Abstract Fuzzy Sets and Systems 118 (2001) 479–487 Cardinality concepts for type-two fuzzy
Vague集凝熵的注记

(4) 设 A = [t A 1 - f A]和 B = [t B 1 - f B], 令 mA = 1 - tA - fA, mB =
还有中立情况, 按直觉判断,A 的模糊性肯定比 B 的模糊性要 大。然而, 由上面结果可以看到 A 的模糊性比 B 的模糊性要 小, 这是不合理的。 针对上述不足, 文献[9]给出了一个改进的模糊熵计算方法去 避免文献[8]的不合理情况, 然而文献[10]也指出了文献[9]的一些 错误之处, 给出了一个新的计算方法如下: 已知一个 Vague 值 其中 0<k≤1。
集 的推广, 也是一种直觉的模糊集
一个真隶属函数 t( ) 和一个假隶属函数 f( ) 来描述其隶属度 v u v u 的边界, 这两个边界就构成[0, 1]中的一个子区间[t( ) , 1-f( ) ], v u v u 其中一个对象的支持度、 反对度和未知度分别为 t( ) 、 f( ) 和 v u v u 1-t( ) -f( ) , 这使得 Vague 集在处理不确定性信息时比传统 v u v u 的 模 糊 集 有 更 强 的 表 达 能 力 以 及 更 具 灵 活 性 [7]。 然 而 对 于 Vague 集的模糊熵的研究, 与 Fuzzy 集相比存在一定的困难, 原 因在于 Vague 集的模糊熵不仅来自 Vague 集本身的未知性, 而 且来自 Vague 集自身存在的不确定性, 如在投票模型中, 支持 者与反对者各占一半时, 人们很难做出合理判断。近年来, 虽 然已有一些学者提出 Vague 集模糊熵的概念并给出了 Vague 集模糊熵的构造方法, 但是他们对 Vague 集模糊熵的约束条件 有共识但也有分歧, 也就是 Vague 集的未知性和不确定性如何 有效合理地形成直觉客观的模糊熵还没有统一, 特别是如何 把不确定性因素转化为模糊熵的一部分, 因此 Vague 集模糊熵 也就没有一个全面严谨的界定, 其定义中的约束条件也应改 进。在文献 [8] 中给出了一个定理计算 Vague 集的模糊熵, 文 献 [9] 指出了其存在的不足之处, 并给出了 Vague 集模糊熵的
Fuzzy Systems and Control

Fuzzy Systems and Control Fuzzy systems and control are essential components of modern engineering and technology. These systems are designed to handle complex and uncertain information, making them suitable for a wide range of applications such as industrial automation, robotics, and decision-making processes. However, despite their potential, fuzzy systems and control face several challenges that need to be addressed. One of the main issues with fuzzy systems and control is their complexity. Developing and implementing these systems requires a deep understanding of fuzzy logic, control theory, and mathematical modeling. This complexity can be a barrier for engineers and researchers who are not familiarwith these concepts, limiting the widespread adoption of fuzzy systems and control in various industries. Another challenge is the lack of standardized methodologies for designing and evaluating fuzzy systems and control. Unlike traditional control systems, which have well-established design and analysis techniques, fuzzy systems and control lack a unified framework for development. This makes it difficult to compare different approaches and assess their performance, hindering the advancement of the field. Furthermore, the interpretability of fuzzy systems and control is a concern. While these systems excel at handling vague and imprecise information, their decision-making processes can be difficult to understand and interpret. This lack of transparency can leadto skepticism and reluctance to adopt fuzzy systems and control in critical applications where explainability is crucial. In addition, the integration of fuzzy systems and control with other technologies, such as machine learning and artificial intelligence, poses a significant challenge. While these fields have shown great promise in addressing complex problems, the combination of fuzzy systems and control with these technologies requires careful consideration of compatibility, scalability, and performance. Moreover, the real-time implementation of fuzzy systems and control in dynamic environments is a challenging task. These systems need to adapt to changing conditions and make decisions in a timely manner, which requires efficient algorithms and hardware support. Ensuring the reliability and robustness of fuzzy systems and control in such environments is a critical area of research and development. Despite thesechallenges, there are ongoing efforts to overcome them and advance the field of fuzzy systems and control. Researchers are working on developing simplified design methodologies and tools to facilitate the implementation of fuzzy systems and control in practical applications. Moreover, there is a growing interest in explainable artificial intelligence, which aims to enhance the interpretability of fuzzy systems and control and address the concerns related to transparency. Furthermore, advancements in hardware technology, such as the emergence of high-performance computing and edge computing, are enabling the real-time implementation of fuzzy systems and control in dynamic environments. These developments are paving the way for the integration of fuzzy systems and control with other emerging technologies, opening up new possibilities for their application in diverse fields. In conclusion, while fuzzy systems and control face several challenges, there are promising developments and research efforts aimed at addressing these issues. By overcoming the complexity, standardizing methodologies, improving interpretability, integrating with other technologies, and enhancing real-time implementation, the potential of fuzzy systems and control can be fully realized, leading to significant advancements in engineering and technology.。
PID流量控制
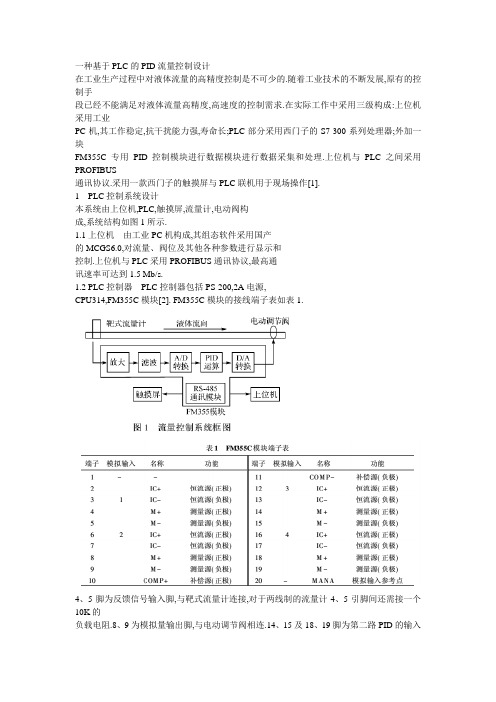
一种基于PLC的PID流量控制设计在工业生产过程中对液体流量的高精度控制是不可少的.随着工业技术的不断发展,原有的控制手段已经不能满足对液体流量高精度,高速度的控制需求.在实际工作中采用三级构成:上位机采用工业PC机,其工作稳定,抗干扰能力强,寿命长;PLC部分采用西门子的S7-300系列处理器;外加一块FM355C专用PID控制模块进行数据模块进行数据采集和处理.上位机与PLC之间采用PROFIBUS通讯协议.采用一款西门子的触摸屏与PLC联机用于现场操作[1].1PLC控制系统设计本系统由上位机,PLC,触摸屏,流量计,电动阀构成,系统结构如图1所示.1.1上位机由工业PC机构成,其组态软件采用国产的MCGS6.0,对流量、阀位及其他各种参数进行显示和控制.上位机与PLC采用PROFIBUS通讯协议,最高通讯速率可达到1.5 Mb/s.1.2 PLC控制器PLC控制器包括PS-200,2A电源,CPU314,FM355C模块[2]. FM355C模块的接线端子表如表1.4、5脚为反馈信号输入脚,与靶式流量计连接,对于两线制的流量计4、5引脚间还需接一个10K的负载电阻.8、9为模拟量输出脚,与电动调节阀相连.14、15及18、19脚为第二路PID的输入与输出.1.3传感器和动作机构流量采集采用数字靶式流量计,该种流量计采用累计计数的工作方式,1 s钟累计1次,工作范围40~1 000 L/h,对大流量的采集较为精确.V型调节球阀利用球芯转动与阀座打开相割打开面积(V形窗口)来调节介质流量,调节性能、自洁性能好,适用于高粘度、悬浮液、纸浆告示不干净、含纤维介质场合.采用直连方式与执行机构连接,具有结构紧凑、尺寸小、重量轻、阻力小、动作稳定可靠等优点.流量计和调节阀的信号范围为4~20 mA,与PLC连接.1.4触摸屏采用西门子的TD100触摸屏,与PLC通过PROFIBUS总线相连.使用PROTOOLS6.0编辑界面监控各种参数.当上位机出现故障时,触摸屏可替代上位机操作,提高了系统的可靠性.2PID算法当被控对象的结构和参数不能完全掌握,或得不到精确的数学模型时,控制理论的其他技术难以采用时,系统控制器的结构和参数必须领先经验和现场调试来确定,这时应用PID控制技术最为方便,即利用比例、积分、微分计算出控制量进行控制[3].PID控制为3环节叠加,公式为:m(t) = Kpe(t)+Kpτde(t)dt+KiTi∫i0e(t)dt,其中Kp为比例系数,Kd为微分系数,τ为微分时间常数,Ki为积分系数.对于离散系统的PID公式为:P(k) = Kp{E(k)+TTi∑kj=0E(j)+TdT[E(k)-E(k-1)]}.3配置PID程序模块对西门子的PLC采用SIMA TIC STEP7 V5.3编程.进入STEP7的编程环境后首先通过“工程向导”配置硬件和网络参数,选用的电源模块为PS-200 2A ,中央处理器为CPU 314IFM,PID控制器为FM355 C型.完成配置后打开OB1主程序块(图2),调用FB31模块(STEP7中有LAD,STL ,FBD三种编程方式,STL为语句表编程方式,其他两种为图形调用方式).其中DB31为分配给FB31的背景块.FB31有如下几个参数必须设置:COM_RST参数地址DB31.DBX44. 0 BOOL型. FM355的启动开关.CHANNEL参数地址DB31.DBX2.0 BOOL型.控制端的通道号(每块FM355含两个通道).LMN_RE参数地址DB31.DBX52BOOL,参数类型为REAL型(32位浮点数).存储的是在未启动PID控制时的阀位值(即手动控制值),取值范围为0~100(系统将默认这些数值为电动阀的开度百分数),该参数在PID控制启动后不起作用.LMN_REON参数地址DB31.DBX6.4,参数类型BOOL型(在STEP7中为1位二进制数).当为1时PID控制关闭,LMN_RE的值作为输出值送给电动调节阀.当为0时,PID控制超作用,LMN_RE无效.PHASE参数地址DB31.DBD4,参数类型INT型(16位无符号整数).PID的相位控制,为1时控制相位反向180°.SP_RE参数地址DB31.DBD48,参数类型REAL型.PID 控制的设定值,取值范围为0~100(%).PID控制启动后模块通过计算该值与采集值的差值ER来改变输出值,仅当LMN_REON为0时有效.DEADB_W参数地址DB31.DBD104,参数类型REAL型.默认值为0,单位值为0,单位Hz.不工作区带宽设定值,差值ER将通过这个参数滤波.它关系到PID控制的性湖北大学学报(自然科学版)第28卷能.GAIN参数地址为DB31.DBD108,参数类型为REAL型.默认值为1.增益控制值,增益过大会提高系统的趋近速度,但同时会增大系统波动,导致系统不稳定.增益过小则会使系统的趋近速度变慢.TI参数地址为DB31.DBD112,参数类型REAL型.默认值为3000,单位s.积分时间常数.TI=0时,无积分环节.TD参数地址为DB31.DBD116,参数类型REAL型.默认值为0,单位s.微分时间常数.TD=0时,无微分环节.TM_LAG参数地址为DB31.DBD120,参数类型REAL型.默认值为5,单位s.微分时间延迟设置.LOAD_PAR参数地址为DB31.DBX44.3,参数类型为BOOL型.PID控制的启动开关,每次启动PID或改变PID参数后必须将此位置1,系统每次检查到此位为1,则将所有参数下载到FM355模块,然后将此位复位[4].4PID参数的调整方法PID参数的设置一方面是要根据控制对象的具体情况而定;另一方面是经验.Kp可控制幅值震荡,Kp大则会出现幅值震荡的幅度大,但震荡频率小,系统达到稳定时间长;Ki是解决动作响应的速度快慢的,Ki大了响应速度慢,反之则快;Kd是消除静态误差的,一般Kd设置都比较小,而且对系统影响比较小[5].5试验结论本系统在葛店的新武大有机硅厂通过测试.测试中流体采用甲醇,测试范围为50~250 L/h.以设定值为200 L/h的系统阶跃响应曲线为例,系统延迟时间td=3.5 s,上升时间tΓ=5 s,峰值时间tp=7 s,调节时间ts=28.5 s,超调量公式为:δ%=h(tp)-h(∞)h(∞)×100%,在试验中h(tp)=290,h(∞)=200,所以δ%=45%.经过调试后,本系统被证明完全能胜任有机硅生产过程中,对甲醇流量的精确控制. 参考文献:[1]周军,海心.电气控制及PLC[M].北京:机械工业出版社,2001:90 135.[2]余雷声,方宗达.电器控制与PLC应用[M].北京:机械工业出版社,1999:126 152.[3]陶永华,尹怕欣,葛芦生.新型PID控制及其应用[M].北京:机械工业出版社,1998.[4] Kember G, Dubay R. PID gain scheduling using fuzzy logic[J]. JSA Transactions, 2000,39(3):317 325.[5] Liu G P, Daley S. Optimal-tuning nonlinear PID controllers for unstable processes based on gain and phase marginspecifications: a fuzzy neural approach[J]. Fuzzy Sets and Systems, 2002: 128(1):95 106.The PID control system of flow measuring based on PLCXIAO Lei, XIE Ju-fang(School of Phisics and Electronic Technology, Hubei University, Wuhan 430062, China) Abstract:Described a kind of PID control system based on PIC. The system is composed of supervisor PC, PLC control block, the touch screen ,the flow measuring probe and the electrically operated valve. The system reads the flow measuring from the probe and then calculate the output value by using PID algorithm. The value output to the electrically operated valve to control the flow.The system also can be used in hard condition with high quality.Key words:flow measuring control system; PID; PLC; FM355C(责任编辑晏建章)。
