependency Graphs (EDG), which allowed us to characterize stable models of a program in terms of distinguished 2-colorings of its EDG. These colorings are called admissible. In other words, there is a one-to-one correspondence between the answer sets of a kernel program Ker( ) and admissible 2-colorings of the related Extended Dependency Graph EDG(Ker( )). Therefore, the ASP problem is rephrased in terms of nding particular colorings of directed graphs; this problem will be called Admissible Coloring Problem (ACP). Since the ASP problem is NP-complete, so is ACP, hence exact solutions to ACP cannot be found in polynomial time (of course, in the worst case). Our approach, described in the rest of the paper, is to relax the problem to that of optimizing a suitable objective function (called tness from now on). More precisely, we try to solve ACP by means of a continuous model of computation. We propose: 1. to relax ACP to combinatorial optimization problem, by associating each instance of the problem to a suitable tness function, so that admissible colorings are exactly global maxima of every tness function, 2. to maximize a tness function by using the genetic model proposed in (Bertoni et al., 2000). In this model, the tness function corresponds to a discretetime system whose attractors are local maxima of the tness function itself. In this Section we show a reduction from ASP computation to a particular type of graph coloring introduced in (Brignoli et al., 1999). The rst step consists in reducing the original program to a new, smaller program Ker( ) which is equivalent to the original as far as existence and number of answer sets are concerned.
不确定理论及应用总结
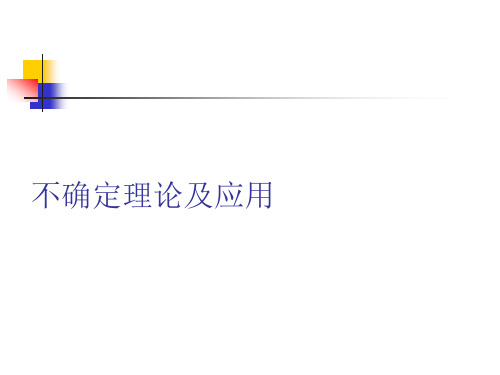
6、证据理论在中国的发展情况
段新生:在1993年出版了一本专门论述证据理论的专 著《证据理论与决策、人工智能》。【注:由于此书出版时间较
3、证据理论的核心、优点及适用领域
核心:Dempster合成规则,这是Dempster在研究 统计问题时首先提出的,随后Shafer把它推广到更为一 般的情形。 优点:由于在证据理论中需要的先验数据比概率推 理理论中的更为直观、更容易获得,再加上Dempster合 成公式可以综合不同专家或数据源的知识或数据,这使 得证据理论在专家系统、信息融合等领域中得到了广泛 应用。
Bel(2000, 4000)=0.7
Pl(1000, 2000)=0.1
Pl(2000, 3000)=0.7 Pl(3000, 4000)=1.0 Pl(4000, 5000)=0.3
Bel A
Pl A
D A
mD
mD
D A 0
Pl(2000, 4000)=1-Bel(1000, 2000) – Bel(4000, 5000) = 1 – 0 – 0 = 1
10(3): 277-282. 注:此处的“约集”即为“粗糙集”(Rough set)】
证据理论在中国的发展情况(续)
曾成等人:研究了不完备的识别框架下的证据合成 问题,并提出相应的证据合成公式。 顾伟康等人:对证据合成公式进行扩展,提出一种 改进的证据合成公式。 徐从富等人:1999-2001总结国内外关于证据理论及 其应用的代表性文献,先后发表2篇关于证据理论及其应 用的综述文章。 ……
写一篇逻辑与悖论的英语作文
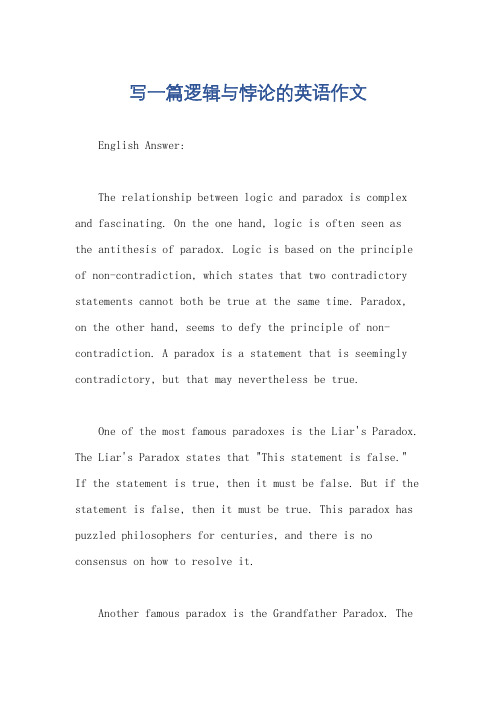
写一篇逻辑与悖论的英语作文English Answer:The relationship between logic and paradox is complex and fascinating. On the one hand, logic is often seen as the antithesis of paradox. Logic is based on the principle of non-contradiction, which states that two contradictory statements cannot both be true at the same time. Paradox, on the other hand, seems to defy the principle of non-contradiction. A paradox is a statement that is seemingly contradictory, but that may nevertheless be true.One of the most famous paradoxes is the Liar's Paradox. The Liar's Paradox states that "This statement is false."If the statement is true, then it must be false. But if the statement is false, then it must be true. This