《自动化学报》稿件编排规范
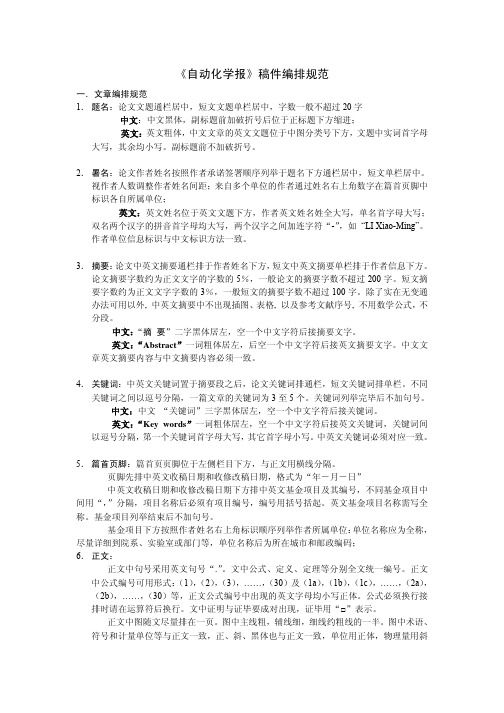
《自动化学报》稿件编排规范一.文章编排规范1.题名:论文文题通栏居中,短文文题单栏居中,字数一般不超过20字中文:中文黑体,副标题前加破折号后位于正标题下方缩进;英文:英文粗体,中文文章的英文文题位于中图分类号下方,文题中实词首字母大写,其余均小写。
副标题前不加破折号。
2.署名:论文作者姓名按照作者承诺签署顺序列举于题名下方通栏居中,短文单栏居中。
视作者人数调整作者姓名间距;来自多个单位的作者通过姓名右上角数字在篇首页脚中标识各自所属单位;英文:英文姓名位于英文文题下方,作者英文姓名姓全大写,单名首字母大写;双名两个汉字的拼音首字母均大写,两个汉字之间加连字符“-”,如 “LI Xiao-Ming”。
作者单位信息标识与中文标识方法一致。
3.摘要:论文中英文摘要通栏排于作者姓名下方,短文中英文摘要单栏排于作者信息下方。
论文摘要字数约为正文文字的字数的5%,一般论文的摘要字数不超过200字。
短文摘要字数约为正文文字字数的3%,一般短文的摘要字数不超过100字。
除了实在无变通办法可用以外, 中英文摘要中不出现插图、表格, 以及参考文献序号, 不用数学公式,不分段。
中文:“摘 要”二字黑体居左,空一个中文字符后接摘要文字。
英文:“Abstract”一词粗体居左,后空一个中文字符后接英文摘要文字。
中文文章英文摘要内容与中文摘要内容必须一致。
4.关键词:中英文关键词置于摘要段之后,论文关键词排通栏,短文关键词排单栏。
不同关键词之间以逗号分隔,一篇文章的关键词为3至5个。
关键词列举完毕后不加句号。
中文:中文“关键词”三字黑体居左,空一个中文字符后接关键词。
英文:“Key words”一词粗体居左,空一个中文字符后接英文关键词,关键词间以逗号分隔,第一个关键词首字母大写,其它首字母小写。
中英文关键词必须对应一致。
5.篇首页脚:篇首页页脚位于左侧栏目下方,与正文用横线分隔。
页脚先排中英文收稿日期和收修改稿日期,格式为“年-月-日”中英文收稿日期和收修改稿日期下方排中英文基金项目及其编号,不同基金项目中间用“,”分隔,项目名称后必须有项目编号,编号用括号括起。
LOGIC AND FUZZY SYSTEM Fuzzy Analogy of Linear Systems
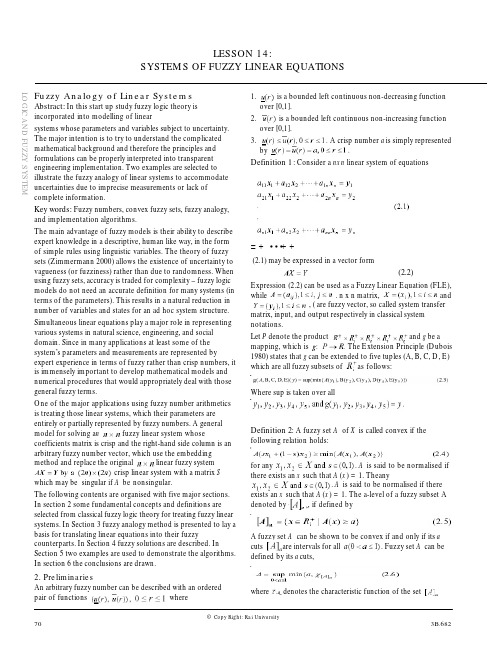
LOGIC AND FUZZY SYSTEMLESSON 14:SYSTEMS OF FUZZY LINEAR EQUATIONSFuzzy Analogy of Linear SystemsAbstract: In this start up study fuzzy logic theory is incorporated into modelling of linearsystems whose parameters and variables subject to uncertainty.The major intention is to try to understand the complicated mathematical background and therefore the principles and formulations can be properly interpreted into transparent engineering implementation. Two examples are selected to illustrate the fuzzy analogy of linear systems to accommodate uncertainties due to imprecise measurements or lack of complete information.Key words: Fuzzy numbers, convex fuzzy sets, fuzzy analogy,and implementation algorithms.The main advantage of fuzzy models is their ability to describe expert knowledge in a descriptive, human like way, in the form of simple rules using linguistic variables. The theory of fuzzy sets (Zimmermann 2000) allows the existence of uncertainty to vagueness (or fuzziness) rather than due to randomness. When using fuzzy sets, accuracy is traded for complexity – fuzzy logic models do not need an accurate definition for many systems (in terms of the parameters). This results in a natural reduction in number of variables and states for an ad hoc system structure.Simultaneous linear equations play a major role in representing various systems in natural science, engineering, and social domain. Since in many applications at least some of the system’s parameters and measurements are represented byexpert experience in terms of fuzzy rather than crisp numbers, it is immensely important to develop mathematical models and numerical procedures that would appropriately deal with those general fuzzy terms.One of the major applications using fuzzy number arithmetics is treating those linear systems, which their parameters are entirely or partially represented by fuzzy numbers. A general model for solving a n fuzzy linear system whosecoefficients matrix is crisp and the right-hand side column is an arbitrary fuzzy number vector, which use the embedding method and replace the original linear fuzzy systemcrisp linear system with a matrix Swhich may be singular if A be nonsingular.The following contents are organised with five major sections.In section 2 some fundamental concepts and definitions are selected from classical fuzzy logic theory for treating fuzzy linear systems. In Section 3 fuzzy analogy method is presented to lay a basis for translating linear equations into their fuzzycounterparts. In Section 4 fuzzy solutions are described. InSection 5 two examples are used to demonstrate the algorithms.In section 6 the conclusions are drawn.2. PreliminariesAn arbitrary fuzzy number can be described with an ordered pair of functions where1. is a bounded left continuous non-decreasing functionover [0,1].2. is a bounded left continuous non-increasing function over [0,1].3.A crisp number a is simply representedbyDefinition 1 : Consider a nxnlinear system of equations= + · · · + +(2.1) may be expressed in a vector form(2.2)Expression (2.2) can be used as a Fuzzy Linear Equation (FLE),while . n x n matrix, and, ( are fuzzy vector, so called system transfermatrix, input, and output respectively in classical system notations.Let P denote the product and g be a mapping, which is The Extension Principle (Dubois 1980) states that g can be extended to five tuples (A, B, C, D, E)which are all fuzzy subsets ofas follows:Where sup is taken over allDefinition 2: A fuzzy set A of X is called convex if thefollowing relation holds:for any . A is said to be normalised if there exists an x such that A (x ) = 1. Theany. A is said to be normalised if thereexists an x such that A (x ) = 1. The a-level of a fuzzy subset A denoted by, if defined byA fuzzy set A can be shown to be convex if and only if its a cuts are intervals for all . Fuzzy set A can be defined by its acuts,wheredenotes the characteristic function of the setLOGIC AND FUZZY SYSTEMDefinition 3 Fuzzy number A is called a number of the R L .type if its membership functionhas the following form:where L and R are continuous non-increasing functions, defined on ), strictly decreasing to zero in those subintervals of the interval ) , in which they are positive, and fulfilling the condition The parameters a and â are non-negative real numbers.3. Fuzzy AnalogyIf X is the set of real numbers, by a fuzzy number x N , this means a fuzzy subset of X where •, if and only if y = x.•is continuous.• is convex.•vanishes at infinity..denotes the degree of belief that the value of x is infact y. Consider a class of functional equations of the formwhere f is unknown, may be either addition or multiplication of real numbers. (3.1) may be put in a generalformwhereis a function relating the unknownquantities stands for andstands forLet denote the degree of belief that x equals to y . Using extension principle (Dubois 1980), (3.2) can be expressed in thefuzzy formwhere the sup is taken over all y 1and y2 for whichAccording to Nguyen (1978), (3.3) and (3.1) may be the implies as following For the alevels are equalis a closed and bounded interval. Hence,,where are the left endpointand the right endpoint respectively associated with the a level of the fuzzy number w . If F is non-decreasing in its argument and Fis continuous, then it givesMoreover solutions to (3.5) and (3.6), say functions H 1and H2, respectively, are continuous and no-decreasing. ThusSo, the solution of (3.3) is given bywhere H 1 and H 2 are the solutions of (3.5) and (3.6),respectively. According to (3.3), the solution of (3.8) may alsobe represented bywhere the sup is taken over allis thecharacteristic function over the interval4. Fuzzy Solutions A solution tto (2.1) one should recall that forarbitrary fuzzy numbers and realnumber k ,•x = yif and only if•Definition 4: A fuzzy number vector givenby is called a solution ofthe FSLE ifConsider the ith equation of the system (2.1)it hasFrom (2.4) two crisp linear systems for all i that there can be extended to. crisp linear system as follows:LOGIC AND FUZZY SYSTEMThus FLE (2.1) is extended to a crisp (2.5) where A= S 2 + S 1.