paradox has puzzled philosophers for centuries, and there is no consensus on how to resolve it.Another famous paradox is the Grandfather Paradox. TheGrandfather Paradox states that if you travel back in time and kill your grandfather before he meets your grandmother, then you will never be born. But if you are never born, then you cannot travel back in time to kill your grandfather. This paradox is also known as the "Bootstrap Paradox," because it seems to create a self-referential loop.Paradoxes can be frustrating and counterintuitive, but they can also be enlightening. Paradoxes can help us to see the world in new ways, and they can challenge our assumptions about reality. In fact, some philosophers believe that paradoxes are essential for the progress of knowledge.中文回答:逻辑与悖论之间的关系既复杂又引人入胜。
逻辑新引 判别是非 读后感
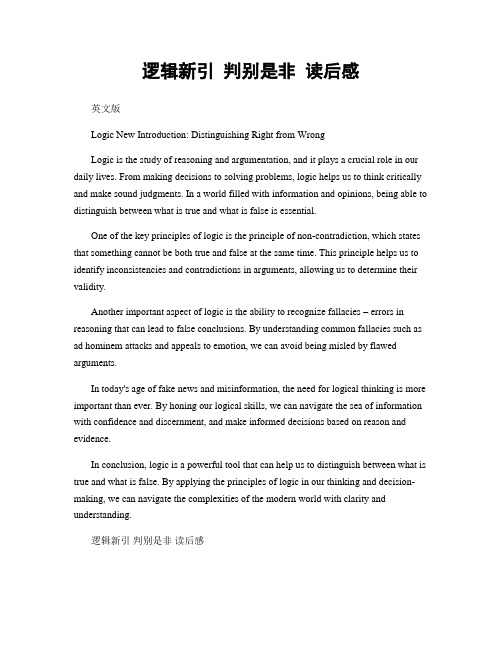
逻辑新引判别是非读后感英文版Logic New Introduction: Distinguishing Right from WrongLogic is the study of reasoning and argumentation, and it plays a crucial role in our daily lives. From making decisions to solving problems, logic helps us to think critically and make sound judgments. In a world filled with information and opinions, being able to distinguish between what is true and what is false is essential.One of the key principles of logic is the principle of non-contradiction, which states that something cannot be both true and false at the same time. This principle helps us to identify inconsistencies and contradictions in arguments, allowing us to determine their validity.Another important aspect of logic is the ability to recognize fallacies – errors in reasoning that can lead to false conclusions. By understanding common fallacies such as ad hominem attacks and appeals to emotion, we can avoid being misled by flawed arguments.In today's age of fake news and misinformation, the need for logical thinking is more important than ever. By honing our logical skills, we can navigate the sea of information with confidence and discernment, and make informed decisions based on reason and evidence.In conclusion, logic is a powerful tool that can help us to distinguish between what is true and what is false. By applying the principles of logic in our thinking and decision-making, we can navigate the complexities of the modern world with clarity and understanding.逻辑新引判别是非读后感逻辑是研究推理和论证的学科,在我们的日常生活中扮演着重要的角色。
- 1、下载文档前请自行甄别文档内容的完整性,平台不提供额外的编辑、内容补充、找答案等附加服务。
- 2、"仅部分预览"的文档,不可在线预览部分如存在完整性等问题,可反馈申请退款(可完整预览的文档不适用该条件!)。
- 3、如文档侵犯您的权益,请联系客服反馈,我们会尽快为您处理(人工客服工作时间:9:00-18:30)。
1 Introduction
In the recent decades, probabilistic reasoning has become an important research topic in artificial intelligence. In particular, extensive research has been carried out on probabilistic reasoning with interval restrictions for conditional probabilities, also called conditional constraints [40]. One important approach for handling conditional constraints is model-theoretic probabilistic logic, which comes
∗ Alternate address: Institut f¨ ur Informationssysteme, Technische Universit¨ at Wien, Favoritenstraße 9-11, 1040 Wien, Austria. E-mail: lukasiewicz@kr.tuwien.ac.at.