(4.5) can be write as follows:where the matrix S is nonsingular if and only if the matrices A=S1+S2 and S1-S2 are both non-singular.Definition 5: Let denote the unique solution of SX = Y . The fuzzy number vectoris defined byis called the fuzzy solution of SX = Y . Ifare all triangular fuzzy numbers thenand U is called a strongfuzzy solution. Otherwise, U is a weak fuzzy solution.In the general, the structure of S implies thatand thatWhere B contains the positive entries of A , C the absolute values of the negative entries of A , and A=B-C If linear system are fuzzy variables, for the inputsdenotes the degree of,inputThesolution of linear system are givenIf linear system are fuzzy coefficients and fuzzy variables, whichis in system (2.1)are fuzzy numbers, denotes the degree ofcoefficients and variable respectively.where so the solution of systemsAX = Yis5. Examples and SimulationsTwo examples were selected to demonstrate the understanding of the fuzzy of analogy of linear systems.Example 1:Consider a simple linear system B AX Y + = withfuzzy output and input variablesFig.1 shows a fuzzy line, which denotes what a line looks like with a fuzzy input.Example 2 :Consider a two inputs & single output fuzzy system with fuzzy outputsY = AX + B(5.3)Where .The extended 4 x 4 . matrix isThe fuzzy solution isFig.2 shows that variables x 1and x 2 are determined by the output y which is the ácut, when y = 1, an accurate value for thesolution can be obtained.LOGIC AND FUZZY SYSTEM6. ConclusionsIn this paper a general model structure is presented for solving of linear equations (systems) with fuzzy variables andparameters. With this structure, a fuzzy system with a matrix Ais transformed into a crisp linear system S . The system is then solved with crisp variables and parameters and thesolution vector is either a strong fuzzy solution or a weak fuzzy solution. Solutions of linear fuzzy equations have been well addressed in mathematics. However it is still a long distance to arrive in engineering field for applications. This study has attempted to bridge the two domains. Additionally fuzzy analogy of nonlinear systems is a new area and will be studied as the expansion of linear systems.References1.Zimmermann, H.J., Fuzzy Set Theory-and Its Applications,3rd Edition, Kluwer Academic Publishers, Dordrecht, 2000.2.Wang, L.X., Adaptive Fuzzy Systems and Control, New Jersey 07632, 1999.3.Klir, G.J., Folger, T.A., Fuzzy Sets, Uncerainty andInformation, Prentice-Hall, Englewood, Cli.s, NJ, 1988.4.Dubois, D., and Prade, H., Fuzzy Sets and Systems: Theory and Applications, Academic Press, New York, 1980.5.Deeba, E., On a fuzzy difference equation. IEEE Trans.Fuzzy Systems 3(3):469-472, 1995.6.Deeba, E., On a fuzzy logistic difference equation.Differential Equations Dynam. Systems 4(2):149-156, 19967.Deschrijver, G., On the relationship between someextensions of fuzzy set theory, Fuzzy Sets and Systems 133:227-235, 2003.8.Friedman, M., Fuzzy Linear Systems, Fuzzy Sets and Systems 96(2): 201-209, 1998.9.Soliman, S.A., Fuzzy linear parameter estimation algorithms:a new formulation, International Journal of Electrical Power & Energy Systems 24(5): 415-420, 200210.Nguyen, H.T., A note on the extension principle for fuzzy sets, J. Math. Anl. 64(2): 369-380, 1978Notes。
Fuzzy Logic and Systems

Fuzzy Logic and SystemsFuzzy Logic and Systems: A Comprehensive OverviewIn the realm of computational intelligence, fuzzy logic and systems have emerged as a powerful tool for dealing with uncertainty and imprecision. This essay aims to provide a comprehensive overview of fuzzy logic and its applications in various systems, highlighting the significance of this approach in modern technology.Introduction to Fuzzy LogicFuzzy logic, introduced by Lotfi A. Zadeh in 1965, is a mathematical logicthat deals with approximate reasoning. Unlike traditional binary logic, which is based on crisp values of true and false, fuzzy logic allows for a range of truth values between 0 and 1. This flexibility enables fuzzy logic to model human reasoning more accurately, particularly in situations where data is incomplete or ambiguous.Fundamentals of Fuzzy LogicAt the core of fuzzy logic is the concept of a fuzzy set, which is a generalization of a classical set. A fuzzy set is characterized by a membership function that assigns each element a degree of membership between 0 and 1. This degree reflects the extent to which an element belongs to the set. Fuzzy logic also employs linguistic variables, which are used to express concepts in a natural language-like manner, making it easier for humans to interact with the system.Fuzzy Logic OperationsFuzzy logic operations include fuzzy union, intersection, and complement. These operations are analogous to classical set operations but are adapted to handle the fuzzy nature of the membership values. The union of two fuzzy sets, for example, combines the membership values of their elements, while the intersectionfinds the minimum membership value among the elements of both sets. The complement operation inverts the membership values, assigning a value of 1 minus the original membership to each element.Applications of Fuzzy LogicFuzzy logic has found applications in a wide range of fields, includingcontrol systems, decision-making, and artificial intelligence. In control systems, fuzzy logic controllers (FLCs) are used to manage complex processes byinterpreting input data and generating appropriate control actions. FLCs are particularly useful in situations where traditional control methods struggle with non-linear or time-varying systems.In decision-making, fuzzy logic helps to address the uncertainty inherent in human judgments. By incorporating fuzzy sets and linguistic variables, decision-makers can create more nuanced and context-sensitive decision models. This approach is particularly valuable in fields such as finance, where decisions must be made with incomplete or uncertain information.Artificial intelligence also benefits from fuzzy logic, as it allows systems to process and interpret ambiguous or imprecise data. This capability is crucialfor developing systems that can interact with humans in a more natural andintuitive manner.Challenges and LimitationsDespite its many advantages, fuzzy logic also faces challenges and limitations. One of the primary concerns is the difficulty in designing and tuning fuzzy systems, particularly when dealing with complex problems. The process of determining appropriate membership functions and rule sets can be time-consuming and requires a deep understanding of the problem domain.Another challenge is the interpretability of fuzzy systems. While fuzzy logic can handle uncertainty and imprecision, it can sometimes be difficult for users tounderstand the reasoning behind the system's decisions. This lack of transparency can be a barrier to the widespread adoption of fuzzy logic in certain applications.Future DirectionsAs technology continues to advance, the role of fuzzy logic in systems is likely to grow. With the increasing prevalence of big data and the need to make sense of complex, uncertain information, fuzzy logic offers a valuable approach to processing and analyzing this data. Additionally, as systems become more integrated into our daily lives, the ability to handle ambiguity and uncertainty will be crucial for creating more human-like interactions.In the future, we can expect to see more research and development in the area of fuzzy logic, with a focus on improving the design and tuning processes, as well as enhancing the interpretability of fuzzy systems. This will help to overcome some of the current challenges and limitations, paving the way for even broader applications of fuzzy logic in various fields.ConclusionFuzzy logic and systems have proven to be a valuable tool for managing uncertainty and imprecision in a wide range of applications. From control systems to decision-making and artificial intelligence, fuzzy logic offers a flexible and powerful approach to dealing with complex problems. While challenges and limitations exist, ongoing research and development are likely to address these issues and further expand the potential of fuzzy logic in the years to come.In conclusion, fuzzy logic and systems represent an important area of study within the field of computational intelligence. As we continue to explore the potential of this approach, we can expect to see its influence grow, shaping the way we interact with technology and making our systems more adaptable and responsive to the complexities of the real world.。
PID流量控制
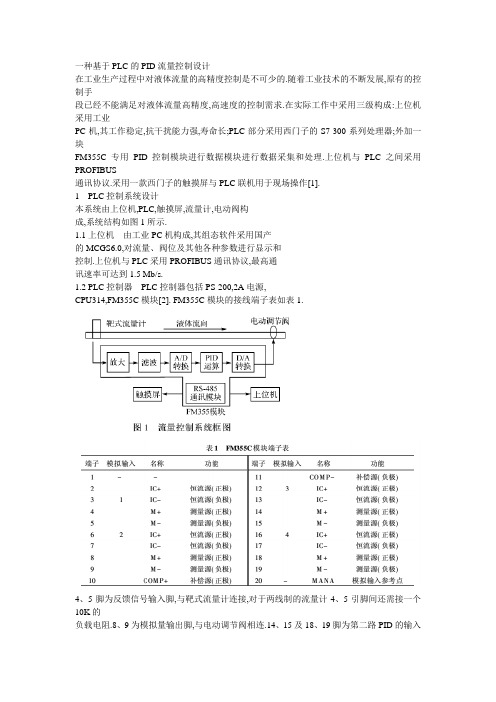
一种基于PLC的PID流量控制设计在工业生产过程中对液体流量的高精度控制是不可少的.随着工业技术的不断发展,原有的控制手段已经不能满足对液体流量高精度,高速度的控制需求.在实际工作中采用三级构成:上位机采用工业PC机,其工作稳定,抗干扰能力强,寿命长;PLC部分采用西门子的S7-300系列处理器;外加一块FM355C专用PID控制模块进行数据模块进行数据采集和处理.上位机与PLC之间采用PROFIBUS通讯协议.采用一款西门子的触摸屏与PLC联机用于现场操作[1].1PLC控制系统设计本系统由上位机,PLC,触摸屏,流量计,电动阀构成,系统结构如图1所示.1.1上位机由工业PC机构成,其组态软件采用国产的MCGS6.0,对流量、阀位及其他各种参数进行显示和控制.上位机与PLC采用PROFIBUS通讯协议,最高通讯速率可达到1.5 Mb/s.1.2 PLC控制器PLC控制器包括PS-200,2A电源,CPU314,FM355C模块[2]. FM355C模块的接线端子表如表1.4、5脚为反馈信号输入脚,与靶式流量计连接,对于两线制的流量计4、5引脚间还需接一个10K的负载电阻.8、9为模拟量输出脚,与电动调节阀相连.14、15及18、19脚为第二路PID的输入与输出.1.3传感器和动作机构流量采集采用数字靶式流量计,该种流量计采用累计计数的工作方式,1 s钟累计1次,工作范围40~1 000 L/h,对大流量的采集较为精确.V型调节球阀利用球芯转动与阀座打开相割打开面积(V形窗口)来调节介质流量,调节性能、自洁性能好,适用于高粘度、悬浮液、纸浆告示不干净、含纤维介质场合.采用直连方式与执行机构连接,具有结构紧凑、尺寸小、重量轻、阻力小、动作稳定可靠等优点.流量计和调节阀的信号范围为4~20 mA,与PLC连接.1.4触摸屏采用西门子的TD100触摸屏,与PLC通过PROFIBUS总线相连.使用PROTOOLS6.0编辑界面监控各种参数.当上位机出现故障时,触摸屏可替代上位机操作,提高了系统的可靠性.2PID算法当被控对象的结构和参数不能完全掌握,或得不到精确的数学模型时,控制理论的其他技术难以采用时,系统控制器的结构和参数必须领先经验和现场调试来确定,这时应用PID控制技术最为方便,即利用比例、积分、微分计算出控制量进行控制[3].PID控制为3环节叠加,公式为:m(t) = Kpe(t)+Kpτde(t)dt+KiTi∫i0e(t)dt,其中Kp为比例系数,Kd为微分系数,τ为微分时间常数,Ki为积分系数.对于离散系统的PID公式为:P(k) = Kp{E(k)+TTi∑kj=0E(j)+TdT[E(k)-E(k-1)]}.3配置PID程序模块对西门子的PLC采用SIMA TIC STEP7 V5.3编程.进入STEP7的编程环境后首先通过“工程向导”配置硬件和网络参数,选用的电源模块为PS-200 2A ,中央处理器为CPU 314IFM,PID控制器为FM355 C型.完成配置后打开OB1主程序块(图2),调用FB31模块(STEP7中有LAD,STL ,FBD三种编程方式,STL为语句表编程方式,其他两种为图形调用方式).其中DB31为分配给FB31的背景块.FB31有如下几个参数必须设置:COM_RST参数地址DB31.DBX44. 0 BOOL型. FM355的启动开关.CHANNEL参数地址DB31.DBX2.0 BOOL型.控制端的通道号(每块FM355含两个通道).LMN_RE参数地址DB31.DBX52BOOL,参数类型为REAL型(32位浮点数).存储的是在未启动PID控制时的阀位值(即手动控制值),取值范围为0~100(系统将默认这些数值为电动阀的开度百分数),该参数在PID控制启动后不起作用.LMN_REON参数地址DB31.DBX6.4,参数类型BOOL型(在STEP7中为1位二进制数).当为1时PID控制关闭,LMN_RE的值作为输出值送给电动调节阀.当为0时,PID控制超作用,LMN_RE无效.PHASE参数地址DB31.DBD4,参数类型INT型(16位无符号整数).PID的相位控制,为1时控制相位反向180°.SP_RE参数地址DB31.DBD48,参数类型REAL型.PID 控制的设定值,取值范围为0~100(%).PID控制启动后模块通过计算该值与采集值的差值ER来改变输出值,仅当LMN_REON为0时有效.DEADB_W参数地址DB31.DBD104,参数类型REAL型.默认值为0,单位值为0,单位Hz.不工作区带宽设定值,差值ER将通过这个参数滤波.它关系到PID控制的性湖北大学学报(自然科学版)第28卷能.GAIN参数地址为DB31.DBD108,参数类型为REAL型.默认值为1.增益控制值,增益过大会提高系统的趋近速度,但同时会增大系统波动,导致系统不稳定.增益过小则会使系统的趋近速度变慢.TI参数地址为DB31.DBD112,参数类型REAL型.默认值为3000,单位s.积分时间常数.TI=0时,无积分环节.TD参数地址为DB31.DBD116,参数类型REAL型.默认值为0,单位s.微分时间常数.TD=0时,无微分环节.TM_LAG参数地址为DB31.DBD120,参数类型REAL型.默认值为5,单位s.微分时间延迟设置.LOAD_PAR参数地址为DB31.DBX44.3,参数类型为BOOL型.PID控制的启动开关,每次启动PID或改变PID参数后必须将此位置1,系统每次检查到此位为1,则将所有参数下载到FM355模块,然后将此位复位[4].4PID参数的调整方法PID参数的设置一方面是要根据控制对象的具体情况而定;另一方面是经验.Kp可控制幅值震荡,Kp大则会出现幅值震荡的幅度大,但震荡频率小,系统达到稳定时间长;Ki是解决动作响应的速度快慢的,Ki大了响应速度慢,反之则快;Kd是消除静态误差的,一般Kd设置都比较小,而且对系统影响比较小[5].5试验结论本系统在葛店的新武大有机硅厂通过测试.测试中流体采用甲醇,测试范围为50~250 L/h.以设定值为200 L/h的系统阶跃响应曲线为例,系统延迟时间td=3.5 s,上升时间tΓ=5 s,峰值时间tp=7 s,调节时间ts=28.5 s,超调量公式为:δ%=h(tp)-h(∞)h(∞)×100%,在试验中h(tp)=290,h(∞)=200,所以δ%=45%.经过调试后,本系统被证明完全能胜任有机硅生产过程中,对甲醇流量的精确控制. 参考文献:[1]周军,海心.电气控制及PLC[M].北京:机械工业出版社,2001:90 135.[2]余雷声,方宗达.电器控制与PLC应用[M].北京:机械工业出版社,1999:126 152.[3]陶永华,尹怕欣,葛芦生.新型PID控制及其应用[M].北京:机械工业出版社,1998.[4] Kember G, Dubay R. PID gain scheduling using fuzzy logic[J]. JSA Transactions, 2000,39(3):317 325.[5] Liu G P, Daley S. Optimal-tuning nonlinear PID controllers for unstable processes based on gain and phase marginspecifications: a fuzzy neural approach[J]. Fuzzy Sets and Systems, 2002: 128(1):95 106.The PID control system of flow measuring based on PLCXIAO Lei, XIE Ju-fang(School of Phisics and Electronic Technology, Hubei University, Wuhan 430062, China) Abstract:Described a kind of PID control system based on PIC. The system is composed of supervisor PC, PLC control block, the touch screen ,the flow measuring probe and the electrically operated valve. The system reads the flow measuring from the probe and then calculate the output value by using PID algorithm. The value output to the electrically operated valve to control the flow.The system also can be used in hard condition with high quality.Key words:flow measuring control system; PID; PLC; FM355C(责任编辑晏建章)。
Tutorial On Fuzzy Logic
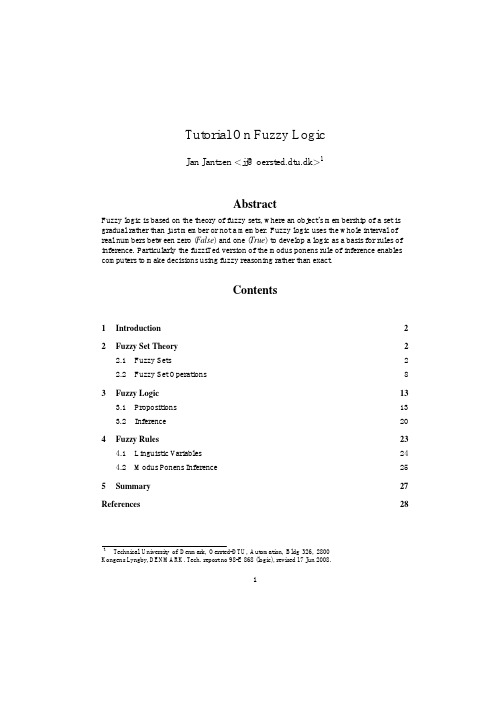
Tutorial On Fuzzy LogicJan Jantzen<jj@oersted.dtu.dk>1AbstractFuzzy logic is based on the theory of fuzzy sets,where an object’s membership of a set is gradual rather than just member or not a member.Fuzzy logic uses the whole interval of real numbers between zero(False)and one(True)to develop a logic as a basis for rules of inference.Particularly the fuzzified version of the modus ponens rule of inference enables computers to make decisions using fuzzy reasoning rather than exact.Contents1Introduction2 2Fuzzy Set Theory22.1Fuzzy Sets22.2Fuzzy Set Operations8 3Fuzzy Logic133.1Propositions133.2Inference20 4Fuzzy Rules234.1Linguistic Variables244.2Modus Ponens Inference25 5Summary27 References281Technical University of Denmark,Oersted-DTU,Automation,Bldg326,2800Kongens Lyngby,DENMARK.Tech.report no98-E868(logic),revised17Jun2008.11IntroductionAn assertion can be more or less true in fuzzy logic.In classical logic an assertion is either true or false—not something in between—and fuzzy logic extends classical logic by allowing intermediate truth values between zero and one.Fuzzy logic enables a computer to interpret a linguistic statement such as’if the washing machine is half full,then use less water.’It adds intelligence to the washing machine since the computer infers an action from a set of such if-then rules.Fuzzy logic is’computing with words,’quoting the creator of fuzzy logic, LotfiA.Zadeh.The objective of this tutorial is to explain the necessary and sufficient parts of the theory, such that engineering students understand how fuzzy logic enables fuzzy reasoning by computers.2Fuzzy Set TheoryFuzzy sets are a further development of mathematical set theory,first studied formally by the German mathematician Georg Cantor(1845-1918).It is possible to express mostof mathematics in the language of set theory,and researchers are today looking at the consequences of’fuzzifying’set theory resulting in for example fuzzy logic,fuzzy numbers, fuzzy intervals,fuzzy arithmetic,and fuzzy integrals.Fuzzy logic is based on fuzzy sets, and with fuzzy logic a computer can process words from natural language,such as’small’,’large’,and’approximately equal’.Although elementary,the following sections include the basic definitions of classical set theory.This is to