Thomas Lukasiewicz∗ Dipartimento di Informatica e Sistemistica, Universit` a di Roma “La Sapienza” Via Salaria 113, 00198 Roma, Italy lukasiewicz@dphilosophy and logic, and whose roots go back to Boole’s book of 1854 “The Laws of Thought” [11]. There is a wide spectrum of formal languages that have been explored in model-theoretic probabilistic logic, ranging from constraints for unconditional and conditional events [16, 20, 2, 53, 19, 23, 33, 45, 39, 40, 42, 46] to rich languages that specify linear inequalities over events [22]. The main problems related to model-theoretic probabilistic logic are checking satisfiability, deciding logical consequence, and computing tight logically entailed intervals. Another important approach to probabilistic reasoning with conditional constraints, which has been extensively explored in the area of statistics, is based on the coherence principle of de Finetti and suitable generalizations of it [8, 12, 13, 14, 15, 27, 28, 29, 50], or on similar principles that have been adopted for lower and upper probabilities [49, 54]. Two important aspects of dealing with uncertainty in this framework are (i) checking the consistency of a probabilistic assessment, and (ii) the propagation of a given assessment to further uncertain quantities. Recently, the relationship between model-theoretic probabilistic logic and probabilistic logic under coherence has been thoroughly explored in [10]. In particular, it turned out that probabilistic entailment under coherence is weaker than model-theoretic probabilistic entailment. Moreover, it has been shown in [10] that probabilistic entailment under coherence is a generalization of classical default entailment in System P , while model-theoretic probabilistic entailment is well-known to be a generalization of modeltheoretic entailment in classical propositional logics. In this paper, we continue this line of research by presenting probabilistic generalizations of more sophisticated formalisms for default reasoning from conditional knowledge bases, which lie between probabilistic entailment under coherence and model-theoretic probabilistic entailment. That is, the new notions of entailment generalize their classical counterparts, they are stronger than entailment under coherence, and weaker than model-theoretic entailment.
The literature contains several different proposals for default reasoning from conditional knowledge bases and extensive work on its desired properties. The core of these properties are the rationality postulates of System P proposed by Kraus et al. [34]. It turned out that these rationality postulates constitute a sound and complete axiom system for several classical model-theoretic entailment relations under uncertainty measures on worlds. In detail, they characterize classical model-theoretic entailment under preferential structures [51, 34], infinitesimal probabilities [1, 47], possibility measures [17], and world rankings [52, 31]. They also characterize an entailment relation based on conditional objects [18]. A survey of all these relationships is given in [6, 24]. Mainly to solve problems with irrelevant information, the notion of rational closure as a more adventurous notion of entailment was introduced by Lehmann [36, 38]. It is equivalent to entailment in System Z by Pearl [48], to the least specific possibility entailment by Benferhat et al. [5], and to a conditional (modal) logic-based entailment by Lamarre [35]. Finally, mainly to solve problems with property inheritance from classes to exceptional subclasses, the maximum entropy approach to default entailment was proposed by Goldszmidt et al. [30]; lexicographic entailment was introduced by Lehmann [37] and Benferhat et al. [4]; conditional entailment was proposed by Geffner [25, 26]; and an infinitesimal belief function approach was suggested by Benferhat et al. [7]. In this paper, we define probabilistic generalizations of entailment in Pearl’s System Z and Lehmann’s lexicographic entailment that lie between entailment in model-theoretic probabilistic logic and entailment in probabilistic logic under coherence. Roughly speaking, the main difference between model-theoretic probabilistic entailment and probabilistic entailment under coherence is that the former realizes an inheritance of logical knowledge, while the latter does not. Intuitively, the new formalisms now add a strategy for resolving inconsistencies to model-theoretic probabilistic logic, and a restricted form of inheritance of logical knowledge to probabilistic logic under coherence. This is why the new notions of entailment are weaker than entailment in model-theoretic probabilistic logic and stronger than entailment in probabilistic logic under coherence. Hence, the new formalisms are refinements of both modeltheoretic probabilistic entailment and probabilistic entailment under coherence. They can be used in place of modeltheoretic probabilistic entailment, when we want to resolve probabilistic inconsistencies related to conditioning on zero events. Here, the new formalisms are especially well-suited as they coincide with model-theoretic probabilistic entailment as long as we condition on non-zero events. Furthermore, the new formalisms can be used in place of proba-