shed light on the original ideas,and thus provide a deeper understanding. But only those basic definitions that are necessary and sufficient will be presented;students interested in delving deeper into set theory and logic,can for example read the book by Stoll (1979[10]);it provides a precise and comprehensive treatment.2.1Fuzzy SetsAccording to Cantor a set X is a collection of definite,distinguishable objects of our intuition which can be treated as a whole.The objects are the members of X.The concept ’objects of our intuition’gives us great freedom of choice,even sets with infinitely many members.Objects must be’definite’:given an object and a set,it must be possible to determine whether the object is,or is not,a member of the set.Objects must also be’distinguishable’:given a set and its members,it must be possible to determine whether any two members are different,or the same.The members completely define a set.To determine membership,it is necessary that the sentence’x is a member of X’,where x is replaced by an object and X by the name of a set, is either true or false.We use the symbol∈and write x∈X if object x is a member of the set X.The assumption that the members determine a set is equivalent to saying:Two sets X and Y are equal,X=Y,iff(if and only if)they have the same members.The set whose members are the objects x1,x2,...,x n is written2{x1,x2,...,x n}.In particular,the set with no members is the empty set symbolized by∅.The set X is included in Y,X⊆Yiff each member of X is a member of Y.We also say that X is a subset of Y,and it means that,for all x,if x∈X,then x∈Y.The empty set is a subset of every set.Almost anything called a set in ordinary conversation is acceptable as a mathematical set,as the next example indicates.Example1Classical setsThe following are lists or collections of definite and distinguishable objects,and therefore sets in the mathematical sense.(a)The set of non-negative integers less than3.This is afinite set with three members {0,1,2}.(b)The set of live dinosaurs in the basement of the British Museum.This set has no members,it is the empty set∅.(c)The set of measurements greater than10volts.Even though this set is infinite,it is possible to determine whether a given measurement is a member or not.(d)The set{0,1,2}is the set from(a).Since{0,1,2}and{2,1,0}have the same mem-bers,they are equal sets.Moreover,{0,1,2}={0,1,1,2}for the same reason.(e)The members of a set may themselves be sets.The setX={{1,3},{2,4},{5,6}}is a set with three members,namely,{1,3},{2,4},and{5,6}.Matlab supports sets of sets, or nested sets,in cell arrays.The notation in Matlab for assigning the above sets to a cell array x is the same.(f)It is possible in Matlab to assign an empty set,for instance:x={[]}.Although the brace notation{·}is practical for listing sets of a few elements,it is impractical for large sets and impossible for infinite sets.How do we then define a set with a large number of members?An answer requires a few more concepts.A proposition is an assertion(declarative statement)which can be classified as either true or false.By a predicate in x we understand an assertion formed using a formula in x.For instance,’0<x≤3’,or’x>10volts’are predicates.They are not propositions,however,since they are not necessarily true or false.Only if we assign a value to the variable x,each predicate becomes a proposition.A predicate P(x)in x defines a set X by the convention that the members of X are exactly those objects a such that P(a)is true.In mathematical notation:{x|P(x)},read’the set of all x such that P(x).’Thus a∈{x|P(x)}iff P(a)is a true proposition.A system in which propositions must be either true or false,but not both,uses atwo-valued logic.As a consequence,what is not true is false and vice versa;that is the law of the excluded middle.This is only an approximation to human reasoning,as Zadeh observed:3Clearly,the"class of all real numbers which are much greater than1,"or"the class of beautiful women,"or"the class of tall men,"do not constitute classes or sets in the usual mathematical sense of these terms.(Zadeh,1965[12])Zadeh’s challenge we might call it,because for instance’tall’is an elastic property.To define the set of tall men as a classical set,one would use a predicate P(x),for instancex≥176,where x is the height of a person,and the right hand side of the inequality a threshold value in centimeters(176centimeters'5foot9inches).This is an abrupt approximation to the concept’tall’.From an engineering viewpoint,it is likely that the measurement is uncertain,due to a source of noise in the equipment.Thus,measurements within the narrow band176±ε,whereεexpresses variation in the noise,could fall on either side of the threshold randomly.Following Zadeh a membership grade allowsfiner detail,such that the transition from membership to non-membership is gradual rather than abrupt.The membership grade for all members defines a fuzzy set(Fig.1).Given a collection of objects U,a fuzzy set A in U is defined as a set of ordered pairsA≡{h x,μA(x)i|x∈U}(1) whereμA(x)is called the membership function for the set of all objects x in U—for the symbol’≡’read’defined as’.The membership function relates to each x a membership gradeμA(x),a real number in the closed interval[0,1].Notice it is now necessary to work with pairs h x,μA(x)i whereas for classical sets a list of objects suffices,since their membership is understood.An ordered pair h x,y i is a list of two objects,in which the object x is consideredfirst and y second(note:in the set{x,y}the order is insignificant).The term’fuzzy’(indistinct)suggests an image of a boundary zone,rather than an abrupt frontier.Indeed,fuzzy logicians speak of classical sets being crisp sets,to distinguish them from fuzzy sets.As with crisp sets,we are only guided by intuition in deciding which objects are members and which are not;a formal basis for how to determine the membership grade of a fuzzy set is absent.The membership grade is a precise,but arbitrary measure:it rests on personal opinion,not reason.The definition of a fuzzy set extends the definition of a classical set,because membership valuesμare permitted in the interval0≤μ≤1,and the higher the value,the higher the membership.A classical set is consequently a special case of a fuzzy set,with membership values restricted toμ∈{0,1}.A single pair h x,μ(x)i is a fuzzy singleton;thus the whole set can be viewed as the union of its constituent singletons.Example2Fuzzy setsThe following are sets which could be described by fuzzy membership functions.(a)The set of real numbers xÀ1(x much greater than one).(b)The set of high temperatures,the set of strong winds,or the set of nice days are fuzzy sets in weather reports.(c)The set of young people.A one year old baby will clearly be a member of the set of young people,and a100-year-old person will not be a member of this set.A person aged304Figure1.Two definitions of the set of"tall men",a crisp set and a fuzzy set.(figtall.m) might be young to the degree0.5.(d)The set of adults.The Danish railways allow children under the age of15to travel at half price.An adult is thus defined by the set of passengers aged15or older.By their definition the set of adults is a crisp set.(e)A predicate may be crisp,but perceived as fuzzy:a speed limit of60kilometres per hour is by some drivers taken to be an elastic range of more or less acceptable speeds within, say,60−70kilometres per hour('37−44miles per hour).Notice how,on the one hand, the traffic law is crisp while,on the other hand,those drivers’s understanding of the law is fuzzy.Members of a fuzzy set are taken from a universe of discourse,or universe for short.The universe is all objects that can come into consideration,confer the set U in(1).The universe depends on the context,as the next example shows.Example3Universes(a)The set xÀ1could have as a universe all real numbers,alternatively all positive integers.(b)The set of young people could have all human beings in the world as its universe. Alternatively it could have the numbers between0and100;these would then represent age in years.(c)The universe depends on the measuring unit;a duration in time depends on whether it is measured in hours,days,or weeks.(d)A non-numerical quantity,for instance taste,must be defined on a psychological continuum;an example of such a universe is U={bitter,sweet,sour,salt,hot}.A programmer can exploit the universe to suppress faulty measurement data,for instance negative values for a duration of time,by making the program consult the universe.5Figure 2.Around noon.Four possible membership functions representing the time ’around noon’:a)trapeziodal,b)triangular,c)smooth trapezoid,and d)smooth triangular.The universe is the hours of the day in 24-hour format.(figmf0.m)There are two alternative ways to represent a membership function:continuous ordiscrete.A continuous fuzzy set A is de fined by means of a continuous membership function μA (x ).A trapezoidal membership function is a piecewise linear,continuous function,controlled by four parameters {a,b,c,d }(Jang et al.,1997[4])μtrapezoid (x ;a,b,c,d )=⎧⎪⎪⎪⎪⎨⎪⎪⎪⎪⎩0,x ≤a x −a ,a ≤x ≤b 1,b ≤x ≤c d −x d −c ,c ≤x ≤d 0,d ≤x⎫⎪⎪⎪⎪⎬⎪⎪⎪⎪⎭,x ∈R (2)The parameters a ≤b ≤c ≤d de fine four breakpoints,here designated:left footpoint (a ),left shoulderpoint (b ),right shoulderpoint (c ),and right footpoint (d ).Figure 2(a)illustrates a trapezoidal membership function.A triangular membership function is piecewise linear,and derived from the trapezoidal membership function by merging the two shoulderpoints into one,that is,setting b =c,Fig.2(b).Smooth,differentiable versions of the trapezoidal and triangular membership functions can be obtained by replacing the linear segments corresponding to the intervals a ≤x ≤b6and c ≤x ≤d by a nonlinear function,for instance a half period of a cosine function,μST rapezoid (x ;a,b,c,d )=⎧⎪⎪⎪⎪⎨⎪⎪⎪⎪⎩0,x ≤a 1+1cos(x −b π),a ≤x ≤b 1,b ≤x ≤c 12+12cos(x −c d −c π),c ≤x ≤d 0,d ≤x⎫⎪⎪⎪⎪⎬⎪⎪⎪⎪⎭,x ∈R We call it here STrapezoid for ’smooth trapezoid ’or ’soft trapezoid ’.Figures 2(c-d)illustrate the smooth membership functions.Other possibilities exist for generating smooth trapezoidal functions,for example Gaussian,generalized bell,and sigmoidal membership functions (Jang et al.,1997[4]).Discrete fuzzy sets are de fined by means of a discrete variable x i (i =1,2,...).A discrete fuzzy set A is de fined by ordered pairs,A ={h x 1,μ(x 1)i ,h x 2,μ(x 2)i ,...|x i ∈U ,i =1,2,...}Each membership value μ(x i )is an evaluation of the membership function μat a discrete point x i in the universe U ,and the whole set is a collection,usually finite,of pairs h x i ,μ(x i )i .Example 4Discrete membership functionTo achieve a discrete triangular membership function from the trapezoid (2)assume the uni-verse is a vector u of 7elements.In Matlab notation,u=[9101112131415]Assume the de fining parameters are a =10,b =12,c =12,and d =14then,by (2),the corresponding membership values are a vector of 7elements,000.510.500Each membership value corresponds to one element of the universe,more clearly displayed aswith the universe in the top row.When this is impractical,in a program,the universe and the membership values can be kept in separate vectors.As a crude rule of thumb,the continuous form is more computing intensive,but less storage demanding than the discrete form.Zadeh writes that a fuzzy set induces a possibility distribution on the universe,meaning,one can interpret the membership values as possibilities.How are then possibilities related to probabilities?First of all,probabilities must add up to one,or the area under a density curve must be one.Memberships may add up to anything (discrete case),or the area under the membership function may be anything (continuous case).Secondly,a probability distribution concerns the likelihood of an event to occur,based on observations,whereas7a possibility distribution(membership function)is subjective.The word’probably’is synonymous with presumably,assumably,doubtless,likely,presumptively.The word’possible’is synonymous with doable,feasible,practicable,viable,workable.Their relationship is best described in the sentence:what is probable is always possible,but not vice versa.This is illustrated next.Example5Probability versus possibilitya)Consider the statement’Hans ate x eggs for breakfast’,where x∈U=h1,2,...,8i(Zadeh in Zimmermann,1993[16]).We may associate a probability distribution p by observing Hans eating breakfast for100days,A fuzzy set expressing the grade of ease with which Hans can eat x eggs may be the following possibility distributionπ,Where the possibilityπ(3)=1,the probability p(3)=0.1only.b)Consider a universe of four car modelsU={T rabant,F iat Uno,BMW,F errari}.We may associate a probability p(x)of each car model driving100miles per hour(161 kilometres per hour)on a motorway,by observing cars for100days,p(T rabant)=0,p(F iat Uno)=0.1,p(BMW)=0.4,p(F errari)=0.5The possibilities may beπ(T rabant)=0,π(F iat Uno)=0.5,π(BMW)=1,π(F errari)=1 Notice that each possibility is at least as high as the corresponding probability.Equality and inclusion are defined by means of membership functions.Two fuzzy sets A and B are equal,iff they have the same membership function,A=B≡μA(x)=μB(x)(3) for all x.A fuzzy set A is a subset of(included in)a fuzzy set B,iff the membership of A is less than equal to that of B,A⊆B≡μA(x)≤μB(x)(4) for all x.2.2Fuzzy Set OperationsIn order to generate new sets from existing sets we define two operations,in certain respects analogous to addition and multiplication.The(classical)union of the sets X and Y, symbolized by X∪Y and read’X union Y’,is the set of all objects which are members of8Figure3.Set operations.The top row are classical Venn diagrams;the universe is repre-sented by the points within the rectangle,and sets by the interior of the circles.The bottom row their fuzzy equivalents;the universal set is represented by a horisontal line at member-shipμ=1,and sets by membership functions.The shaded areas are:a)and d)union A∪B, b)and e)intersection A∩B,and c)and f)complement A∪B.(figvenn2.m)X or Y,or both.That is,X∪Y≡{x|x∈X or x∈Y}Thus,by definition,x∈X∪Y iff x is a member of at least one of X and Y.For example,{1,2,3}∪{1,3,4}={1,2,3,4}The(classical)intersection of the sets X and Y,symbolized by X∩Y and read’X intersection Y’,is the set of all objects which are members of both X and Y.That is,X∩Y≡{x|x∈X and y∈Y}For example,{1,2,3}∩{1,3,4}={1,3}The(classical)complement of a set X,symbolized by X and read’the complement of X’isX≡{x|x/∈X}That is,the set of those members of the universe which are not members(/∈)of X.Venn diagrams clearly illustrate the set operations,Fig.3(a-c).When turning to fuzzy sets,the gradual membership complicates matters.Figure3(d-f)9shows an intuitively acceptable modification of the Venn diagrams.The following fuzzy set operations are defined accordingly:Let A and B be fuzzy sets defined on a mutual universe U.The fuzzy union of A and B isA∪B≡{h x,μA∪B(x)i|x∈U andμA∪B(x)=max(μA(x),μB(x))}The fuzzy intersection of A and B isA∩B≡{h x,μA∩B(x)i|x∈U andμA∩B(x)=min(μA(x),μB(x))}The fuzzy complement of A isA≡{h x,μ(x)i|x∈U andμ(x)=1−μA(x)}While the notation may look cumbersome,it is in practice easy to apply the fuzzy set operations max,min,and1−μ.Example6Buying a house(after Zimmermann,1993[16])A four-person family wishes to buy a house.An indication of their level of comfort is the number of bedrooms in the house.But they also wish a large house.The universe U= h1,2,3,4,5,6,7,8,9,10i is the set of houses to be considered described by their number of bedrooms.The fuzzy set Comfortable may be described as a vector c,or in Matlabc=£0.20.50.810.70.30000¤Let l describe the fuzzy set Large,defined asl=£000.20.40.60.81111¤The intersection of Comfortable and Large is then min(c,l),000.20.40.60.30000To interpret,five bedrooms is optimal having the largest membership value0.6.It is,however, not fully satisfactory,since the membership is less than1.The second best solution is four bedrooms,membership0.4.If the market is a buyer’s market,the family will probably wait until a better offer comes up,thus hoping to achieve full satisfaction(membership1).The union of Comfortable and Large is max(c,l)0.20.50.810.70.81111Here four bedrooms is fully satisfactory(membership1)because it is comfortable.Also7-10 bedrooms are satisfactory,because the house is large.If the market is a seller’s market,the family might buy the house,being content that at least one of the criteria is fulfilled.If the children are about to move away from the family within the next couple of years,the parents may wish to buy a house that is Comfortable and Not Large,or min(c,1-l)0.20.50.80.60.40.20000In that case,three bedrooms is satisfactory to the degree0.8.The example indicates how fuzzy sets can be used for computer aided decision support.In mathematics the word’relation’is used in the sense of relationship,for example the10predicates:x is less than y,or y is a function of x.A binary relation R is a set of ordered pairs.We may write it x R y which reads:’x is related to y.’There are established symbols for various relations,for example x=y,x<y.One simple relation is the set of all pairs h x,y i,such that x is a member of a set X and y is a member of a set Y.This is the(classical) cartesian product of X and Y,X×Y={h x,y i|x∈X,y∈Y}In fact,any binary relation x R y is a subset of the cartesian product X×Y,and we can think of those instances of X×Y,that are members of R as having membership1.By analogy,a binary fuzzy relation consists of pairs h x,y i with an associated fuzzy membership value.For example,given X=Y={1,2,3}we can set up a relation’approximately equal’between all pairs of the three numbers,most clearly displayed in a tabular arrangement,Y1X23In the fuzzy cartesian product each pair:the object,which is a pair itself,and its membership.Let A and B be fuzzy sets defined on X and Y respectively,then the cartesian product A×B is a fuzzy set in X×Y with the membership functionA×B=©h x,y i,μA×B(x,y)®|x∈X,y∈Y,μA×B(x,y)=min(μA(x),μB(y))ªFor example,assume X and Y are as above,andμA(x i)=h0,0.5,1i,with i=1,2,3,and μB(y j)=h1,0.5,0i,with j=1,2,3,then A×B is a two-dimensional fuzzy setBA0.51The element at row i and column j is the intersection ofμA(x i)andμB(y j).Again we note that to each object h x i,y j i is associated a membershipμA×B(x i,y j),whereas the classical cartesian product consists of objects h x i,y j i only.In order to see how to combine relations,let us look at an example from the cartoon Donald Duck.Example7Donald Duck’s familyAssume that Donald Duck’s nephew Huey resembles nephew Dewey to the grade0.8,and Huey resembles nephew Louie to the grade0.9.We have therefore a relation between two subsets of the nephews in the family.This is conveniently represented in a matrix,with one row and two columns(and additional headings),R1=Huey11Let us assume another relation between nephews Dewey and Louie on the one side,and uncle Donald on the other,a matrix with two rows and one column,R2=DeweyLouieWe wish tofind out how much Huey resembles Donald by combining the information in the two matrices.Observe that(i)Huey resembles Dewey(R1(1,1)=0.8),and Dewey in turn resembles Donald(R2(1,1)=0.5),or(ii)Huey resembles Louie(R1(1,2)=0.9),and Louie in turn resembles Donald(R2(2,1)= 0.6).Assertion(i)contains two relationships combined by’and’;it seems reasonable to takethe intersection.With our definition,this corresponds to choosing the smallest membership value for the(transitive)Huey-Donald relationship,or min(0.8,0.5)=0.5.Similarly with statement(ii).Thus from chains(i)and(ii),respectively,we deduce that(iii)Huey resembles Donald to the degree0.5,or(iv)Huey resembles Donald to the degree0.6.Although the results in(iii)and(iv)differ,we are equally confident in either result;we haveto choose either one or the other,so it seems reasonable to take the union.With our definition, this corresponds to choosing the largest membership value,or max(0.5,0.6)=0.6.Thus,the answer is that Huey resembles Donald to the degree0.6.Generally speaking,this was an example of composition of relations.Let R and S betwo fuzzy relations defined on X×Y and Y×Z respectively.Their composition is a fuzzyset defined byR◦S=(*h x,z i,[yμR(x,y)∩μS(y,z)+|x∈X,y∈Y,z∈Z)When R and S are expressed as matrices R and S,the composition is equivalent to aninner product.The inner product is similar to an ordinary matrix(dot)product,except multiplication is replaced by any function and summation by any function.Suppose R isan m×p matrix and S is a p×n matrix.Then the inner∪−∩product(read’cup-cap product’)is an m×n matrix T=(t ij)whose ij-entry is obtained by combining the i throw of R with the j th column of S,such thatt ij=(r i1∩s1j)∪(r i2∩s2j)∪...∪(r ip∩s pj)=p[k=1r ik∩s kj(5)With our definitions of the set operations,the composition is specifically called max-min composition(Zadeh in Zimmermann,1993[16]).Sometimes the min operation is replaced by*for multiplication,then it is called max-star composition.Example8Inner product12For the tables R1and R2above,the inner product yields,R1◦R2==(0.8∩0.5)∪(0.9∩0.6)=0.5∪0.6=0.6 which agrees with the previous result.3Fuzzy LogicLogic started as the study of language in arguments and persuasion,and it can be used to judge the correctness of a chain of reasoning—in a mathematical proof for instance. The goal of the theory is to reduce principles of reasoning to a code.The’truth’or’falsity’assigned to a proposition is its truth-value.In fuzzy logic a proposition may be true or false,or an intermediate truth-value such as maybe true.The sentence’John is a tall man’is an example of a fuzzy proposition.For convenience we shall here restrict the possible truth-values to a discrete domain{0,0.5,1}for false,maybe true,and true.In doing so we are in effect restricting the theory to multi-valued logic,or rather three-valued logic to be specific.In practice one would subdivide the unit interval intofiner divisions,or work with a continous truth-domain.Nevertheless,much of what follows is valid even in a continuous domain as we shall see.3.1PropositionsIn daily conversation and mathematics,sentences are connected with the words and,or,if-then(or implies),and if and only if.These are called connectives.A sentence which is modified by the word’not’is called the negation of the original sentence.The word’and’is used to join two sentences to form the conjunction of the two sentences.The word’or’is used to join two sentences to form the disjunction of the two sentences.From two sentences we may construct one,of the form’If...then...’;this is called an implication.The sentence following’If’is the antecedent,and the sentence following’then’is the consequent.Other idioms which we shall regard as having the same meaning are’p implies q’,’p only if q’,’q if p’,etc.Letters and special symbols make the connective structure stand out.Our choice of symbols is¬for’not’∧for’and’∨for’or’⇒for’if-then’(implication)⇔for’if and only if’(equivalence)The next example illustrates how the symbolic forms expose the underlying logical structure. Example9Baseball betting(Stoll,1979[10])13Consider the assertion about four baseball teams:If either the Pirates or the Cubs loose and the Giants win,then the Dodgers will be out of first place,and I will loose a bet.Since it is an implication,it may be symbolised in the form r ⇒s.The antecedent is composed from the three sentences p (The Pirates lose),c (The Cubs lose),and g (The Giants win).The consequent is the conjunction of d (The Dodgers will be out of first place)and b (I will lose a bet).The original sentence may thus be represented by ((p ∨c )∧g )⇒(d ∧b ).An assertion which contains at least one propositional variable is called a propositional form .The main difference between proposition and propositional form is that everyproposition has a truth-value,whereas a propositional form is an assertion whose truth-value cannot be determined until propositions are substituted for its propositional variables.But when no confusion results,we will refer to propositional forms as propositions.A truth-table summarises the possible truth-values of an assertion.Take for example the truth-table for the two-valued propositional form p ∨q .The truth-table (below,left)lists all possible combinations of truth-values —the Cartesian product —of the arguments p and q in the two leftmost columns.The rightmost column holds the truth-values of the proposition.Alternatively,the truth-table can be rearranged into a two-dimensional array,a so-called Cayley table (below,right).is equivalent to Or p ∨q →q 01↓p Along the vertical axis in the Cayley table,symbolized by arrow ↓,are the possible values 0and 1of the first argument p .Along the horizontal axis,symbolized by arrow →,are the possible values 0and 1of the second argument q .Above the table,the proposition p ∨q reminds us that the table concerns disjunction.At the intersection of row i and column j (only counting the inside of the box)is the truth-value of the expression p i ∨q j .By inspection,one entry renders p ∨q false,while three entries render p ∨q true.Truth-tables for binary connectives are thus given by two-by-two matrices.A total of 16such tables can be constructed,and each has been associated with a connective.We can derive the truth-table for ’nand’(’not and’)from ’or’.By the de finition (¬p )∨(¬q )we negate each variable of the previous truth-table,which is equivalent to reversing the axes and permuting the entries back in the usual ascending order on the axes,Nand(¬p )∨(¬q )→q01↓p14。
- 1、下载文档前请自行甄别文档内容的完整性,平台不提供额外的编辑、内容补充、找答案等附加服务。
- 2、"仅部分预览"的文档,不可在线预览部分如存在完整性等问题,可反馈申请退款(可完整预览的文档不适用该条件!)。
- 3、如文档侵犯您的权益,请联系客服反馈,我们会尽快为您处理(人工客服工作时间:9:00-18:30)。
Abstract In this paper, we deÿne generalized concepts of cardinality of a fuzzy-valued function and obtained some properties of these new concepts. Also, we investigate examples for the calculation of two generalized cardinality of fuzzy-valued functions and compared with concepts of cardinality of a fuzzy set and a fuzzy-valued function. c 2001 Elsevier Science B.V. All rights reserved. Keywords: Fuzzy set; Fuzzy number; Fuzzy cardinality; Sigma count; Fuzzy-valued function; Fuzzy-valued cardinality; Fuzzy-valued sigma count
F; a(1)
x2
F; a(2)
··· ···
xn
F; a(n)
:
This notation will be made clear in Section 2. We will use the following concept of fuzzy number. Let R be the real numbers and let [0, 1] be the unit interval in R. Let F(R) denote the set of all fuzzy sets in R.
F; a(k ) ; c(k ) ; d(k )
∧
F; 1−a(k +1) ; 1−d(k +1) ; 1−c(k +1) F; 0 (x ) = 1;
where a(0) ¿a(1) ¿ · · · ¿a(n) are the ordered values of the ai ’s, a(0) = 1 and a(n+1) = 0, and F; 1 (x ) = 0 for all x ∈ R. Furthermore, we deÿne the operation ∧ by
Fuzzy Sets and Systems 118 (2001) 479–487
/locate/fss
ቤተ መጻሕፍቲ ባይዱ
Cardinality concepts for type-two fuzzy sets
Lee-Chae Jang ∗; 1 , Dan Ralescu
Department of Mathematical Sciences, University of Cincinnati, Cincinnati, Ohio 45221-0025, USA Received April 1998; received in revised form January 1999
480
L.-C. Jang, D. Ralescu / Fuzzy Sets and Systems 118 (2001) 479–487
Deÿnition 1.1. A fuzzy set A ∈ F(R) is called a fuzzy number, if and only if (1) A is normal, i.e. there exists x0 ∈ R such that A (x0 ) = 1. (2) A is convex, i.e. A ( x + (1 − )y)¿ min( A (x); A (y)) for all x; y ∈ R and ∈ [0; 1]. (3) For any ∈ (0; 1]; A = {x: A (x)¿ } is a closed interval and cl(A0 ) = cl({x: A (x)¿0}) is compact. Here, cl(A0 ) is the closure of A0 . It follows from the deÿnition above that A ∈ F(R) is a fuzzy number if and only if there exists a closed interval [r; s] such that x ∈ [r; s] = ∅; 1; L(x); x¡ r; A (x ) = R(x); x¿s where L(x) is a right continuous function and R(x) is a left continuous function (see [6]). 2. Cardinality and f-cardinality Let X = {x1 ; x2 ; : : : ; xn } be a ÿnite set, and let A be a fuzzy subset of X , represented by its membership function A : X → [0; 1]. We denote the -level set of A by L (A) = {x ∈ X | A (x)¿ } for 06 61. The complement A of A has membership function A = 1 − A . Ralescu [3] and Ralescu [2] discussed some properties of card A, the cardinality of fuzzy set A. This should be a fuzzy subset of {0; 1; : : : ; n}, with card A(k ) being interpreted as the possibility that A has exactly k elements (06k 6n). Also, Ralescu [2] investigated that card A was k to the extent to which exactly k elements of X belong to A while the other (n − k ) elements do not belong to A and obtained the following explicit formular for the fuzzy cardinality: card A(k ) =
(k )
∧ (1 −
(k +1) );
k = 0; 1; : : : ; n
where (1) ; (2) ; : : : ; (n) are the values of A (x1 ); : : : ; A (xn ) arranged in decreasing order of magnitude, and (0) = 1; (n+1) = 0. Now we will consider a new deÿnition of f-card F , the fuzzy-valued cardinality of a fuzzy set-valued function F : X → F(R) with the following membership functions F (xi ) (x)= F; ai (x) of each F (xi ), for i = 1; 2; : : : ; n; x ∈ [ri ; si ] = ∅; 1; Li (x); x¡ ri ; F; ai ; ci ; di (x ) = Ri (x); x¿si where ai (i = 1; 2; : : : ; n) are some real numbers, si = ((m − 1)=m)ai and ti = ((m + 1)=m)ai , the functions Li are straight lines through points (1; si ); (ci ; 0) and the functions Ri are straight lines through points (1; ti ); (di ; 0), for some numbers ci ; di and i = 1; 2; : : : ; n. We denote the set of such fuzzy numbers by F 0. Deÿnition 2.1. The f-cardinality of a fuzzy-valued function F is the fuzzy-valued function f-card F : {0; 1; : : : ; n} →F 0 (R) deÿned for every k by f-card F (k ) =
F; a; c; d
∧
F; b; e; f
=
F; a∧b; c∧e; d∧f :
L.-C. Jang, D. Ralescu / Fuzzy Sets and Systems 118 (2001) 479–487
481
Notes 2.2. (1) Let a1 = · · · = ar = 1 and ar +1 = · · · = an = 0 and let Li = Ri = 0, we deÿne the membership functions F; ai ; ai ; ai of xi by
1. Preliminaries A fuzzy set is deÿned by a function f : X → [0; 1], where X is an ordinary set. Zadeh [7], Ralescu [2], Ralescu [3], Wygralak [5], Dubois and Prade [1], Yager [6] investigated concepts of cardinality of a fuzzy set and obtained some properties of these new concepts. A statement such as “most students are smart” has a truth value between 0 and 1; such a statement is of the general form “Qx’s are A” where Q is a fuzzy quantiÿer and A is a fuzzy subset of a (ÿnite) universe of discourse X = {x1 ; x2 ; : : : ; xn }. An explicit formula for the truth-value of such a statement was given for the ÿrst time in [2]. In this paper, we consider a fuzzy-valued function F : X → F(R) instead of a fuzzy set A, that is, instead of a real-valued function on X . We denote such a fuzzy-valued function by F= x1